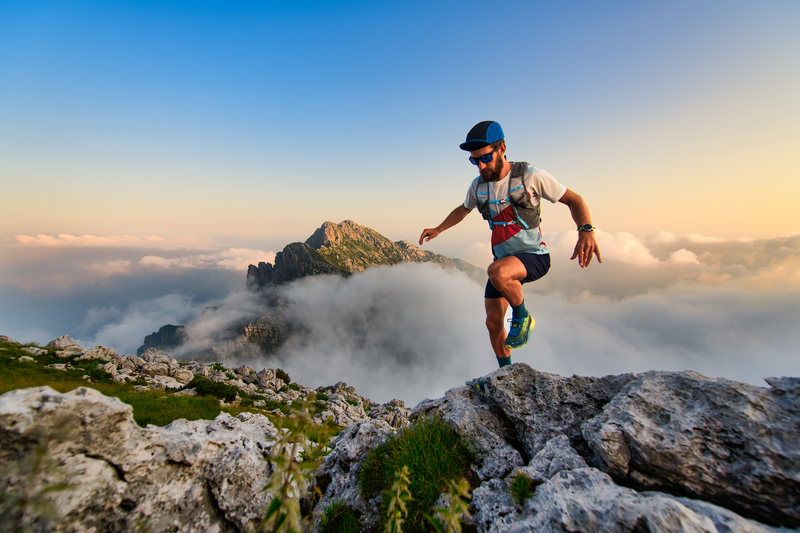
95% of researchers rate our articles as excellent or good
Learn more about the work of our research integrity team to safeguard the quality of each article we publish.
Find out more
MINI REVIEW article
Front. Aging Neurosci. , 17 July 2023
Sec. Alzheimer's Disease and Related Dementias
Volume 15 - 2023 | https://doi.org/10.3389/fnagi.2023.1150512
This article is part of the Research Topic Advancing Clinical Neuroscience by Multi-Omic Driven Approaches Towards Personalized Medicine: Opportunity, Challenges, and the Future View all 9 articles
Alzheimer’s disease (AD) is the most common form of neurological dementia, specified by extracellular β-amyloid plaque deposition, neurofibrillary tangles, and cognitive impairment. AD-associated pathologies like cerebral amyloid angiopathy (CAA) are also affiliated with cognitive impairment and have overlapping molecular drivers, including amyloid buildup. Discerning the complexity of these neurological disorders remains a significant challenge, and the spatiomolecular relationships between pathogenic features of AD and AD-associated pathologies remain poorly understood. This review highlights recent developments in spatial omics, including profiling and molecular imaging methods, and how they are applied to AD. These emerging technologies aim to characterize the relationship between how specific cell types and tissue features are organized in combination with mapping molecular distributions to provide a systems biology view of the tissue microenvironment around these neuropathologies. As spatial omics methods achieve greater resolution and improved molecular coverage, they are enabling deeper characterization of the molecular drivers of AD, leading to new possibilities for the prediction, diagnosis, and mitigation of this debilitating disease.
Alzheimer’s disease (AD) is the most common form of neurological dementia, with an estimated 6.07 million adults in the United States diagnosed with the disease by 2020 and a predicted 13.85 million adults by 2060 (Rajan et al., 2021). A progressive disability, initial symptoms of AD include mild memory loss, followed by gradually increasing symptoms of disorientation, mood and behavior change, and deepening confusion while the disease spreads throughout regions of the brain (Rajan et al., 2021). The characteristic neuropathologies associated with AD include neuritic plaques consisting of extracellular β-amyloid deposits and neurofibrillary tangles (NFTs) composed of aggregated hyperphosphorylated tau protein (p-tau) (Long and Holtzman, 2019). Unfortunately, most drug candidates in the last few decades targeting β-amyloid and p-tau failed to show clinical efficacy. One exception is lecanemab, a monoclonal antibody that targets β-amyloid and modestly slows AD progression, which was recently approved by the FDA. However, there are still no preventative treatments for AD (van Dyck et al., 2022). The failure of many of these putative therapeutic candidates coincides with the lack of understanding of the molecular underpinnings of AD onset and progression. Therefore, more research into the complex and heterogeneous nature of AD is necessary to better treat this disease.
