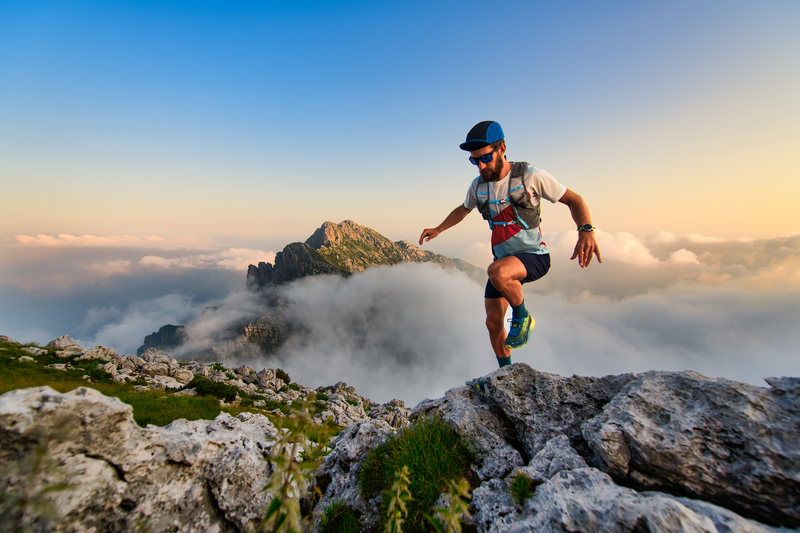
95% of researchers rate our articles as excellent or good
Learn more about the work of our research integrity team to safeguard the quality of each article we publish.
Find out more
ORIGINAL RESEARCH article
Front. Aging Neurosci. , 12 October 2023
Sec. Alzheimer's Disease and Related Dementias
Volume 15 - 2023 | https://doi.org/10.3389/fnagi.2023.1278998
Amyloid-beta (Aβ) is a pathological hallmark of Alzheimer’s disease (AD). We aimed to identify genes related to Aβ uptake in the Korean population and investigate the effects of these novel genes on clinical outcomes, including neurodegeneration and cognitive impairments. We recruited a total of 759 Korean participants who underwent neuropsychological tests, brain magnetic resonance imaging, 18F-flutemetamol positron emission tomography, and microarray genotyping data. We performed gene-based association analysis, and also performed expression quantitative trait loci and network analysis. In genome-wide association studies, no single nucleotide polymorphism (SNP) passed the genome-wide significance threshold. In gene-based association analysis, six genes (LCMT1, SCRN2, LRRC46, MRPL10, SP6, and OSBPL7) were significantly associated with Aβ standardised uptake value ratio in the brain. The three most significant SNPs (rs4787307, rs9903904, and rs11079797) on these genes are associated with the regulation of the LCMT1, OSBPL7, and SCRN2 genes, respectively. These SNPs are involved in decreasing hippocampal volume and cognitive scores by mediating Aβ uptake. The 19 enriched gene sets identified by pathway analysis included axon and chemokine activity. Our findings suggest novel susceptibility genes associated with the uptake of Aβ, which in turn leads to worse clinical outcomes. Our findings might lead to the discovery of new AD treatment targets.
Aging population is growing worldwide. By 2050, the world’s population with age over 65 is anticipated to increase to approximately 1.5 billion (United Nations Department of Economic and Social Affairs, 2021). WHO reported in 2012 that about 150 million people would be impacted by dementia by 2050 (Patterson, 2018), which would subsequently increase the total costs of Alzheimer’s disease (AD), the most common cause of dementia, to over $1 trillion (Prince et al., 2015). In South Korea, the number of patients with dementia in 2021 was estimated at 910,726 and the prevalence rate over 86 years old was estimated at 36.66%, but it is anticipated to exceed 3 million by 2050 (Ministry of Health and Welfare, 2020). Therefore, early diagnosis and intervention are critical to reduce all the burdens that dementia causes.
AD is caused by the accumulation of β-amyloid (Aβ) plaques and neurofibrillary tangles, with subsequent neurodegeneration and cognitive decline (Serrano-Pozo et al., 2011). The heritability of AD is reported to be approximately 60–80% (Gatz et al., 2006). AD also has a complex genetic aetiology. In addition to apolipoprotein E (APOE), a well-known risk factor for AD, previous large-scale genome-wide association studies (GWAS) have identified more than 20 genes for late-onset AD (LOAD) (Jun, 2010; Lambert et al., 2013; Wang et al., 2016). However, since their discovery, AD-related genetic variants could account for only about 30% of the clinical diagnosis (phenotype) of AD (Adams et al., 2016). Recent studies have focused on AD endophenotypes, including Aβ and neurodegeneration markers. This approach related to endophenotypes may help identify additional AD-related genetic variants (Potkin et al., 2009; Ramanan et al., 2015; Maxwell et al., 2018; Elsheikh et al., 2020; Homann et al., 2022).
With the development of Aβ positron emission tomography (PET), there is increasing evidence showing the relationships between Aβ uptake and genetic variations (Ramanan et al., 2014, 2015; Li et al., 2015; Apostolova et al., 2018; Raghavan et al., 2020; Kim H. R. et al., 2021). Previous studies based on non-Hispanic whites (NHWs) identified novel genetic variants associated with Aβ uptake in the AD brain (Ramanan et al., 2014, 2015; Li et al., 2015; Apostolova et al., 2018; Raghavan et al., 2020). The latest studies have reported differences in the frequency of Aβ positivity according to various ethnicities (Deters et al., 2021; Kim J. et al., 2021; Wilkins et al., 2022); compared to NHWs, African Americans and Asians had a lower frequency of Aβ positivity. As a result, investigations on the relationships between Aβ uptake and genetic variations in different populations are necessary. However, it is difficult to examine the previously identify genetic variants in Asians, and genetic association studies related to Asians are still insufficient.
Recent genetic association studies have increased our understanding of the underlying genetic architecture of AD. However, identified genetic factors only account for a small fraction of heritability (Maher, 2008; Manolio et al., 2009; Adams et al., 2016). Missing heritability results from the lower explanation power of each causal variant that did not meet the GWAS’s strict statistical threshold or/and the incomplete linkage disequilibrium (LD) between causal and genotyped variants (Yang et al., 2010). However, the gene-based analysis considers genes as units of association, and this analysis may be a valuable complement to GWAS. This analysis may be useful in identifying novel genes associated with traits by reducing multiple comparison corrections. Additionally, this analysis might be helpful to replicate findings, particularly for genetic variants with allelic heterogeneity between populations (Yang et al., 2010; Hong et al., 2012).
