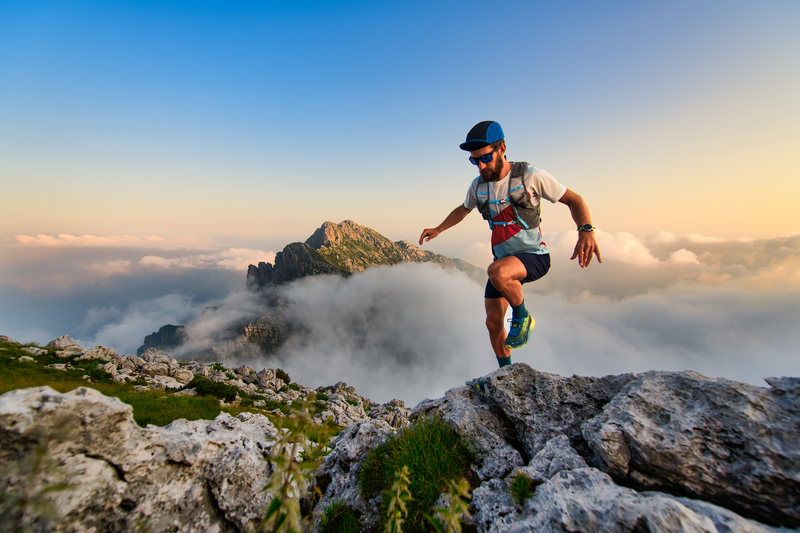
94% of researchers rate our articles as excellent or good
Learn more about the work of our research integrity team to safeguard the quality of each article we publish.
Find out more
ORIGINAL RESEARCH article
Front. Aging Neurosci. , 17 September 2024
Sec. Alzheimer's Disease and Related Dementias
Volume 16 - 2024 | https://doi.org/10.3389/fnagi.2024.1338179
This article is part of the Research Topic Emotional Affective Disorders in Aging and Alzheimer’s Disease and Their Impact on Higher Order Cognition View all 6 articles
Background: Although depression symptoms are commonly reported in patients with subcortical vascular mild cognitive impairment (svMCI), their impact on brain functions remains largely unknown, with diagnoses mainly dependent on behavioral assessments.
Methods: In this study, we analyzed resting-state fMRI data from a cohort of 34 svMCI patients, comprising 18 patients with depression symptoms (svMCI+D) and 16 patients without (svMCI-D), along with 34 normal controls (NC). The study used the fraction of the amplitude of low-frequency fluctuations (fALFF), resting-state functional connectivity, correlation analyses, and support vector machine (SVM) techniques.
Results: The fALFF of the right cerebellum (CERE.R) differed among the svMCI+D, svMCI-D, and NC groups. Specifically, the regional mean fALFF of CERE. R was lower in svMCI-D patients compared to NC but higher in svMCI+D patients compared to svMCI-D patients. Moreover, the adjusted fALFF of CERE. R showed a significant correlation with Montreal Cognitive Assessment (MOCA) scores in svMCI-D patients. The fALFF of the right orbital part of the superior frontal gyrus was significantly correlated with Hamilton Depression Scale scores in svMCI+D patients, whereas the fALFF of the right postcingulate cortex (PCC.R) showed a significant correlation with MOCA scores in svMCI-D patients. Furthermore, RSFC between PCC. R and right precuneus, as well as between CERE. R and the right lingual gyrus (LING.R), was significantly reduced in svMCI-D patients compared to NC. In regional analyses, the adjusted RSFC between PCC. R and PreCUN. R, as well as between CERE. R and LING. R, was decreased in svMCI-D patients compared to NC but increased in svMCI+D patients compared to svMCI-D. Further SVM analyses achieved good performances, with an area under the curve (AUC) of 0.82 for classifying svMCI+D, svMCI-D, and NC; 0.96 for classifying svMCI+D and svMCI-D; 0.82 for classifying svMCI+D and NC; and 0.92 for classifying svMCI-D and NC.
Conclusion: The study revealed disruptive effects of cognitive impairment, along with both disruptive and complementary effects of depression symptoms on spontaneous brain activity in svMCI. Moreover, these findings suggest that the identified features might serve as potential biomarkers for distinguishing between svMCI+D, svMCI-D, and NC, thereby guiding clinical treatments such as transcranial magnetic stimulation for svMCI.
Mild cognitive impairment (MCI) has been considered a preclinical stage of dementia or Alzheimer’s disease (AD) (Lyketsos et al., 2002). In addition to cognitive impairment, neuropsychiatric symptoms (Donaghy et al., 2018; Lo et al., 2020; Gallagher et al., 2017), especially depression, have been commonly observed in MCI, as well as in frontotemporal dementia and Lewy-body disease, with an overall pooled prevalence of 32% (Ismail et al., 2017; Cotta et al., 2021).
Moreover, several meta-analyses have consistently shown that depression is associated with a higher likelihood of progression from MCI to AD (Ismail et al., 2017; Gao et al., 2013; Cooper et al., 2015; Mourao et al., 2016). As one of the common subtypes of MCI, subcortical vascular mild cognitive impairment (svMCI) is clinically characterized by several neuroimaging features, including white matter hyperintensities, lacunar infarction, micro-infarcts, micro-bleeds, enlarged perivascular spaces, and brain atrophy. Among them, vascular lesions in the deep and periventricular white matter and in the deep gray matter, as well as a greater number of perivascular spaces in the basal ganglia, are particularly implicated in cognitive decline, potentially leading to vascular dementia (Seo et al., 2009; Lee et al., 2018; Román et al., 2002; Ramusino et al., 2022; Wardlaw et al., 2013). Similar to MCI, depression in svMCI has been reported not only as a predictive marker for conversion to subcortical vascular dementia (SvaD) (Kim et al., 2013) but also as a factor that exacerbates cognitive impairments (Steffens, 2012; Cinar et al., 2014).
Given that depression in svMCI may be reversible through the management of risk factors (Ravaglia et al., 2005; Jak et al., 2009), exploring the underlying neurobiological mechanisms of depression in the svMCI is crucial for preventing the progression from svMCI to SvaD.
The amplitude of low-frequency fluctuation (ALFF) and fractional ALFF (fALFF) (Zou et al., 2008) have been widely and effectively adopted to explore changing patterns in spontaneous brain activity in the low-frequency range in patients with MCI (Han et al., 2011; Han et al., 2012; Zhao et al., 2015; Yang et al., 2018; Wang et al., 2019) and svMCI (Yi et al., 2012). These measures have proven effective in characterizing early and gradual physiological changes associated with AD (Yang et al., 2018). However, these studies showed inconsistent results, showing both increased and/or decreased activity patterns in different brain regions. The potential effect of co-existing neuropsychiatric symptoms, particularly depression, on brain function is an important factor that must not be overlooked.
Recently, there has been growing interest in understanding how depression symptoms affect brain activity in MCI (Li et al., 2017; Liu et al., 2018; Yu et al., 2019). For example, one study showed significant differences in fALFF across the full frequency, slow-5, and slow-4 bands in MCI patients with depression (Li et al., 2017). Another study identified decreased fALFF in regions such as the temporal gyrus, frontal gyrus, inferior occipital gyrus, middle frontal gyrus, and cerebellum, alongside increases in the cuneus, calcarine, and lingual gyrus in MCI with depression (Yu et al., 2019).