There are several emerging but less-understood AD-associated pathologies, including cerebral amyloid angiopathy (CAA), dystrophic neurites, neuropil threads, granulovacuolar degenerating bodies, and gliosis (Long and Holtzman, 2019). CAA occurs when toxic β-amyloid deposits form within cerebral arterioles and capillaries in the central nervous system leading to cognitive impairment and intracerebral hemorrhage (Biffi and Greenberg, 2011; van Etten et al., 2014; Boyle et al., 2015). Dystrophic neurites contain an accumulation of dysfunctional lysosomes within distending axons that surround β-amyloid plaques, while granulovacuolar degenerating bodies are pathologic neuronal organelles with lysosome-related proteins thought to be clogged autophagosomes (Schrag et al., 2020). In addition, astrocytes and microglia surrounding neural tissue demonstrate forms of gliosis in AD. Despite the varied molecular and cellular drivers of these localized sites of dysfunction, each is highly correlated with AD. For example, CAA is reported to occur in 82 to 98% of AD patients (Attems and Jellinger, 2014). Additional studies have demonstrated that CAA correlates to neurodegenerative disease and cognitive impairments, more generally (Debette and Markus, 2010; Schrag et al., 2010; Carare et al., 2014; Lee et al., 2018). Researchers also suggest that defective neuronal endolysosomes accumulating within dystrophic neurites surrounding β-amyloid plaques might have a molecular interaction with the autophagic organelles in granulovacuolar degenerating bodies (Schrag et al., 2020). While these contributory pathologies are observed frequently among patients with AD, the heterogeneous nature and interactions between them remain unclear. Traditional multi-omic strategies, which include integrated genomics, epigenomics, transcriptomics, proteomics, and metabolomics/lipidomics studies, have identified AD-associated molecular drivers and biomarkers of the neuropathologies mentioned above (Badhwar et al., 2020; Johnson et al., 2020; Clark et al., 2021, 2022; François et al., 2022; Gao et al., 2022; Kodam et al., 2023). Still, future analyses must discern the spatial relationship and interconnectedness between AD biomarkers, cell types, and contributing pathologies in order to develop more precise therapeutics. Recent technological advances in spatial omics can illuminate these meaningful relationships across time and space. This review will focus on novel spatial multi-omics technologies, comparing spatial molecular profiling strategies with molecular imaging (See Table 1). Close attention will be paid to imaging mass spectrometry (IMS) as a modality primed to help untangle the spatial complexity of Alzheimer’s disease.
Understanding the complex and concerted molecular drivers of diseases such as AD requires insight into how cellular neighborhoods and molecular distributions are altered near sites of dysfunction. Spatial profiling experiments achieve this by performing omics measurements on specific tissue structures, cell types, and/or single cells through discrete surface sampling approaches (Moffitt et al., 2022). These spatially derived samples are then analyzed using advanced omics technologies, including next-generation sequencing or liquid chromatography-mass spectrometry (LC-MS), Moffitt et al. (2022) producing generate deep molecular profiles (i.e., hundreds to thousands of molecular species) that span a wide range of molecular classes. Profiling experiments often sacrifice spatial information by collecting data from larger tissue areas (>100 μm2) or from dispersed cells to allow for enough material to maximize molecular coverage, sensitivity, and dynamic range. This contrasts with imaging approaches that provide more complete spatial distributions at higher resolution, often with diminished molecular coverage and sensitivity. For Alzheimer’s disease, this approach can be used target neuropathological foci such as neuritic plaques or NFTs to understand better how these features change cellular interactions and biomolecular mechanisms in situ.
Spatial profiling methods can be coupled to proteomics workflows to determine the abundance of proteins produced or modified at specific locations in tissue. Often spatial proteomics experiments are performed in tandem with complementary transcriptomics experiments allowing for relationships between gene expression and protein abundance to be better understood (Gry et al., 2009). Spatial proteomics utilizing mass spectrometry (MS) is advantageous over other approaches, such as antibody-based techniques, because it enables untargeted, rapid, highly specific analysis of thousands of proteins and proteoforms (Bai et al., 2021). Additionally, it does not require any a priori information. It is optimal for detecting post-translational modifications (PTMs) that often cannot be observed with antibody protein labeling techniques, which bind to a specific epitope (Bai et al., 2021). These highly specific MS-based methods are important when assessing molecular heterogeneity associated with neuropathologies as PTMs have been found to play an important role in Alzheimer’s disease (Deture and Dickson, 2019). For example, hyperphosphorylated tau is a hallmark feature of neuropil threads and neurofibrillary tangles, which correlate to disease severity (Giannakopoulos et al., 2003). One strategy for producing spatially resolved LC-MS results is to utilize laser capture microdissection (LCM). This is a standard method that uses a cutting laser to isolate discrete tissue regions (>10 μm in diameter) for MS analysis (Datta et al., 2015). Isolated tissue regions are then prepared and analyzed using of proteomics, lipidomics, and metabolomics (Drummond et al., 2018; Knittelfelder et al., 2018). In one study, researchers quantified ∼900 proteins in plaques and ∼500 proteins in NFTs in human Alzheimer’s tissue using LCM followed by LC-MS (Hughes et al., 2014). Recent advances have been made to maximize tissue collection efficiency for LCM experiments, improving the overall sensitivity and molecular coverage of the workflow. Many of these new methods utilize single tubes or droplets for sample collection and processing, which minimizes sample loss and reduces the total amount of tissue needed for the subsequent analyses (Hughes et al., 2014; Kulak et al., 2014; Moggridge et al., 2018). Techniques like NanoPOTS (Nanodroplet Processing in One pot for Trace Samples) employ a robotic/microfluidic platform that collects tissue and performs processing steps in ∼200 nL droplets in order to improve overall sensitivity and map protein expression at higher spatial resolutions resolution (Xu et al., 2019). Another profiling approach used to identify proteins in a particular tissue region while maintaining spatial integrity is micro-liquid extraction surface analysis (microLESA) (Ryan et al., 2019; Guiberson et al., 2021). This technique utilizes a piezo-electric spotter to deposit nanoliter droplets of trypsin onto defined tissue regions, allowing for bottom-up proteomics to be performed from foci as small as 80 μm (Ryan et al., 2019; Guiberson et al., 2021). MicroLESA can be performed more rapidly than LCM but with reduced spatial resolution. The trajectory of spatial molecular profiling toward smaller detection areas puts these technologies in a unique position to discover altered pathways and signaling networks associated with neuropathogenic structures like neuritic plaques and NFTs, which require cell-type or even single-cell differentiation.