Because the ethnic differences in frequency of Aβ and genetic variants have been reported, more genetic studies trying to identify genetic variants associated with AD in the Asian population are needed. The objective of study is to identify novel genes associated with Aβ uptake in the Korean population. In the present study, we used Aβ uptake as an endophenotype of AD that lies along the pathway from genes to disease (Figure 1) and hypothesised that genes associated with Aβ uptake might affect the development of AD. The endophenotype conceptual analysis and gene-based analysis may help to increase statistical power and facilitate the biological interpretation of results. By adopting these strategies, we expected to identify novel associations between genes and Aβ uptake in the Korean population. Firstly, we performed genetic association analysis on a Korean population-based AD sample. Secondly, we also conducted expression quantitative trait loci (eQTL) analysis to identify the functional role of genetic variants. Furthermore, we investigated the relationships between these SNPs and clinical outcomes. Finally, we performed pathway enrichment and network analysis with gene expression levels in the brain tissue to identify potential AD-related genes.
Figure 1. A conceptual diagram of endophenotype conceptual analysis envisioned by Gottesman and Gould (2003). The possible relationship between gene, endophenotype, and disease. Endophenotypes lies along the pathway from gene to disease.
The 759 participants were enrolled from the Korea-Registries to Overcome and Accelerate Dementia research project (K-ROAD). The K-ROAD aims to develop a genotype–phenotype cohort to accelerate the development of novel diagnostic and therapeutic techniques for Alzheimer’s and concomitant cerebrovascular diseases. Nationwide, 25 university-affiliated hospitals in South Korea are participating in the K-ROAD. All participants underwent neuropsychological tests, brain magnetic resonance imaging (MRI), Aβ PET (18F-flutemetamol (FMM)), APOE genotyping, and microarray genotyping data. The 759 participants consist of Alzheimer’s disease (AD; n = 246), amnestic mild cognitive impairment (aMCI; n = 255), and cognitively unimpaired (CU) individuals (n = 258). All participants with CU met the following criteria: (1) no medical history that was likely to affect cognitive function based on Christensen’s health screening criteria; (2) no objective cognitive impairment in any cognitive domain on a comprehensive neuropsychological test battery (at least −1.0 SD above age-adjusted norms on any cognitive test); and (3) independence in daily living activities. All participants with MCI met the criteria for MCI with the following modifications (Albert et al., 2011); (1) subjective cognitive complaints by the participants or caregivers; (2) objective memory impairment below −1·0 SD on verbal or visual memory tests; (3) no significant impairment in daily living activities; and (4) non-demented status. Participants with dementia met the core clinical criteria of probable AD dementia proposed by the National Institute on Aging-Alzheimer’s Association (NIA-AA; McKhann et al., 2011).
Participants with significant white matter hyperintensities (cap or band >10 mm and longest diameter of deep white matter lesion >25 mm), structural lesions, including cerebral infarction, intracranial haemorrhage, brain tumours, and hydrocephalus on MRI, and abnormal laboratory results on complete blood count, electrolyte, vitamin B12, and folate levels, syphilis serology, and liver, kidney, or thyroid function tests were excluded from the study.
The institutional review board of the Samsung Medical Center approved this study. Written informed consent was obtained from all participants.
Genotyping was performed using the Illumina Asian Screening Array BeadChip (Illumina, CA, United States). The quality control (QC) procedures were conducted using PLINK software, and samples that did not satisfy the following criteria were excluded: call rate < 95%, sex-mismatch, excess heterozygosity rate (5 standard deviation from the mean), and identify-by-descent ≥0.125. Additionally, individual markers with the following criteria were excluded: call rate < 98%, minor allele frequency (MAF) < 1%, Hardy–Weinberg equilibrium (HWE) p < 10−6. Following QC, un-genotyped markers were imputed using Minimac4 and reference haplotypes from HRC-r1.1 on the University of Michigan Imputation Server (Das et al., 2016). Furthermore, post-imputation QC was performed using the following criteria: poor imputation, r2 ≤ 0.8 and MAF < 1%. Finally, bi-allelic 4,906,407 SNPs in autosomal chromosomes (sex chromosome, mitochondrial, and pseudo autosomal SNPs were excluded) were used for the following analyses.
All participants underwent Aβ PET (18F-flutemetamol PET) scans using a Discovery STe PET/CT scanner (GE Medical Systems, Milwaukee, WI, United States). For 18F-flutemetamol PET, a 20-min emission PET scan in dynamic mode (consisting of 4 × 5 min frames) was performed 90 min after an injection of a mean dose of 311.5 MBq 18F-florbetaben or 197.7 MBq 18F-flutemetamol. Three-dimensional PET images were reconstructed in a 128 × 128 × 48 matrix with 2 mm × 2 mm × 3·27 mm voxel size using the ordered-subsets expectation maximisation algorithm (iteration = 4 and subset = 20).
For image processing, we used SPM81 running on MATLAB.2 T1-weighted images were corrected for nonuniformity (Sled et al., 1998) and normalised to standard space using a linear transformation. PET images were co-registered, and a Gaussian kernel with an 8 mm full width at half maximum was used for spatial smoothing. Using the whole cerebellum as a reference region, the standardised uptake value ratio (SUVR) images were calculated. The mask of the reference region was obtained from the Global Alzheimer’s Association Information Network (GAAIN) websites3 (Klunk et al., 2015). The mean SUVR of cerebral cortical areas was determined using the masks of the standard cortical target region (Klunk et al., 2015) downloaded from GAAIN.
We acquired standardised three-dimensional T1 Turbo Field Echo and three-dimensional fluid-attenuated inversion recovery (FLAIR) images using a 3.0 T MRI scanner (Philips 3.0 T Achieva; Philips Healthcare, Andover, MA, United States), as previously described (Kang et al., 2021).
Images were processed using the CIVET anatomical pipeline (version 2.1.0). The native MRI images were registered to the MNI-152 template by a linear transformation and corrected for intensity nonuniformities using the N3 algorithm (Sled et al., 1998). We used an automated hippocampus segmentation method described in an earlier study that combined a graph cut algorithm with atlas-based segmentation and morphological opening to measure hippocampal volume (Kwak et al., 2013).
