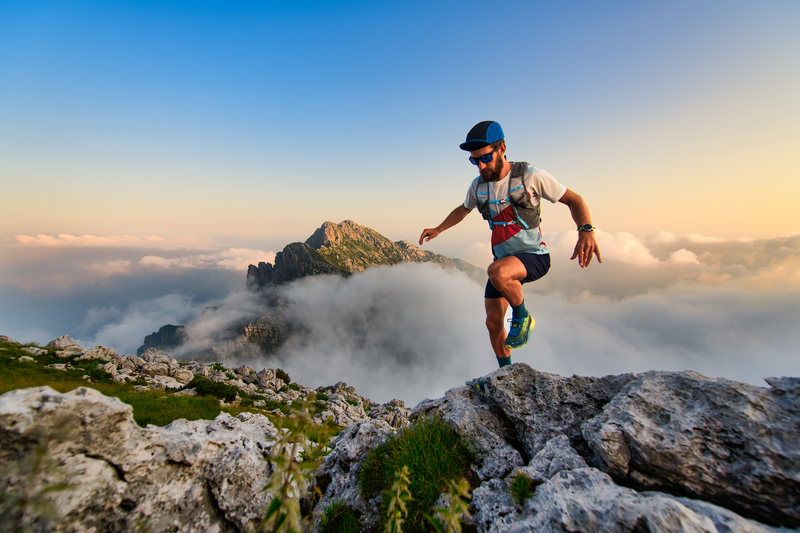
94% of researchers rate our articles as excellent or good
Learn more about the work of our research integrity team to safeguard the quality of each article we publish.
Find out more
ORIGINAL RESEARCH article
Front. Aging Neurosci. , 24 January 2024
Sec. Neurocognitive Aging and Behavior
Volume 16 - 2024 | https://doi.org/10.3389/fnagi.2024.1307204
This article is part of the Research Topic Neuroscience and Neurological Machine Learning for Cognitive Assessment: Advancements, Challenges, and Future Directions View all 8 articles
We investigated a screening method for mild cognitive impairment (MCI) that combined bioimpedance features and the Korean Mini-Mental State Examination (K-MMSE) score. Data were collected from 539 subjects aged 60 years or older at the Gwangju Alzheimer’s & Related Dementias (GARD) Cohort Research Center, A total of 470 participants were used for the analysis, including 318 normal controls and 152 MCI participants. We measured bioimpedance, K-MMSE, and the Seoul Neuropsychological Screening Battery (SNSB-II). We developed a multiple linear regression model to predict MCI by combining bioimpedance variables and K-MMSE total score and compared the model’s accuracy with SNSB-II domain scores by the area under the receiver operating characteristic curve (AUROC). We additionally compared the model performance with several machine learning models such as extreme gradient boosting, random forest, support vector machine, and elastic net. To test the model performances, the dataset was divided into a training set (70%) and a test set (30%). The AUROC values of SNSB-II scores were 0.803 in both sexes, 0.840 for males, and 0.770 for females. In the combined model, the AUROC values were 0.790 (0.773) for males (and females), which were significantly higher than those from the model including MMSE scores alone (0.723 for males and 0.622 for females) or bioimpedance variables alone (0.640 for males and 0.615 for females). Furthermore, the accuracies of the combined model were comparable to those of machine learning models. The bioimpedance-MMSE combined model effectively distinguished the MCI participants and suggests a technique for rapid and improved screening of the elderly population at risk of cognitive impairment.
Population aging is a global phenomenon because of improvement in hygiene, nutritional status, and medical technology. Consequently, the number of elderly individuals at risk of dementia or mild cognitive impairment (MCI) is increasing at an alarming rate. Dementia imposes a substantial social burden due to its challenging treatment and the suffering experienced by patients and their care givers. Early detection of cognitive impairment may help to prevent or slow the progression of dementia.
To identify cognitive dysfunction, clinics often employ neuropsychological assessment questionnaires, such as the Consortium to Establish a Registry for Alzheimer’s Disease (CERAD), Dementia Rating Scale (DRS), Alzheimer’s Disease Assessment Scale-Cognitive Subscale (ADAS-Cog), and Seoul Neuropsychological Screening Battery II (SNSB II). These assessments are widely utilized for the comprehensive cognition test due to their accessibility, cost-effectiveness, convenience, and the use of neuroimaging techniques such as magnetic resonance imaging (MRI) or positron emission tomography (PET) to determine the underlying causes of cognitive impairment (Benedict and Zivadinov, 2011; Risacher et al., 2017; Petracca et al., 2021).
However, these comprehensive neuropsychological assessments may not be readily available to many participants due to their time-consuming nature and the need for a professionally trained practitioner. As a result, brief neuropsychological questionnaires such as the Mini-Mental State Examination (MMSE) and the Montreal Cognitive Assessment (MoCA) have gained widespread popularity as screening tools for dementia (Folstein et al., 1975; Nasreddine et al., 2005; Smith et al., 2007; Roalf et al., 2013). These tools are easily administered with minimal training, and can be completed within 10 min and have demonstrated diagnostic utility. The MMSE, in particular, is the most widely adopted measure for screening cognitive function in medical and neuropsychological research. However, the MMSE is known to lack its accuracy to earlier cognitive impairment from cognitively normal elderly (Hoops et al., 2009; Lee et al., 2009; Arevalo-Rodriguez et al., 2015; Ciesielska et al., 2016).
