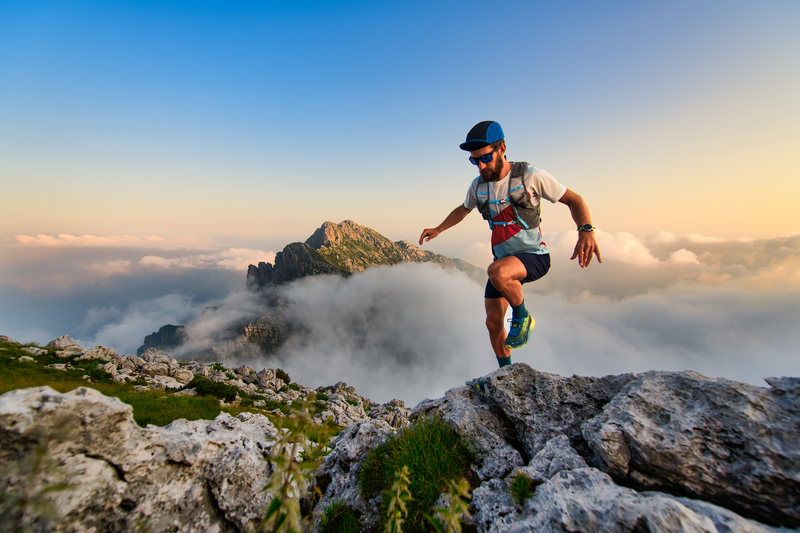
94% of researchers rate our articles as excellent or good
Learn more about the work of our research integrity team to safeguard the quality of each article we publish.
Find out more
MINI REVIEW article
Front. Aging Neurosci. , 28 July 2023
Sec. Neurocognitive Aging and Behavior
Volume 15 - 2023 | https://doi.org/10.3389/fnagi.2023.1231410
Cognitive control is one of the most fundamental aspects of human life. Its ageing is an important contemporary research area due to the needs of the growing ageing population, such as prolonged independence and quality of life. Traditional ageing research argued for a global decline in cognitive control with age, typically characterised by slowing processing speed and driven by changes in the frontal cortex. However, recent advances questioned this perspective by demonstrating high heterogeneity in the ageing data, domain-specific declines, activity changes in resting state networks, and increased functional connectivity. Moreover, improvements in neuroimaging techniques have enabled researchers to develop compensatory models of neural reorganisation that helps negate the effects of neural losses and promote cognitive control. In this article on typical ageing, we review recent behavioural and neural findings related to the decline in cognitive control among older adults. We begin by reviewing traditional perspectives and continue with how recent work challenged those perspectives. In the discussion section, we propose key areas of focus for future research in the field.
One of the most fundamental human skills is cognitive control—the ability to regulate and coordinate one’s thoughts, actions, and emotions to achieve goals and adapt to changing environmental demands. Lifespan research has focussed on the emergence of cognitive control from infancy (Zelazo et al., 2004) and in recent years, it has become a pressing research goal to identify what behavioural, neural, and computational factors drive developmental improvements in cognitive control (Lindenberger, 2014).
Cognitive control has been defined as an inherently variable dual-mechanism framework that allows individuals to switch between proactive and reactive control strategies, or modes, to adapt to the needs of a task or goal (Braver and West, 2008; Braver, 2012; Grady, 2012). Others have highlighted the role of cognitive control in the regulation and allocation of cognitive resources to process information (Fan, 2014). Because of the vast amount of information transmitted both internally (physiologically) and from the external environment, cognitive control is necessary for continuously adapting responses according to present goals (Braver, 2012; Fan, 2014). Environmental manipulations have been found to cause major changes in cognitive-control strategies and corresponding brain regions, and models like these are important for considering age-related changes in cognitive strategy (Braver, 2012). Even more so when cognitive demands are high during times of increased competition for resources, conflict, or distraction (Ludwig et al., 2010; Korotkevich et al., 2015).
However, understanding how cognitive control fades is as essential as understanding its emergence. The decline in cognitive control with ageing affects foundational skills in daily life, such as preserving independence, completing job-related tasks, and maintaining a healthy active lifestyle (Wiles et al., 2012; Bacsu et al., 2014; Denburg and Hedgcock, 2015; Strout et al., 2018). As a society we must promote quality of life alongside longer living, therefore it is critical to ensure sufficient knowledge to protect and conserve cognitive control in older adults, and to support the efforts of researchers and healthcare professionals to develop interventions and treatments (Lindenberger, 2014).
During the past two decades, a growing body of literature in psychology and neuroscience has challenged traditional perspectives on the decline of cognitive control with age, emphasising the heterogeneity in research data and the role of brain plasticity during ageing (Lövdén et al., 2010a; Bherer et al., 2013; Park and McDonough, 2013), as well as shifts in cognitive control strategies to compensate for age-related neurocognitive changes (Jimura and Braver, 2010). We begin this article by reviewing traditional perspectives and continue with how recent advances affect those perspectives. Finally, we propose key areas of focus for future research in the field. Atypical ageing is beyond the scope of this short review.
Cognitive control has been used interchangeably with executive processes, executive functioning, or executive control (Jurado and Rosselli, 2007; Vaughan and Giovanello, 2010; Diamond, 2013). The exact sub-domains under this umbrella term remain under debate (Jurado and Rosselli, 2007; Maldonado et al., 2020), but some of the core behavioural processes have been identified as inhibitory control (inhibiting or stopping pre-potent responses or resistance to interference), mental task or set shifting (the ability to quickly shift between two goals), memory updating (relating to holding and updating relevant information in working memory), and coordination (for example, aiding motor control; Verhaeghen, 2011; Zelinski et al., 2011; Maldonado et al., 2020). These processes are at the core of ageing research because they dominate vital aspects of life quality, including mental and physical health, career, academic ambitions, social cohesion, and the fostering of relationships (Jurado and Rosselli, 2007; Diamond, 2013).
Past research has argued for a global decline of cognitive control, typically underlined by slowing processing speed with age (Sorel and Pennequin, 2008). This deceleration in information processing affects cognitive skills, such as attention, memory, and executive functions (Korotkevich et al., 2015), making it harder to keep up in tasks that require simultaneous processing of multiple pieces of information (Li et al., 2001; Verhaeghen and Cerella, 2002). The cause of this decline relates to changes in brain structure and function over time (Adnan et al., 2019), and factors such as decreased blood flow and oxidative stress (Glade, 2010; Insel et al., 2012). Due to slow processing, older adults are also less likely to encode new information effectively, causing difficulties in retrieving information from long-term memory (Salthouse et al., 2003; Salthouse, 2009; Spreng and Turner, 2019).
Global declines also affect working memory—the ability to temporarily hold and manipulate information in mind. Working memory declines with age (Borella et al., 2010; Köstering et al., 2016), impacting a variety of daily activities, such as decision making and problem solving (Royall et al., 2005; Vaughan and Giovanello, 2010). The decrease in working memory limits the amount of information that can be actively processed, making it harder to switch between tasks and manipulate information to solve problems (Thornton and Dumke, 2005; Spieler et al., 2006).