To assess the effect of genes on Aβ SUVR, a gene-based association analysis was performed by combining the SNP-based p-values from a GWAS, which was performed using the PLINK software (Purcell et al., 2007). The statistical analysis was performed on 759 participants from three diagnostic groups (CU, aMCI, and AD) and allelic effects for the Aβ SUVR were measured using an additive model with age, sex, and APOE ε4 counts as covariates. Gene-based association analysis was conducted using the extended Simes procedure (GATES; Li et al., 2011). SNPs that mapped within 20 kb of the 3′ and 5′ untranslated regions were considered “within” the genes. The linkage disequilibrium (LD) was calculated using data for East Asian ancestry from the 1,000 Genomes Project, and SNPs with r2 < 0.005 were ignored in gene annotation.
We performed an eQTL analysis to determine the functional effect of genetic variants on gene expression. Genotype data and exon-specific expression in the hippocampus, frontal, and temporal cortex were downloaded from the Braineac database4 (Ramasamy et al., 2014). The eQTL analysis was performed with the most significant SNPs mapped to identified genes from gene-based association analysis. Multiple comparison correction for the number of tissues was performed, and eQTLs with q < 0.05 were considered significant.
We hypothesised that the identified genetic variants were related to biomarkers that indicate the severity of disease symptoms. The effects of the most significant SNPs mapped to identified genes on cognitive scores [Mini-Mental State Exam (MMSE) and Clinical Dementia Rating Sum of Boxes (CDR-SB)] and hippocampal volume were investigated. After applying inverse normal transformations to cognitive scores for data normality, associations were tested, and age, sex, education, and ICV volume were used as covariates, if appropriate. In addition, the mediation package in R was used for mediation analysis in order to identify the SUVR-mediated pathway from SNP to AD biomarkers (Imai et al., 2010). The genotypes were defined as independent variables, the SUVR as a mediator variable, and the clinical outcomes as continuous dependent variables. The mediate() function generated the direct and indirect effects as well as paths from the mediator variable to dependent variables by linear regression.
We performed pathway analysis using GSA-SNP (Nam et al., 2010) to identify functional gene sets associated with Aβ SUVR. The pathway annotations from the Gene Ontology (GO) resource5 were downloaded, and gene sets containing 5–200 genes were used for analysis. The summary statistics of GWAS were used, and SNPs that fell within 20 kb of the boundary of a gene were annotated to the gene on the human genome (hg19) coordinate. Gene sets were evaluated by Z-statistics for the identification of enriched gene sets, with gene sets having q < 0.05 considered significant.
We generated a protein–protein interaction network using the WEB-based gene set analysis toolkit (Liao et al., 2019) with the human PPI data from the Biological General Repository for Interaction Datasets. For the seed genes identified in gene-based association analysis, random walk analysis was performed to expand the network. The network was expanded by ranking genes based on their network proximity with the seed genes, and the resulting network consisted of the seed genes and the top 50 neighbouring genes. Functional module detection was performed using HumanBase (Greene et al., 2015) to find the functional gene cluster in human brain tissue. Genes were clustered into the functional modules with tissue-specific networks based on the shared k-nearest nearest-neighbours (SKNN) and the Louvain community-finding algorithm. Functional enrichment analysis was performed for the resulting functional modules using Go terms. Statistical significance was tested by a one-sided Fisher’s exact test and Go terms with q < 0.05 were considered significant.
The demographics and genotypic characteristics of the final samples are listed in Table 1. The age (mean [±standard deviation]) of the participants was 70.6 (±8.5) years; the proportions of female and Aβ + participants were 58.6 and 49.4%, respectively. The proportions of apolipoprotein ε4 carriers were 38.1%.
The considerable association of APOE4 with Aβ SUVR led us to conduct GWAS with APOE ε4 counts as a covariate, where no SNP passed the genome-wide significance threshold of 5 × 10−8 (Supplementary Figure S1). Ten SNPs on chromosomes 13, 16, 17, and 21 showed genome-wide suggestive significance (p < 1 × 10−6) and there is no genomic inflation (λ = 1.0).
In gene-based association analysis, 3,936,415 SNPs mapped to 26,443 genes on the human genome. One gene on chromosome 16, LCMT1 (Leucine Carboxyl Methyltransferase 1), and five genes on chromosome 17, SCRN2 (Secernin 2), LRRC46 (Leucine Rich Repeat Containing 46), MRPL10 (Mitochondrial Ribosomal Protein L10), SP6 (Sp6 transcription factor), and OSBPL7 (Oxysterol Binding Protein Like 7), were significantly associated with the Aβ SUVR (Figure 2). SCRN2 on chromosome 17 showed the strongest associations with Aβ SUVR (q = 2.33 × 10−2). Four additional genes, LRRC46, MRPL10, SP6, and OSBPL7, close to SCRN2, were found to be related to Aβ SUVR (p = 2.46 × 10−6, p = 2.64 × 10−6, p = 5.39 × 10−6, p = 6.71 × 10−6, respectively). Furthermore, the LCMT1 gene on chromosome 16 was observed to be significantly related to Aβ SUVR (p = 5.90 × 10−6).
Figure 2. Manhattan plot of gene-based association analysis. The horizontal axis (x-axis) shows the start position of genes on chromosomes, and the vertical axis (y-axis) shows the observed −log10 (value of p). The red horizontal line indicates a genome-wide significant threshold (value of p <1 × 10−5, which approximately corresponds to a threshold of the false discovery rate (FDR) corrected value of p <0.05). Genes with FDR-corrected value of p < 0.05 are coloured in red.
The genetic effects of the most significant SNPs mapped to the identified six genes are shown in Figures 3A–C. The rs4787307 on the LCMT1 gene has a risk effect on Aβ SUVR (β = 0.258, p = 3.91 × 10−7), which means that participants with minor alleles have a higher Aβ SUVR than those without minor alleles. The rs9903904 mapped to SCRN2, LRRC46, and SP6 genes, and the rs11079797 mapped to MRPL10 and OSBPL7 also have risk effects (β = 0.655, p = 9.73 × 10−8 and β = 0.623, p = 1.14 × 10−7, respectively).
Figure 3. Effects of the most significant SNPs on Aβ SUVR and gene expression. Regional association plots for Aβ standardised uptake value ratio (SUVR) (A–C). All single nucleotide polymorphisms (SNPs) within 200 kb upstream and downstream of rs4787307 on chromosome 16 (A), rs11079797 (B), and rs9903904 (C) on chromosome 17 are plotted based on their GWAS −log10 (value of ps). The most significant SNPs mapped to identified genes in gene-based association analysis are highlighted in violet. The colour scale of the squared correlation (r2) value is used to label SNPs based on their degree of linkage disequilibrium with the highlighted SNPs. Genes in the region are labelled with arrows denoting the 5′-3′ orientation. All plots are adapted from LocusZoom results. Expression quantitative trait loci (eQTL) plots of association between rs4787307 and the expression levels of LCMT1 (D), rs11079797 and MRPL10 (E) and OSBPL7 (F), and rs9903904 and SCRN2 (G). The x-axes are the genotype of SNP and y-axes are exon-specific gene expression obtained from United Kingdom brain expression consortium (UKBEC).
