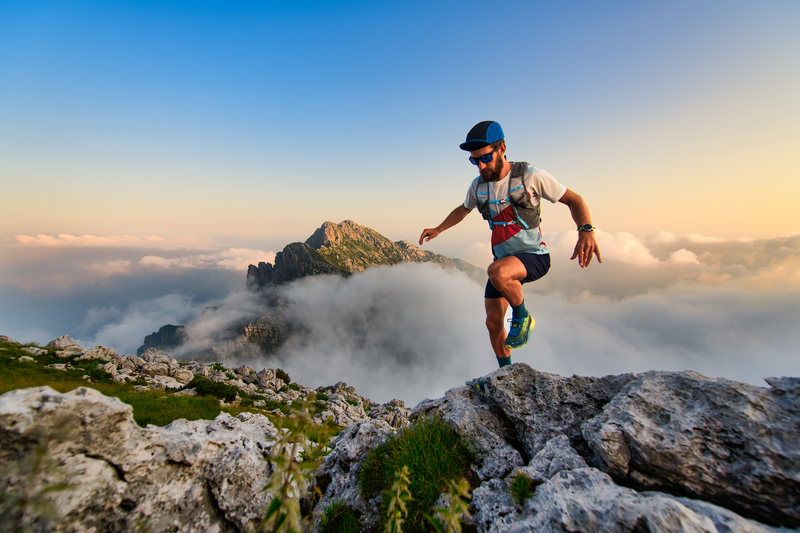
95% of researchers rate our articles as excellent or good
Learn more about the work of our research integrity team to safeguard the quality of each article we publish.
Find out more
ORIGINAL RESEARCH article
Front. Aging Neurosci. , 20 November 2023
Sec. Neurocognitive Aging and Behavior
Volume 15 - 2023 | https://doi.org/10.3389/fnagi.2023.1285375
This article is part of the Research Topic Learning and Memory 2023 - Volume II View all 30 articles
Introduction: Separate unimodal magnetic resonance imaging (MRI) literatures have shown that hippocampal gray matter macrostructure (volume) and microstructure (diffusion) decline with age and relate to episodic memory performance, with multimodal MRI studies reporting that episodic memory may be better explained by a combination of these metrics. However, these effects are often assessed independent of age or only within older adults and therefore do not address whether these distinct modalities explain variance in (i.e, mediate) the effect of age on episodic memory.
Methods: Here, we simultaneously examined the unique and joint contribution of hippocampal volume and diffusion to age-related differences in episodic memory in 83 younger and 61 older adults who underwent a T1- and diffusion-weighted MRI and completed the Rey Auditory Verbal Learning Test.
Results: As expected, older age was significantly related to smaller volume and higher diffusion (intracellular, dispersion, and free) in bilateral hippocampus and to worse episodic memory performance (immediate and delayed free recall, recognition). Structural equation modelling revealed that the age-memory relationship was significantly mediated by hippocampal diffusion, but not volume. A non-significant influential indirect effect further revealed that the structural metrics did not jointly mediate the age-memory relationship.
Discussion: Together, these findings indicate that hippocampal microstructure uniquely contributes to age-related differences in episodic memory and suggest that volume and diffusion capture distinct neurobiological properties of hippocampal gray matter.
The neurocognitive aging field seeks to identify the neural basis of age-related declines in various cognitive abilities. A key function known to decline in otherwise cognitively normal older adults is the ability to remember past events (Craik, 1994; Nyberg et al., 1996). These episodic memories are often assessed using list learning tasks, such as the Rey Auditory Verbal Learning Task (RAVLT) (Rey, 1941), in which participants study a list of words and are later tested on their ability to generate those words without cues (free recall) or identify them among distractor words (recognition). Older adults consistently recall fewer words than younger adults, particularly after a delay, but there are mixed findings regarding age effects for recognition memory (Craik and McDowd, 1987; Parker et al., 2004; Rhodes et al., 2019). These age-related differences in episodic memory are often attributed to degradation of the hippocampus in older adults (Van Petten, 2004; Leal and Yassa, 2015), which can be assessed using structural magnetic resonance imaging (MRI). Whereas most studies report on a single MRI modality, multimodal approaches are needed to determine which modalities are more sensitive to the effect of age on memory performance.
One of the most commonly used structural MRI modalities is T1-weighted imaging, which measures differences in tissue contrast and can be used to assess macrostructural properties of individual brain regions, such as their size or shape (Orrison et al., 1995). Unimodal T1-weighted MRI studies have consistently shown age-related decreases in volume of the hippocampus (Raz et al., 2005; Walhovd et al., 2005; Du et al., 2006; Nobis et al., 2019) and that smaller hippocampal volume relates to worse delayed free recall (Golomb et al., 1994; De Leon et al., 1997; Lye et al., 2004; Bruno et al., 2016) and recognition memory (Shing et al., 2011; Bender et al., 2013; Bennett et al., 2019). However, these volume-memory relationships are primarily seen within older adults whereas studies of adults across the lifespan do not observe significant relationships between hippocampal volume and episodic memory (Sullivan et al., 1995; Raz et al., 1998; Tisserand et al., 2000; Rodrigue and Raz, 2004).
