- 1Department of Neurology, Affiliated Hospital of Weifang Medical University, School of Clinical Medicine, Weifang Medical University, Weifang, China
- 2Department of Neurology, Qingdao Municipal Hospital, Qingdao University, Qingdao, China
- 3Department of Neurology, Qingdao Municipal Hospital, University of Health and Rehabilitation Sciences, Qingdao, China
Background: Clusterin, a glycoprotein implicated in Alzheimer’s disease (AD), remains unclear. The objective of this study was to analyze the effect of cerebrospinal fluid (CSF) clusterin in relation to AD biomarkers using a longitudinal cohort of non-demented individuals.
Methods: We gathered a sample comprising 86 individuals under cognition normal (CN) and 134 patients diagnosed with MCI via the Alzheimer’s Disease Neuroimaging Initiative (ADNI) database. To investigate the correlation of CSF clusterin with cognitive function and markers of key physiological changes, we employed multiple linear regression and mixed-effect models. We undertook a causal mediation analysis to inspect the mediating influence of CSF clusterin on cognitive abilities.
Results: Pathological characteristics associated with baseline Aβ42, Tau, brain volume, exhibited a correlation with initial CSF clusterin in the general population, Specifically, these correlations were especially prominent in the MCI population; CSF Aβ42 (PCN = 0.001; PMCI = 0.007), T-tau (PCN < 0.001; PMCI < 0.001), and Mid temporal (PCN = 0.033; PMCI = 0.005). Baseline CSF clusterin level was predictive of measurable cognitive shifts in the MCI population, as indicated by MMSE (β = 0.202, p = 0.029), MEM (β = 0.186, p = 0.036), RAVLT immediate recall (β = 0.182, p = 0.038), and EF scores (β = 0.221, p = 0.013). In MCI population, the alterations in brain regions (17.87% of the total effect) mediated the effect of clusterin on cognition. It was found that variables such as age, gender, and presence of APOE ε4 carrier status, influenced some of these connections.
Conclusion: Our investigation underscored a correlation between CSF clusterin concentrations and pivotal AD indicators, while also highlighting clusterin’s potential role as a protective factor for cognitive abilities in MCI patients.
1. Introduction
Dementia, a neurological disorder marked by a steady deterioration of cognitive abilities, impacts memory, thought processes, and behavior. Alzheimer’s disease (AD) predominates as the most frequently diagnosed type of dementia, comprising about 70% of all cases (Duong et al., 2017). The amyloid cascade theory suggests that the accumulation of amyloid-beta (Aβ) peptide in cerebral regions marks a critical incident in AD’s progression. This event, occurring quite early, has the potential to set off tau-related pathology, provoking detrimental cellular reactions that may result in neuronal dysfunction and cellular death (Karran et al., 2011; Pooler et al., 2015).
The tau-related pathology observed in neurons aligns closely with the cognitive deterioration typical of the disease’s final stage. There are theories that this process occurs in a continuous cascade (Karran and De Strooper, 2022). Clusterin is a multifunctional glycoprotein encoded by the CLU gene (a single copy gene located on chromosome 8 at the p21-p12 locus), also known as apolipoprotein J (Foster et al., 2019; Yuste-Checa et al., 2022). The protective effect of this protein against cellular stress has been reported in studies of cardiac injury and tumor survival (Djeu and Wei, 2009; Foster et al., 2019). Apart from curtailing the development of amyloid polypeptide fibrils, a key marker of Alzheimer’s pathology, it further mitigates apoptosis and oxidative stress. It exhibits the capability to bind Aβ polypeptides, thus thwarting excessive inflammation (Wu et al., 2012).
One study observed a significant association between single nucleotide polymorphisms (SNPs) at the CLU gene (also known as APOJ) (rs11136000) at a genome-wide level (Harold et al., 2009). It has been reported that clusterin is associated with neuroprotective effects in AD (Wojtas et al., 2020) and has been associated with major AD pathological markers (Tang et al., 2022). Recent researches suggested that the CLU protein may promote the propagation of tau pathology, and an animal study also observed an increase in tau levels in mice treated with CLU (Martin-Rehrmann et al., 2005; Yuste-Checa et al., 2021). The processes through which clusterin influences AD pathology remain ambiguous. The primary focus of our research was to find the correlation between clusterin in CSF, AD pathophysiological processes, and cognitive abilities. Furthermore, we sought to determine the risk factors for AD, such as age, gender, and presence of APOE ε4 carrier status, influence these correlations (Rosselli et al., 2022).
Additionally, our goal was to determine the degree to which other pertinent biomarkers might influence these correlations. Gaining a comprehensive understanding of how clusterin ties into the onset and clinical presentation of AD could also serve as a beneficial guide for early detection and management of AD. To achieve our goals, we analyzed several biomarkers related to AD pathophysiology such as Aβ42, tau protein abnormalities (phosphorylated tau protein, P-tau protein), and indicators of neurodegeneration including total tau protein (T-tau protein) and MRI findings. These biomarkers were chosen for their representation of crucial stages in the AD progression and their extensive research in the context of this disorder (Wang et al., 2020). Our analyses were conducted on a population of non-demented individuals to better understand the early stages of the disease (Giau et al., 2019; Talwar et al., 2021).
2. Materials and methods
2.1. ADNI database
ADNI is a large-scale research effort launched in 2003, an international multicenter, ongoing, observational object, helps improve our understanding of AD and other disorders of the nervous system through a longitudinal study. Participants undergo regular clinical assessments, neuroimaging scans, and blood and CSF tests. The data collected from participants are stored in a central database and made available to researchers worldwide, see the ADNI website for the latest information https://adni.loni.usc.edu/ (Veitch et al., 2019, 2022).
2.2. Participants
Data was retrieved from the ADNI database, encompassing clinical attributes, CSF details, MRI scan results, and cognitive information from 220 participants. The ADNI database classifies subjects as clinically cognitively normal (CN), including those with subjective memory symptoms (MMSE >24, CDR = 0), those with mild cognitive impairment (MCI; MMSE >24, CDR = 0.5), and those with dementia. Participants underwent periodic evaluations for clinical data collection and participation in biomarker studies, which included CSF extraction. The inclusion of 220 individuals necessitated the presence of clusterin protein, demographic information, APOE gene carriage status, cognitive diagnosis. Simultaneously, we incorporated cognitive assessment scores of participants meeting these criteria and brain structural organizational data, despite the potential for missing data. Following the baseline examination, biomarker measurements and clinical cognitive assessments were conducted at baseline (BL), 12, 48, 72, 96, 120, 144, 168, and 180 months (Supplementary Table S1). All participants were made aware of the study’s aim and granted their approval via signed consent forms (Aisen et al., 2010).
2.3. Measurements of biomarkers
Measurement of Aβ42, T-tau, and P-tau in CSF by the INNO-BIA AlzBio3 kit (Shaw et al., 2009). CSF samples were analyzed using an automated Roche Elecsys® platform at the University of Pennsylvania. Further details can be found in previously published literature (Schindler et al., 2018). Repeated biomarker assays in CSF were averaged, and CSF clusterin was quantified using liquid chromatography-tandem mass spectrometry multiple reaction monitoring (LC/MS-MRM) with the peptide sequence IDSLLENDR. The ADNI database was used to collect all observed data (Kennedy et al., 2014; Liu et al., 2022).
2.4. MRI assessment
The MRI Core has established a standardized and publicly accessible data analysis set through the implementation of precise and fully automated brain and hippocampal segmentation algorithms, which have been validated in previously published studies. In our study, we focused on the following regions of interest (ROI): the whole brain, hippocampus, entorhinal, fusiform, and middle temporal areas. Previous large cohort studies, such as the Add Neuro Med project in Europe and the ADNI database study, have demonstrated the research value of these brain regions (Mangialasche et al., 2013; Falahati et al., 2017; Yu et al., 2021).