To the best of our knowledge, no studies to date have explored the impact of depression symptoms on spontaneous brain activity in svMCI.
In the current study, we aimed to explore (1) whether and how depression symptoms affect spontaneous brain activity in patients with svMCI and (2) whether these altered neural indices can serve as biomarkers for diagnosing svMCI. To address these two questions, resting-state fMRI data were obtained from 34 svMCI patients, comprising 18 svMCI patients with depression symptoms (svMCI+D), 16 svMCI patients without depression symptoms (svMCI-D), and 34 normal controls (NC).
Then, several main steps were performed in the study (Figure 1): (1) comparisons of ALFF and fALFF between svMCI and NC groups; (2) comparisons of ALFF and fALFF among svMCI-D, svMCI-D, and NC groups; (3) correlation analyses between ALFF/fALFF and Hamilton Depression Scale (HAMD)/Montreal Cognitive Assessment (MOCA) scores in the svMCI+D group, as well as between ALFF/fALFF and MOCA scores in the svMCI-D group; (4) analysis of resting-state functional connectivity (RSFC) to identify related spontaneous brain functional patterns; and (5) multivariate pattern analyses using a linear support vector machine (SVM) to determine whether these altered neural indices can serve as biomarkers for diagnosing svMCI.
Figure 1. Flowchart of study design. ALFF, amplitude of low-frequency fluctuations, fALFF, fractions of ALFF; svMCI, subcortical vascular mild cognitive impairment; NC, normal controls; svMCI+D, svMCI with depression symptoms; svMCI-D patients, svMCI patients without depression symptoms; HAMD, Hamilton Depression Scale; MOCA, Montreal Cognitive Assessment; ANCOVA, analysis of covariance; RSFC, resting state functional connectivity; and SVM, support vector machine.
This study was conducted at the Shenzhen Traditional Chinese Medicine Hospital and approved by the Institutional Review Board. Patients and normal controls (NCs) were recruited through advertisements at the hospital, and all participants provided written informed consent. The svMCI patients were required to meet specific diagnostic, exclusion, and MRI criteria, whereas NC participants only needed to meet the exclusion criteria.
The diagnostic criteria for svMCI patients included: (1) reported cognitive impairments involving memory and/or other cognitive domains lasting for at least 3 months; (2) not classified as normal nor demented, with a clinical dementia rating of (CDR) > = 0.5 in at least one domain (Hughes et al., 1982) and a global score of <= 0.5 in the three functional CDR domains (Salloway et al., 2004); and (3) a MOCA score of <= 26.
The exclusion criteria were as follows: (1) history of psychiatric illness in any first-to third-degree biological relatives; (2) history of strokes or transient ischemic attack within the past 3 months; (3) history of seizures, schizophrenia, or major depressive disorder; (4) inherited or inflammatory small vessel disease; (5) previous head trauma with the loss of consciousness; (6) use of medications (e.g., Donepezil and Kabalatine) that may affect cognition; (7) serious medical or surgical illness; (8) physical disabilities that could prevent completion of neuropsychological testing; and (9) contraindications for MRI.
The MRI criterion was based on T2 FLAIR images, and patients needed to meet at least one of the following: (1) at least three supratentorial subcortical small infarcts (diameters ranged from 3 to 20 mm) with or without white matter lesions of any degree; (2) moderate to severe white matter lesions (a score of > = 2 according to the Fazekas rating scale (FRS)) (Fazekas et al., 1987) with/without small infarct; (3) one or more strategically located subcortical small infarcts in the caudate nucleus, globus pallidus, or thalamus.
The severity of depression symptoms in patients was assessed using the 17-item HAMD. Patients were then categorized into two groups: svMCI+D (HAMD score 8–17) and svMCI-D (HAMD score <7) (Zimmerman et al., 2013). Ultimately, 34 svMCI patients (18 svMCI+D and 16 svMCI-D) and 34 NC were included in this study (Table 1).
MRI data of all participants were acquired using a GE medical system 3 T scanner (MR750) in the radiology department. The fMRI images were obtained with the following parameters: repetition time (TR) = 2000 ms, echo time (TE) = 35 ms, flip angle = 90 degrees, matrix size = 64 × 64, slice thickness = 4 mm, voxel size = 4 × 4 × 4 mm3, field of view = 220 × 220 mm2, and volumes = 240. The parameters for T2 FLAIR images were as follows: TR = 9,000 ms, TE = 92.544 ms, flip angle = 160 degrees, matrix size = 256 × 224, slice thickness = 6.5 mm, and voxel size = 0.47 × 0.47 × 6.5 mm3.
The resting-state fMRI data were preprocessed using DPABI1 following these steps: (1) removal of the first 10 volumes; (2) slice timing correction; (3) realignment (subjects with head motion exceeding 3 mm in any dimension or 3° of angular motion were removed, leading to the removal of one svMCI-D patient); (4) spatial normalization using an echo planar imaging (EPI) template; (5) resampling to 3 mm isotropic voxels; (6) smoothing with a Gaussian kernel of 6 mm full-width at half maximum; (7) regressing of linear and quadratic trends, signals of 24 head motion parameters (Satterthwaite et al., 2013; Yan et al., 2013), as well as signals from the white matter and cerebrospinal fluid. For the RSFC analysis, additional three steps were performed: (1) regressing out global signals; (2) temporal band-pass filtering (0.01–0.1 Hz); and (3) “scrubbing” two-time points before and one-time points after bad images, with a frame displacement (FD) of >0.5 (Power et al., 2012).
Following preprocessing, voxel-wise ALFF and fALFF were calculated using DPABI. Similar to previous studies (Zou et al., 2008; Yang et al., 2007), the ALFF was defined as the mean square root of the power spectrum of time series within the 0.01–0.1 Hz frequency range, whereas fALFF was calculated as the ratio of the power spectrum of time series within the 0.01–0.01 Hz range to that of the entire frequency range. These values were further normalized using Fisher’s z transformation to improve normality.
For altered brain regions, RSFC of the whole brain was further calculated to investigate functional changing patterns. First, Pearson correlation coefficients were calculated between the mean time series of each altered brain region and that of each voxel in the rest of the brain. Then, each correlation coefficient was converted to z values using Fisher’s z transformation to improve normality.
Several main statistical steps were taken using DPABI: (1) two sample t-tests to examine differences in the voxel-wise ALFF and fALFF maps between svMCI and NC with age, sex, and education as covariates; (2) one-way analysis of covariance (ANCOVA) and post-hoc two-sample t-tests between any two groups to examine between-group differences in the voxel-wise ALFF, fALFF and RSFC maps among scMCI+D, svMCI-D, and NC patients with age, sex, and education as covariates; (3) correlation analyses between HAMD scores and voxel-wise ALFF and fALFF maps, with age, sex, education, and MOCA scores as covariates in the svMCI+D; (4) correlation analyses between MOCA scores and voxel-wise ALFF and fALFF maps, with age, sex, education, and HAMD scores as covariates in the svMCI+D; (5) correlation analyses between MOCA scores and voxel-wise ALFF and fALFF maps, with age, sex, education, and HAMD scores as covariates in the svMCI+D. All these statistical analyses were corrected using the Gaussian Random Field (GRF) corrections, with a voxel level of p < 0.001 and a cluster level of p < 0.05.
