- 1The Affiliated Jiangsu Shengze Hospital of Nanjing Medical University, Suzhou, China
- 2Medical Laboratory, Suzhou Psychiatric Hospital, The Affiliated Guangji Hospital of Soochow University, Suzhou, China
- 3The University of New South Wales, Kensington, NSW, Australia
- 4The University of Melbourne, Parkville, VIC, Australia
Introduction: Amyotrophic Lateral Sclerosis (ALS) is a neurodegenerative disease with poorly understood pathophysiology. Recent studies have highlighted systemic inflammation, especially the role of circulating inflammatory proteins, in ALS.
Methods: This study investigates the potential causal link between these proteins and ALS. We employed a two-sample Mendelian Randomization(MR) approach, analyzing data from large-scale genome-wide association studies to explore the relationship between 91 circulating inflammatory proteins and ALS. This included various MR methods like MR Egger, weighted median, and inverse-variance weighted, complemented by sensitivity analyses for robust results.
Results: Significant associations were observed between levels of inflammatory proteins, including Adenosine Deaminase, Interleukin-17C, Oncostatin-M, Leukemia Inhibitory Factor Receptor, and Osteoprotegerin, and ALS risk. Consistencies were noted across different P-value thresholds. Bidirectional MR suggested that ALS risk might influence levels of certain inflammatory proteins.
Discussion: Our findings, via MR analysis, indicate a potential causal relationship between circulating inflammatory proteins and ALS. This sheds new light on ALS pathophysiology and suggests possible therapeutic targets. Further research is required to confirm these results and understand the specific roles of these proteins in ALS.
1 Introduction
Amyotrophic Lateral Sclerosis (ALS), also known as Lou Gehrig’s disease, is a neurodegenerative condition that primarily affects motor neurons. This disease is characterized by the progressive degeneration of motor neurons, leading to fatal paralysis. ALS manifests in two forms: familial and sporadic, differentiated by family history. Despite significant research efforts, the underlying pathogenesis of ALS remains elusive, and effective treatments are scarce (Ralli et al., 2019; Masrori and Van Damme, 2020; Yang et al., 2021).
In the last decade, substantial progress has been made in understanding the genetic architecture, pathophysiological mechanisms, and potential biomarkers of ALS (Yang et al., 2021; Witzel et al., 2022; Mead et al., 2023). These advancements have opened new avenues for therapeutic intervention, particularly in the domain of neuroinflammation, which is increasingly associated with ALS. Recent focus in ALS research has centered on systemic inflammation, especially the role of circulating inflammatory proteins (Henkel et al., 2004; Murdock et al., 2015; Zondler et al., 2016). Studies have linked proteins like PIKFYVE kinase and TDP-43 to neuronal damage in ALS, associating their inhibition or misfolding with neuronal injury (Gleixner et al., 2022; Shao et al., 2022; Tejwani et al., 2023). This growing body of evidence underscores the importance of these proteins as both biomarkers and potential therapeutic targets (Akiyama et al., 2022; Jiang et al., 2022; Hosaka et al., 2023).
Concurrently, the application of bioinformatics, molecular biology, and genetics in ALS research has significantly enhanced our understanding of the disease’s complexity (Sankaran et al., 2021; Carroll, 2022; Sari et al., 2022). Innovations in these fields have led to the identification of new genetic and molecular pathways in ALS, laying the groundwork for targeted therapies (Lehrach et al., 2011; Akinduro et al., 2021; Wu and Lin, 2022). One key methodology employed in this context is Mendelian Randomization (MR). MR leverages genetic variations as instrumental variables to establish causal relationships between exposures (inflammatory proteins) and outcomes (ALS), thus enhancing the credibility of causal inferences drawn from observational studies by reducing common confounders and reverse causation (Song et al., 2022; Lee et al., 2023; Liu et al., 2023; Rajasundaram and Gill, 2023).
Our study employs a two-sample MR approach, utilizing multiple p-value thresholds to increase the accuracy of our findings while acknowledging the trade-offs involved. Lower p-values, such as <5.0E−08, are typically used to ensure robustness of associations, reduce heterogeneity, and improve study precision. However, such stringent criteria may also exclude potentially meaningful associations. Therefore, by adopting different p-value thresholds, our analysis aims to strike a balance between minimizing false positives and not overlooking significant associations that could be crucial for understanding the pathophysiology of ALS (Liu et al., 2023; Soremekun et al., 2023).
2 Methods
The research process is illustrated in the flowchart figure (Figure 1).
2.1 Data sources
Data on 91 circulating inflammatory proteins were sourced from GWAS data measured using the Olink Target Inflammation panel across 11 cohorts, involving a total of 14,824 European ancestry participants (Zhao et al., 2023). Data on Amyotrophic Lateral Sclerosis were derived from a meta-analysis of GWAS by van Rheenen et al., “GCST90027164”,1 including 27,205 ALS cases and 110,881 European ancestry controls (van Rheenen et al., 2021) (Supplementary Table S1). The ALS validation group comprises two GWAS datasets curated by van Rheenen and Nicolas. The initial dataset consists of sporadic ALS cases, while the validation sets include one sporadic ALS dataset and another dataset that does not differentiate between familial or sporadic ALS (Supplementary Table S1). Specific details regarding data curation are thoroughly explained in the original articles.
2.2 Mendelian randomization
We utilized a two-sample MR (TSMR) analysis to explore the causal relationship between circulating inflammatory proteins and ALS. In our MR analysis, inflammatory proteins were the exposure of interest, ALS was the outcome, and SNPs were used as instrumental variables. The TSMR approach was based on the following assumptions: (I) instrumental variables are closely associated with the risk of inflammatory proteins; (II) instrumental variables affect ALS risk only through their impact on inflammatory proteins; (III) instrumental variables are independent of confounding factors (Davey Smith and Hemani, 2014).
We selected SNPs associated with inflammatory proteins across the whole genome (p < 5 × 10−8), (p < 5 × 10−7), and (p < 5 × 10−6) for forward TSMR analysis. For reverse TSMR analysis of ALS GWAS instrumental variables, we used SNPs (p < 5 × 10−8). Additionally, PLINK clumping was employed to calculate linkage disequilibrium between each exposure’s SNPs on the basis of the 1,000 Genomes European panel, using an r2 < 0.01 (clumping distance = 5,000 kb) as the threshold for SNPs in linkage equilibrium. The F-statistic was calculated using F = beta2/se2, with all F-statistics >10, indicating robustness of the instrumental variables.
Several MR methods were used, including MR Egger, weighted median, IVW, Wald ratio, simple mode, and weighted mode. IVW was selected as the primary analysis method, using Wald ratio when snp < 2, with a p-value <0.05 considered significant (Burgess et al., 2013; Verbanck et al., 2018). Cochran’s Q statistic was used to assess heterogeneity between individual SNPs. If no significant heterogeneity was observed (p < 0.05), a fixed-effect model was adopted (Hemani et al., 2018); otherwise, the causal significance relationship needed cautious interpretation. Sensitivity analyses were also conducted to verify the robustness of our results. Furthermore, MR-Egger and MR-PRESSO methods were employed to determine the presence of pleiotropy. The intercept obtained from MR-Egger regression was used to measure directional pleiotropy, and MR-PRESSO was used for enhanced detection of pleiotropy (Bowden et al., 2016). Steiger testing was performed to determine the direction of causality. Leave-one-out sensitivity analysis was conducted to determine whether individual SNPs had a significant impact on MR results.
2.3 Statistical software
All statistical analyses were performed using R software version 4.3.0.2 MR analysis and Steiger filtering were performed using the “TwoSampleMR” R package (Smith and Ebrahim, 2003; Emdin et al., 2017; The Telomeres Mendelian Randomization Collaboration et al., 2017). MR-PRESSO was carried out using the “MRPRESSO” R package.
2.4 Selection of external datasets for validation
During the initial exploration phase of the study, we refrained from using p-value correction to capture more potential associations. To ensure the reliability of our preliminary results, we chose to validate them using two external datasets. One dataset focuses on sporadic ALS, while the other does not distinguish between familial or sporadic ALS. By including both sporadic ALS and non-differentiated familial or sporadic ALS datasets, we can comprehensively assess the generalizability of our findings. In the preliminary screening process, we incorporated potentially key proteins previously identified, including 12 inflammation-related proteins. By utilizing these datasets, we are able to validate the associations observed in our study (van Rheenen et al., 2016; Nicolas et al., 2018).