2.5. Cognitive assessment
We retrieved the composite memory score (MEM), as well as the commonly used cognitive measures, including ADAS-Cog which full name is the AD Assessment Scale-Cognitive subscale and executive functioning (EF) scores, the Brief Mental State Evaluation Scale (MMSE), and the Rey Auditory Word Learning Immediate Test (RAVLT immediate), from the ADNI Neuropsychological Test to monitor the cognitive measures’ trajectory. All of them are psychometrically optimized composite scores, which have been previously validated and proven to be reliable and externally valid (Folstein et al., 1975; Crane et al., 2012; Gibbons et al., 2012; Xu et al., 2022).
2.6. Statistical analysis
CSF clusterin concentrations were analyzed and found to be approximately normally distributed (Supplementary Table S1). To ensure the reliability of the results, We defined outliers as those with a standard deviation of 4 SD above or below the mean, and all analyses in this study were performed using transformed log10 values. We compared continuous demographics, clinical outcomes, and biomarkers using Student’s t-test or Wilcoxon rank sum tests, while nominal variables were compared with chi-square test or one-way ANOVA, followed by post hoc comparisons. The correlation of baseline CSF clusterin with biomarker and cognitive data was assessed using linear regression models. In each model, CSF clusterin levels were used as the form of independent variables, and dependent variables included cognitive measures and biomarkers. We employed a technique previously adopted by other researchers wherein a Linear Mixed Effects (LME) model was fitted, designating different measures as the dependent variable, The independent variable is time, while adjusting for random slope and intercept (Ma et al., 2021).
We also used the model to extract the rate of change of each variable for further subsequent analysis. We used the main biomarkers mentioned above as our hypothetical mediators to analyze the mediation between CSF clusterin and multiple cognitive performance, all tests are bootstrapped with 10,000 replications and adjusted for covariates. The cumulative risk of cognitive progression during follow-up was compared between groups stratified by clusterin level using Kaplan–Meier curves. Furthermore, the relationship between clusterin and the incidence of cognitive progression during follow-up was analyzed using multivariate Cox regression models. In the present study, we introduce a novel variable derived from the product of AD risk factors and CSF clusterin to the model to evaluate the interactive effects of clusterin levels and known AD risk factors (such as age, gender, and APOE ε4 status) on the dependent variables. Moreover, we utilized a pre-established and verified cut-off value: a critical concentration of 976.6 pg./mL of CSF Aβ42, enabling us to categorize subjects into A+ and A− groups for further analys (Tang et al., 2022).
All statistical analyses were performed in R 0.4.2.2. All regression analyses were corrected for age level, participant gender, educational level, and presence of APOE ε4 carrier status.
3. Results
The main characteristics of the study population, according to cognitive state, are reported in Table 1. We divided the participants into two groups: normal cognitive ability (CN; n = 86) and mild cognitive impairment (MCI; n = 134).
As expected, cognitive test scores differed significantly between the CN and MCI groups (ADAS11, p < 0.001; ADAS13, p < 0.001; ADASQ4, p < 0.001; MMSE, p < 0.001, AVLT immediate, p < 0.001; MEM, p < 0.001; EF, p < 0.001).
Almost all neuroimaging variables, except the whole brain volume and fusiform volume, showed significant differences between the two cognitive groups (ventricles volume, p = 0.001; hippocampus volume, p < 0.001; entorhinal volume, p < 0.001; mid temporal volume, p < 0.001). MCI group had higher T-tau (p < 0.001), P-tau (p < 0.001), and relatively lower levels of CSF Aβ42 (p < 0.001). There was no clear difference in CSF clusterin and years of education between the two groups which closely resembled previous findings (Tang et al., 2022). The MCI population had a higher proportion of APOE ε4 carriers compared to the CN group. Additionally, we observed a progressive increase in dementia prevalence from the CN (11.5%) to the MCI group (63.9%), which is by previously reported findings in the literature (Chen et al., 2022).
3.1. Cross-sectional CSF biomarker analysis
In our primary analysis, we computed the correlations between clusterin and all biomarkers as well as cognitive measures after adjustment of age, gender, educational level, APOE ε4 carriers’ status, and intracranial volume. A positive correlation was found between clusterin and biomarkers remained significant in the MCI group (CSF Aβ, β = 0.225, p = 0.007; Total tau, β = 0.440, p < 0.001; P-tau, β = 0.384, p < 0.001). EF was found to have a positive correlation with clusterin (β = 0.191, p = 0.035), whereas other cognitive factors had no correlation with clusterin. The high level of clusterin correlated with the bigger volumes of the hippocampus (p = 0.02), fusiform (p = 0.001), mid-temporal (p = 0.005), and smaller volumes of ventricles (p < 0.001). No significant associations were listed between baseline clusterin and other neuroimaging variables in a cross-sectional study. Further analyses suggested that CSF Aβ, tau, MRI imaging markers, and clusterin directly impact cognitive measurement (Figures 1, 2).
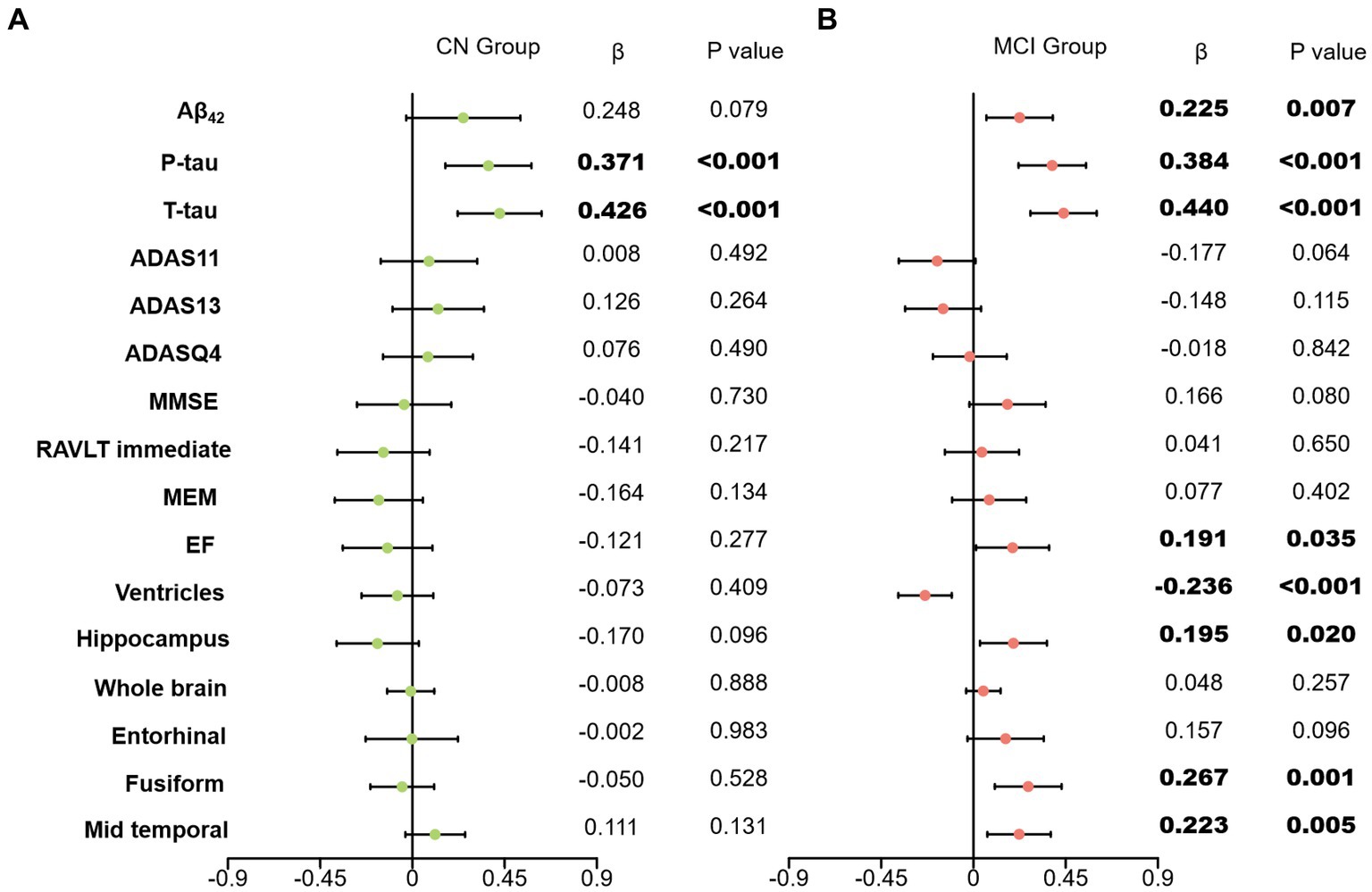
Figure 1. Effects of clusterin with biomarkers and cognitive data. Associations of baseline clusterin on baseline biomarkers and cognitive measurements in two group. All analyses were corrected for age, gender, educational level, APOE ε4 status, and intracranial volume. (A) results of CN group; (B) results of MCI group; CN, cognitively normal; MCI, mild cognitive impairment; Aβ42, Amyloid-β42; T-tau, Total tau; P-tau, Phosphorylated tau; ADAS, Alzheimer’s disease assessment scale-cognitive; ADASQ4, ADAS delayed word recall; MMSE, mini-mental state examination; RAVLT immediate, Rey auditory verbal learning test immediate recall; MEM, memory function composite score; EF, executive function composite score.