In addition, we calculated the regional mean fALFF and RSFC for the regions that showed significant differences among the three groups. Group differences were compared among three groups using ANCOVA and post-hoc t-tests between any two groups, with age, sex, and education as covariates, using IBM SPSS 19. We also conducted correlation analyses between regional mean fALFF and clinical measures for the svMCI+D and svMCI-D groups, respectively, using IBM SPSS 19. For the svMCI+D patients, age, sex, education, and MOCA scores were regressed out from the fALFF, whereas for the svMCI-D patients, age, sex, education, and HAMD scores were regressed out from the fALFF. The resulting values were defined as adjusted fALFF, following the same adjustment method applied to the RSFC. The significance level was set at p < 0.05.
To determine whether the identified neural indices might serve as biomarkers for the classification of svMCI+D, svMCI-D, and NC, we employed a linear support vector machine (SVM) using the LIBSVMs toolkit (Chang and Lin, 2011). The features used for classification include the mean regional fALFF of the right orbitofrontal cortex (OFC.R), right posterior cingulate cortex (PCC.R), and right cerebellum (CERE.R), as well as the functional connectivity between PCC. R and the right precuneus (PreCUN.R), and between CERE. R and the right lingual gyrus (LING.R). We conducted classifications among svMCI+D, svMCI-D, and NC, as well as between svMCI+D and svMCI-D, between svMCI+D and NC, and between svMCI-D and NC. Given the relatively small sample size, we used a leave-one-out cross-validation strategy to estimate the generalization ability of our classifiers. The performance of classifiers was assessed using the area under curve (AUC).
The normality of the distribution was analyzed using the Shapiro–Wilk test. The education years, MOCA scores, and HAMD scores were all normally distributed. Therefore, demographic and clinical measures between the two groups were analyzed using two-sample t-tests, whereas the three groups were analyzed using one-way ANOVA. Sex was analyzed using the chi-square (χ2) test. As a result, no significant difference was found in age, sex, or education, whereas significant differences were found in MOCA and HAMD scores between svMCI and NC, among svMCI+D, svMCI-D, and NC (Table 1).
No significant differences in ALFF and fALFF were observed between svMCI patients and NC. However, the fALFF of the CERE. R showed significant alterations among the svMCI+D, svMCI-D, and NC patients (Figure 2A). Specifically, the regional mean fALFF of CERE. R was decreased in svMCI-D patients compared to NC, but it increased in svMCI+D patients compared to svMCI-D patients (Figure 2B).
Figure 2. (A) ANCOVA of fALFF among svMCI+D, svMCI-D, and NC using DPABI with age, sex, and education as covariates. The results were corrected by Gaussian Random Field (GRF) with a voxel level p < 0.001 and a cluster level p < 0.05. (B) ANCOVA and post-hoc two-sample t-tests of mean fALFF were performed using SPSS.
No significant correlations were found between ALFF and clinical measurements in either the svMCI+D or svMCI-D patients. However, the fALFF of the OFC. R was negatively correlated with HAMD scores in svMCI+D patients after controlling for age, sex, education, and MOCA scores (Figures 3A,B). Additionally, the fALFF of the PCC. R was negatively correlated with MOCA scores in svMCI-D patients while controlling for age, sex, education, and HAMD scores (Figures 3C,D).
Figure 3. Results of correlation analyses. Correlation analyses between HAMD and whole brain fALFF in svMCI+D patients (A) with age, sex, education, and MOCA as covariates and between MOCA and whole brain fALFF in the svMCI-D (C) with age, sex, education, and HAMD as covariates were obtained using DPABI (see text footnote 1). The results were corrected by GRF with a voxel level p < 0.001 and a cluster level p < 0.05. Correlation analyses between HAMD and adjusted fALFF regressed by age, sex, education, and MOCA in the svMCI+D (B) and between MOCA and adjusted fALFF regressed by age, sex, education, and HAMD in the svMCI-D (D) were obtained using SPSS. The significant level was set at p < 0.05.
In voxel-wise analyses, RSFC between PCC. R and PreCUN. R, as well as between CERE. R and LING. R, was significantly decreased in svMCI-D patients compared to NC (Figures 4A,C). In regional analyses, the adjusted RSFC between PCC. R and PreCUN. R, as well as between CERE. R and LING. R, was decreased in svMCI-D patients compared to NC but increased in svMCI+D patients compared to svMCI-D patients (Figures 4B,D).
Figure 4. Differences in RSFC among the three groups. (A) The PreCUN.R showed significantly decreased RSFC with PCC. R in the svMCI-D compared to NC. (B) The mean RSFC between PCC. R and PreCUN. R was the lowest in the svMCI-D. (C) The LING. R showed significantly decreased RSFC with CERE. R in the svMCI-D compared to NC. (D) The mean RSFC between CERE. R and LING. R was the lowest in the svMCI-D. Abbreviations: PreCUN. R, the right precuneus; PCC. R, the right postcingulate cortex; CERE. R, the right cerebellum; and LINGnnn R, the right lingual gyrus.
The SVM classifier demonstrated strong performance, achieving an AUC of 0.82 for distinguishing among svMCI+D, svMCI-D, and NC; an AUC of 0.96 for distinguishing between svMCI+D and svMCI-D patients; an AUC of 0.82 for distinguishing between svMCI+D and NC; and an AUC of 0.92 for distinguishing between svMCI-D and NC (Figure 5).
In the present study, we conducted ALFF, fALFF, RSFC, and correlation analyses to investigate the impact of depression symptoms on spontaneous brain activity in svMCI patients and used SVM to assess whether the identified neural indices might serve as biomarkers for classifying svMCI+D, svMCI-D, and NC patients. Four main results emerged: (1) fALFF in CERE. R, RSFC between CERE. R and LING. R, and RSFC between PCC. R and PreCUN. R were decreased in svMCI-D patients compared to NC but increased in svMCI+D patients compared to svMCI-D patients; (2) fALFF in OFC. R was significantly correlated with depression symptoms in svMCI+D patients; (3) fALFF in PCC. R was significantly correlated with cognitive impairment in svMCI-D patients; and (4) further SVM analyses achieved good performances, with an AUC of 0.96 for distinguishing svMCI+D patients from svMCI-D patients.
