- 1Department of Neurology, The Affiliated Hospital of Jining Medical University, Jining, China
- 2Department of Neurology, China-Japan Friendship Hospital, Beijing, China
- 3Institute of Microbiology, Chinese Academy of Sciences, Beijing, China
- 4Department of Neurology, Sir Run Run Shaw Hospital, School of Medicine, Zhejiang University, Hangzhou, China
A key role of the gut microbiota in the pathogenesis of neurodegenerative diseases, such as Alzheimer’s disease (AD), has been identified over the past decades. Increasing clinical and preclinical evidence implicates that there is bidirectional communication between the gut microbiota and the central nervous system (CNS), which is also known as the microbiota-gut-brain axis. Nevertheless, current knowledge on the interplay between gut microbiota and the brain remains largely unclear. One of the primary mediating factors by which the gut microbiota interacts with the host is peripheral metabolites, including blood or gut-derived metabolites. However, mechanistic knowledge about the effect of the microbiome and metabolome signaling on the brain is limited. Neuroimaging techniques, such as multi-modal magnetic resonance imaging (MRI), and fluorodeoxyglucose-positron emission tomography (FDG-PET), have the potential to directly elucidate brain structural and functional changes corresponding with alterations of the gut microbiota and peripheral metabolites in vivo. Employing a combination of gut microbiota, metabolome, and advanced neuroimaging techniques provides a future perspective in illustrating the microbiota-gut-brain pathway and further unveiling potential therapeutic targets for AD treatments.
1. Introduction
Alzheimer’s disease (AD) is the most common form of dementia, leading to a globally heavy healthcare and economic burden (Jia et al., 2018, 2020). According to the National Institute on Aging and Alzheimer’s Association (NIA-AA) research framework, AD-related biomarkers are mainly classified into three existing groups, including amyloid-β (Aβ) deposition, pathologic tau aggregation, and neurodegeneration [AT(N)] (Jack et al., 2018; Scheltens et al., 2021). However, the failure or unsatisfactory results of recent clinical trials targeting Aβ clearance underscores the importance of understanding new disease-driving mechanisms and seeking other available disease-modifying therapies.
Gut microbial dysbiosis may serve as a key factor for developing AD (Cryan et al., 2020). A large number of studies have presented compelling evidence that there is bidirectional communication between the gut microbiota and brain (Cryan et al., 2019). Indeed, alterations in the composition and function of the gut microbiota have been reported both in human AD patients (Vogt et al., 2017; Zhuang et al., 2018; Li et al., 2019; Liu et al., 2019b; Sheng et al., 2021, 2022) and mouse models of AD (Zhang et al., 2017; Chen et al., 2020; Kim et al., 2020; Chen et al., 2022). However, knowledge about underlying mechanisms of the gut microbiota in regulating the brain of AD remain unclear. A growing evidence supports that AD appears to be a metabolic disease (Kaddurah-Daouk et al., 2013; Varma et al., 2018; Whiley et al., 2021). One of the primary mediating pathways by which the gut microbiota interacts with the host brain is via peripheral metabolites, which are small molecules that are derived from bacterial metabolism of the non-digestible carbohydrates, the products of cholesterol metabolism, or directly from gastrointestinal bacteria, etc (Luan et al., 2019; Lavelle and Sokol, 2020). Many metabolites play a series of roles in maintaining host health, including brain energy homeostasis, gut mucosal integrity, the host immune system and membrane lipid metabolism (Lavelle and Sokol, 2020). Gut microbiota can also give rise to metabolites, such as tryptophan derivatives and short-chain fatty acids (SCFAs), which may play an important role in modulating cognitive function and behavior (Silva et al., 2020; Doifode et al., 2021). Recently, advanced metabolomics techniques have been used to identify key AD-related metabolites and define changed metabolic pathways during AD disease trajectory (Toledo et al., 2017; Sun et al., 2020). Therefore, the integration of microbiome and metabolomics can help reveal the potential impact of altered microbial communities on host metabolism. Nevertheless, how do gut microbiome and peripheral metabolism impact brain health, and further cognitive function?
Interestingly, the advances in neuroimaging techniques may provide a promising approach to directly mirror the impact of the gut microbiota on human brain via the mediation role of peripheral metabolites (Cryan et al., 2019; Liu et al., 2019a; Arnoriaga-Rodríguez et al., 2020). Multi-modal magnetic resonance imaging (MRI), including structural MRI (sMRI), functional MRI (fMRI) and diffusion tensor imaging (DTI), as well as [18F] fluorodeoxyglucose-positron emission tomography (FDG-PET), have offered an avenue that allows non-invasively investigating abnormalities of brain structure, spontaneous functional activities and glucose metabolism in AD (Wang et al., 2020). Therefore, the combination of neuroimaging, microbiome and metabolomics, which is also called neuroimaging-omics, has the potential to identify multi-dimensional biological signatures essential to reveal the complex gut microbiota-metabolites-brain interaction mechanism.
In this review, we aim to summarize: (1) the microbiota-gut-brain axis and AD; (2) the potential mediator of the gut microbiota-brain pathway: peripheral (blood and gut) metabolites; (3) the integrative neuroimaging-omics method for studying AD. This review will facilitate a better understanding of the interplay between microbiota, metabolites and neurodegeneration along the gastrointestinal-brain axis in AD, possibly providing future targets for AD precision therapeutic modulation (see Table 1).