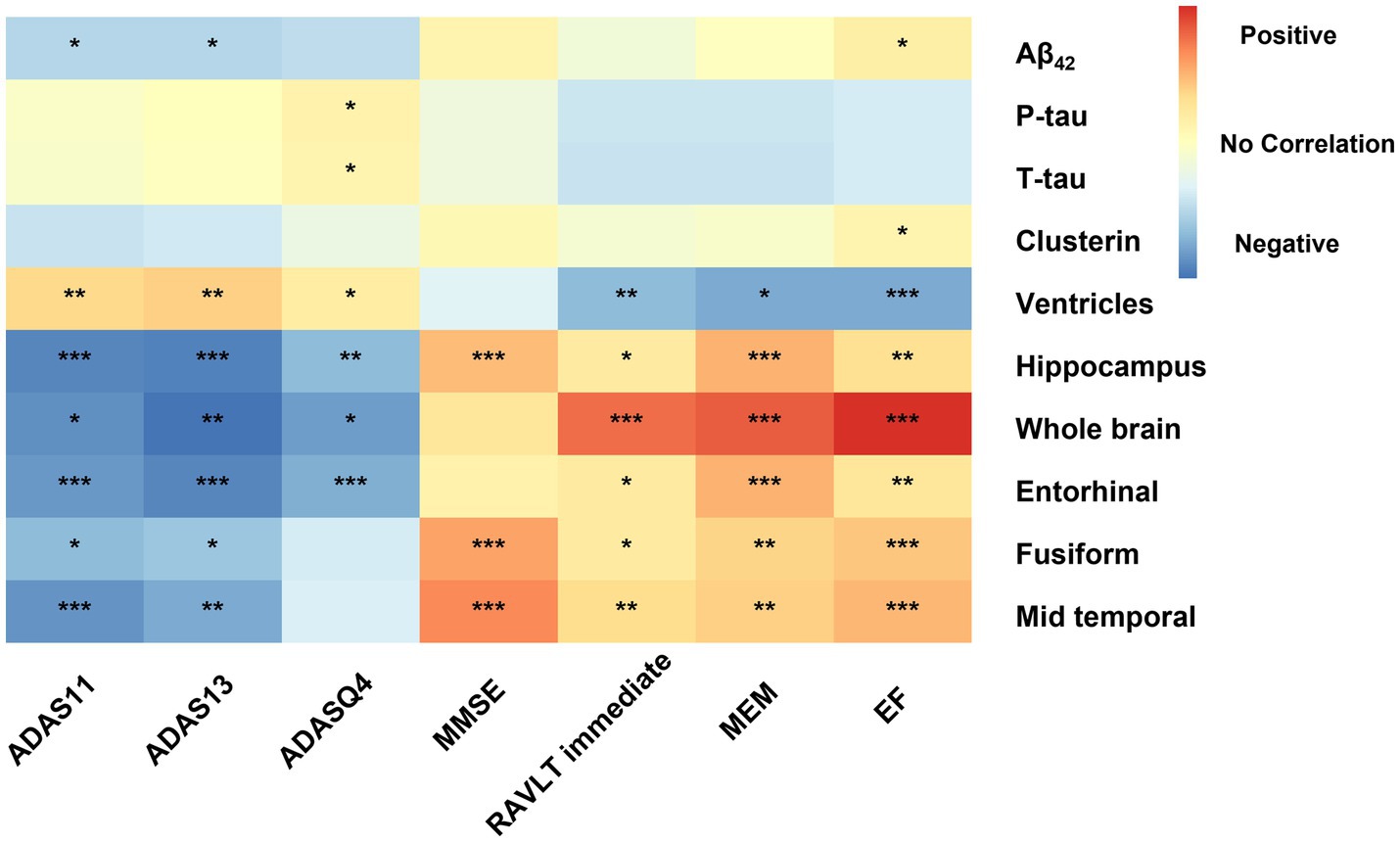
Figure 2. Effects of biomarkers on cognitive measures within MCI participants. Correlation of AD biomarker cognitive measurements at baseline. All analyses were corrected for age, gender, educational level, APOE ε4 status, and intracranial volume. Significance have been marked with the symbol ***, **, and *: p value<0.001 and p value <0.01, and p value<0.05. Aβ42, Amyloid-β42; T-tau, Total tau; P-tau, Phosphorylated tau; ADAS, Alzheimer’s disease assessment scale-cognitive; ADASQ4, ADAS delayed word recall; MMSE, mini-mental state examination; RAVLT immediate, Rey auditory verbal learning test immediate recall; MEM, memory function composite score; EF, executive function composite score.
3.2. Age, gender, and APOE ε4 interactions with CSF clusterin on biomarkers
In this analysis, we aimed to examine the interactions between CSF clusterin levels and age, gender, and APOE ε4 status on various biomarkers in the CN and MCI groups. The main findings were as follows:
Our observations revealed an interaction between CSF clusterin levels and age on T-tau, MRI (hippocampus volume, whole brain volume, and mid-temporal volume), and cognitive measurements (EF) exclusively in MCI participants. In the CN group, the initial interaction effect we examined involved the correlation between CSF clusterin and other measures, with APOE ε4 status affecting baseline MRI (mid-temporal volume). Interaction effects were noted for longitudinal MRIand cognitive measurements (EF). Interactions are statistically significant between CSF clusterin and gender on longitudinal ADAS11. As for the relationship between age and CSF clusterin, it was noteworthy for longitudinal MRI (ventricles and mid temporal) (Supplementary Tables S8, S9).
3.3. Prediction of longitudinal changes of CSF biomarkers, cognitive score, and neuroimaging variables using baseline CSF clusterin
In the longitudinal analysis, we examined the relationship between baseline CSF clusterin levels and changes in CSF biomarkers, cognitive scores, and neuroimaging variables over time. We hypothesized that the upregulation of clusterin would be related to an increase in overall cognitive ability. After adjusting for relevant covariates, we found the following results for the MCI group:
A higher baseline CSF clusterin level was significantly associated with a higher increased rate not only of global cognition (MMSE, β = 0.202, p = 0.029), composite memory (MEM, β = 0.186, p = 0.036) but also of short-term auditory-verbal memory (i.e., RAVLT immediate, β = 0.182, p = 0.038), executive function (EF, β = 0.221, p = 0.013), Additionally, lower baseline CSF clusterin level predicted greater decline in hippocampus volume (β = 0.170, p = 0.043), the whole brain volume (β = 0.176, p = 0.047), mid temporal volume (β = 0.177, p = 0.039). A higher baseline clusterin level was significantly associated with a higher increased rate of CSF Aβ (β = 0.243, p = 0.003). Meanwhile, the upregulation of baseline clusterin predicted a decrease in the rate of change of P-tau (β = −0.227, p = 0.013). However the effects of baseline clusterin on longitudinal biomarkers and cognitive were not statistically significant in cognitively normal populations (CN) (Figure 3).
