- 1Federal Research and Clinical Centre of Intensive Care Medicine and Rehabilitology, Department of Clinical Trials, Moscow, Russia
- 2Department of Anesthesiology, First Moscow State Medical University, Moscow, Russia
- 3Department of Anesthesiology, City Clinical Oncological Hospital No. 1, Moscow, Russia
Background: Postoperative delirium (POD) significantly affects patient outcomes after surgery, leading to increased morbidity, extended hospital stays, and potential long-term cognitive decline. This study assessed the predictive value of intraoperative electroencephalography (EEG) patterns for POD in adults.
Methods: This systematic review and meta-analysis followed the PRISMA and Cochrane Handbook guidelines. A thorough literature search was conducted using PubMed, Medline, and CENTRAL databases focusing on intraoperative native EEG signal analysis in adult patients. The primary outcome was the relationship between the burst suppression EEG pattern and POD development.
Results: From the initial 435 articles identified, 19 studies with a total of 7,229 patients were included in the systematic review, with 10 included in the meta-analysis (3,705 patients). In patients exhibiting burst suppression, the POD incidence was 22.1% vs. 13.4% in those without this EEG pattern (p=0.015). Furthermore, an extended burst suppression duration associated with a higher likelihood of POD occurrence (p = 0.016). Interestingly, the burst suppression ratio showed no significant association with POD.
Conclusions: This study revealed a 41% increase in the relative risk of developing POD in cases where a burst suppression pattern was present. These results underscore the clinical relevance of intraoperative EEG monitoring in predicting POD in older patients, suggesting its potential role in preventive strategies.
Systematic Review Registration: This study was registered on International Platform for Registered Protocols for Systematic Reviews and Meta-Analyses: INPLASY202420001, https://doi.org/10.37766/inplasy2024.2.0001.
1 Introduction
Postoperative delirium (POD) emerges as a multifaceted organic cerebral syndrome, presenting itself as a neuropsychiatric complication following surgical procedures (World Health Organisation, 1994). Marked by abrupt shifts in attention, cognition, and consciousness, POD poses a considerable challenge in perioperative care (O'Regan et al., 2013; Berikashvili et al., 2023). Its implications include heightened patient morbidity, prolonged hospitalization, and an elevated susceptibility to long-term cognitive decline, especially in older patients (Yan et al., 2023).
Given the organic underpinnings of postoperative delirium, monitoring brain activity through electroencephalography (EEG) during surgery emerges as a valuable approach for predicting this complication (Sun et al., 2020). Consequently, recent European guidelines on postoperative delirium management advocate for intraoperative monitoring of anesthesia depth and EEG patterns, specifically the burst suppression pattern, despite the limited quality of evidence supporting this recommendation (Aldecoa et al., 2023).
While a recent meta-analysis suggests that anesthesia guided by bispectral index (BIS) has not significantly reduced the incidence of postoperative delirium (Chew et al., 2022), it is crucial to recognize that excessively deep anesthesia remains a critical risk factor for its development (Evered et al., 2021). The pathological processes induced by overly deep anesthesia, contributing to the clinical manifestation of postoperative delirium, could potentially be discerned through the identification of EEG burst suppression patterns. Moreover, various other EEG signal characteristics may serve as potential predictors of postoperative delirium (Baron Shahaf et al., 2023; Khalifa et al., 2023; Kinoshita et al., 2023). Further research and exploration of EEG monitoring techniques hold promise in refining our understanding and prediction of postoperative delirium in clinical practice.
This systematic review and meta-analysis aim to assess the predictive value of intraoperative EEG for postoperative delirium in adults.
2 Materials and methods
This study was conducted in accordance with the Preferred Reporting Items for Systematic Reviews and Meta-Analyses (PRISMA) (Liberati et al., 2009) and Cochrane Handbook guidelines (Higgins et al., 2019). The meta-analysis protocol was registered with the International Platform for Registered Protocols for Systematic Reviews and Meta-Analyses (INPLASY) under registration number INPLASY202420001, https://doi.org/10.37766/inplasy2024.2.0001. The completed PRISMA checklist is presented in Supplementary Table S1.
2.1 Search strategy
A comprehensive systematic search was conducted to identify relevant studies published between January 1, 2003, and October 23, 2023. This search encompassed databases such as PubMed, Medline, and the Cochrane Central Register of Controlled Trials (CENTRAL) and was performed by three independent researchers. Additionally, the authors employed forward and backward snowballing methods [Litmaps service (Literature Map Software for Lit Reviews and Research and Litmaps, 2024)]. We did not restrict the search by language. The detailed search strategy and queries are available in Supplementary Appendix A.