2. Microbiota-gut-brain axis and AD
Accumulating evidence suggests a close correlation between altered gut microbiota and brain, which is also called the microbiota-gut-brain axis. Alterations of the gut microbiota are associated with abnormal brain Aβ deposition in both AD animal models (Chen et al., 2020, 2022) and humans (Cattaneo et al., 2017; Sheng et al., 2022), and the transfer of a healthy fecal microbiota reduces Aβ and tau pathology in AD animal models (Kim et al., 2020). Gut microbiota-host interactions may lead to the release of numerous substances, such as cytokines, neurotransmitters, neuropeptides, and gut-derived metabolites, into the blood and lymphatic system. These substances can permeate into the brain through the blood brain barrier (BBB), or directly influence the neural messages transmission carried by the vagal and spinal afferent neurons (Cryan et al., 2019), which may further regulate brain function and behavior. Thus, targeting gut microbiota would provide a critical opportunity for therapeutic intervention of AD.
Recently, cross-sectional preclinical and clinical studies have revealed the significantly altered gut microbiota in AD. Compared with cognitively normal individuals, patients with AD and MCI showed significantly decreased microbial diversity and changed relative abundance of phylum Firmicutes and its corresponding bacteria (Vogt et al., 2017; Sheng et al., 2021, 2022). Similarly, amnestic mild cognitive impairment (MCI) patients also exhibited significant alterations in the microbial compositions, such as Gammaproteobacteria, Enterobacteriales and Enterobacteriaceae, the relative abundance of which were between cognitively normal adults and AD patients (Liu et al., 2019b). Additionally, one recent study reported the decreased anti-inflammatory genus Faecalibacterium in individuals with subjective cognitive decline (SCD), providing the preliminary evidence of altered gut microbiota in elderly adults at risk of AD (Sheng et al., 2021). For Aβ positive cognitively normal individuals, the relative abundance of phylum Bacteroidetes was enriched, whereas taxa in Firmicutes and Proteobacteria phyla were reduced (Sheng et al., 2022). Besides, the global brain Aβ burden was negatively associated with the Desulfovibrionaceae, Bilophila and Faecalibacterium, which confirmed the association of gut microbial dysbiosis with AD. Thus, gut microbiota may serve as a potential peripheral biomarker in identifying AD. Nevertheless, the regulatory mechanism of gut microbiota on the brain is unclear.
3. The potential mediator of the gut microbiota-brain pathway: peripheral metabolites
Currently, there is increasing interest in the interaction of gut microbiota with the brain in vivo. Metabolomics may provide an approach to understand why specific strains/probiotic interventions have the potential to modulate cognitive function. Metabolomics is an omics analysis that measures thousands of small molecule metabolites simultaneously (Luan et al., 2019; Lavelle and Sokol, 2020). Advances in high-throughput metabolomics techniques have provided new biochemical insights into disease mechanisms and revealed early systemic changes in disease. Given that the process of AD includes many biochemical changes, such as phosphorylation of tau, oxidative stress, and membrane lipid dysregulation (Toledo et al., 2017), the application of metabolomics may offer a promising perspective in AD diagnosis and functional pathway elucidation.
AD is now considered a metabolic dysfunction disease, with significant peripheral metabolic disturbances (Toledo et al., 2017; Varma et al., 2018; MahmoudianDehkordi et al., 2019; Arnold et al., 2020; Huo et al., 2020). Blood- and gut microbiota-derived metabolites are regarded as the potential peripheral metabolites, which may play the important role in microbiota-host crosstalk in AD. Lipid metabolism dysfunction is a hallmark in the whole spectrum of AD, with the declined level of fasting serum sphingomyelin (SM) and ether-containing phosphatidylcholines (PC) in the asymptomatic stage of AD, as well as the decreased acylcarnitines, valine and α-aminoadipic acid (α-AAA) mainly in the symptomatic stage of AD (Toledo et al., 2017). To our knowledge, ether-linked PCs and SMs are rich in the lipid rafts of the membrane. Lipid rafts are fluctuating assemblies composed of sphingolipid, cholesterol and proteins, forming platforms for membrane signaling and trafficking (Lingwood and Simons, 2010). In addition, accumulating evidence suggests that lipid rafts act as a common platform for the progression of AD pathology, which may be associated with amyloid precursor protein (APP) processing, Aβ accumulation and tau oligomers production (Rushworth and Hooper, 2010; Kawarabayashi et al., 2022). Therefore, changed lipid metabolism may provide a novel target for AD treatment. Moreover, current evidence from pre-clinical studies indicates that several lipid substances, such as bioactive sphingolipids, have the potential to modulate the gut microbiota and gut barrier function (Norris et al., 2016, 2019; Rohrhofer et al., 2021). For instance, intake of SMs shows protective properties against gut dysbiosis (Norris et al., 2019), indicating the interaction between gut microbiota and metabolites.
Short-chain fatty acids (SCFAs), as the most common form of gut microbiota-derived metabolites, also build a vital communication “bridge” between the gut and brain (Dalile et al., 2019; Colombo et al., 2021; Doifode et al., 2021; Ameen et al., 2022). About 90% SCFAs are derived from indigestible carbohydrates in the cecum and colon through gut microbial fermentation. SCFAs play a key role in the homeostasis of CNS by maintaining the integrity of intestinal epithelium barriers (Feng et al., 2018), regulating the metabolism of glucose and lipid (Ge et al., 2008; Canfora et al., 2015), and modifying immune function (Corrêa-Oliveira et al., 2016). Previous studies showed that the concentration of fecal SCFAs significantly declined in AD mice (Zhang et al., 2017). Wu et al. Wu et al. (2021) initially investigate the fecal metabolomics in the spectrum of AD, characterized by the progressively decreased trend of SCFAs from healthy controls to aMCI and AD patients. We speculate that the declined SCFAs level in AD may be due to the significant reduction of key SCFA-producing bacteria belonging to phylum Firmicutes, such as class Clostridia, order Clostridiales, family Ruminococcaceae and family Lachnospiraceae (Liu et al., 2019b; Sheng et al., 2021, 2022; Chen et al., 2023). Thus, SCFAs also exhibit the mediating impact between the gut microbiota and the brain.