3 Results
3.1 Forward Mendelian randomization results
Using the threshold of SNPs (p < 5 × 10−8), instrumental variables were extracted for 73 inflammatory proteins for TSMR analysis. The results indicated that increased levels of Adenosine Deaminase are associated with a higher risk of ALS (OR = 1.068, PIVW = 0.048). This analysis showed no significant heterogeneity (MR Egger Q = 5.658, Q p-value = 0.059) and no horizontal pleiotropy (P Egger Intercept = 0.806, P MR Presso = 0.361). An increase in Interleukin-17C levels was also found to increase ALS risk (OR = 1.199, PIVW = 0.047) (SNPs <3). Higher Oncostatin-M levels were associated with a decreased risk of ALS (OR = 0.84, PIVW = 0.016), with the analysis showing no significant heterogeneity (MR Egger Q = 0.359, Q p-value = 0.836) and no horizontal pleiotropy (P Egger Intercept = 0.596, P MR Presso = 0.864). Increased levels of Leukemia inhibitory factor receptor were associated with a decreased risk of ALS (OR = 0.903, PIVW = 0.017), with no significant heterogeneity (MR Egger Q = 2.064, Q p-value = 0.151) or horizontal pleiotropy (P Egger Intercept = 0.913) observed (Figure 2) (Supplementary Tables S2, S3, S8).
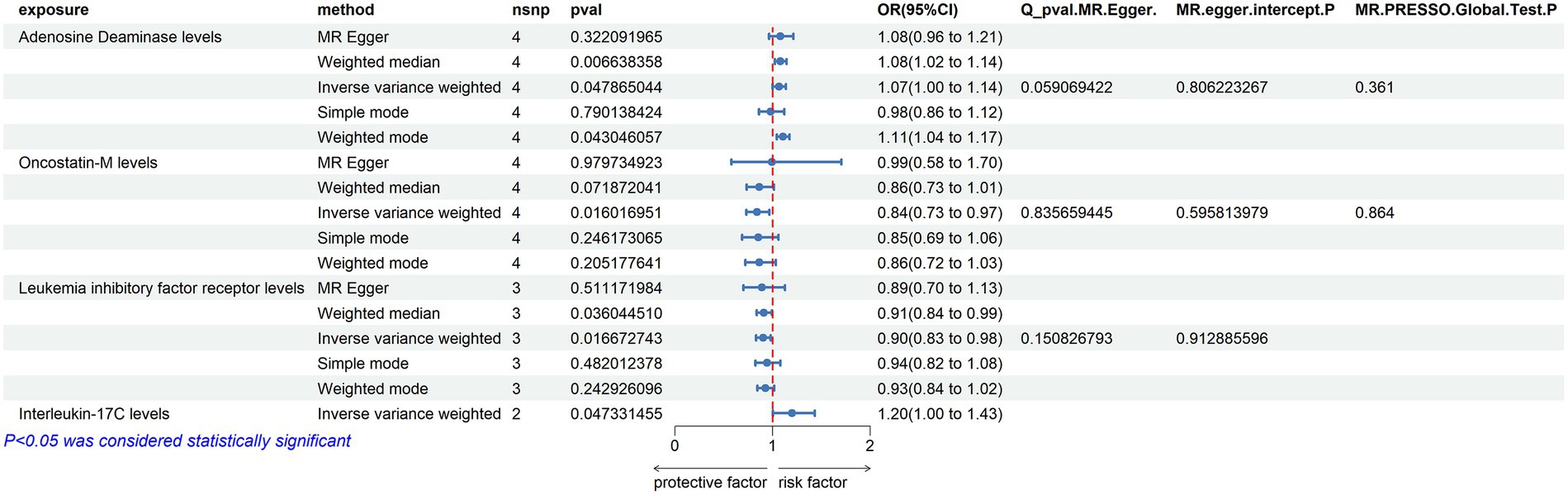
Figure 2. Adopt a significance threshold for selecting SNPs of p < 5 × 10−8. In this Mendelian randomization analysis, inflammatory proteins are analyzed as the exposure factor, with ALS as the resultant outcome. Significant findings are denoted by a P_IVW value less than 0.05.
Due to the initial selection criteria, some proteins did not yield SNPs, leading us to relax the conditions. We selected SNPs with a threshold of p < 5 × 10−7 as instrumental variables, and conducted TSMR analysis on 86 inflammatory proteins. The results revealed the following:
Adenosine Deaminase Levels: an increase in Adenosine Deaminase levels was associated with an increased risk of ALS (OR = 1.07, PIVW = 0.025). The analysis showed no significant heterogeneity (MR Egger Q = 6.654, Q p-value = 0.084) and no evidence of horizontal pleiotropy (P Egger Intercept = 0.820, P MR Presso = 0.339).
Interleukin-5 Levels: higher levels of Interleukin-5 were also linked to an increased risk of ALS (OR = 1.5, PIVW = 0.015), with fewer than three SNPs involved.
SIR2-like Protein 2 Levels: an elevation in SIR2-like protein 2 levels was correlated with an increased risk of ALS (OR = 1.24, PIVW = 0.024), again with fewer than three SNPs.
Neurturin Levels: increased levels of Neurturin were found to raise the risk of ALS (OR = 1.237, PIVW = 0.040), with fewer than three SNPs.
Leukemia Inhibitory Factor Receptor Levels: conversely, an increase in Leukemia inhibitory factor receptor levels was associated with a decreased risk of ALS (OR = 0.912, PIVW = 0.029). This analysis also showed no significant heterogeneity (MR Egger Q = 2.966, Q p-value = 0.227) and no horizontal pleiotropy (P Egger Intercept = 0.713, P MR Presso = 0.566).
Osteoprotegerin Levels: higher levels of Osteoprotegerin were linked to a reduced risk of ALS (OR = 0.89, PIVW = 0.020). The analysis indicated no significant heterogeneity (MR Egger Q = 16.163, Q p-value = 0.064) and no horizontal pleiotropy (P Egger Intercept = 0.979, P MR Presso = 0.118) (Figure 3) (Supplementary Tables S2, S4, S9).
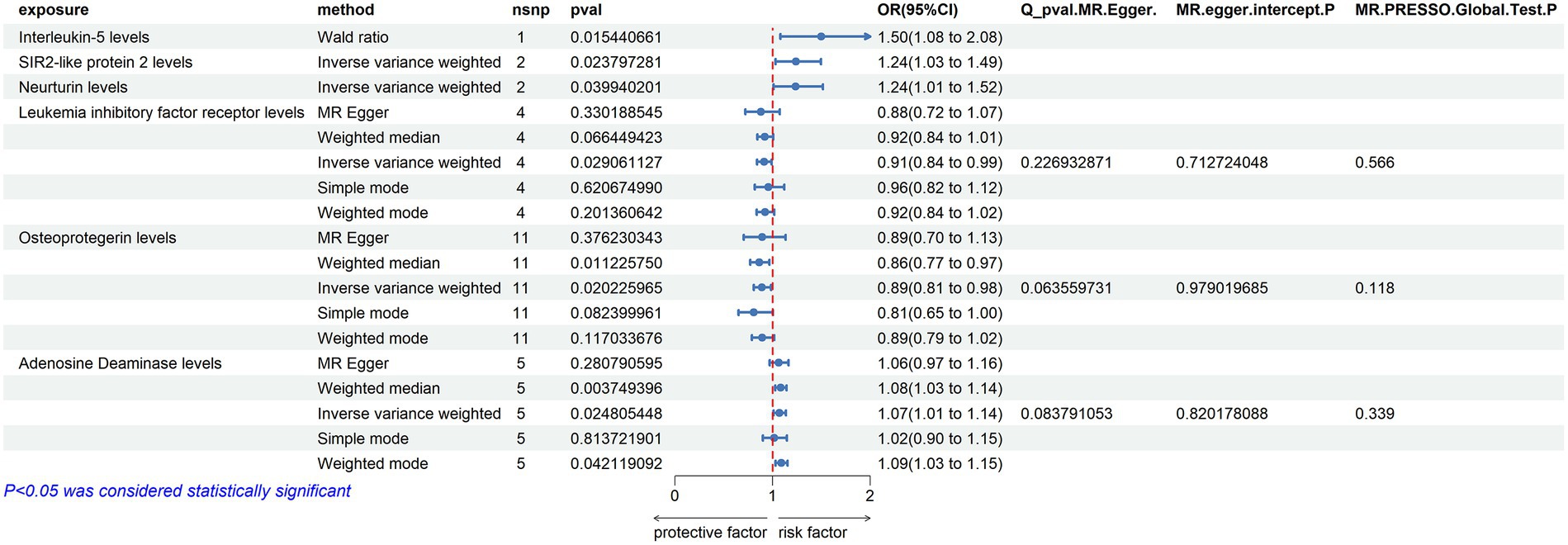
Figure 3. Adopt a significance threshold for selecting SNPs of p < 5 × 10−7. In this Mendelian randomization analysis, inflammatory proteins are analyzed as the exposure factor, with ALS as the resultant outcome. Significant findings are denoted by a P_IVW value less than 0.05.