There are also emerging profiling-based spatial transcriptomics technologies that provide insights into localized gene expression in targeted cells and tissue features (Lovatt et al., 2014; Nichterwitz et al., 2018; Chen et al., 2020; Merritt et al., 2020; Qian et al., 2020; Rao et al., 2021; Walker et al., 2022). Traditional single-cell and single-nuclei RNA sequencing approaches (scRNA-seq/snRNA-seq) enable cell phenotyping by generating deep molecular information for individual cells but do not provide spatial context (Rao et al., 2021). LCM coupled with sequencing workflows (LCM-seq) offers the ability to target tissue regions while allowing for the flexibility to perform most sequencing approaches. For example, LCM-seq has been used to perform polyA-based RNA sequencing on individual neurons, preserving information regarding the location of each cell relative to the tissue environment (Nichterwitz et al., 2018). Similar to proteomics, this approach has challenges associated with tissue loss and collection efficiency during the LCM process. A different tissue profiling approach has been commercialized by NanoString using a platform called the GeoMx digital spatial profiler (Merritt et al., 2020). This technology uses RNA probes bound to oligo barcodes using a UV-cleavable linker. Probes are hybridized to endogenous RNA in tissue sections, the barcodes are released from targeted tissue regions using focused UV light, and those barcodes are aspirated and sequenced using next-generation sequencing. When coupled with immunohistochemistry, this technology allows for highly precise and accurate targeting of specific cell types and tissue features while assessing expression for >18,000 genes in human and murine tissues. The GeoMx digital spatial profiler is already being employed in the study of AD. For example, Walker et al. (2022) used a cocktail of 86 antibodies conjugated to unique UV-photocleavable oligonucleotide tags, allowing researchers to determine differential gene expression in hippocampal regions between dementia patients with AD neuropathological changes and cognitively normal patients (termed resilient) with AD neuropathological changes (Figures 1A, B). Although powerful, this approach is limited to studies of species of which probe sets are available. Currently, nanoString only offers probe sets for the human and mouse genome. Overall, transcriptomic spatial profiling techniques have advanced significantly over a short period of time. They will undoubtedly become more prominent in understanding transcript-level alterations at sites of AD-associated pathologies. With the ability to analyze a wide range of molecular classes and link observations to specific tissue features and cell types, spatial molecular profiling technologies are well-positioned to help decipher the complexity associated with AD and related diseases.
Figure 1. Spatial profiling and imaging technologies used in brain studies. (A,B) The GeoMx platform allows for highly multiplexed analysis of gene and protein expression [modified from Walker et al. (2022)]. (A) The Hippocampal CA1 subregion labeled with morphology markers (AT8, green; β-amyloid, aqua; IBA-1, red; nuclear marker SYTO13, blue) to highlight targeted NFTs and surrounding areas. (B) Heatmap of differentially expressed proteins when comparing NFTs to normal neurons (p < 0.01). (C) Representative highly multiplexed CycIF images of astroscytes from a AD donor highlighting ALDH1L1, EAAT2, GFAP, and Aβ [modified from Muñoz-Castro et al. (2022)]. (D) MALDI imaging mass spectrometry lipid data from murine brain collected at 10 μm spatial resolution. Selected images include m/z 834.5437 ([PS(40:6)-H]–, –0.12 ppm error), m/z 883.5683 ([PI(38:5)-H]–, –0.45 ppm error), m/z 885.5498 ([PI(38:4)-H]–, –0.23 ppm error), and m/z 906.6431 ([SHexCer(t42:1)-H]–, –1.9 ppm error) [modified from Spraggins et al. (2019)].
Molecular imaging combines the spatial characteristics of traditional histology with the molecular specificity of modern omics technologies. Although imaging approaches provide reduced sensitivity and coverage compared to bulk or profiling measurements, they more completely capture the spatial context of biomolecular processes in situ. This is critically important for complex diseases like AD as localized disorder of molecular and cellular neighborhoods often drive disease pathology (Mrdjen et al., 2019).
Revealing spatial distributions of proteins in biological tissue is traditionally driven by antibody-based technologies, including immunohistochemistry (IHC) and immunofluorescence (IF), providing the ability to map targeted proteins at high spatial resolution (Weissleder, 2002; Goltsev et al., 2018; Saka et al., 2019; Black et al., 2021; Hickey et al., 2022; Murray et al., 2022; Muñoz-Castro et al., 2022; Neumann et al., 2022; Wang et al., 2022). IF allows the detection of multiple protein targets, but plexity is typically limited to ∼4 to 7 markers due to spectral overlap of the emission spectra for individual fluorochromes (Tsurui et al., 2000). However, new approaches have been developed that enable highly multiplexed antibody-based imaging. Cyclic IF (CycIF) uses cycles of antibody staining and stripping to produce high plexity molecular images, typically with 3–5 markers in each cycle. An 8-cylce cyclic IF workflow allowed researchers to discover a new third state of glial cells found near plaques and tangles (Muñoz-Castro et al., 2022; Figure 1C). This state was hypothesized to be an “intermediate” cell population between homeostatic and reactive microglia. Murray et al. (2022) have developed an optimized CycIF workflow for brain tissue using commercially available primary and secondary antibodies termed multiplexed fluorescence-based immunohistochemistry (MP-IHC). They used this approach to screen 100 markers in the olfactory bulb from post-mortem tissues of Alzheimer’s disease and Parkinson’s disease patients. Leveraging pixel-wise unsupervised machine learning, the resulting multiplexed imaging data were used to segment anatomical features and group samples based on disease type (Murray et al., 2022). The ability to distinguish specific cell types and states is essential for understanding how cellular neighborhoods are altered at sites of AD neuropathology. However, most CycIF strategies are performed manually and are prone to tissue loss during the cycling steps.