As individuals age, their cognitive resources become increasingly taxed, leading to greater attention costs, particularly in tasks requiring cognitive resources such as attention shifting. Some researchers have proposed that performance level in two tasks simultaneously is a predictor of cognitive impairments in older adults (Downey et al., 2022). While researchers have pointed to divided attention—the ability to attend to multiple tasks simultaneously—as the source of this decline (McDowd and Craik, 1988; Craik et al., 1996; Verhaeghen and Cerella, 2002), selective attention—the ability to ignore unrelated information when performing a task—is vulnerable to ageing due to differences suppressing or filtering task-irrelevant information (Gazzaley et al., 2005; Schmitz et al., 2010; Jost et al., 2011). Additionally, difficulties in cognitively demanding situations have been related to an overall slowing of the cognitive-control network (Sorel and Pennequin, 2008; Wunsch et al., 2017) as shown in cases where older adults manage attentional control poorly during situations with salient distraction (Mayas et al., 2012).
Finally, older adults exhibit a decline in their ability to ignore irrelevant information or inhibit impulses to focus on relevant information (Hasher and Zacks, 1988; Reuter-Lorenz et al., 2021). Age has also been associated with retaining information that is no longer task relevant, which can hinder or benefit performance depending on the task (Amer et al., 2016, 2022). Inefficient inhibitory control leads to more distractibility and decreased attentional control. For example, older adults perform poorly when required to suppress prepotent actions of dominant responses (Park and Reuter-Lorenz, 2009; Zelinski et al., 2011). A large-scale cross-sectional study featuring more than 3,000 older adults suggested that the effects of ageing on inhibitory control result from a decline in fine motor skills, processing speed, and visuospatial abilities (Hoogendam et al., 2014) which are strong early markers for the general decline in cognitive control (Wunsch et al., 2017; Glover et al., 2021).
At the neural level, evidence points to the pre-frontal cortex (PFC) as the central brain region that is responsible for cognitive control (Vaughan and Giovanello, 2010; Park and McDonough, 2013). The PFC is involved in a wide range of functions, including working memory (Diamond, 2013; Köstering et al., 2016), decision making (Craik and Bialystok, 2006; Denburg and Hedgcock, 2015), attentional regulation (Diamond, 2013), regulation of thoughts (Vaughan and Giovanello, 2010), and the processing of conflicting information and resolution of cognitive conflicts (Mayas et al., 2012).
Evidence linking PFC activation and cognitive control led to the frontal lobe hypothesis of ageing (West, 1996; Reuter-Lorenz et al., 2021). The main argument in this hypothesis is that the frontal lobe has a greater decline with age compared to other brain regions and it deteriorates at an accelerated rate (Rosselli and Torres, 2019), thereby leading to poorer cognitive control in older adults. Supporting empirical evidence includes structural and functional changes in the frontal lobe as people age (Park and McDonough, 2013; Cabeza et al., 2018), specifically decreased neural activity in the PFC (Jimura and Braver, 2010) and decreased grey matter volume (Rosselli and Torres, 2019). Because the PFC is one of the first areas to show decreased activity when under strain (e.g., during high stress, depression, or lack of sleep), it has been identified as a “warning system” for cognitive ageing (Diamond, 2013).
The frontal lobe hypothesis is also supported by ageing changes in neurotransmitter systems which are involved in the regulation of PFC functions. For example, the dopamine system is known to play a role in executive functioning, working memory, and attention (Zelazo et al., 2004; Park and Reuter-Lorenz, 2009), and ageing is associated with decreased dopamine modulation in the PFC, which contributes to age-related declines in cognitive control (Grady, 2012; Gutchess, 2014; Lindenberger, 2014). Other important neurotransmitters involved in PFC maturation and its effects on cognitive control are the acetylcholine (involved in attention, learning, and memory; Blokland, 1995) and norepinephrine systems (involved in attention, arousal, and the regulation of mood).
For more than a decade, researchers have challenged the idea of a global cognitive decline by emphasising the heterogeneity found in the ageing research data (Lindenberger and Baltes, 1997; Wilson et al., 2002; Raz et al., 2005; Verhaeghen, 2011). While attention allocation and inhibitory control may decline with age (Rozas et al., 2008; Zanto and Gazzaley, 2017), working memory and reasoning abilities may be preserved or even improved (Hoogendam et al., 2014; Maldonado et al., 2020). Older adults even triumph over younger adults in certain crystallised cognitive control tasks, specifically remembering task-relevant information in a real-world multiple errands task (Kliegel et al., 2007). High variability was also found in the onset of decline across different cognitive-control processes (Jurado and Rosselli, 2007), and the onset of declines could be exaggerated by cross-sectional data due to cohort effects, such as age-related similarities in education level (Nyberg et al., 2012).
Heterogeneity can depend on the experimental tasks. For example, a recent meta-analysis indicated that common tasks used to measure cognitive control, such as Stroop and flanker, do not show reliable age-related deficits, whereas go/no-go and stop-signal tasks are associated with impairments in inhibitory control (Fan, 2014; Rey-Mermet and Gade, 2018). Even within inhibitory control, impairments depend on the type of task used (Borella et al., 2009; Mayas et al., 2012). These cross-task differences suggest that the ageing process of cognitive control is not a univariate reduction in performance but rather a more complex, unsynchronised change among cognitive processes.
Significant heterogeneity is also observed across individuals due to variability in socio-economic status (SES) and quality of living factors such as education, lifestyle, and general health (Migeot et al., 2022). Processes that are sensitive to stress or environmental adversity (e.g., attention allocation, emotional regulation, and decision making) are affected more by differences in SES compared to other processes. These effects relate to the limited access of individuals to quality education, healthcare, and other protective resources, alongside increased exposure to environmental toxins and other life stressors that can have a negative impact on brain function. Historically, protective factors, such as higher education and occupation attainment levels, have been discussed as promoting individual cognitive reserve levels (Stern, 2002). Reuter-Lorenz and Park (2014) suggested that factors such as education level, physical fitness, and multilingualism could protect cognitive functioning by improving brain structure and function and providing neural and cognitive support against age-related cognitive declines. Likewise, individuals with higher levels of education (Lenehan et al., 2015) and better health practices (Woods et al., 2012) exhibit fewer declines in inhibitory control and a slower decline in cognitive control.
Taken together, recent advances challenge the global decline approach by suggesting a domain-specific decline, affecting some cognitive-control processes more than others, with varying degrees of decline in different stages of ageing (Cabeza et al., 2018). These findings highlight the importance of considering specific domains when studying age-related changes and developing interventions to improve cognitive control in older adults.
That said, the lack of consensus on the definition of cognitive control contributes to the discussion about heterogeneity (Rey-Mermet and Gade, 2018; Heckner et al., 2021). For some researchers, cognitive control refers to the ability to focus attention and ignore distractions (Mayas et al., 2012), while for others it encompasses a wider range of functions. Some researchers use working memory (Zelazo et al., 2004) or attention to measure cognitive control, while others use inhibitory control (Persad et al., 2002; Salthouse et al., 2003; Vaughan and Giovanello, 2010). Theories need to account for these inconsistencies and form a more complex view of what cognitive control is and how its ageing should be measured.