In an effort to include more inflammatory proteins, the criteria were adjusted by setting the SNP threshold to p < 5 × 10−6. This enabled the extraction of instrumental variables for all 91 inflammatory proteins, which were then analyzed using TSMR. The findings were as follows:
ADA (Adenosine Deaminase) Levels: an increase in ADA levels was associated with an increased risk of ALS (OR = 1.072, PIVW = 0.037). This analysis indicated the presence of heterogeneity (MR Egger Q = 29.224, Q p-value = 0.004) and no evidence of horizontal pleiotropy (P Egger Intercept = 0.735, P MR Presso = 0.389).
TNF-beta Levels: elevated TNF-beta levels were associated with a reduced risk of ALS (OR = 0.951, PIVW = 0.012). The analysis showed heterogeneity (MR Egger Q = 34.518, Q p-value = 0.184) and no horizontal pleiotropy (p = 0.454, P MR Presso = 0.723).
Osteoprotegerin Levels: an increase in Osteoprotegerin levels was linked to a decreased risk of ALS (OR = 0.916, PIVW = 0.031). The analysis did not show significant heterogeneity (MR Egger Q = 27.915, Q p-value = 0.085) and no horizontal pleiotropy was found (P Egger Intercept = 0.440, P MR Presso = 0.125).
Interleukin-10 Levels: higher levels of Interleukin-10 were associated with a decreased risk of ALS (OR = 0.901, PIVW = 0.011). This analysis indicated no significant heterogeneity (MR Egger Q = 11.062, Q p-value = 0.853) and no horizontal pleiotropy (P Egger Intercept = 0.751, P MR Presso = 0.056) (Figure 4) (Supplementary Tables S2, S5, S10).
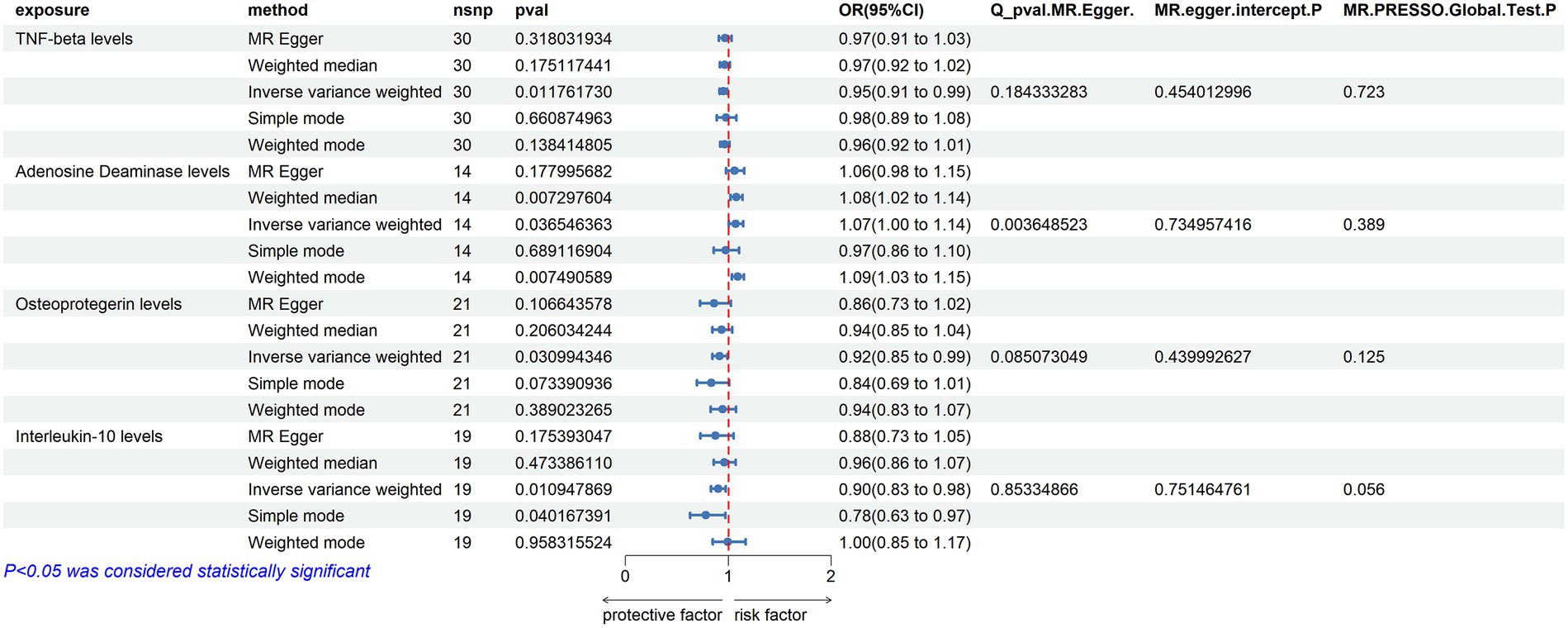
Figure 4. Adopt a significance threshold for selecting SNPs of p < 5 × 10−6. In this Mendelian randomization analysis, inflammatory proteins are analyzed as the exposure factor, with ALS as the resultant outcome. Significant findings are denoted by a P_IVW value less than 0.05.
From the three sets of analyses conducted, we can draw several conclusions:
ADA Levels: an increase in ADA levels was found to heighten the risk of ALS. This finding was significant across all three sets of analyses (P IVW < 0.05). Although there was heterogeneity in the results with the threshold at p < 5 × 10−6, the IVW results were relatively stable.
Leukemia Inhibitory Factor Receptor Levels: higher levels of the Leukemia inhibitory factor receptor were associated with a decreased risk of ALS. This was significantly observed in the first two sets of analyses (P IVW < 0.05).
Osteoprotegerin Levels: an increase in Osteoprotegerin levels also appeared to reduce the risk of ALS. This outcome was significant in the latter two sets of analyses (P IVW < 0.05).
Next, we conducted a leave-one-out analysis on the three key results mentioned above. This involved sequentially excluding each SNP and estimating the effect sizes for the remaining SNPs. For both Leukemia inhibitory factor receptor levels and Osteoprotegerin levels, the analysis showed no significant difference in effect size before and after exclusion, indicating that no single SNP had a significant impact on the MR estimates. However, in the three sets of analyses for ADA levels, the exclusion of the SNP “rs112665079” led to a deviation in results, suggesting that rs112665079 has a significant influence on the MR estimation results (Figure 5) (Supplementary Table S7).