4. An integrative neuroimaging-omics method for AD study
4.1. The framework of the neuroimaging-omics analysis
Neuroimaging techniques have the potential to reflect the microbiota-gut-brain interaction, and evaluate the effect of interventions targeting the gut microbiota (Tillisch et al., 2008; Tillisch and Labus, 2014). To date, several studies have explored the correlation between the gut and brain in other diseases using neuroimaging techniques (Wang et al., 2019; Arnoriaga-Rodríguez et al., 2020). For instance, a prior study has investigated the linkages of gut microbiota, systematic inflammation, amygdala-based functional connectivity, and cognitive performance in patients with end-stage renal disease. Causal mediation analysis revealed that the disrupted Roseburia indirectly regulated the amygdala-based functional connectivity through systematic tumor necrosis factor-alpha (Zheng et al., 2020). Tillisch and colleagues once reported that a four-week intake of a fermented milk product with probiotics by healthy women affected the activity of brain regions that control the central processing of emotion and sensation (Tillisch et al., 2013), providing direct evidence that intestinal bacteria can influence brain activity in healthy adults.
Currently, multimodal neuroimaging techniques, including sMRI, fMRI, DTI, and FDG-PET, can reveal subtle brain structural, functional, and glucose metabolism changes in AD (Wang et al., 2020). During the neuroimaging data preprocessing and analysis, multi-dimension features will be extracted, such as gray matter volume, amplitude of low frequency fluctuation (ALFF), fractional ALFF (fALFF), fractional anisotropy (FA), mean diffusivity (MD), etc. Using the radiomics method, high-throughput features (e.g., gray level histogram, texture, and wavelet transform) are also extracted from different brain tissues (Feng and Ding, 2020; Li et al., 2020), which will provide more precise evidence for the diagnosis of AD.
Advances in high-throughput sequencing have promoted the rapid development of microbiome research. Intestinal microbiome sequencing analyses mainly includes 16 ribosome DNA (rDNA) amplicon and metagenomic sequencing. 16S rDNA amplicon sequencing is the most commonly used method for neurological diseases. The advantages of 16S rDNA amplicon sequencing are cost effective, relatively small data amount, and quick analysis. However, it can only reach genus-level resolution, and it is sensitive to the specific primers and the number of PCR cycles (Liu et al., 2020). Metagenomic sequencing analysis, with higher taxonomic resolution (species- or strain-level) and potential functional information, will provide more microbial information than traditional 16S rDNA amplicon sequencing (Quince et al., 2017; Liu et al., 2020).
Peripheral metabolites (fecal and plasma/serum) exert diverse effects on host physiology and are likely to be the key mediators between the gut microbiota and brain. Thus, the combination of brain imaging techniques with microbiome and metabolomics will greatly enhance our understanding of the microbiota-gut-brain axis in regulating cognition in AD (Cryan et al., 2019). The proposed framework of the neuroimaging-omics analysis is shown in Figure 1.
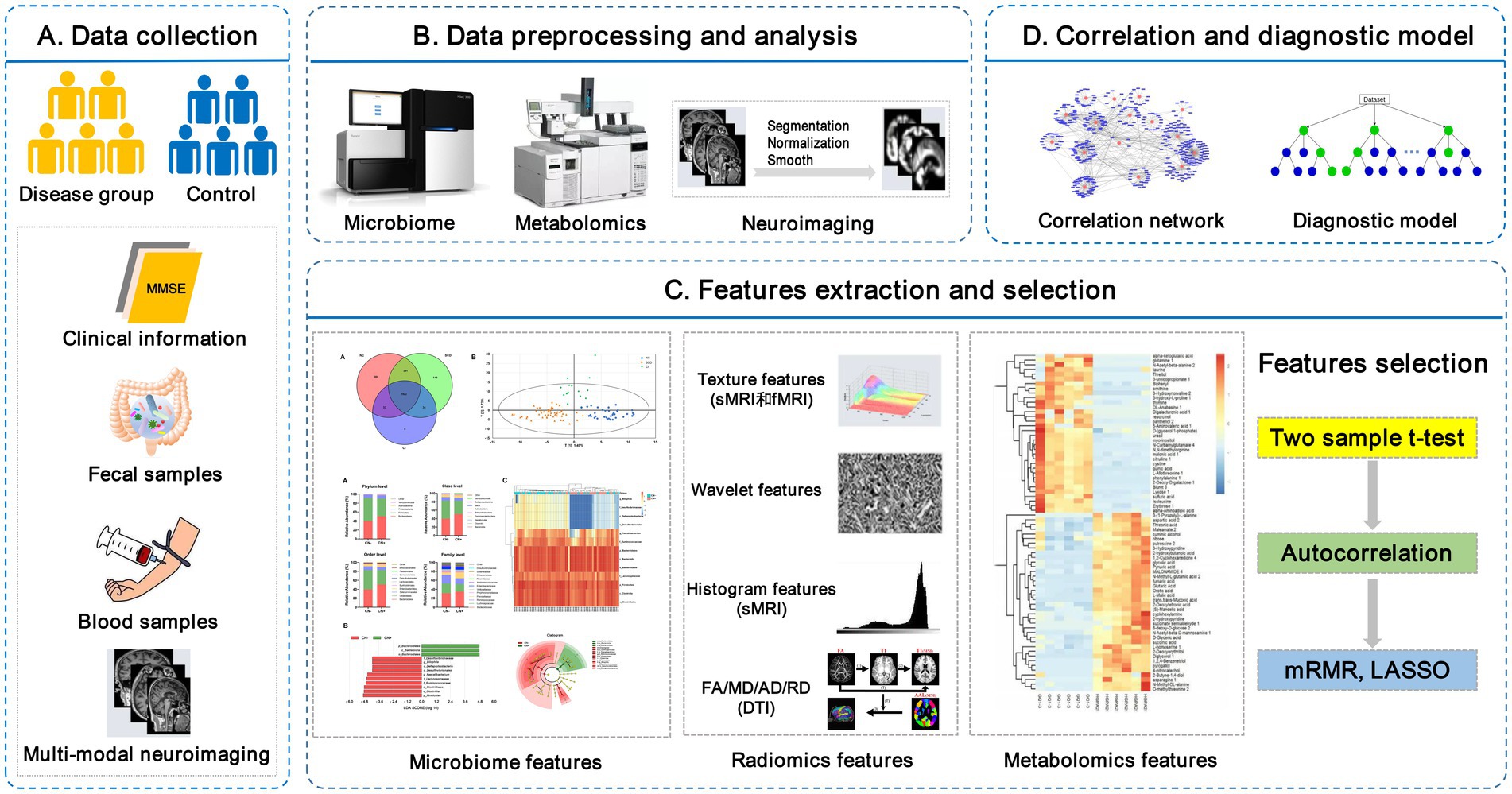
Figure 1. The framework of the neuroimaging-omics analysis. (A) Data collection: recruitment of the disease group and control group, collection of the clinical information, fecal samples, fasting blood samples, and multi-modal neuroimaging scan data. (B) Data preprocessing and analysis: including microbiome high-throughput sequencing, untargeted/targeted metabolomics, images segmentation, normalization, etc. (C) Features extraction and selection: key multi-omics features are extracted and further selected using some algorithms, such as Max-Relevance and Min-Redundancy (mRMR) and least absolute shrinkage and selection operator (LASSO). (D) Correlation and diagnostic model: the correlation network is used to investigate the complex relationship between different omics features, and the establishment of diagnostic models is based on the selected key multi-omics features. LASSO, least absolute shrinkage and selection operator; mRMR, Max-Relevance and Min-Redundancy; sMRI, structural MRI; fMRI, functional MRI; DTI, diffusion tensor imaging.