To identify the functional effect of genetic variants on gene expression, cis-eQTL analysis was performed. We performed eQTL analysis with three SNPs (rs4787307, rs9903904, and rs11079797) as well as the expression levels of the mapped genes in the frontal and temporal cortex and hippocampus (Figures 3D–G). Our findings indicated that rs4787307 on chromosome 16 significantly regulates the expression of LCMT1 in the temporal cortex (q = 4.20 × 10−2), while rs11079797 on chromosome 17 significantly regulates the expression of MRPL10 (q = 6.90 × 10−3) and OSBPL7 (q = 4.80 × 10−2) in the frontal cortex and hippocampus. In addition, rs9903904 on chromosome 17 influences the expression of SCRN2 in the hippocampus (q = 4.80 × 10−2).
We tested the association between the most significant SNPs on six genes and cognitive scores and hippocampal volume (Table 2). The association tests are shown in Table 2. The rs4787307 in LCMT1 was significantly associated with CDR-SB (β = 0.129, p = 2.25 × 10−2), left (β = −93.19, p = 6.39 × 10−3) and right hippocampal volume decline (β = −106.51, p = 1.00 × 10−2). The rs11079797 in MRPL10 and OSBPL7 was significantly associated with MMSE (β = −0.372, p = 2.61 × 10−3), left (β = −181.2, p = 1.90 × 10−2) and right hippocampal volume decline (β = −164.56, p = 3.83 × 10−2). In addition, rs9903904 in SCRN2, LRRC46, and SP6 was significantly associated with MMSE (β = −0.453, p = 4.74 × 10−4), CDR-SB (β = 0.321, p = 1.48 × 10−2), left (β = −213.2, p = 8.72 × 10−3), and right hippocampal volume (β = −203.45, p = 2.34 × 10−2).
To identify the Aβ uptake-mediated pathways from SNP to clinical outcomes, we performed mediation analysis with SUVR as the mediator variable and clinical outcomes as dependent variables (Figure 4; Supplementary Table S1). Three SNPs had significant indirect effects when CDR-SB was set as the outcome (rs4787307, effect = 0.08, p < 0.001; rs11079797, effect = 0.21, p < 0.001; and rs9903904, effect = 0.22, p < 0.001; respectively), while only rs9903904 had a significant direct effect (effect = 0.1, p < 3.65 × 10−2). In addition, when left hippocampal volume was set as the outcome, we found significant indirect effects of three SNPs (rs4787307, effect = −60.54, p < 0.001; rs11079797, effect = −124.94, p < 0.001; rs9903904, effect = −130.63, p < 0.001; respectively), but the direct effects were not significant. The mediation results for MMSE and right hippocampal volume are presented in Supplementary Table S1. These findings indicate that the SNP could affect hippocampal volume and cognitive scores, primarily through the mediation of Aβ SUVR.
Figure 4. The diagram of mediation analyses for the relation of SNP with cognitive scores and hippocampal volume. The mediation implemented by Aβ standardised uptake value ratio (SUVR) from single nucleotide polymorphisms (SNPs) on Clinical Dementia Rating Sum of Boxes (CDR-SB) (A–C) and from SNP on left hippocampal volume (D–F). X, M, and Y indicate predictor, mediator, and outcome variables in mediation analyses, respectively. The direct and indirect effects are presented as coefficients (95% confidence interval) with a value of p.
To identify functional gene sets associated with Aβ SUVR, we performed pathway analysis using SNP p-values from GWAS. The 6,432 gene sets have been examined, and 19 gene sets were enriched for Aβ SUVR (Figure 5). Gene sets related to axon parts, cytokine, and chemokine activity were enriched. More detailed results and a list of genes contained in the gene set are presented in Supplementary Table S2.
Figure 5. Enriched pathways associated with Aβ SUVR. The y-axis is the name of gene sets from gene ontology (GO), the x-axis ‘count’ indicates the number of genes included in the gene sets, and the colours indicate the pathway analysis p-values from GSA-SNP2.
We constructed a network consisting of six seed genes identified in the gene-based association analysis and 50 top-ranking neighbour genes (Supplementary Figure S2). The 56 genes in the constructed PPI network were clustered into five functional modules in the cerebral cortex. Modules 1, 2, 3, 4, and 5 included 6, 10, 6, 8, and 10 genes, respectively (Supplementary Figure S3; Supplementary Table S3). In enrichment analysis, eight genes clustered into M1 were enriched for DNA repair, recombination-related processes, and dephosphorylation (Table 3). M2 and M4 genes were enriched for cell cycle-related processes, and M5 was enriched for the proteolysis-related process.
In the present study, we performed a genetic association study to identify novel genes associated with Aβ uptake in the brain. Our major findings are as follows. First, six genes, LCMT1, SCRN2, LRRC46, MRPL10, SP6, and OSBPL7, were associated with Aβ uptake independent of the APOE effects. Second, the most significant SNPs (rs4787307, rs9903904, and rs11079797) mapped to identified genes were predictive of neurodegeneration and cognitive outcomes with the mediation of Aβ uptake. Finally, the genes identified by pathway-based analysis and by combining network and functional enrichment analysis, are related to AD pathophysiology. Overall, our findings indicated that these six genes might be novel potential targets of AD.