Researchers are also working to improve robustness and throughput for multiplexed protein imaging. Although yet to be applied to the study of AD, one approach that is gaining traction is co-detection by indexing (CODEX) IF microsocopy (Goltsev et al., 2018; Saka et al., 2019; Black et al., 2021; Hickey et al., 2022; Neumann et al., 2022). CODEX is performed using DNA-conjugated antibodies and then cycling the fluorescently labeled complementary oligo barcodes allowing for >50 markers to be imaged from a single tissue section. One drawback of CODEX is the preparation and validation required to conjugate primary antibodies. However, the process is less prone to tissue loss and more amenable to cycling, increasing the number of markers that can be imaged reproducibly. There are also MS-based approaches that use antibodies labeled with elemental mass reporters, such as cytometry time-of-flight (CyTOF) (Wang et al., 2022) imaging and multiplexed ion beam imaging by time-of-flight (MIBI-TOF) (Vijayaragavan et al., 2022). With MIBI, a high-energy ion beam is used to sample the tissue surface, sputtering elemental reporters, and detecting the reporters using a TOF mass spectrometer at each pixel location. Using MIBI, authors validated and imaged 36 brain-abundant targets while comparing healthy and AD hippocampus tissue regions (Vijayaragavan et al., 2022). The results of this study identified persistent neurons expressing MFN2 that were adjacent to NFTs, suggesting that this mitochondrial membrane protein carries a survival advantage for neurons evading tau-induced pathology (Vijayaragavan et al., 2022). MS-based technologies allow for higher plexity within a single scan without the challenges of overcoming overlapping fluorescence emission bands. However, these approaches are typically lower throughput, limited to only imaging small tissue areas, and completely ablate the tissue eliminating the ability for subsequent experiments. On the other hand, fluorescence microscopy-based approaches require complex cycling methods to achieve high plexity but allow for whole-slide imaging.
Imaging mass spectrometry (IMS) is another multiplexed molecular imaging technology that enables untargeted mapping of proteins, glycans, lipids, and metabolites (Caprioli et al., 1997; Spengler and Hubert, 2002; Gode and Volmer, 2013; Norris and Caprioli, 2013; Wu et al., 2013; Nilsson et al., 2015; Spraggins et al., 2019; Djambazova et al., 2020, 2023). This label-free approach can simultaneously detect 100s to 1,000s of endogenous biomolecules in a pixel-wise manner, generating molecular tissue maps (Caprioli et al., 1997). Although numerous IMS technologies exist, matrix-assisted laser desorption/ionization (MALDI) and desorption electrospray ionization (DESI) have most extensively been applied to AD. MALDI IMS utilizes an applied chemical matrix and laser ablation to desorb and ionize analyte molecules from tissue (Norris and Caprioli, 2013; Figure 1D). Significant advances in laser and stage technologies have allowed for higher spatial resolution (≤10 μm pixel size) (Spraggins et al., 2019), in some cases ablating tissue areas of less than 1 μm (Spengler and Hubert, 2002). This makes MALDI IMS a powerful tool for studying changes associated with Alzheimer’s disease at cellular resolution. Recent studies targeting the tissue microenvironment of amyloid plaques have observed various molecular signatures, distinct lipid accumulations, and even monitored Aβ-peptide content within and around β-amyloid plaques (Casadonte et al., 2015; Kaya et al., 2018; Michno et al., 2018, 2021, 2022; Kelley et al., 2020; Koutarapu et al., 2022). The Hanreider group used MALDI combined with plaque staining to identify molecular heterogeneous plaques in a mouse model of AD and identified differential lipid signatures across multiple stages of plaque development (Michno et al., 2022). For example, the data revealed inositol phospholipids, lyso-phosphatidylinositols, and ganglioside (i.e., GM2 and GM3) lipids localized to immature AD plaques. Whereas phosphatidylethanolamines and phosphatidic acids were found in higher abundance in the core regions of mature plaques. Their research has revealed the vast molecular heterogeneity during plaque development. DESI IMS is another common ionization source for performing IMS experiments. DESI is performed by spraying charged droplets onto the tissue surface, which dissolve endogenous molecules and are deflected into the mass spectrometer inlet (Ifa et al., 2010; Eberlin et al., 2011). This approach allows for rapid analysis due to not requiring specific sample preparations; however, spatial resolution is typically limited to 30–50 μm pixel sizes (Qi et al., 2021). DESI IMS is especially effective for imaging lipids (Roach et al., 2010; Chew et al., 2020; Zhang et al., 2021), which are implicated in the progression of Alzheimer’s disease. One study incorporating DESI IMS detected significant changes in glycerophospholipid metabolism in correlation with the early stages of AD (Zhang et al., 2021). Finally, secondary ion mass spectrometry (SIMS) (Wu and Odom, 1996) is being utilized for high resolution molecular imaging studies of AD (Lazar et al., 2013). SIMS utilizes a focused ion beam allowing for pixel sizes of 100 nm–1 μm (Wu and Odom, 1996). However, depending on the ion beam used, fragmentation of endogenous molecules can occur during desorption and ionization, typically limiting SIMS analysis to low molecular weight species (Heeren et al., 2006). One example application of SIMS to AD found heterogeneous distributions of cholesterol in the cerebral cortex (Lazar et al., 2013). They found a significant increase in cholesterol signal in the external granular layer/external pyramidal layer and the internal granular layer (Lazar et al., 2013). Advances to SIMS, such as surface modification, matrix-enhanced SIMS and metal-assisted SIMS, and polyatomic ions, are helping to expand the applicability of SIMS to a broader range of molecular classes (Wu and Odom, 1996; Heeren et al., 2006; McDonnell et al., 2006).