A complementary structural MRI modality is diffusion-weighted imaging, which measures the movement of molecular water and can be used to assess microstructural properties of tissue, such as the presence and organization of neurons and glia (Beaulieu, 2002; Afzali et al., 2021). Neurite Orientation Dispersion and Density Imaging (NODDI) is a multi-compartment modeling approach that yields separate estimates of diffusion within (intracellular) and between (dispersion) cells and from non-cellular sources (free) (Zhang et al., 2012). These NODDI metrics are more sensitive to age differences in diffusion in gray matter than single tensor metrics (Venkatesh et al., 2020). NODDI studies have shown age-related increases in all diffusion metrics in the hippocampus (Nazeri et al., 2015; Metzler-Baddeley et al., 2019; Radhakrishnan et al., 2020; Venkatesh et al., 2020, 2021; Franco et al., 2021) and that higher hippocampal diffusion relates to worse delayed free recall in younger and older adults (Radhakrishnan et al., 2020; Venkatesh et al., 2021). Thus, whereas hippocampal macrostructure (volume) only relates to episodic memory performance within older adults, hippocampal microstructure (diffusion) is sensitive to individual differences in memory performance across the adult lifespan. What remains untested is the extent to which either of these MRI modalities in hippocampal gray matter explains variance in (i.e., mediate) the effect of age on episodic memory.
An important follow up question is whether macrostructural and microstructural properties of hippocampal gray matter are differentially sensitive to effects of age on episodic memory. The multimodal MRI literature has primarily provided evidence that diffusion captures unique variance that is more sensitive to episodic memory than volume, although these studies have been limited to older adults or assessed the structure-memory relationships independent of age. For example, one study that compared these MRI modalities in the same group of older adults found that single tensor metrics of hippocampal diffusion, but not hippocampal volume, related to memory performance (den Heijer et al., 2012). Another study reported that episodic memory performance across younger and older adults was better predicted when multi-compartment diffusion in the hippocampus was added to a model of hippocampal volume and single tensor diffusion metrics (Radhakrishnan et al., 2022). A third multimodal MRI study instead focused on their shared variance, finding that episodic memory in older adults was related to a latent construct of structural MRI modalities, including T1- and diffusion-weighted metrics, in the hippocampus (Köhncke et al., 2021). The combination of unique and shared variance in memory performance may indicate that volume and diffusion capture at least some different underlying neurobiological substrate (s), such as neurodegeneration, demyelination, or inflammation, or perhaps the same substrates but to different degrees.
Given that prior work had separately related hippocampal macrostructure (volume) and microstructure (diffusion) to episodic memory performance without testing whether they mediate the effect of age on episodic memory as well as questions about whether these MRI modalities capture different underlying neurobiological substrates, the current study used serial multiple mediation structural equation modeling (SEM) to simultaneously examine the extent to which one (specific indirect effects) or both (influential indirect effect) latent variables of each MRI modality (volume, diffusion) can explain variance in the effect of age on multiple forms of episodic memory (immediate free recall, delayed free recall, yes/no recognition) in a large sample of younger and older adults (n = 144). Note that mediation is used here to test whether volume and/or diffusion explain cross-sectional variance in memory that is related to age (statistical mediation) and not to make claims about their causal influences (causal mediation). Specific indirect effects were expected for diffusion, with weaker or non-significant effects for volume given that prior studies have not found significant volume-memory relationships in samples with younger and older adults (Sullivan et al., 1995; Raz et al., 1998; Tisserand et al., 2000; Rodrigue and Raz, 2004). A significant influential indirect effect would further indicate that these distinct MRI modalities explain shared variance in the effect of age on memory performance, as would be expected if microstructure and macrostructure capture common neurobiological substrates. Additional analyses used raw volume instead of normalized volume as normalization methods have been shown to influence volume-memory relationships in older adults (Van Petten, 2004) and separate models for each memory metric as prior work was largely limited to free recall. An exploratory analysis reran the original model within older adults only for comparison to prior work.
Eighty-nine healthy younger adults and 83 older adults were recruited from the University of California, Riverside undergraduate research pool and surrounding Riverside community, respectively. All participants were screened for conditions that would prevent them from safely entering an MRI scanner (e.g., ferrous mental implants, claustrophobia, pregnancy). Participants were excluded for having no behavioral or MRI data (1 younger, 6 older) data or poor general cognition assessed using the Mini-Mental State Exam (scores <26; 3 younger) (Folstein et al., 1975) or Montreal Cognitive Assessment (scores <21; 2 older) (Pendlebury et al., 2013). Extensive quality checks were conducted on the raw and processed behavioral and MRI data to identify artifacts and outliers that could bias the variables of interest. This resulted in additional exclusions for extremely poor episodic memory performance (RAVLT recognition scores >3 standard deviations below the sample mean corresponding to negative values indicating that participants had more false alarms than hits; 1 younger, 3 older), significant lesions in bilateral hippocampus (more than two lesions that were each >2 voxels in diameter; 6 older), uncorrectable MRI registration or segmentation errors (1 younger, 3 older), and having structural metric values exceeding 3 standard deviations from the sample mean (2 older). The final sample included 83 younger (18–29 years) and 61 older (65–86 years) adults (see Table 1).
All participants gave consent and received either course credit or monetary compensation for their participation. Study procedures were approved by the Institutional Review Board of the University of California, Riverside.
Participants completed the Rey Auditory Verbal Learning Task (RAVLT) (Rey, 1941) to assess free recall and yes/no recognition. On each on five recall trials, the experimenter read the same list of 15 common words (List A) after which the participant was asked to freely recall as many words as possible. On a subsequent interference trial, the experimenter read a different list of 15 common words (List B) that the participant had to freely recall. Immediate and delayed recall scores were calculated as the number of words correctly recalled from List A immediately after the interference trial and after a 20-min delay, respectively. Less commonly used immediate recall measures include total recall (average number of words correctly recalled on the five recall trials from List A) and interference list recall (average number of words correctly recalled from List B). The experimenter then read a list of 50 words and the participant had to indicate if they were (“yes”) or were not (“no”) from List A. A recognition score was calculated as the difference between the number of correctly (hits) and incorrectly (false alarms) identified List A words.