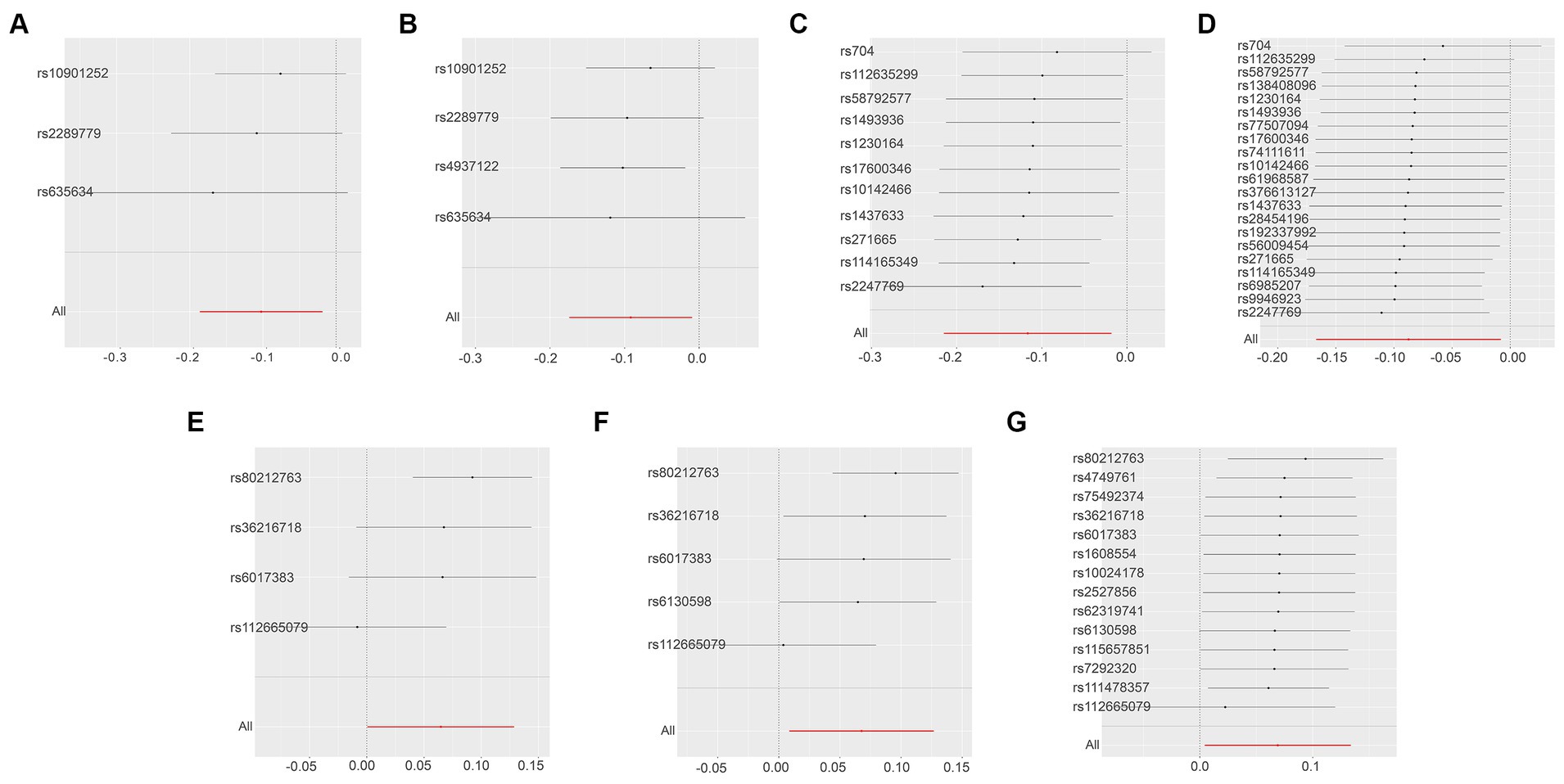
Figure 5. Displays the leave-one-out analysis results using the IVW method, assessing the impact of individual SNPs on the overall MR findings by sequentially excluding each SNP. The Y-axis corresponds to each excluded rsID and the aggregate IVW method result without any SNP exclusions. The X-axis represents the specific IVW values, where black and red dots denote beta effect values, and the lines indicate the confidence intervals of the beta values. Specifically, (A,B) illustrate the leukemia inhibitory factor receptor levels as the exposure, with SNP thresholds set at p < 5 × 10−8 and p < 5 × 10−7, respectively. (C,D) focus on osteoprotegerin levels as the exposure, applying SNP thresholds of p < 5 × 10−7 and p < 5 × 10−6. Finally, the levels of adenosine deaminase as the exposure factor are examined in (E–G), with SNP thresholds set at p < 5 × 10−8, p < 5 × 10−7, and p < 5 × 10−6, respectively.
After excluding rs112665079 and reanalyzing TSMR with ADA levels as the exposure and ALS as the outcome, the results were contrary to the previous findings, showing no significant correlation. This indeed demonstrates the significant impact of rs112665079 on the MR estimation results (Figure 6).
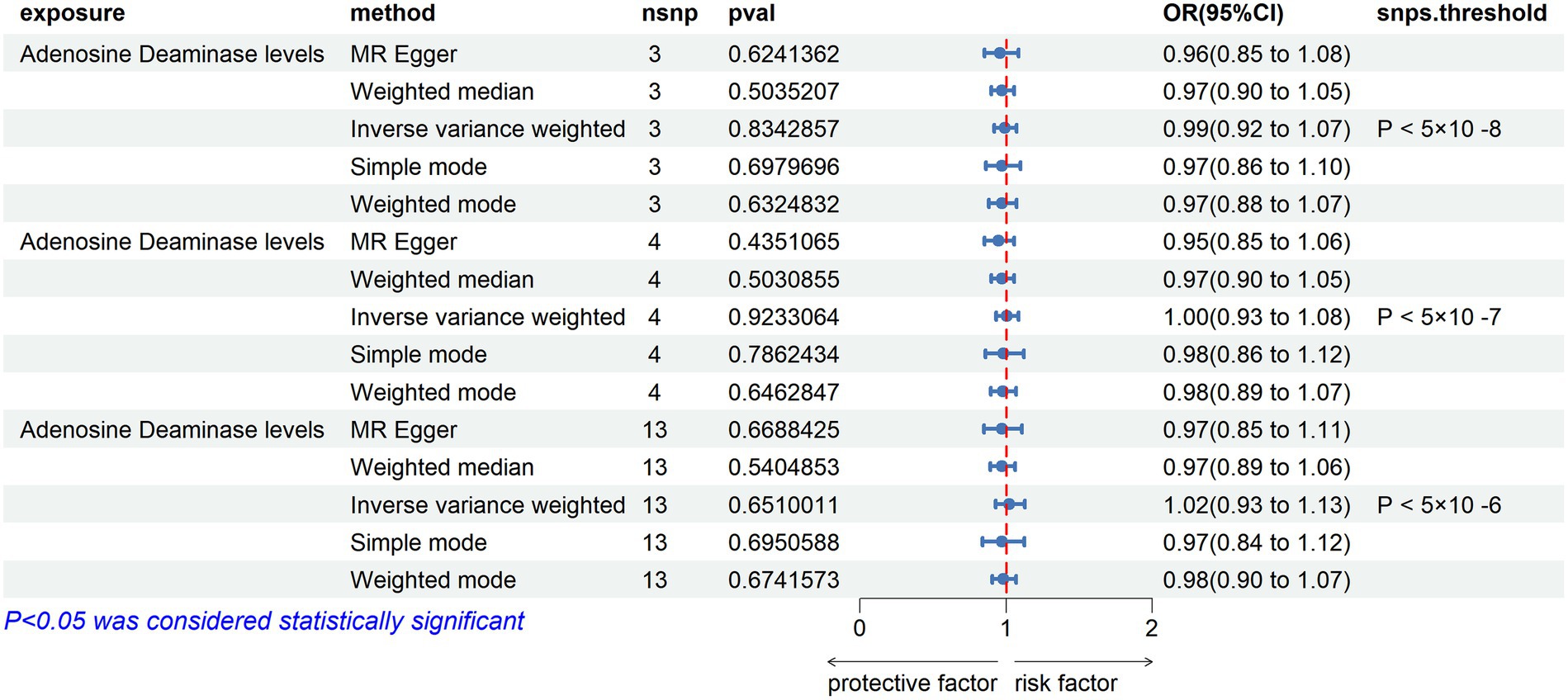
Figure 6. MR Results with Adenosine Deaminase Correction. SNP selection thresholds are applied with p-values <5 × 10−8, p < 5 × 10−7, and p < 5 × 10−6, respectively. Adenosine Deaminase protein is considered as the exposure factor, and ALS as the outcome in the Mendelian randomization analysis.
3.2 Reverse Mendelian randomization results
In the reverse Mendelian randomization analysis involving ALS and 91 inflammatory proteins, the following results were obtained:
C-C Motif Chemokine 20 Levels: an increase in the risk of ALS was associated with elevated levels of C-C motif chemokine 20 (OR = 1.089, PIVW = 0.020). The analysis showed no significant heterogeneity (MR Egger Q = 9.884, Q p-value = 0.626) and no horizontal pleiotropy (P Egger Intercept = 0.742, P MR Presso = 0.725).
Tumor Necrosis Factor Ligand Superfamily Member 12 Levels: similarly, an increased risk of ALS was associated with higher levels of Tumor necrosis factor ligand superfamily member 12 (OR = 1.097, PIVW = 0.010). No significant heterogeneity was observed in this analysis (MR Egger Q = 7.787, Q p-value = 0.802), and there was no evidence of horizontal pleiotropy (P Egger Intercept = 0.127, P MR Presso = 0.586).
Interleukin-5 Levels: in contrast, an increased risk of ALS was associated with decreased levels of Interleukin-5 (OR = 0.915, PIVW = 0.031). This analysis also showed no significant heterogeneity (MR Egger Q = 10.272, Q p-value = 0.592) and no horizontal pleiotropy (P Egger Intercept = 0.898, P MR Presso = 0.686) (Figure 7).