Similar to profiling technologies, novel imaging approaches have been developed for decoding localized gene expression in relation to cell types and tissue features (Chen et al., 2015; Rodriques et al., 2019; Stickels et al., 2021; Lebrigand et al., 2023). Techniques such as Slide-seq and Slide-seqV2 provide genome-wide expression information at high spatial resolution by capturing RNA onto a surface covered with DNA-barcoded microbeads (Rodriques et al., 2019; Stickels et al., 2021). This approach has been used to map gene expression in the cerebellum and hippocampus from murine brain tissues (Rodriques et al., 2019; Stickels et al., 2021). Another useful method for imaging RNAs is multiplexed error-robust fluorescence in situ hybridization (MERFISH), which detected ∼1,000 RNA species within a single cell (Chen et al., 2015). Sequential imaging details genes that are co-expressed and co-regulated in various tissue features. This technique was applied to the primary motor cortex of a mouse, encompassing the transcriptomic information of 300,000 cells and their spatial orientations. Furthermore, in situ sequencing technologies are being advanced by commercial vendors like 10x genomics, whose Visium platform performs Spatial Isoform Transcriptomics (SiT), which was able to collect full-length transcript sequences from mouse brain tissue while maintaining spatial context (Lebrigand et al., 2023). Many research groups are working to improve sensitivity, molecular coverage, and spatial resolution for transcriptomic imaging. As spatial transcriptomics technologies advance, these innovative imaging strategies will undoubtedly be applied to AD and associated pathologies to better understand how cellular neighborhoods and biomolecular pathways are altered in normal aging and diseased tissues.
The rapid development of spatial molecular profiling and imaging technologies gives scientists a new toolbox for addressing complex diseases at a systems level. Elucidating the interconnectedness between the cellular and molecular organization of neuropathologies associated with Alzheimer’s disease have the potential to bring about breakthroughs in the prediction, detection, and treatment of AD. These breakthroughs are being driven by improvements in the microscopy, mass spectrometry, and transcriptomics assays described here, but advancements are being accelerated through the development of integrated technologies that synergistically combine these assays into multimodal workflows. Multimodal spatial omics experiments provide the ability to understand relationships between a broad range of molecular classes (e.g., RNAs, proteins, lipids, and metabolites) across a wide range of spatial scales (e.g., whole organs to single cells). This will enable a deeper understanding of how cellular neighborhoods and molecular distributions are reorganized around specific neuropathologies found in different tissue microenvironments. In addition to advanced analytical capabilities, this requires novel computational approaches for processing, integrating, and analyzing data from multiple imaging modalities. The future of Alzheimer’s disease research will certainly rely heavily on machine learning to integrate and mine information-rich molecular imaging technologies in both 2- and 3-dimensional space.
Computational strategies to integrate multi-omic information are already providing novel insights into the molecular underpinnings of AD. One study combined transcriptomics, proteomics, and epigenomics approaches to reveal histone modifications specific to AD (Nativio et al., 2020). Using STRING (Search Tool for Retrieval of Interacting Genes/Proteins) analysis, which draws from a protein-protein interaction database and is visualized using the Cytoscape software, researchers showed how epigenetic dysregulation could be a target for early stage AD prevention (Nativio et al., 2020). Taga et al. (2020) used regression analysis to integrate immunohistochemistry data from post-mortem brain tissue with mRNA expression levels. They discovered that BIN1 protein isoforms are differentially expressed in neuronal cell types. In particular, decreased expression of BIN1 isoforms containing exon 7 is associated with a greater accumulation of tangles and subsequent cognitive decline. Researchers have also integrated spatial omics to improve spatial resolution, which is especially important for analyzing smaller features of AD, like plaques and neurofibrillary tangles. One study utilizing a supervised machine learning method called deep data fusion integrated spatial transcriptomics and histology, which allowed for the characterization of the transcriptome of a mouse olfactory bulb on a micrometer scale (Bergenstråhle et al., 2022). Analogous approaches have been applied to IMS data to spatially “sharpen” ion images through data-driven image fusion with microscopy using highly multivariate linear regression (Van de Plas et al., 2015). This method can predict ion distributions with up to 10× higher spatial resolution and in tissue areas not measured by IMS. In the context of AD, where pathological features like plaques and NFTs are on the order of 1–20 um, achieving cellular resolution with the aid of computational tools like image fusion will be extremely valuable. Other supervised machine learning approaches are being used for classification tasks to allow for data-driven recognition of diseased tissues (Lazova et al., 2012; Meding et al., 2012; Hanselmann et al., 2013; Casadonte et al., 2014; Veselkov et al., 2014; Verbeeck et al., 2020). Tideman et al. (2021) recently have shown that supervised and interpretable machine learning could be used for biomarker discovery when applied to untargeted, highly multiplexed MALDI IMS datasets, including for central nervous system tissue. On the other hand, unsupervised machine learning with factorization, clustering, and manifold learning methods are being used for exploratory analysis and interpretation of high dimensionality spatial omics datasets (Verbeeck et al., 2020).