Imaging data were acquired using a 3-T Siemens MRI (Siemens Healthineers, Malvern, PA) scanner with a 32-channel receive-only head coil at the Center for Advanced Neuroimaging at the University of California, Riverside. Head movement was minimized by placing a fitted padding around each participant’s head.
A single T1-weighted high-resolution magnetization prepared rapid acquisition gradient echo (MP-RAGE) sequence was acquired using the following parameters: echo time (TE)/repetition time (TR) = 2.72/2400 ms, 208 axial slices, field of view = 256 × 256 × 208 mm, flip angle = 8 degrees, and spatial resolution = 0.8 mm3.
A pair of diffusion-weighted scans with opposite phase encoding directions were also acquired. Each scan had two gradient strengths (b = 1,500 and 3,000 s/mm2) applied in 64 orthogonal directions, with six images having no diffusion weighting (b = 0) and using the following acquisition parameters: TE/TR = 102/3500 ms, field of view = 212 × 182 mm, 72 interleaved slices, and spatial resolution = 1.7 mm3.
Bilateral hippocampus was automatically segmented on each participant’s MP-RAGE using FMRIB Software Library (FSL) (Jenkinson et al., 2012) Integrated Registration and Segmentation Tool (FIRST) (Patenaude et al., 2011). The three-stage affine registration flag was used to optimize fitting of the standard space model to subject space. Outputs were visually inspected by trained researchers blind to participants’ age, and participants were excluded for excessive (>1 voxel) and uncorrectable over- or under-fitting.
For each participant, raw hippocampal volumes were calculated separately in each hemisphere from the FIRST-segmented structures in MP-RAGE space (Volumeraw). Individual differences in brain size were then corrected using the residual normalization method (Jack et al., 1989). Intracranial volume was measured for each participant using the Estimated Total Intracranial Volume (eTIVindiv) generated by FreeSurfer (v.6.0)1 and then averaged within younger participants (eTIVmean). The effect of brain size on hippocampal volume was estimated using the slope of the regression line between eTIVindiv and Volumeraw, separately for each hemisphere (β) within younger participants to get estimates of these measures in the absence of age-related atrophy. Normalized volumes (Volumenorm) were then calculated separately for bilateral hippocampus in each participant using the equation:
For each participant, diffusion data were pre-processed using Analysis of Functional Neuro Images (AFNI) (Cox and Hyde, 1997) to remove non-brain tissue and generate a whole brain mask. FSL’s Topup was then used to generate a field map and Eddy was used to correct for motion, eddy-current induced distortions, and susceptibility induced distortions.
Pre-processed diffusion data were analyzed using the NODDI MATLAB toolbox2, which generates voxel-wise estimates of three metrics thought to reflect different sources of the diffusion signal (Zhang et al., 2012; Tariq et al., 2016). For better modeling within gray matter, the intrinsic diffusivity metric was adjusted to 1.1 μM3 (Guerrero et al., 2019). Intracellular diffusion (also known as neurite density index, NDI, or intracellular volume fraction, fICVF) is modelled as a set of sticks, dispersion of diffusion (also known as orientation dispersion index, ODI) is modelled as the dispersion of those sticks, and free diffusion (also known as fraction of isotropic diffusion, fISO) is modeled as an isotropic sphere.
These diffusion metrics were extracted from bilateral hippocampus for each participant. Segmented hippocampus masks were registered to diffusion space by applying the inverse of a rigid body transformation (6 degrees of freedom, boundary-based registration cost function) from a linear alignment between the brain extracted diffusion (distortion-corrected average b0) and MP-RAGE images. Mean diffusion metrics were then calculated separately for each hemisphere by multiplying each registered hippocampus mask by the corresponding voxel-wise NODDI image and taking the average across voxels. All diffusion metrics were limited to voxels with sufficient signal (excluding voxels with intracellular diffusion >0.99 that are found in regions with low signal-to-noise ratios) (Emmenegger et al., 2021) and the intracellular and dispersion of diffusion metrics were further limited to voxels with sufficient cellular sources of the diffusion signal (excluding voxels with free diffusion >0.9) as values may not be meaningful when the cellular source is too low (G. Zhang personal communication, January 25, 2018).
Latent constructs of hippocampal volume and diffusion and episodic memory were generated using AMOS by SPSS (version 27.0, IBM, 2020). The latent volume construct was built from measures of normalized hippocampal volume from each hemisphere. The latent diffusion construct was built from latent constructs of the hippocampal NODDI metrics (intracellular, dispersion, free), each of which was built from the corresponding mean diffusion metrics from each hemisphere. Separate measures from the left and right hemisphere were used to estimate bilateral latent factors based on similar prior work (Foster et al., 2019) and to better capture shared variance between the hemispheres in the absence of hemisphere-specific predictions. Finally, the latent construct of episodic memory was built from the RAVLT immediate and delayed free recall and recognition scores. Confirmatory factor analyses were used to determine whether the measured variables significantly represented their respective latent constructs (see Supplementary Figures S1A,B).