Bioelectrical impedance analysis (BIA), also known as bioimpedance analysis, is widely acknowledged to be a safe, rapid, reliable, easy-to-use, portable, noninvasive, and cost-effective technique. BIA has extensive applications in measuring body composition (Abu Khaled et al., 1988; Jackson et al., 1988; Shafer et al., 2009). Recently, numerous studies have reported the potential of using BIA measurements to assess health indicators and clinical outcomes among various patient populations. Specifically, BIA has been extensively used as a valuable index for monitoring and screening different diseases and conditions, including mortality, nutrition status, diabetes, hemodialysis, chronic heart failure, and liver cirrhosis (Selberg and Selberg, 2002; Colin-Ramirez et al., 2012; Beberashvili et al., 2014; Dittmar et al., 2015; Genton et al., 2017; Kuchnia et al., 2017; Jun et al., 2018). Some studies have explored the association between cognitive function, such as MCI or dementia related to Alzheimer’s disease (AD), and BIA variables. Notably, BIA variables were significantly different in individuals with AD compared to controls. Participants with AD exhibited lower lean tissue mass and higher percent fat mass than healthy elderly individuals. Reactance and the ratio of reactance to height were approximately 21% lower in individuals with dementia than in older adults without dementia (Buffa et al., 2010, 2014; Camina Martin et al., 2015). Additionally, AD patients demonstrated significantly higher height-normalized impedance values and lower phase angles (indicative of body cell mass) than healthy controls. Men with MCI exhibited a higher ratio of impedance to height than healthy controls (Cova et al., 2017). Furthermore, a study reported positive correlations between muscle mass percentage and cognitive function measures such as attention and executive function (Crespillo-Jurado et al., 2019). Recent research has also revealed associations between MCI and increased segmental water and lean mass in the lower extremities, as well as decreased resistance and reactance in the lower extremities (Doan et al., 2022b).
Numerous studies have highlighted the relationship between cognitive function and body composition, which can be influenced by factors such as nutrition status and physical activity. However, a screening method for cognitive in a manner that is easy, cost-effective, rapid, and relatively accurate is challenging. In this study, we propose a new approach to screen for MCI by combining MMSE with BIA measurements.
To evaluate the classification accuracy of prediction models that distinguish individuals with MCI from cognitively normal controls (NCs), we plotted a receiver operating characteristic (ROC) curve for the combined model (including MMSE scores and BIA measurements) and calculated the corresponding area under the ROC (AUROC). Specifically, we compared the AUROC value of the model combining the MMSE scores and BIA measures with that of a model with SNSB II scores. Furthermore, we explored the possibility of diagnosing MCI using various machine learning algorithms, by comparing their performance via AUROC values.
The results of this study have the potential to facilitate easy, rapid, and relatively accurate screening of individuals suspected to have cognitive impairment within a large population. It can help identify individuals who need for further precision neuropsychological tests and/or neuroimaging in clinical settings, thereby saving energy and medical cost.
From December 2017 to February 2020, participants were recruited from the GARD Cohort Research Center for the early diagnosis and progression of Alzheimer’s disease for local citizens aged 60 years or older from Gwangju city, South Korea. A total of 539 participants were involved in this study, and data from 470 participants were used for the analysis, excluding 69 individuals with no data, failure to meet the criteria, or deferred diagnosis. There were 152 individuals with clinical consensus regarding a diagnosis of MCI and 318 elderly normal individuals, for a total of 470 participants. The sex ratio of the analyzed sample consisted of 219 males and 251 females. The study was approved by the Institutional Review Board (IRB) of Chonnam National University Hospital and Chosun University Hospital (IRB number: CNUH-2019-279, CHOSUN 2016–10–005-017). All participants were informed of the objectives and methods of the research, and they provided written informed consent. The study was performed according to the Declaration of Helsinki guidelines.
The Korean version of MMSE (K-MMSE) and the SNSB-II were used for neuropsychological evaluations. The K-MMSE incorporates a range of cognitive domains that include orientation in time (5 points), orientation in place (5 points), memory registration (3 points), attention and calculation (5 points), memory recall (3 points), language (8 points), and spatiotemporal configuration (1 point) (Kang et al., 2016). This creates a total score of 30 points. The SNSB-II assesses attention, language, memory, visuospatial skills, and frontal/executive function; The memory domain includes the Seoul Verbal Learning Test (SVLT) and the Rey Complex Figure Test (RCFT) (Jahng et al., 2015). The frontal/executive function domain includes motor impersistence, contrasting program, the go-no-go task, the Luria test, alternating hand movements, the alternating square and triangle task, the Luria loop task, Controlled Oral Word Association Test (COWAT), Korean-Color Word Stroop Test (K-CWST), Digit Symbol Coding (DSC), and Korean-Trail Making Test. The language domain includes the Korean-Boston Naming Test (K-BNT), right–left orientation, and calculation. The attention domain includes the Digit Span Test (DST), letter cancellation, and vigilance test. The visuospatial domain is composed of the clock drawing test (CDT) and RCFT. It also includes other related tests, such as the Clinical Dementia Rating Scale (CDR), Barthel-Activities of Daily Living (B-ADL), Korean-Instrumental Activities of Daily Living (K-IADL), and Geriatric Depression Scale (GDS). The estimated completion time of the whole battery is one and a half hours to two hours.
The diagnostic criteria for MCI proposed by the International Working Group on MCI were the absence of dementia according to the criteria of the Diagnostic and Statistical Manual of Mental Disorders, fifth edition (DSM-V) (Edition F, 2013). Detailly, we evaluated all participants using comprehensive clinical consultations involving a combination of a neuropsychological battery and the Clinical Dementia Rating (CDR) scale. Individuals categorized as cognitively normal (CN) were clinically identified with a CDR score of zero and displayed no cognitive impairment. Meanwhile, those with a CDR score of 0.5 and evidence of cognitive decline in one or more domains, were classified as having MCI. MCI patients exhibited a Seoul Neuropsychological Screening Battery-Second Edition (SNSB-II) z score of less than −1.5 in at least one cognitive domain.
Bioelectrical impedance was measured using a direct segmental multifrequency bioelectrical impedance analyzer with tetrapolar 8-point electrodes (InBody S10, InBody, Korea). We measured the impedance at six frequencies (1, 5, 50, 250, 500, and 1,000 kHz) and reactance and phase angle (PhA) values at three frequencies (5, 50, and 250 kHz). Eight electrodes were used to measure segmental impedance in five body segments (the two arms, two legs, and trunk). After the readings sufficiently stabilized, BIA measurements were collected from the limbs of the body with the subjects in a supine position, which is one of the standard measurement positions.
All statistical analyses were performed with R (version 4.0.3, released on 2020-10-10) (Team RC, 2019). Individuals with invalid data (69 participants) or AD dementia (three participants) were excluded. An independent-sample t-test was carried out to assess the differences in means of variables between participants with MCI and healthy controls. The correlations between BIA variables and domain scores and total scores on the neuropsychological questionnaires were analyzed. A prediction model for MCI was constructed by combining BIA variables and the K-MMSE total score, and AUROC values of the combined model were compared to those of the model with the SNSB-II scores. Although the data were not extensive, the classification accuracy of several machine learning algorithms (constructed in R) was calculated in terms of AUROC values, and the differences between the prediction models with various variables and the variables included by the machine learning algorithms were compared.