First, we observed that the fALFF of CERE. R decreased in svMCI-D patients compared to NC but increased in svMCI+D patients compared to svMCI-D patients. Although the CERE is widely known to play an important role in regulating motor coordination, accumulating evidence has highlighted its significant involvement in cognitive regulation (Baillieux et al., 2008) and emotion processing (Stoodley and Schmahmann, 2009). Indeed, functional abnormalities in the CERE have been reported not only in patients with depression (Liu et al., 2013; Guo et al., 2015; Xu et al., 2017) but also across all various subtypes of MCI, including aMCI (Wang et al., 2016; Lin et al., 2020), vascular mild cognitive impairment (VaMCI) (Zuo et al., 2019; Diciotti et al., 2017), and svMCI (Seo et al., 2009; Yoon et al., 2013). Recent studies on MCI with depression have also noted decreased fALFF and greater loss of gray matter volume in the CERE (Yu et al., 2019; Capogna et al., 2019). In addition, two of our previous studies showed similar patterns of RSFC and white matter microstructure in the CERE. R in svMCI+D patients (Lyu et al., 2019; Xu et al., 2020).
The decreased brain activity in svMCI-D patients may be linked to the detrimental effects of cognitive impairment. However, the increased brain activity observed in svMCI+D patients could suggest compensatory effects related to depression symptoms, although we found no significant correlation between these alterations and HAMD scores. This lack of correlation may be due to the relatively small sample size, and caution is warranted when interpreting these findings. Nonetheless, identifying increased brain activity in svMCI+D patients is valuable for a better understanding of the underlying mechanism of depression in svMCI patients.
Then, we also found that the fALFF of the OFC. R was negatively correlated with HAMD scores in svMCI+D patients. The OFC is believed to be involved in the non-reward attractor theory of depression, a concept supported by many neurophysiological, functional neuroimaging, and lesion studies. Additionally, the OFC is a key component of the frontal-limbic circuit, which is closely associated with depressive symptoms (Rolls, 2016; Cotta Ramusino et al., 2024). Many previous studies showed decreased gray matter volume in the OFC among patients with depression (Lavretsky et al., 2005; Salvadore et al., 2011; Grieve et al., 2013; Kandilarova et al., 2019; Harada et al., 2016). Moreover, the efficiency of the right orbital part of the superior frontal gyrus (part of OFC) was also found to be significantly correlated with the severity of depression (Qin et al., 2014). Similarly, another study showed that depressive symptoms in MCI are associated with gray matter volume loss in the OFC (Xie et al., 2012). This body of evidence supports our finding that the decreased fALFF in the OFC. R is strongly associated with the detrimental effects of depression symptoms in svMCI+D patients.
In addition, we found that the fALFF of the PCC. R was negatively correlated with MOCA scores, suggesting that cognitive impairments in svMCI-D patients may have damaging effects. As a key region in the default mode network (Davey et al., 2016; Satpute and Lindquist, 2019), the PCC plays an important role in various cognitive functions, such as episodic memory, spatial attention, and self-evaluation (Davey et al., 2016; Bagurdes et al., 2008; Natu et al., 2019). Moreover, numerous histopathological (Pivtoraiko et al., 2015; Mitolo et al., 2019), metabolic (Johnson et al., 2006; Frings et al., 2010; Gusnard et al., 2001), structural (Zhao et al., 2015; Choo et al., 2010), and functional imaging (Wang et al., 2016; Krajcovicova et al., 2017; Teipel et al., 2017; Papma et al., 2017) studies have consistently shown that the PCC is highly associated with the pathophysiology of MCI and may even serve as an imaging predictor for further cognitive decline into clinical AD (Huang et al., 2002; Petrella et al., 2007; Zhou et al., 2008). A three-year longitudinal analysis also found that altered functional connectivity of the PCC was significantly correlated with cognitive performances in MCI (Wang et al., 2012). Although these results primarily focus on MCI, they are similar to our results, providing additional evidence that alterations in the PCC may be linked to cognitive impairment in svMCI patients.
To the best of our knowledge, only three previous studies have reported functional alterations of the PCC in svMCI patients. First, Sun et al. (2011) identified abnormal functional connectivity of the PCC using resting-state fMRI in patients with vascular cognitive impairment, no dementia (VCIND), and a clinical state with a vascular etiology similar to that of svMCI. Subsequently, Yi et al. (2012) observed significantly increased low-frequency oscillation amplitudes in the PCC in svMCI patients. Finally, significantly decreased rsFC of the PCC and its association with memory scores were identified in svMCI patients (Ding et al., 2015). Given all this evidence, it is much more reasonable to suggest that the fALFF of PCC. R might be associated with the damaging effects of cognitive impairment in svMCI patients.
Numerous previous studies have explored the effectiveness of machine learning in distinguishing NC from patients with SVaD, svMCI, AD, and MCI using multimodal MRI data, including diffusion, morphometry, functional, and combined features (Yang et al., 2018; Diciotti et al., 2015; Long et al., 2016; Guo et al., 2017). Among these, SVM with a linear kernel has been identified as the most effective classification algorithm for distinguishing AD from NC (Mao et al., 2017). Among all these studies, one study that used the Hurst exponent, ALFF, regional homogeneity, and gray matter density as classification features achieved an AUC of up to 0.97 in differentiating 29 MCI patients from 33 NC (Long et al., 2016). Additionally, two other studies confirmed that a combination of resting-state measures such as ALFF, fALFF, and RSFC improves classification accuracy (Yang et al., 2018; de Vos et al., 2018).
However, our study is the first to classify svMCI+D patients from svMCI-D patients using SVM, achieving a relatively high AUC of 0.96 with only five functional features: the mean regional fALFF of OFC. R, PCC. R, and CERE. R, as well as RSFC between PCC. R and PreCUN. R, and RSFC between CERE. R and LING.R. These features may serve as potential biomarkers for distinguishing svMCI+D patients from svMCI-D patients and could be instrumental in guiding the clinical treatment of svMCI+D patients.
Finally, two major limitations of this study need to be stressed. First, although we used GRF corrections with strict parameters (a voxel level of p < 0.001 and a cluster level of p < 0.05) to enhance statistical power, future studies with larger sample sizes are highly recommended to validate our results. Second, while we identified increased brain activity, we did not find a significant relationship with HAMD scores in svMCI+D patients. This lack of correlation may be due to the relatively small sample size, and therefore, caution is warranted in interpreting these results.
In conclusion, our results showed distinct patterns of the detrimental impact of cognitive impairment and depression symptoms on spontaneous brain activity in svMCI patients. However, further research is required to directly confirm the compensatory effects of depression symptoms in svMCI patients. In addition, features derived from spontaneous brain activity hold potential as biomarkers for differentiating between svMCI+D patients, svMCI-D patients, and NC, which could be valuable in guiding clinical treatment for svMCI patients, such as transcranial magnetic stimulation.
The raw data supporting the conclusions of this article can be accessed upon request from the corresponding author, provided that the privacy of the participants is protected and ethical approval from the hospital has been obtained.
The studies involving humans were approved by the Review Board of Shenzhen Traditional Chinese Medicine Hospital. The studies were conducted in accordance with the local legislation and institutional requirements. The participants provided their written informed consent to participate in this study.
LH: Writing – original draft. JC: Writing – review & editing. XL: Writing – review & editing. HZ: Writing – review & editing. JZ: Writing – review & editing. YL: Writing – review & editing. JL: Writing – review & editing. HY: Writing – review & editing. NY: Writing – review & editing. JW: Writing – review & editing. HL: Writing – review & editing. JX: Writing – original draft.