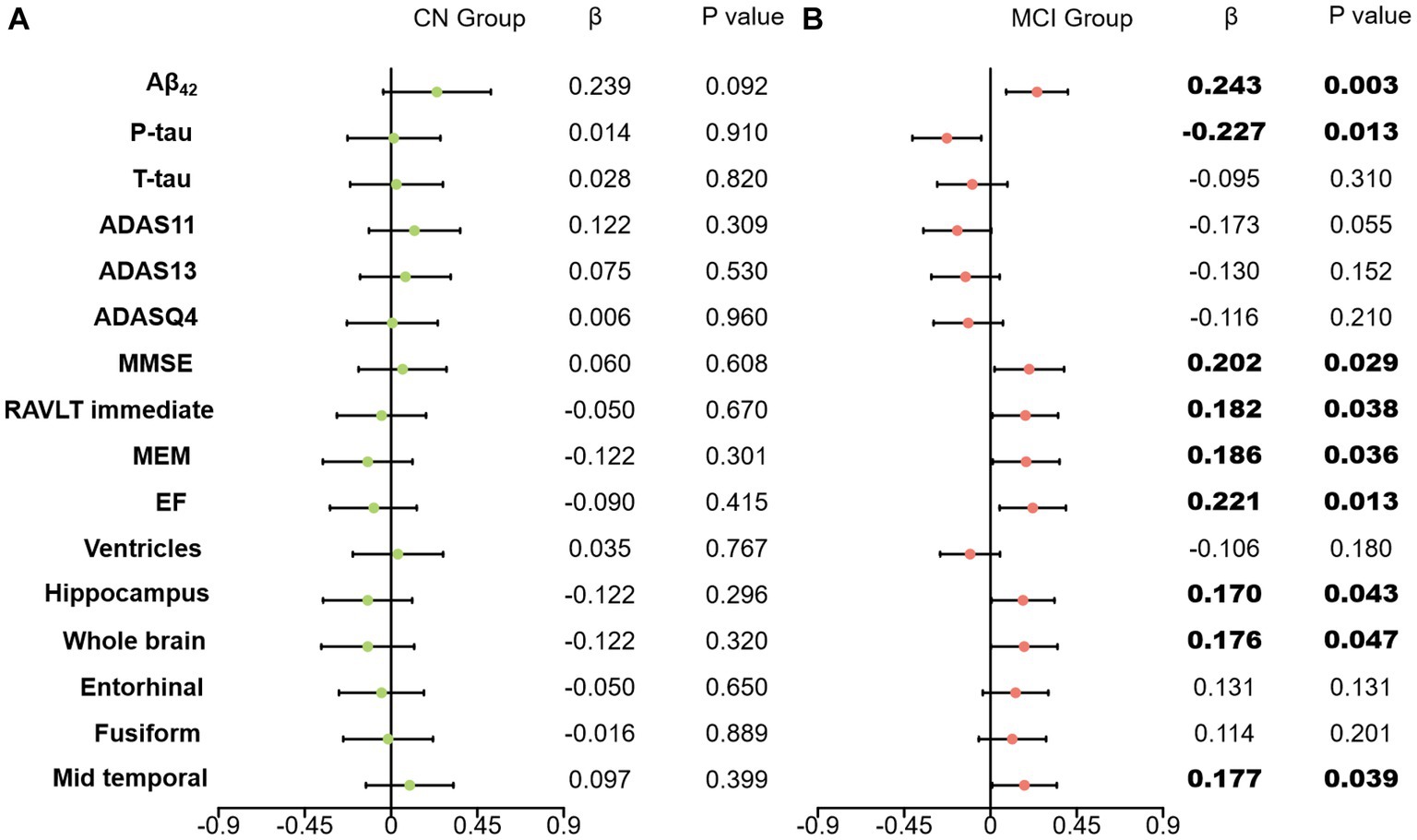
Figure 3. Effects of clusterin on longitudinal biomarkers and cognitive score. All analyses were corrected for age, gender, educational level, APOE ε4 status, and intracranial volume. (A) results of CN group; (B) results of MCI group; CN, cognitively normal; MCI, mild cognitive impairment; Aβ42, Amyloid-β42; T-tau, Total tau; P-tau, Phosphorylated tau; ADAS, Alzheimer’s disease assessment scale-cognitive; ADASQ4, ADAS delayed word recall; MMSE, mini-mental state examination; RAVLT immediate, Rey auditory verbal learning test immediate recall; MEM, memory function composite score; EF, executive function composite score.
3.4. Mediation analyses
Our initial regression studies across various groups unveiled the relationships between the index of pathology and cognitive parameters within a model that accounted for factors such as age, gender, education level, and APOE ε4 status. We delved into whether the connection between baseline CSF clusterin and cognitive parameters was influenced by factors such as CSF Aβ42, tau-related pathology, and neuroimaging variables. For a graphical representation of the mediation concept, see Figure 4A. There is no evidence found to suggest that the effects of CSF clusterin on cognitive impairments are mediated by its modulation of other AD markers in cross-sectional.
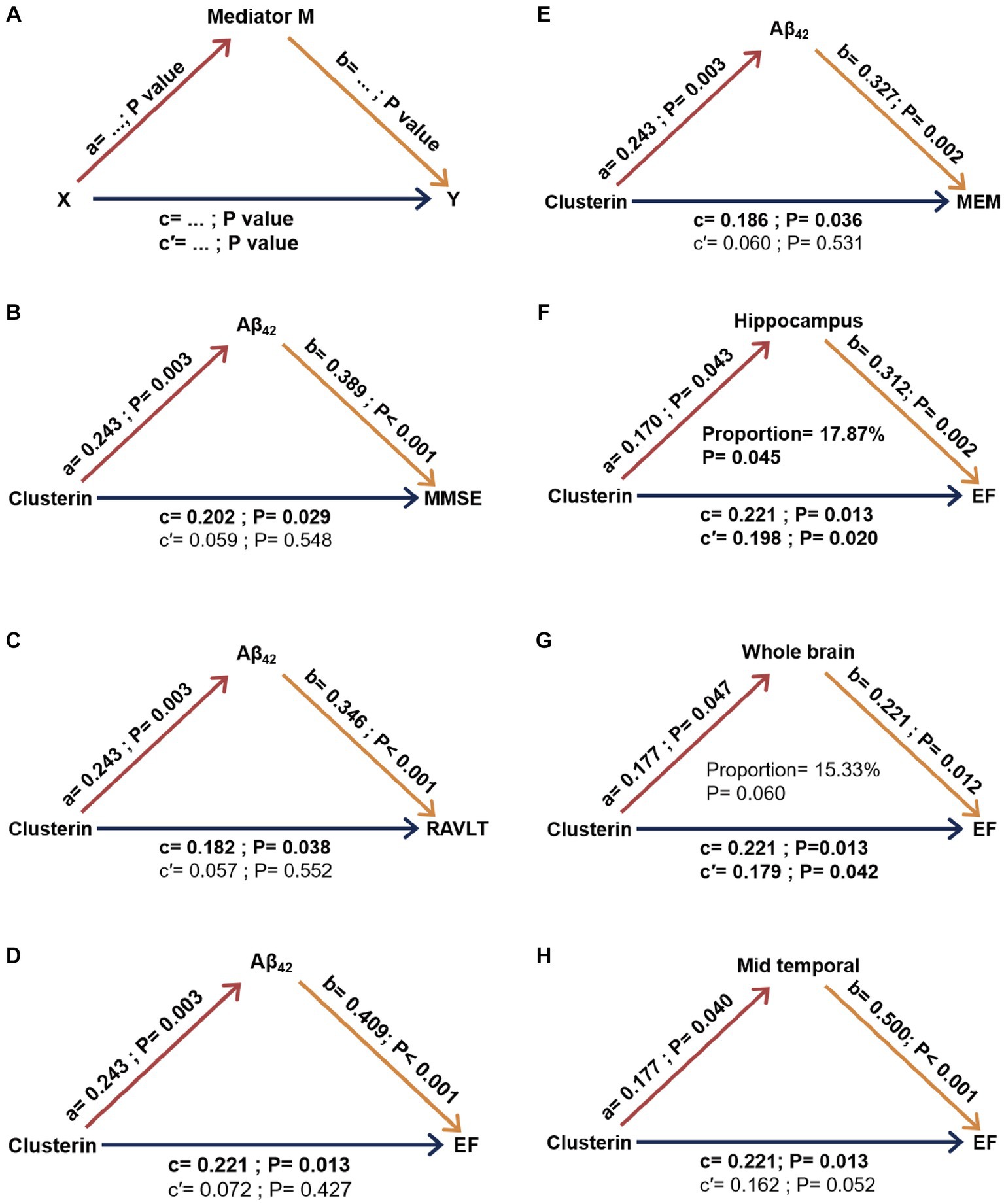
Figure 4. Mediation analysis results concerning clusterin’s impact on longitudinal cognition with biomarkers serving as mediators in MCI. The concept of mediation is visually interpreted in part (A). Specific results in part (B-H). a, b, c, c’ were took into account factors such as age, gender, education level, APOE ε4 carrier status, and intracranial volume. Aβ42, Amyloid-β42; MMSE, Mini-Mental State Examination; RAVLT, Rey auditory verbal learning test immediate recall; MEM, memory function composite score; EF, executive function composite score. X, independent variable; Y, dependent variable; M, mediating variable; proportion, percentage of mediation (obtained using a bootstrap method). a: clusterin’s effect on biomarker levels. b: biomarkers’ effect on cognitive scores. c: Total effect of clusterin on cognitive scores without mediation. c’: direct effect of clusterin on cognitive scores considering mediation.
