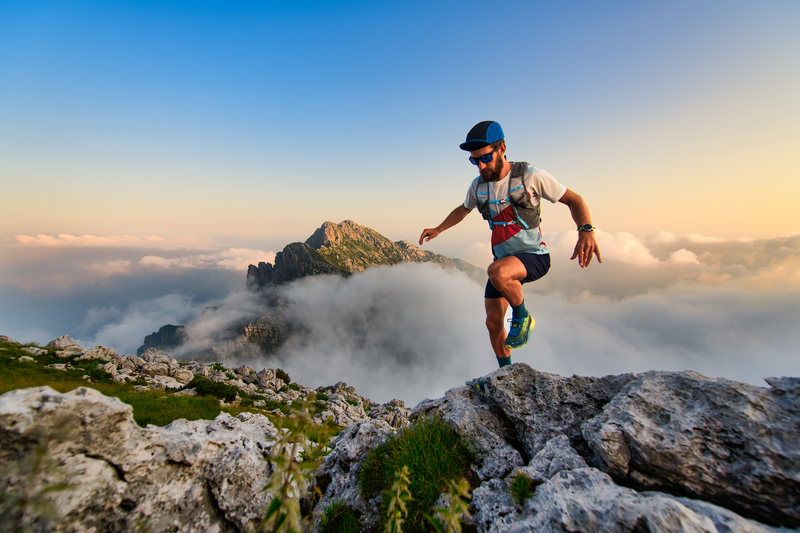
95% of researchers rate our articles as excellent or good
Learn more about the work of our research integrity team to safeguard the quality of each article we publish.
Find out more
ORIGINAL RESEARCH article
Front. Aging Neurosci. , 06 September 2023
Sec. Neuroinflammation and Neuropathy
Volume 15 - 2023 | https://doi.org/10.3389/fnagi.2023.1227203
Introduction: Advanced age is a significant factor in changes to brain physiology and cognitive functions. Recent research has highlighted the critical role of the gut microbiome in modulating brain functions during aging, which can be influenced by various factors such as apolipoprotein E (APOE) genetic variance, body mass index (BMI), diabetes, and dietary intake. However, the associations between the gut microbiome and these factors, as well as brain structural, vascular, and metabolic imaging markers, have not been well explored.
Methods: We recruited 30 community dwelling older adults between age 55-85 in Kentucky. We collected the medical history from the electronic health record as well as the Dietary Screener Questionnaire. We performed APOE genotyping with an oral swab, gut microbiome analysis using metagenomics sequencing, and brain structural, vascular, and metabolic imaging using MRI.
Results: Individuals with APOE e2 and APOE e4 genotypes had distinct microbiota composition, and higher level of pro-inflammatory microbiota were associated higher BMI and diabetes. In contrast, calcium- and vegetable-rich diets were associated with microbiota that produced short chain fatty acids leading to an anti-inflammatory state. We also found that important gut microbial butyrate producers were correlated with the volume of the thalamus and corpus callosum, which are regions of the brain responsible for relaying and processing information. Additionally, putative proinflammatory species were negatively correlated with GABA production, an inhibitory neurotransmitter. Furthermore, we observed that the relative abundance of bacteria from the family Eggerthellaceae, equol producers, was correlated with white matter integrity in tracts connecting the brain regions related to language, memory, and learning.
Discussion: These findings highlight the importance of gut microbiome association with brain health in aging population and could have important implications aimed at optimizing healthy brain aging through precision prebiotic, probiotic or dietary interventions.
Aging is associated with significant alterations in brain structure, blood flow, and metabolism, leading to declines in cognitive function and increased risk for neurodegenerative diseases. The most notable structural change is brain atrophy, particularly in the hippocampus and other regions associated with memory and cognitive processes (Raz and Rodrigue, 2006; Fjell and Walhovd, 2010). Aging also leads to a decline in white matter (WM) integrity through various processes, including myelin degradation, and the development of white matter lesions (Molloy et al., 2021). Cerebral blood flow (CBF) is another critical aspect of brain function that changes with age. Older adults typically exhibit reduced CBF, which can negatively impact cognition (Tarumi and Zhang, 2018). Aging also affects brain metabolism, with older individuals displaying reduced glucose and oxygen metabolic rates, and the production of essential brain metabolites (Lin et al., 2014; Brinton et al., 2015; Mattson and Arumugam, 2018; Turner et al., 2022).
Accumulating evidence has implicated the gut microbiome in brain health (Cox and Weiner, 2018). Gut dysbiosis is associated with many neurological disorders, including Alzheimer’s disease, stroke, and traumatic brain injury (Vogt et al., 2017; Borsom et al., 2020; Yanckello et al., 2022a; Hammond et al., 2022b). Aging is associated with significant alterations in the gut microbiome (Hoffman et al., 2017). One prominent age-related change is the decline of beneficial bacteria, such as Bifidobacteria, and an increase in opportunistic pathogens like bacteria from the phylum Proteobacteria (Biagi et al., 2010). This shift may contribute to chronic low-grade inflammation, known as “inflammaging,” which has been linked to various age-related conditions, including cardiovascular diseases, cognitive decline, and metabolic disorders (Franceschi and Campisi, 2014). Additionally, older adults often experience reduced production of short-chain fatty acids (SCFAs) due to changes in the gut microbiota (Badal et al., 2020). SCFAs play essential roles in maintaining gut health, immune function, and metabolism. The decline in SCFAs production may contribute to age-related immune dysregulation and increases susceptibility to infections and chronic diseases (Buford, 2017).
There are other factors that may impact gut-brain interactions in aging, such as apolipoprotein E (APOE) variance, diet, body mass index (BMI), obesity, and diabetes. Individuals with APOE ε4 alleles (APOE4) display altered brain function, such as reduced amyloid-beta clearance (Castellano et al., 2011) and increased inflammation (Shi et al., 2017). Recent studies show connections between the APOE4 genotype and gut microbiome composition, with APOE4 carriers exhibiting increased pro-inflammatory microbes (Hoffman et al., 2019; Tran et al., 2019; Parikh et al., 2020; Yanckello et al., 2022b). Diet, BMI, and diabetes all have significant impacts on brain function and the gut microbiome (Asadi et al., 2022; Wachsmuth et al., 2022). A diet high in sugar and saturated fat can contribute to inflammation in the brain, impairing cognitive function and increasing the risk of dementia (Gómez-Pinilla, 2008). Additionally, obesity and diabetes are associated with decreased cognitive performance, and an altered gut microbiome, which can contribute to inflammation and neurodegenerative diseases (Arnoldussen et al., 2014; Gomes et al., 2014).
While there is growing evidence to support a link between the gut microbiome and brain health in aging, the specific associations between microbiome composition, brain imaging features, APOE genotype, BMI, diabetes, and dietary intake remain unclear. To shed light on these relationships, we performed an analysis of 30 community-swelling older adults. Our study aims to establish a foundation for understanding how gut microbes are associated with brain health. These findings could have important implications for developing precision nutrition interventions to optimize healthy brain aging older adults.
We recruited participants for this study in Kentucky, United States. The inclusion criteria for the study required that participants be between age 55–85. Exclusion criteria required that participants not have an acute disease of chronic, clinically significant (unresolved, requiring on-going medical management or medication) pulmonary, gastrointestinal, dermatologic, hepatic, or renal functional abnormality. Participants were required to not have had cancer or a positive test for HIV, HBV, or HCV. Participants were required to not be immunosuppressed or have had major surgery of the GI tract in the past 5 years. Participants also had to be MRI compatible. The sample size was determined based on the relative abundance comparisons of the gut microbiome with the highly specific species level resolution provided by whole genome sequencing technology. With alpha = 0.05, a sample size of 30 and continuous variables, we will have 80% power to detect Pearson correlation coefficient ρ of 50% or more. Although the pandemic has imposed challenges for recruitment and retention, especially for the aging population, we were able to complete the study with 30 participants by taking into consideration the population distribution of Kentucky – 85.08% White Non-Hispanic and 7.88% Black or African American Non-Hispanic (Source: US Census 2017 5-Year American Community Survey). Participants were recruited through researchmatch.org and from advertisements posted by the Center for Clinical and Translational Science at the University of Kentucky. Table 1 describes the major demographic characteristics of our participants known to influence brain aging, with a mean age of 65.7 years, 17.23 years of education, and BMI of 26.95 kg/m2. Our population was 83.33% female, 90% white, 10% had diabetes, 43.33% had hypertension, and 30% had hyperlipidemia. 53% were genotype APOE ε3/ε3, 33% were ε4 carriers, and 13% were ε2 carriers. Participants were required to not all research activities were monitored by the Institutional Review Board at the University of Kentucky.