The author(s) declare financial support was received for the research, authorship, and/or publication of this article. This study was supported by the Shenzhen Science and Technology Research Program (nos. JCYJ20170412174037594 and JCYJ20200109114816594), Construction of High-level Hospital in Shenzhen Hospital of Traditional Chinese Medicine Program (no. G3030202116), Ningxia Key Research Program (no. 2022BEG03158), Zhongshan Key Technology Research Program (no. 2019B1005), Sanming Project of Medicine in Shenzhen (SZZYSM202311002), Construction Project of Guangdong Famous Traditional Chinese Medicine Inheritance Studio (Document No. 108 [2023] issued by the Guangdong Traditional Chinese Medicine Administration).
We appreciate the statistical guidance provided by Chao Wang from Shenzhen University.
The authors declare that the research was conducted in the absence of any commercial or financial relationships that could be construed as a potential conflict of interest.
All claims expressed in this article are solely those of the authors and do not necessarily represent those of their affiliated organizations, or those of the publisher, the editors and the reviewers. Any product that may be evaluated in this article, or claim that may be made by its manufacturer, is not guaranteed or endorsed by the publisher.
Bagurdes, L. A., Mesulam, M., Gitelman, D., Weintraub, S., and Small, D. (2008). Modulation of the spatial attention network by incentives in healthy aging and mild cognitive impairment. Neuropsychologia 46, 2943–2948. doi: 10.1016/j.neuropsychologia.2008.06.005
Baillieux, H., Smet, H., Paquier, P., de, P., and Mariën, P. (2008). Cerebellar neurocognition: insights into the bottom of the brain. Clin. Neurol. Neurosurg. 110, 763–773. doi: 10.1016/j.clineuro.2008.05.013
Capogna, E., Manca, R., de, M., Hall, A., Soininen, H., and Venneri, A. (2019). Understanding the effect of cognitive/brain reserve and depression on regional atrophy in early Alzheimer’s disease. Postgrad. Med. 131, 533–538. doi: 10.1080/00325481.2019.1663127
Chang, C.-C., and Lin, C.-J. (2011). LIBSVM. ACM Trans. Intell. Syst. Technol. 2, 1–27. doi: 10.1145/1961189.1961199
Choo, I. H., Lee, D., Oh, J., Lee, J., Lee, D., Song, I., et al. (2010). Posterior cingulate cortex atrophy and regional cingulum disruption in mild cognitive impairment and Alzheimer’s disease. Neurobiol. Aging 31, 772–779. doi: 10.1016/j.neurobiolaging.2008.06.015
Cinar, M. A., Sertoglu, E., and Oztin, H. (2014). Impact of oxidative stress on cognition and depressive symptoms in mild cognitive impairment in the elderly. Int. J. Neuropsychopharmacol. 17:71.
Cooper, C., Sommerlad, A., Lyketsos, C., and Livingston, G. (2015). Modifiable predictors of dementia in mild cognitive impairment: a systematic review and meta-analysis. Am. J. Psychiatry 172, 323–334. doi: 10.1176/appi.ajp.2014.14070878
Cotta, M., Perini, G., Vaghi, G., Dal, B., Capelli, M., Picascia, M., et al. (2021). Correlation of frontal atrophy and CSF tau levels with neuropsychiatric symptoms in patients with cognitive impairment: a memory clinic experience. Front. Aging Neurosci. 13:595758. doi: 10.3389/fnagi.2021.595758
Cotta Ramusino, M., Imbimbo, C., Capelli, M., Cabini, R. F., Bernini, S., and Lombardo, F. P. (2024). Role of fronto-limbic circuit in neuropsychiatric symptoms of dementia: clinical evidence from an exploratory study. Front. Psych. 15:1231361. doi: 10.3389/fpsyt.2024.1231361
Davey, C. G., Pujol, J., and Harrison, B. J. (2016). Mapping the self in the brain’s default mode network. NeuroImage 132, 390–397. doi: 10.1016/j.neuroimage.2016.02.022
de Vos, F., Koini, M., Schouten, T. M., Seiler, S., van der Grond, J., Lechner, A., et al. (2018). A comprehensive analysis of resting state fMRI measures to classify individual patients with Alzheimer’s disease. NeuroImage 167, 62–72. doi: 10.1016/j.neuroimage.2017.11.025
Diciotti, S., Ciulli, S., Ginestroni, A., Salvadori, E., Poggesi, A., and Pantoni, L. (2015). Multimodal MRI classification in vascular mild cognitive impairment. Conf. Proc. IEEE Eng. Med. Biol. Soc. 2015, 4278–4281. doi: 10.1109/EMBC.2015.7319340
Diciotti, S., Orsolini, S., Salvadori, E., Giorgio, A., Toschi, N., Ciulli, S., et al. (2017). Resting state fMRI regional homogeneity correlates with cognition measures in subcortical vascular cognitive impairment. J. Neurol. Sci. 373, 1–6. doi: 10.1016/j.jns.2016.12.003
Ding, W., Cao, W., Wang, Y., Sun, Y., Chen, X., Zhou, Y., et al. (2015). Altered functional connectivity in patients with subcortical vascular cognitive impairment--a resting-state functional magnetic resonance imaging study. PLoS One 10:e0138180. doi: 10.1371/journal.pone.0138180
Donaghy, P. C., Taylor, J. P., O’Brien, J., Barnett, N., Olsen, K., Colloby, S., et al. (2018). Neuropsychiatric symptoms and cognitive profile in mild cognitive impairment with Lewy bodies. Psychol. Med. 48, 2384–2390. doi: 10.1017/S0033291717003956
Fazekas, F., Chawluk, J. B., Alavi, A., Hurtig, H. I., and Zimmerman, R. A. (1987). MR signal abnormalities at 1.5 T in Alzheimer’s dementia and normal aging. AJR Am. J. Roentgenol. 149, 351–356. doi: 10.2214/ajr.149.2.351
Frings, L., Dressel, K., Abel, S., Saur, D., Kümmerer, D., Mader, I., et al. (2010). Reduced precuneus deactivation during object naming in patients with mild cognitive impairment, Alzheimer’s disease, and frontotemporal lobar degeneration. Dement. Geriatr. Cogn. Disord. 30, 334–343. doi: 10.1159/000320991
Gallagher, D., Fischer, C. E., and Iaboni, A. (2017). Neuropsychiatric symptoms in mild cognitive impairment. Can. J. Psychiatr. 62, 161–169. doi: 10.1177/0706743716648296
Gao, Y., Huang, C., Zhao, K., Ma, L., Qiu, X., Zhang, L., et al. (2013). Depression as a risk factor for dementia and mild cognitive impairment: a meta-analysis of longitudinal studies. Int. J. Geriatr. Psychiatry 28, 441–449. doi: 10.1002/gps.3845