Figure 4 demonstrates the ultimate models of MCI participants, wherein some mediators exhibited a minimum of the trend toward significance in the mediation pathway. Our findings suggest that the association between baseline clusterin and cognitive impairment, specifically executive function (EF), was partially mediated by longitudinal neurodegeneration as indicated by hippocampus volume, accounting for approximately 17.84% of the total effect. As for other possible mediation relationships, no meaningful mediating effects were observed in models with these biomarkers as mediating variables (CSF Aβ, tau pathology, whole brain volume, and mid-temporal volume) (Figure 4).
An estimation of hazard ratios (HRs) was performed using Cox proportional hazards regression analysis based on tertiles of baseline clustering. However, no significant results was observed in the analysis (Supplementary Table S7). We examined the correlation between CSF clusterin and biomarkers in both A− and A+ subjects. Our analysis showed a significant association between CSF clusterin and both CSF Aβ and tau pathology in the A+ subgroup, which is similar to the results observed in the whole MCI population (Supplementary Tables S11–S13).
4. Discussion
Current cohort studies of CSF clusterin were few and most have focused on plasma clusterin (Lidström et al., 1998; Thambisetty et al., 2010). The association between clusterin and AD pathology is unclear, and we used this study, a large longitudinal cohort of non-demented individuals was used to comprehensively investigate the predictive value of CSF clusterin in relation to various indicators of AD pathology. The findings revealed that baseline CSF clusterin was a predictor of longitudinal cognitive impairment in individuals with MCI, and that baseline CSF clusterin concentrations were more predictive in females under the age of 65. These results provide valuable insights into the potential role of CSF clusterin in the pathogenesis and progression of AD.
A significant negative correlation was found between plasma clusterin concentration and cognitive score in a study investigating the association between plasma clusterin and AD cognition (Thambisetty et al., 2010). However, in contrast to their result, our findings revealed a significant association between CSF clusterin levels and executive function in the MCI population in cross-sectional. In a study of plasma clusterin levels, lower clusterin groups were also found to have lower cognitive scores, which was consistent with our findings (Romagnoli et al., 2021).
Our findings suggest that CSF clusterin levels are associated with changes in pathology related to AD: decreased CSF clusterin levels in early AD (MCI) is associated with abnormal Aβ pathology, previous research has suggested that the correlation between clusterin and CSF Aβ42 may be related to the specific interaction of clusterin with amyloid. Several mechanisms may underlie these associations. Firstly, clusterin has been found to interact with prefibrillar species, inhibiting amyloid plaque formation in a substrate-dependent manner when the ratio of clusterin to Aβ peptide is relatively high (Yerbury et al., 2007). This association is more likely to be detected in the early stages of AD, consistent with our findings. Secondly, clusterin is sensitive to oxidative stress and has been reported to have several oxidative stress-related loci on its gene promoter. It is involved in various cellular stress responses such as the acute myocardial injury response and enhanced antioxidation in tumor cells. In the context of AD pathology, Aβ can trigger the generation of reactive oxygen species (ROS) by activating NMDA receptors. This stressful environment may lead to the upregulation of clusterin (Tiwari et al., 2019; Kalvaityte et al., 2022).
Rik Ossenkoppele et al. suggested that tau protein aggregation plays a pivotal role in the development of clinical AD. Their study revealed a strong link between a high burden of tau protein and cognitive decline. In vitro studies further support this relationship, showing that tau pathology can induce synaptic loss and reduce neural network function, thereby emphasizing the close association between tau protein pathology, neurodegeneration, and the onset of clinical symptoms (Ossenkoppele et al., 2022). Hyperphosphorylated tau can impair synaptic function and increase excitotoxicity and is also implicated in Aβ-induced neuronal death (Leschik et al., 2007; Khan and Bloom, 2016).
The causal relationship between increased CSF clusterin aggregates and tau protein pathology, changes in neurons is unclear. Patricia Yuste-Checa et al. found elevated CSF clusterin levels in individuals with tau pathology and Manifestations of neurodegeneration (Martin-Rehrmann et al., 2005; Yuste-Checa et al., 2021).
In our cross-sectional study, the tau pathology and neurodegeneration are associated with increased CSF clusterin levels. These findings are consistent with previous studies (Martin-Rehrmann et al., 2005; Deming et al., 2016). The cross-sectional correlation between tau and clusterin may suggest an upregulated response of clusterin to tau pathology (Nuutinen et al., 2009; Wojtas et al., 2020). Furthermore, this relationship is observed in cognitively normal populations and clusterin correlates with age, suggesting that this effect may be associated with normal aging (O'Bryan et al., 1993).
AD pathology typically begins in the temporal lobe region, particularly the medial temporal lobe (MTL), this particular area is involved in learning memory, stress and emotional response, consisting of structures such as the parahippocampal cortices, hippocampus and entorhinal (Jin et al., 2022), then spreads to the limbic regions of the medial and inferior temporal lobes, the posterior cingulate cortex, and finally to the isocortical areas of the brain (Serrano-Pozo et al., 2011). Clusterin was associated with several brain regions associated with cognition in our study, such as the hippocampus and middle temporal gyrus, which are mentioned in the Results section.
A previous meta-analysis showed that the mean volume of the hippocampus was smaller in the MCI population compared to the normal elderly group, which is consistent with our finding (Shi et al., 2009). Hippocampal atrophy has been found in people with MCI and has been linked to cognitive decline in a meta-analysis, which is consistent with our findings (Nickl-Jockschat et al., 2012).
Higher levels of clusterin at baseline correlate with a baseline volume of the hippocampus, similar associated brain regions also encompass the middle temporal gyrus, which primarily functions in explicit memory, language processing, and social cognition (Xu et al., 2019) suggesting that processing clusterin may be involved in the protection of neurons in relevant brain regions. Previous research indicates that clusterin may have neuroprotective properties, and in AD, reduced levels of this protein may be linked to neuronal degeneration and cell death (Giannakopoulos et al., 1998).