To verify the models developed in this study, we conducted machine learning experiments with several well-known machine learning algorithms on different feature sets. The candidate feature sets consisted of the following:
1. Demographic characteristics (DM), including age, sex, education level, height, weight, and BMI
2. BIA variables (including raw signals) with effect sizes greater than 0.2 (BIA feat. filtered)
3. DM + MMSE scores
4. DM + MMSE scores + BIA feat. filtered
5. DM + BIA feat. filtered
6. Features identified with a greedy search algorithm and a binary logistic regression model, previously used in the proposed models
All models based on different feature sets in this experiment were evaluated using datasets stratified by sex and the entire dataset. The candidate feature sets were randomly and identically divided into a training set comprising 70% of the data (females: 172, males: 157, total participants: 329) and a test set comprising 30% of the data (females: 79, males: 62, total participants: 141), with a similar distribution of normal individuals and MCI participants as in the original dataset. To compensate for the imbalance of data in the distribution of NC and MCI participants, the synthetic minority oversampling technique (SMOTE) was applied to all candidate feature sets.
All continuous features were transformed using two methods, z-transformation and min-max transformation, to examine the effect of the different normalization methods. We trained four different machine learning (ML) algorithms on each feature set: Elastic-net logistic regression (ELASTIC-NET) (Zou and Hastie, 2005), support vector machine with a radial basis kernel (svmRBF) (Scholkopf et al., 1997), random forest (RF) (Breiman, 2001), and extreme gradient boosting (XGBOOST) (Chen and Guestrin, 2016). The optimal hyperparameters in the training of each candidate feature set were selected via two rounds of 5-fold cross-validation. The performance of the models was evaluated using the AUROC values of the test sets, considering the combination of the sex-stratified datasets, entire dataset, candidate feature sets, candidate models, and normalization methods. All pipelines in this machine learning experiment were implemented using the tidymodels package within R.
The ages (mean ± SD) of male participants with MCI (n = 78) and normal controls (NCs) (n = 141) were 73.78 ± 6.35 years and 75.33 ± 6.61 years, respectively, and the ages of female participants with MCI (n = 74) and NCs (n = 177) were 70.77 ± 6.29 years and 71.43 ± 6.21 years, respectively. The demographic characteristics, family history, medical history, and neuropsychology test data, according to sex and cognitive status, are summarized in Table 1. BIA data are presented in Table 2 according to sex due to the large difference in body composition between males and females. The age, weight, height, body mass index (BMI), and years of education of participants with MCI and healthy controls were not significantly different according to t tests for males or females. Neuropsychological test scores (on the K-MMSE and SNSB-II) showed significant differences between participants with MCI and NCs in both sexes.
AUROC values were used to compare the classification accuracies of individual variables from neuropsychological screening tools and BIA measurements for the diagnosis of MCI, as shown in Figure 1. The AUROC values of the scores on the SNSB-II memory, frontal, language, and attention domains and the K-MMSE in the male group were more than 0.7, and the maximum AUROC value was obtained with the SNSB-II memory score (0.832). In the female group, AUROC values for SNSB II memory and language were more than 0.7, and the maximum value was obtained with the SNSB-II memory score (0.752), similar to the male group. In the classification model for both sexes, AUROC values of more than 0.7 were obtained for the SNSB-II memory, frontal, and language scores, and the maximum AUROC value was 0.794 (SNSB-II memory score). The AUROC values of K-MMSE scores in males, females, and both sexes were 0.723, 0.622, and 0.663, respectively. According to previous studies, the AUROC values of MMSE scores used for screening for MCI were approximately 0.69–0.78 (Ahn et al., 2010; Shim et al., 2015; Lee et al., 2018). The AUROC value of classification accuracy using the MMSE score from our data was 0.663, showing similar accuracy to that of previous reports.
Figure 1. The area under the receiver operating curve (AUROC) for each variable, including variables of the neuropsychological screening tools and BIA, and DeLong’s 95% confidence intervals for AUROC values.
A prediction model for multiple regression analysis was constructed with the 5 domain scores of SNSB-II (attention, language, memory, visuospatial, and frontal/executive function), and the AUROC values were calculated to evaluate the accuracy of MCI diagnosis. The AUROC values of the prediction model including the 5 domain scores of the SNSB-II were 0.803 in both sexes, 0.840 in the male group, and 0.770 in the female group. In addition, the accuracy of MCI diagnosis was calculated with the AUROC values of the prediction model including the K-MMSE total score and demographic information as variables. The AUROC values of the prediction model including the K-MMSE scores and demographic information were 0.712 in both sexes, 0.756 in the male group, and 0.704 in the female group. The ROC curves of prediction models for MCI diagnosis including SNSB-II and K-MMSE scores are shown in Figure 2.
Figure 2. ROC curves of prediction models according to sex (both sexes, males, and females, from top to bottom). (A) AUROC results of the prediction models including the 5 domain scores of the SNSB-II. (B) AUROC results of the prediction models including K-MMSE scores and demographic information.
The correlations between BIA variables and neuropsychological test scores are shown in a heatmap in Figure 3. The highest correlation coefficient was between extra-cellular water/total body water (ECW/TBW) (BIA variable) and age; this value was 0.449. The correlation coefficient of total body water/fat free mass (TBW/FFM) (BIA variable) and years of education was −0.190, that of the reactance of the right leg at 5 kHz (BIA variable) and SNSB-II attention scores was 0.268, that of TBW/FFM (BIA variable) and SNSB-II language scores was −0.226, that of the phase angle of the left leg at 50 kHz (BIA variable) and SNSB-II visuospatial scores was 0.238, that of ECW/TBW (BIA variable) and SNSB-II memory scores was −0.270, that of the phase angle of the right leg at 50 kHz (BIA variable) and SNSB-II frontal scores was 0.336, and that of ECW/TBW (BIA variable) and K-MMSE scores was −0.254. Previous studies have reported that the correlation coefficients between some of the variables of resting-state EEG data and K-MMSE scores were in the range of 0.3–0.6 (Choi et al., 2019; Doan et al., 2021). The present correlation analyses show that the BIA variables reflected both body composition and characteristic differences in cognitive decline, as indicated by neuropsychological test scores. Therefore, it is expected that the accuracy of MCI prediction can be increased by combining BIA variables and K-MMSE scores.