Grieve, S. M., Korgaonkar, M., Koslow, S., Gordon, E., and Williams, L. (2013). Widespread reductions in gray matter volume in depression. Neuroimage Clin. 3, 332–339. doi: 10.1016/j.nicl.2013.08.016
Guo, S., Lai, C., Wu, C., and Cen, G. (2017). Conversion discriminative analysis on mild cognitive impairment using multiple cortical features from MR images. Front. Aging Neurosci. 9:146. doi: 10.3389/fnagi.2017.00146
Guo, W., Liu, F., Liu, J., Yu, M., Zhang, Z., and Liu, G. (2015). Increased cerebellar-default-mode-network connectivity in drug-naive major depressive disorder at rest. Medicine 94:e560. doi: 10.1097/MD.0000000000000560
Gusnard, D. A., Raichle, M. E., and Raichle, M. E. (2001). Searching for a baseline: functional imaging and the resting human brain. Nat. Rev. Neurosci. 2, 685–694. doi: 10.1038/35094500
Han, Y., Lui, S., Kuang, W., Lang, Q., Zou, L., and Jia, J. (2012). Anatomical and functional deficits in patients with amnestic mild cognitive impairment. PLoS One 7:e28664. doi: 10.1371/journal.pone.0028664
Han, Y., Wang, J., Zhao, Z., Min, B., Lu, J., Li, K., et al. (2011). Frequency-dependent changes in the amplitude of low-frequency fluctuations in amnestic mild cognitive impairment: a resting-state fMRI study. NeuroImage 55, 287–295. doi: 10.1016/j.neuroimage.2010.11.059
Harada, K., Matsuo, K., Nakashima, M., Hobara, T., Higuchi, N., Higuchi, F., et al. (2016). Disrupted orbitomedial prefrontal limbic network in individuals with later-life depression. J. Affect. Disord. 204, 112–119. doi: 10.1016/j.jad.2016.06.031
Huang, C., Wahlund, L. O., Svensson, L., Winblad, B., and Julin, P. (2002). Cingulate cortex hypoperfusion predicts Alzheimer’s disease in mild cognitive impairment. BMC Neurol. 2:9. doi: 10.1186/1471-2377-2-9
Hughes, C. P., Berg, L., Danziger, W., Coben, L., and Martin, R. (1982). A new clinical scale for the staging of dementia. Br. J. Psychiatry 140, 566–572. doi: 10.1192/bjp.140.6.566
Ismail, Z., Elbayoumi, H., Fischer, C., Hogan, D., Millikin, C., Schweizer, T., et al. (2017). Prevalence of depression in patients with mild cognitive impairment. JAMA Psychiatry 74, 58–67. doi: 10.1001/jamapsychiatry.2016.3162
Jak, A. J., Bondi, M. W., Delano-Wood, L., Wierenga, C., Corey-Bloom, J., Salmon, D. P., et al. (2009). Quantification of five neuropsychological approaches to defining mild cognitive impairment. Am. J. Geriatr. Psychiatr. 17, 368–375. doi: 10.1097/JGP.0b013e31819431d5
Johnson, S. C., Schmitz, T. W., Moritz, C. H., Meyerand, M. E., Rowley, H. A., Alexander, A. L., et al. (2006). Activation of brain regions vulnerable to Alzheimer’s disease: the effect of mild cognitive impairment. Neurobiol. Aging 27, 1604–1612. doi: 10.1016/j.neurobiolaging.2005.09.017
Kandilarova, S., Stoyanov, D., Sirakov, N., Maes, M., and Specht, K. (2019). Reduced grey matter volume in frontal and temporal areas in depression: contributions from voxel-based morphometry study. Acta Neuropsychiatr. 31, 252–257. doi: 10.1017/neu.2019.20
Kim, S. H., Kang, H., Kim, H., Ryu, H., Kim, M., Seo, S., et al. (2013). Neuropsychiatric predictors of conversion to dementia both in patients with amnestic mild cognitive impairment and those with subcortical vascular MCI. Clin. Neurol. Neurosurg. 115, 1264–1270. doi: 10.1016/j.clineuro.2012.11.029
Krajcovicova, L., Barton, M., Elfmarkova-Nemcova, N., Mikl, M., Marecek, R., and Rektorova, I. (2017). Changes in connectivity of the posterior default network node during visual processing in mild cognitive impairment: staged decline between normal aging and Alzheimer’s disease. J. Neural Transm. 124, 1607–1619. doi: 10.1007/s00702-017-1789-5
Lavretsky, H., Roybal, D., Ballmaier, M., Toga, A., and Kumar, A. (2005). Antidepressant exposure may protect against decrement in frontal gray matter volumes in geriatric depression. J. Clin. Psychiatry 66, 964–967. doi: 10.4088/JCP.v66n0801
Lee, J., Seo, S., Yang, J. J., Jang, Y., Lee, J., Kim, Y., et al. (2018). Longitudinal cortical thinning and cognitive decline in patients with early‐ versus late‐stage subcortical vascular mild cognitive impairment. Eur. J. Neurol. 25, 326–333. doi: 10.1111/ene.13500
Li, Y., Jing, B., Liu, H., Li, Y., Gao, X., Li, Y., et al. (2017). Frequency-dependent changes in the amplitude of low-frequency fluctuations in mild cognitive impairment with mild depression. J. Alzheimers Dis. 58, 1175–1187. doi: 10.3233/JAD-161282
Lin, C. Y., Chen, C. H., Tom, S., and Kuo, S. H. (2020). Cerebellar volume is associated with cognitive decline in mild cognitive impairment: Results from ADNI. Cerebellum 19, 217–225. doi: 10.1007/s12311-019-01099-1
Liu, X., Chen, J., Shen, B., Wang, G., Li, J., Hou, H., et al. (2018). Altered intrinsic coupling between functional connectivity density and amplitude of low-frequency fluctuation in mild cognitive impairment with depressive symptoms. Neural Plast. 2018, 1–8. doi: 10.1155/2018/1672708
Liu, F., Guo, W., Liu, L., Long, Z., Ma, C., Xue, Z., et al. (2013). Abnormal amplitude low-frequency oscillations in medication-naive, first-episode patients with major depressive disorder: a resting-state fMRI study. J. Affect. Disord. 146, 401–406. doi: 10.1016/j.jad.2012.10.001
Lo, T. W. B., Karameh, W., Barfett, J., Fornazzari, L., Munoz, D., Schweizer, T., et al. (2020). Association between neuropsychiatric symptom trajectory and conversion to Alzheimer disease. Alzheimer Dis. Assoc. Disord. 34, 141–147. doi: 10.1097/WAD.0000000000000356
Long, Z., Jing, B., Yan, H., Dong, J., Liu, H., and Mo, X. (2016). A support vector machine-based method to identify mild cognitive impairment with multi-level characteristics of magnetic resonance imaging. Neuroscience 331, 169–176. doi: 10.1016/j.neuroscience.2016.06.025
Lyketsos, C. G., Lopez, O., Jones, B., Fitzpatrick, A., Breitner, J., and DeKosky, S. (2002). Prevalence of neuropsychiatric symptoms in dementia and mild cognitive impairment. JAMA 288, 1475–1483. doi: 10.1001/jama.288.12.1475