To assess whether hippocampal volume and diffusion uniquely or jointly explained variance in the effect of age on episodic memory performance, we conducted serial multiple mediation structural equation models using data from all younger and older participants (or older adults only) with AMOS by SPSS (see Figure 1). The models depicted two specific indirect pathways (separately for latent constructs of diffusion and volume) as well as an influential indirect pathway (from the latent construct of diffusion to volume) between age and the latent memory construct (or each memory metric separately). The influential indirect pathway was calculated as the product of the direct effect estimates of age on diffusion, diffusion on volume, and volume on memory. Prior to model fitting and analyses, age was mean centered and all diffusion metrics were multiplied by −1 to match the direction of age effects across modalities. Modification indices were used to adjust the model if necessary.
Figure 1. Structural equation model used to test the mediating effects of hippocampal diffusion (intracellular, dispersion, free) and volume on age-related differences in episodic memory performance (immediate free recall, delayed free recall, recognition). Circles represent latent variables and rectangles represent measured variables. Bolded values depict significant path estimates. L/R = left/right hemisphere.
Standardized path estimates were derived from bootstrapping using 5,000 iterations with 95% confidence intervals. Model fit was evaluated using established thresholds across several indices: non-significant chi-square (χ2) value, root mean square error of approximation (RMSEA) ≤ 0.05, comparative fit index (CFI) > 0.90, and standardized root mean residual (SRMR) < 0.08 (Hu and Bentler, 1999; Raykov and Marcoulides, 2006). Improvements in model fit were assessed by comparing Akaike information criterion (AIC) and Bayesian information criterion (BIC) measures, with smaller values indicating better fit. Specific indirect effects were tested with the James and Brett (1984) method, by which a significant indirect effect is sufficient to support an indirect relationship by means of an intermediate variable.
The effect of age on each measured variable was assessed using separate Pearson correlations (Table 2). Significant effects survived Bonferroni correction for 11 comparisons per measure, p < 0.0045. Results revealed that older age was significantly related to higher hippocampal diffusion, smaller hippocampal volume, and worse episodic memory performance. Moreover, all memory scores were significantly related to all hippocampal diffusion metrics, but not to hippocampal volume. Similar analyses conducted separately within younger and older adults are presented in Supplementary Tables S1, S2.
Prior to model fitting, separate first- and second-order confirmatory factor analyses confirmed that all measured variables significantly represented their respective latent constructs (Table 3; Supplementary Figures S1A,B; Supplementary Table S3). As such, latent constructs for hippocampal diffusion, hippocampal volume, and episodic memory were used in the subsequent structural equation models.
The original SEM in all participants (Figure 1) initially resulted in fits (AIC = 288.3, BIC = 383.3) that were improved after implementing a modification index correlating the residuals of right hemisphere hippocampal intracellular diffusion and right hemisphere hippocampal free diffusion (AIC = 207.0, BIC = 305.0). This resulted in acceptable, but not strong, model fits as they were significant according to standard thresholds for some indices (CFI, SRMR), but not others (χ2, RMSEA). Although these findings are mixed, the acceptability of our model is further supported by it being a significantly better fit to the data than a model of covariances (Supplementary Figure S1C; Supplementary Table S4).
As seen in Table 4 (column for All Participants, Original), significant path effects were seen for the total effect of age on memory, but not the direct effect of age on memory after accounting for the mediators. The specific indirect effect on the age-memory relationship was significant for hippocampal diffusion, but not normalized hippocampal volume. Moreover, the influential indirect pathway capturing the joint mediating effects of hippocampal diffusion and volume on the age-memory relationship was not significant. Standardized and unstandardized path effects and 95% confidence intervals are presented in Supplementary Table S5.
Results of the original model in all participants remained unchanged when using raw volume instead of normalized volume. Model fits were comparable to the original model (AIC = 202.1, BIC = 300.1) and the same standardized path effects were significant (Table 4 column for All Participants, Raw Volume).
Results of the original model in all participants remained unchanged when separate models were conducted for each memory measure rather than using the latent memory construct. Model fits were comparable to the original model (immediate free recall: AIC = 182.1, BIC = 265.3; delayed free recall: AIC = 183.4, BIC = 266.5; recognition: AIC = 185.7, BIC = 268.9) and similar standardized path effects were significant, except that the total indirect effect was not significant for the immediate free recall or recognition models and the direct effect was significant for the immediate free recall model (Table 4 column for All Participants, Immediate Recall, Delayed Recall, and Recognition).
Given that relationships between hippocampal volume and episodic memory had been primarily reported in studies of older adults, rather than adults across the lifespan, the original SEM was run again using data from only older adults. Using the same modification index, model fits for this exploratory SEM were comparable to the original model (AIC = 139.0, BIC = 208.6), with acceptable model fits according to CFI and SRMR, but not the other indices (χ2, RMSEA). However, no standardized path effects were significant (Table 4 column for Older Adults, Original).
The current study assessed the unique and joint contributions of hippocampal microstructure (diffusion) and macrostructure (volume) to age-related differences in multiple forms of episodic memory using SEM in a large sample of younger and older adults. In addition to replicating previously observed negative effects of age on hippocampal diffusion (Nazeri et al., 2015; Metzler-Baddeley et al., 2019; Radhakrishnan et al., 2020; Venkatesh et al., 2020; Franco et al., 2021), hippocampal volume (Raz et al., 2005; Walhovd et al., 2005; Du et al., 2006), and memory performance (Craik and McDowd, 1987; Nyberg et al., 1996; Rönnlund et al., 2005), our results revealed two key findings. First, a significant indirect effect for hippocampal diffusion, but not volume, revealed that microstructure explained variance in the effect of age on memory performance, extending the literature by replicating similar findings from unimodal MRI studies within the same sample and using multi-compartment diffusion metrics. Second, the influential indirect pathway was not significant, indicating that hippocampal diffusion and volume made independent contributions to the age-episodic memory relationship.