2.2 Eligibility criteria and study selection
After the automatic exclusion of duplicate records, three researchers independently screened the remaining studies for eligibility based on their titles and abstracts utilizing the PICOS criteria (detailed in Supplementary Appendix B). We focused on prospective and retrospective observational studies that explored predictors of POD using intraoperative native EEG signal analysis in adult patients. The final inclusion in this study was determined after a thorough full-text article analysis. Studies were excluded if they met one of the following criteria: (1) were review articles, case reports or letters to the editors; (2) followed EEG-guided anesthesia; (3) reported no outcome data; (4) utilized non-intraoperative EEG; or (5) evaluated the BIS.
Any disagreements were resolved by consultation with the involvement of the supervisor until a consensus was reached.
2.3 Outcome measures and data extraction
For this review, a dedicated data collection form was developed. This form was utilized independently by three authors to independently assess the full manuscripts and supplemental or additional files of all included studies and extract the data. The following data were extracted: study design, sample size, first author, publication year, journal name, POD assessment method, study setting, participant age and sex, American Society of Anesthesiologists (ASA) score, type of anesthesia used, duration of surgery and anesthesia, length of intensive care unit (ICU) and hospital stay, intraoperative EEG timing, and the types and characteristics of EEG patterns in both the POD and non-POD groups. After independent data extraction, the researchers consulted with each other to identify disagreements and reach consensus through discussion.
In instances where the data were presented solely in graphical format, numerical values were extracted using the WebPlotDigitizer tool (Rohatgi, 2010). For studies presenting continuous data in non-standard formats (e.g., median, interquartile range, or 95% confidence intervals), we employed established methods to calculate the mean ± standard deviation (SD). These methods included the statistical techniques proposed by Wan et al. (2014) and Luo et al. (2018), as well as the Cochrane Handbook recommendations 6.5.2.2 and 6.5.2.5 (Higgins and Green, 2011).
This meta-analysis specifically focused on the burst suppression pattern in EEG signals, examining its duration, ratio, and presence (incidence). In this meta-analysis, the BSR is defined as the time EEG shows BSP divided by the total EEG monitoring time. This differs from the clinical BSR, typically a time-varying percentage of BSP over a short period. Our BSR calculation reflects the overall incidence of burst suppression during the entire EEG session, providing a distinct measure for our analysis.
2.4 Data analysis and synthesis
In this meta-analysis, STATA 17.0 (StataCorp LLC, Texas, US) was used for both calculations and visualizations. We assessed interstudy heterogeneity using the I-squared (I2) statistic and the Cochrane Q test. Standardized mean differences (SMDs) with 95% confidence intervals (CIs) were calculated for quantitative data. In accordance with the Cochrane handbook guidelines, SMDs were categorized into effect sizes: small (< 0.40), moderate (0.40 to 0.70), and large (>0.70) (Higgins and Green, 2011). For categorical outcomes, logarithmic odds ratios (log ORs) and 95% CIs were determined.
A fixed-effects inverse-variance model was applied in cases of low statistical heterogeneity (I2 < 60% and p > 0.05), while a random-effects model [restricted maximum likelihood (REML)] was used for I2 ≥ 60% and/or p < 0.05. Statistical significance was set at p < 0.05.
The diagnostic accuracy of burst suppression presence was evaluated through pooled metrics, sensitivity, specificity, and positive and negative likelihood ratios, along with the summary receiver operating characteristic (SROC) area under the curve (AUC), employing the 'midas' module in STATA 17.0 (Dwamena, 2007). All the EEG patterns were categorized into six distinct groups based on the specific characteristics of the EEG signals being studied: (1) wave patterns (alpha, beta, delta, theta), (2) burst suppression pattern, and (3) unclassified patterns (Supplementary Table S6). We calculated a weighted average AUCs for the wave patterns.
2.5 Internal validity and risk of bias assessment
The internal validity and risk of bias were assessed by two independent reviewers using the “Tool to assess risk of bias in cohort studies” contributed by the CLARITY Group at McMaster University (CLARITY-group. Tool to Assess Risk of Bias in Case Control Studies Hamilton, 2023). An explanation of the risk of bias assessment is presented in Supplementary Table S2. The results were visualized using the “risk-of-bias visualization tool” (McGuinness and Higgins, 2021). Publication bias and small-study effects were assessed using Egger's test and funnel plot analysis.
The certainty of evidence was assessed with the GRADE systematic approach (Guyatt et al., 2008).
2.6 Sensitivity analysis
For sensitivity analysis and a more convenient way of comparing effect sizes, direct mean difference (MD), odds ratio (OR) and risk ratio (RR) values were additionally calculated and analyzed.