Despite strong evidence supporting the frontal lobe hypothesis of ageing, the theory does not explain all the neural effects and it is still a matter of scientific debate (Verhaeghen, 2011). To that end, researchers raised questions about whether declines in cognitive control result solely from frontal lobe changes and investigated the role of other relevant brain regions, such as the parietal and temporal lobes (Jimura and Braver, 2010; Vallesi et al., 2011). There are also knowledge gaps concerning the relation between declines in the frontal lobe and various environmental factors (e.g., lifestyle; Lövdén et al., 2010a).
Recent advances have attempted to challenge the focus on the frontal cortex. Large-scale neural changes in older adults’ regulation of default network (DN) activity have been related to the ageing of cognitive control (Park and Reuter-Lorenz, 2009; Grady, 2012). The DN refers to areas of the brain that are active when an individual is “at rest” or involved in tasks that are internal, such as self-reflective thoughts (Park and Reuter-Lorenz, 2009). When the brain moves to more demanding tasks, the DN is suppressed (Spreng and Turner, 2019). This suppression is reduced in older adults and this reduction is associated with underperformance on cognitive control tasks. Moreover, declines in DN activity and its functional connectivity have been associated with impaired resource allocation, accounting for observed age-related differences in cognitive performance (Park and Reuter-Lorenz, 2009).
In younger adult brains, neural networks are highly specialised, meaning they are densely connected within specific subnetworks but more sparsely connected between other networks (Chan et al., 2014; Geerligs et al., 2015). With age, networks become less specialised, as seen in a reduction of within-network connectivity and increase in internetwork activity both during specific tasks and in resting state conditions (Chan et al., 2014; Geerligs et al., 2015). The role of resting state networks and their functional segregation during cognitive control declines have been tested longitudinally. A recent study tested changes in brain networks over a 4-year period during older adulthood (Malagurski et al., 2020). The segregation of the DN, salience network, and frontoparietal control network deteriorated. Moreover, the drop in segregation of the frontoparietal control network was associated with declines in processing speed. Mixed patterns in network connectivity, including increases in DN and in between-network connectivity has been associated with strong cognitive functioning (La Corte et al., 2016). While resting state network activity has provided these insights and is useful for investigating whole-brain connectivity patterns, it has been recognised that an over-reliance on resting state data may withdraw from the focus on task-relevant cognitive measures, and the two should be used as complementary approaches (Campbell and Schacter, 2017).
Finally, older adults exhibit longer stop-signal reaction times compared to young adults and those age-related differences are associated with functional changes in the supramarginal gyrus, anterior insula, right inferior frontal cortex, and pre-supplementary motor areas (Coxon et al., 2016). These findings tie in with existing research showing the relationship between brain structure maintenance and improved cognitive control. For example, in task-switching studies, connectivity of task-relevant frontoparietal regions in younger adults was increased compared to older adults (Madden et al., 2010). Taken together, neural mechanisms underlying ageing of cognitive control goes beyond the functionality of the frontal cortex.
Another focal point in the neuroscience of cognitive-control ageing is plasticity—the ability of the neural networks to change and adapt in response to experiences and learning (Park and McDonough, 2013; Gutchess, 2014). Plasticity allows the brain to respond to environmental demands by way of structural changes that alter brain function and behaviour over time (Lövdén et al., 2010b; Lindenberger, 2018). Neuroplasticity models have helped to explain how structural changes occur due to a metaphoric mismatch between the scope of current functioning and the demands of the environment (Lövdén et al., 2010b; Lindenberger, 2018). This mismatch must occur for enough time and be intense enough to surpass a threshold, causing the changes necessary for a new state of balance between supply and demand (Lövdén et al., 2010b; Lindenberger, 2018; Lindenberger and Lövdén, 2019).
The exploration-selection-refinement (ESR) model of neuroplasticity posits three phases of learning (Lindenberger and Lövdén, 2019). Following heightened levels of activity, structural neural changes are supported by myelination and the formation of new dendric spines leading to an increase in new circuits formed and trialled. The best performing microcircuit is selected, variability decreases, and then the microcircuitry is stabilised and unselected microcircuits are withdrawn (Lindenberger and Lövdén, 2019).
Plastic changes in cognitive abilities may require both local plasticity (for example within the PFC) and widespread changes, such as improved network reorganisation aided by myelination of white matter tracts (Lindenberger and Lövdén, 2019). When considering neuroplasticity models in relation to neural activity patterns related to ageing, older age is more strongly associated with neural maintenance and cognitive flexibility than growth (Braver et al., 2014; Coxon et al., 2016; Kühn and Lindenberger, 2016), and neural patterns in older adults are less distinctive than in younger networks (Li et al., 2000). This could be due to an increased reliance on stronger network reorganisation which can be observed through increased bi-hemispheric activation (Cabeza, 2002).
Cabeza et al. (2002) theorised that if activity in specific regions of the brain declines with age, it would be reasonable to assume that compensatory mechanisms could gradually permit adaptive neurocognitive functioning to ageing of cognitive control. This idea has been supported by the notion of adaptations as driven by neurological supply deficits and environmental demand mismatches, which lead to plasticity-related changes in old age (Lövdén et al., 2010b). Increased neurological generalisation has been shown to counter neurocognitive declines, acting as a supportive adaptation (Cabeza et al., 2002; Park and McDonough, 2013).
Increasing age is associated with losses in controlled or fluid cognition (Zelazo et al., 2004; Thornton and Dumke, 2005; Wang et al., 2020). However, researchers have suggested older adults’ gains in crystalised knowledge (Kramer and Willis, 2002) could be due to shifts in cognitive architecture, with crystallised gains reflected in functional network architecture changes in the brain (Spreng and Turner, 2019). Increased reliance on prior knowledge has been highlighted as a compensatory mechanism that allows older adults to offset restrictions in cognitive resources, for example in decision making and planning tasks (Denburg and Hedgcock, 2015), and when strategically prioritising higher-value information in memory (Castel, 2007; Knowlton and Castel, 2022). Neural activity in older adults has shown increased recruitment of PFC regions and reduced suppression of the DN (Park and Reuter-Lorenz, 2009; Grady, 2012; Spreng and Turner, 2019). Thus, with age, cognition becomes increasingly influenced by existing knowledge, engaging the DN and lateral prefrontal brain regions to compensate for cognitive supply limitations (Lövdén et al., 2010b; Spreng and Turner, 2019).
Researchers have refined the compensatory model by examining how plasticity negates the effects of neural losses and promotes cognitive control in situations where cognitive demands are high (Vallesi et al., 2011; Park and McDonough, 2013; Cabeza et al., 2018). During demanding tasks older adults show increased bilateral activation in the frontal gyrus, the inferior parietal lobule, and posterior cerebellum. These activations are associated with over-recruitment of additional regions during more difficult tasks. Over-recruitment may lead older adults to reach their peak performance during difficult tasks at an earlier rate than younger adults, with quick improvements followed by plateaus in performance during inhibitory control tasks [for an explanation of neural activation and level of task demand, see the compensation-related utilisation of neural circuits hypothesis (CRUNCH), Reuter-Lorenz and Cappell, 2008; Vallesi et al., 2011; Reuter-Lorenz et al., 2021]. Thus, compensatory mechanisms include neural reorganisation in response to cognitive losses; upregulation of existing processes; and selection/promotion of an existing cognitive strategy without the development of a new structure or process (Cabeza et al., 2018).