Figure 3. Heatmap of Pearson’s correlation coefficients between neuropsychological examination tools and BIA measures.
The effect size of the difference between NCs and MCI was also analyzed to select BIA variables to include in the prediction model, as shown in Figure 4. There was a difference in the effect size according to sex; the effect size was 0.4 or more in the phase angles in the male group and in the reactance in the female group. There were also many variables with an effect size of 0.2 or more. Based on the correlation and effect size results, prediction models were developed to distinguish between NCs and individuals with MCI. The developed prediction models included the K-MMSE score and BIA variables; these combined models were compared (according to AUROC values) with prediction models including SNSB-II scores only. Three combined models were constructed: one each for males, females, and both sexes. This was because BIA variables showed significant differences between males and females and to enable comparison. The multiple regression equation of the combined model for males is as follows:
Figure 4. Effect sizes of each BIA measurement and their 95% confidence intervals according to sex. Effect sizes (Cohen’s d) were calculated to compare NC and MCI participants.
where Edu is years of education, MMSE is the K-MMSE total score, FFM is fat-free mass, ECWR is the ratio of extracellular water to total body water (ECW/TBW), 50 kHz_LA_Xc is the reactance of the left arm at 50 kHz, 250 kHz_LA_Xc is the reactance of the left arm at 250 kHz frequency, 50 kHz_LA_PhA is the phase angle of the left arm at 50 kHz, 250kHz_LA_PhA is the phase angle of the left arm at 250 kHz, and 50kHz_WB_PhA is the phase angle of the whole body at 50 kHz. To validate the developed prediction model, the AUROC values were calculated by dividing all male participants into a training set (of 154 participants; 70%) and a test set (of 65 participants; 30%). The AUROC values of the training and test sets for the male combined model, MCIprediction_M, were 0.819 and 0.790, respectively, and the ROC curve and AUROC values are shown in Figure 5A. The multiple regression equation of the combined model for females is as follows:
Figure 5. ROC curves of the training and test sets of the combined models including K-MMSE scores and BIA variables: (A) combined model for males, (B) combined model for females, and (C) combined model for both sexes.
where wt is weight, TBW is total body water, PBF is percent body fat, ECWRTR is the ratio of extracellular water to segmental water in the trunk [extra-cellular water of trunk (ECWTR)/segmental water of trunk (SWTR)], ECWRLL is the ratio of extracellular water to segmental water in the left leg [ECWLL/segmental water of the left leg (SWLL)], BCM is body cell mass, 50kHz_LL_Z is the impedance of the left leg at 50 kHz, 250 kHz_LL_Z is the impedance of the left leg at 250 kHz, and 5kHz_RL_PhA is the phase angle of the right leg at 5 kHz. The AUROC values were calculated by dividing all female participants into a training set (of 176 participants; 70%) and a test set (of 75 participants; 30%). The AUROC values of the training and test sets of the female combined model, MCIprediction_F, were 0.811 and 0.773, respectively, and the ROC curve and AUROC values are shown in Figure 5B. The multiple regression equation of the combined model for both sexes is as follows.
where male is 1 and female is 0 and ICW is intracellular water. The AUROC values were calculated by dividing all participants into a training set (of 329 participants; 70%) and a test set (of 141 participants; 30%). The AUROC values of the training and test sets of the combined model, MCIprediction_B, were 0.776 and 0.751, respectively, and the ROC curve and AUROC values are shown in Figure 5C.
The combined model of MMSE and BIA variables for MCI screening exhibited an AUROC value in Figure 5, comparable to that of the comprehensive neuropsychological battery. While slight variations were observed based on dataset sizes or the extent of separation between training and test sets, it was evident that this model possesses sufficient potential for effective screening MCI. Given the current trend of 10–20% MCI incidence (Langa and Levine, 2014) and the rapid aging of populations, there’s an increasing need for prompt and cost-effective MCI screening technologies. This study’s results showed the feasibility of utilizing the developed models.
Next, to develop an improved MCI screening technique that combined K-MMSE scores and BIA variables, we further employed various machine learning algorithms beyond regression approach. The prediction models generated by these algorithms were compared according to the obtained AUROC values. The training data for machine learning consisted of SNSB-II and K-MMSE scores, BIA variables, and their combinations. ELASTIC-NET, svmRBF, RF, and XGBOOST algorithms were utilized for MCI screening.
Figure 6 presents the classification models developed with machine learning algorithms and their corresponding AUROC values. Among these prediction models, the svmRBF algorithm achieved the highest AUROC value of 0.76; the AUROC values of the RF algorithm for females and the svmRBF algorithm for males were 0.73 and 0.75, respectively. The AUROC values of the other machine learning models ranged from 0.65 to 0.73, with no significant difference from those of the svmRBF model and RF algorithm. Notably, the AUROC values of the prediction models developed with machine learning algorithms did not exhibit significant improvements from the subset regression models developed. This could be attributed to the limited amount of data available for the analysis, which might not have been sufficient for robust machine learning analysis.
Figure 6. The best predictive models (evaluated by test sets) according to the combination of sex-stratified datasets, feature sets, normalization methods and machine learning algorithms.
Expanding the sample size will likely lead to more accurate and reliable prediction models using machine learning techniques. Further studies with more diverse datasets including various ethnics, cultural background and body fat mass will improve clinical utility of the machine learning models for MCI prediction.
With the rapid aging of the population, there’s a growing need for cognitive impairment detection tests among the elderly. However, while simple tools like MMSE or MoCA are less reliable for MCI diagnosis, comprehensive neuropsychological batteries like CERAD and SNSB-II, commonly used for diagnosis, present drawbacks: lengthy administration (around 1.5 h), costly, and need specialized experts. A rapid and accurate MCI screening method enables the prompt and precise identification of individuals at risk of MCI. Directing those identified to in-depth hospital examinations for precise diagnoses saves time and resources compared to extensive testing for more general population group. Therefore, there’s a demand for technology that enables relatively accurate, rapid, and cost-effective MCI screening. Diagnosing mild Cognitive Impairment (MCI) requires a comprehensive approach involving various criteria (Langa and Levine, 2014). These criteria serve as guidelines for clinicians in making a comprehensive judgment regarding the diagnosis of MCI, particularly when considering various potential underlying causes and clinical presentations. Certainly, diagnosing Mild Cognitive Impairment (MCI) relies on an expert’s assessment, combining multiple indicators. Relying on a single indicator for diagnosis is challenging. Having a reliable and simple screening tool for identifying individuals who require detailed MCI diagnosis would significantly aid in the diagnostic process.
