- 1Structural Brain Mapping Group, Department of Neurology, Jena University Hospital, Jena, Germany
- 2Structural Brain Mapping Group, Department of Psychiatry and Psychotherapy, Jena University Hospital, Jena, Germany
- 3Brain and Behaviour (INM-7), Institute of Neuroscience and Medicine, Forschungszentrum Jülich, Jülich, Germany
- 4Institute of Systems Neuroscience, Medical Faculty, Heinrich Heine University Düsseldorf, Düsseldorf, Germany
- 5German Center for Mental Health (DZPG), Jena-Halle-Magdeburg, Germany
Objectives: Previous research has found an association of low bone mineral density (BMD) and regional gray matter (GM) volume loss in Alzheimer’s disease (AD). We were interested whether BMD is associated with GM volume decrease in brains of a healthy elderly population from the UK Biobank.
Materials and methods: T1-weighted images from 5,518 women (MAge = 70.20, SD = 3.54; age range: 65–82 years) and 7,595 men (MAge = 70.84, SD = 3.68; age range: 65–82 years) without neurological or psychiatric impairments were included in voxel-based morphometry (VBM) analysis in CAT12 with threshold-free-cluster-enhancement (TFCE) across the whole brain.
Results: We found a significant decrease of GM volume in women in the superior frontal gyri, middle temporal gyri, fusiform gyri, temporal poles, cingulate gyri, precunei, right parahippocampal gyrus and right hippocampus, right ventral diencephalon, and right pre- and postcentral gyrus. Only small effects were found in men in subcallosal area, left basal forebrain and entorhinal area.
Conclusion: BMD is associated with low GM volume in women but less in men in regions afflicted in the early-stages of AD even in a sample without neurodegenerative diseases.
1. Introduction
In the fields of neuroscience and neurology, the role of bone tissue on brain metabolism has long been neglected, however, novel insights in the bone-brain crosstalk are starting to pique interest of a wider research community. Burgeoning findings show that the bone is an important endocrine organ with intensive bidirectional communication with the brain (and other organs) (Guntur and Rosen, 2012; Rousseaud et al., 2016), is implicated in mood and cognition (Oury et al., 2013; Khrimian et al., 2017; Obri et al., 2018; Nakamura et al., 2021) as well as in multiple neurological, psychiatric and neurodevelopmental disorders (Kelly et al., 2020), such as Parkinson’s disease (Sleeman et al., 2016; Tassorelli et al., 2017), multiple sclerosis (Bisson et al., 2019), autism spectrum disorder (Neumeyer et al., 2015).
The connection between bone health (reflected by bone mineral density and/or bone mineral content, measured by dual-energy X-ray absorptiometry (DXA) or estimated by quantitative ultrasound) and cognition was intensively investigated in women during the 90’s and early 00’s. Bone mineral density was then identified as a surrogate marker of low estrogen levels in postmenopausal women (Tan et al., 2005). Results from these studies indicated a connection between low BMD and an increased risk of Alzheimer’s disease (AD) in women (Lui et al., 2003; Tan et al., 2005), and later also in men (Zhou et al., 2011). The role of BMD in AD was further considered by VBM studies that found an association between low BMD and a decreased gray matter volume in hypothalamic and limbic regions (Loskutova et al., 2010) and in a recent study from Takano et al. (2020), which showed an association between regional gray matter decline in left precuneus and BMD loss in male AD patients but not in women.
Given the consistent reports on the connection of bone measures and brain aging from the researchers associated with the UK Biobank research consortium (Miller et al., 2016; Smith et al., 2019), we wanted to test whether BMD is associated with gray matter volume changes also on a larger healthy sample from the UK Biobank.1
2. Methods
2.1. Participants
This research has been conducted using data from UK Biobank under the application number 41655. The UK Biobank is a biomedical database and research resource that contains genetic, lifestyle and health information from half a million UK Biobank participants (see text footnote 1). UKB holds the ethical approval from the North West Multi-Centre Research Ethics Committee (MREC) and is in possession of informed consents from the study cohort participants.
Due to a predominantly white sample in the UK Biobank and with regard to a possible effect of ethnic background on bone measures (Jorgetti et al., 2014; Zengin et al., 2015; Popp et al., 2017), the analyses were applied only to a sample of participants of British and Irish descent who underwent brain magnetic resonance (MR) imaging and had available BMD measures. Participants with pre-existing neurological or psychiatric diagnoses were excluded from the analyses. Further exclusion criteria were based on ICD-10 diagnosis of bone disorders and the use of medication that can affect bone metabolism (e.g., glucocorticoids, see Supplementary material for detailed exclusion criteria). We limited our analyses to a subset of subjects older than 65 years as men and women start to lose bone mass at a similar rate in this age range (National Institutes of Health Osteoporosis and Related Bone Diseases National Resource Center, 2023).
The analyses were conducted on a sample of 5,518 women (MAge = 70.20, SD = 3.54; age range: 65–82 years) and 7,595 men (MAge = 70.84, SD = 3.68; age range: 65–82 years).