4.2. Neuroimaging and microbiome in AD
De Santis has provided the definition of radiomicrobiomics, which is an approach designed to extract quantitative parameters by combining brain imaging and gut microbiome (e.g., gut microbial composition, alpha diversity) (De Santis et al., 2019), mining these big data to develop diagnostic biomarkers of diseases and further elucidate the causal nature of the gut-brain interaction. Accumulating evidence supports that alterations of the gut microbiome are associated with brain structural and functional changes. Using structural MRI and 16S rRNA analysis, researchers have confirmed that specific gut microbial features are associated with cognitive dysfunction and decreased hippocampal volume (Saji et al., 2019; Liang et al., 2022). A cross-sectional study including AD, MCI and cognitively normal individuals found that the higher abundance of Lachnospiraceae NK4A136 group spp. and Anaerostipes spp. was correlated with lower global cortical atrophy visual scores (Verhaar et al., 2021). In addition, MCI patients with more Bacteroides were more likely to present with cortical and hippocampal atrophy (Saji et al., 2019). Resting-state fMRI can also build the crosstalk between the gut microbiota and the human brain. For instance, in patients with aMCI, gut microbiota, such as Bacteroides, Clostridiaceae, and Blautia, was confirmed to interact with intrinsic brain activity at different bands (Liu et al., 2021). Moreover, one study has investigated the effect of the probiotic strain Bifidobacterium breve MCC1274 (A1) on preventing brain atrophy in patients with MCI (Asaoka et al., 2022). The results showed that probiotics Bifidobacterium breve consumption for 24 weeks suppressed brain atrophy progression in MCI patients, validating that manipulating gut microbial compositions can ameliorate the pathogenesis of AD.
4.3. Neuroimaging and metabolomics in AD
An increasing amount of findings in vivo suggest that alterations of blood- and gut-derived metabolites, such as lipid metabolites, amino acids, bile acids, and SCFAs, may be closely linked to neuroimaging biomarkers of AD. Using targeted metabolomics, the declined concentrations of sphingomyelin (SM) and ether-containing phosphatidylcholines (PC) were associated with brain atrophy, suggesting that the dysfunction in lipid metabolism was linked to the brain morphological changes (Toledo et al., 2017). Female sex and APOE ε4 genotype are regarded as the major risk factors for AD. Interestingly, both APOE ε4 genotype and sex profoundly impact metabolism. Chang et al. revealed the sex- and APOE genotype-specific metabolic signatures in AD (Chang et al., 2023). Changes in amino acids were mainly in males, and PCs and tryptophan were in females. The APOE ɛ4 allele specifically affected the metabolism of PCs. In addition, Arnold et al. further confirmed that sex had the potential to modify the association of metabolites with AD-related biomarkers. A significant negative association between the level of palmitoleic acid (C16:1) and glucose uptake measured by FDG-PET was found mainly in the male group (Arnold et al., 2020). Thus, in future metabolomic studies, sex and APOE ɛ4 allele should be considered as confounding factors.
Peripheral metabolic changes may influence central abnormalities through the liver and gut-brain axis. Bile acids (BAs) appear to play a role in AD pathogenesis. When combining the structural MRI, [18F] FDG-PET and targeted metabolomics, Nho and colleagues found that bile acids levels were associated with hippocampal volume, cortical thickness and brain glucose metabolism in AD (Nho et al., 2019). For one primary bile acid metabolite, lower cholic acid (CA) levels were associated with decreased hippocampal volume and reduced glucose metabolism. Nevertheless, for bacterially produced conjugated secondary bile acid metabolites, including glycodeoxycholic acid (GDCA), glycolithocholic acid (GLCA), taurodeoxycholic acid (TDCA) and taurolithocholic acid (TLCA), higher levels were associated with decreased hippocampal volume and reduced glucose metabolism.