Recently, several papers have reported an association between MCI or dementia due to AD and BIA measures. These studies have shown a significant association (ranging from 0.2 to 0.4, value of p <0.05) of BIA variables with MCI or dementia due to AD (Bae et al., 2017; Fujisawa et al., 2017; Mathys et al., 2017). However, the specific BIA variables that vary are inconsistent across papers. Furthermore, the underlying physiological mechanism explaining why BIA variables differ between MCI, AD, and normal controls has not been clearly elucidated. Therefore, further studies are needed to investigate these mechanisms. In summary, there is still a need for reliable and efficient MCI screening techniques given population aging. Although BIA variables have shown promising associations with MCI and AD, more comprehensive research is needed to identify the specific BIA variables indicative of cognitive impairment and to better understand the underlying physiological mechanisms.
The MMSE has been widely utilized in clinical practice as an effective and sensitive test for detecting and screening cognitive impairment and dementia (Benson et al., 2005; Arevalo-Rodriguez et al., 2015). It has a high accuracy of 92% in detecting dementia, with sensitivity ranging from 78 to 84% and specificity ranging from 87 to 91% (Tsoi et al., 2015). However, the MMSE does have some limitations; it can be influenced by factors such as the socio-educational backgrounds of participants and practice, and it has low sensitivity for the early stage of cognitive decline (Scazufca et al., 2009; Duff et al., 2012; Carnero-Pardo, 2014). By integrating the MMSE with BIA variables, which can be easily and quickly performed, it is possible to provide a more convenient and accessible means to detect cognitive impairment in its early stage and recommend for MCI screening. This combination has the potential to overcome some of the limitations associated with use of the MMSE alone, providing a more comprehensive and reliable assessment of cognitive status especially in the early stage.
The comparison of BIA variables among individuals with MCI or dementia is a relatively recent research topic. While there may be minimal disparities in muscle mass between individuals with cognitive impairment and normal cognition, differences in nutritional status or water ratios appear in the reactance or phase angles of segmental BIA measurements. Based on these observations, we constructed a prediction model for MCI that combined BIA variables with MMSE scores. The aim of incorporating BIA variables into the prediction model alongside MMSE scores was to identify new factors for detecting cognitive impairment. The study showed some BIA variables related to muscle mass, extra/intra-cellular water ratio and lower level of bioimpedance features such as reactance and phase angle as risk factors of cognitive impairment. Through the development and evaluation of the prediction model, we aimed to provide evidence of the feasibility of using BIA as a screening tool.
The screening accuracy of the MMSE for cognitive impairment varies with ethnic, language, and demographic factors. To address this variability, compensation tables with MMSE cut-off scores for MCI and dementia screening have been utilized accounting for differences in sex, age, and education level. On the other hand, BIA variables are influenced by factors such as sex, height, weight, age, and ethnicity. Previous studies demonstrated differences in some BIA variables for MCI even after adjusting for age, sex, height, and weight (Doan et al., 2022a). However, there is currently no standardized approach for predicting dementia or MCI using BIA variables. This study used the results of previous studies as a reference to develop an MCI screening model. It is the first to propose combining MMSE scores with BIA variables to enhance the model’s predictive power for detecting MCI. Future studies aiming at developing refined prediction models by accounting for various demographic factors can facilitate more accurate and reliable screening tools for dementia and MCI. Healthcare professionals would effectively screen individuals with diverse backgrounds.
It’s reported that BIA variables serve as valuable indicators for monitoring and screening various diseases and conditions. However, it remains unclear how much these disease-dependent BIA variables would work as confounding factors in MCI screening. Previous studies have shown differences in BIA variables between individuals with diabetes and those without, particularly in PhA (Jun et al., 2018). Considering the previous research findings (Doan et al., 2022a,b, 2023), differences in BIA variables between MCI and normal groups persist even after adjusting for conditions like hyperlipidemia, diabetes, and CNS disorders. In our study, there was a difference in diabetes prevalence between the MCI and NC groups in the male group, but no significant difference was observed in the female group. Nevertheless, when looking at the model’s AUC values, the difference between the male and female groups was not substantial. This indicates that the model’s ability to screen for MCI remains consistent regardless of diabetes status.
In this study, we investigated combinations of machine learning algorithms, BIA variables, and K-MMSE total scores for screening for MCI. However, we did not observe a significant improvement in diagnostic accuracy with machine learning algorithms compared to more classical regression models. Importantly, the small size of the dataset used in this study may have influenced the results. With a larger dataset, machine learning models may be able to capture more patterns and relationships, leading to improved diagnostic accuracy. Additionally, including individuals with a wider range of demographic factors and considering other relevant variables may also contribute to better results in future research.
One limitation of this study is the application of a matched-samples design, which may restrict the generalizability of the findings. The sample used in this study may not be representative of the entire Korean population, further limiting the external validity of the results. Additionally, as BIA variables can vary based on geographical region, participant race, age, sex, BMI, and other factors, it is essential to develop a prediction model for MCI screening based on data from more diverse populations, considering a wider range of regions, age, sex, BMI values, and other factors. This will ensure the broader applicability of a screening tool to predict mild cognitive impairment.
While it is widely acknowledged that MoCA is more effective in detecting MCI than MMSE, our cohort center, the GARD Cohort Research Center, has been collecting data including MMSE, Seoul Neuropsychological Screening Battery (SNSB) II, and BIA since 2017. MoCA is not widely used in Korea yet and it is one of our study limitations.