The reviewed research clarifies the need to provide a more nuanced understanding of the complex interaction between cognitive processes during ageing. To achieve this, we propose key areas of focus for future research, along with challenges in this field.
Studies on the role of plasticity and its relation to compensation during ageing suggest that the same brain regions can play different roles in older vs. younger adults depending on the connections with other intra-network regions. Yet, the structure of brain activity changes with age and their origins remain under debate and researchers have noted the need for any interpretations to be supported by behavioural evidence.
Building on the positive connections between behavioural measures, structural brain changes, and neural changes in functional connectivity (Lövdén et al., 2010a; Grady, 2012; de Lange et al., 2017), further examination of how neural plasticity underlies shifts in cognitive control during ageing holds promise for identifying ways to promote healthy ageing and maintain cognitive function throughout the lifespan. Specifically, similar to recent work in child development (Ossmy and Adolph, 2020), future ageing research would benefit from using advanced analytic techniques to (1) describe the heterogeneity in cognitive control ageing using a multivariate approach; (2) identify factors that contribute to different types of age-related declines; and (3) use identified factors and patterns to generalise findings from one group of older adults to another and help explain individual differences. When considering structural brain changes, researchers should also investigate structural invariance, as longitudinal stability has been associated with greater performance on different cognitive performance measures (de Frias et al., 2009).
Developmental researchers have increasingly examined child development as a cascadic effect, acknowledging that multiple processes and factors during development are at play during any given moment (Oakes and Rakison, 2019). This is also true for cognitive changes in later life. A person’s environment, behaviours, and cognitive resources continue to change from adulthood into old age, contributing to the significant individual differences in ageing.
The ageing research to-date has supported the notion of a multidimensional change in cognitive control with age and has acknowledged how individual experiences, such as education level, have been shown to impact brain network segregation (Chan et al., 2021). However, research has neglected a cascadic approach that considers how experiences influence ageing outcomes through a series of interconnected and reciprocal effects across multiple domains and their cumulative effect (Oakes and Rakison, 2019). Cognitive control is an ideal candidate for exploring ageing cascades because of the involvement of multiple processes and functions and their potential interactions (Oakes and Rakison, 2019). Specific attention should be paid to the cascading effects of life experiences on neural plasticity (Gutchess, 2014).
A longitudinal study design could be used to investigate the cascadic effects of life experiences on neural plasticity. As with developmental studies with infants and children, participants would be recruited from different age groups and researchers would follow them over an extended period, collecting data at multiple time points. Education, occupation, physical activity, social engagement, stress levels, and cognitive stimulation would be collected and analysed in a multivariate approach (i.e., full patterns from multiple data sources are used to predict changes in neural activity). Cognitive function could be assessed using neuropsychological tests, while neuroimaging techniques such as structural MRI, fMRI, and Diffusion Tensor Imaging would provide insight into brain structure and connectivity. Statistical analysis techniques, including regression models and mediation/moderation analyses, should be used to explore the relationships between life experiences and neural measures while accounting for confounding factors. Cross-sectional comparisons between individuals with diverse life trajectories and intervention studies, such as cognitive training programmes or physical exercise interventions, could further elucidate the impact of specific life experiences on neural plasticity in ageing.
Despite much evidence from psychology and neuroscience, the exact mechanisms that contribute to age-related declines in cognitive control are still unclear. Computational modelling of cognition—the use of mathematical and computational approaches to simulate and explain neural and cognitive processes—has a high potential to achieve such understanding.
Previous work used computational modelling to formulate cognitive control as a process of controlling the flow of information between perceptual and executive systems, feeding internal system and external environment information to the controller (Haykin et al., 2012). In their review of the literature on computational modelling in ageing, Dully et al. (2018) discussed the useful nature of models for explaining individual differences by identifying age-associated processes related to multiple cognitive domains. One useful example is sequential sampling modelling, which explains decision making as a threshold that is passed once sufficient pre-determined sensory information is gathered, leading to a decision (Forstmann et al., 2016; Dully et al., 2018). In the context of cognitive-control ageing, sequential sampling models are useful in considering behavioural data associated with relevant latent variables such as the quantity and quality of evidence entering the decision-making process, and associated processes including sensory encoding and motor execution (Dully et al., 2018).
Utilising computational models could provide a quantitative framework for understanding the role of different brain regions and neurotransmitter systems in changes that occur with ageing and how they affect cognitive control performance. Moreover, simulating different ageing models and comparing the results to empirical data may provide the most likely explanations for observed changes in cognitive control with ageing, thereby guiding the development of interventions to maintain cognitive control in older adults. Finally, computational modelling facilitates communication and collaboration between researchers in different fields, such as cognitive neuroscience, psychology, and computer science, by providing a shared language and conceptual framework for understanding the ageing of cognitive control.
Our final proposal for future direction involves the investigation of brain connectivity patterns at a whole-brain level. Recent advancements have initiated the exploration of how ageing affects whole-brain functional connectivity by examining patterns of segregation and integration throughout the entire brain (Park and Reuter-Lorenz, 2009; Campbell et al., 2013), as well as connectivity strength in relation to age and task performance (Lugtmeijer et al., 2023). This comprehensive approach holds significant promise for testing the role of age-related changes in brain connectivity in shaping cognitive control abilities (Geerligs et al., 2017).
By employing whole-brain analyses, researchers can unravel the intricate interactions and communication between segregated brain regions, shedding light on a spread of underlying mechanisms of cognitive control during ageing. Critically, it will enable the identification of specific networks or pathways that may be particularly susceptible or resilient to the effects of ageing. Furthermore, by considering the concept of cognitive reserve, which pertains to the brain’s capacity to cope with age-related decline through efficient neural processing or alternative strategies, researchers can gain insights into the individual differences observed in cognitive ageing trajectories. Finally, whole-brain approaches can potentially lead to the discovery of novel biomarkers and therapeutic targets for interventions aimed at promoting healthy cognitive ageing.
Pushing the envelope of ageing research of cognitive control also comes with major challenges. One key challenge is the need for cross-sectional and longitudinal covariation studies. Such studies are crucial for capturing the dynamic nature of cognitive control and its changes across the lifespan. Examining both within-subject changes and individual differences in cognitive control abilities over time allows for identification of age-related trajectories and the exploration of potential predictors or moderators that contribute to individual differences in cognitive ageing.
Another critical challenge lies in unravelling the interplay between maturation, senescence, plasticity, and flexibility in cognitive control processes. The question of how age-related changes in brain maturation and senescence influence the contributions of plasticity and flexibility remains unanswered. Understanding these dynamics is crucial for developing effective interventions. Future research should aim to elucidate the complex interactions between these factors, providing insights into how cognitive control can be maintained or enhanced throughout the ageing process.