Spatial profiling and imaging is a rapidly expanding field that will continue to see growth. On one end, assays will see further developments as techniques such as imaging mass spectrometry and spatial transcriptomics will achieve greater resolution and become capable of detecting a wider range of molecules. On the other end, data integration and mining with machine learning are now regularly deployed and becoming increasingly necessary for working with large-scale multimodal molecular imaging data. The ability to capture relationships between data modalities in mathematical models and open them for biological interpretation will offer new opportunities for spatially specific biomarker discovery. Using the spatial dimension to connect multi-omic studies will these integrated technologies to be harnessed to reveal how cellular organization and molecular distributions are altered during AD. This will be critical for untangling the mechanisms underlying the diverse array of AD-associated neuropathologies and informing future therapeutic strategies.
CM, MF, KD, and JS conceptualized and wrote the manuscript. All authors contributed to the article and approved the submitted version.
The authors gratefully acknowledge support from the NIH National Institute on Aging (NIA) (R01AG078803 awarded to JS). KD was supported by the National Institute of Diabetes and Digestive and Kidney Diseases (NIDDK) training grant T32DK007569.
We also thank Drs. Martin Dufresne and Elizabeth Neumann from the Spraggins research group at Vanderbilt University for generating CODEX and MALDI IMS brain images highlighted in Figure 1.
The authors declare that the research was conducted in the absence of any commercial or financial relationships that could be construed as a potential conflict of interest.
All claims expressed in this article are solely those of the authors and do not necessarily represent those of their affiliated organizations, or those of the publisher, the editors and the reviewers. Any product that may be evaluated in this article, or claim that may be made by its manufacturer, is not guaranteed or endorsed by the publisher.
AD, Alzheimer’s disease; CAA, cerebral amyloid angiopathy; CODEX, Co-detection by indexing; CycIF, cyclic immunofluorescence; CyTOF, cytometry time of flight; DESI, desorption electrospray ionization; DNA, deoxyribonucleic acid; FDA, food and drug administration; IHC, immunohistochemistry; IF, immunofluorescence; immuno-SABER, immunostaining with signal amplification by exchange reaction; IMS, imaging mass spectrometry; LC-MS, liquid chromatography–mass spectrometry; LCM, laser capture microdissection; MALDI, matrix-assisted laser desorption/ionization; merfish, multiplexed error-robust fluorescence in situ hybridization; MIBI, multiplex ion beam imaging; microLESA, micro liquid extraction surface analysis; MRI, magnetic resonance imaging; mRNA, messenger ribonucleic acid; MS, mass spectrometry; NFT, neurofibrillary tangle; pciSeq, probabilistic cell typing by in Situ sequencing; PET, positron emission tomography; PTM, post translational modification; RNA, ribonucleic acid; scRNA-seq, single-cell ribonucleic acid sequencing; SIMS, secondary ion mass spectrometry; SiT, spatial isoform transcriptomics; snRNA-seq, single-nucleus ribonucleic acid sequencing; STRING, search tool for retrieval of interacting genes/proteins; timsTOF, trapped ion mobility spectrometry time-of-flight.
Attems, J., and Jellinger, K. A. (2014). The overlap between vascular disease and Alzheimer’s disease - lessons from pathology. BMC Med. 12:206. doi: 10.1186/s12916-014-0206-2
Badhwar, A., McFall, G., Sapkota, S., Black, S., Chertkow, H., Duchesne, S., et al. (2020). A multiomics approach to heterogeneity in Alzheimer’s disease: focused review and roadmap. Brain 143, 1315–1331. doi: 10.1093/brain/awz384
Bai, B., Vanderwall, D., Li, Y., Wang, X., Poudel, S., Wang, H., et al. (2021). Proteomic landscape of Alzheimer’s Disease: novel insights into pathogenesis and biomarker discovery. Mol. Neurodegener. 16:55. doi: 10.1186/s13024-021-00474-z
Bergenstråhle, L., He, B., Bergenstråhle, J., Abalo, X., Mirzazadeh, R., Thrane, K., et al. (2022). Super-resolved spatial transcriptomics by deep data fusion. Nat. Biotechnol. 40, 476–479. doi: 10.1038/s41587-021-01075-3
Biffi, A., and Greenberg, S. M. (2011). Cerebral amyloid angiopathy: A systematic review. J. Clin. Neurol. 7, 1–9.