Each individual received a verbal and written explanation of the purposes, procedures, and potential hazards of the study, and written consent was obtained. Study personnel consented subjects using the University of California, San Diego Brief Assessment of Capacity to Consent (UBACC) to ensure capacity. Signed consent was obtained before definite enrollment of the subject in this study. Following consent, we obtained past medical history from the electronic health record and questionnaires. These data were used solely for research purposes. Variables including age, gender, racial/ethnic background, education level, Body Mass Index, history of conventional vascular risk factors (hypertension, diabetes mellitus, atrial fibrillation, hyperlipoproteinemia, and smoking habit), pre-stroke therapy, and acute treatment (i.e., oral anticoagulants, antiplatelet agents, tPA, IV thrombolysis and/or mechanical thrombectomy, and/or antibiotics) were recorded and used as covariates for our analyses.
Stool samples were collected in Zymo DNA stabilization solution with Sarstedt feces tubes from feces catcher placed on toilet seat. Genomic DNA was extracted from 0.25 grams of stool using ZymoBIOMICS™ DNA Mini Kit and shipped to the Genomics and Microbiome Core Facility (GMCF) at Rush University. Shotgun metagenome libraries were constructed using an Illumina DNA prep kit according to the manufacturer’s instructions. Deep sequencing of libraries was performed on an Illumina NovaSeq6000 instrument, using paired end 2 × 150 base sequencing reads. Unassembled sequencing reads were analyzed by the Research Informatics Core at the University of Illinois Chicago for microbiome analysis. We used MetaPhlAn (Metagenomic Phylogenetic Analysis) to profile the composition of microbial communities using unique clade-specific marker genes identified from ~17,000 reference genomes (~13,500 bacterial and archaeal, ~3,500 viral, and ~ 110 eukaryotic) (Beghini et al., 2021).
We assessed diet history using the Dietary Screener Questionnaire in the National Health and Nutrition Examination Survey (DSQ) (NHANES 2009-10). DSQ was used to measure dietary intake over the last month to include estimated intake of fiber, calcium, whole grains, sugar, dairy, fruits and vegetables, and sugar sweetened beverages.
We collected oral swabs from all participants and placed them in Zymo DNA stabilization solution. We sent the oral swabs to the Research Informatics Core at the University of Illinois Chicago for DNA extraction and amplification. The Core performed PCR to amplify and measure SNPs rs429358 and rs7412 that define the common allelic variants of Apolipoprotein E.
MRI images were collected from all participants on a 3 T Prisma MR scanner (Siemens, Germany) at UK’s Magnetic Resonance Imaging & Spectroscopy Center.
High-resolution, 3D anatomic images were acquired using an MP-RAGE sequence [repetition time (TR) = 2,530 ms, echo time (TE) = 2.26 ms, flip angle (FA) = 7°, 1 mm isotropic voxels, 6:19 min]. We used the FreeSurfer Software Suite to segment and quantify brain volumes.
Brain metabolites were measured with a chemical shift imaging (CSI) sequence that incorporates localization by adiabatic selective refocusing (LASER) for FOV-reduction (McNab and Bartha, 2006). Semi-laser sequence [TR = 1700 ms and TE = 40 ms]. All MRS slices were placed parallel to the anterior commissure-posterior commissure line. The volume of interest was centered to the medial to posterior part of the corpus callosum, with VOI = 80 (l-r) × 80 (a-p) and field of view (FOV) = 160 × 160 mm3. The acquired matrix size was 10 × 10 × 15 mm. Two voxels that contained pure white matter were selected from each participant and averaged for analysis. Spectra were calculated using LCModel Software (Provencher, 1993) to determine the concentration of brain metabolites.
Quantitative CBF (with units of mL/g per minute) was measured using a pulsed arterial spin labeling (PASL) PICORE Q2T sequence with a TR = 4,400 ms and a TE = 20.8 ms. We used FreeSurfer software to process relative cerebral blood flow (relCBF) data produced by the Siemens scanner.1 The automated software provides relCBF averages over the FreeSurferColorLUT.txt set of ROIs.
White matter integrity was measured using an axial double refocused spin echo, echo planar imaging (Yang et al., 2015) Diffusion Tensor Imaging (DTI) sequence with the following parameters: TR = 3,400 ms, TE = 71 ms, field of view = 232 mm, 81 slices, 2 mm isotropic resolution. The DTI images were acquired with 128 noncollinear encoding directions (b = 2000 s/mm2) and 6 images without diffusion weighting (b = 0 s/mm2, b0). DTI data were analyzed with FSL (Functional MRI of the Brain software library, FMRIB) to calculate fractional anisotropy (FA) values.
All statistical analyses were completed using JMP Statistical Software (SAS, Cary, NC, USA) and R Statistical Software (R Core Team, 2020). Relative abundance comparisons were utilized to provide detailed analyses of the samples concordant with the highly specific species level resolution provided by whole genome sequencing technology. Shapiro-Wilks testing revealed that there were no significant departures from normality. For all comparisons, Two-sample t-test and two-way ANOVA were used to determine differences between groups with a continuous response. The MaAsLin2 R Package was used to normalize all variables and employ linear regression analysis to associate various variables with microbiome measures (Mallick et al., 2021). A false discovery rate (FDR) of q < 0.25 was used as part of our exploratory approach of identifying differentially abundant microbial features due to a small dataset. With alpha = 0.05, a sample size of 30 and continuous variables, we will have 80% power to detect Pearson correlation coefficient ρ of 50% or more.
We detected significant associations between gut microbial community structure with APOE genotype, BMI, diabetes, and hypertension in our community dwelling older adult cohort. The relative abundance of bacterial species from the phylum Firmicutes, including Eubacterium eligens (Figure 1A), Oscillibacter (Figure 1B), and Faecalibacterium prausnitzii (Figure 1C), were all lower in the APOE ε2/ε3 (APOE2 carriers) genotype and that of the genus Lachnoclostridium was higher (Figure 1D). In the APOE ε3/ε4 (APOE4 carriers) genotype, the relative abundance of Roseburia (Figure 1E) and Holdemania filiformis (Figure 1F), both from the phylum Firmicutes, was lower. The relative abundance of Bacteroides dorei (phylum Bacteroidetes) was higher (Figure 1G), while that of Akkermansia muciniphila (phylum Verrucomicrobia) was lower in obesity (Figure 1H). The relative abundance of Escherichia coli (E. coli; phylum Proteobacteria) was higher in participants with diabetes (Figure 1I). The relative abundance of Phascolarctobacterium (phylum Firmicutes) was lower in participants with hypertension (Figure 1J).
Figure 1. Microbial taxa associated with demographic features. The Apolipoprotein E (APOE) ε2 genotype was associated with lower (A) Eubacterium eligens, (B) the genus Oscillibacter, and (C) Faecalibacterium prausnitzii and higher (D) genus Lachnoclostridium. The APOE ε4 genotype was associated with lower (E) genus Roseburia and (F) Holdemania filiformis. Obesity was associated with higher (G) Bacteroides dorei and lower (H) Akkermansia muciniphila. Diabetes was associated with higher (I) Escherichia coli (E. coli). Hypertension was associated with lower (J) genus Phascolarctobacterium.