Our conclusion that these identified genes are associated with Aβ uptake, which in turn leads to poor clinical outcomes, is supported by the following observations: (1) Six genes (LCMT1, SCRN2, LRRC46, MRPL10, SP6, and OSBPL7) were associated with Aβ uptake; (2) eQTL analysis revealed that the SNPs in these genes were associated with the regulation of genes; (3) these SNPs were predictive of neurodegeneration and cognitive impairments. Previously, studies reported that these genes were associated with AD pathophysiology. Particularly, LCMT1 increases PP2A activity, which in turn leads to decreased tau hyperphosphorylation in the AD (Torrent and Ferrer, 2012). A previous study also reported that microglial expression of LRRC46 in the CA1 region of the hippocampus was significantly different between the AD group and the control group (Mastroeni et al., 2018). Furthermore, the SCRN2, MRPL10, and OSBPL7 are related to proteolysis, mitochondrial translation, and cholesterol metabolism, respectively, which play important roles in AD pathogenesis (Bishop et al., 2010; Strooper, 2010; Swerdlow, 2011; Picard and McEwen, 2014; Xue et al., 2018). In fact, a previous study suggested that genetic variants in MRLP10 and OSBL7 are associated with cognitive decline in AD (Sherva et al., 2014). However, to our knowledge, there are no studies showing that these genes influence the pathophysiology of AD through increased Aβ uptake. Notably, in this study, Aβ uptake mediated the relationships between these SNPs and neurodegeneration or between these SNPs and cognitive outcomes. As a result, our findings might help achieve a better understanding of AD pathophysiology and help uncover novel therapeutic targets for AD.
The mechanisms by which these genetic variants are predictive of worse clinical outcomes remain to be elucidated. These findings, however, might be explained by our other findings from pathway-based analysis and the combination of network analysis and functional enrichment. The 19 gene sets identified through pathway-based analysis are associated with axon part, cytokine receptor activity, modulation of excitatory postsynaptic potential, and chemokine receptor activity, which is related to AD pathophysiology (Figure 4; Akiyama et al., 2000; Ardiles et al., 2012; Millecamps and Julien, 2013; Liu et al., 2014; Nagae and Araki, 2016; Sleigh et al., 2019). Additionally, enriched pathways in functional modules identified by a combination of network analysis and functional enrichment revealed that the genes consisting of networks were related to critical AD processes, such as DNA repair and recombination processes, cell cycle-related processes, and dephosphorylation (Table 3; Sheng et al., 1998; Iqbal et al., 2010; Shanbhag et al., 2019). Cell cycle deficits are associated with various neurogenerative disorders, including AD. Several genes and proteins, such as DKs and cyclins, have been linked to cell cycle dysregulation in AD (Yang et al., 2003; Hamdane and Buée, 2007; Zhang et al., 2012), and cell cycle proteins have been reported to be related to tau phosphorylation (Conejero-Goldberg et al., 2008; Seward et al., 2013; Kim et al., 2016).
The strength of our study is the recruitment of participants using a standardised diagnostic protocol, including detailed neuropsychological tests, Aβ PET, and brain MRI. However, the present study had some limitations. We identified novel genes related to AD using gene-based association analysis, but our sample size was moderate for a genetic association study. As a result, the replication of our findings in larger, independent datasets is needed. In addition, we used only the Korean population; further studies in a racially diverse sample are needed to generalise our findings. Nevertheless, the fact that so little research has been conducted on the Asian population makes our current study notable. We have identified genes associated with Aβ uptake whose involvement in Aβ uptake was not reported in previous European studies, highlighting the importance of genetic association studies in a diverse population.
In conclusion, by utilising gene-based association analysis, we identified new APOE-independent associations of six genes, LCMT1, SCRN2, LRRC46, MRPL10, SP6, and OSBPL7, with Aβ uptake in the Korean cohort. The SNPs on these genes were also related to decreased hippocampal volume and cognitive scores. Furthermore, we identified several functional gene sets that may contribute to AD through pathway enrichment and network analysis. Our findings may contribute to understanding the genetic architecture underlying Aβ uptake and its relationships with Alzheimer’s disease.
The original contributions presented in the study are publicly available. This data can be found here: https://www.ebi.ac.uk/eva/?eva-study=PRJEB66172.
All procedures performed in studies involving human participants were in accordance with the ethical standards of the institutional and/or national research committee and with the 1964 Helsinki Declaration and its later amendments or comparable ethical standards. Informed consent was obtained from all individual participants included in the study. The Institutional Review Board of Samsung Medical Center approved the study protocol, and all methods were performed according to the approved guidelines. The studies were conducted in accordance with the local legislation and institutional requirements. The participants provided their written informed consent to participate in this study.
B-HK: Conceptualization, Formal analysis, Methodology, Visualization, Writing – original draft. HL: Formal analysis, Methodology, Writing – original draft. HH: Formal analysis, Methodology, Writing – original draft. HK: Data curation, Writing – review & editing. HJ: Data curation, Writing – review & editing. JK: Data curation, Writing – review & editing. YP: Formal analysis, Methodology, Writing – original draft. MK: Conceptualization, Supervision, Writing – review & editing. SS: Conceptualization, Supervision, Writing – review & editing.
The author(s) declare financial support was received for the research, authorship, and/or publication of this article. This work was partly supported by Institute of Information and communications Technology Planning and Evaluation (IITP) grant funded by the Korea government (MSIT) (No. 2021-0-02068, Artificial Intelligence Innovation Hub), a grant of the Korea Dementia Research Project through the Korea Dementia Research Center (KDRC), funded by the Ministry of Health and Welfare and Ministry of Science and ICT, Republic of Korea (grant number: HU20C0111), a grant of the Korea Health Technology R&D Project through the Korea Health Industry Development Institute (KHIDI), funded by the Ministry of Health and Welfare and Ministry of science and ICT, Republic of Korea (grant number: HU22C0170), the National Research Foundation of Korea (NRF) grant funded by the Korea government (MSIT) (NRF-2019R1A5A2027340), Future Medicine 20*30 Project of the Samsung Medical Center [#SMX1230081], the “National Institute of Health” research project (2021-ER1006-02), the National Research Foundation of Korea under Grant NRF-2022R1F1A1068529.
The authors declare that the research was conducted in the absence of any commercial or financial relationships that could be construed as a potential conflict of interest.
All claims expressed in this article are solely those of the authors and do not necessarily represent those of their affiliated organizations, or those of the publisher, the editors and the reviewers. Any product that may be evaluated in this article, or claim that may be made by its manufacturer, is not guaranteed or endorsed by the publisher.