Black, S., Phillips, D., Hickey, J., Kennedy-Darling, J., Venkataraaman, V., Samusik, N., et al. (2021). CODEX multiplexed tissue imaging with DNA-conjugated antibodies. Nat. Protoc. 16, 3802–3835. doi: 10.1038/s41596-021-00556-8
Boyle, P., Yu, L., Nag, S., Leurgans, S., Wilson, R., Bennett, D., et al. (2015). Cerebral amyloid angiopathy and cognitive outcomes in community-based older persons. Neurology 85, 1930–1936. doi: 10.1212/WNL.0000000000002175
Caprioli, R. M., Farmer, T. B., and Gile, J. (1997). Molecular imaging of biological samples: localization of peptides and proteins using MALDI-TOF MS. Anal. Chem. 69, 4751–4760. doi: 10.1021/ac970888i
Carare, R., Teeling, J., Hawkes, C., Püntener, U., Weller, R., Nicoll, J., et al. (2014). Immune complex formation impairs the elimination of solutes from the brain: implications for immunotherapy in Alzheimer’s disease. Acta Neuropathol. Commun. 1:48. doi: 10.1186/2051-5960-1-48
Casadonte, R., Kriegsmann, M., Deininger, S., Amann, K., Paape, R., Belau, E., et al. (2015). Imaging mass spectrometry analysis of renal amyloidosis biopsies reveals protein co-localization with amyloid deposits. Anal. Bioanal. Chem. 407, 5323–5331. doi: 10.1007/s00216-015-8689-z
Casadonte, R., Kriegsmann, M., Zweynert, F., Friedrich, K., Baretton, G., Otto, M., et al. (2014). Imaging mass spectrometry to discriminate breast from pancreatic cancer metastasis in formalin-fixed paraffin-embedded tissues. Proteomics 14, 956–964. doi: 10.1002/pmic.201300430
Chen, K., Boettiger, A., Moffitt, J., Wang, S., and Zhuang, X. (2015). RNA imaging. Spatially resolved, highly multiplexed RNA profiling in single cells. Science 348, aaa6090. doi: 10.1126/science.aaa6090
Chen, W., Lu, A., Craessaerts, K., Pavie, B., Sala Frigerio, C., Corthout, N., et al. (2020). Spatial transcriptomics and in situ sequencing to study Alzheimer’s Disease. Cell 182, 976–991.e. doi: 10.1016/j.cell.2020.06.038
Chew, H., Solomon, V., and Fonteh, A. (2020). Involvement of lipids in Alzheimer’s Disease pathology and potential therapies. Front. Physiol. 11:598. doi: 10.3389/fphys.2020.00598
Clark, C., Dayon, L., Masoodi, M., Bowman, G., and Popp, J. (2021). An integrative multi-omics approach reveals new central nervous system pathway alterations in Alzheimer’s disease. Alzheimers Res. Ther. 13:71. doi: 10.1186/s13195-021-00814-7
Clark, C., Rabl, M., Dayon, L., and Popp, J. (2022). The promise of multi-omics approaches to discover biological alterations with clinical relevance in Alzheimer’s disease. Front. Aging Neurosci. 14:1065904. doi: 10.3389/fnagi.2022.1065904
Datta, S., Malhotra, L., Dickerson, R., Chaffee, S., Sen, C., and Roy, S. (2015). Laser capture microdissection: Big data from small samples. Histol. Histopathol. 30, 1255–1269. doi: 10.14670/HH-11-622
Debette, S., and Markus, H. S. (2010). The clinical importance of white matter hyperintensities on brain magnetic resonance imaging: Systematic review and meta-analysis. BMJ 341:288. doi: 10.1136/bmj.c3666
Deture, M. A., and Dickson, D. W. (2019). The neuropathological diagnosis of Alzheimer’s disease. Mol. Neurodegener. 14:32. doi: 10.1186/s13024-019-0333-5
Djambazova, K., Dufresne, M., Migas, L., Kruse, A., Van de Plas, R., Caprioli, R., et al. (2023). MALDI TIMS IMS of Disialoganglioside Isomers-GD1a and GD1b in Murine Brain Tissue. Anal. Chem. 95, 1176–1183. doi: 10.1021/acs.analchem.2c03939
Djambazova, K., Klein, D., Migas, L., Neumann, E., Rivera, E., Van de Plas, R., et al. (2020). Resolving the Complexity of Spatial Lipidomics Using MALDI TIMS Imaging Mass Spectrometry. Anal. Chem. 92, 13290–13297. doi: 10.1021/acs.analchem.0c02520
Drummond, E., Nayak, S., Pires, G., Ueberheide, B., and Wisniewski, T. (2018). “Isolation of Amyloid Plaques and Neurofibrillary Tangles from Archived Alzheimer’s Disease Tissue Using Laser-Capture Microdissection for Downstream Proteomics,” in Laser Capture Microdissection: Methods and Protocols, ed. G. I. Murray (New York, NY: Springer), 319–334. doi: 10.1007/978-1-4939-7558-7_18
Eberlin, L. S., Ferreira, C. R., Dill, A. L., Ifa, D. R., and Cooks, R. G. (2011). Desorption electrospray ionization mass spectrometry for lipid characterization and biological tissue imaging. Biochim. Biophys. Acta 1811, 946–960. doi: 10.1016/j.bbalip.2011.05.006
François, M., Karpe, A., Liu, J., Beale, D., Hor, M., Hecker, J., et al. (2022). Multi-Omics, an integrated approach to identify novel blood biomarkers of Alzheimer’s Disease. Metabolites 12:949. doi: 10.3390/metabo12100949