3 Results
3.1 Study characteristics
In the initial search, a total of 435 articles were identified. Following an abstract screening process, 51 articles were selected for full-text evaluation. After careful reading of the full-text articles, 32 studies were excluded (Supplementary Table S3). Ultimately, this systematic review included 19 studies published between 2015 and 2023 (Soehle et al., 2015; Fritz et al., 2016; Hesse et al., 2019; Momeni et al., 2019; Pedemonte et al., 2020; Jung et al., 2021; Koch et al., 2021, 2023; Lele et al., 2022; Li et al., 2022; Lutz et al., 2022; Röhr et al., 2022; Windmann et al., 2022; Baron Shahaf et al., 2023; Dragovic et al., 2023; Khalifa et al., 2023; Kinoshita et al., 2023; Reese et al., 2023; Ostertag et al., 2024). Additionally, 10 of these articles were included in the meta-analysis (Soehle et al., 2015; Hesse et al., 2019; Pedemonte et al., 2020; Jung et al., 2021; Koch et al., 2021, 2023; Lele et al., 2022; Lutz et al., 2022; Röhr et al., 2022; Ostertag et al., 2024). A flowchart illustrating the study selection process is presented in Figure 1.
In this systematic review, a total of 7,229 patients were analyzed, comprising 1,370 patients with POD (POD+) and 5,859 without (POD-). The data extracted from the included articles are detailed in Supplementary Tables S4–S6. The characteristics of the studies included in the meta-analysis are summarized in Table 1. In the meta-analysis of 3,705 patients, 18.8% (696) developed POD. The meta-analysis included five prospective observational studies, two post-hoc analyses of randomized trials, two retrospective observational studies, and one post-hoc analysis of a prospective observational trial. The mean age ranged from 59.8 to 72.9 years, and the proportion of patients with ASA III-V varied from 23.7% to 95.6% (Table 1).
3.2 Presence of burst suppression
According to a meta-analysis of five studies encompassing 1,182 patients and reporting the burst suppression episodes on EEG, the incidence was significantly higher in patients who experienced POD (22.1% vs. 13.4%) [OR = 1.68 (1.22; 2.32), p = 0.015; log OR = 0.52 (0.2; 0.84), p = 0.002; RR = 1.41 (1.1; 1.8), p = 0.006] (Figure 2, Table 2, Supplementary Figures S1-S3).
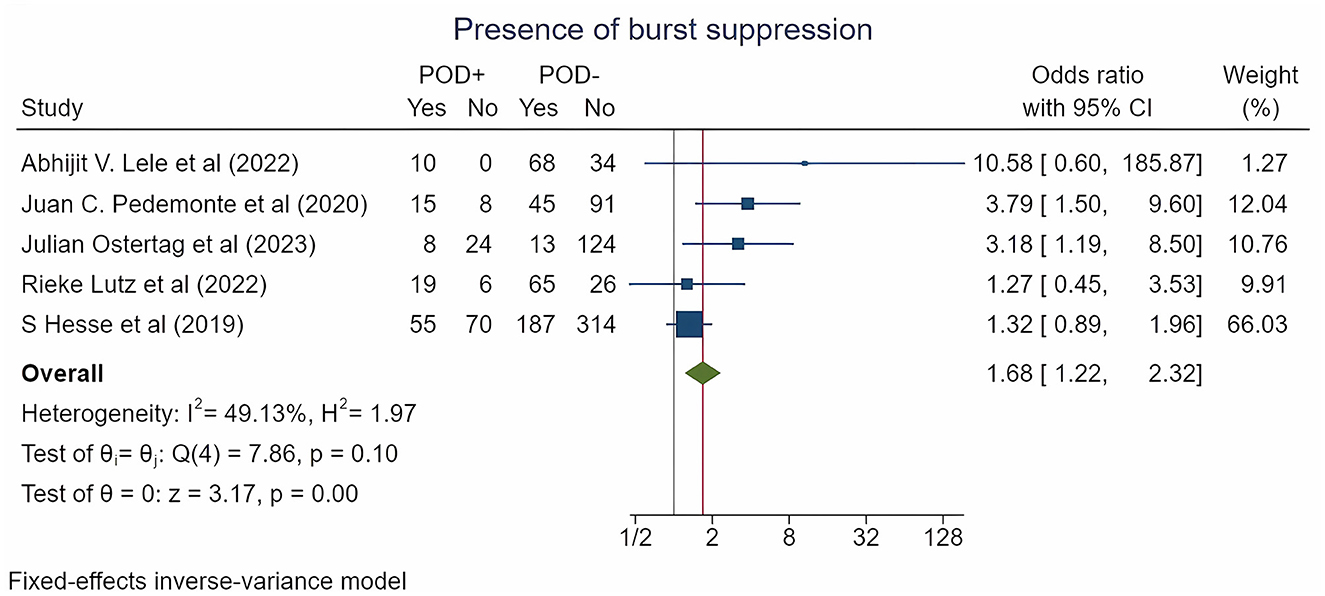
Figure 2. Forest plot for burst suppression incidence by POD type. The plot displays the studies, sample sizes, odds ratios, confidence intervals (CIs), and p-values. The size of the squares indicates the weight of the studies (considering sample size and standard deviations); the diamond represents the pooled odds ratio with CIs.
The area under the SROC curve for the presence of burst suppression was 0.620 (0.580; 0.660) (Figure 3), with a pooled sensitivity of 0.60 (0.34; 0.81) and a specificity of 0.59 (0.35; 0.79) (Supplementary Figure S4).