The Supplementary material for this article can be found online at: https://www.frontiersin.org/articles/10.3389/fnagi.2023.1278998/full#supplementary-material
Adams, P. M., Albert, M. S., Albin, R. L., Apostolova, L. G., Arnold, S. E., Asthana, S., et al. (2016). Assessment of the genetic variance of late-onset Alzheimer’s disease. Neurobiol. Aging 41, 200.e13–200.e20. doi: 10.1016/j.neurobiolaging.2016.02.024
Akiyama, H., Barger, S., Barnum, S., Bradt, B., Bauer, J., Cole, G. M., et al. (2000). Inflammation and Alzheimer’s disease. Neurobiol. Aging 21, 383–421. doi: 10.1016/S0197-4580(00)00124-X
Albert, M. S., DeKosky, S. T., Dickson, D., Dubois, B., Feldman, H. H., Fox, N. C., et al. (2011). The diagnosis of mild cognitive impairment due to Alzheimer’s disease: recommendations from the National Institute on Aging-Alzheimer’s association workgroups on diagnostic guidelines for Alzheimer’s disease. Alzheimers Dement. 7, 270–279. doi: 10.1016/j.jalz.2011.03.008
Apostolova, L. G., Risacher, S. L., Duran, T., Stage, E. C., Goukasian, N., West, J. D., et al. (2018). Associations of the top 20 Alzheimer disease risk variants with brain amyloidosis. JAMA Neurol. 75:328. doi: 10.1001/jamaneurol.2017.4198
Ardiles, Á. O., Tapia-Rojas, C. C., Mandal, M., Alexandre, F., Kirkwood, A., Inestrosa, N. C., et al. (2012). Postsynaptic dysfunction is associated with spatial and object recognition memory loss in a natural model of Alzheimer’s disease. Proc. Natl. Acad. Sci. U. S. A. 109, 13835–13840. doi: 10.1073/pnas.1201209109
Bishop, N. A., Lu, T., and Yankner, B. A. (2010). Neural mechanisms of ageing and cognitive decline. Nature 464, 529–535. doi: 10.1038/nature08983
Conejero-Goldberg, C., Townsend, K., and Davies, P. (2008). Effects of cell cycle inhibitors on tau phosphorylation in N2aTau3R cells. J. Mol. Neurosci. 35, 143–150. doi: 10.1007/s12031-008-9044-z
Das, S., Forer, L., Schönherr, S., Sidore, C., Locke, A. E., Kwong, A., et al. (2016). Next-generation genotype imputation service and methods. Nat. Genet. 48, 1284–1287. doi: 10.1038/ng.3656
Deters, K. D., Napolioni, V., Sperling, R. A., Greicius, M. D., Mayeux, R., Hohman, T., et al. (2021). Amyloid PET imaging in self-identified non-Hispanic black participants of the anti-amyloid in asymptomatic Alzheimer’s disease (A4) study. Neurology 96, e1491–e1500. doi: 10.1212/WNL.0000000000011599
Elsheikh, S. S. M., Chimusa, E. R., Mulder, N. J., and Crimi, A. (2020). Genome-wide association study of brain connectivity changes for Alzheimer’s disease. Sci. Rep. 10:1433. doi: 10.1038/s41598-020-58291-1
Gatz, M., Reynolds, C. A., Fratiglioni, L., Johansson, B., Mortimer, J. A., Berg, S., et al. (2006). Role of genes and environments for explaining Alzheimer’s disease. Arch. Gen. Psychiatry 63, 168–174. doi: 10.1001/archpsyc.63.2.168
Gottesman, I. L, and Gould, T. D. (2003). The endophenotype concept in psychiatry: Etymology and strategic intentions. Am. J. Psychiatry 160, 636–645. doi: 10.1176/appi.ajp.160.4.636
Greene, C. S., Krishnan, A., Wong, A. K., Ricciotti, E., Zelaya, R. A., Himmelstein, D. S., et al. (2015). Understanding multicellular function and disease with human tissue-specific networks. Nat. Genet. 47, 569–576. doi: 10.1038/ng.3259
Hamdane, M., and Buée, L. (2007). The complex p25/Cdk5 kinase in neurofibrillary degeneration and neuronal death: the missing link to cell cycle. Biotechnol. J. 2, 967–977. doi: 10.1002/biot.200700059
Homann, J., Osburg, T., Ohlei, O., Dobricic, V., Deecke, L., Bos, I., et al. (2022). Genome-wide association study of Alzheimer’s disease brain imaging biomarkers and neuropsychological phenotypes in the European medical information framework for Alzheimer’s disease multimodal biomarker discovery dataset. Front. Aging Neurosci 14:840651. doi: 10.3389/fnagi.2022.840651
Hong, M. G., Reynolds, C. A., Feldman, A. L., Kallin, M., Lambert, J. C., Amouyel, P., et al. (2012). Genome-wide and gene-based association implicates FRMD6 in alzheimer disease. Hum. Mutat. 33, 521–529. doi: 10.1002/humu.22009
Imai, K., Keele, L., and Yamamoto, T. (2010). Identification, inference and sensitivity analysis for causal mediation effects. Stat. Sci. 25, 17–21. doi: 10.1214/10-STS321
Iqbal, K., Liu, F., Gong, C.-X., and Grundke-Iqbal, I. (2010). Tau in Alzheimer disease and related tauopathies. Curr. Alzheimer Res. 7, 656–664. doi: 10.2174/156720510793611592
Jun, G. (2010). Meta-analysis confirms CR1, CLU, and PICALM as Alzheimer disease risk loci and reveals interactions with APOE genotypes. Arch. Neurol. 67, 1473–1484. doi: 10.1001/archneurol.2010.201
Kang, S. H., Kim, M. E., Jang, H., Kwon, H., Lee, H., Kim, H. J., et al. (2021). Amyloid positivity in the Alzheimer/subcortical-vascular Spectrum. Neurology 96, e2201–e2211. doi: 10.1212/WNL.0000000000011833
Kim, J., Jung, S., Choe, Y., Kim, S., Kim, B., Kim, H., et al. (2021). Ethnic differences in the frequency of β-amyloid deposition in cognitively normal individuals. Alzheimers Dement. 17:57488. doi: 10.1002/alz.057488