In conclusion, recent studies on the effects of typical ageing on cognitive control have revealed valuable insights into the complex interplay between brain function and cognitive abilities. However, there are still important knowledge gaps that concern the complexity of these effects in the space (whole brain networks) and in time (ageing cascades). Those knowledge gaps should be addressed by integrating computational, neural, behavioural perspectives, and methods from different disciplines to push the ageing cognition research forward.
MD and OO contributed equally to conception and creation of this manuscript. Both authors contributed to manuscript writing, revision, read, and approved the submitted version.
This work was supported by the ESRC New Investigator grant ES/W009242/1, the BA Talent Award TDA21\210038, the Waterloo Foundation grant 917-4975, and the Birkbeck/Wellcome Trust Institutional Strategic Support Fund to OO.
The authors declare that the research was conducted in the absence of any commercial or financial relationships that could be construed as a potential conflict of interest.
All claims expressed in this article are solely those of the authors and do not necessarily represent those of their affiliated organizations, or those of the publisher, the editors and the reviewers. Any product that may be evaluated in this article, or claim that may be made by its manufacturer, is not guaranteed or endorsed by the publisher.
Adnan, A., Beaty, R., Silvia, P., Spreng, R. N., and Turner, G. R. (2019). Creative aging: functional brain networks associated with divergent thinking in older and younger adults. Neurobiol. Aging 75, 150–158. doi: 10.1016/j.neurobiolaging.2018.11.004
Amer, T., Campbell, K. L., and Hasher, L. (2016). Cognitive control as a double-edged sword. Trends Cogn. Sci. 20, 905–915. doi: 10.1016/j.tics.2016.10.002
Amer, T., Wynn, J. S., and Hasher, L. (2022). Cluttered memory representations shape cognition in old age. Trends Cogn. Sci. 26, 255–267. doi: 10.1016/j.tics.2021.12.002
Bacsu, J., Jeffery, B., Abonyi, S., Johnson, S., Novik, N., Martz, D., et al. (2014). Healthy aging in place: Perceptions of rural older adults. Educ. Gerontol. 40, 327–337. doi: 10.1080/03601277.2013.802191
Bherer, L., Erickson, K. I., and Liu-Ambrose, T. (2013). A review of the effects of physical activity and exercise on cognitive and brain functions in older adults. J. Aging Res. 2013:657508. doi: 10.1155/2013/657508
Blokland, A. (1995). Acetylcholine: A neurotransmitter for learning and memory? Brain Res. Rev. 21, 285–300. doi: 10.1016/0165-0173(95)00016-X
Borella, E., Delaloye, C., Lecerf, T., Renaud, O., and De Ribaupierre, A. (2009). Do age differences between young and older adults in inhibitory tasks depend on the degree of activation of information? Eur. J. Cogn. Psychol. 21, 445–472. doi: 10.1080/09541440802613997
Borella, E., Ludwig, C., Dirk, J., and De Ribaupierre, A. (2010). The influence of time of testing on interference, working memory, processing speed, and vocabulary: Age differences in adulthood. Exp. Aging Res. 37, 76–107. doi: 10.1080/0361073X.2011.536744
Braver, T. S. (2012). The variable nature of cognitive control: A dual mechanisms framework. Trends Cogn. Sci. 16, 106–113. doi: 10.1016/j.tics.2011.12.010
Braver, T. S., Krug, M. K., Chiew, K. S., Kool, W., Westbrook, J. A., Clement, N. J., et al. (2014). Mechanisms of motivation–cognition interaction: Challenges and opportunities. Cogn. Affect. Behav. Neurosci. 14, 443–472. doi: 10.3758/s13415-014-0300-0
Braver, T. S., and West, R. (2008). “Working memory, executive control, and aging,” in The handbook of aging and cognition, eds F. I. M. Craik and T. A. Salthouse (London: Psychology Press), 311–372.
Cabeza, R. (2002). Hemispheric asymmetry reduction in older adults: The HAROLD model. Psychol. Aging 17, 85–100. doi: 10.1037/0882-7974.17.1.85
Cabeza, R., Albert, M., Belleville, S., Craik, F. I., Duarte, A., Grady, C. L., et al. (2018). Maintenance, reserve and compensation: The cognitive neuroscience of healthy ageing. Nat. Rev. Neurosci. 19, 701–710. doi: 10.1038/s41583-018-0068-2
Cabeza, R., Anderson, N. D., Locantore, J. K., and McIntosh, A. R. (2002). Aging gracefully: Compensatory brain activity in high-performing older adults. Neuroimage 17, 1394–1402. doi: 10.1006/nimg.2002.1280
Campbell, K. L., Grigg, O., Saverino, C., Churchill, N., and Grady, C. L. (2013). Age differences in the intrinsic functional connectivity of default network subsystems. Front. Aging Neurosci. 5:73. doi: 10.3389/fnagi.2013.00073
Campbell, K. L., and Schacter, D. L. (2017). Ageing and the resting state: Is cognition obsolete? Lang. Cogn. Neurosci. 32, 661–668. doi: 10.1080/23273798.2016.1227858
Castel, A. D. (2007). The adaptive and strategic use of memory by older adults: Evaluative processing and value-directed remembering. Psychol. Learn. Motiv. 48, 225–270. doi: 10.1016/S0079-7421(07)48006-9
Chan, M. Y., Han, L., Carreno, C. A., Zhang, Z., Rodriguez, R. M., LaRose, M., et al. (2021). Long-term prognosis and educational determinants of brain network decline in older adult individuals. Nat. Aging 1, 1053–1067. doi: 10.1038/s43587-021-00125-4
Chan, M. Y., Park, D. C., Savalia, N. K., Petersen, S. E., and Wig, G. S. (2014). Decreased segregation of brain systems across the healthy adult lifespan. Proc. Natl. Acad. Sci. U.S.A. 111, E4997–E5006. doi: 10.1073/pnas.1415122111
Coxon, J. P., Goble, D. J., Leunissen, I., Van Impe, A., Wenderoth, N., and Swinnen, S. P. (2016). Functional brain activation associated with inhibitory control deficits in older adults. Cerebral Cortex 26, 12–22. doi: 10.1093/cercor/bhu165
Craik, F. I., Govoni, R., Naveh-Benjamin, M., and Anderson, N. D. (1996). The effects of divided attention on encoding and retrieval processes in human memory. J. Exp. Psychol. Gen. 125, 159–180. doi: 10.1037/0096-3445.125.2.159
Craik, F. I. M., and Bialystok, E. (2006). Cognition through the lifespan: Mechanisms of change. Trends Cogn. Sci. 10, 131–138. doi: 10.1016/j.tics.2006.01.007
de Frias, C. M., Dixon, R. A., and Strauss, E. (2009). Characterizing executive functioning in older special populations: From cognitively elite to cognitively impaired. Neuropsychology 23, 778–791. doi: 10.1037/a0016743