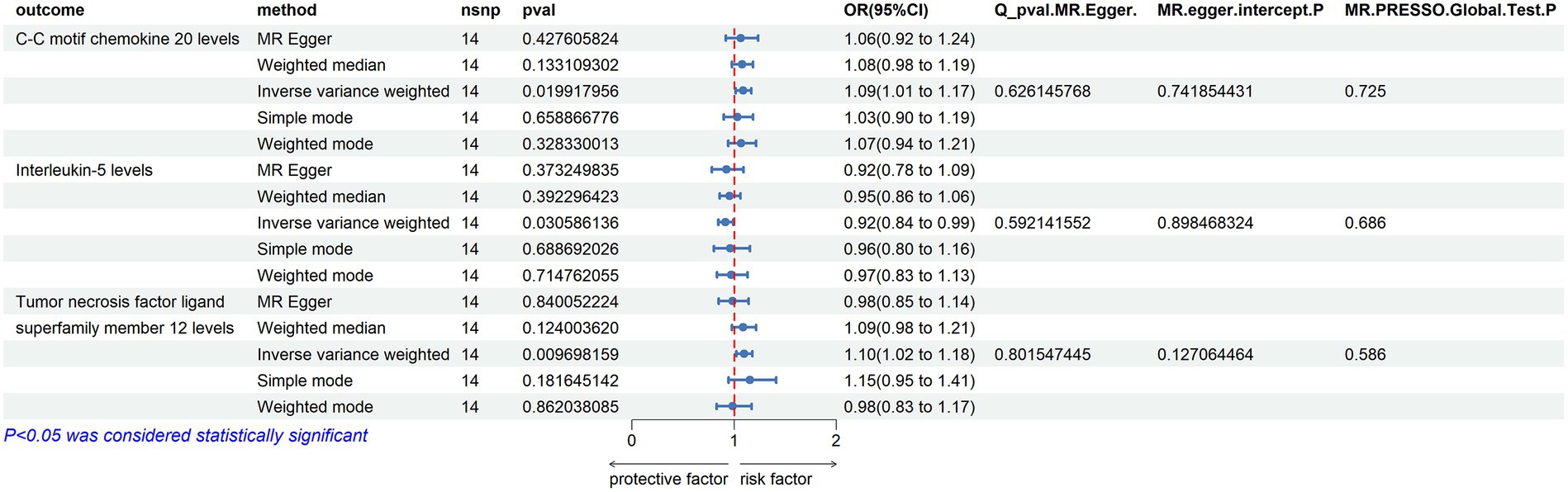
Figure 7. Reverse MR Results. Mendelian randomization analysis with ALS as the exposure factor and inflammatory proteins as the outcome, considering significance with p_ivw < 0.05.
A sensitivity analysis using the leave-one-out approach demonstrated robust results (Figure 8) (Supplementary Tables S2, S6, S11).
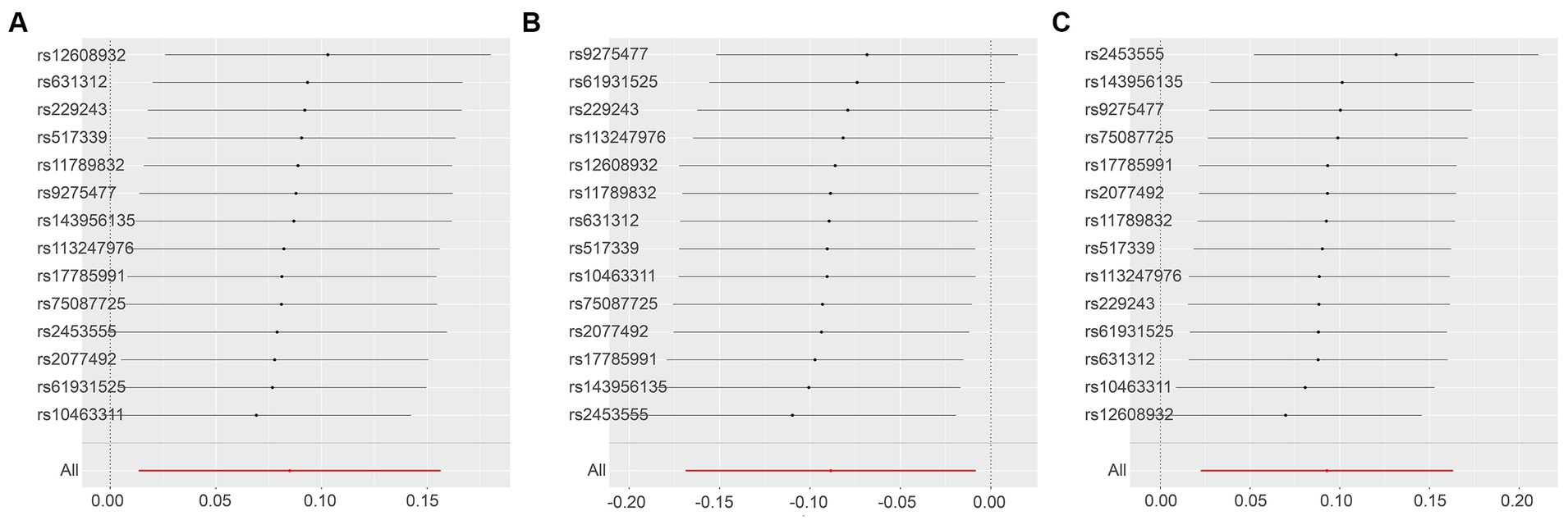
Figure 8. Leave-One-Out Analysis Results for Various Outcomes with ALS Exposure. This series illustrates the influence of ALS as an exposure factor on different inflammatory markers, using the leave-one-out methodology to assess the impact of individual SNPs on the overall results. (A): C-C motif chemokine 20 levels as the outcome, with ALS as the exposure; (B): Interleukin-5 levels as the outcome, with ALS as the exposure; (C): Tumor necrosis factor ligand superfamily member 12 levels as the outcome, with ALS as the exposure.
3.3 Validation group results
We selected three SNP thresholding tool variables (p < 5 × 10−8, p < 5 × 10−7, p < 5 × 10−6) and 12 inflammatory proteins as exposures, with ALS as the outcome, for two-sample Mendelian randomization analysis. The clumping conditions are the same as those for the test set. We were surprised to find that, whether in the initial exploration phase or in the validation set, the levels of osteoprotegerin showed significance for sporadic ALS (OR < 1, PIVW < 0.05) (Figure 9) (Supplementary Tables S2–S4). This result suggests that there may be an association between Osteoprotegerin levels and sporadic ALS, where Osteoprotegerin levels may play a protective role.
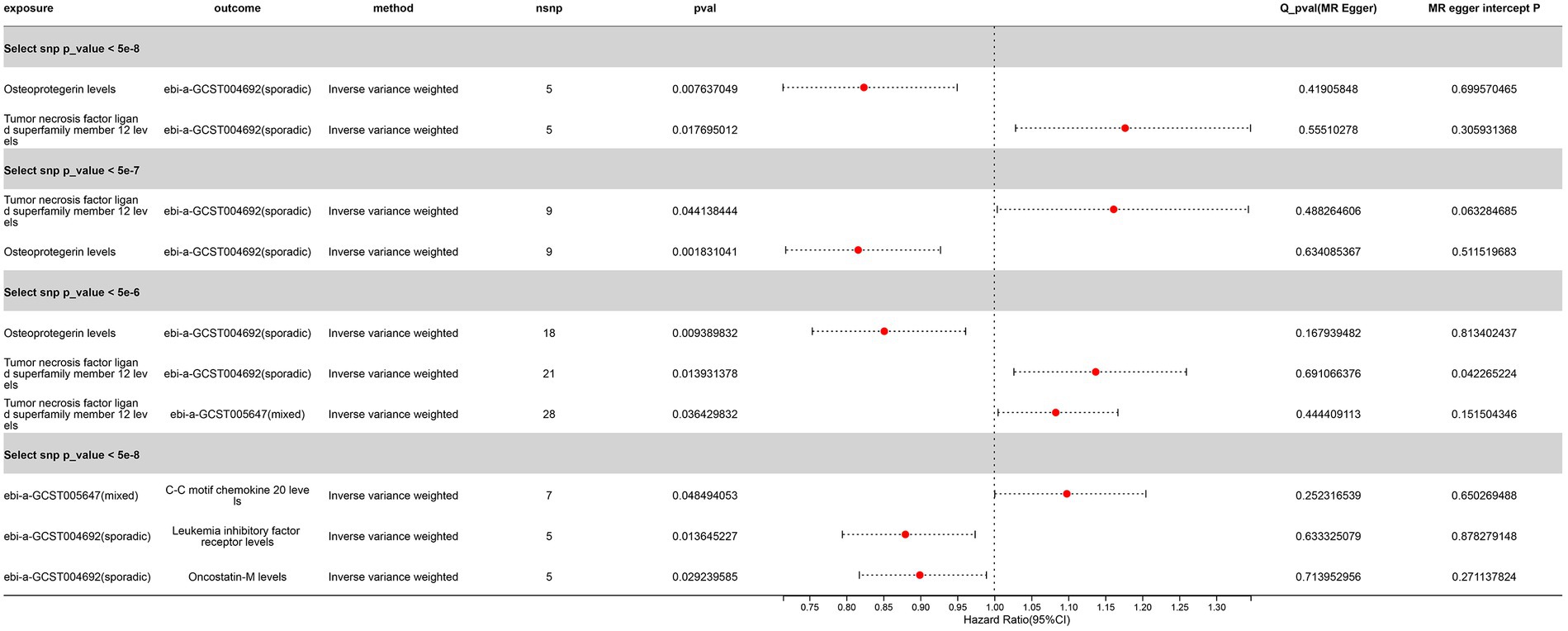
Figure 9. Significant Mendelian Randomization Results of Inflammatory Proteins with ALS Validation Sets (p_ivw < 0.05 denotes significance). “Sporadic” denotes a cohort of sporadic ALS, while “Mixed” refers to a combined cohort of familial and sporadic ALS.