Currently, there was only one study combining fecal microbiome, targeted serum metabolomics and structural MRI data. Based on a large human cohort, the main finding was that Odoribacter was positively associated with hippocampal volume, which might be mediated by acetic acids (Liang et al., 2022). To date, the number of studies for revealing the interactions between gut microbiota, brain and cognition in humans is few. Future studies are needed to elucidate the effect of microbial modulation on cognition and behavior via the combination of metabolomics and neuroimaging.
5. Discussion and future perspectives
Although accumulating literatures support the contribution of gut microbial dysbiosis to the pathogenesis of AD, the complex interaction mechanisms involving the microbiota-gut-brain axis remain to be fully established. Peripheral metabolites may be a vital intermediate factor in communicating gut microbiota and the brain. Neuroimaging techniques can quantitatively mirror brain structural and functional changes in AD, which can directly reflect the effect of altered gut microbiota and metabolites on the human brain. Currently, most studies have profiled the association of altered microbiome or metabolites with neuroimaging parameters in patients with AD or MCI, whereas few studies focus on their causative correlations and the establishment of diagnostic models using integrated multi-omics techniques, especially in preclinical AD. In the future, the application of integrated neuroimaging-omics analysis has the potential for the identification of early AD. Notably, in the revised NIA-AA diagnostic criteria for AD,1 the value of plasma and inflammatory/immune biomarkers are also highlighted. Therefore, the combination of multi-omics indicators with new AD biomarkers may provide more diagnostic information for AD. In addition, longitudinal studies with a larger sample size from multiple centers are also needed, which may reveal the dynamic changes of these multi-omics biomarkers and further validate the robustness of findings. Moreover, the lack of data regarding neuroimaging modalities other than sMRI, DTI, and fMRI also deserves due attention. In conclusion, microbiota-gut-brain interactions may reveal novel insights into the mechanism of AD, and further provide the potential therapeutic target for AD interventions and treatments.
Author contributions
CS, XW, and SJ conceived the review. CS, WD, and XW drafted the initial manuscript. QD, XC, YL searched literatures. PX and CS revised the manuscript. All authors contributed to the article and approved the submitted version.
Funding
This article was supported by the National Natural Science Foundation of China (grant no. 81801052), the Natural Science Foundation of Shandong Province (grant no. ZR2023MH170), Science and Technology Development Plan Project of Shandong Medical and Health (grant no. 202203070774) and Doctoral Research Fund Project in the Affiliated Hospital of Jining Medical University (grant no. 2022-BS-02).
Conflict of interest
The authors declare that the research was conducted in the absence of any commercial or financial relationships that could be construed as a potential conflict of interest.
Publisher’s note
All claims expressed in this article are solely those of the authors and do not necessarily represent those of their affiliated organizations, or those of the publisher, the editors and the reviewers. Any product that may be evaluated in this article, or claim that may be made by its manufacturer, is not guaranteed or endorsed by the publisher.
Footnotes
References
Ameen, A. O., Freude, K., and Aldana, B. I. (2022). Fats, friends or foes: investigating the role of short- and medium-chain fatty acids in Alzheimer's disease. Biomedicine 10:10112778. doi: 10.3390/biomedicines10112778
Arnold, M., Nho, K., Kueider-Paisley, A., Massaro, T., Huynh, K., Brauner, B., et al. (2020). Sex and APOE ε4 genotype modify the Alzheimer's disease serum metabolome. Nat. Commun. 11:1148. doi: 10.1038/s41467-020-14959-w
Arnoriaga-Rodríguez, M., Mayneris-Perxachs, J., Burokas, A., Contreras-Rodríguez, O., Blasco, G., Coll, C., et al. (2020). Obesity impairs short-term and working memory through gut microbial metabolism of aromatic amino acids. Cell Metab. 32, 548–560.e7. doi: 10.1016/j.cmet.2020.09.002
Asaoka, D., Xiao, J., Takeda, T., Yanagisawa, N., Yamazaki, T., Matsubara, Y., et al. (2022). Effect of probiotic Bifidobacterium breve in improving cognitive function and preventing brain atrophy in older patients with suspected mild cognitive impairment: results of a 24-week randomized, double-blind, placebo-controlled trial. J. Alzheimers Dis. 88, 75–95. doi: 10.3233/JAD-220148
Canfora, E. E., Jocken, J. W., and Blaak, E. E. (2015). Short-chain fatty acids in control of body weight and insulin sensitivity. Nat. Rev. Endocrinol. 11, 577–591. doi: 10.1038/nrendo.2015.128
Cattaneo, A., Cattane, N., Galluzzi, S., Provasi, S., Lopizzo, N., Festari, C., et al. (2017). Association of brain amyloidosis with pro-inflammatory gut bacterial taxa and peripheral inflammation markers in cognitively impaired elderly. Neurobiol. Aging 49, 60–68. doi: 10.1016/j.neurobiolaging.2016.08.019