We measured dietary intake over the previous month using the Dietary Screener Questionnaires (DSQ) in the National Health and Nutrition Examination Survey (NHANES) 2009-10. While there were no significant associations with fiber, whole grain, added sugar, dairy, significant associations existed with calcium intake and fruit and vegetable intake. An increased intake of calcium was associated a higher relative abundance of Bifidobacterium adolescentis, Eubacterium eligens, the family Acidaminococcaceae, and Haemophilus parainfluenzae (Figures 2A–D) and a lower abundance of Clostridium asparagiforme, Eubacterium ventriosum, and Sellimonas intestinalis (Figures 2E–G). An increased intake of vegetable was positively associated Agathobaculum butyriciproducens (Figure 2H), and negatively associated with Eggerthella lenta (Figure 2I).
Figure 2. Microbial taxa associations with dietary calcium and vegetable intakes. Calcium intake is positively associated with (A) Bifidobacterium adolescentis, (B) Eubacterium eligens, (C) family Acidaminococcaceae, and (D) Haemophilus parainfluenzae, but negatively associated with (E) Clostridium asparagiforme, (F) Eubacterium ventriosum, and (G) Sellimonas intestinalis. Vegetable intake is positively associated with (H) Agathobaculum butyriciproducens, but negatively associated with (I) Eggerthella lenta.
The relative abundance of Bacteroides ovatus was positively associated with the thalamus volume (Figure 3A) and that of Bacteroides uniformis was negatively associated with white matter hypointensities (Figure 3B). The relative abundance of bacteria from the family Acidaminococcaceae was positively associated the volume of the mid posterior portion of the corpus callosum (Figure 3C).
Figure 3. Microbial taxa associations with structural imaging and brain metabolites in the white matter of the corpus callosum. (A) The thalamus volume is positively associated with Bacteroides ovatus abundance. (B) White Matter (WM) hypointensities are positively associated with Bacteroides uniformis abundance. (C) The volume of the mid-posterior portion of the corpus callosum (CC_Mid_Posterior) is positively associated with the family Acidaminococcaceae abundance. (D) Gamma-aminobutyric acid (GABA) is negatively associated with the genus Collinsella and (E) positively associated with Parasutterella excrementihominis. (F) Glycerophosphorylcholine (GPC) is positively associated with Alistipes putredinis and (G) the family Acidaminococcaceae. (H) N-acetylaspartate (NAA) and N-acetylaspartylglutamate (NAAG) are positively associated with Ruminococcus lactaris.
The relative abundance Collinsella aerofaciens (q < 0.05) was negatively associated with gamma-aminobutyric acid (GABA) (Figure 3D) and that of Parasutterella excrementihominis was positively correlated with GABA (Figure 3E). The relative abundance of Alistipes putredinis and that of the family Acidaminococcaceae were positively correlated with glycerophosphocholine (GPC) (Figures 3F,G). The relative abundance of Ruminococcus lactaris was positively correlated with N-acetylaspartate and N-acetylaspartylglutamate (NAA + NAAG) (Figure 3H).
Several taxa from the Actinobacteria were correlated with white matter integrity. These included the family Eggerthellaceae (Figure 4A), which was positively correlated with white matter tracts in the middle cerebellar peduncle and the left external capsule, which contains corticocortical association fibers. Specifically, the relative abundance of Gordonibacter pamelaeae (Figures 4B–E), Asaccharobacter celatus (Figure 4F), and Adlercreutzia equolifaciens (Figures 4G–I), were positively correlated with several tracts that connects brain regions responsible for language function and the limbic system. In the Bacteroidetes phylum, the relative abundance of Coprobacter fastidiosus was negatively correlated with the superior longitudinal fasciculus and that of Bacteroides uniformis was positively correlated with the tapetum. The Firmicutes phylum has several taxa that were positively correlated with white matter tracts, including Clostridium innocuum (Figure 5A), the genus Lactobacillus (Figure 5B), Lachnospira pectinoschiza (Figure 5C), Roseburia hominis (Figures 5D,E).
Figure 4. Species from the Eggerthellaceae family are positively correlated with white matter integrity (WMI) in language, memory, and limbic brain circuits. Eggerthellaceae spp. are positively associated with WMI in (A) the external capsule, (B) the middle cerebellar peduncle, (C) the corticospinal tract, (D) the tapetum, (E) the pontine crossing tract, (F,G) corticospinal tracts, (H) the fornix, and (I) the inferior fronto-occipital fasciculus.
Figure 5. Notable microbial taxa associations with white matter integrity (WMI) in limbic and memory regions. (A) Clostridium innocuum is positively associated with WMI in the corticospinal tract. (B) The genus Lactobacillus is positively associated with WMI in the medial lemniscus. (C) Lachnospira pectinoschiza is positively associated with WMI in the medial lemniscus. Roseburia hominis is positively associated with WMI in (D) the cingulum and (E) the inferior fronto-occipital fasciculus.
Table 2 shows the microbial taxa that were associated with CBF in various brain regions. The relative abundance of Collinsella stercoris, a bile-acid conjugator (Doden et al., 2021), was positively correlated with brain regions related language function and the limbic system, such as temporal cortex. The relative abundance of bacteria from the genus, part of the Bacteroidetes phylum and related to altered dopamine signaling through its synthetization of GABA (Strandwitz et al., 2019; Kramer et al., 2020), were negatively correlated with CBF in the basal ganglia regions and hippocampal regions, both implicated in learning and memory. In addition, the relative abundance of sulfate-reducing bacteria from the family Desulfovibrionaceae, known for inducing inflammatory responses and promoting atherosclerotic plaque formation, were negatively correlated with CBF in memory and learning areas.
We conducted a study on the gut microbiome of 30 older adults who reside in the community. Figure 6 presents a summary of our findings, which indicate a strong association between microbiome composition in this group and several factors including APOE genotype, BMI, obesity, and diabetes, as well as brain imaging markers obtained from brain volume, CBF, metabolites, and WMI. Our analysis reveals that APOE2 and APOE4 carriers had a very distinctive microbiota composition are consistent with a number of studies examining gut microbiome in murine models (Weng et al., 2019; Parikh et al., 2020; Zajac et al., 2022). Specifically, the APOE4 genotype had relatively lower abundance of Roseburia, but higher in Eubacterium eligens and Faecalibacterium prausnitzii. On the other hand, the APOE2 genotype had higher levels of Lachnoclostridium species and lower levels of Eubacterium eligens, Oscillibacter, and Faecalibacterium prausnitzii (Chung et al., 2017). Roseburia, a well-known butyrate producer that is associated with a decreased risk of cognitive decline, was found to be decreased in the APOE4 genotype (Jin et al., 2021). This is an interesting finding as APOE2 is protective against Alzheimer’s disease (AD) while APOE4 increase the risk (Yamazaki et al., 2019).
Figure 6. The relationship of gut microorganisms to various disease variables and brain function: Microbiome in aging is associated with (Left) APOE genotype, calcium intake, vegetable intake, diabetes, and obesity, as well as with (Right) brain imaging makers, including brain volume, brain metabolites, cerebral blood flow, and white matter integrity.
While the functions of some species identified between APOE2 and APOE4 carriers in this study still need to be explored, our results align with existing literature, suggesting that APOE variants may shape the structure of the gut microbiome (Weng et al., 2019; Parikh et al., 2020; Zajac et al., 2022). In earlier work, we demonstrated that asymptomatic APOE4 carriers experience more pronounced gut dysbiosis than non-carriers, including those with APOE e3 alleles (APOE3), and prebiotic diet may mitigate the risk for AD by reducing the gut dysbiosis in the APOE4 mice (Hoffman et al., 2019; Yanckello et al., 2021). Other research has shown associations between APOE genotype differences and significant variations in the relative abundance of families like Prevotellaceae and Ruminococcaceae, as well as several butyrate-producing genera (Tran et al., 2019). A recent investigation even revealed that disruptions in gut microbiome composition can modify immune pathways, leading to the tauopathy underlying Alzheimer’s disease in an APOE genotype-dependent manner (Seo et al., 2023). These insights suggest that microbiome composition and diversity may play a substantial role in AD development, influenced by variations in APOE genetics. Moving forward, future studies should delve deeper into the differential effects of APOE-genotype dependent variations in the microbiome.