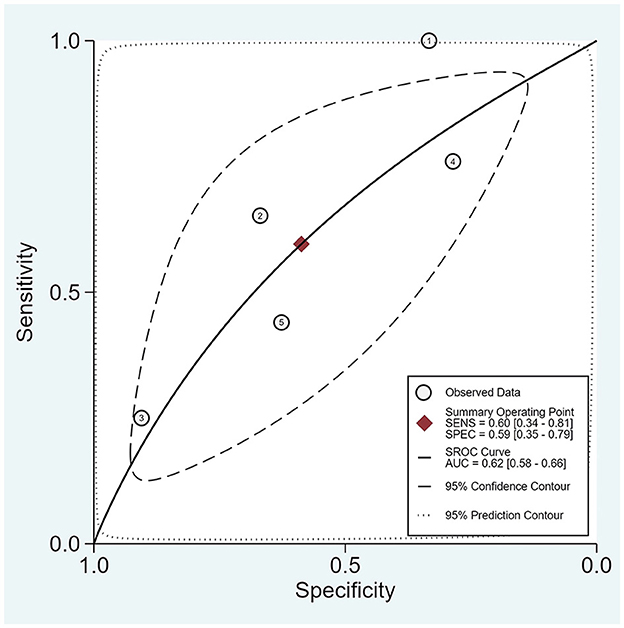
Figure 3. SROC for the presence of burst suppression. The plot displays the summary ROC curve (SROC) and presents AUC with 95% confidence interval (CI), 95% prediction and confidence contours, sensitivity, and specificity with CIs.
3.3 Duration of burst suppression
According to a pooled analysis of five studies reporting this outcome and involving 1,568 patients, patients who experienced POD had a significantly extended duration of burst suppression on EEG [MD = 15.86 (3.02; 28.70) minutes, p = 0.016; SMD = 0.36 (0.23; 0.49), p < 0.001] (Table 2, Supplementary Figures S5, S6).
3.4 Burst suppression ratio
In a pooled analysis of two studies involving 2,125 patients and reporting the burst suppression ratio on EEG, we found no significant relationship with POD [MD = 0.007 (−0.004; 0.018), p = 0.217; SMD = 0.07 (−0.04; 0.18), p = 0.215] (Table 2, Supplementary Figures S7, S8).
3.5 Weighted average AUC values
The weighted average AUC values for the alpha (0.676), beta (0.670), delta (0.660), and theta (0.685) wave patterns were determined from studies included in the systematic review (Supplementary Table S6).
3.6 Risk of bias and GRADE assessment
The overall risk of bias of the 10 enrolled studies was judged as ‘low' in three studies and ‘some concerns' in 7 studies (Supplementary Figure S9). The main sources of bias identified were the lack of matching for confounding variables and inconsistency in exposure assessment. Egger's test and funnel plot analysis did not reveal small-study effects for the majority of the study outcomes (Supplementary Figure S10). Publication bias was statistically significant for the presence of burst suppression (Table 2, Supplementary Figure S10). According to the GRADE approach, a moderate level of evidence supported the association between duration of burst suppression and POD, while evidence for the incidence of burst suppression was considered very low (Supplementary Table S7).
4 Discussion
4.1 Key findings
This is the first systematic review and meta-analysis to evaluate various aspects of burst suppression pattern of EEG signals as prognostic factors of postoperative delirium in older adults. The meta-analysis revealed that the duration of burst suppression is extended in patients who have developed postoperative delirium. In addition, the occurrence of a burst suppression pattern was associated with a 1.4-fold increased risk of developing POD (a relative risk increases of 41%). However, the SROC value for presence of burst suppression pattern was only 0.62, indicating that this pattern was a satisfactory prognostic factor for POD development. Investigations into other various wave patterns also demonstrated similar satisfactory predictive capabilities (the average weighted area under the curve (AUC) varied from 0.660 to 0.685).
4.2 Relationship with previous studies
This meta-analysis is the first to comprehensively examine the relationship between various EEG patterns and POD. Despite finding an association between the presence of the burst-suppression EEG pattern and the development of postoperative delirium, these results were not consistent across all studies (Hesse et al., 2019; Lele et al., 2022; Lutz et al., 2022). However, since the primary cause of this pattern is excessively deep anesthesia, our findings likely explain the results of the meta-analysis by Sumner M et al., which showed that EEG-guided anesthesia is associated with a reduced risk of POD (Sumner et al., 2023). Additionally, our results regarding the duration of burst suppression and its link to POD may also elucidate the findings of Sumner et al. (2023). In the systematic review conducted by Bruzzone et al. (2023) a detailed analysis was presented showing associations between specific intraoperative EEG parameters and the development of POD. The study found that increased magnitude and longer durations of EEG suppression, alongside a reduction in higher frequency activity, were significant indicators of POD risk. Furthermore, it was noted that an increased incidence and duration of BSR and lower BIS values are also predictive of POD development. Nonetheless, several studies have not demonstrated an association between this parameter and POD (Koch et al., 2021, 2023; Lele et al., 2022).