The estimated prevalence of mild cognitive impairment (MCI) in those over 65 is approximately 10 to 20%. As comprehensive testing for all suspected MCI cases is challenging, a simple and accurate screening tool is needed. To examine the possibility of screening for cognitive impairment in elderly people, we developed a prediction model for mild cognitive impairment (MCI) based on the multivariate regressing model by combining multifrequency bioimpedance analysis (MF-BIA) variables and total scores of the Mini-Mental State Examination (MMSE). Pearson correlation analysis was performed to examine the associations between MF-BIA variables and scores on the MMSE and Seoul Neuropsychological Screening Battery Scores II (SNSB II). Weak correlations were observed with the highest value of 0.336.
To assess the model performance, the AUROC values of the combined model of MF-BIA and MMSE were compared with that of the model using the SNSB-II scores, which were popularly used diagnostic tool for cognitive impairment in South Korea, by dividing the dataset into training and test sets (70 and 30%, respectively). The AUROC values of the SNSB-II were 0.803 in both sexes, 0.840 in the male group, and 0.770 in the female group. The AUROC values of the training and test sets of the combined model were 0.776 and 0.751 in both sexes, 0.819 and 0.790 in the male group, and 0.811 and 0.773 in the female group, respectively. The maximum AUROC value of the model developed with machine learning was 0.76 (for the svmRBF algorithm), which did not significantly differ from the combined model.
The accuracy reduction of the combined model was not significant compared to that of the model based on a standard diagnostic battery (SNSB-II). Accounting for the ease of use, short measurement time, and the low cost to implement in clinics, the BIA and MMSE combined model is considered to have sufficient potential for screening for MCI.
The original contributions presented in the study are included in the article/Supplementary material, further inquiries can be directed to the corresponding author.
The studies involving humans were approved by The Institutional Review Board (IRB) of Chonnam National University Hospital and Chosun University Hospital (IRB number: CNUH-2019-279, CHOSUN 2016-10-005-017). The studies were conducted in accordance with the local legislation and institutional requirements. The participants provided their written informed consent to participate in this study.
M-HJ: Conceptualization, Formal analysis, Investigation, Methodology, Writing – original draft, Writing – review & editing. BK: Formal analysis, Writing – review & editing. KK: Data curation, Writing – review & editing. KL: Data curation, Writing – review & editing. JK: Funding acquisition, Project administration, Writing – original draft, Writing – review & editing.
The author(s) declare financial support was received for the research, authorship, and/or publication of this article. This work was supported by a grant (KSN1824130) from the Korea Institute of Oriental Medicine (KIOM), funded by the Korean government, and the KBRI Basic Research Program through the Korea Brain Research Institute, funded by the Ministry of Science and ICT (24-BR-03-05).
The authors declare that the research was conducted in the absence of any commercial or financial relationships that could be construed as a potential conflict of interest.
All claims expressed in this article are solely those of the authors and do not necessarily represent those of their affiliated organizations, or those of the publisher, the editors and the reviewers. Any product that may be evaluated in this article, or claim that may be made by its manufacturer, is not guaranteed or endorsed by the publisher.
The Supplementary material for this article can be found online at: https://www.frontiersin.org/articles/10.3389/fnagi.2024.1307204/full#supplementary-material
Abu Khaled, M., Mccutcheon, M. J., Reddy, S., Pearman, P. L., Hunter, G. R., and Weinsier, R. L. (1988). Electrical impedance in assessing human body composition: the Bia method. Am. J. Clin. Nutr. 47, 789–792. doi: 10.1093/ajcn/47.5.789
Ahn, H. J., Chin, J., Park, A., Lee, B. H., Suh, M. K., Seo, S. W., et al. (2010). Seoul neuropsychological screening battery-dementia version (Snsb-D): a useful tool for assessing and monitoring cognitive impairments in dementia patients. J. Korean Med. Sci. 25, 1071–1076. doi: 10.3346/jkms.2010.25.7.1071
Arevalo-Rodriguez, I., Smailagic, N., Roque, I. F. M., Ciapponi, A., Sanchez-Perez, E., Giannakou, A., et al. (2015). Mini-mental state examination (MMSE) for the detection of Alzheimer's disease and other dementias in people with mild cognitive impairment (MCI). Cochrane Database Syst. Rev. :Cd010783
Bae, S., Shimada, H., Park, H., Lee, S., Makizako, H., Doi, T., et al. (2017). Association between body composition parameters and risk of mild cognitive impairment in older Japanese adults. Geriatr Gerontol Int 17, 2053–2059. doi: 10.1111/ggi.13018
Beberashvili, I., Azar, A., Sinuani, I., Kadoshi, H., Shapiro, G., Feldman, L., et al. (2014). Longitudinal changes in bioimpedance phase angle reflect inverse changes in serum Il-6 levels in maintenance hemodialysis patients. Nutrition 30, 297–304. doi: 10.1016/j.nut.2013.08.017
Benedict, R. H. B., and Zivadinov, R. (2011). Risk factors for and management of cognitive dysfunction in multiple sclerosis. Nat. Rev. Neurol. 7, 332–342. doi: 10.1038/nrneurol.2011.61
Benson, A. D., Slavin, M. J., Tran, T.-T., Petrella, J. R., and Doraiswamy, P. M. (2005). Screening for early Alzheimer’s disease: is there still a role for the Mini-mental state examination. Prim Care Companion J. Clin. Psychiatry 7, 62–69. doi: 10.4088/pcc.v07n0204
Buffa, R., Mereu, R. M., Putzu, P. F., Floris, G., and Marini, E. (2010). Bioelectrical impedance vector analysis detects low body cell mass and dehydration in patients with Alzheimer's disease. J. Nutr. Health Aging 14, 823–827. doi: 10.1007/s12603-010-0115-9