Indirect effects revealed that the age-related difference in episodic memory was significantly explained by hippocampal diffusion, but not hippocampal volume. In other words, cross-sectional variance in memory performance that was related to age was better captured by diffusion in the hippocampus. These finding are consistent with previous unimodal multi-compartment diffusion-weighted (Radhakrishnan et al., 2020; Venkatesh et al., 2021) and T1-weighted (Raz et al., 1998; Tisserand et al., 2000; Rodrigue and Raz, 2004) studies that included younger and older adults. However, an advantage of the current multimodal approach is that these unique effects for each structural MRI modality were assessed within the same sample, revealing that diffusion was more sensitive to memory deficits in older adults than volume. At least one multimodal imaging study within older adults similarly found that hippocampal diffusion, but not hippocampal volume, predicted episodic memory performance when using separate regression models for each structural modality (den Heijer et al., 2012), although they did not assess whether either structural metric mediated the effect of age on episodic memory, which was of interest here. The current study further extends these literatures by having used a latent memory construct that captured shared variance across multiple episodic memory metrics, not all of which were included in previous studies.
The non-significant influential indirect effect revealed that hippocampal diffusion and volume did not jointly contribute to the age-related decline in episodic memory. This finding is consistent with some multimodal MRI studies that focused on structure-memory relationships independent of age, which reported independent contributions of hippocampal diffusion and volume to episodic memory performance (den Heijer et al., 2012), but not with others whose approaches focused on the additive (Radhakrishnan et al., 2022) or shared (Köhncke et al., 2021) variance across these structural MRI measures. Similar results have also been observed in multimodal MRI studies that examined the contribution of volume in hippocampal gray matter and diffusion in white matter emanating from the hippocampus (e.g., fornix) to memory performance in aging (Gorbach et al., 2017; Foster et al., 2019; Hayek et al., 2020), with one study in adults across the lifespan finding that the effect of age on associative memory was mediated by fornix diffusion, but not volume of medial temporal structures that included the hippocampus (Foster et al., 2019). Finding similar relationships to age, but different relationships to episodic memory performance, supports the notion that diffusion- and T1-weighted MRI modalities are capturing at least some distinct neurobiological substrates in hippocampal gray matter (Wolf et al., 2015), or perhaps the same substrates but to different degrees. We speculate that microstructural metrics may be more influenced by subtle individual differences or early-stage age-related differences in neuronal (e.g., neuron density, myelination, arborization) or glial (e.g., swelling) tissue properties compared to macrostructural metrics that may capture more advanced or late-stage differences, making diffusion more sensitive to individual- and age-related differences in memory performance.
One caveat of our study is that the small and non-significant contribution of hippocampal volume to the age-memory relationship across all participants may have affected our ability to detect an influential indirect effect. Unimodal T1-weighted studies in older adults have observed significant relationships between hippocampal volume and episodic memory (Golomb et al., 1994; De Leon et al., 1997; Lye et al., 2004; Shing et al., 2011; Bender et al., 2013; Bruno et al., 2016; Bennett et al., 2019), but the current sample of older adults may be too small to reliably test with our SEM model, as seen by there being no significant standardized path effects in the exploratory model within older adults only. Another possibility is that these effects may be localized to the dentate gyrus/cornu ammonis 3 subfields of the hippocampus, as seen in prior work (Shing et al., 2011; Bender et al., 2013; Bennett et al., 2019), and may be obscured here by our focus on volume and diffusion of the whole hippocampus.
In summary, the current study revealed that, although episodic memory performance and both hippocampal macrostructure (volume) and microstructure (diffusion) were worse in older than younger adults, only hippocampal microstructure contributed to the effect of age on episodic memory performance. These findings cannot be interpreted as demonstration of a causal effect of age on diffusion or memory, given well-known limitations of mediation, particularly when using age as a predictor in cross-sectional designs (e.g., Lindenberger et al., 2011). Instead, our approach has identified promising relationships among age, brain structure, and memory that should be explored in future longitudinal studies that can speak to age-related change. These findings would be further strengthened by replication in a sample of adults across the lifespan, as well as studies showing generalization to other forms of memory. Nonetheless, the results for NODDI diffusion metrics have implications for biomarker research aimed at establishing reliable neural metrics that vary with age and memory performance. We further demonstrate that SEM is an important tool for multimodal MRI, enabling the simultaneous characterization of unique (specific indirect effects) and joint (influential indirect effect) variance explained by multiple imaging modalities to age-related differences in cognition.
The raw data supporting the conclusions of this article will be made available by the authors, without undue reservation.
The studies involving humans were approved by Institutional Review Board of the University of California, Riverside. The studies were conducted in accordance with the local legislation and institutional requirements. The participants provided their written informed consent to participate in this study.
KI: Conceptualization, Formal analysis, Investigation, Visualization, Writing – original draft. IB: Conceptualization, Data curation, Formal analysis, Funding acquisition, Investigation, Methodology, Project–administration, Resources, Software, Supervision, Validation, Visualization, Writing – review & editing.
The author(s) declare financial support was received for the research, authorship, and/or publication of this article. This work was supported by R00 AG047334 (IB) and R21 AG054804 (IB) from the National Institutes of Health/National Institute on Aging.
The authors wish to thank Justino Flores for his role in data collection.
The authors declare that the research was conducted in the absence of any commercial or financial relationships that could be construed as a potential conflict of interest.