Chang, R., Trushina, E., Zhu, K., Zaidi, S. S. A., Lau, B. M., Kueider-Paisley, A., et al. (2023). Predictive metabolic networks reveal sex- and APOE genotype-specific metabolic signatures and drivers for precision medicine in Alzheimer's disease. Alzheimers Dement. 19, 518–531. doi: 10.1002/alz.12675
Chen, C., Ahn, E. H., Kang, S. S., Liu, X., Alam, A., and Ye, K. (2020). Gut dysbiosis contributes to amyloid pathology, associated with C/EBPβ/AEP signaling activation in Alzheimer's disease mouse model. Sci. Adv. 6:eaba0466. doi: 10.1126/sciadv.aba0466
Chen, C., Liao, J., Xia, Y., Liu, X., Jones, R., Haran, J., et al. (2022). Gut microbiota regulate Alzheimer's disease pathologies and cognitive disorders via PUFA-associated neuroinflammation. Gut 71, 2233–2252. doi: 10.1136/gutjnl-2021-326269
Chen, G., Zhou, X., Zhu, Y., Shi, W., and Kong, L. (2023). Gut microbiome characteristics in subjective cognitive decline, mild cognitive impairment and Alzheimer's disease: a systematic review and meta-analysis. Eur. J. Neurol. doi: 10.1111/ene.15961
Colombo, A. V., Sadler, R. K., Llovera, G., Singh, V., Roth, S., Heindl, S., et al. (2021). Microbiota-derived short chain fatty acids modulate microglia and promote Aβ plaque deposition. elife 10:59826. doi: 10.7554/eLife.59826
Corrêa-Oliveira, R., Fachi, J. L., Vieira, A., Sato, F. T., and Vinolo, M. A. (2016). Regulation of immune cell function by short-chain fatty acids. Clin Transl Immunology 5:e73. doi: 10.1038/cti.2016.17
Cryan, J. F., O'riordan, K. J., Cowan, C. S. M., Sandhu, K. V., Bastiaanssen, T. F. S., Boehme, M., et al. (2019). The microbiota-gut-brain Axis. Physiol. Rev. 99, 1877–2013. doi: 10.1152/physrev.00018.2018
Cryan, J. F., O'riordan, K. J., Sandhu, K., Peterson, V., and Dinan, T. G. (2020). The gut microbiome in neurological disorders. Lancet Neurol. 19, 179–194. doi: 10.1016/S1474-4422(19)30356-4
Dalile, B., Van Oudenhove, L., Vervliet, B., and Verbeke, K. (2019). The role of short-chain fatty acids in microbiota-gut-brain communication. Nat. Rev. Gastroenterol. Hepatol. 16, 461–478. doi: 10.1038/s41575-019-0157-3
De Santis, S., Moratal, D., and Canals, S. (2019). Radiomicrobiomics: advancing along the gut-brain Axis through big data analysis. Neuroscience 403, 145–149. doi: 10.1016/j.neuroscience.2017.11.055
Doifode, T., Giridharan, V. V., Generoso, J. S., Bhatti, G., Collodel, A., Schulz, P. E., et al. (2021). The impact of the microbiota-gut-brain axis on Alzheimer's disease pathophysiology. Pharmacol. Res. 164:105314. doi: 10.1016/j.phrs.2020.105314
Feng, Q., and Ding, Z. (2020). MRI Radiomics classification and prediction in Alzheimer's disease and mild cognitive impairment: a review. Curr. Alzheimer Res. 17, 297–309. doi: 10.2174/1567205017666200303105016
Feng, Y., Wang, Y., Wang, P., Huang, Y., and Wang, F. (2018). Short-chain fatty acids manifest Stimulative and protective effects on intestinal barrier function through the inhibition of NLRP3 Inflammasome and autophagy. Cell. Physiol. Biochem. 49, 190–205. doi: 10.1159/000492853
Ge, H., Li, X., Weiszmann, J., Wang, P., Baribault, H., Chen, J. L., et al. (2008). Activation of G protein-coupled receptor 43 in adipocytes leads to inhibition of lipolysis and suppression of plasma free fatty acids. Endocrinology 149, 4519–4526. doi: 10.1210/en.2008-0059
Huo, Z., Yu, L., Yang, J., Zhu, Y., Bennett, D. A., and Zhao, J. (2020). Brain and blood metabolome for Alzheimer's dementia: findings from a targeted metabolomics analysis. Neurobiol. Aging 86, 123–133. doi: 10.1016/j.neurobiolaging.2019.10.014
Jack, C. R., Bennett, D. A., Blennow, K., Carrillo, M. C., Dunn, B., Haeberlein, S. B., et al. (2018). NIA-AA research framework: toward a biological definition of Alzheimer's disease. Alzheimers Dement. 14, 535–562. doi: 10.1016/j.jalz.2018.02.018
Jia, J., Wei, C., Chen, S., Li, F., Tang, Y., Qin, W., et al. (2018). The cost of Alzheimer's disease in China and re-estimation of costs worldwide. Alzheimers Dement. 14, 483–491. doi: 10.1016/j.jalz.2017.12.006
Jia, L., Quan, M., Fu, Y., Zhao, T., Li, Y., Wei, C., et al. (2020). Dementia in China: epidemiology, clinical management, and research advances. Lancet Neurol. 19, 81–92. doi: 10.1016/S1474-4422(19)30290-X
Kaddurah-Daouk, R., Zhu, H., Sharma, S., Bogdanov, M., Rozen, S. G., Matson, W., et al. (2013). Alterations in metabolic pathways and networks in Alzheimer's disease. Transl. Psychiatry 3:e244. doi: 10.1038/tp.2013.18
Kawarabayashi, T., Nakamura, T., Sato, K., Seino, Y., Ichii, S., Nakahata, N., et al. (2022). Lipid rafts act as a common platform for amyloid-β oligomer-induced Alzheimer's disease pathology. J. Alzheimers Dis. 87, 1189–1203. doi: 10.3233/JAD-215662
Kim, M. S., Kim, Y., Choi, H., Kim, W., Park, S., Lee, D., et al. (2020). Transfer of a healthy microbiota reduces amyloid and tau pathology in an Alzheimer's disease animal model. Gut 69, 283–294. doi: 10.1136/gutjnl-2018-317431
Lavelle, A., and Sokol, H. (2020). Gut microbiota-derived metabolites as key actors in inflammatory bowel disease. Nat. Rev. Gastroenterol. Hepatol. 17, 223–237. doi: 10.1038/s41575-019-0258-z
Liang, X., Fu, Y., Cao, W. T., Wang, Z., Zhang, K., Jiang, Z., et al. (2022). Gut microbiome, cognitive function and brain structure: a multi-omics integration analysis. Transl Neurodegener 11:49. doi: 10.1186/s40035-022-00323-z