We found that obesity was inversely associated with Akkermansia muciniphila, which has previously been found to be decreased in obesity and is believed to be protective against metabolic disorders through its excretion of endocannabinoids that control inflammation, gut barrier function, and gut peptide secretion (Everard et al., 2013). Further, proinflammatory state was associated with higher BMI and diabetes. Diabetes was associated with increased levels of E. coli and Parabacteroides goldsteinii. While E. coli is a normal commensal gut bacteria, it has many pathologic variants that can cause disease (Leimbach et al., 2013), and increases in infectious E. coli have previously been reported in diabetes (Suri et al., 2009) and diabetes-related neurological disorders, such as stroke (Hammond et al., 2022a,b).
Our findings suggest that a healthy diet is strongly associated with a microbiota that produces SCFAs, leading to an anti-inflammatory state. We used the Dietary Screener Questionnaire from the National Health and Nutrition Examination Survey to measure effects of the diet on the microbiome. Interestingly, we observed an inverse relationship between calcium and Clostridium asparagiforme, a species that has been found to be reduced in smokers with hypertension (Wang et al., 2021). Conversely, we found that calcium was positively associated with Acidaminococcaceae, Phascolarctobacterium and Eubacterium eligens. The former two taxa are known to produce SCFAs (Wu et al., 2017), while Eubacterium eligens has anti-inflammatory effects on the host (Chung et al., 2017). Although the precise mechanisms underlying the effects of calcium on these microbial taxa remain unknown, previous research has shown that a high calcium diet increases Firmicutes species and levels of lactic, acetic, and butyric acid (Fuhren et al., 2021). We also observed a positive relationship between vegetable intake and Agathobaculum butyriciproducens, a butyrate producer, which has been shown to improve performance in behavioral tests, decrease Aβ plaque deposition and microglial activation APP/PS1 transgenic mice (Go et al., 2021). A negative effect with Eggerthella lenta, an infectious species, was found in our data (Gardiner et al., 2015; Go et al., 2021). It is interesting that fiber, whole grains, added sugars, dairy, and fruit intake did not have any significant associations with the microbial taxa in our data. Many studies have highlighted the beneficial effects of a vegetarian diet on the microbiome by limiting the abundance of inflammatory species and promoting SCFA-producing species (Tang et al., 2019; Sakkas et al., 2020). Other studies have investigated the effects of Western, Mediterranean, vegetarian, high carbohydrate, high fat, whole food, ultra-processed, and low-calorie sweetener diets on the microbiome (Dahl et al., 2020). Specific Dietary effects on the microbiome should be explored in future studies.
The results also imply that strengthening the immune barriers of the gut were associated with the volume of brain structures related to processing high levels of information. Bacteroides ovatus is related to the thalamus volume. The thalamus is an important relay station in the brain that communicates information from the spinal cord and cerebellum to the cerebral cortex (Sherman, 2007). Bacteroides ovatus is a commensal microbe that uses various carbohydrates and proteins as its fuel source (Fultz et al., 2021), is a prominent inducer of IgA (Yang et al., 2020), and promotes the reparative cytokine IL-22 (Ihekweazu et al., 2021), thereby stimulating epithelial recovery (Ihekweazu et al., 2019). The family Acidaminococcaceae is related to the volume of the mid posterior portion of the corpus callosum. The region is responsible for connecting portions of the parietal and temporal cortices on each side of the brain. This is consistent with a previous finding that Acidaminococcaceae is associated with memory performance (Khine et al., 2020).
Our results also indicated that microbiota associated with SCFAs production are highly associated with less vascular disease in the brain. Bacteroides uniformis correlates inversely with white matter hypointensities (WMH), which are a marker of vascular disease in the brain (Graff-Radford et al., 2019) and are often associated with cognitive impairment (Hu et al., 2021). Bacteroides uniformis prefers wheat bran extract as a fuel source and is a butyrate producer; it strengthens the first line of immune defense against unhealthy diets (Lopez-Almela et al., 2021) and improves glucose tolerance (Fabersani et al., 2021). This microbe has also been associated with increased dopamine transporters (Hartstra et al., 2020) and normalizing the brain reward response to reduce anxiety in rats (Agusti et al., 2021). Interestingly, the microbiota-derived phenylacetylglutamine has previously been associated with the amount of WMH in the brain (Yu et al., 2021).
Our data also suggests that gut microbiota that produce GABA, the main inhibitory neurotransmitter in the brain (Petroff, 2002), are highly associated with the amount of GABA present in the corpus callosum. Collinsella aerofaciens is negatively associated with GABA production, and Parasutterella excrementihominis is positively associated with GABA production. Collinsella aerofaciens is an obligate anaerobe (Bag et al., 2017; Qin et al., 2019) and a proinflammatory species associated with Crohn’s disease (Joossens et al., 2011; Kalinkovich and Livshits, 2019). Parasutterella excrementihominis is strictly anaerobic (Nagai et al., 2009) and has been associated with impaired GI health (Fart et al., 2020). While Collinsella aerofaciens and Parasutterella excrementihominis have not previously been identified as GABA producers or consumers, Bacteroides has been identified as a dominant GABA producer and Pseudomonas as a prominent GABA consumer (Strandwitz et al., 2019).
It was observed that microbial species Ruminococcus lactaris, a producer of SCFAs, is associated with the brain metabolites related to mitochondrial function and cognition. NAA correlates with neuronal mitochondrial function and survival (Paslakis et al., 2014), and NAAG has procognitive properties (Neale and Olszewski, 2019). Ruminococcus lactaris is a butyrate producer (Sato et al., 2021) and is negatively correlated with the inflammatory cytokine IL-8 (Shintouo et al., 2020). A previous group found a link between Ruminococcus and NAA thought to be mediated by Ruminococcus decreasing cortisol, which impacts brain NAA (Mudd et al., 2017).
Our analysis indicates a positive correlation between Collinsella stercoris, a producer for conjugated bile acid, with CBF. Previous research has shown that the conjugated bile acid Tauroursodeoxycholic acid (TUDCA) can alleviate early brain injury by reversing cerebrovascular dysfunction and ER-stress-mediated apoptosis (Chen et al., 2020). TUDCA was found to enhance neurological function and treated SAH-related cerebrovascular dysfunction by increasing cerebral cortical perfusion and decreasing blood–brain barrier permeability. These reports are in line with our findings that presence of Collinsella stercoris may be beneficial for maintaining CBF. We further showed that microbiota related to equol producers are highly associated with WMI that connecting brain regions associated with language and memory. The superior temporal sulcus is commonly referred to as Wernicke’s area and is a central area for speech recognition and processing (Nourski et al., 2021). The left pars triangularis is commonly referred to as Broca’s area and is a central area for speech production (Foundas et al., 1996) Members of the family Eggerthellaceae are able to convert the isoflavone daidzein (a soy product) into equol, an estrogen (Soukup et al., 2021). Specific members of this family, including Adlercreutzia equolifaciens, Asaccharobacter celatus, and Gordonibacter pamelaeae all have negative associations with these same areas responsible for language circuits, in addition to areas of the brain responsible for memory, learning, and reward processing. Equol supplementation has been shown to improve long and short-term memory in rats by increasing brain antioxidant activity and improving blood pressure (Liu and Tsai, 2016; Caliskan et al., 2021).
Collectively, the findings in the present study indicated that microbiome composition in aging may play an important role in determining brain metabolism and integrity, and APOE status could also be a key player. This is in line with studies showing that metabolism may play a more critical role in driving neurodegeneration than amyloid beta plaques and tau tangles in the progression of Alzheimer’s disease (AD) (Hammond et al., 2020; Hammond and Lin, 2022), and that APOE4 carriers and non-carriers develop AD through different metabolic pathways (Hammond et al., 2021). Therefore, it will be critical for to identify interventions that can promote healthy microbiome in aging, taking into consideration of APOE genotype, to protect brain function in aging and potentially mitigate AD risk for APOE4 carriers. This is in good agreement with our recent studies showing that supplementing a diet with the prebiotic inulin, a fermentable prebiotic fiber, can positively impact gut microbiome composition, boost the production of SCFAs, enhance mitochondrial function, and decrease neuroinflammation in young, asymptomatic APOE4 mice (Hoffman et al., 2019). Our results also demonstrate that these effects of dietary inulin supplementation revealed an APOE genotype-dependent response (Yanckello et al., 2021). These findings are also consistent with what we observed in the current study that higher fiber (vegetable) intake can improve microbiome in aging. Our study further provides other potential interventions in the future, such as equol or butyrate supplementation, as well as probiotic interventions with butyrate and equol producers like Roseburia, which have shown promise as easily implemented, inexpensive tools to optimize healthy brain aging.