All claims expressed in this article are solely those of the authors and do not necessarily represent those of their affiliated organizations, or those of the publisher, the editors and the reviewers. Any product that may be evaluated in this article, or claim that may be made by its manufacturer, is not guaranteed or endorsed by the publisher.
The Supplementary material for this article can be found online at: https://www.frontiersin.org/articles/10.3389/fnagi.2023.1285375/full#supplementary-material
Afzali, M., Pieciak, T., Newman, S., Garyfallidis, E., Özarslan, E., Cheng, H., et al. (2021). The sensitivity of diffusion MRI to microstructural properties and experimental factors. J. Neurosci. Methods 347:108951. doi: 10.1016/j.jneumeth.2020.108951
Beaulieu, C. (2002). The basis of anisotropic water diffusion in the nervous system - a technical review. NMR Biomed. 15, 435–455. doi: 10.1002/nbm.782
Bender, A. R., Daugherty, A. M., and Raz, N. (2013). Vascular risk moderates associations between hippocampal subfield volumes and memory. J. Cogn. Neurosci. 25, 1851–1862. doi: 10.1162/jocn_a_00435
Bennett, I. J., Stark, S. M., and Stark, C. E. L. (2019). Recognition memory dysfunction relates to hippocampal subfield volume: a study of cognitively normal and mildly impaired older adults. J. Gerontol. B Psychol. Sci. Soc. Sci. 74, 1132–1141. doi: 10.1093/geronb/gbx181
Bruno, D., Grothe, M. J., Nierenberg, J., Sidtis, J. J., Teipel, S. J., and Pomara, N. (2016). Output order and variability in free recall are linked to cognitive ability and hippocampal volume in elderly individuals. Neuropsychologia 80, 126–132. doi: 10.1016/j.neuropsychologia.2015.11.014
Cox, R. W., and Hyde, J. S. (1997). Software tools for analysis and visualization of fMRI data. NMR Biomed. 10, 171–178. doi: 10.1002/(SICI)1099-1492(199706/08)10:4/5<171::AID-NBM453>3.0.CO;2-L
Craik, F. I. M. (1994). Memory changes in normal aging. Curr. Dir. Psychol. Sci. 3, 155–158. doi: 10.1111/1467-8721.ep10770653
Craik, F. I. M., and McDowd, J. M. (1987). Age differences in recall and recognition. J. Exp. Psychol. Learn. Mem. Cogn. 13, 474–479. doi: 10.1037/0278-7393.13.3.474
De Leon, M. J., George, A. E., Golomb, J., Tarshish, C., Convit, A., Kluger, A., et al. (1997). Frequency of hippocampal formation atrophy in normal aging and Alzheimer’s disease. Neurobiol. Aging 18, 1–11. doi: 10.1016/S0197-4580(96)00213-8
den Heijer, T., der Lijn, F., Vernooij, M. W., de Groot, M., Koudstaal, P. J., der Lugt, A., et al. (2012). Structural and diffusion MRI measures of the hippocampus and memory performance. NeuroImage 63, 1782–1789. doi: 10.1016/j.neuroimage.2012.08.067
Du, A. T., Schuff, N., Chao, L. L., Kornak, J., Jagust, W. J., Kramer, J. H., et al. (2006). Age effects on atrophy rates of entorhinal cortex and hippocampus. Neurobiol. Aging 27, 733–740. doi: 10.1016/j.neurobiolaging.2005.03.021
Emmenegger, T. M., David, G., Ashtarayeh, M., Fritz, F. J., Ellerbrock, I., Helms, G., et al. (2021). The influence of radio-frequency transmit field inhomogeneities on the accuracy of g-ratio weighted imaging. Front. Neurosci. 15:674719. doi: 10.3389/fnins.2021.674719
Folstein, M. F., Folstein, S. E., and McHugh, P. R. (1975). “Mini-mental state”. A practical method for grading the cognitive state of patients for the clinician. J. Psychiatr. Res. 12, 189–198. doi: 10.1016/0022-3956(75)90026-6
Foster, C. M., Kennedy, K. M., Hoagey, D. A., and Rodrigue, K. M. (2019). The role of hippocampal subfield volume and fornix microstructure in episodic memory across the lifespan. Hippocampus 29, 1206–1223. doi: 10.1002/hipo.23133
Franco, C. Y., Petok, J. R., Langley, J., Hu, X., and Bennett, I. J. (2021). Implicit associative learning relates to basal ganglia gray matter microstructure in young and older adults. Behav. Brain Res. 397:112950. doi: 10.1016/j.bbr.2020.112950
Golomb, J., Kluger, A., de Leon, M. J., Ferris, S. H., Convit, A., Mittelman, M. S., et al. (1994). Hippocampal formation size in normal human aging: a correlate of delayed secondary memory performance. Learn Mem 1, 45–54. doi: 10.1101/lm.1.1.45
Gorbach, T., Pudas, S., Lundquist, A., Orädd, G., Josefsson, M., Salami, A., et al. (2017). Longitudinal association between hippocampus atrophy and episodic-memory decline. Neurobiol. Aging 51, 167–176. doi: 10.1016/j.neurobiolaging.2016.12.002
Guerrero, J. M., Adluru, N., Bendlin, B. B., Goldsmith, H. H., Schaefer, S. M., Davidson, R. J., et al. (2019). Optimizing the intrinsic parallel diffusivity in NODDI: an extensive empirical evaluation. PLoS One 14:e0217118. doi: 10.1371/journal.pone.0217118