de Lange, A. M. G., Bråthen, A. C. S., Rohani, D. A., Grydeland, H., Fjell, A. M., and Walhovd, K. B. (2017). The effects of memory training on behavioral and microstructural plasticity in young and older adults. Hum. Brain Mapp. 38, 5666–5680. doi: 10.1002/hbm.23756
Denburg, N. L., and Hedgcock, W. M. (2015). “Age-associated executive dysfunction, the prefrontal cortex, and complex decision making,” in Aging and decision making, eds T. M. Hess, J. Strough, and C. E. Löckenhoff (Amsterdam: Elsevier), 79–101. doi: 10.1016/B978-0-12-417148-0.00005-4
Diamond, A. (2013). Executive functions. Annu. Rev. Psychol. 64, 135–168. doi: 10.1146/annurev-psych-113011-143750
Downey, R., Bherer, L., Pothier, K., Vrinceanu, T., Intzandt, B., Berryman, N., et al. (2022). Multiple routes to help you roam: A comparison of training interventions to improve cognitive-motor dual-tasking in healthy older adults. Front. Aging Neurosci. 14:710958. doi: 10.3389/fnagi.2022.710958
Dully, J., McGovern, D. P., and O’Connell, R. G. (2018). The impact of natural aging on computational and neural indices of perceptual decision making: A review. Behav. Brain Res. 355, 48–55. doi: 10.1016/j.bbr.2018.02.001
Fan, J. (2014). An information theory account of cognitive control. Front. Hum. Neurosci. 8:680. doi: 10.3389/fnhum.2014.00680
Forstmann, B. U., Ratcliff, R., and Wagenmakers, E.-J. (2016). Sequential sampling models in cognitive neuroscience: Advantages, applications, and extensions. Annu. Rev. Psychol. 67, 641–666. doi: 10.1146/annurev-psych-122414-033645
Gazzaley, A., Cooney, J., Rissman, J., and D’Esposito, M. (2005). Top-down suppression deficit underlies working memory impairment in normal aging. Nat. Neurosci. 8, 1298–1300. doi: 10.1038/nn1543
Geerligs, L., Renken, R. J., Saliasi, E., Maurits, N. M., and Lorist, M. M. (2015). A brain-wide study of age-related changes in functional connectivity. Cereb. Cortex 25, 1987–1999. doi: 10.1093/cercor/bhu012
Geerligs, L., Tsvetanov, K. A., and Henson, R. N. (2017). Challenges in measuring individual differences in functional connectivity using fMRI: The case of healthy aging. Hum. Brain Mapp. 38, 4125–4156. doi: 10.1002/hbm.23653
Glade, M. J. (2010). Oxidative stress and cognitive longevity. Nutrition 26, 595–603. doi: 10.1016/j.nut.2009.09.014
Glover, C. M., Yu, L., Stewart, C. C., Wilson, R. S., Bennett, D. A., and Boyle, P. A. (2021). The association of late life cognitive activity with healthcare and financial decision-making in community-dwelling, nondemented older adults. Am. J. Geriatr. Psychiatry 29, 117–125. doi: 10.1016/j.jagp.2020.06.010
Grady, C. (2012). Trends in neurocognitive aging. Nat. Revi. Neurosci. 13, 491–505. doi: 10.1038/nrn3256
Gutchess, A. (2014). Plasticity of the aging brain: New directions in cognitive neuroscience. Science 346, 579–582. doi: 10.1126/science.1254604
Hasher, L., and Zacks, R. T. (1988). Working memory, comprehension, and aging: A review and a new view. Psychol. Learn. Motiv. 22, 193–225. doi: 10.1016/S0079-7421(08)60041-9
Haykin, S., Fatemi, M., Setoodeh, P., and Xue, Y. (2012). Cognitive control. Proc. IEEE 100, 3156–3169. doi: 10.1109/JPROC.2012.2215773
Heckner, M. K., Cieslik, E. C., Eickhoff, S. B., Camilleri, J. A., Hoffstaedter, F., and Langner, R. (2021). The aging brain and executive functions revisited: Implications from meta-analytic and functional-connectivity evidence. J. Cogn. Neurosci. 33, 1716–1752. doi: 10.1162/jocn_a_01616
Hoogendam, Y. Y., van der Lijn, F., Vernooij, M. W., Hofman, A., Niessen, W. J., van der Lugt, A., et al. (2014). Older age relates to worsening of fine motor skills: A population-based study of middle-aged and elderly persons. Front. Aging Neurosci. 6:259. doi: 10.3389/fnagi.2014.00259
Insel, K. C., Merkle, C. J., Hsiao, C.-P., Vidrine, A. N., and Montgomery, D. W. (2012). Biomarkers for cognitive aging part I: Telomere length, blood pressure and cognition among individuals with hypertension. Biol. Res. Nurs. 14, 124–132. doi: 10.1177/1099800411406433
Jimura, K., and Braver, T. S. (2010). Age-related shifts in brain activity dynamics during task switching. Cereb. Cortex 20, 1420–1431. doi: 10.1093/cercor/bhp206
Jost, K., Bryck, R. L., Vogel, E. K., and Mayr, U. (2011). Are old adults just like low working memory young adults? Filtering efficiency and age differences in visual working memory. Cereb. Cortex 21, 1147–1154. doi: 10.1093/cercor/bhq185
Jurado, M. B., and Rosselli, M. (2007). The elusive nature of executive functions: A review of our current understanding. Neuropsychol. Rev. 17, 213–233. doi: 10.1007/s11065-007-9040-z
Kliegel, M., Martin, M., McDaniel, M. A., and Phillips, L. H. (2007). Adult age differences in errand planning: The role of task familiarity and cognitive resources. Exp. Aging Res. 33, 145–161. doi: 10.1080/03610730601177395
Knowlton, B. J., and Castel, A. D. (2022). Memory and reward-based learning: A value-directed remembering perspective. Annu. Rev. Psychol. 73, 25–52. doi: 10.1146/annurev-psych-032921-050951
Korotkevich, Y., Trewartha, K. M., Penhune, V. B., and Li, K. Z. (2015). Effects of age and cognitive load on response reprogramming. Exp. Brain Res. 233, 937–946. doi: 10.1007/s00221-014-4169-5
Köstering, L., Leonhart, R., Stahl, C., Weiller, C., and Kaller, C. P. (2016). Planning decrements in healthy aging: Mediation effects of fluid reasoning and working memory capacity. J. Gerontol. Ser. B Psychol. Sci. Soc. Sci. 71, 230–242. doi: 10.1093/geronb/gbu107
Kramer, A. F., and Willis, S. L. (2002). Enhancing the cognitive vitality of older adults. Cur. Direct. Psychol. Sci. 11, 173–177. doi: 10.1111/1467-8721.00194
Kühn, S., and Lindenberger, U. (2016). “Research on human plasticity in adulthood: A lifespan agenda,” in Handbook of the psychology of aging, eds K. W. Schaie and S. L. Willis (Cambridge, MA: Academic Press.), 105–123. doi: 10.1016/B978-0-12-411469-2.00006-6
La Corte, V., Sperduti, M., Malherbe, C., Vialatte, F., Lion, S., Gallarda, T., et al. (2016). Cognitive decline and reorganization of functional connectivity in healthy aging: The pivotal role of the salience network in the prediction of age and cognitive performances. Front. Aging Neurosci. 8:204. doi: 10.3389/fnagi.2016.00204