Another remarkable discovery is the correlation of Tumor Necrosis Factor Ligand Superfamily Member 12 levels with the sporadic ALS validation set across three different SNP threshold groups, with a potential association observed with the mixed ALS set at SNP (p < 5 × 10−6) (OR > 1, P IVW < 0.05) (Figure 9) (Supplementary Tables S2–S4). This suggests that an increase in Tumor necrosis factor ligand superfamily member 12 levels may be a risk factor for ALS. However, we observed the opposite causal relationship in the initial GWAS set.
These results suggest complex interactions between ALS and Tumor Necrosis Factor Ligand Superfamily Member 12 levels, possibly involving bidirectional causal relationships.
4 Discussion
This study utilized the TSMR approach to explore the potential causal relationship between circulating inflammatory proteins and ALS. Our analysis revealed several significant associations between inflammatory proteins and the risk of ALS, offering new insights into the pathophysiology of ALS and potentially unveiling new therapeutic targets.
In our study, different p-value thresholds significantly impacted the results. Lower p-value thresholds (e.g., < 5.0E−08) are commonly employed to ensure robustness of associations, reduce heterogeneity, and enhance the accuracy of the research. However, such stringent criteria might also exclude potentially meaningful associations (Bottigliengo et al., 2022; Chen et al., 2023; Liu et al., 2023; Ren et al., 2023). Our analysis indicated that some associations, previously insignificant, became significant when the p-value threshold was relaxed, underscoring the importance and complexity of p-value selection in research on the relationship between inflammatory proteins and ALS.
Our study employed both forward and reverse MR analyses, a method that allows for a more comprehensive exploration of the potential causal relationship between inflammatory proteins and ALS (Perry et al., 2021; Huimei Huang et al., 2022; Wang et al., 2022; Yin et al., 2023). The forward MR analysis revealed associations between increased levels of specific inflammatory proteins and an increased risk of ALS, whereas the reverse MR analysis provided evidence that an increased risk of ALS could lead to changes in certain inflammatory protein levels. These findings suggest a possible bidirectional causal relationship between inflammatory proteins and ALS, further complicating their interaction.
In our research, multiple inflammatory proteins identified across various p-value thresholds showed significant positive results related to ALS risk. For instance, increased levels of ADA were significantly associated with an increased risk of ALS. Previous studies have suggested that ADA may play an important role in neurodegenerative diseases, linked to neuronal damage and inflammatory responses. Allen et al. identified a defect in adenosine to inosine deamination in astrocytes of ALS patients caused by reduced ADA expression. This defect led to increased sensitivity to adenosine-mediated toxicity (Allen et al., 2019). Supplementing inosine could reverse motor neuron toxicity observed in co-cultured patient astrocytes (Allen et al., 2018). Song et al. explored gene therapy for ALS by upregulating ADAR2 in mouse motor neurons using adeno-associated viral vectors. This treatment prevented progressive motor dysfunction and rescued motor neurons from death by normalizing TDP-43 expression, suggesting a potential gene therapy approach for ALS (Song and Pan, 2014).
In summary, this section of the study discusses the significant associations found between changes in Leukemia inhibitory factor receptor and Osteoprotegerin levels and the risk of ALS. It highlights the diverse roles of cytokines, including LIFR, in skeletal muscle physiology and their impact on muscle cell growth, differentiation, metabolism, nerve innervation, and inflammatory cell recruitment to muscle injury sites (Hunt and White, 2016). The research also touches on the limited but emerging findings linking AIFR and Osteoprotegerin to ALS, as well as their roles in neuropsychiatric disorders, emphasizing the importance of inflammation and immune mechanisms in these conditions (Ham et al., 2018; Hashioka et al., 2019; Novellino et al., 2020; Xu et al., 2023).
Furthermore, the study finds significant correlations between ALS risk and changes in levels of various inflammatory proteins, such as Interleukin-17C, Oncostatin-M, Interleukin-5 levels, SIR2-like protein 2 levels, Neurturin levels, TNF-beta levels and Interleukin-10, under different p-value thresholds. Reverse MR analysis suggests that increased ALS risk could lead to changes in certain inflammatory protein levels, such as motif chemokine 20 levels, Tumor necrosis factor ligand superfamily member 12 levels and Interleukin-5 levels. These findings offer new perspectives for research into the roles of these proteins in neuroprotection, neuroregeneration, and inflammation, potentially contributing to understanding and treating ALS and related neuropsychiatric disorders.
In summary, our study, based on GWAS data from European populations, suggests that Osteoprotegerin levels confer a protective effect against sporadic ALS, validated in two datasets. Additionally, we observed a complex bidirectional relationship between Tumor Necrosis Factor Ligand Superfamily Member 12 levels and sporadic ALS. Furthermore, some correlations were found in the GWAS dataset combining Tumor Necrosis Factor Ligand Superfamily Member 12 with familial and sporadic ALS, highlighting the potential complex bidirectional association between Tumor Necrosis Factor Ligand Superfamily Member 12 levels and ALS. Future research can delve into the specific roles of Osteoprotegerin and Tumor Necrosis Factor Ligand Superfamily Member 12 in the pathogenesis of ALS, assess their potential as biomarkers, and explore therapeutic strategies targeting them.
The findings of this study rely on data from the European population, implying that the applicability of its conclusions may have certain limitations. Although these inflammatory proteins show significant associations for some ALS patients within the European population, we must acknowledge that ALS patients in other populations worldwide may exhibit different levels of correlation and significance. Therefore, to comprehensively understand the role of these inflammatory proteins and their differences across diverse populations, future research should focus on collecting and analyzing data from more varied population groups. Such research endeavors will help uncover the population-specific aspects of ALS pathogenesis, thereby laying the groundwork for the discovery of universally applicable therapeutic strategies.
5 Conclusion
In summary, our study offers new insights into the role of circulating inflammatory proteins in ALS and paves the way for future research and the development of therapeutic strategies. Future research should focus on validating these findings and exploring the relationships between other potential inflammatory proteins and ALS. Furthermore, a deeper investigation into the specific roles of these inflammatory proteins in ALS pathophysiology will be crucial.
Data availability statement
The original contributions presented in the study are included in the article/Supplementary material, further inquiries can be directed to the corresponding author.
Author contributions
CX: Conceptualization, Data curation, Formal analysis, Funding acquisition, Investigation, Methodology, Project administration, Resources, Software, Supervision, Validation, Visualization, Writing – original draft, Writing – review & editing. XG: Writing – original draft, Writing – review & editing. YF: Writing – original draft, Writing – review & editing. JS: Conceptualization, Data curation, Formal analysis, Funding acquisition, Investigation, Methodology, Project administration, Resources, Software, Supervision, Validation, Visualization, Writing – original draft, Writing – review & editing.