Hayek, D., Thams, F., Flöel, A., and Antonenko, D. (2020). Dentate gyrus volume mediates the effect of fornix microstructure on memory formation in older adults. Front. Aging Neurosci. 12, 1–11. doi: 10.3389/fnagi.2020.00079
Hu, L., and Bentler, P. M. (1999). Cutoff criteria for fit indexes in covariance structure analysis: conventional criteria versus new alternatives. Struct. Equ. Model. Multidiscip. J. 6, 1–55. doi: 10.1080/10705519909540118
Jack, C. R., Twomey, C. K., Zinsmeister, A. R., Sharbrough, F. W., Petersen, R. C., and Cascino, G. D. (1989). Anterior temporal lobes and hippocampal formations: normative volumetric measurements from MR images in young adults. Radiology 172, 549–554. doi: 10.1148/radiology.172.2.2748838
James, L. R., and Brett, J. M. (1984). Mediators, moderators, and tests for mediation. J. Appl. Psychol. 69, 307–321. doi: 10.1037/0021-9010.69.2.307
Jenkinson, M., Beckmann, C. F., Behrens, T. E. J., Woolrich, M. W., and Smith, S. M. (2012). FSL. Neuroimage 62, 782–790. doi: 10.1016/j.neuroimage.2011.09.015
Köhncke, Y., Düzel, S., Sander, M. C., Lindenberger, U., Kühn, S., and Brandmaier, A. M. (2021). Hippocampal and parahippocampal gray matter structural integrity assessed by multimodal imaging is associated with episodic memory in old age. Cereb. Cortex 31, 1464–1477. doi: 10.1093/cercor/bhaa287
Leal, S. L., and Yassa, M. A. (2015). Neurocognitive aging and the Hippocampus across species. Trends Neurosci. 38, 800–812. doi: 10.1016/j.tins.2015.10.003
Lindenberger, U., von Oertzen, T., Ghisletta, P., and Hertzog, C. (2011). Cross-sectional age variance extraction: What’s change got to do with it? Psychol. Aging 26, 34–47. doi: 10.1037/a0020525
Lye, T. C., Piguet, O., Grayson, D. A., Creasey, H., Ridley, L. J., Bennett, H. P., et al. (2004). Hippocampal size and memory function in the ninth and tenth decades of life: the Sydney older persons study. J. Neurol. Neurosurg. Psychiatry 75, 548–554. doi: 10.1136/jnnp.2003.010223
Metzler-Baddeley, C., Mole, J. P., Sims, R., Fasano, F., Evans, J., Jones, D. K., et al. (2019). Fornix white matter glia damage causes hippocampal gray matter damage during age-dependent limbic decline. Sci. Rep. 9:1060. doi: 10.1038/s41598-018-37658-5
Nazeri, X., Chakravart, M., Rotenberg, D. J., Rajji, T. K., Rathi, X., Michailovich, O. V., et al. (2015). Functional consequences of neurite orientation dispersion and density in humans across the adult lifespan. J. Neurosci. 35, 1753–1762. doi: 10.1523/JNEUROSCI.3979-14.2015
Nobis, L., Manohar, S. G., Smith, S. M., Alfaro-Almagro, F., Jenkinson, M., Mackay, C. E., et al. (2019). Hippocampal volume across age: nomograms derived from over 19,700 people in UK biobank. Neuroimage Clin. 23:101904. doi: 10.1016/j.nicl.2019.101904
Nyberg, L., Bäckman, L., Erngrund, K., Olofsson, U., and Nilsson, L. G. (1996). Age differences in episodic memory, semantic memory, and priming: relationships to demographic, intellectual, and biological factors. J. Gerontol. B Psychol. Sci. Soc. Sci. 51:P234-40. doi: 10.1093/geronb/51B.4.P234
Orrison, W., Lewine, J., Sanders, J., and Hartshorne, M. (1995). Functional brain imaging. St Louis, MO: Mosby-Year Book, Inc.
Parker, E. S., Landau, S. M., Whipple, S. C., and Schwartz, B. L. (2004). Aging, recall and recognition: a study on the sensitivity of the University of Southern California Repeatable Episodic memory test (USC-REMT). J. Clin. Exp. Neuropsychol. 26, 428–440. doi: 10.1080/13803390490510130
Patenaude, B., Smith, S. M., Kennedy, D. N., and Jenkinson, M. (2011). A Bayesian model of shape and appearance for subcortical brain segmentation. NeuroImage 56, 907–922. doi: 10.1016/j.neuroimage.2011.02.046
Pendlebury, S. T., Welch, S. J. V., Cuthbertson, F. C., Mariz, J., Mehta, Z., and Rothwell, P. M. (2013). Telephone assessment of cognition after transient ischemic attack and stroke. Stroke 44, 227–229. doi: 10.1161/strokeaha.112.673384
Radhakrishnan, H., Bennett, I. J., and Stark, C. E. L. (2022). Higher-order multi-shell diffusion measures complement tensor metrics and volume in gray matter when predicting age and cognition. NeuroImage 253:119063. doi: 10.1016/j.neuroimage.2022.119063
Radhakrishnan, H., Stark, S. M., and Stark, C. E. L. (2020). Microstructural alterations in hippocampal subfields mediate age-related memory decline in humans. Front. Aging Neurosci. 12:94. doi: 10.3389/fnagi.2020.00094
Raykov, T., and Marcoulides, G. A. (2006). “A first course in structural equation modeling” in A first course in structural equation modeling. (2nd ed.). Mahwah, NJ: Lawrence Erlbaum Associates.