2.2. Bone mineral density data acquisition
Bone mineral density measures of the left femoral neck were obtained by dual energy X-ray absorptiometry (DXA) scanning with iDXA instrument (GE-Lunar, Madison, WI). See https://biobank.ndph.ox.ac.uk/showcase/ukb/docs/DXA_explan_doc.pdf for a detailed report of the acquisition procedure. DXA scanning is a state-of-the-art assessment method for diagnosing osteoporosis (Arabi et al., 2007; Blake and Fogelman, 2007). A T-score instead of a raw score was used for femoral neck bone mineral density, representing a person’s relative bone stiffness of femoral neck in comparison to that of a healthy young adult of their sex.
2.3. MR data acquisition and processing
All the information regarding MR data acquisition is available in the UK Biobank Imaging documentation.2 In short, T1-weighted MR images were acquired on a Siemens Skyra 3T scanner with a 32-channel RF receive head coil. The MPRAGE sequence was used with 1-mm isotropic resolution, inversion/repetition time = 880/2000 ms, acquisition time: 5 min, FOV: 208 × 256 × 256 matrix, in-plane acceleration factor = 2.
Raw structural MR images were processed using default parameters in CAT12.7 Toolbox (Gaser et al., 2022)3 running under standalone MCR version of SPM12 (Wellcome Center for Human Neuroimaging)4 massively parallelized on the JURECA High Performance Computing system (Jülich Supercomputing Centre, 2018).
The images were segmented into gray matter, white matter, and cerebrospinal fluid, non-linearly registered and warped to MNI152NLin2009cAsym standard space with 1.5 × 1.5 × 1.5 mm3, where a Gaussian filtering (FWHM = 4 mm) was applied.
The CAT12 preprocessing has utilized a unified segmentation (Ashburner and Friston, 2005) to remove B0-inhomogeneities and create an initial segmentation that has been used for (local) intensity scaling and adaptive non-local means denoising (Manjon et al., 2010). An adaptive maximum a posteriori (AMAP, Rajapakse et al., 1997) segmentation with a hidden Markov random field (Cuadra et al., 2005) and a partial volume effect model (Tohka et al., 2004) has been adopted to create the final segmentation. Non-linear registration was performed using the Shooting method with modulation (Ashburner and Friston, 2011) as implemented in CAT12.
2.4. Statistical analysis
A multiple regression model was applied in CAT12.8 Toolbox (Gaser et al., 2022, see text footnote 3) running in SPM12 (Wellcome Center for Human Neuroimaging, see text footnote 4) and corrected for different brain sizes by applying a global scaling with total intracranial volume.
In order to obtain more comparable results to Takano et al. (2020), we modeled brain volume changes based on T-scores of BMD of the left femoral neck (UKB data field #23300–2.0) for women and men separately, with age as a covariate. A voxel-wise general linear model (GLM) was applied with threshold-free-cluster-enhancement (TFCE with 10,000 permutations) (Smith and Nichols, 2009), accounting for multiple comparisons across the whole brain using an FWE-correction.
Due to possible effects of lifestyle factors and hormonal changes in women, we ran additional sensitivity analyses. The model for women included age, history of hormone replacement therapy (UKB data field #2814-2.0), hysterectomy (i.e., womb removal; #3591-2.0), bilateral oophorectomy (i.e., removal of both ovaries; #2834-2.0), age at menopause (#3581-2.0) and years since the last menstrual period, number of live births (#2734-2.0), frequency of alcohol intake (#1558-2.0), and pack years of smoking (#20161-2.0) as covariates of non-interest. The sensitivity analysis for men included age, pack years of smoking, and alcohol intake frequency as nuisance parameters.
3. Results
We report the results with FWE-rate of p < 0.01 for voxel-based morphometry (VBM) analysis based on BMD of the left femoral neck for women and men without neurologic or psychiatric impairment (see the sample’s characteristics in Table 1).
As shown in Figure 1, we identified significant TFCE-corrected suprathreshold clusters of decreased GM volume in women with lower BMD of femoral neck in the superior frontal gyri, middle temporal gyri, fusiform gyri, temporal poles, cingulate gyri, precunei, right parahippocampal gyrus and right hippocampus, right ventral diencephalon, and right pre- and postcentral gyrus.