4.3 Significance of the study findings
The significance of our study findings can be understood in two key aspects.
First, this inaugural meta-analysis investigated the effects of deep anesthesia, characterized by the presence of a burst suppression pattern on EEG signals, on the incidence of POD. Identifying statistically significant differences in burst suppression pattern, in terms of incidence and duration, between patients with and without POD is promising for advancing our understanding of postoperative delirium prediction and prevention strategies.
Second, our meta-analysis revealed a lack of comprehensive data on intraoperative EEG patterns. A major challenge in studying the prognostic qualities of EEG patterns is the absence of a standardized set of metrics for evaluation. This limitation hinders the possibility of conducting extensive meta-analyses. Additionally, the lack of standardization in the aspects of recording periods further diminishes the quality of the existing evidence. Despite current recommendations advocating for perioperative EEG monitoring, especially concerning the burst suppression pattern, the shift in clinical practices necessitates robust, high-quality evidence to adhere to evidence-based clinical decision-making principles. Our study provides, for the first time, high-quality evidence supporting the integration of perioperative EEG monitoring in the diagnostic framework for POD. Our results align with and reinforce the latest guidelines, thereby enhancing the evidence quality in this domain. In summary, this research not only highlights the clinical value of intraoperative EEG monitoring but also underscores the need for further high-quality studies to strengthen the evidence base in this area.
4.4 Strengths and limitations
To the best of our knowledge, this is the first meta-analysis in this field. Despite the inability to conduct a meta-analysis on a wide range of other diverse EEG patterns, we successfully grouped them based on their relation to one of the EEG waves (alpha, beta, theta, delta) and calculated the weighted average AUCs for each group. While the values of the weighted average AUCs were comparable, a more in-depth exploration of the existing patterns is of significant scientific interest. All studies included in the meta-analysis exhibited a moderate or low risk of bias, enhancing the quality of the obtained results.
Nevertheless, it is important to acknowledge the limitations in this analysis. The high heterogeneity among the studies presented in this work complicates the process of data synthesis resulting from the analysis. Additionally, the burst suppression ratio did not demonstrate statistical significance in predicting POD, which may be attributed to the limited availability of data and studies examining this pattern. We cannot overlook that the development of POD is influenced by a multitude of factors, such as patients' medical histories, characteristics of the intraoperative period, and surgical complications during the perioperative period. To gain a more precise understanding of the nature of POD onset, these factors must be considered in the analysis. We also observed significant publication bias for burst suppression incidence, which suggests that the findings should be interpreted with caution. Moreover, using EEG to predict POD during the intraoperative period has several limitations. Individual variability in brain activity, influenced by factors like age and neurological history, can complicate EEG signal interpretation. The intraoperative setting introduces artifacts from surgical and medical equipment, challenging the clarity and reliability of EEG data. Additionally, anesthetic agents alter EEG patterns, necessitating careful consideration of their effects in POD prediction. Standardization issues in EEG protocol, such as electrode placement and signal processing, further limit the consistency and generalizability of findings across studies.
4.5 Future studies and prospects
A comprehensive analysis of the conducted studies has highlighted the need for further exploration of the burst suppression pattern through the execution of high-quality prospective observational studies dedicated to its examination. The substantial heterogeneity among the studied patterns raises considerations about standardizing the methods for assessing individual types of EEG waves, potentially facilitating subsequent meta-analyses to assess the prognostic significance of these parameters in predicting postoperative delirium.
5 Conclusion
This systematic review and meta-analysis demonstrated that the occurrence of the burst suppression pattern on EEG was associated with a 41% increase in the relative risk of POD development in older patients. Additionally, the duration of burst suppression was also extended in patients with POD. Our research provides strong evidence for expanding the use of intraoperative EEG monitoring in current guidelines. This study highlights EEG's value in improving perioperative care by assessing brain activity and detecting delirium risk. Our findings advocate for integrating EEG monitoring into routine intraoperative procedures to enhance patient outcomes and support more personalized anesthetic management.
Data availability statement
The original contributions presented in the study are included in the article/Supplementary material, further inquiries can be directed to the corresponding author.
Author contributions
VL: Conceptualization, Methodology, Project administration, Writing – original draft, Writing – review & editing. LB: Conceptualization, Formal analysis, Methodology, Writing – original draft, Writing – review & editing. AS: Data curation, Investigation, Writing – original draft, Writing – review & editing. PP: Formal analysis, Methodology, Writing – original draft, Writing – review & editing. MY: Formal analysis, Writing – original draft, Writing – review & editing. NG: Data curation, Investigation, Writing – original draft, Writing – review & editing. OR: Data curation, Investigation, Writing – original draft, Writing – review & editing. IA: Writing – original draft, Writing – review & editing. MS: Project administration, Writing – original draft, Writing – review & editing. AK: Project administration, Writing – original draft, Writing – review & editing.