We identified a significant positive correlation between executive function (EF) and the level of clusterin. This finding suggests that higher levels of clusterin are associated with better executive functioning. The positive correlation with EF might be grounded on the neuroprotective role of clusterin, where higher levels could be indicative of a better neuronal health and, consequently, better cognitive performance, particularly in tasks requiring executive control.
Only a few cohort studies have systematically investigated longitudinal changes in biomarkers within MCI populations. A previous study identified a correlation between clusterin levels and rates of change in cognitively relevant brain regions (Thambisetty et al., 2012). Their research demonstrated that elevated clusterin levels correlated with a slower pace of brain atrophy. We observed similar results to theirs, particularly in the region of the temporal lobe and hippocampus. Our results echo previous research findings, showcasing that elevated levels of clusterin are associated with a slower rate of brain atrophy, notably in critical regions implicated in cognitive functions such as the hippocampus and the broader brain structure. The results indicate that higher clusterin levels are associated with a slower decline in the volume of both the hippocampus and the whole brain over time, suggesting a potential protective role of clusterin in preserving brain volume. Additionally, we found that clusterin levels were associated with cognitive changes in the MCI population, especially in memory and executive function. Over time, higher levels of clusterin proteins are associated with a slower decline in cognitive scores. Interestingly, we found a correlation between clusterin and longitudinal changes in tau pathology. Higher levels of clusterin were associated with a slower accumulation of tau pathology over time. The interaction mechanism between clusterin and tau pathology remains a subject of debate. One possible explanation is that the body responds to stressors, such as phosphorylated tau protein aggregation or cerebrovascular disease induction, by increasing clusterin concentration. The presence of clusterin impeded the formation and accumulation of tau aggregates, as reported in a cellular experiment (Mok et al., 2018; Dhiman et al., 2019; Wojtas et al., 2020; Yuste-Checa et al., 2022).
Based on these results, we conducted mediation analyses to determine which factors mediate the relationship between clusterin levels and cognitive performance. In the CN population, we did not observe a significant effect. However, in the mild cognitive impairment (MCI) population, our analysis suggested that the impact of clusterin on cognitive measures was partially mediated by neurodegeneration, specifically in the hippocampus. Previous research has also demonstrated that hippocampal atrophy can be observed in individuals with MCI and is associated with cognitive decline. Moreover, hippocampal atrophy has been established as a sensitive and specific marker for early detection of AD (Hampel et al., 2005). While we conducted a mediation analysis with AD biomarkers as intermediaries, and the results indicate that clusterin does not exert its influence on brain regions through the major biomarkers, including tau protein (Supplementary Table S10).
Our study’s findings imply that clusterin may exhibit neuroprotective effects during the initial stages of AD. Nonetheless, additional research is required to validate these findings and elucidate the underlying mechanisms connecting clusterin, hippocampal atrophy, and cognitive function.
Several limitations to our study should be acknowledged. Firstly, the lack of complete follow-up in our cohort may limit the reliability of our findings. Secondly, our analyses were based on CSF protein measurements which may not be as accurate as PET imaging data. Thirdly, there is variability in the results of different studies on clusterin which may require clarification through larger and more diverse cohorts. Lastly, the sampling of CSF clusterin is an invasive procedure that is not accessible to all patients, which may limit the generalizability of our findings. Due to the absence of long-term follow-up data on clusterin protein, we are unable to provide a comprehensive explanation for causality.
To sum up, our study has revealed a link between clusterin and important biomarkers of AD and has evaluated the protein’s long-term effect on the disease’s pathology using a longitudinal cohort. Our findings pave the way for further investigations into the complex role of this glycoprotein.
Data availability statement
The original contributions presented in the study are included in the article/Supplementary material, further inquiries can be directed to the corresponding authors.
Ethics statement
The studies involving human participants were reviewed and approved by the Alzheimer’s Disease Neuroimaging Initiative (ADNI). All participants were made aware of the study’s aim and granted their approval via signed consent forms.
Author contributions
HW: Data curation, Formal analysis, Investigation, Methodology, Software, Writing – original draft. L-ZM: Formal analysis, Conceptualization, Data curation, Methodology, Writing – review & editing. Z-HS: Data curation, Formal analysis, Methodology, Supervision, Validation, Writing – original draft. J-YL: Methodology, Writing – original draft, Data curation. W-YY: Data curation, Writing – original draft. FG: Data curation, Writing – original draft. WZ: Conceptualization, Supervision, Writing – review & editing. LT: Conceptualization, Supervision, Writing – review & editing, Project administration.
Funding
The author(s) declare financial support was received for the research, authorship, and/or publication of this article. This study was supported by grants from the National Natural Science Foundation of China (grant numbers 82271475 and 82201587).
Acknowledgments
Data used in the preparation of this article were obtained from the Alzheimer’s Disease Neuroimaging Initiative (ADNI) database (adni.loni.usc.edu). As such, the investigators within the ADNI contributed to the design and implementation of ADNI and/or provided data but did not participate in the analysis or writing of this report. A complete listing of ADNI investigators can be found at: http://adni.loni.usc.edu/wp-content/uploads/how_to_apply/ADNI_Acknowledgement_List.pdf. Data collection and sharing for this project was funded by the Alzheimer’s Disease Neuroimaging Initiative (ADNI) (National Institutes of Health grant U01 AG024904) and DOD ADNI (Department of Defense award number W81XWH-12-2-0012).
Conflict of interest
The authors declare that the research was conducted in the absence of any commercial or financial relationships that could be construed as a potential conflict of interest.
The handling editor J-TY declared a past co-authorship with the author LT.
Publisher’s note
All claims expressed in this article are solely those of the authors and do not necessarily represent those of their affiliated organizations, or those of the publisher, the editors and the reviewers. Any product that may be evaluated in this article, or claim that may be made by its manufacturer, is not guaranteed or endorsed by the publisher.