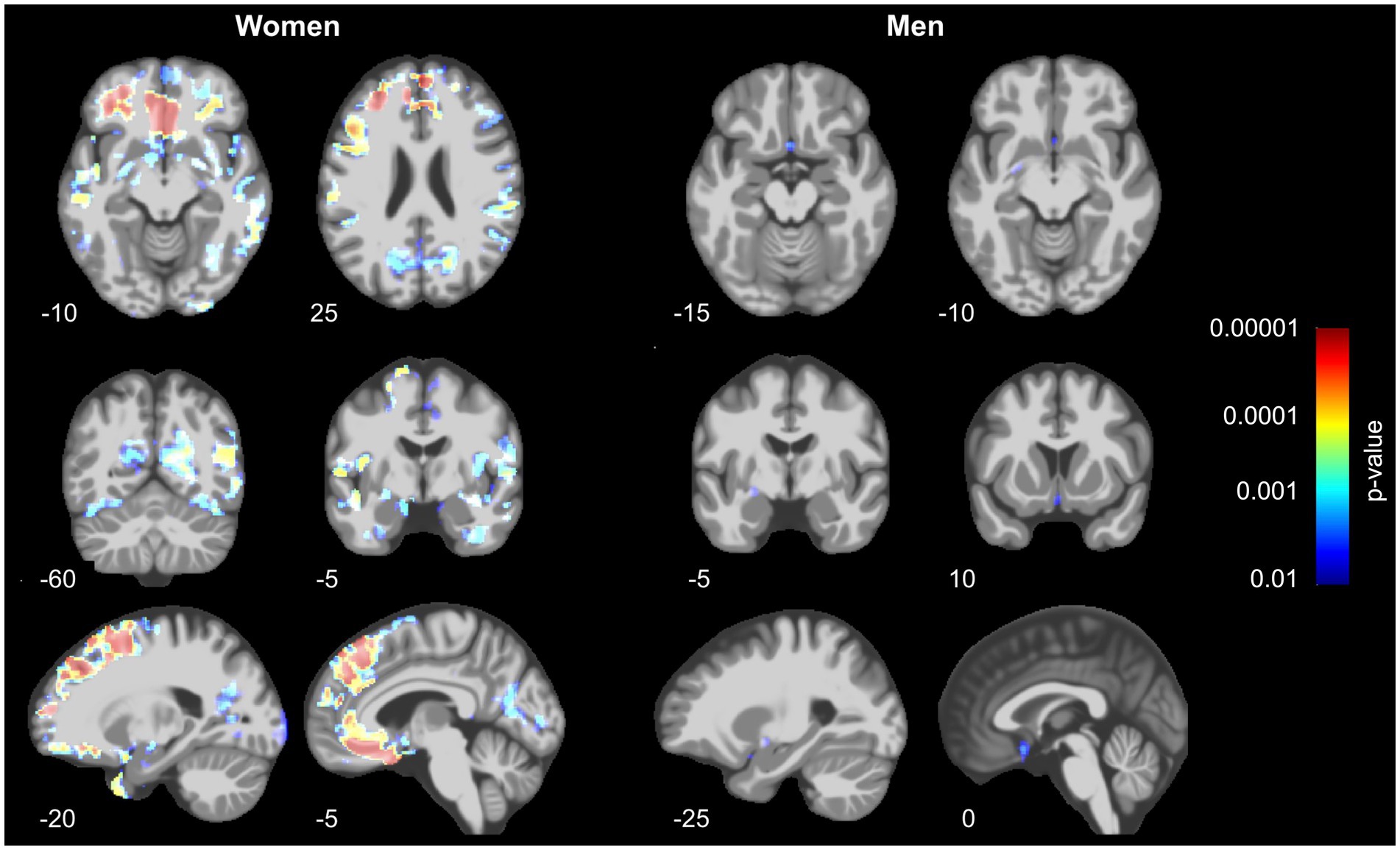
Figure 1. Decrease of gray matter volume in women (left) and men (right) in relation to bone mineral density assessed at the left femoral neck. Prominent results can be seen in women, especially in the prefrontal, parietal and temporo-occipital regions, whereas men show only small effects in a subcallosal area, left basal forebrain and entorhinal area. The results were similar even after controlling for history of hormonal- and lifestyle factors (see Supplementary Figure S1).
In men, a significant decrease of GMV associated with BMD of the femoral neck was found in subcallosal area, left pallidum and basal forebrain, as well as left entorhinal area.
The inclusion of covariates related to hormonal- and lifestyle factors did not change the results (Figure 2 and Supplementary Figure S1). See the Supplementary material for an in-depth overview of the results from VBM (sensitivity) analyses.