Funding
The author(s) declare that no financial support was received for the research, authorship, and/or publication of this article.
Conflict of interest
The authors declare that the research was conducted in the absence of any commercial or financial relationships that could be construed as a potential conflict of interest.
Publisher's note
All claims expressed in this article are solely those of the authors and do not necessarily represent those of their affiliated organizations, or those of the publisher, the editors and the reviewers. Any product that may be evaluated in this article, or claim that may be made by its manufacturer, is not guaranteed or endorsed by the publisher.
Supplementary material
The Supplementary Material for this article can be found online at: https://www.frontiersin.org/articles/10.3389/fnagi.2024.1386669/full#supplementary-material
References
Aldecoa, C., Bettelli, G., Bilotta, F., Sanders, R. D., Aceto, P., Audisio, R., et al. (2023). Update of the European society of anaesthesiology and intensive care medicine evidence-based and consensus-based guideline on postoperative delirium in adult patients. Eur. J. Anaesthesiol. 41:1876 doi: 10.1097/EJA.0000000000001876
Baron Shahaf, D., Hight, D., Kaiser, H., and Shahaf, G. (2023). Association between risk of stroke and delirium after cardiac surgery and a new electroencephalogram index of interhemispheric similarity. J. Cardiothorac. Vasc. Anesth. 37, 1691–1699. doi: 10.1053/j.jvca.2023.05.033
Berikashvili, L. B., Kadantseva, K. K., Ermokhina, N. V., Yadgarov, M. Y., Makarevich, D. G., Smirnova, A. V., et al. (2023). Postoperative neurocognitive disorders: the legacy of almost 400 years of history (Review). Obs. Reanimatol. 19, 29–42. doi: 10.15360/1813-9779-2023-4-29-42
Bruzzone, M., Walker, J., Chapin, B., Firme, M. S., Kimmet, F., Ahmed, S., et al. (2023). The role of the perioperative use of EEG as a predictor/diagnostic tool for post-operative delirium: systematic review (P10-1.008). Neurology 100:204149. doi: 10.1212/WNL.0000000000204149
Chew, W. Z., Teoh, W. Y., Sivanesan, N., Loh, P. S., Shariffuddin, I. I., Ti, L. K., et al. (2022). Bispectral index (BIS) monitoring and postoperative delirium in elderly patients undergoing surgery: a systematic review and meta-analysis with trial sequential analysis. J. Cardiothorac. Vasc. Anesth. 36, 4449–4459. doi: 10.1053/j.jvca.2022.07.004
CLARITY-group. Tool to Assess Risk of Bias in Case Control Studies Hamilton Ontario: McMaster University. (2023). Available online at: https://www.evidencepartners.com/wp-content/uploads/2021/03/Tool-to-Assess-Risk-of-Bias-in-Case-Control-Studies-DistillerSR.pdf (accessed July 14, 2023).
Dragovic, S., Schneider, G., García, P. S., Hinzmann, D., Sleigh, J., Kratzer, S., et al. (2023). Predictors of low risk for delirium during anesthesia emergence. Anesthesiology 139, 757–768. doi: 10.1097/ALN.0000000000004754
Dwamena, B. (2007). MIDAS: Stata Module for Meta-Analytical Integration of Diagnostic Test Accuracy Studies. Stat. Softw. Components, 1–2. Available online at: https://www.researchgate.net/publication/4998122_Meta-analytical_Integration_of_Diagnostic_Accuracy_Studies_in_Stata (accessed January 31, 2024).
Evered, L. A., Chan, M. T. V., Han, R., Chu, M. H. M., Cheng, B. P., Scott, D. A., et al. (2021). Anaesthetic depth and delirium after major surgery: a randomised clinical trial. Br. J. Anaesth. 127, 704–712. doi: 10.1016/j.bja.2021.07.021
Fritz, B. A., Kalarickal, P. L., Maybrier, H. R., Muench, M. R., Dearth, D., Chen, Y., et al. (2016). Intraoperative electroencephalogram suppression predicts postoperative delirium. Anesth. Analg. 122, 234–242. doi: 10.1213/ANE.0000000000000989
Guyatt, G. H., Oxman, A. D., Vist, G. E., Kunz, R., Falck-Ytter, Y., Alonso-Coello, P., et al. (2008). GRADE: an emerging consensus on rating quality of evidence and strength of recommendations. BMJ 336, 924–926. doi: 10.1136/bmj.39489.470347.AD
Hesse, S., Kreuzer, M., Hight, D., Gaskell, A., Devari, P., Singh, D., et al. (2019). Association of electroencephalogram trajectories during emergence from anaesthesia with delirium in the postanaesthesia care unit: an early sign of postoperative complications. Br. J. Anaesth. 122, 622–634. doi: 10.1016/j.bja.2018.09.016
Higgins, J. P. T., and Green, S. (2011). Cochrane Handbook for Systematic Reviews of Interventions | Cochrane Training. Handbook, 649. Available online at: https://training.cochrane.org/handbook (accessed January 31, 2024).