While this study provides further understanding between gut microbiome and brain health in the aging population, it has many limitations. The observational nature of this study helps us to make many associations between gut bacteria and markers of brain health on imaging, but we are unable to determine causation. Additionally, due to the pandemic, we were only able to recruit 30 participants for the study. The relatively small sample size for a human study with a heterogeneous population potentially leaves the study underpowered to detect smaller associations and changes in the microbiome. Future work should utilize a larger sample size to allow adjusting for potential confounders and to identify which demographic factors (e.g., BMI, gender, age, APOE genotype) most strongly associate with the composition of the microbiome. Future work should investigate the long-term nature of the gut microbiome in this population. Future studies can also further include other more advanced MRI methods to measure brain oxygen metabolism or perivascular space, which will provide further insight for brain metabolism and inflammation, respectively (Lin et al., 2008, 2010; Feldman et al., 2018; Langan et al., 2022).
In summary, we demonstrated the significant correlations of microbiome in older adults with their brain imaging markers, APOE genotypes, calcium intake, vegetable intakes, diabetes, and obesity. The findings could have important implications in the future for promoting brain health through microbiome modulation and developing precision nutrition interventions to optimize healthy brain aging older adults.
The datasets presented in this article are not publicly available due to national legislation that restricts the deposition of human data. Requests to access the datasets should be directed to A-LL YWktbGluZy5saW5AaGVhbHRoLm1pc3NvdXJpLmVkdQ==.
The studies involving humans were approved by Institutional Review Board at the University of Kentucky. The studies were conducted in accordance with the local legislation and institutional requirements. The participants provided their written informed consent to participate in this study.
TH, AS, and A-LL designed the research. TH, SG, GC, AC, and A-LL conducted the research. TH, AC, YJ, XX, and AS performed the statistical analysis. TH, SG, GC, YJ, SH, AC, CA, XX, AF, AS, PB, and A-LL wrote the manuscript. TH, SG, AS, and A-LL had primary responsibility for final content. All authors contributed to the article and approved the submitted version.
This research was supported by grants from NIH/NIA, NIH/ODS, and American Federation for Aging Research to A-LL (R01AG054459 and RF1AG062480) and TH (T32AG057461).
We would like to thank the participants in the study for donating their time and effort to this research. We would also like to thank David Powell at the UK MRISC for assisting MRI/MRS data acquisition, and Shawn Caudill of UK Internal Medicine assisting participant recruitment. Bioinformatics analysis in the project described was performed by the UIC Research Informatics Core, supported in part by NCATS through Grant UL1TR002003.
The authors declare that the research was conducted in the absence of any commercial or financial relationships that could be construed as a potential conflict of interest.
All claims expressed in this article are solely those of the authors and do not necessarily represent those of their affiliated organizations, or those of the publisher, the editors and the reviewers. Any product that may be evaluated in this article, or claim that may be made by its manufacturer, is not guaranteed or endorsed by the publisher.
Agusti, A., Campillo, I., Balzano, T., Benitez-Paez, A., Lopez-Almela, I., Romani-Perez, M., et al. (2021). Bacteroides uniformis CECT 7771 modulates the brain reward response to reduce binge eating and anxiety-like behavior in rat. Mol. Neurobiol. 58, 4959–4979. doi: 10.1007/s12035-021-02462-2
Arnoldussen, I. A., Kiliaan, A. J., and Gustafson, D. R. (2014). Obesity and dementia: adipokines interact with the brain. Eur. Neuropsychopharmacol. 24, 1982–1999. doi: 10.1016/j.euroneuro.2014.03.002
Asadi, A., Shadab Mehr, N., Mohamadi, M. H., Shokri, F., Heidary, M., Sadeghifard, N., et al. (2022). Obesity and gut-microbiota-brain axis: a narrative review. J. Clin. Lab. Anal. 36:e24420. doi: 10.1002/jcla.24420
Badal, V. D., Vaccariello, E. D., Murray, E. R., Yu, K. E., Knight, R., Jeste, D. V., et al. (2020). The gut microbiome, aging, and longevity: a systematic review. Nutrients 12:3759. doi: 10.3390/nu12123759
Bag, S., Ghosh, T. S., and Das, B. (2017). Complete genome sequence of Collinsella aerofaciens isolated from the gut of a healthy Indian subject. Genome Announc. 5:e01361-17. doi: 10.1128/genomeA.01361-17
Beghini, F., McIver, L. J., Blanco-Miguez, A., Dubois, L., Asnicar, F., Maharjan, S., et al. (2021). Integrating taxonomic, functional, and strain-level profiling of diverse microbial communities with bioBakery 3. eLife 10:e65088. doi: 10.7554/eLife.65088
Biagi, E., Nylund, L., Candela, M., Ostan, R., Bucci, L., Pini, E., et al. (2010). Through ageing, and beyond: gut microbiota and inflammatory status in seniors and centenarians. PLoS One 5:e10667. doi: 10.1371/journal.pone.0010667
Borsom, E. M., Lee, K., and Cope, E. K. (2020). Do the bugs in your gut eat your memories? Relationship between gut microbiota and Alzheimer's Disease. Brain Sci. 10:814. doi: 10.3390/brainsci10110814
Brinton, R. D., Yao, J., Yin, F., Mack, W. J., and Cadenas, E. (2015). Perimenopause as a neurological transition state. Nat. Rev. Endocrinol. 11, 393–405. doi: 10.1038/nrendo.2015.82
Buford, T. W. (2017). (Dis)trust your gut: the gut microbiome in age-related inflammation, health, and disease. Microbiome 5:80. doi: 10.1186/s40168-017-0296-0
Caliskan, G., Raza, S. A., Demiray, Y. E., Kul, E., Sandhu, K. V., and Stork, O. (2021). Depletion of dietary phytoestrogens reduces hippocampal plasticity and contextual fear memory stability in adult male mouse. Nutr. Neurosci. 24, 951–962. doi: 10.1080/1028415X.2019.1698826
Castellano, J. M., Kim, J., Stewart, F. R., Jiang, H., DeMattos, R. B., Patterson, B. W., et al. (2011). Human apoE isoforms differentially regulate brain amyloid-b peptide clearance. Sci. Transl. Med. 3:89ra57. doi: 10.1126/scitranslmed.3002156