Li, B., He, Y., Ma, J., Huang, P., Du, J., Cao, L., et al. (2019). Mild cognitive impairment has similar alterations as Alzheimer's disease in gut microbiota. Alzheimers Dement. 15, 1357–1366. doi: 10.1016/j.jalz.2019.07.002
Lingwood, D., and Simons, K. (2010). Lipid rafts as a membrane-organizing principle. Science 327, 46–50. doi: 10.1126/science.1174621
Li, T. R., Wu, Y., Jiang, J. J., Lin, H., Han, C. L., Jiang, J. H., et al. (2020). Radiomics analysis of magnetic resonance imaging facilitates the identification of preclinical Alzheimer's disease: An exploratory study. Front. Cell Dev. Biol. 8:605734. doi: 10.3389/fcell.2020.605734
Liu, P., Jia, X. Z., Chen, Y., Yu, Y., Zhang, K., Lin, Y. J., et al. (2021). Gut microbiota interacts with intrinsic brain activity of patients with amnestic mild cognitive impairment. CNS Neurosci. Ther. 27, 163–173. doi: 10.1111/cns.13451
Liu, P., Peng, G., Zhang, N., Wang, B., and Luo, B. (2019a). Crosstalk between the gut microbiota and the brain: An update on neuroimaging findings. Front. Neurol. 10:883. doi: 10.3389/fneur.2019.00883
Liu, P., Wu, L., Peng, G., Han, Y., Tang, R., Ge, J., et al. (2019b). Altered microbiomes distinguish Alzheimer's disease from amnestic mild cognitive impairment and health in a Chinese cohort. Brain Behav. Immun. 80, 633–643. doi: 10.1016/j.bbi.2019.05.008
Liu, Y. X., Qin, Y., Chen, T., Lu, M., Qian, X., Guo, X., et al. (2020). A practical guide to amplicon and metagenomic analysis of microbiome data. Protein Cell 12, 315–330. doi: 10.1007/s13238-020-00724-8
Luan, H., Wang, X., and Cai, Z. (2019). Mass spectrometry-based metabolomics: targeting the crosstalk between gut microbiota and brain in neurodegenerative disorders. Mass Spectrom. Rev. 38, 22–33. doi: 10.1002/mas.21553
MahmoudianDehkordi, S., Arnold, M., Nho, K., Ahmad, S., Jia, W., Xie, G., et al. (2019). Altered bile acid profile associates with cognitive impairment in Alzheimer's disease-An emerging role for gut microbiome. Alzheimers Dement. 15, 76–92. doi: 10.1016/j.jalz.2018.07.217
Nho, K., Kueider-Paisley, A., Mahmoudiandehkordi, S., Arnold, M., Risacher, S. L., Louie, G., et al. (2019). Altered bile acid profile in mild cognitive impairment and Alzheimer's disease: relationship to neuroimaging and CSF biomarkers. Alzheimers Dement. 15, 232–244. doi: 10.1016/j.jalz.2018.08.012
Norris, G. H., Jiang, C., Ryan, J., Porter, C. M., and Blesso, C. N. (2016). Milk sphingomyelin improves lipid metabolism and alters gut microbiota in high fat diet-fed mice. J. Nutr. Biochem. 30, 93–101. doi: 10.1016/j.jnutbio.2015.12.003
Norris, G. H., Milard, M., Michalski, M. C., and Blesso, C. N. (2019). Protective properties of milk sphingomyelin against dysfunctional lipid metabolism, gut dysbiosis, and inflammation. J. Nutr. Biochem. 73:108224. doi: 10.1016/j.jnutbio.2019.108224
Quince, C., Walker, A. W., Simpson, J. T., Loman, N. J., and Segata, N. (2017). Shotgun metagenomics, from sampling to analysis. Nat. Biotechnol. 35, 833–844. doi: 10.1038/nbt.3935
Rohrhofer, J., Zwirzitz, B., Selberherr, E., and Untersmayr, E. (2021). The impact of dietary sphingolipids on intestinal microbiota and gastrointestinal immune homeostasis. Front. Immunol. 12:635704. doi: 10.3389/fimmu.2021.635704
Rushworth, J. V., and Hooper, N. M. (2010). Lipid rafts: linking Alzheimer's amyloid-β production, aggregation, and toxicity at neuronal membranes. Int. J. Alzheimers Dis. 2011:603052
Saji, N., Murotani, K., Hisada, T., Tsuduki, T., Sugimoto, T., Kimura, A., et al. (2019). The relationship between the gut microbiome and mild cognitive impairment in patients without dementia: a cross-sectional study conducted in Japan. Sci. Rep. 9:19227. doi: 10.1038/s41598-019-55851-y
Scheltens, P., De Strooper, B., Kivipelto, M., Holstege, H., Chételat, G., Teunissen, C. E., et al. (2021). Alzheimer's disease. Lancet 397, 1577–1590. doi: 10.1016/S0140-6736(20)32205-4
Sheng, C., Lin, L., Lin, H., Wang, X., Han, Y., and Liu, S. L. (2021). Altered gut microbiota in adults with subjective cognitive decline: the SILCODE study. J. Alzheimers Dis. 82, 513–526. doi: 10.3233/JAD-210259
Sheng, C., Yang, K., He, B., Du, W., Cai, Y., and Han, Y. (2022). Combination of gut microbiota and plasma amyloid-β as a potential index for identifying preclinical Alzheimer's disease: a cross-sectional analysis from the SILCODE study. Alzheimers Res. Ther. 14:35. doi: 10.1186/s13195-022-00977-x
Silva, Y. P., Bernardi, A., and Frozza, R. L. (2020). The role of short-chain fatty acids from gut microbiota in gut-brain communication. Front Endocrinol (Lausanne) 11:25. doi: 10.3389/fendo.2020.00025
Simpson, B. N., Kim, M., Chuang, Y. F., Beason-Held, L., Kitner-Triolo, M., Kraut, M., et al. (2016). Blood metabolite markers of cognitive performance and brain function in aging. J. Cereb. Blood Flow Metab. 36, 1212–1223. doi: 10.1177/0271678X15611678
Sun, C., Gao, M., Wang, F., Yun, Y., Sun, Q., Guo, R., et al. (2020). Serum metabolomic profiling in patients with Alzheimer disease and amnestic mild cognitive impairment by GC/MS. Biomed. Chromatogr. 34:e4875. doi: 10.1002/bmc.4875
Tillisch, K., and Labus, J. S. (2014). Neuroimaging the microbiome-gut-brain axis. Adv. Exp. Med. Biol. 817, 405–416.