Higgins, J. P. T., Thomas, J., Chandler, J., Cumpston, M., Li, T., Page, M. J., et al. (2019). Cochrane Handbook for Systematic Reviews of Interventions. New York, NY: John Wiley and Sons.
Jung, C., Hinken, L., Fischer-Kumbruch, M., Trübenbach, D., Fielbrand, R., Schenk, I., et al. (2021). Intraoperative monitoring parameters and postoperative delirium: results of a prospective cross-sectional trial. Med 100:E24160. doi: 10.1097/MD.0000000000024160
Khalifa, C., Lenoir, C., Robert, A., Watremez, C., Kahn, D., Mastrobuoni, S., et al. (2023). Intra-operative electroencephalogram frontal alpha-band spectral analysis and postoperative delirium in cardiac surgery: a prospective cohort study. Eur. J. Anaesthesiol. 40, 777–787. doi: 10.1097/EJA.0000000000001895
Kinoshita, H., Saito, J., Kushikata, T., Oyama, T., Takekawa, D., Hashiba, E., et al. (2023). The perioperative frontal relative ratio of the alpha power of electroencephalography for predicting postoperative delirium after highly invasive surgery: a prospective observational study. Anesth. Analg. 137, 1279–1288. doi: 10.1213/ANE.0000000000006424
Koch, S., Blankertz, B., Windmann, V., Spies, C., Radtke, F. M., and Röhr, V. (2023). Desflurane is risk factor for postoperative delirium in older patients' independent from intraoperative burst suppression duration. Front. Aging Neurosci. 15:1067268 doi: 10.3389/fnagi.2023.1067268
Koch, S., Windmann, V., Chakravarty, S., Kruppa, J., Yürek, F., Brown, E. N., et al. (2021). Perioperative electroencephalogram spectral dynamics related to postoperative delirium in older patients. Anesth. Analg. 133, 1598–1607. doi: 10.1213/ANE.0000000000005668
Lele, A. V., Furman, M., Myers, J., Kinney, G., Sharma, D., and Hecker, J. (2022). Inadvertent burst suppression during total intravenous anesthesia in 112 consecutive patients undergoing spinal instrumentation surgery: a retrospective observational quality improvement project. J. Neurosurg. Anesthesiol. 34, 300–305. doi: 10.1097/ANA.0000000000000754
Li, N., Liu, X., Gao, Y., Yin, L., Zhao, W., Ma, R., et al. (2022). Association of electroencephalogram epileptiform discharges during cardiac surgery with postoperative delirium: an observational study. Front. Surg. 9:900122. doi: 10.3389/fsurg.2022.900122
Liberati, A., Altman, D. G., Tetzlaff, J., Mulrow, C., Gøtzsche, P. C., Ioannidis, J. P. A., et al. (2009). The PRISMA statement for reporting systematic reviews and meta-analyses of studies that evaluate health care interventions: explanation and elaboration. J. Clin. Epidemiol. 62, e1–e34. doi: 10.1016/j.jclinepi.2009.06.006
Literature Map Software for Lit Reviews and Research and Litmaps (2024). Available online at: https://www.litmaps.com/ (accessed January 31, 2024).
Luo, D., Wan, X., Liu, J., and Tong, T. (2018). Optimally estimating the sample mean from the sample size, median, mid-range, and/or mid-quartile range. Stat. Methods Med. Res. 27, 1785–1805. doi: 10.1177/0962280216669183
Lutz, R., Müller, C., Dragovic, S., Schneider, F., Ribbe, K., Anders, M., et al. (2022). The absence of dominant alpha-oscillatory EEG activity during emergence from delta-dominant anesthesia predicts neurocognitive impairment- results from a prospective observational trial. J. Clin. Anesth. 82:110949 doi: 10.1016/j.jclinane.2022.110949
McGuinness, L. A., and Higgins, J. P. T. (2021). Risk-of-bias VISualization (robvis): an R package and Shiny web app for visualizing risk-of-bias assessments. Res. Synth. Methods 12, 55–61. doi: 10.1002/jrsm.1411
Momeni, M., Meyer, S., Docquier, M. A., Lemaire, G., Kahn, D., Khalifa, C., et al. (2019). Predicting postoperative delirium and postoperative cognitive decline with combined intraoperative electroencephalogram monitoring and cerebral near-infrared spectroscopy in patients undergoing cardiac interventions. J. Clin. Monit. Comput. 33, 999–1009. doi: 10.1007/s10877-019-00253-8
O'Regan, N. A., Fitzgerald, J., Timmons, S., O'Connell, H., and Meagher, D. (2013). Delirium: a key challenge for perioperative care. Int. J. Surg. 11, 136–144. doi: 10.1016/j.ijsu.2012.12.015
Ostertag, J., Engelhard, A., Nuttall, R., Aydin, D., Schneider, G., García, P. S., et al. (2024). Development of postanesthesia care unit delirium is associated with differences in aperiodic and periodic alpha parameters of the electroencephalogram during emergence from general anesthesia: results from a prospective observational cohort study. Anesthesiology 140, 73–84. doi: 10.1097/ALN.0000000000004797
Pedemonte, J. C., Plummer, G. S., Chamadia, S., Locascio, J. J., Hahm, E., Ethridge, B., et al. (2020). Electroencephalogram burst-suppression during cardiopulmonary bypass in elderly patients mediates postoperative delirium. Anesthesiology 133, 280–292. doi: 10.1097/ALN.0000000000003328
Reese, M., Christensen, S., Anolick, H., Roberts, K. C., Wong, M. K., Wright, M. C., et al. (2023). EEG pre-burst suppression: characterization and inverse association with preoperative cognitive function in older adults. Front. Aging Neurosci. 15:1229081. doi: 10.3389/fnagi.2023.1229081
Rohatgi, A. (2010). WebPlotDigitizer - Extract Data From Plots, Images, and Maps. Arohatgi. Available online at: http://arohatgi.info/WebPlotDigitizer/ (accessed January 31, 2024).