Lyu, H., Wang, J., Xu, J., Zheng, H., Yang, X., Lin, S., et al. (2019). Structural and functional disruptions in subcortical vascular mild cognitive impairment with and without depressive symptoms. Front. Aging Neurosci. 11:241. doi: 10.3389/fnagi.2019.00241
Mao, S., Zhang, C., Gao, N., Wang, Y., Yang, Y., Guo, X., et al. (2017). A study of feature extraction for Alzheimer’s disease based on resting-state fMRI. Conf. Proc. IEEE Eng. Med. Biol. Soc. 2017, 517–520. doi: 10.1109/EMBC.2017.8036875
Mitolo, M., Stanzani-Maserati, M., Capellari, S., Testa, C., Rucci, P., Poda, R., et al. (2019). Predicting conversion from mild cognitive impairment to Alzheimer’s disease using brain 1H-MRS and volumetric changes: a two- year retrospective follow-up study. Neuroimage Clin. 23:101843. doi: 10.1016/j.nicl.2019.101843
Mourao, R. J., Mansur, G., Malloy-Diniz, L., Castro, E., and Diniz, B. (2016). Depressive symptoms increase the risk of progression to dementia in subjects with mild cognitive impairment: systematic review and meta-analysis. Int. J. Geriatr. Psychiatry 31, 905–911. doi: 10.1002/gps.4406
Natu, V. S., Lin, J. J., Burks, A., Arora, A., Rugg, M., and Lega, B. (2019). Stimulation of the posterior cingulate cortex impairs episodic memory encoding. J. Neurosci. 39, 7173–7182. doi: 10.1523/JNEUROSCI.0698-19.2019
Papma, J. M., Smits, M., de, M., Mattace, F., van, A., Vrooman, H., et al. (2017). The effect of hippocampal function, volume and connectivity on posterior cingulate cortex functioning during episodic memory fMRI in mild cognitive impairment. Eur. Radiol. 27, 3716–3724. doi: 10.1007/s00330-017-4768-1
Petrella, J. R., Wang, L., Krishnan, S., Slavin, M., Prince, S., Tran, T. T., et al. (2007). Cortical deactivation in mild cognitive impairment: high-field-strength functional MR imaging. Radiology 245, 224–235. doi: 10.1148/radiol.2451061847
Pivtoraiko, V. N., Abrahamson, E., Leurgans, S., DeKosky, S., Mufson, E., and Ikonomovic, M. (2015). Cortical pyroglutamate amyloid-β levels and cognitive decline in Alzheimer’s disease. Neurobiol. Aging 36, 12–19. doi: 10.1016/j.neurobiolaging.2014.06.021
Power, J. D., Barnes, K., Snyder, A., Schlaggar, B., and Petersen, S. (2012). Spurious but systematic correlations in functional connectivity MRI networks arise from subject motion. NeuroImage 59, 2142–2154. doi: 10.1016/j.neuroimage.2011.10.018
Qin, J., Wei, M., Liu, H., Yan, R., Luo, G., Yao, Z., et al. (2014). Abnormal brain anatomical topological organization of the cognitive-emotional and the frontoparietal circuitry in major depressive disorder. Magn. Reson. Med. 72, 1397–1407. doi: 10.1002/mrm.25036
Ramusino, M. C., Vitali, P., Anzalone, N., Melazzini, L., Lombardo, F., Farina, L., et al. (2022). Vascular lesions and brain atrophy in Alzheimer’s, vascular and mixed dementia: an optimized 3T MRI protocol reveals distinctive radiological profiles. Curr. Alzheimer Res. 19, 449–457. doi: 10.2174/1567205019666220620112831
Ravaglia, G., Forti, P., Maioli, F., Martelli, M., Servadei, L., Brunetti, N., et al. (2005). Conversion of mild cognitive impairment to dementia: predictive role of mild cognitive impairment subtypes and vascular risk factors. Dement. Geriatr. Cogn. Disord. 21, 51–58. doi: 10.1159/000089515
Rolls, E. T. (2016). A non-reward attractor theory of depression. Neurosci. Biobehav. Rev. 68, 47–58. doi: 10.1016/j.neubiorev.2016.05.007
Román, G., Erkinjuntti, T., Wallin, A., Pantoni, L., and Chui, H. (2002). Subcortical ischaemic vascular dementia. Lancet Neurol. 1, 426–436. doi: 10.1016/S1474-4422(02)00190-4
Salloway, S., Ferris, S., Kluger, A., Goldman, R., Griesing, T., Kumar, D., et al. (2004). Efficacy of donepezil in mild cognitive impairment. Neurology 63, 651–657. doi: 10.1212/01.WNL.0000134664.80320.92
Salvadore, G., Nugent, A., Lemaitre, H., Luckenbaugh, D., Tinsley, R., Cannon, D., et al. (2011). Prefrontal cortical abnormalities in currently depressed versus currently remitted patients with major depressive disorder. NeuroImage 54, 2643–2651. doi: 10.1016/j.neuroimage.2010.11.011
Satpute, A. B., and Lindquist, K. A. (2019). The default mode Network’s role in discrete emotion. Trends Cogn. Sci. 23, 851–864. doi: 10.1016/j.tics.2019.07.003
Satterthwaite, T. D., Elliott, M., Gerraty, R., Ruparel, K., Loughead, J., Calkins, M., et al. (2013). An improved framework for confound regression and filtering for control of motion artifact in the preprocessing of resting-state functional connectivity data. NeuroImage 64, 240–256. doi: 10.1016/j.neuroimage.2012.08.052
Seo, S. W., Cho, S., Park, A., Chin, J., and Na, D. (2009). Subcortical vascular versus amnestic mild cognitive impairment: comparison of cerebral glucose metabolism. J. Neuroimaging 19, 213–219. doi: 10.1111/j.1552-6569.2008.00292.x
Steffens, D. C. (2012). Depressive symptoms and mild cognitive impairment in the elderly: an ominous combination. Biol. Psychiatry 71, 762–764. doi: 10.1016/j.biopsych.2012.02.002
Stoodley, C. J., and Schmahmann, J. D. (2009). Functional topography in the human cerebellum: a meta-analysis of neuroimaging studies. NeuroImage 44, 489–501. doi: 10.1016/j.neuroimage.2008.08.039
Sun, Y. W., Qin, L. D., Zhou, Y., Xu, Q., Qian, L. J., Tao, J., et al. (2011). Abnormal functional connectivity in patients with vascular cognitive impairment, no dementia: a resting-state functional magnetic resonance imaging study. Behav. Brain Res. 223, 388–394. doi: 10.1016/j.bbr.2011.05.006
Teipel, S. J., Wohlert, A., Metzger, C., Grimmer, T., Sorg, C., Ewers, M., et al. (2017). Multicenter stability of resting state fMRI in the detection of Alzheimer’s disease and amnestic MCI. Neuroimage Clin. 14, 183–194. doi: 10.1016/j.nicl.2017.01.018
Wang, P., Li, R., Liu, B., Wang, C., Huang, Z., Dai, R., et al. (2019). Altered static and temporal dynamic amplitude of low-frequency fluctuations in the background network during working memory states in mild cognitive impairment. Front. Aging Neurosci. 11:152. doi: 10.3389/fnagi.2019.00152