Kim, H. R., Jung, S. H., Kim, J., Jang, H., Kang, S. H., Hwangbo, S., et al. (2021). Identifying novel genetic variants for brain amyloid deposition: a genome-wide association study in the Korean population. Alzheimers Res. Ther. 13:117. doi: 10.1186/s13195-021-00854-z
Kim, H., Kwon, Y. A., Ahn, I. S., Kim, S., Kim, S., Jo, S. A., et al. (2016). Overexpression of cell cycle proteins of peripheral lymphocytes in patients with alzheimer’s disease. Psychiatry Investig. 13, 127–134. doi: 10.4306/pi.2016.13.1.127
Klunk, W. E., Koeppe, R. A., Price, J. C., Benzinger, T. L., Devous, M. D., Jagust, W. J., et al. (2015). The Centiloid project: standardizing quantitative amyloid plaque estimation by PET. Alzheimers Dement. 11, 1–15.e4. doi: 10.1016/j.jalz.2014.07.003
Kwak, K., Yoon, U., Lee, D. K., Kim, G. H., Seo, S. W., Na, D. L., et al. (2013). Fully-automated approach to hippocampus segmentation using a graph-cuts algorithm combined with atlas-based segmentation and morphological opening. Magn. Reson. Imaging 31, 1190–1196. doi: 10.1016/j.mri.2013.04.008
Lambert, J. C., Ibrahim-Verbaas, C. A., Harold, D., Naj, A. C., Sims, R., Bellenguez, C., et al. (2013). Meta-analysis of 74,046 individuals identifies 11 new susceptibility loci for Alzheimer’s disease. Nat. Genet. 45, 1452–1458. doi: 10.1038/ng.2802
Li, M. X., Gui, H. S., Kwan, J. S. H., and Sham, P. C. (2011). GATES: a rapid and powerful gene-based association test using extended Simes procedure. Am. J. Hum. Genet. 88, 283–293. doi: 10.1016/j.ajhg.2011.01.019
Li, J., Zhang, Q., Chen, F., Yan, J., Kim, S., Wang, L., et al. (2015). Genetic interactions explain variance in cingulate amyloid burden: An AV-45 PET genome-wide association and interaction study in the ADNI cohort. Biomed Res. Int 2015:647389. doi: 10.1155/2015/647389
Liao, Y., Wang, J., Jaehnig, E. J., Shi, Z., and Zhang, B. (2019). Web gestalt 2019: gene set analysis toolkit with revamped UIs and APIs. Nucleic Acids Res. 47, W199–W205. doi: 10.1093/nar/gkz401
Liu, C., Cui, G., Zhu, M., Kang, X., and Guo, H. (2014). Neuroinflammation in Alzheimer’s disease: Chemokines produced by astrocytes and chemokine receptors. Int. J. Clin. Exp. Pathol. 7, 8342–8355.
Manolio, T. A., Collins, F. S., Cox, N. J., Goldstein, D. B., Hindorff, L. A., Hunter, D. J., et al. (2009). Finding the missing heritability of complex diseases. Nature 461, 747–753. doi: 10.1038/nature08494
Mastroeni, D., Nolz, J., Sekar, S., Delvaux, E., Serrano, G., Cuyugan, L., et al. (2018). Laser-captured microglia in the Alzheimer’s and Parkinson’s brain reveal unique regional expression profiles and suggest a potential role for hepatitis B in the Alzheimer’s brain. Neurobiol. Aging 63, 12–21. doi: 10.1016/j.neurobiolaging.2017.10.019
Maxwell, T. J., Corcoran, C., Del-Aguila, J. L., Budde, J. P., Deming, Y., Cruchaga, C., et al. (2018). Genome-wide association study for variants that modulate relationships between cerebrospinal fluid amyloid-beta 42, tau, and p-tau levels. Alzheimers Res. Ther. 10:86. doi: 10.1186/s13195-018-0410-y
McKhann, G. M., Knopman, D. S., Chertkow, H., Hyman, B. T., Jack, C. R., Kawas, C. H., et al. (2011). The diagnosis of dementia due to Alzheimer’s disease: recommendations from the National Institute on Aging-Alzheimer’s association workgroups on diagnostic guidelines for Alzheimer’s disease. Alzheimers Dement. 7, 263–269. doi: 10.1016/j.jalz.2011.03.005
Millecamps, S., and Julien, J. P. (2013). Axonal transport deficits and neurodegenerative diseases. Nat. Rev. Neurosci. 14, 161–176. doi: 10.1038/nrn3380
Ministry of Health and Welfare (2020). The 4th National Dementia Plan. Sejong: Ministry of Health and Welfare
Nagae, T., and Araki, K. (2016). Cytokines and cytokine receptors involved in the pathogenesis of Alzheimer’s disease. J. Clin. Cell. Immunol. 7:441. doi: 10.4172/2155-9899.1000441
Nam, D., Kim, J., Kim, S. Y., and Kim, S. (2010). GSA-SNP: a general approach for gene set analysis of polymorphisms. Nucleic Acids Res. 38, W749–W754. doi: 10.1093/nar/gkq428
Patterson, C. (2018). World Alzheimer Report 2018. The state of the art of dementia research: new frontiers. London: Alzheimer’s Disease International.
Picard, M., and McEwen, B. S. (2014). Mitochondria impact brain function and cognition. Proc. Natl. Acad. Sci. U. S. A. 111, 7–8. doi: 10.1073/pnas.1321881111
Potkin, S. G., Guffanti, G., Lakatos, A., Turner, J. A., Kruggel, F., Fallon, J. H., et al. (2009). Hippocampal atrophy as a quantitative trait in a genome-wide association study identifying novel susceptibility genes for Alzheimer’s disease. PLoS One 4:e6501. doi: 10.1371/journal.pone.0006501
Prince, M., Wimo, A., Guerchet, M., Ali, G.-C., Wu, Y.-T., Prina, M., et al. (2015). World Alzheimer report 2015: the global impact of dementia: an analysis of prevalence, incidence, cost and trends. Alzheimer’s Dis. Int.