Raz, N., Gunning-Dixon, F. M., Head, D., Dupuis, J. H., and Acker, J. D. (1998). Neuroanatomical correlates of cognitive aging: evidence from structural magnetic resonance imaging. Neuropsychology 12, 95–114. doi: 10.1037//0894-4105.12.1.95
Raz, N., Lindenberger, U., Rodrigue, K. M., Kennedy, K. M., Head, D., Williamson, A., et al. (2005). Regional brain changes in aging healthy adults: general trends, individual differences and modifiers. Cereb. Cortex 15, 1676–1689. doi: 10.1093/cercor/bhi044
Rey, A. (1941). L’examen psychologique dans les cas d’encéphalopathie traumatique. Arch. Psychol. 28, 215–285.
Rhodes, S., Greene, N. R., and Naveh-Benjamin, M. (2019). Age-related differences in recall and recognition: a meta-analysis. Psychon. Bull. Rev. 26, 1529–1547. doi: 10.3758/s13423-019-01649-y
Rodrigue, K. M., and Raz, N. (2004). Shrinkage of the entorhinal cortex over five years predicts memory performance in healthy adults. J. Neurosci. 24, 956–963. doi: 10.1523/JNEUROSCI.4166-03.2004
Rönnlund, M., Nyberg, L., Bäckman, L., and Nilsson, L. G. (2005). Stability, growth, and decline in adult life span development of declarative memory: cross-sectional and longitudinal data from a population-based study. Psychol. Aging 20, 3–18. doi: 10.1037/0882-7974.20.1.3
Shing, Y. L., Rodrigue, K. M., Kennedy, K. M., Fandakova, Y., Bodammer, N., Werkle-Bergner, M., et al. (2011). Hippocampal subfield volumes: age, vascular risk, and correlation with associative memory. Front. Aging Neurosci. 3, 1–8. doi: 10.3389/fnagi.2011.00002
Sullivan, E. V., Marsh, L., Mathalon, D. H., Lim, K. O., and Pfefferbaum, A. (1995). Age-related decline in MRI volumes of temporal lobe gray matter but not hippocampus. Neurobiol. Aging 16, 591–606. doi: 10.1016/0197-4580(95)00074-O
Tariq, M., Schneider, T., Alexander, D. C., Gandini Wheeler-Kingshott, C. A., and Zhang, H. (2016). Bingham-NODDI: mapping anisotropic orientation dispersion of neurites using diffusion MRI. NeuroImage 133, 207–223. doi: 10.1016/j.neuroimage.2016.01.046
Tisserand, D. J., Visser, P. J., Van Boxtel, M. P. J., and Jolles, J. (2000). The relation between global and limbic brain volumes on MRI and cognitive performance in healthy individuals across the age range. Neurobiol. Aging 21, 569–576. doi: 10.1016/S0197-4580(00)00133-0
Van Petten, C. (2004). Relationship between hippocampal volume and memory ability in healthy individuals across the lifespan: review and meta-analysis. Neuropsychologia 42, 1394–1413. doi: 10.1016/j.neuropsychologia.2004.04.006
Venkatesh, A., Daugherty, A. M., and Bennett, I. J. (2021). Neuroimaging measures of iron and gliosis explain memory performance in aging. Hum. Brain Mapp. 42, 5761–5770. doi: 10.1002/hbm.25652
Venkatesh, A., Stark, S. M., Stark, C. E. L., and Bennett, I. J. (2020). Age- and memory- related differences in hippocampal gray matter integrity are better captured by NODDI compared to single-tensor diffusion imaging. Neurobiol. Aging 96, 12–21. doi: 10.1016/j.neurobiolaging.2020.08.004
Walhovd, K. B., Fjell, A. M., Reinvang, I., Lundervold, A., Dale, A. M., Eilertsen, D. E., et al. (2005). Effects of age on volumes of cortex, white matter and subcortical structures. Neurobiol. Aging 26, 1261–1270. doi: 10.1016/j.neurobiolaging.2005.05.020
Wolf, D., Fischer, F. U., de Flores, R., Chételat, G., and Fellgiebel, A. (2015). Differential associations of age with volume and microstructure of hippocampal subfields in healthy older adults. Hum. Brain Mapp. 36, 3819–3831. doi: 10.1002/hbm.22880
Keywords: hippocampus, diffusion, NODDI, volume, episodic memory, aging
Citation: Ibrahim K and Bennett IJ (2023) Hippocampal microstructure, but not macrostructure, mediates age differences in episodic memory. Front. Aging Neurosci. 15:1285375. doi: 10.3389/fnagi.2023.1285375
Received: 29 August 2023; Accepted: 07 November 2023;
Published: 20 November 2023.
Edited by:
Brice Alan Kuhl, University of Oregon, United StatesReviewed by:
Christine Coughlin, University of Illinois Chicago, United StatesCopyright © 2023 Ibrahim and Bennett. This is an open-access article distributed under the terms of the Creative Commons Attribution License (CC BY). The use, distribution or reproduction in other forums is permitted, provided the original author(s) and the copyright owner(s) are credited and that the original publication in this journal is cited, in accordance with accepted academic practice. No use, distribution or reproduction is permitted which does not comply with these terms.
*Correspondence: Ilana J. Bennett, aWxhbmFiQHVjci5lZHU=
Disclaimer: All claims expressed in this article are solely those of the authors and do not necessarily represent those of their affiliated organizations, or those of the publisher, the editors and the reviewers. Any product that may be evaluated in this article or claim that may be made by its manufacturer is not guaranteed or endorsed by the publisher.
Research integrity at Frontiers
Learn more about the work of our research integrity team to safeguard the quality of each article we publish.