Buffa, R., Mereu, E., Putzu, P., Mereu, R. M., and Marini, E. (2014). Lower lean mass and higher percent fat mass in patients with Alzheimer's disease. Exp. Gerontol. 58, 30–33. doi: 10.1016/j.exger.2014.07.005
Camina Martin, M. A., De Mateo Silleras, B., Nescolarde Selva, L., Barrera Ortega, S., Dominguez Rodriguez, L., and Redondo Del Rio, M. P. (2015). Bioimpedance vector analysis and conventional bioimpedance to assess body composition in older adults with dementia. Nutrition 31, 155–159. doi: 10.1016/j.nut.2014.06.006
Carnero-Pardo, C. (2014). Should the Mini-mental state examination be retired? Neurologia 29, 473–481. doi: 10.1016/j.nrl.2013.07.003
Chen, T., and Guestrin, C. (2016) Xgboost: a scalable tree boosting system. In Proceedings of the 22nd Acm Sigkdd international conference on knowledge discovery and data mining, 785–794. doi: 10.1145/2939672.2939785
Choi, J., Ku, B., You, Y. G., Jo, M., Kwon, M., Choi, Y., et al. (2019). Resting-state prefrontal Eeg biomarkers in correlation with MMSE scores in elderly individuals. Sci. Rep. 9:10468. doi: 10.1038/s41598-019-46789-2
Ciesielska, N., Sokolowski, R., Mazur, E., Podhorecka, M., Polak-Szabela, A., and Kedziora-Kornatowska, K. (2016). Is the Montreal cognitive assessment (MOCA) test better suited than the mini-mental state examination (MMSE) in mild cognitive impairment (MCI) detection among people aged over 60? Meta-analysis. Psychiatr. Pol. 50, 1039–1052. doi: 10.12740/PP/45368
Colin-Ramirez, E., Castillo-Martinez, L., Orea-Tejeda, A., Vazquez-Duran, M., Rodriguez, A. E., and Keirns-Davis, C. (2012). Bioelectrical impedance phase angle as a prognostic marker in chronic heart failure. Nutrition 28, 901–905. doi: 10.1016/j.nut.2011.11.033
Cova, I., Pomati, S., Maggiore, L., Forcella, M., Cucumo, V., Ghiretti, R., et al. (2017). Nutritional status and body composition by bioelectrical impedance vector analysis: a cross sectional study in mild cognitive impairment and Alzheimer's disease. PLoS One 12:E0171331. doi: 10.1371/journal.pone.0171331
Crespillo-Jurado, M., Delgado-Giralt, J., Reigal, R. E., Rosado, A., Wallace-Ruiz, A., Juarez-Ruiz De Mier, R., et al. (2019). Body composition and cognitive functioning in a sample of active elders. Front. Psychol. 10:1569. doi: 10.3389/fpsyg.2019.01569
Dittmar, M., Reber, H., and Kahaly, G. J. (2015). Bioimpedance phase angle indicates catabolism in type 2 diabetes. Diabet. Med. 32, 1177–1185. doi: 10.1111/dme.12710
Doan, D. N. T., Kim, K., Kim, S. G., Lee, S., Lee, K. H., and Kim, J. (2022a). Segmental bioelectrical impedance analysis for Korean older population with cold pattern. Front. Nutr. 9:975464. doi: 10.3389/fnut.2022.975464
Doan, D. N. T., Kim, K., Ku, B., Lee, K. H., and Kim, J. U. (2023). Reduced body cell mass and functions in lower extremities are associated with mild cognitive impairment and Alzheimer's dementia. Sci. Rep. 13:13389. doi: 10.1038/s41598-023-39110-9
Doan, D. N. T., Ku, B., Choi, J., Oh, M., Kim, K., Cha, W., et al. (2021). Predicting dementia with prefrontal electroencephalography and event-related potential. Front. Aging Neurosci. 13:659817. doi: 10.3389/fnagi.2021.659817
Doan, D. N. T., Ku, B., Kim, K., Jun, M., Choi, K. Y., Lee, K. H., et al. (2022b). Segmental bioimpedance variables in association with mild cognitive impairment. Front. Nutr. 9:873623. doi: 10.3389/fnut.2022.873623
Duff, K., Chelune, G., and Dennett, K. (2012). Within-session practice effects in patients referred for suspected dementia. Dement. Geriatr. Cogn. Disord. 33, 245–249. doi: 10.1159/000339268
Folstein, M. F., Folstein, S. E., and Mchugh, P. R. (1975). "Mini-mental state". A practical method for grading the cognitive state of patients for the clinician. J. Psychiatr. Res. 12, 189–198. doi: 10.1016/0022-3956(75)90026-6
Fujisawa, C., Umegaki, H., Okamoto, K., Nakashima, H., Kuzuya, M., Toba, K., et al. (2017). Physical function differences between the stages from normal cognition to moderate Alzheimer disease. J. Am. Med. Dir. Assoc. 18, 368.e9–368.e15. doi: 10.1016/j.jamda.2016.12.079
Genton, L., Norman, K., Spoerri, A., Pichard, C., Karsegard, V. L., Herrmann, F. R., et al. (2017). Bioimpedance-derived phase angle and mortality among older people. Rejuvenation Res. 20, 118–124. doi: 10.1089/rej.2016.1879
Hoops, S., Nazem, S., Siderowf, A. D., Duda, J. E., Xie, S. X., Stern, M. B., et al. (2009). Validity of the Moca and Mmse in the detection of mci and dementia in Parkinson disease. Neurology 73, 1738–1745. doi: 10.1212/WNL.0b013e3181c34b47
Jackson, A. S., Pollock, M. L., Graves, J. E., and Mahar, M. T. (1988). Reliability and validity of bioelectrical impedance in determining body composition. J. Appl. Physiol. 1985, 529–534.
Jahng, S., Na, D. L., and Kang, Y. (2015). Constructing a composite score for the Seoul neuropsychological screening battery-Core. Dementia Neurocogn. Disord. 14, 137–142. doi: 10.12779/dnd.2015.14.4.137
Jun, M. H., Kim, S., Ku, B., Cho, J., Kim, K., Yoo, H. R., et al. (2018). Glucose-independent segmental phase angles from multi-frequency bioimpedance analysis to discriminate diabetes mellitus. Sci. Rep. 8:648. doi: 10.1038/s41598-017-18913-7
Kang, I. W., Beom, I. G., Cho, J. Y., and Son, H. R. (2016). Accuracy of Korean-Mini-mental status examination based on Seoul neuro-psychological screening battery ii results. Korean J. Fam. Med. 37, 177–181. doi: 10.4082/kjfm.2016.37.3.177
Kuchnia, A. J., Teigen, L. M., Cole, A. J., Mulasi, U., Gonzalez, M. C., Heymsfield, S. B., et al. (2017). Phase angle and impedance ratio: reference cut-points from the United States National Health and Nutrition Examination Survey 1999-2004 from bioimpedance spectroscopy data. JPEN J. Parenter. Enteral Nutr. 41, 1310–1315. doi: 10.1177/0148607116670378
Langa, K. M., and Levine, D. A. (2014). The diagnosis and management of mild cognitive impairment: a clinical review. JAMA 312, 2551–2561. doi: 10.1001/jama.2014.13806
Lee, K. S., Cheong, H. K., Oh, B. H., and Hong, C. H. (2009). Comparison of the validity of screening tests for dementia and mild cognitive impairment of the elderly in a community: K-MMSE, MMSE-K, MMSE-KC, and K-HDS. J. Korean Neuropsychiatr. Assoc. 48, 61–69.