Chen, X., Wang, J., Gao, X., Wu, Y., Gu, G., Shi, M., et al. (2020). Tauroursodeoxycholic acid prevents ER stress-induced apoptosis and improves cerebral and vascular function in mice subjected to subarachnoid hemorrhage. Brain Res. 1727:146566. doi: 10.1016/j.brainres.2019.146566
Chung, W. S. F., Meijerink, M., Zeuner, B., Holck, J., Louis, P., Meyer, A. S., et al. (2017). Prebiotic potential of pectin and pectic oligosaccharides to promote anti-inflammatory commensal bacteria in the human colon. FEMS Microbiol. Ecol. 93:fix127. doi: 10.1093/femsec/fix127
Cox, L. M., and Weiner, H. L. (2018). Microbiota signaling pathways that influence neurologic Disease. Neurotherapeutics 15, 135–145. doi: 10.1007/s13311-017-0598-8
Dahl, W. J., Rivero Mendoza, D., and Lambert, J. M. (2020). Diet, nutrients and the microbiome. Prog. Mol. Biol. Transl. Sci. 171, 237–263. doi: 10.1016/bs.pmbts.2020.04.006
Doden, H. L., Wolf, P. G., Gaskins, H. R., Anantharaman, K., Alves, J. M. P., and Ridlon, J. M. (2021). Completion of the gut microbial epi-bile acid pathway. Gut Microbes 13, 1–20. doi: 10.1080/19490976.2021.1907271
Everard, A., Belzer, C., Geurts, L., Ouwerkerk, J. P., Druart, C., Bindels, L. B., et al. (2013). Cross-talk between Akkermansia muciniphila and intestinal epithelium controls diet-induced obesity. Proc. Natl. Acad. Sci. U. S. A. 110, 9066–9071. doi: 10.1073/pnas.1219451110
Fabersani, E., Portune, K., Campillo, I., Lopez-Almela, I., la Paz, S. M., Romani-Perez, M., et al. (2021). Bacteroides uniformis CECT 7771 alleviates inflammation within the gut-adipose tissue axis involving TLR5 signaling in obese mice. Sci. Rep. 11:11788. doi: 10.1038/s41598-021-90888-y
Fart, F., Rajan, S. K., Wall, R., Rangel, I., Ganda-Mall, J. P., Tingo, L., et al. (2020). Differences in gut microbiome composition between senior orienteering athletes and community-dwelling older adults. Nutrients 12:2610. doi: 10.3390/nu12092610
Feldman, R. E., Rutland, J. W., Fields, M. C., Marcuse, L. V., Pawha, P. S., Delman, B. N., et al. (2018). Quantification of perivascular spaces at 7T: a potential MRI biomarker for epilepsy. Seizure 54, 11–18. doi: 10.1016/j.seizure.2017.11.004
Fjell, A., and Walhovd, K. B. (2010). Structural brain changes in aging: courses, causes and cognitive consequences. Rev. Neurosci. 21, 187–221. doi: 10.1515/REVNEURO.2010.21.3.187
Foundas, A. L., Leonard, C. M., Gilmore, R. L., Fennell, E. B., and Heilman, K. M. (1996). Pars triangularis asymmetry and language dominance. Proc. Natl. Acad. Sci. U. S. A. 93, 719–722. doi: 10.1073/pnas.93.2.719
Franceschi, C., and Campisi, J. (2014). Chronic inflammation (inflammaging) and its potential contribution to age-associated diseases. J. Gerontol. A Biol. Sci. Med. Sci. 69, S4–S9. doi: 10.1093/gerona/glu057
Fuhren, J., Schwalbe, M., Boekhorst, J., Rosch, C., Schols, H. A., and Kleerebezem, M. (2021). Dietary calcium phosphate strongly impacts gut microbiome changes elicited by inulin and galacto-oligosaccharides consumption. Microbiome 9:218. doi: 10.1186/s40168-021-01148-0
Fultz, R., Ticer, T., Ihekweazu, F. D., Horvath, T. D., Haidacher, S. J., Hoch, K. M., et al. (2021). Unraveling the metabolic requirements of the gut commensal Bacteroides ovatus. Front. Microbiol. 12:745469. doi: 10.3389/fmicb.2021.745469
Gardiner, B. J., Tai, A. Y., Kotsanas, D., Francis, M. J., Roberts, S. A., Ballard, S. A., et al. (2015). Clinical and microbiological characteristics of Eggerthella lenta bacteremia. J. Clin. Microbiol. 53, 626–635. doi: 10.1128/JCM.02926-14
Go, J., Chang, D. H., Ryu, Y. K., Park, H. Y., Lee, I. B., Noh, J. R., et al. (2021). Human gut microbiota Agathobaculum butyriciproducens improves cognitive impairment in LPS-induced and APP/PS1 mouse models of Alzheimer's disease. Nutr. Res. 86, 96–108. doi: 10.1016/j.nutres.2020.12.010
Gomes, A. C., Bueno, A. A., de Souza, R. G., and Mota, J. F. (2014). Gut microbiota, probiotics and diabetes. Nutr. J. 13:60. doi: 10.1186/1475-2891-13-60
Gómez-Pinilla, F. (2008). Brain foods: the effects of nutrients, on brain function. Nat. Rev. Neurosci. 9, 568–578. doi: 10.1038/nrn2421
Graff-Radford, J., Arenaza-Urquijo, E. M., Knopman, D. S., Schwarz, C. G., Brown, R. D., Rabinstein, A. A., et al. (2019). White matter hyperintensities: relationship to amyloid and tau burden. Brain 142, 2483–2491. doi: 10.1093/brain/awz162
Hammond, T. C., and Lin, A. L. (2022). Glucose metabolism is a better marker for predicting clinical Alzheimer's Disease than amyloid or tau. J. Cell Immunol. 4, 15–18. doi: 10.33696/immunology.4.128
Hammond, T. C., Messmer, S., Frank, J. A., Lukins, D., Colwell, R., Lin, A. L., et al. (2022a). Gut microbial dysbiosis correlates with stroke severity markers in aged rats. Front. Stroke 1:1026066. doi: 10.3389/fstro.2022.1026066
Hammond, T. C., Powell, E., Green, S. J., Chlipala, G., Frank, J., Yackzan, A. T., et al. (2022b). Functional recovery outcomes following acute stroke is associated with abundance of gut microbiota related to inflammation, butyrate and secondary bile acid. Front. Rehabil. Sci. 3:1017180. doi: 10.3389/fresc.2022.1017180
Hammond, T. C., Xing, X., Wang, C., Ma, D., Nho, K., Crane, P. K., et al. (2020). beta-amyloid and tau drive early Alzheimer's disease decline while glucose hypometabolism drives late decline. Commun. Biol. 3:352. doi: 10.1038/s42003-020-1079-x
Hammond, T. C., Xing, X., Yanckello, L. M., Stromberg, A., Chang, Y. H., Nelson, P. T., et al. (2021). Human gray and white matter metabolomics to differentiate APOE and stage dependent changes in Alzheimer's Disease. J. Cell Immunol. 3, 397–412. doi: 10.33696/immunology.3.123
Hartstra, A. V., Schuppel, V., Imangaliyev, S., Schrantee, A., Prodan, A., Collard, D., et al. (2020). Infusion of donor feces affects the gut-brain axis in humans with metabolic syndrome. Mol. Metab. 42:101076. doi: 10.1016/j.molmet.2020.101076
Hoffman, J. D., Parikh, I., Green, S. J., Chlipala, G., Mohney, R. P., Keaton, M., et al. (2017). Age drives distortion of brain metabolic, vascular and cognitive functions, and the gut microbiome. Front. Aging Neurosci. 9:298. doi: 10.3389/fnagi.2017.00298
Hoffman, J. D., Yanckello, L. M., Chlipala, G., Hammond, T. C., McCulloch, S. D., Parikh, I., et al. (2019). Dietary inulin alters the gut microbiome, enhances systemic metabolism and reduces neuroinflammation in an APOE4 mouse model. PLoS One 14:e0221828. doi: 10.1371/journal.pone.0221828
Hu, H. Y., Ou, Y. N., Shen, X. N., Qu, Y., Ma, Y. H., Wang, Z. T., et al. (2021). White matter hyperintensities and risks of cognitive impairment and dementia: a systematic review and meta-analysis of 36 prospective studies. Neurosci. Biobehav. Rev. 120, 16–27. doi: 10.1016/j.neubiorev.2020.11.007