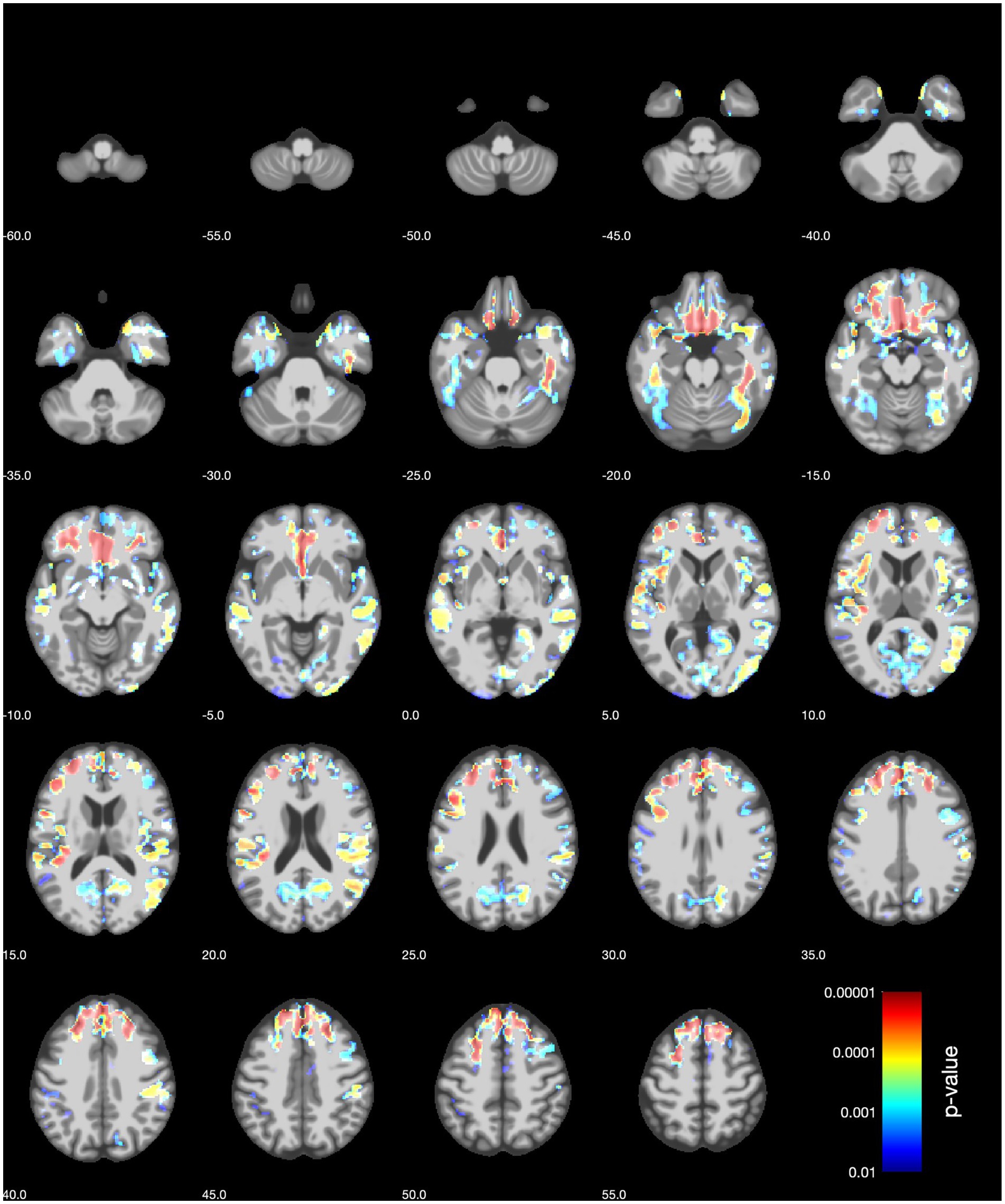
Figure 2. VBM sensitivity analysis results (axial slices) for women for the BMD of the left femoral neck. The covariates included age, history of hormone replacement therapy, hysterectomy, bilateral oophorectomy, age at menopause and years since the last menstrual period, number of live births, frequency of alcohol intake, and pack years of smoking.
4. Discussion
In the present study, we investigated whether bone mineral density is associated with gray matter volume in healthy older adults of British and Irish descent from the UK Biobank population-based cohort. We found a significant association of decrease in BMD and GM volume in women for the superior frontal gyri, middle frontal and temporal gyri, fusiform gyri, temporal poles, cingulate gyri, precunei, right parahippocampal gyrus and right hippocampus, right ventral diencephalon, and right pre- and postcentral gyrus. Minimal effects could be observed in men in the subcallosal area, left pallidum and basal forebrain, as well as the left entorhinal area.
Previous studies investigating the connection of BMD and brain structure found a decrease of GM volume in hypothalamus, cingulate, parahippocampal gyri, left superior temporal gyrus and left inferior parietal cortex in patients with early AD (Loskutova et al., 2010) and in the left precuneus in male AD patients (Takano et al., 2020). Differences in our results to previous findings from Takano et al. (2020) could stem from several possible factors, namely the sample structure and characteristics (AD patients vs. healthy subjects, calcium supplementation in AD women, sample size, ethnicity). Nevertheless, higher effects in women are expected due to an abrupt hormonal change with onset of menopause, with estrogens being a common denominator of bone remodeling as well as changes in brain structure and function (Kalervo Väänänen and Härkönen, 1996; Morrison et al., 2006; Schatz et al., 2020).
Among the predominantly affected regions were those that are commonly involved in cognitive rather than motor functions (Li et al., 2013; Koyama et al., 2017; Herlin et al., 2021). The region of superior frontal gyri was shown to be affected in aging (Bakkour et al., 2013), but also in the early stages of AD (Apostolova et al., 2008). Furthermore, the superior frontal gyri form a part of the default mode network (Li et al., 2013), which is subjected to alterations in AD, especially in the hippocampal formation and PCC/precuneal region (Karas et al., 2007; Mevel et al., 2011; Yokoi et al., 2018). In the present study, GM volume shrinkage was found in PCC and both precunei only in women, however, a decrease in (para)hippocampal regions was found in both men and women. Our results in men showed a GM volume decrease in the subcallosal area and basal forebrain, which was previously shown to be connected to early stages of AD (Hall et al., 2008; Grothe et al., 2012; Kilimann et al., 2014). However, the effects in men were weaker and potentially affected by possible segmentation issues of blood vessels in those parts.