Funding
The author(s) declare that financial support was received for the research, authorship, and/or publication of this article. This work was supported by basic research in medical application and applied research in medical innovation project (SKY2023101) and Suzhou Science and Technology Bureau - Key Technology (SS202089 and SZFCXK202107).
Acknowledgments
In the vastness of space and immensity of time, it is my joy to spend a planet and an epoch with her.
Conflict of interest
The authors declare that the research was conducted in the absence of any commercial or financial relationships that could be construed as a potential conflict of interest.
Publisher’s note
All claims expressed in this article are solely those of the authors and do not necessarily represent those of their affiliated organizations, or those of the publisher, the editors and the reviewers. Any product that may be evaluated in this article, or claim that may be made by its manufacturer, is not guaranteed or endorsed by the publisher.
Supplementary material
The Supplementary material for this article can be found online at: https://www.frontiersin.org/articles/10.3389/fnagi.2024.1367106/full#supplementary-material
Footnotes
References
Akinduro, O. O., Suarez-Meade, P., Garcia, D., Brown, D. A., Sarabia-Estrada, R., Attia, S., et al. (2021). Targeted therapy for Chordoma: key molecular Signaling pathways and the role of multimodal therapy. Target. Oncol. 16, 325–337. doi: 10.1007/s11523-021-00814-5
Akiyama, T., Koike, Y., Petrucelli, L., and Gitler, A. (2022). Cracking the cryptic code in amyotrophic lateral sclerosis and frontotemporal dementia: towards therapeutic targets and biomarkers. Clin. Transl. Med. 12:e818. doi: 10.1002/ctm2.818
Allen, S. P., Hall, B., Castelli, L., Francis, L., Woof, R., Higginbottom, A., et al. (2018). Inosine reverses motor neuron toxicity observed in amyotrophic lateral sclerosis patient astrocytes with an adenosine deaminase deficiency. Biochimica et Biophysica Acta 1859:e23. doi: 10.1016/J.BBABIO.2018.09.071
Allen, S. P., Hall, B., Castelli, L., Francis, L., Woof, R., Siskos, A., et al. (2019). Astrocyte adenosine deaminase loss increases motor neuron toxicity in amyotrophic lateral sclerosis. Brain 142:586. doi: 10.1093/brain/awy353
Bottigliengo, D., Foco, L., Seibler, P., Klein, C., König, I., and Del Greco, M. (2022). A Mendelian randomization study investigating the causal role of inflammation on Parkinson’s disease. Brain 145, 3444–3453. doi: 10.1093/brain/awac193
Bowden, J., Davey Smith, G., Haycock, P. C., and Burgess, S. (2016). Consistent estimation in Mendelian randomization with some invalid instruments using a weighted median estimator. Genet. Epidemiol. 40, 304–314. doi: 10.1002/gepi.21965
Burgess, S., Butterworth, A., and Thompson, S. G. (2013). Mendelian randomization analysis with multiple genetic variants using summarized data. Genet. Epidemiol. 37, 658–665. doi: 10.1002/gepi.21758
Carroll, D. (2022). Integrating experience with databases, bioinformatics, and wet lab exercises for students in an introductory genetics course. Biochem. Mol. Biol. Educ. 50, 457–459. doi: 10.1002/bmb.21649
Chen, J., Song, B., Feng, Q., Feng, Y., Yu, Q., He, P., et al. (2023). AB0154 MENDELIAN randomization study implies causal linkage between POLYMYOSITIS and physical ACTIVITY. Ann. Rheum. Dis. 82, 1256.2–1256.1256. doi: 10.1136/annrheumdis-2023-eular.4215
Davey Smith, G., and Hemani, G. (2014). Mendelian randomization: genetic anchors for causal inference in epidemiological studies. Hum. Mol. Genet. 23, R89–R98. doi: 10.1093/hmg/ddu328
Emdin, C. A., Khera, A. V., and Kathiresan, S. (2017). Mendelian Randomization. JAMA 318, 1925–1926. doi: 10.1001/jama.2017.17219
Gleixner, A., Morris Verdone, B., Otte, C. G., Anderson, E. N., Ramesh, N., Shapiro, O. R., et al. (2022). NUP62 localizes to ALS/FTLD pathological assemblies and contributes to TDP-43 insolubility. Nat. Commun. 13, 3380–3317. doi: 10.1038/s41467-022-31098-6
Ham, S., Kim, T., Hong, H., Kim, Y. S., Tang, Y., and Im, H. I. (2018). Big data analysis of genes associated with neuropsychiatric disorders in an Alzheimer’s disease animal model. Front. Neurosci. 12:407. doi: 10.3389/fnins.2018.00407
Hashioka, S., Inoue, K., Miyaoka, T., Hayashida, M., Wake, R., Oh-Nishi, A., et al. (2019). The possible causal link of periodontitis to neuropsychiatric disorders: more than psychosocial mechanisms. Int. J. Mol. Sci. 20:3723. doi: 10.3390/ijms20153723
Hemani, G., Bowden, J., and Davey Smith, G. (2018). Evaluating the potential role of pleiotropy in Mendelian randomization studies. Hum. Mol. Genet. 27, R195–r208. doi: 10.1093/hmg/ddy163
Henkel, J. S., Engelhardt, J. I., Siklós, L., Simpson, E. P., Kim, S. H., Pan, T., et al. (2004). Presence of dendritic cells, MCP-1, and activated microglia/macrophages in amyotrophic lateral sclerosis spinal cord tissue. Ann. Neurol. 55, 221–235. doi: 10.1002/ana.10805
Hosaka, T., Tsuji, H., and Kwak, S. (2023). Roles of aging, circular RNAs, and RNA editing in the pathogenesis of amyotrophic lateral sclerosis: potential biomarkers and therapeutic targets. Cells 12:1443. doi: 10.3390/cells12101443
Huimei Huang, S., Cheng, C.’e. L., Cheng, B., Liu, L., Xuena Yang, P., Meng, Y. Y., et al. (2022). Dissecting the association between psychiatric disorders and neurological proteins: a genetic correlation and two-sample bidirectional Mendelian randomization study. Neuropsychopharmacology 34, 311–317. doi: 10.1017/neu.2022.10
Hunt, L., and White, J. (2016). The role of Leukemia inhibitory factor receptor Signaling in skeletal muscle growth, injury and disease. Advances in experimental medicine and biology, 900, 45–59. Springer, Cham
Jiang, Z. Z., Wang, Z., Wei, X. J., and Yu, X. F. (2022). Inflammatory checkpoints in amyotrophic lateral sclerosis: from biomarkers to therapeutic targets. Front. Immunol. 13:59994. doi: 10.3389/fimmu.2022.1059994
Lee, G., Yao, C., Hwang, S.-J., Ma, J., Joehanes, R., Lee, D. H., et al. (2023). Integrative Mendelian randomization reveals the soluble receptor for advanced glycation end products as protective in relation to rheumatoid arthritis. Sci. Rep. 13:8002. doi: 10.1038/s41598-023-35098-4
Lehrach, H., Schäfer, R., and Schlag, P. M. (2011). “Deep sequencing”und prädiktive Modellierung als Konzept therapeutischer Entscheidungsfindungen in der Onkologie [deep sequencing and predictive modeling as a concept for therapeutic decision-making in oncology]. Onkologe 17, 477–486. doi: 10.1007/s00761-011-2025-9
Liu, R., Shi, X., Feng, J., Piao, J., Yang, Z., Zhao, Y., et al. (2023). Ischemic stroke and cerebral microbleeds: a two-sample bidirectional Mendelian randomization study. Neurol Ther 12, 1299–1308. doi: 10.1007/s40120-023-00500-w
Liu, W.-S., Zhang, Y.-R., Ge, Y.-J., Wang, H., Cheng, W., and Yu, J. (2023). Inflammation and brain structure in Alzheimer’s disease and other neurodegenerative disorders: A Mendelian randomization study
Masrori, P., and Van Damme, P. (2020). Amyotrophic lateral sclerosis: a clinical review. Eur. J. Neurol. 27, 1918–1929. doi: 10.1111/ene.14393
Mead, R. J., Shan, N., Reiser, J., Marshall, F., and Shaw, P. J. (2023). Amyotrophic lateral sclerosis: a neurodegenerative disorder poised for successful therapeutic translation. Nat. Rev. Drug Discov. 22, 185–212. doi: 10.1038/s41573-022-00612-2
Murdock, B. J., Bender, D. E., Segal, B. M., and Feldman, E. L. (2015). The dual roles of immunity in ALS: injury overrides protection. Neurobiol. Dis. 77, 1–12. doi: 10.1016/j.nbd.2014.12.015
Nicolas, A., Kenna, K. P., Renton, A. E., Ticozzi, N., Faghri, F., Chia, R., et al. (2018). Genome-wide analyses identify KIF5A as a novel ALS gene. Neuron 97, 1268–1283.e6. doi: 10.1016/j.neuron.2018.02.027
Novellino, F., Sacca, V., Donato, A., Zaffino, P., Spadea, M., Vismara, M., et al. (2020). Innate immunity: a common denominator between neurodegenerative and neuropsychiatric diseases. Int. J. Mol. Sci. 21:1115. doi: 10.3390/ijms21031115
Perry, B., Burgess, S., Jones, H., Zammit, S., Upthegrove, R., Mason, A., et al. (2021). The potential shared role of inflammation in insulin resistance and schizophrenia: a bidirectional two-sample mendelian randomization study. PLoS Med. 18:e1003455. doi: 10.1371/journal.pmed.1003455
Rajasundaram, S., and Gill, D. (2023). Tumour necrosis factor receptor 1 inhibition and cardiovascular disease: A cis-Mendelian randomization study
Ralli, M., Lambiase, A., Artico, M., de Vincentiis, M., and Greco, A. (2019). Amyotrophic lateral sclerosis: autoimmune pathogenic mechanisms, clinical features, and therapeutic perspectives. Israel Med. Assoc. J. 21, 438–443.