Ihekweazu, F. D., Engevik, M. A., Ruan, W., Shi, Z., Fultz, R., Engevik, K. A., et al. (2021). Bacteroides ovatus promotes IL-22 production and reduces Trinitrobenzene sulfonic acid-driven colonic inflammation. Am. J. Pathol. 191, 704–719. doi: 10.1016/j.ajpath.2021.01.009
Ihekweazu, F. D., Fofanova, T. Y., Queliza, K., Nagy-Szakal, D., Stewart, C. J., Engevik, M. A., et al. (2019). Bacteroides ovatus ATCC 8483 monotherapy is superior to traditional fecal transplant and multi-strain bacteriotherapy in a murine colitis model. Gut Microbes 10, 504–520. doi: 10.1080/19490976.2018.1560753
Jin, X., He, W., Zhang, Y., Gong, E., Niu, Z., Ji, J., et al. (2021). Association of APOE epsilon4 genotype and lifestyle with cognitive function among Chinese adults aged 80 years and older: a cross-sectional study. PLoS Med. 18:e1003597. doi: 10.1371/journal.pmed.1003597
Joossens, M., Huys, G., Cnockaert, M., De Preter, V., Verbeke, K., Rutgeerts, P., et al. (2011). Dysbiosis of the faecal microbiota in patients with Crohn's disease and their unaffected relatives. Gut 60, 631–637. doi: 10.1136/gut.2010.223263
Kalinkovich, A., and Livshits, G. (2019). A cross talk between dysbiosis and gut-associated immune system governs the development of inflammatory arthropathies. Semin. Arthritis Rheum. 49, 474–484. doi: 10.1016/j.semarthrit.2019.05.007
Khine, W. W. T., Voong, M. L., Ng, T. K. S., Feng, L., Rane, G. A., Kumar, A. P., et al. (2020). Mental awareness improved mild cognitive impairment and modulated gut microbiome. Aging 12, 24371–24393. doi: 10.18632/aging.202277
Kramer, P. F., Twedell, E. L., Shin, J. H., Zhang, R., and Khaliq, Z. M. (2020). Axonal mechanisms mediating gamma-aminobutyric acid receptor type a (GABA-A) inhibition of striatal dopamine release. eLife 9:e55729. doi: 10.7554/eLife.55729
Langan, M. T., Smith, D. A., Verma, G., Khegai, O., Saju, S., Rashid, S., et al. (2022). Semi-automated segmentation and quantification of perivascular spaces at 7 tesla in COVID-19. Front. Neurol. 13:846957. doi: 10.3389/fneur.2022.846957
Leimbach, A., Hacker, J., and Dobrindt, U. (2013). E. coli as an all-rounder: the thin line between commensalism and pathogenicity. Curr. Top. Microbiol. Immunol. 358, 3–32. doi: 10.1007/82_2012_303
Lin, A. L., Coman, D., Jiang, L., Rothman, D. L., and Hyder, F. (2014). Caloric restriction impedes age-related decline of mitochondrial function and neuronal activity. J. Cereb. Blood Flow Metab. 34, 1440–1443. doi: 10.1038/jcbfm.2014.114
Lin, A. L., Fox, P. T., Hardies, J., Duong, T. Q., and Gao, J. H. (2010). Nonlinear coupling between cerebral blood flow, oxygen consumption, and ATP production in human visual cortex. Proc. Natl. Acad. Sci. U. S. A. 107, 8446–8451. doi: 10.1073/pnas.0909711107
Lin, A. L., Fox, P. T., Yang, Y., Lu, H., Tan, L. H., and Gao, J. H. (2008). Evaluation of MRI models in the measurement of CMRO2 and its relationship with CBF. Magn. Reson. Med. 60, 380–389. doi: 10.1002/mrm.21655
Liu, T. H., and Tsai, T. Y. (2016). Effects of equol on deoxycorticosterone acetate salt-induced hypertension and associated vascular dementia in rats. Food Funct. 7, 3444–3457. doi: 10.1039/C6FO00223D
Lopez-Almela, I., Romani-Perez, M., Bullich-Vilarrubias, C., Benitez-Paez, A., Gomez Del Pulgar, E. M., Frances, R., et al. (2021). Bacteroides uniformis combined with fiber amplifies metabolic and immune benefits in obese mice. Gut Microbes 13, 1–20. doi: 10.1080/19490976.2020.1865706
Mallick, H., Rahnavard, A., McIver, L. J., Ma, S., Zhang, Y., Nguyen, L. H., et al. (2021). Multivariable association discovery in population-scale meta-omics studies. PLoS Comput. Biol. 17:e1009442. doi: 10.1371/journal.pcbi.1009442
Mattson, M. P., and Arumugam, T. V. (2018). Hallmarks of brain aging: adaptive and pathological modification by metabolic states. Cell Metab. 27, 1176–1199. doi: 10.1016/j.cmet.2018.05.011
McNab, J. A., and Bartha, R. (2006). Quantitative short echo-time 1H LASER-CSI in human brain at 4 T. NMR Biomed. 19, 999–1009. doi: 10.1002/nbm.1053
Molloy, C. J., Nugent, S., and Bokde, A. L. W. (2021). Alterations in diffusion measures of white matter integrity associated with healthy aging. J. Gerontol. A Biol. Sci. Med. Sci. 76, 945–954. doi: 10.1093/gerona/glz289
Mudd, A. T., Berding, K., Wang, M., Donovan, S. M., and Dilger, R. N. (2017). Serum cortisol mediates the relationship between fecal Ruminococcus and brain N-acetylaspartate in the young pig. Gut Microbes 8, 589–600. doi: 10.1080/19490976.2017.1353849
Nagai, F., Morotomi, M., Sakon, H., and Tanaka, R. (2009). Parasutterella excrementihominis gen. Nov., sp. nov., a member of the family Alcaligenaceae isolated from human faeces. Int. J. Syst. Evol. Microbiol. 59, 1793–1797. doi: 10.1099/ijs.0.002519-0
Neale, J. H., and Olszewski, R. (2019). A role for N-acetylaspartylglutamate (NAAG) and mGluR3 in cognition. Neurobiol. Learn. Mem. 158, 9–13. doi: 10.1016/j.nlm.2019.01.006
Nourski, K. V., Steinschneider, M., Rhone, A. E., Kovach, C. K., Banks, M. I., Krause, B. M., et al. (2021). Electrophysiology of the human superior temporal sulcus during speech processing. Cereb. Cortex 31, 1131–1148. doi: 10.1093/cercor/bhaa281
Parikh, I. J., Estus, J. L., Zajac, D. J., Malik, M., Maldonado Weng, J., Tai, L. M., et al. (2020). Murine gut microbiome association with APOE alleles. Front. Immunol. 11:200. doi: 10.3389/fimmu.2020.00200
Paslakis, G., Traber, F., Roberz, J., Block, W., and Jessen, F. (2014). N-acetyl-aspartate (NAA) as a correlate of pharmacological treatment in psychiatric disorders: a systematic review. Eur. Neuropsychopharmacol. 24, 1659–1675. doi: 10.1016/j.euroneuro.2014.06.004
Petroff, O. A. (2002). GABA and glutamate in the human brain. Neuroscientist 8, 562–573. doi: 10.1177/1073858402238515
Provencher, S. W. (1993). Estimation of metabolite concentrations from localized in vivo proton NMR spectra. Magn. Reson. Med. 30, 672–679. doi: 10.1002/mrm.1910300604
Qin, P., Zou, Y., Dai, Y., Luo, G., Zhang, X., and Xiao, L. (2019). Characterization a novel butyric acid-producing bacterium Collinsella aerofaciens Subsp. Shenzhenensis Subsp. Nov. Microorganisms 7:78. doi: 10.3390/microorganisms7030078
R Core Team (2020). "R: A language and environment for statistical computing." R Foundation for Statistical Computing, Vienna, Austria.