Röhr, V., Blankertz, B., Radtke, F. M., Spies, C., and Koch, S. (2022). Machine-learning model predicting postoperative delirium in older patients using intraoperative frontal electroencephalographic signatures. Front. Aging Neurosci. 14:911088. doi: 10.3389/fnagi.2022.911088
Soehle, M., Dittmann, A., Ellerkmann, R. K., Baumgarten, G., Putensen, C., and Guenther, U. (2015). Intraoperative burst suppression is associated with postoperative delirium following cardiac surgery: A prospective, observational study. BMC Anesthesiol. 15:51. doi: 10.1186/s12871-015-0051-7
Sumner, M., Deng, C., Evered, L., Frampton, C., Leslie, K., Short, T., et al. (2023). Processed electroencephalography-guided general anaesthesia to reduce postoperative delirium: a systematic review and meta-analysis. Br. J. Anaesth. 130, e243–e253. doi: 10.1016/j.bja.2022.01.006
Sun, Y., Wei, C., Cui, V., Xiu, M., and Wu, A. (2020). Electroencephalography: clinical applications during the perioperative period. Front. Med. 7:251. doi: 10.3389/fmed.2020.00251
Wan, X., Wang, W., Liu, J., and Tong, T. (2014). Estimating the sample mean and standard deviation from the sample size, median, range and/or interquartile range. BMC Med. Res. Methodol. 14, 1–13. doi: 10.1186/1471-2288-14-135
Windmann, V., Dreier, J. P., Major, S., Spies, C., Lachmann, G., and Koch, S. (2022). Increased direct current-electroencephalography shifts during induction of anesthesia in elderly patients developing postoperative delirium. Front. Aging Neurosci. 14:921139. doi: 10.3389/fnagi.2022.921139
World Health Organisation (1994). The ICD-10 Classification of Mental and Behavioural Disorders: Clinical Descriptions and Diagnostic Guidelines, Tenth Revision. Null Null, Null. Available online at: https://iris.who.int/bitstream/10665/246208/1/9789241549165-V1-eng.pdf (accessed February 3, 2024).
Keywords: postoperative delirium, intraoperative EEG, burst suppression, systematic review, meta-analysis, surgery, anesthesia
Citation: Likhvantsev VV, Berikashvili LB, Smirnova AV, Polyakov PA, Yadgarov MY, Gracheva ND, Romanova OE, Abramova IS, Shemetova MM and Kuzovlev AN (2024) Intraoperative electroencephalogram patterns as predictors of postoperative delirium in older patients: a systematic review and meta-analysis. Front. Aging Neurosci. 16:1386669. doi: 10.3389/fnagi.2024.1386669
Received: 15 February 2024; Accepted: 30 April 2024;
Published: 13 May 2024.
Edited by:
Kevin Nicholas Hascup, Southern Illinois University School of Medicine, United StatesReviewed by:
Somchai Amornyotin, Mahidol University, ThailandSeyed A. Safavynia, Cornell University, United States
Copyright © 2024 Likhvantsev, Berikashvili, Smirnova, Polyakov, Yadgarov, Gracheva, Romanova, Abramova, Shemetova and Kuzovlev. This is an open-access article distributed under the terms of the Creative Commons Attribution License (CC BY). The use, distribution or reproduction in other forums is permitted, provided the original author(s) and the copyright owner(s) are credited and that the original publication in this journal is cited, in accordance with accepted academic practice. No use, distribution or reproduction is permitted which does not comply with these terms.
*Correspondence: Valery V. Likhvantsev, lik0704@gmail.com