Ren, F., Jin, Q.-Y., Qian, Y., Ren, X., Liu, T., and Zhan, Y. (2023). Genetic evidence supporting the causal role of gut microbiota in chronic kidney disease and chronic systemic inflammation in CKD: a bilateral two-sample Mendelian randomization study. Front. Immunol. 14:87698. doi: 10.3389/fimmu.2023.1287698
Sankaran, S. M., Smith, J. D., and Roy, K. R. (2021). CRISPR-Cas9 gene editing in yeast: a molecular biology and bioinformatics laboratory module for undergraduate and high school students. J. Microbiol. Biol. Educ. 22:e21. doi: 10.1128/jmbe.00106-21
Sari, I. J., Pongsophon, P., Vongsangnak, W., Pimthong, P., and Pitiporntapin, S. (2022). The development of molecular genetics concept test for senior high school students using Rasch analysis. Int. J. Eval. Res. Educ. 11, 1687–1458. doi: 10.11591/ijere.v11i4.21846
Shao, W., Todd, T. W., Wu, Y., Jones, C. Y., Tong, J., Jansen-West, K. R., et al. (2022). Two FTD-ALS genes converge on the endosomal pathway to induce TDP-43 pathology and degeneration. Science 378:94. doi: 10.1126/science.abq7860
Smith, G. D., and Ebrahim, S. (2003). Mendelian randomization: can genetic epidemiology contribute to understanding environmental determinants of disease? Int. J. Epidemiol. 32, 1–22. doi: 10.1093/ije/dyg070
Song, C. J., Bian, M. Y., Lei, L., and Chen, L. L. (2022). Mendelian randomization and its application in periodontitis
Song, Y., and Pan, W. (2014). Exploration of the pathogenesis of amyotrophic lateral sclerosis from the perspective of motor neuron TDP-43 protein expression and ADAR2 Activity. Neurodegener. Dis. 1, 119–125. doi: 10.1159/000368927
Soremekun, O. S., Musanabaganwa, C., Uwineza, A., Ardissino, M., Rajasundaram, S., Wani, A., et al. (2023). Transl Psychiatry 13:2542. doi: 10.1038/s41398-023-02542-y
Tejwani, L., Jung, Y., Kokubu, H., Sowmithra, S., Ni, L., Lee, C., et al. (2023). Reduction of nemo-like kinase increases lysosome biogenesis and ameliorates TDP-43–related neurodegeneration. J. Clin. Invest. 133:e138207. doi: 10.1172/JCI138207
The Telomeres Mendelian Randomization CollaborationHaycock, P. C., Burgess, S., Nounu, A., Zheng, J., Okoli, G. N., et al. (2017). Association between telomere length and risk of cancer and non-neoplastic diseases: a Mendelian randomization study. JAMA Oncol. 3, 636–651. doi: 10.1001/jamaoncol.2016.5945
van Rheenen, W., Shatunov, A., Dekker, A. M., McLaughlin, R. L., Diekstra, F. P., Pulit, S. L., et al. (2016). Genome-wide association analyses identify new risk variants and the genetic architecture of amyotrophic lateral sclerosis. Nat. Genet. 48, 1043–1048. doi: 10.1038/ng.3622
van Rheenen, W., van der Spek, R. A. A., Bakker, M. K., van Vugt, J. J. F. A., Hop, P. J., Zwamborn, R. A. J., et al. (2021). Common and rare variant association analyses in amyotrophic lateral sclerosis identify 15 risk loci with distinct genetic architectures and neuron-specific biology. Nat. Genet. 53, 1636–1648. doi: 10.1038/s41588-021-00973-1
Verbanck, M., Chen, C. Y., Neale, B., and do, R. (2018). Detection of widespread horizontal pleiotropy in causal relationships inferred from Mendelian randomization between complex traits and diseases. Nat. Genet. 50, 693–698. doi: 10.1038/s41588-018-0099-7
Wang, Q., Shi, Q., Jiawen, L., Wang, Z., and Hou, J. (2022). Causal relationships between inflammatory factors and multiple myeloma: a bidirectional Mendelian randomization study. Int. J. Cancer 151, 1750–1759. doi: 10.1002/ijc.34214
Witzel, S., Mayer, K., and Oeckl, P. (2022). Biomarkers for amyotrophic lateral sclerosis. Curr. Opin. Neurol. 35, 699–704. doi: 10.1097/WCO.0000000000001094
Wu, J., and Lin, Z. (2022). Non-small cell lung cancer targeted therapy: drugs and mechanisms of drug resistance. Int. J. Mol. Sci. 23:15056. doi: 10.3390/ijms232315056
Xu, H., Zheng, L., Wang, L., Gao, H., Wei, Y., and Chen, J. (2023). Albumin and associated biomarkers in severe neuropsychiatric disorders: acute-phase schizophrenia and bipolar disorder. Neuropsychiatr. Dis. Treat. 19, 2027–2037. doi: 10.2147/NDT.S423399
Yang, X., Ji, Y., Wang, W., Zhang, L., Chen, Z., Yu, M., et al. (2021). Amyotrophic lateral sclerosis: molecular mechanisms, biomarkers, and therapeutic strategies. Antioxidants (Basel) 10:1012. doi: 10.3390/antiox10071012
Yin, Z., Chen, J., Xia, M., Zhang, X.-y., Li, Y., Chen, Z.-h., et al. (2023). Assessing causal relationship between circulating cytokines and age-related neurodegenerative diseases: a bidirectional two-sample Mendelian randomization analysis. Sci. Rep. 13:12325. doi: 10.1038/s41598-023-39520-9
Zhao, J. H., Stacey, D., Eriksson, N., Macdonald-Dunlop, E., Hedman, Å. K., Kalnapenkis, A., et al. (2023). Genetics of circulating inflammatory proteins identifies drivers of immune-mediated disease risk and therapeutic targets. Nat. Immunol. 24, 1540–1551. doi: 10.1038/s41590-023-01588-w
Keywords: amyotrophic lateral sclerosis, circulating inflammatory protein, two-sample mendelian randomization, osteoprotegerin, tumor necrosis factor
Citation: Xiao C, Gu X, Feng Y and Shen J (2024) Two-sample Mendelian randomization analysis of 91 circulating inflammatory protein levels and amyotrophic lateral sclerosis. Front. Aging Neurosci. 16:1367106. doi: 10.3389/fnagi.2024.1367106
Edited by:
Robert Weissert, University of Regensburg, GermanyReviewed by:
Deepti Lall, Cedars Sinai Medical Center, United StatesFuyi Xu, University of Tennessee Health Science Center (UTHSC), United States
Copyright © 2024 Xiao, Gu, Feng and Shen. This is an open-access article distributed under the terms of the Creative Commons Attribution License (CC BY). The use, distribution or reproduction in other forums is permitted, provided the original author(s) and the copyright owner(s) are credited and that the original publication in this journal is cited, in accordance with accepted academic practice. No use, distribution or reproduction is permitted which does not comply with these terms.
*Correspondence: Jing Shen, MTY1MzQ0NDQwQHFxLmNvbQ==
†These authors have contributed equally to this work and share first authorship