Lenehan, M. E., Summers, M. J., Saunders, N. L., Summers, J. J., and Vickers, J. C. (2015). Relationship between education and age-related cognitive decline: A review of recent research. Psychogeriatrics 15, 154–162. doi: 10.1111/psyg.12083
Li, K. Z., Lindenberger, U., Freund, A. M., and Baltes, P. B. (2001). Walking while memorizing: Age-related differences in compensatory behavior. Psychol. Sci. 12, 230–237. doi: 10.1111/1467-9280.00341
Li, S. C., Lindenberger, U., and Frensch, P. A. (2000). Unifying cognitive aging: From neuromodulation to representation to cognition. Neurocomputing 32, 879–890. doi: 10.1016/S0925-2312(00)00256-3
Lindenberger, U. (2014). Human cognitive aging: Corriger la fortune? Science 346, 572–578. doi: 10.1126/science.1254403
Lindenberger, U. (2018). “Plasticity beyond early development: Hypotheses and questions,” in Emergent brain dynamics: Prebirth to adolescence, 1st Edn, eds A. A. Benasich and U. Ribary (Cambridge, MA: MIT Press), 207–223. doi: 10.7551/mitpress/11957.003.0015
Lindenberger, U., and Baltes, P. B. (1997). Intellectual functioning in old and very old age: Cross-sectional results from the Berlin aging study. Psychol. Aging 12, 410–432. doi: 10.1037/0882-7974.12.3.410
Lindenberger, U., and Lövdén, M. (2019). Brain plasticity in human lifespan development: The exploration–selection–refinement model. Annu. Rev. Dev. Psychol. 1, 197–222. doi: 10.1146/annurev-devpsych-121318-085229
Lövdén, M., Bodammer, N. C., Kühn, S., Kaufmann, J., Schütze, H., Tempelmann, C., et al. (2010a). Experience-dependent plasticity of white-matter microstructure extends into old age. Neuropsychologia 48, 3878–3883. doi: 10.1016/j.neuropsychologia.2010.08.026
Lövdén, M., Bäckman, L., Lindenberger, U., Schaefer, S., and Schmiedek, F. (2010b). A theoretical framework for the study of adult cognitive plasticity. Psychol. Bull. 136, 659–676. doi: 10.1037/a0020080
Ludwig, C., Borella, E., Tettamanti, M., and De Ribaupierre, A. (2010). Adult age differences in the color Stroop test: A comparison between an Item-by-item and a blocked version. Arch. Gerontol. Geriatr. 51, 135–142. doi: 10.1016/j.archger.2009.09.040
Lugtmeijer, S., Geerligs, L., Tsvetanov, K. A., Mitchell, D. J., and Campbell, K. L. (2023). Lifespan differences in visual short-term memory load-modulated functional connectivity. NeuroImage 270:119982. doi: 10.1016/j.neuroimage.2023.119982
Madden, D. J., Costello, M. C., Dennis, N. A., Davis, S. W., Shepler, A. M., Spaniol, J., et al. (2010). Adult age differences in functional connectivity during executive control. Neuroimage 52, 643–657. doi: 10.1016/j.neuroimage.2010.04.249
Malagurski, B., Liem, F., Oschwald, J., Mérillat, S., and Jäncke, L. (2020). Functional dedifferentiation of associative resting state networks in older adults–a longitudinal study. Neuroimage 214:116680. doi: 10.1016/j.neuroimage.2020.116680
Maldonado, T., Orr, J. M., Goen, J. R., and Bernard, J. A. (2020). Age differences in the subcomponents of executive functioning. J. Gerontol. Ser. B 75, e31–e55. doi: 10.1093/geronb/gbaa005
Mayas, J., Fuentes, L., and Ballesteros, S. (2012). Stroop interference and negative priming (NP) suppression in normal aging. Arch. Gerontol. Geriatr. 54, 333–338. doi: 10.1016/j.archger.2010.12.012
McDowd, J. M., and Craik, F. I. (1988). Effects of aging and task difficulty on divided attention performance. J. Exp. Psychol. 14, 267–280. doi: 10.1037/0096-1523.14.2.267
Migeot, J., Calivar, M., Granchetti, H., Ibáñez, A., and Fittipaldi, S. (2022). Socioeconomic status impacts cognitive and socioemotional processes in healthy ageing. Sci. Rep. 12, 1–11. doi: 10.1038/s41598-022-09580-4
Nyberg, L., Lövdén, M., Riklund, K., Lindenberger, U., and Bäckman, L. (2012). Memory aging and brain maintenance. Trends Cogn. Sci. 16, 292–305. doi: 10.1016/j.tics.2012.04.005
Oakes, L. M., and Rakison, D. H. (2019). Developmental cascades: Building the infant mind. New York, NY: Oxford University Press. doi: 10.1093/oso/9780195391893.001.0001
Ossmy, O., and Adolph, K. E. (2020). Real-time assembly of coordination patterns in human infants. Curr. Biol. 30, 4553–4562. doi: 10.1016/j.cub.2020.08.073
Park, D. C., and McDonough, I. M. (2013). The dynamic aging mind: Revelations from functional neuroimaging research. Perspect. Psychol. Sci. 8, 62–67. doi: 10.1177/1745691612469034
Park, D. C., and Reuter-Lorenz, P. (2009). The adaptive brain: Aging and neurocognitive scaffolding. Annu. Rev. Psychol. 60, 173–196. doi: 10.1146/annurev.psych.59.103006.093656
Persad, C. C., Abeles, N., Zacks, R. T., and Denburg, N. L. (2002). Inhibitory changes after age 60 and the relationship to measures of attention and memory. J. Gerontol. Ser. B Psychol. Sci. Soc. Sci. 57, 223–232. doi: 10.1093/geronb/57.3.P223
Raz, N., Lindenberger, U., Rodrigue, K. M., Kennedy, K. M., Head, D., Williamson, A., et al. (2005). Regional brain changes in aging healthy adults: General trends, individual differences and modifiers. Cereb. Cortex 15, 1676–1689. doi: 10.1093/cercor/bhi044
Reuter-Lorenz, P. A., and Cappell, K. A. (2008). Neurocognitive aging and the compensation hypothesis. Curr. Direct. Psychol. Sci. 17, 177–182. doi: 10.1111/j.1467-8721.2008.00570.x
Reuter-Lorenz, P. A., Festini, S. B., and Jantz, T. K. (2021). “Executive functions and neurocognitive aging,” in Handbook of the psychology of aging, eds K. W. Schaie and S. L. Willis (Cambridge, MA: Academic Press), 67–81. doi: 10.1016/B978-0-12-816094-7.00019-2
Reuter-Lorenz, P. A., and Park, D. C. (2014). How does it STAC up? Revisiting the scaffolding theory of aging and cognition. Neuropsychol. Rev. 24, 355–370. doi: 10.1007/s11065-014-9270-9
Rey-Mermet, A., and Gade, M. (2018). Inhibition in aging: What is preserved? What declines? A meta-analysis. Psychon. Bull. Rev. 25, 1695–1716. doi: 10.3758/s13423-017-1384-7
Rosselli, M., and Torres, V. L. (2019). “Executive dysfunction during normal and abnormal aging,” in Dysexecutive syndromes: Clinical and experimental perspectives, eds A. Ardila, S. Fatima, and M. Rosselli (Berlin: Springer Nature), 155–175. doi: 10.1007/978-3-030-25077-5_8