Supplementary material
The Supplementary material for this article can be found online at: https://www.frontiersin.org/articles/10.3389/fnagi.2023.1256389/full#supplementary-material
References
Aisen, P. S., Petersen, R. C., Donohue, M. C., Gamst, A., Raman, R., Thomas, R. G., et al. (2010). Clinical core of the Alzheimer's disease neuroimaging initiative: Progress and plans. Alzheimers Dement. 6, 239–246. doi: 10.1016/j.jalz.2010.03.006
Chen, Y., Qian, X., Zhang, Y., Su, W., Huang, Y., Wang, X., et al. (2022). Prediction models for conversion from mild cognitive impairment to Alzheimer's disease: a systematic review and meta-analysis. Front. Aging Neurosci. 14:840386. doi: 10.3389/fnagi.2022.840386
Crane, P. K., Carle, A., Gibbons, L. E., Insel, P., Mackin, R. S., Gross, A., et al. (2012). Development and assessment of a composite score for memory in the Alzheimer's disease neuroimaging initiative (ADNI). Brain Imaging Behav. 6, 502–516. doi: 10.1007/s11682-012-9186-z
Deming, Y., Xia, J., Cai, Y., Lord, J., Holmans, P., Bertelsen, S., et al. (2016). A potential endophenotype for Alzheimer's disease: cerebrospinal fluid clusterin. Neurobiol. Aging 37, 208.e1–208.e9. doi: 10.1016/j.neurobiolaging.2015.09.009
Dhiman, K., Blennow, K., Zetterberg, H., Martins, R. N., and Gupta, V. B. (2019). Cerebrospinal fluid biomarkers for understanding multiple aspects of Alzheimer’s disease pathogenesis. Cell. Mol. Life Sci. 76, 1833–1863. doi: 10.1007/s00018-019-03040-5
Djeu, J. Y., and Wei, S. (2009). Clusterin and chemoresistance. Adv. Cancer Res. 105, 77–92. doi: 10.1016/S0065-230X(09)05005-2
Duong, S., Patel, T., and Chang, F. (2017). Dementia: what pharmacists need to know. Can. Pharm. J. (Ott) 150, 118–129. doi: 10.1177/1715163517690745
Falahati, F., Ferreira, D., Muehlboeck, J. S., Eriksdotter, M., Simmons, A., Wahlund, L. O., et al. (2017). Monitoring disease progression in mild cognitive impairment: associations between atrophy patterns, cognition, APOE and amyloid. Neuroimage Clin. 16, 418–428. doi: 10.1016/j.nicl.2017.08.014
Folstein, M. F., Folstein, S. E., and McHugh, P. R. (1975). “Mini-mental state”: a practical method for grading the cognitive state of patients for the clinician. J. Psychiatr. Res. 12, 189–198. doi: 10.1016/0022-3956(75)90026-6
Foster, E. M., Dangla-Valls, A., Lovestone, S., Ribe, E. M., and Buckley, N. J. (2019). Clusterin in Alzheimer's disease: mechanisms, genetics, and lessons from other pathologies. Front. Neurosci. 13:164. doi: 10.3389/fnins.2019.00164
Giannakopoulos, P., Kövari, E., French, L. E., Viard, I., Hof, P. R., and Bouras, C. (1998). Possible neuroprotective role of clusterin in Alzheimer’s disease: a quantitative immunocytochemical study. Acta Neuropathol. 95, 387–394. doi: 10.1007/s004010050815
Giau, V. V., Bagyinszky, E., and An, S. S. A. (2019). Potential fluid biomarkers for the diagnosis of mild cognitive impairment. Int. J. Mol. Sci. 20:4149. doi: 10.3390/ijms20174149
Gibbons, L. E., Carle, A. C., Mackin, R. S., Harvey, D., Mukherjee, S., Insel, P., et al. (2012). A composite score for executive functioning, validated in Alzheimer's disease neuroimaging initiative (ADNI) participants with baseline mild cognitive impairment. Brain Imaging Behav. 6, 517–527. doi: 10.1007/s11682-012-9176-1
Hampel, H., Bürger, K., Pruessner, J. C., Zinkowski, R., DeBernardis, J., Kerkman, D., et al. (2005). Correlation of cerebrospinal fluid levels of tau protein phosphorylated at threonine 231 with rates of hippocampal atrophy in Alzheimer disease. Arch. Neurol. 62, 770–773. doi: 10.1001/archneur.62.5.770
Harold, D., Abraham, R., Hollingworth, P., Sims, R., Gerrish, A., Hamshere, M. L., et al. (2009). Genome-wide association study identifies variants at CLU and PICALM associated with Alzheimer's disease. Nat. Genet. 41, 1088–1093. doi: 10.1038/ng.440
Jin, W., Jie, F., Wenwei, Z., Bin, Z., Chen, S., Wei, S., et al. (2022). The medial temporal lobe structure and function support positive affect. Neuropsychologia 176:108373. doi: 10.1016/j.neuropsychologia.2022.108373
Kalvaityte, U., Matta, C., Bernotiene, E., Pushparaj, P. N., Kiapour, A. M., and Mobasheri, A. (2022). Exploring the translational potential of clusterin as a biomarker of early osteoarthritis. J. Orthop. Translat. 32, 77–84. doi: 10.1016/j.jot.2021.10.001
Karran, E., and De Strooper, B. (2022). The amyloid hypothesis in Alzheimer disease: new insights from new therapeutics. Nat. Rev. Drug Discov. 21, 306–318. doi: 10.1038/s41573-022-00391-w
Karran, E., Mercken, M., and De Strooper, B. (2011). The amyloid cascade hypothesis for Alzheimer's disease: an appraisal for the development of therapeutics. Nat. Rev. Drug Discov. 10, 698–712. doi: 10.1038/nrd3505
Kennedy, J. J., Abbatiello, S. E., Kim, K., Yan, P., Whiteaker, J. R., Lin, C., et al. (2014). Demonstrating the feasibility of large-scale development of standardized assays to quantify human proteins. Nat. Methods 11, 149–155. doi: 10.1038/nmeth.2763
Khan, S. S., and Bloom, G. S. (2016). Tau: the Center of a Signaling Nexus in Alzheimer's disease. Front. Neurosci. 10:31. doi: 10.3389/fnins.2016.00031
Leschik, J., Welzel, A., Weissmann, C., Eckert, A., and Brandt, R. (2007). Inverse and distinct modulation of tau-dependent neurodegeneration by presenilin 1 and amyloid-beta in cultured cortical neurons: evidence that tau phosphorylation is the limiting factor in amyloid-beta-induced cell death. J. Neurochem. 101, 1303–1315. doi: 10.1111/j.1471-4159.2006.04435.x
Lidström, A. M., Bogdanovic, N., Hesse, C., Volkman, I., Davidsson, P., and Blennow, K. (1998). Clusterin (apolipoprotein J) protein levels are increased in hippocampus and in frontal cortex in Alzheimer's disease. Exp. Neurol. 154, 511–521. doi: 10.1006/exnr.1998.6892
Liu, Y., Zhang, H., Zhong, X., Li, Z., Zetterberg, H., and Li, L. (2022). Isotopic N,N-dimethyl leucine tags for absolute quantification of clusterin and apolipoprotein E in Alzheimer's disease. J. Proteome 257:104507. doi: 10.1016/j.jprot.2022.104507
Ma, L.-Z., Zhang, C., Wang, H., Ma, Y.-H., Shen, X.-N., Wang, J., et al. (2021). Serum neurofilament dynamics predicts cognitive progression in de novo Parkinson’s disease. J. Parkinsons Dis. 11, 1117–1127. doi: 10.3233/JPD-212535
Mangialasche, F., Westman, E., Kivipelto, M., Muehlboeck, J. S., Cecchetti, R., Baglioni, M., et al. (2013). Classification and prediction of clinical diagnosis of Alzheimer's disease based on MRI and plasma measures of α−/γ-tocotrienols and γ-tocopherol. J. Intern. Med. 273, 602–621. doi: 10.1111/joim.12037
Martin-Rehrmann, M. D., Hoe, H.-S., Capuani, E. M., and Rebeck, G. W. (2005). Association of apolipoprotein J-positive β-amyloid plaques with dystrophic neurites in alzheimer’s disease brain. Neurotox. Res. 7, 231–241. doi: 10.1007/BF03036452
Mok, S.-A., Condello, C., Freilich, R., Gillies, A., Arhar, T., Oroz, J., et al. (2018). Mapping interactions with the chaperone network reveals factors that protect against tau aggregation. Nat. Struct. Mol. Biol. 25, 384–393. doi: 10.1038/s41594-018-0057-1