Wang, P., Li, R., Yu, J., Huang, Z., and Li, J. (2016). Frequency-dependent brain regional homogeneity alterations in patients with mild cognitive impairment during working memory state relative to resting state. Front. Aging Neurosci. 8:60. doi: 10.3389/fnagi.2016.00060
Wang, Z., Liang, P., Jia, X., Jin, G., Song, H., Han, Y., et al. (2012). The baseline and longitudinal changes of PCC connectivity in mild cognitive impairment: a combined structure and resting-state fMRI study. PLoS One 7:e36838. doi: 10.1371/journal.pone.0036838
Wardlaw, J. M., Smith, E. E., Biessels, G. J., Cordonnier, C., Fazekas, F., Frayne, R., et al. (2013). Neuroimaging standards for research into small vessel disease and its contribution to ageing and neurodegeneration. Lancet Neurol. 12, 822–838. doi: 10.1016/S1474-4422(13)70124-8
Xie, C., Li, W., Chen, G., Douglas, B., Franczak, M., Jones, J., et al. (2012). The co-existence of geriatric depression and amnestic mild cognitive impairment detrimentally affect gray matter volumes: voxel-based morphometry study. Behav. Brain Res. 235, 244–250. doi: 10.1016/j.bbr.2012.08.007
Xu, Z., Wang, J., Lyu, H., Wang, R., Hu, Y., Guo, Z., et al. (2020). Alterations of white matter microstructure in subcortical vascular mild cognitive impairment with and without depressive symptoms. J. Alzheimers Dis. 73, 1565–1573. doi: 10.3233/JAD-190890
Xu, L. Y., Xu, F. C., Liu, C., Ji, Y. F., Wu, J. M., Wang, Y., et al. (2017). Relationship between cerebellar structure and emotional memory in depression. Brain Behav. 7:e00738. doi: 10.1002/brb3.738
Yan, C. G., Cheung, B., Kelly, C., Colcombe, S., Craddock, R., di, A., et al. (2013). A comprehensive assessment of regional variation in the impact of head micromovements on functional connectomics. NeuroImage 76, 183–201. doi: 10.1016/j.neuroimage.2013.03.004
Yang, H., Long, X. Y., Yang, Y., Yan, H., Zhu, C. Z., Zhou, X. P., et al. (2007). Amplitude of low frequency fluctuation within visual areas revealed by resting-state functional MRI. NeuroImage 36, 144–152. doi: 10.1016/j.neuroimage.2007.01.054
Yang, L., Yan, Y., Wang, Y., Hu, X., Lu, J., Chan, P., et al. (2018). Gradual disturbances of the amplitude of low-frequency fluctuations (ALFF) and fractional ALFF in Alzheimer Spectrum. Front. Neurosci. 12:975. doi: 10.3389/fnins.2018.00975
Yi, L., Wang, J., Jia, L., Zhao, Z., Lu, J., Li, K., et al. (2012). Structural and functional changes in subcortical vascular mild cognitive impairment: a combined voxel-based morphometry and resting-state fMRI study. PLoS One 7:e44758. doi: 10.1371/journal.pone.0044758
Yoon, C. W., Seo, S., Park, J. S., Kwak, K. C., Yoon, U., Suh, M., et al. (2013). Cerebellar atrophy in patients with subcortical-type vascular cognitive impairment. Cerebellum 12, 35–42. doi: 10.1007/s12311-012-0388-0
Yu, Y., Li, Z., Lin, Y., Yu, J., Peng, G., Zhang, K., et al. (2019). Depression affects intrinsic brain activity in patients with mild cognitive impairment. Front. Neurosci. 13:1333. doi: 10.3389/fnins.2019.01333
Zhao, Z. L., Fan, F. M., Lu, J., Li, H. J., Jia, L. F., Han, Y., et al. (2015). Changes of gray matter volume and amplitude of low-frequency oscillations in amnestic MCI: an integrative multi-modal MRI study. Acta Radiol. 56, 614–621. doi: 10.1177/0284185114533329
Zhou, Y., Dougherty, J. Jr., Hubner, K., Bai, B., Cannon, R., and Hutson, R. (2008). Abnormal connectivity in the posterior cingulate and hippocampus in early Alzheimer’s disease and mild cognitive impairment. Alzheimers Dement. 4, 265–270. doi: 10.1016/j.jalz.2008.04.006
Zimmerman, M., Martinez, J., Young, D., Chelminski, I., and Dalrymple, K. (2013). Severity classification on the Hamilton depression rating scale. J. Affect. Disord. 150, 384–388. doi: 10.1016/j.jad.2013.04.028
Zou, Q. H., Zhu, C. Z., Yang, Y., Zuo, X. N., Long, X. Y., Cao, Q. J., et al. (2008). An improved approach to detection of amplitude of low-frequency fluctuation (ALFF) for resting-state fMRI: fractional ALFF. J. Neurosci. Methods 172, 137–141. doi: 10.1016/j.jneumeth.2008.04.012
Keywords: subcortical vascular mild cognitive impairment, depression symptoms, fractions of amplitude of low-frequency fluctuations, resting-state functional connectivity, support vector machine
Citation: Hu L, Chen J, Li X, Zhang H, Zhang J, Lu Y, Lian J, Yu H, Yang N, Wang J, Lyu H and Xu J (2024) Disruptive and complementary effects of depression symptoms on spontaneous brain activity in the subcortical vascular mild cognitive impairment. Front. Aging Neurosci. 16:1338179. doi: 10.3389/fnagi.2024.1338179
Received: 14 November 2023; Accepted: 26 August 2024;
Published: 17 September 2024.
Edited by:
Yuanchao Zhang, University of Electronic Science and Technology of China, ChinaReviewed by:
Yuanchao Zhang, University of Electronic Science and Technology of China, ChinaCopyright © 2024 Hu, Chen, Li, Zhang, Zhang, Lu, Lian, Yu, Yang, Wang, Lyu and Xu. This is an open-access article distributed under the terms of the Creative Commons Attribution License (CC BY). The use, distribution or reproduction in other forums is permitted, provided the original author(s) and the copyright owner(s) are credited and that the original publication in this journal is cited, in accordance with accepted academic practice. No use, distribution or reproduction is permitted which does not comply with these terms.
*Correspondence: Jinping Xu, anAueHVAc2lhdC5hYy5jbg==; Hanqing Lyu, bHZoYW5xaW5nMjAxOUAxNjMuY29t; Jianjun Wang, dGluY3Ryb3dAMTYzLmNvbQ==
†These authors have contributed equally to this work
Disclaimer: All claims expressed in this article are solely those of the authors and do not necessarily represent those of their affiliated organizations, or those of the publisher, the editors and the reviewers. Any product that may be evaluated in this article or claim that may be made by its manufacturer is not guaranteed or endorsed by the publisher.
Research integrity at Frontiers
Learn more about the work of our research integrity team to safeguard the quality of each article we publish.