Raz, N., and Rodrigue, K. M. (2006). Differential aging of the brain: patterns, cognitive correlates and modifiers. Neurosci. Biobehav. Rev. 30, 730–748. doi: 10.1016/j.neubiorev.2006.07.001
Sakkas, H., Bozidis, P., Touzios, C., Kolios, D., Athanasiou, G., Athanasopoulou, E., et al. (2020). Nutritional status and the influence of the vegan diet on the gut microbiota and human health. Medicina (Kaunas) 56:88. doi: 10.3390/medicina56020088
Sato, N., Kakuta, M., Hasegawa, T., Yamaguchi, R., Uchino, E., Murashita, K., et al. (2021). Metagenomic profiling of gut microbiome in early chronic kidney disease. Nephrol. Dial. Transplant. 36, 1675–1684. doi: 10.1093/ndt/gfaa122
Seo, D. O., O'Donnell, D., Jain, N., Ulrich, J. D., Herz, J., Li, Y., et al. (2023). ApoE isoform- and microbiota-dependent progression of neurodegeneration in a mouse model of tauopathy. Science 379:eadd1236. doi: 10.1126/science.add1236
Sherman, S. M. (2007). The thalamus is more than just a relay. Curr. Opin. Neurobiol. 17, 417–422. doi: 10.1016/j.conb.2007.07.003
Shi, Y., Yamada, K., Liddelow, S. A., Smith, S. T., Zhao, L., Luo, W., et al. (2017). ApoE4 markedly exacerbates tau-mediated neurodegeneration in a mouse model of tauopathy. Nature 549, 523–527. doi: 10.1038/nature24016
Shintouo, C. M., Mets, T., Beckwee, D., Bautmans, I., Ghogomu, S. M., Souopgui, J., et al. (2020). Is inflammageing influenced by the microbiota in the aged gut? A systematic review. Exp. Gerontol. 141:111079. doi: 10.1016/j.exger.2020.111079
Soukup, S. T., Stoll, D. A., Danylec, N., Schoepf, A., Kulling, S. E., and Huch, M. (2021). Metabolism of Daidzein and Genistein by gut Bacteria of the class Coriobacteriia. Foods 10:2741. doi: 10.3390/foods10112741
Strandwitz, P., Kim, K. H., Terekhova, D., Liu, J. K., Sharma, A., Levering, J., et al. (2019). GABA-modulating bacteria of the human gut microbiota. Nat. Microbiol. 4, 396–403. doi: 10.1038/s41564-018-0307-3
Suri, R. S., Mahon, J. L., Clark, W. F., Moist, L. M., Salvadori, M., and Garg, A. X. (2009). Relationship between Escherichia coli O157:H7 and diabetes mellitus. Kidney Int. 75, S44–S46. doi: 10.1038/ki.2008.619
Tang, Z. Z., Chen, G., Hong, Q., Huang, S., Smith, H. M., Shah, R. D., et al. (2019). Multi-Omic analysis of the microbiome and metabolome in healthy subjects reveals microbiome-dependent relationships between diet and metabolites. Front. Genet. 10:454. doi: 10.3389/fgene.2019.00454
Tarumi, T., and Zhang, R. (2018). Cerebral blood flow in normal aging adults: cardiovascular determinants, clinical implications, and aerobic fitness. J. Neurochem. 144, 595–608. doi: 10.1111/jnc.14234
Tran, T. T. T., Corsini, S., Kellingray, L., Hegarty, C., Le Gall, G., Narbad, A., et al. (2019). APOE genotype influences the gut microbiome structure and function in humans and mice: relevance for Alzheimer's disease pathophysiology. FASEB J. 33, 8221–8231. doi: 10.1096/fj.201900071R
Turner, M. P., Zhao, Y., Abdelkarim, D., Liu, P., Spence, J. S., Hutchison, J. L., et al. (2022). Altered linear coupling between stimulus-evoked blood flow and oxygen metabolism in the aging human brain. Cereb. Cortex 33, 135–151. doi: 10.1093/cercor/bhac057
Vogt, N. M., Kerby, R. L., Dill-McFarland, K. A., Harding, S. J., Merluzzi, A. P., Johnson, S. C., et al. (2017). Gut microbiome alterations in Alzheimer's disease. Sci. Rep. 7:13537. doi: 10.1038/s41598-017-13601-y
Wachsmuth, H. R., Weninger, S. N., and Duca, F. A. (2022). Role of the gut-brain axis in energy and glucose metabolism. Exp. Mol. Med. 54, 377–392. doi: 10.1038/s12276-021-00677-w
Wang, P., Dong, Y., Jiao, J., Zuo, K., Han, C., Zhao, L., et al. (2021). Cigarette smoking status alters dysbiotic gut microbes in hypertensive patients. J. Clin. Hypertens. (Greenwich) 23, 1431–1446. doi: 10.1111/jch.14298
Weng, M. J., Parikh, I., Naqib, A., York, J., Green, S. J., Estus, S., et al. (2019). Synergistic effects of APOE and sex on the gut microbiome of young EFAD transgenic mice. Mol. Neurodegener. 14:47. doi: 10.1186/s13024-019-0352-2
Wu, F., Guo, X., Zhang, J., Zhang, M., Ou, Z., and Peng, Y. (2017). Phascolarctobacterium faecium abundant colonization in human gastrointestinal tract. Exp. Ther. Med. 14, 3122–3126. doi: 10.3892/etm.2017.4878
Yamazaki, Y., Zhao, N., Caulfield, T. R., Liu, C. C., and Bu, G. (2019). Apolipoprotein E and Alzheimer disease: pathobiology and targeting strategies. Nat. Rev. Neurol. 15, 501–518. doi: 10.1038/s41582-019-0228-7
Yanckello, L. M., Chang, Y.-H., Sun, M., Chlipala, G., Green, S. J., Lei, Z., et al. (2022a). Inulin supplementation prior to mild traumatic brain injury mitigates gut dysbiosis, and brain vascular and white matter deficits in mice. Front. Microbiomes 1:986951. doi: 10.3389/frmbi.2022.986951
Yanckello, L. M., Hoffman, J. D., Chang, Y.-H., Lin, P., Nehra, G., Chlipala, G., et al. (2021). Apolipoprotein E genotype-dependent nutrigenetic effects to prebiotic inulin for modulating systemic metabolism and neuroprotection in mice via gut-brain axis. Nutr. Neurosci. 25, 1669–1679. doi: 10.1080/1028415X.2021.1889452
Yanckello, L. M., Hoffman, J. D., Chang, Y. H., Lin, P., Nehra, G., Chlipala, G., et al. (2022b). Apolipoprotein E genotype-dependent nutrigenetic effects to prebiotic inulin for modulating systemic metabolism and neuroprotection in mice via gut-brain axis. Nutr. Neurosci. 25, 1669–1679. doi: 10.1080/1028415X.2021.1889452
Yang, C., Mogno, I., Contijoch, E. J., Borgerding, J. N., Aggarwala, V., Li, Z., et al. (2020). Fecal IgA levels are determined by strain-level differences in Bacteroides ovatus and are modifiable by gut microbiota manipulation. Cell Host Microbe 27, 467–475.e6. doi: 10.1016/j.chom.2020.01.016
Yang, T., Santisteban, M. M., Rodriguez, V., Li, E., Ahmari, N., Carvajal, J. M., et al. (2015). Gut dysbiosis is linked to hypertension. Hypertension 65, 1331–1340. doi: 10.1161/HYPERTENSIONAHA.115.05315
Yu, F., Feng, X., Li, X., Luo, Y., Wei, M., Zhao, T., et al. (2021). Gut-derived metabolite Phenylacetylglutamine and white matter Hyperintensities in patients with acute ischemic stroke. Front. Aging Neurosci. 13:675158. doi: 10.3389/fnagi.2021.675158
Keywords: healthy aging, microbiome, MRI, APOE, BMI, diabetes, calcium intake, brain metabolism
Citation: Hammond TC, Green SJ, Jacobs Y, Chlipala GE, Xing X, Heil S, Chen A, Aware C, Flemister A, Stromberg A, Balchandani P and Lin A-L (2023) Gut microbiome association with brain imaging markers, APOE genotype, calcium and vegetable intakes, and obesity in healthy aging adults. Front. Aging Neurosci. 15:1227203. doi: 10.3389/fnagi.2023.1227203
Received: 22 May 2023; Accepted: 17 August 2023;
Published: 06 September 2023.
Edited by:
Zhigang Liu, Northwest A&F University, ChinaCopyright © 2023 Hammond, Green, Jacobs, Chlipala, Xing, Heil, Chen, Aware, Flemister, Stromberg, Balchandani and Lin. This is an open-access article distributed under the terms of the Creative Commons Attribution License (CC BY). The use, distribution or reproduction in other forums is permitted, provided the original author(s) and the copyright owner(s) are credited and that the original publication in this journal is cited, in accordance with accepted academic practice. No use, distribution or reproduction is permitted which does not comply with these terms.
*Correspondence: Ai-Ling Lin, YWktbGluZy5saW5AaGVhbHRoLm1pc3NvdXJpLmVkdQ==
Disclaimer: All claims expressed in this article are solely those of the authors and do not necessarily represent those of their affiliated organizations, or those of the publisher, the editors and the reviewers. Any product that may be evaluated in this article or claim that may be made by its manufacturer is not guaranteed or endorsed by the publisher.
Research integrity at Frontiers
Learn more about the work of our research integrity team to safeguard the quality of each article we publish.