Nickl-Jockschat, T., Kleiman, A., Schulz, J. B., Schneider, F., Laird, A. R., Fox, P. T., et al. (2012). Neuroanatomic changes and their association with cognitive decline in mild cognitive impairment: a meta-analysis. Brain Struct. Funct. 217, 115–125. doi: 10.1007/s00429-011-0333-x
Nuutinen, T., Suuronen, T., Kauppinen, A., and Salminen, A. (2009). Clusterin: a forgotten player in Alzheimer's disease. Brain Res. Rev. 61, 89–104. doi: 10.1016/j.brainresrev.2009.05.007
O'Bryan, M. K., Cheema, S. S., Bartlett, P. F., Murphy, B. F., and Pearse, M. J. (1993). Clusterin levels increase during neuronal development. J. Neurobiol. 24, 421–432. doi: 10.1002/neu.480240402
Ossenkoppele, R., van der Kant, R., and Hansson, O. (2022). Tau biomarkers in Alzheimer's disease: towards implementation in clinical practice and trials. Lancet Neurol. 21, 726–734. doi: 10.1016/S1474-4422(22)00168-5
Pooler, A. M., Polydoro, M., Maury, E. A., Nicholls, S. B., Reddy, S. M., Wegmann, S., et al. (2015). Amyloid accelerates tau propagation and toxicity in a model of early Alzheimer's disease. Acta Neuropathol. Commun. 3:14. doi: 10.1186/s40478-015-0199-x
Romagnoli, T., Ortolani, B., Sanz, J. M., Trentini, A., Seripa, D., Nora, E. D., et al. (2021). Serum apo J as a potential marker of conversion from mild cognitive impairment to dementia. J. Neurol. Sci. 427:117537. doi: 10.1016/j.jns.2021.117537
Rosselli, M., Uribe, I. V., Ahne, E., and Shihadeh, L. (2022). Culture, ethnicity, and level of education in Alzheimer’s disease. Neurotherapeutics 19, 26–54. doi: 10.1007/s13311-022-01193-z
Schindler, S. E., Gray, J. D., Gordon, B. A., Xiong, C., Batrla-Utermann, R., Quan, M., et al. (2018). Cerebrospinal fluid biomarkers measured by Elecsys assays compared to amyloid imaging. Alzheimers Dement. 14, 1460–1469. doi: 10.1016/j.jalz.2018.01.013
Serrano-Pozo, A., Frosch, M. P., Masliah, E., and Hyman, B. T. (2011). Neuropathological alterations in Alzheimer disease. Cold Spring Harb. Perspect. Med. 1:a006189. doi: 10.1101/cshperspect.a006189
Shaw, L. M., Vanderstichele, H., Knapik-Czajka, M., Clark, C. M., Aisen, P. S., Petersen, R. C., et al. (2009). Cerebrospinal fluid biomarker signature in Alzheimer's disease neuroimaging initiative subjects. Ann. Neurol. 65, 403–413. doi: 10.1002/ana.21610
Shi, F., Liu, B., Zhou, Y., Yu, C., and Jiang, T. (2009). Hippocampal volume and asymmetry in mild cognitive impairment and Alzheimer's disease: meta-analyses of MRI studies. Hippocampus 19, 1055–1064. doi: 10.1002/hipo.20573
Talwar, P., Kushwaha, S., Chaturvedi, M., and Mahajan, V. (2021). Systematic review of different neuroimaging correlates in mild cognitive impairment and Alzheimer's disease. Clin. Neuroradiol. 31, 953–967. doi: 10.1007/s00062-021-01057-7
Tang, L., Wang, Z. B., Ma, L. Z., Cao, X. P., Tan, L., and Tan, M. S. (2022). Dynamic changes of CSF clusterin levels across the Alzheimer's disease continuum. BMC Neurol. 22:508. doi: 10.1186/s12883-022-03038-w
Thambisetty, M., An, Y., Kinsey, A., Koka, D., Saleem, M., Guntert, A., et al. (2012). Plasma clusterin concentration is associated with longitudinal brain atrophy in mild cognitive impairment. NeuroImage 59, 212–217. doi: 10.1016/j.neuroimage.2011.07.056
Thambisetty, M., Simmons, A., Velayudhan, L., Hye, A., Campbell, J., Zhang, Y., et al. (2010). Association of Plasma Clusterin Concentration with Severity, pathology, and progression in Alzheimer disease. Arch. Gen. Psychiatry 67, 739–748. doi: 10.1001/archgenpsychiatry.2010.78
Tiwari, S., Atluri, V., Kaushik, A., Yndart, A., and Nair, M. (2019). Alzheimer's disease: pathogenesis, diagnostics, and therapeutics. Int. J. Nanomedicine 14, 5541–5554. doi: 10.2147/IJN.S200490
Veitch, D. P., Weiner, M. W., Aisen, P. S., Beckett, L. A., Cairns, N. J., Green, R. C., et al. (2019). Understanding disease progression and improving Alzheimer's disease clinical trials: recent highlights from the Alzheimer's disease neuroimaging initiative. Alzheimers Dement. 15, 106–152. doi: 10.1016/j.jalz.2018.08.005
Veitch, D. P., Weiner, M. W., Aisen, P. S., Beckett, L. A., DeCarli, C., Green, R. C., et al. (2022). Using the Alzheimer's disease neuroimaging initiative to improve early detection, diagnosis, and treatment of Alzheimer's disease. Alzheimers Dement. 18, 824–857. doi: 10.1002/alz.12422
Wang, X., Huang, W., Su, L., Xing, Y., Jessen, F., Sun, Y., et al. (2020). Neuroimaging advances regarding subjective cognitive decline in preclinical Alzheimer's disease. Mol. Neurodegener. 15:55. doi: 10.1186/s13024-020-00395-3
Wojtas, A. M., Carlomagno, Y., Sens, J. P., Kang, S. S., Jensen, T. D., Kurti, A., et al. (2020). Clusterin ameliorates tau pathology in vivo by inhibiting fibril formation. Acta Neuropathol. Commun. 8:210. doi: 10.1186/s40478-020-01079-1
Wu, Z. C., Yu, J. T., Li, Y., and Tan, L. (2012). Clusterin in Alzheimer's disease. Adv. Clin. Chem. 56, 155–173. doi: 10.1016/b978-0-12-394317-0.00011-x
Xu, J., Lyu, H., Li, T., Xu, Z., Fu, X., Jia, F., et al. (2019). Delineating functional segregations of the human middle temporal gyrus with resting-state functional connectivity and coactivation patterns. Hum. Brain Mapp. 40, 5159–5171. doi: 10.1002/hbm.24763
Xu, J., Yuan, M. Q., and Fang, Y. (2022). Research on predicting the risk of mild cognitive impairment in the elderly based on the joint model. Zhonghua Liu Xing Bing Xue Za Zhi 43, 269–276. doi: 10.3760/cma.j.cn112338-20210620-00484
Yerbury, J. J., Poon, S., Meehan, S., Thompson, B., Kumita, J. R., Dobson, C. M., et al. (2007). The extracellular chaperone clusterin influences amyloid formation and toxicity by interacting with prefibrillar structures. FASEB J. 21, 2312–2322. doi: 10.1096/fj.06-7986com
Yu, Q., Mai, Y., Ruan, Y., Luo, Y., Zhao, L., Fang, W., et al. (2021). An MRI-based strategy for differentiation of frontotemporal dementia and Alzheimer's disease. Alzheimers Res. Ther. 13:23. doi: 10.1186/s13195-020-00757-5
Yuste-Checa, P., Bracher, A., and Hartl, F. U. (2022). The chaperone Clusterin in neurodegeneration-friend or foe? BioEssays 44:e2100287. doi: 10.1002/bies.202100287
Keywords: Alzheimer’s disease, cerebrospinal fluid, biomarkers, cognition, clusterin
Citation: Wang H, Ma L-Z, Sheng Z-H, Liu J-Y, Yuan W-Y, Guo F, Zhang W and Tan L (2023) Association between cerebrospinal fluid clusterin and biomarkers of Alzheimer’s disease pathology in mild cognitive impairment: a longitudinal cohort study. Front. Aging Neurosci. 15:1256389. doi: 10.3389/fnagi.2023.1256389
Edited by:
Jin-Tai Yu, Fudan University, ChinaReviewed by:
Ramhari Kumbhar, Johns Hopkins Medicine, United StatesVenkata Ajay Narendra Talabattula, Delaware State University, United States
Copyright © 2023 Wang, Ma, Sheng, Liu, Yuan, Guo, Zhang and Tan. This is an open-access article distributed under the terms of the Creative Commons Attribution License (CC BY). The use, distribution or reproduction in other forums is permitted, provided the original author(s) and the copyright owner(s) are credited and that the original publication in this journal is cited, in accordance with accepted academic practice. No use, distribution or reproduction is permitted which does not comply with these terms.
*Correspondence: Lan Tan, ZHIudGFubGFuQDE2My5jb20=; Wei Zhang, am56aGFuZ3dlaUAxMjYuY29t
†These authors have contributed equally to this work