Osteoporotic patients or subjects with low BMD have an increased risk for developing AD (Chang et al., 2014; Kostev et al., 2018; Zhang et al., 2022; Xiao et al., 2023). Decrease in BMD/a diagnosis of osteoporosis and an abnormal GM volume loss in neurodegenerative diseases share similar risk factors that could explain our results (Friedman et al., 2010; Kostev et al., 2018). Lifestyle factors, such as reduced physical activity, smoking, and alcohol consumption, can result in lower BMD and pose a higher risk for developing dementia (Ward and Klesges, 2001; Livingston et al., 2020; Shojaa et al., 2020). However, our sensitivity analyses demonstrated that a BMD-associated decrease of GM volume in the aforementioned regions remains stable even after controlling for history of hormonal exposure and lifestyle factors. The results could also be explained by secretion of the pituitary hormones; pronounced effects in women can be related to postmenopausal signaling of follicle-stimulating hormone, which is involved in osteoporosis as well as neurodegeneration (Zaidi et al., 2023).
Our study has shown that the effect of low BMD on GM volume decrease can be seen before the potential onset of neurodegenerative diseases. Since we did not screen for participants’ cognitive status, it is possible we can observe the effects of prodromal neurodegeneration. Neurodegenerative diseases were previously shown to affect bone health and increase the risk of fractures, which pose a major threat to personal independence and mortality in older adults (Friedman et al., 2010; Kelly et al., 2020). Although our study design does not allow us to draw any causal conclusions, it adds to the body of literature showing that bone health could affect brain health and vice-versa. A possible causal connection of brains influencing the bones has been recently discovered in a Mendelian randomization study (Guo et al., 2023). Further studies that would investigate interrelated longitudinal changes in bone and brain health are necessary to expand our knowledge of the bone-brain crosstalk.
Data availability statement
Publicly available datasets were analyzed in this study. This data can be found at: https://www.ukbiobank.ac.uk/.
Ethics statement
The studies involving humans were approved by North West Multi-Centre Research Ethics Committee (MREC). The studies were conducted in accordance with the local legislation and institutional requirements. Written informed consent for participation was not required from the participants or the participants’ legal guardians/next of kin in accordance with the national legislation and institutional requirements.
Author contributions
RD: Software, Writing – review & editing. FH: Data curation, Resources, Writing – review & editing. CG: Formal analysis, Funding acquisition, Software, Supervision, Writing – review & editing. PK: Conceptualization, Writing – original draft.
Funding
The author(s) declare financial support was received for the research, authorship, and/or publication of this article. This work was supported by Carl Zeiss Stiftung as a part of the IMPULS project (IMPULS P2019-01-006), the Federal Ministry of Science and Education (BMBF) under the frame of ERA PerMed (Pattern-Cog ERAPERMED2021-127) and the Marie Skłodowska-Curie Innovative Training Network (SmartAge 859890 H2020-MSCA-ITN2019). We acknowledge support by the German Research Foundation Projekt-Nr. 512648189 and the Open Access Publication Fund of the Thueringer Universitaets- und Landesbibliothek Jena.
Acknowledgments
This research has been conducted using data from UK Biobank under the application number 41655. We gratefully acknowledge the time and effort of the UK Biobank participants as well as the researchers and staff involved in data collection and management. The authors also gratefully acknowledge the computing time granted by the JARA Vergabegremium and provided on the JARA Partition part of the supercomputer JURECA at Forschungszentrum Jülich.
Conflict of interest
The authors declare that the research was conducted in the absence of any commercial or financial relationships that could be construed as a potential conflict of interest.
The author(s) declared that they were an editorial board member of Frontiers, at the time of submission. This had no impact on the peer review process and the final decision
Publisher’s note
All claims expressed in this article are solely those of the authors and do not necessarily represent those of their affiliated organizations, or those of the publisher, the editors and the reviewers. Any product that may be evaluated in this article, or claim that may be made by its manufacturer, is not guaranteed or endorsed by the publisher.