Purcell, S., Neale, B., Todd-Brown, K., Thomas, L., Ferreira, M. A. R., Bender, D., et al. (2007). PLINK: a tool set for whole-genome association and population-based linkage analyses. Am. J. Hum. Genet. 81, 559–575. doi: 10.1086/519795
Raghavan, N. S., Dumitrescu, L., Mormino, E., Mahoney, E. R., Lee, A. J., Gao, Y., et al. (2020). Association between common variants in RBFOX1, an RNA-binding protein, and brain amyloidosis in early and preclinical Alzheimer disease. JAMA Neurol. 77, 1288–1298. doi: 10.1001/jamaneurol.2020.1760
Ramanan, V. K., Risacher, S. L., Nho, K., Kim, S., Shen, L., McDonald, B. C., et al. (2015). GWAS of longitudinal amyloid accumulation on18F-florbetapir PET in Alzheimer’s disease implicates microglial activation gene IL1RAP. Brain 138, 3076–3088. doi: 10.1093/brain/awv231
Ramanan, V. K., Risacher, S. L., Nho, K., Kim, S., Swaminathan, S., Shen, L., et al. (2014). APOE and BCHE as modulators of cerebral amyloid deposition: a florbetapir PET genome-wide association study. Mol. Psychiatry 19, 351–357. doi: 10.1038/mp.2013.19
Ramasamy, A., Trabzuni, D., Guelfi, S., Varghese, V., Smith, C., Walker, R., et al. (2014). Genetic variability in the regulation of gene expression in ten regions of the human brain. Nat. Neurosci. 17, 1418–1428. doi: 10.1038/nn.3801
Serrano-Pozo, A., Frosch, M. P., Masliah, E., and Hyman, B. T. (2011). Neuropathological alterations in Alzheimer disease. Cold Spring Harb. Perspect. Med. 1:a006189. doi: 10.1101/cshperspect.a006189
Seward, M. E., Swanson, E., Norambuena, A., Reimann, A., Nicholas Cochran, J., Li, R., et al. (2013). Amyloid-β signals through tau to drive ectopic neuronal cell cycle re-entry in alzheimer’s disease. J. Cell Sci. 126, 1278–1286. doi: 10.1242/jcs.1125880
Shanbhag, N. M., Evans, M. D., Mao, W., Nana, A. L., Seeley, W. W., Adame, A., et al. (2019). Early neuronal accumulation of DNA double strand breaks in Alzheimer’s disease. Acta Neuropathol. Commun. 7:77. doi: 10.1186/s40478-019-0723-5
Sheng, J. G., Mrak, R. E., and Griffin, W. S. T. (1998). Progressive neuronal DNA damage associated with neurofibrillary tangle formation in Alzheimer disease. J. Neuropathol. Exp. Neurol. 57, 323–328. doi: 10.1097/00005072-199804000-00003
Sherva, R., Tripodis, Y., Bennett, D. A., Chibnik, L. B., Crane, P. K., De Jager, P. L., et al. (2014). Genome-wide association study of the rate of cognitive decline in Alzheimer’s disease. Alzheimers Dement. 10, 45–52. doi: 10.1016/j.jalz.2013.01.008
Sled, J. G., Zijdenbos, A. P., and Evans, A. C. (1998). A nonparametric method for automatic correction of intensity nonuniformity in MRI data. IEEE Trans. Med. Imaging 17, 87–97. doi: 10.1109/42.668698
Sleigh, J. N., Rossor, A. M., Fellows, A. D., Tosolini, A. P., and Schiavo, G. (2019). Axonal transport and neurological disease. Nat. Rev. Neurol. 15, 691–703. doi: 10.1038/s41582-019-0257-2
Strooper, B. D. E. (2010). Proteases and proteolysis in Alzheimer disease: a multifactorial view on the disease process. Physiol. Rev 90, 465–494. doi: 10.1152/physrev.00023.2009
Swerdlow, R. H. (2011). Brain aging, Alzheimer’s disease, and mitochondria. Biochim. Biophys. Acta. Mol. Basis Dis. 1812, 1630–1639. doi: 10.1016/j.bbadis.2011.08.012
Torrent, L., and Ferrer, I. (2012). PP2A and Alzheimer disease. Curr. Alzheimer Res. 9, 248–256. doi: 10.2174/156720512799361682
United Nations Department of Economic and Social Affairs (2021). World population ageing 2020: highlights. United Nations: Living Arrangements of Older Persons.
Wang, H. F., Wan, Y., Hao, X. K., Cao, L., Zhu, X. C., Jiang, T., et al. (2016). Bridging integrator 1 (BIN1) genotypes mediate Alzheimer’s disease risk by altering neuronal degeneration. J. Alzheimers Dis. 52, 179–190. doi: 10.3233/JAD-150972
Wilkins, C. H., Windon, C. C., Dilworth-Anderson, P., Romanoff, J., Gatsonis, C., Hanna, L., et al. (2022). Racial and ethnic differences in amyloid PET positivity in individuals with mild cognitive impairment or dementia: a secondary analysis of the imaging dementia-evidence for amyloid scanning (IDEAS) cohort study. JAMA Neurol. 79, 1139–1147. doi: 10.1001/jamaneurol.2022.3157
Xue, A., Wu, Y., Zhu, Z., Zhang, F., Kemper, K. E., Zheng, Z., et al. (2018). Genome-wide association analyses identify 143 risk variants and putative regulatory mechanisms for type 2 diabetes. Nat. Commun. 9:2941. doi: 10.1038/s41467-018-04951-w
Yang, J., Benyamin, B., McEvoy, B. P., Gordon, S., Henders, A. K., Nyholt, D. R., et al. (2010). Common SNPs explain a large proportion of the heritability for human height. Nat. Genet. 42, 565–569. doi: 10.1038/ng.608
Yang, Y., Mufson, E. J., and Herrup, K. (2003). Neuronal cell death is preceded by cell cycle events at all stages of Alzheimer’s disease. J. Neurosci. 23, 2557–2563. doi: 10.1523/jneurosci.23-07-02557.2003
Keywords: Alzheimer’s disease, PET, GWAS, amyloid-beta (Abeta), gene
Citation: Kim B-H, Lee H, Ham H, Kim HJ, Jang H, Kim JP, Park YH, Kim M and Seo SW (2023) Clinical effects of novel susceptibility genes for beta-amyloid: a gene-based association study in the Korean population. Front. Aging Neurosci. 15:1278998. doi: 10.3389/fnagi.2023.1278998
Received: 17 August 2023; Accepted: 25 September 2023;
Published: 12 October 2023.
Edited by:
Selvakumar Govindhasamy Pushpavathi, The University of Iowa, United StatesReviewed by:
Konstantinos Xanthopoulos, Aristotle University of Thessaloniki, GreeceCopyright © 2023 Kim, Lee, Ham, Kim, Jang, Kim, Park, Kim and Seo. This is an open-access article distributed under the terms of the Creative Commons Attribution License (CC BY). The use, distribution or reproduction in other forums is permitted, provided the original author(s) and the copyright owner(s) are credited and that the original publication in this journal is cited, in accordance with accepted academic practice. No use, distribution or reproduction is permitted which does not comply with these terms.
*Correspondence: Mansu Kim, bWFuc3Uua2ltQGdpc3QuYWMua3I=; Sang Won Seo, c2FuZ3dvbnNlb0BlbXBhbC5jb20=
Disclaimer: All claims expressed in this article are solely those of the authors and do not necessarily represent those of their affiliated organizations, or those of the publisher, the editors and the reviewers. Any product that may be evaluated in this article or claim that may be made by its manufacturer is not guaranteed or endorsed by the publisher.
Research integrity at Frontiers
Learn more about the work of our research integrity team to safeguard the quality of each article we publish.