Lee, S. J., Han, J. H., Hwang, J. W., Paik, J. W., Han, C., and Park, M. H. (2018). Screening for Normal cognition, mild cognitive impairment, and dementia with the Korean dementia screening questionnaire. Psychiatry Investig. 15, 384–389. doi: 10.30773/pi.2017.08.24
Mathys, J., Gholamrezaee, M., Henry, H., Von Gunten, A., and Poppa, J. (2017). Decreasing body mass index is associated with cerebrospinal fluid markers of Alzheimer's pathology in mci and mild dementia. Exp. Gerontol. 100, 45–53. doi: 10.1016/j.exger.2017.10.013
Nasreddine, Z. S., Phillips, N. A., Bedirian, V., Charbonneau, S., Whitehead, V., Collin, I., et al. (2005). The Montreal cognitive assessment, MOCA: a brief screening tool for mild cognitive impairment. J. Am. Geriatr. Soc. 53, 695–699. doi: 10.1111/j.1532-5415.2005.53221.x
Petracca, M., Pontillo, G., Moccia, M., Carotenuto, A., Cocozza, S., Lanzillo, R., et al. (2021). Neuroimaging correlates of cognitive dysfunction in adults with multiple sclerosis. Brain Sci. :11. doi: 10.3390/brainsci11030346
R Core Team (2019). R: A language and environment for statistical computing. R Foundation for Statistical Computing, Vienna, Austria. Available at: https://www.R-project.org/
Risacher, S. L., Anderson, W. H., Charil, A., Castelluccio, P. F., Shcherbinin, S., Saykin, A. J., et al. (2017). Alzheimer disease brain atrophy subtypes are associated with cognition and rate of decline. Neurology 89, 2176–2186. doi: 10.1212/WNL.0000000000004670
Roalf, D. R., Moberg, P. J., Xie, S. X., Wolk, D. A., Moelter, S. T., and Arnold, S. E. (2013). Comparative accuracies of two common screening instruments for classification of Alzheimer's disease, mild cognitive impairment, and healthy aging. Alzheimers Dement. 9, 529–537. doi: 10.1016/j.jalz.2012.10.001
Scazufca, M., Almeida, O. P., Vallada, H. P., Tasse, W. A., and Menezes, P. R. (2009). Limitations of the Mini-mental state examination for screening dementia in a community with low socioeconomic status. Eur. Arch. Psychiatry Clin. Neurosci. 259, 8–15. doi: 10.1007/s00406-008-0827-6
Scholkopf, B., Sung, K.-K., Burges, C. J., Girosi, F., Niyogi, P., Poggio, T., et al. (1997). Comparing support vector machines with Gaussian kernels to radial basis function classifiers. IEEE Trans. Signal Process. 45, 2758–2765. doi: 10.1109/78.650102
Selberg, O., and Selberg, D. (2002). Norms and correlates of bioimpedance phase angle in healthy human subjects, hospitalized patients, and patients with liver cirrhosis. Eur. J. Appl. Physiol. 86, 509–516. doi: 10.1007/s00421-001-0570-4
Shafer, K. J., Siders, W. A., Johnson, L. K., and Lukaski, H. C. (2009). Validity of segmental multiple-frequency bioelectrical impedance analysis to estimate body composition of adults across a range of body mass indexes. Nutrition 25, 25–32. doi: 10.1016/j.nut.2008.07.004
Shim, Y., Ryu, H. J., Lee, D. W., Lee, J. Y., Jeong, J. H., Choi, S. H., et al. (2015). Literacy independent cognitive assessment: assessing mild cognitive impairment in older adults with low literacy skills. Psychiatry Investig. 12, 341–348. doi: 10.4306/pi.2015.12.3.341
Smith, T., Gildeh, N., and Holmes, C. (2007). The Montreal cognitive assessment: validity and utility in a memory clinic setting. Can. J. Psychiatr. 52, 329–332. doi: 10.1177/070674370705200508
Tsoi, K. K. F., Chan, J. Y. C., Hirai, H. W., Wong, S. Y. S., and Kwok, T. C. Y. (2015). Cognitive tests to detect dementia a systematic review and meta-analysis. JAMA Intern. Med. 175, 1450–1458. doi: 10.1001/jamainternmed.2015.2152
Keywords: bioimpedance analysis, mild cognitive impairment, dementia, screening, combination of bioimpedance and MMSE
Citation: Jun M-H, Ku B, Kim K, Lee KH and Kim JU (2024) A screening method for mild cognitive impairment in elderly individuals combining bioimpedance and MMSE. Front. Aging Neurosci. 16:1307204. doi: 10.3389/fnagi.2024.1307204
Received: 04 October 2023; Accepted: 08 January 2024;
Published: 24 January 2024.
Edited by:
Jinping Xu, Chinese Academy of Sciences (CAS), ChinaReviewed by:
Jinhuan Zhang, Guangzhou University of Chinese Medicine, ChinaCopyright © 2024 Jun, Ku, Kim, Lee and Kim. This is an open-access article distributed under the terms of the Creative Commons Attribution License (CC BY). The use, distribution or reproduction in other forums is permitted, provided the original author(s) and the copyright owner(s) are credited and that the original publication in this journal is cited, in accordance with accepted academic practice. No use, distribution or reproduction is permitted which does not comply with these terms.
*Correspondence: Jaeuk U. Kim, amFldWtraW1Aa2lvbS5yZS5rcg==
Disclaimer: All claims expressed in this article are solely those of the authors and do not necessarily represent those of their affiliated organizations, or those of the publisher, the editors and the reviewers. Any product that may be evaluated in this article or claim that may be made by its manufacturer is not guaranteed or endorsed by the publisher.
Research integrity at Frontiers
Learn more about the work of our research integrity team to safeguard the quality of each article we publish.