Supplementary material
The Supplementary material for this article can be found online at: https://www.frontiersin.org/articles/10.3389/fnagi.2023.1287304/full#supplementary-material
Footnotes
1. ^ https://www.ukbiobank.ac.uk/
2. ^ https://biobank.ctsu.ox.ac.uk/crystal/crystal/docs/brain_mri.pdf
References
Apostolova, L. G., Lu, P., Rogers, S., Dutton, R. A., Hayashi, K. M., Toga, A. W., et al. (2008). 3D mapping of language networks in clinical and pre-clinical Alzheimer’s disease. Brain Lang. 104, 33–41. doi: 10.1016/j.bandl.2007.03.008
Arabi, A., Baddoura, R., Awada, H., Khoury, N., Haddad, S., Ayoub, G., et al. (2007). Discriminative ability of dual-energy X-ray absorptiometry site selection in identifying patients with osteoporotic fractures. Bone 40, 1060–1065. doi: 10.1016/j.bone.2006.11.017
Ashburner, J., and Friston, K. J. (2005). Unified segmentation. Neuroimage 26, 839–851. doi: 10.1016/j.neuroimage.2005.02.018
Ashburner, J., and Friston, K. J. (2011). Diffeomorphic registration using geodesic shooting and Gauss–Newton optimisation. Neuroimage 55, 954–967, doi: 10.1016/j.neuroimage.2010.12.049
Bakkour, A., Morris, J. C., Wolk, D. A., and Dickerson, B. C. (2013). The effects of aging and Alzheimer’s disease on cerebral cortical anatomy: specificity and differential relationships with cognition. Neuroimage 76, 332–344. doi: 10.1016/j.neuroimage.2013.02.059
Bisson, E. J., Finlayson, M. L., Ekuma, O., Leslie, W. D., and Marrie, R. A. (2019). Multiple sclerosis is associated with low bone mineral density and osteoporosis. Neurol. Clin. Pract. 9, 391–399. doi: 10.1212/CPJ.0000000000000669
Blake, G. M., and Fogelman, I. (2007). The role of DXA bone density scans in the diagnosis and treatment of osteoporosis. Postgrad. Med. J. 83, 509–517. doi: 10.1136/pgmj.2007.057505
Chang, K.-H., Chung, C.-J., Lin, C.-L., Sung, F.-C., Wu, T.-N., and Kao, C.-H. (2014). Increased risk of dementia in patients with osteoporosis: a population-based retrospective cohort analysis. Age 36, 967–975. doi: 10.1007/s11357-013-9608-x
Cuadra, M. B., Cammoun, L., Butz, T., Cuisenaire, O., and Thiran, J.-P. (2005). Comparison and validation of tissue modelization and statistical classification methods in T1-weighted MR brain images. IEEE Trans. Med. Imaging 24, 1548–1565. doi: 10.1109/TMI.2005.857652
Friedman, S. M., Menzies, I. B., Bukata, S. V., Mendelson, D. A., and Kates, S. L. (2010). Dementia and hip fractures: development of a pathogenic framework for understanding and studying risk. Geriatr. Orthop. Surgery Rehabil. 1, 52–62. doi: 10.1177/2151458510389463
Gaser, C., Dahnke, R., Thompson, P. M., Kurth, F., and Luders, E. (2022). CAT – A computational anatomy toolbox for the analysis of structural MRI data. Preprint. doi: 10.1101/2022.06.11.495736
Grothe, M., Heinsen, H., and Teipel, S. J. (2012). Atrophy of the cholinergic basal forebrain over the adult age range and in early stages of Alzheimer’s disease. Biol. Psychiatry 71, 805–813. doi: 10.1016/j.biopsych.2011.06.019
Guntur, A. R., and Rosen, C. J. (2012). Bone as an endocrine organ. Endocr. Pract. 18, 758–762. doi: 10.4158/EP12141.RA
Guo, B., Wang, C., Zhu, Y., Liu, Z., Long, H., Ruan, Z., et al. (2023). Causal associations of brain structure with bone mineral density: a large-scale genetic correlation study. Bone Res. 11:37. doi: 10.1038/s41413-023-00270-z
Hall, A. M., Moore, R. Y., Lopez, O. L., Kuller, L., and Becker, J. T. (2008). Basal forebrain atrophy is a presymptomatic marker for Alzheimer’s disease. Alzheimers Dement. 4, 271–279. doi: 10.1016/j.jalz.2008.04.005
Herlin, B., Navarro, V., and Dupont, S. (2021). The temporal pole: from anatomy to function—A literature appraisal. J. Chem. Neuroanat. 113:101925. doi: 10.1016/j.jchemneu.2021.101925
Jorgetti, V., Dos Reis, L. M., and Ott, S. M. (2014). Ethnic differences in bone and mineral metabolism in healthy people and patients with CKD. Kidney Int. 85, 1283–1289. doi: 10.1038/ki.2013.443
Jülich Supercomputing Centre (2018). JURECA: data centric and booster modules implementing the modular supercomputing architecture at Jülich Supercomputing Centre. J Large Scale Res Facilities 7:A182. doi: 10.17815/jlsrf-7-182
Kalervo Väänänen, H., and Härkönen, P. L. (1996). Estrogen and bone metabolism. Maturitas 23, S65–S69. doi: 10.1016/0378-5122(96)01015-8
Karas, G., Scheltens, P., Rombouts, S., van Schijndel, R., Klein, M., Jones, B., et al. (2007). Precuneus atrophy in early-onset Alzheimer’s disease: a morphometric structural MRI study. Neuroradiology 49, 967–976. doi: 10.1007/s00234-007-0269-2