Royall, D. R., Palmer, R., Chiodo, L. K., and Polk, M. J. (2005). Normal rates of cognitive change in successful aging: The freedom house study. J. Int. Neuropsychol. Soc. 11, 899–909. doi: 10.1017/s135561770505109x
Rozas, A. X. P., Juncos-Rabadán, O., and González, M. S. R. (2008). Processing speed, inhibitory control, and working memory: Three important factors to account for age-related cognitive decline. Int. J. Aging Hum. Dev. 66, 115–130. doi: 10.2190/AG.66.2.b
Salthouse, T. A. (2009). When does age-related cognitive decline begin? Neurobiol. Aging 30, 507–514. doi: 10.1016/j.neurobiolaging.2008.09.023
Salthouse, T. A., Atkinson, T. M., and Berish, D. E. (2003). Executive functioning as a potential mediator of age-related cognitive decline in normal adults. J. Exp. Psychol. Gen. 132, 566–594. doi: 10.1037/0096-3445.132.4.566
Schmitz, T. W., Cheng, F. H., and De Rosa, E. (2010). Failing to ignore: Paradoxical neural effects of perceptual load on early attentional selection in normal aging. J. Neurosci. 30, 14750–14758. doi: 10.1523/JNEUROSCI.2687-10.2010
Sorel, O., and Pennequin, V. (2008). Aging of the planning process: The role of executive functioning. Brain Cogn. 66, 196–201. doi: 10.1016/j.bandc.2007.07.006
Spieler, D. H., Mayr, U., and LaGrone, S. (2006). Outsourcing cognitive control to the environment: Adult age differences in the use of task cues. Psychon. Bull. Rev. 13, 787–793. doi: 10.3758/bf03193998
Spreng, R. N., and Turner, G. R. (2019). The shifting architecture of cognition and brain function in older adulthood. Perspect. Psychol. Sci. 14, 523–542. doi: 10.1177/1745691619827511
Stern, Y. (2002). What is cognitive reserve? Theory and research application of the reserve concept. J. Int. Neuropsychol. Soc. 8, 448–460. doi: 10.1017/S1355617702813248
Strout, K., Ahmed, F., Sporer, K., Howard, E. P., Sassatelli, E., and Mcfadden, K. (2018). What are older adults wellness priorities? A qualitative analysis of priorities within multiple domains of wellness. Healthy Aging Res. 7:e21. doi: 10.1097/HXR.0000000000000021
Thornton, W. J., and Dumke, H. A. (2005). Age differences in everyday problem-solving and decision-making effectiveness: A meta-analytic review. Psychol. Aging 20, 85–99. doi: 10.1037/0882-7974.20.1.85
Vallesi, A., McIntosh, A. R., and Stuss, D. T. (2011). Overrecruitment in the aging brain as a function of task demands: Evidence for a compensatory view. J. Cogn. Neurosci. 23, 801–815. doi: 10.1162/jocn.2010.21490
Vaughan, L., and Giovanello, K. (2010). Executive function in daily life: Age-related influences of executive processes on instrumental activities of daily living. Psychol. Aging 25, 343–355. doi: 10.1037/a0017729
Verhaeghen, P. (2011). Aging and executive control: Reports of a demise greatly exaggerated. Curr. Dir. Psychol. Sci. 20, 174–180. doi: 10.1177/0963721411408772
Verhaeghen, P., and Cerella, J. (2002). Aging, executive control, and attention: A review of meta-analyses. Neurosci. Biobehav. Rev. 26, 849–857. doi: 10.1016/S0149-7634(02)00071-4
Wang, S., Williams, J., and Wilmut, K. (2020). Constraints on motor planning across the life span: Physical, cognitive, and motor factors. Psychol. Aging 35, 421–433. doi: 10.1037/pag0000408
West, R. L. (1996). An application of prefrontal cortex function theory to cognitive aging. Psychol. Bull. 120, 272–292. doi: 10.1037/0033-2909.120.2.272
Wiles, J. L., Leibing, A., Guberman, N., Reeve, J., and Allen, R. E. (2012). The meaning of “aging in place” to older people. Gerontol. 52, 357–366. doi: 10.1093/geront/gnr098
Wilson, R. S., Beckett, L. A., Barnes, L. L., Schneider, J. A., Bach, J., Evans, D. A., et al. (2002). Individual differences in rates of change in cognitive abilities of older persons. Psychol. Aging 17, 179–193. doi: 10.1037/0882-7974.17.2.179
Woods, S. P., Weinborn, M., Velnoweth, A., Rooney, A., and Bucks, R. S. (2012). Memory for intentions is uniquely associated with instrumental activities of daily living in healthy older adults. J. Int. Neuropsychol. Soc. 18, 134–138. doi: 10.1017/S1355617711001263
Wunsch, K., Weigelt, M., and Stöckel, T. (2017). Anticipatory motor planning in older adults. J. Gerontol. Ser. B 72, 373–382.
Zanto, T. P., and Gazzaley, A. (2017). “Cognitive control and the ageing brain,” in The Wiley handbook of cognitive control, ed. T. Egner (Hoboken, NJ: John Wiley & Sons), 476–490. doi: 10.1002/9781118920497.ch27
Zelazo, P. D., Craik, F. I., and Booth, L. (2004). Executive function across the life span. Acta Psychol. 115, 167–183. doi: 10.1016/j.actpsy.2003.12.005
Keywords: cognitive control, executive functions, ageing, planning, cognitive decline, cognitive development
Citation: Dexter M and Ossmy O (2023) The effects of typical ageing on cognitive control: recent advances and future directions. Front. Aging Neurosci. 15:1231410. doi: 10.3389/fnagi.2023.1231410
Received: 30 May 2023; Accepted: 13 July 2023;
Published: 28 July 2023.
Edited by:
Ian M. McDonough, The University of Alabama, United StatesReviewed by:
Karen Campbell, Brock University, CanadaCopyright © 2023 Dexter and Ossmy. This is an open-access article distributed under the terms of the Creative Commons Attribution License (CC BY). The use, distribution or reproduction in other forums is permitted, provided the original author(s) and the copyright owner(s) are credited and that the original publication in this journal is cited, in accordance with accepted academic practice. No use, distribution or reproduction is permitted which does not comply with these terms.
*Correspondence: Ori Ossmy, b3JpLm9zc215QGJiay5hYy51aw==
Disclaimer: All claims expressed in this article are solely those of the authors and do not necessarily represent those of their affiliated organizations, or those of the publisher, the editors and the reviewers. Any product that may be evaluated in this article or claim that may be made by its manufacturer is not guaranteed or endorsed by the publisher.
Research integrity at Frontiers
Learn more about the work of our research integrity team to safeguard the quality of each article we publish.