Tillisch, K., Labus, J., Kilpatrick, L., Jiang, Z., Stains, J., Ebrat, B., et al. (2013). Consumption of fermented milk product with probiotic modulates brain activity. Gastroenterology 144, 1394–1401.e4. doi: 10.1053/j.gastro.2013.02.043
Tillisch, K., Wang, Z., Kilpatrick, L., Holschneider, D. P., and Mayer, E. A. (2008). Studying the brain-gut axis with pharmacological imaging. Ann. N Y. Acad. Sci. 1144, 256–264. doi: 10.1007/978-1-4939-0897-4_18
Toledo, J. B., Arnold, M., Kastenmüller, G., Chang, R., Baillie, R. A., Han, X., et al. (2017). Metabolic network failures in Alzheimer's disease: a biochemical road map. Alzheimers Dement. 13, 965–984. doi: 10.1016/j.jalz.2017.01.020
Varma, V. R., Oommen, A. M., Varma, S., Casanova, R., An, Y., Andrews, R. M., et al. (2018). Brain and blood metabolite signatures of pathology and progression in Alzheimer disease: a targeted metabolomics study. PLoS Med. 15:e1002482. doi: 10.1371/journal.pmed.1002482
Verhaar, B. J. H., Hendriksen, H. M. A., De Leeuw, F. A., Doorduijn, A. S., Van Leeuwenstijn, M., Teunissen, C. E., et al. (2021). Gut microbiota composition is related to AD pathology. Front. Immunol. 12:794519
Vogt, N. M., Kerby, R. L., Dill-Mcfarland, K. A., Harding, S. J., Merluzzi, A. P., Johnson, S. C., et al. (2017). Gut microbiome alterations in Alzheimer's disease. Sci. Rep. 7:13537. doi: 10.1038/s41598-017-13601-y
Wang, X., Huang, W., Su, L., Xing, Y., Jessen, F., Sun, Y., et al. (2020). Neuroimaging advances regarding subjective cognitive decline in preclinical Alzheimer's disease. Mol. Neurodegener. 15:55. doi: 10.1186/s13024-020-00395-3
Wang, Y. F., Zheng, L. J., Liu, Y., Ye, Y. B., Luo, S., Lu, G. M., et al. (2019). The gut microbiota-inflammation-brain axis in end-stage renal disease: perspectives from default mode network. Theranostics 9, 8171–8181. doi: 10.7150/thno.35387
Whiley, L., Chappell, K. E., D’Hondt, E., Lewis, M. R., Jiménez, B., et al. (2021). Metabolic phenotyping reveals a reduction in the bioavailability of serotonin and kynurenine pathway metabolites in both the urine and serum of individuals living with Alzheimer’s disease. Alzheimers Res. Ther. 13:20. doi: 10.1186/s13195-020-00741-z
Wu, L., Han, Y., Zheng, Z., Peng, G., Liu, P., Yue, S., et al. (2021). Altered gut microbial metabolites in amnestic mild cognitive impairment and Alzheimer’s disease: signals in host–microbe interplay. Nutrients 13:13. doi: 10.3390/nu13010228
Zhang, L., Wang, Y., Xiayu, X., Shi, C., Chen, W., Song, N., et al. (2017). Altered gut microbiota in a mouse model of Alzheimer's disease. J. Alzheimers Dis. 60, 1241–1257. doi: 10.3233/JAD-170020
Zheng, L. J., Lin, L., Zhong, J., Zhang, Z., Ye, Y. B., Zhang, X. Y., et al. (2020). Gut dysbiosis-influence on amygdala-based functional activity in patients with end stage renal disease: a preliminary study. Brain Imaging Behav. 14, 2731–2744. doi: 10.1007/s11682-019-00223-3
Keywords: Alzheimer’s disease, microbiome, metabolome, neuroimaging, microbiota-gut-brain axis
Citation: Sheng C, Du W, Liang Y, Xu P, Ding Q, Chen X, Jia S and Wang X (2023) An integrated neuroimaging-omics approach for the gut-brain communication pathways in Alzheimer’s disease. Front. Aging Neurosci. 15:1211979. doi: 10.3389/fnagi.2023.1211979
Edited by:
Kristy A. Nielson, Marquette University, United StatesReviewed by:
Brett Frye, Wake Forest University, United StatesAimee Parker, Quadram Institute, United Kingdom
Copyright © 2023 Sheng, Du, Liang, Xu, Ding, Chen, Jia and Wang. This is an open-access article distributed under the terms of the Creative Commons Attribution License (CC BY). The use, distribution or reproduction in other forums is permitted, provided the original author(s) and the copyright owner(s) are credited and that the original publication in this journal is cited, in accordance with accepted academic practice. No use, distribution or reproduction is permitted which does not comply with these terms.
*Correspondence: Can Sheng, Y2FueWV3ZWl3dTIwMTNAMTYzLmNvbQ==; Xiaoni Wang, d3huYW5uYUAxNjMuY29t