Kelly, R. R., Sidles, S. J., and LaRue, A. C. (2020). Effects of neurological disorders on bone health. Front. Psychol. 11:612366. doi: 10.3389/fpsyg.2020.612366
Khrimian, L., Obri, A., Ramos-Brossier, M., Rousseaud, A., Moriceau, S., Nicot, A.-S., et al. (2017). Gpr158 mediates osteocalcin’s regulation of cognition. J. Exp. Med. 214, 2859–2873. doi: 10.1084/jem.20171320
Kilimann, I., Grothe, M., Heinsen, H., Alho, E. J. L., Grinberg, L., Amaro, E. Jr., et al. (2014). Subregional basal forebrain atrophy in Alzheimer’s disease: a multicenter study. J. Alzheimers Dis. 40, 687–700. doi: 10.3233/JAD-132345
Kostev, K., Hadji, P., and Jacob, L. (2018). Impact of osteoporosis on the risk of dementia in almost 60,000 patients followed in general practices in Germany. J. Alzheimers Dis. 65, 401–407. doi: 10.3233/JAD-180569
Koyama, M. S., O’Connor, D., Shehzad, Z., and Milham, M. P. (2017). Differential contributions of the middle frontal gyrus functional connectivity to literacy and numeracy. Sci. Rep. 7:17548. doi: 10.1038/s41598-017-17702-6
Li, W., Qin, W., Liu, H., Fan, L., Wang, J., Jiang, T., et al. (2013). Subregions of the human superior frontal gyrus and their connections. Neuroimage 78, 46–58. doi: 10.1016/j.neuroimage.2013.04.011
Livingston, G., Huntley, J., Sommerlad, A., Ames, D., Ballard, C., Banerjee, S., et al. (2020). Dementia prevention, intervention, and care: 2020 report of the lancet commission. Lancet 396, 413–446. doi: 10.1016/S0140-6736(20)30367-6
Loskutova, N., Honea, R. A., Brooks, W. M., and Burns, J. M. (2010). Reduced limbic and hypothalamic volumes correlate with bone density in early Alzheimer’s disease. J. Alzheimers Dis. 20, 313–322. doi: 10.3233/JAD-2010-1364
Lui, L.-Y., Stone, K., Cauley, J. A., Hillier, T., and Yaffe, K. (2003). Bone loss predicts subsequent cognitive decline in older women: the study of osteoporotic fractures. J. Am. Geriatr. Soc. 51, 38–43. doi: 10.1034/j.1601-5215.2002.51007.x
Manjon, J., Coupe, P., Marti-Bonmati, L., Collins, D. L., and Robles, M. (2010). Adaptive non-local means denoising of MR images with spatially varying noise levels. J. Magn. Reson. Imaging 31, 192–203. doi: 10.1002/jmri.22003
Mevel, K., Chételat, G., Eustache, F., and Desgranges, B. (2011). The default mode network in healthy aging and Alzheimer’s disease. Int. J. Alzheimers Dis. 2011, 1–9. doi: 10.4061/2011/535816
Miller, K. L., Alfaro-Almagro, F., Bangerter, N. K., Thomas, D. L., Yacoub, E., Xu, J., et al. (2016). Multimodal population brain imaging in the UK biobank prospective epidemiological study. Nat. Neurosci. 19, 1523–1536. doi: 10.1038/nn.4393
Morrison, J. H., Brinton, R. D., Schmidt, P. J., and Gore, A. C. (2006). Estrogen, menopause, and the aging brain: how basic neuroscience can inform hormone therapy in women. J. Neurosci. 26, 10332–10348. doi: 10.1523/JNEUROSCI.3369-06.2006
Nakamura, M., Imaoka, M., and Takeda, M. (2021). Interaction of bone and brain: osteocalcin and cognition. Int. J. Neurosci. 131, 1115–1123. doi: 10.1080/00207454.2020.1770247
National Institutes of Health Osteoporosis and Related Bone Diseases National Resource Center (2023). Osteoporosis in men. Available at: https://www.bones.nih.gov/sites/bones/files/pdfs/osteopinmen-508.pdf
Neumeyer, A. M., O’Rourke, J. A., Massa, A., Lee, H., Lawson, E. A., McDougle, C. J., et al. (2015). Brief report: bone fractures in children and adults with autism Spectrum disorders. J. Autism Dev. Disord. 45, 881–887. doi: 10.1007/s10803-014-2228-1
Obri, A., Khrimian, L., Karsenty, G., and Oury, F. (2018). Osteocalcin in the brain: from embryonic development to age-related decline in cognition. Nat. Rev. Endocrinol. 14, 174–182. doi: 10.1038/nrendo.2017.181
Oury, F., Khrimian, L., Denny, C. A., Gardin, A., Chamouni, A., Goeden, N., et al. (2013). Maternal and offspring pools of osteocalcin influence brain development and functions. Cells 155, 228–241. doi: 10.1016/j.cell.2013.08.042
Popp, K. L., Hughes, J. M., Martinez-Betancourt, A., Scott, M., Turkington, V., Caksa, S., et al. (2017). Bone mass, microarchitecture and strength are influenced by race/ethnicity in young adult men and women. Bone 103, 200–208. doi: 10.1016/j.bone.2017.07.014
Rajapakse, J. C., Giedd, J. N., and Rapoport, J. L. (1997). Statistical approach to segmentation of single-channel cerebral MR images. IEEE Trans. Med. Imaging 16, 176–186. doi: 10.1109/42.563663
Rousseaud, A., Moriceau, S., Ramos-Brossier, M., and Oury, F. (2016). Bone-brain crosstalk and potential associated diseases. Horm. Mol. Biol. Clin. Invest. 28, 69–83. doi: 10.1515/hmbci-2016-0030
Schatz, M., Saravanan, S., d’Adesky, N. D., Bramlett, H., Perez-Pinzon, M. A., and Raval, A. P. (2020). Osteocalcin, ovarian senescence, and brain health. Front. Neuroendocrinol. 59:100861. doi: 10.1016/j.yfrne.2020.100861
Shojaa, M., Von Stengel, S., Schoene, D., Kohl, M., Barone, G., Bragonzoni, L., et al. (2020). Effect of exercise training on bone mineral density in post-menopausal women: a systematic review and meta-analysis of intervention studies. Front. Physiol. 11:652. doi: 10.3389/fphys.2020.00652
Sleeman, I., Che, Z. C., and Counsell, C. (2016). Risk of fracture amongst patients with Parkinson’s disease and other forms of parkinsonism. Parkinsonism Relat. Disord. 29, 60–65. doi: 10.1016/j.parkreldis.2016.05.026
Smith, S., and Nichols, T. (2009). Threshold-free cluster enhancement: addressing problems of smoothing, threshold dependence and localisation in cluster inference. Neuroimage 44, 83–98. doi: 10.1016/j.neuroimage.2008.03.061
Smith, S. M., Vidaurre, D., Alfaro-Almagro, F., Nichols, T. E., and Miller, K. L. (2019). Estimation of brain age delta from brain imaging. Neuroimage 200, 528–539. doi: 10.1016/j.neuroimage.2019.06.017
Takano, Y., Tatewaki, Y., Mutoh, T., Morota, N., Matsudaira, I., Thyreau, B., et al. (2020). Voxel-based morphometry reveals a correlation between bone mineral density loss and reduced cortical gray matter volume in Alzheimer’s disease. Front. Aging Neurosci. 12:178. doi: 10.3389/fnagi.2020.00178
Tan, Z. S., Seshadri, S., Beiser, A., Zhang, Y., Felson, D., Hannan, M. T., et al. (2005). Bone mineral density and the risk of Alzheimer disease. Arch. Neurol. 62:107. doi: 10.1001/archneur.62.1.107
Tassorelli, C., Berlangieri, M., Buscone, S., Bolla, M., De Icco, R., Baricich, A., et al. (2017). Falls, fractures and bone density in Parkinson’s disease – a cross-sectional study. Int. J. Neurosci. 127, 299–304. doi: 10.1080/00207454.2016.1206897
Tohka, J., Zijdenbos, A., and Evans, A. (2004). Fast and robust parameter estimation for statistical partial volume models in brain MRI. Neuroimage 23, 84–97. doi: 10.1016/j.neuroimage.2004.05.007
Ward, K. D., and Klesges, R. C. (2001). A meta-analysis of the effects of cigarette smoking on bone mineral density. Calcif. Tissue Int. 68, 259–270. doi: 10.1007/BF02390832
Xiao, T., Ghatan, S., Mooldijk, S. S., Trajanoska, K., Oei, L., Gomez, M. M., et al. (2023). Association of bone mineral density and dementia: the Rotterdam study. Neurology 100, e2125–e2133. doi: 10.1212/WNL.0000000000207220
Yokoi, T., Watanabe, H., Yamaguchi, H., Bagarinao, E., Masuda, M., Imai, K., et al. (2018). Involvement of the precuneus/posterior cingulate cortex is significant for the development of Alzheimer’s disease: A PET (THK5351, PiB) and resting fMRI study. Front. Aging Neurosci. 10:304. doi: 10.3389/fnagi.2018.00304
Zaidi, M., Yuen, T., and Kim, S. M. (2023). Pituitary crosstalk with bone, adipose tissue and brain. Nat. Rev. Endocrinol. doi: 10.1038/s41574-023-00894-5, [Online ahead of print]
Zengin, A., Prentice, A., and Ward, K. A. (2015). Ethnic differences in bone health. Front. Endocrinol. 6:24. doi: 10.3389/fendo.2015.00024
Zhang, X., Hsu, W. W. Q., Sing, C. W., Li, G. H. Y., Tan, K. C. B., Kung, A. W. C., et al. (2022). Low bone mineral density with risk of dementia: a prospective cohort study. J. Am. Med. Dir. Assoc. 23:1719.e9. doi: 10.1016/j.jamda.2022.07.012
Keywords: bone mineral density, osteoporosis, VBM, Alzheimer’s disease, UK Biobank
Citation: Kalc P, Dahnke R, Hoffstaedter F and Gaser C (2023) Low bone mineral density is associated with gray matter volume decrease in UK Biobank. Front. Aging Neurosci. 15:1287304. doi: 10.3389/fnagi.2023.1287304
Edited by:
Taher Darreh-Shori, Karolinska Institutet (KI), SwedenReviewed by:
Anusha Jayaraman, National Neuroscience Institute (NNI), SingaporeWooyoung Jang, Gangneung Asan Hospital, Republic of Korea
Copyright © 2023 Kalc, Dahnke, Hoffstaedter and Gaser. This is an open-access article distributed under the terms of the Creative Commons Attribution License (CC BY). The use, distribution or reproduction in other forums is permitted, provided the original author(s) and the copyright owner(s) are credited and that the original publication in this journal is cited, in accordance with accepted academic practice. No use, distribution or reproduction is permitted which does not comply with these terms.
*Correspondence: Polona Kalc, cG9sb25hLmthbGNAbWVkLnVuaS1qZW5hLmRl