Gao, C., Shen, X., Tan, Y., and Chen, S. (2022). Pathogenesis, therapeutic strategies and biomarker development based on “omics” analysis related to microglia in Alzheimer’s disease. J. Neuroinflammation 19:215. doi: 10.1186/s12974-022-02580-1
Giannakopoulos, P., Herrmann, F., Bussière, T., Bouras, C., Kövari, E., Perl, D., et al. (2003). Tangle and neuron numbers, but not amyloid load, predict cognitive status in Alzheimer’s disease. Neurology 60, 1495–1500. doi: 10.1212/01.wnl.0000063311.58879.01
Gode, D., and Volmer, D. A. (2013). Lipid imaging by mass spectrometry-a review. Analyst 138, 1289–1315. doi: 10.1039/c2an36337b
Goltsev, Y., Samusik, N., Kennedy-Darling, J., Bhate, S., Hale, M., Vazquez, G., et al. (2018). Deep Profiling of Mouse Splenic Architecture with CODEX Multiplexed Imaging. Cell 174, 968–981.e. doi: 10.1016/j.cell.2018.07.010
Gry, M., Rimini, R., Strömberg, S., Asplund, A., Pontén, F., Uhlén, M., et al. (2009). Correlations between RNA and protein expression profiles in 23 human cell lines. BMC Genomics 10:365. doi: 10.1186/1471-2164-10-365
Guiberson, E., Weiss, A., Ryan, D., Monteith, A., Sharman, K., Gutierrez, D., et al. (2021). Spatially targeted proteomics of the host-pathogen interface during staphylococcal abscess formation. ACS Infect. Dis. 7, 101–113. doi: 10.1021/acsinfecdis.0c00647
Hanselmann, M., Röder, J., Köthe, U., Renard, B., Heeren, R., and Hamprecht, F. (2013). Active learning for convenient annotation and classification of secondary ion mass spectrometry images. Anal. Chem. 85, 147–155. doi: 10.1021/ac3023313
Heeren, R. M. A., McDonnell, L. A., Amstalden, E., Luxembourg, S. L., Altelaar, A. F. M., and Piersma, S. R. (2006). Why don’t biologists use SIMS?. A critical evaluation of imaging MS. Appl. Surf. Sci. 252, 6827–6835. doi: 10.1016/j.apsusc.2006.02.134
Hickey, J., Neumann, E., Radtke, A., Camarillo, J., Beuschel, R., Albanese, A., et al. (2022). Spatial mapping of protein composition and tissue organization: a primer for multiplexed antibody-based imaging. Nat. Methods 19, 284–295. doi: 10.1038/s41592-021-01316-y
Hughes, C., Foehr, S., Garfield, D., Furlong, E., Steinmetz, L., and Krijgsveld, J. (2014). Ultrasensitive proteome analysis using paramagnetic bead technology. Mol. Syst. Biol. 10:757. doi: 10.15252/msb.20145625
Ifa, D., Wu, C., Ouyang, Z., and Cooks, R. (2010). Desorption electrospray ionization and other ambient ionization methods: current progress and preview. Analyst 135, 669–681. doi: 10.1039/b925257f
Johnson, E., Dammer, E., Duong, D., Ping, L., Zhou, M., Yin, L., et al. (2020). Large-scale proteomic analysis of Alzheimer’s disease brain and cerebrospinal fluid reveals early changes in energy metabolism associated with microglia and astrocyte activation. Nat. Med. 26, 769–780. doi: 10.1038/s41591-020-0815-6
Kaya, I., Zetterberg, H., Blennow, K., and Hanrieder, J. (2018). Shedding light on the molecular pathology of amyloid plaques in transgenic Alzheimer’s Disease mice using multimodal MALDI imaging mass spectrometry. ACS Chem. Neurosci. 9, 1802–1817. doi: 10.1021/acschemneuro.8b00121
Kelley, A., Colley, M., Dyer, S., Bach, S., Zhu, X., and Perry, G. (2020). Ethanol-Fixed, Paraffin-Embedded Tissue Imaging: Implications for Alzheimer’s Disease Research. J. Am. Soc. Mass Spectrom 31, 2416–2420. doi: 10.1021/jasms.0c00195
Knittelfelder, O., Traikov, S., Vvedenskaya, O., Schuhmann, A., Segeletz, S., Shevchenko, A., et al. (2018). Shotgun lipidomics combined with laser capture microdissection: A tool to analyze histological zones in cryosections of tissues. Anal. Chem. 90, 9868–9878. doi: 10.1021/acs.analchem.8b02004
Kodam, P., Sai Swaroop, R., Pradhan, S., Sivaramakrishnan, V., and Vadrevu, R. (2023). Integrated multi-omics analysis of Alzheimer’s disease shows molecular signatures associated with disease progression and potential therapeutic targets. Sci. Rep. 13:3695. doi: 10.1038/s41598-023-30892-6
Koutarapu, S., Ge, J., Jha, D., Blennow, K., Zetterberg, H., Lashley, T., et al. (2022). Correlative chemical imaging identifies amyloid peptide signatures of neuritic plaques and dystrophy in human sporadic Alzheimer’s Disease. Brain Connect. [Epub ahead of print]. doi: 10.1089/brain.2022.0047
Kulak, N., Pichler, G., Paron, I., Nagaraj, N., and Mann, M. (2014). Minimal, encapsulated proteomic-sample processing applied to copy-number estimation in eukaryotic cells. Nat. Methods 11, 319–324. doi: 10.1038/nmeth.2834
Lazar, A., Bich, C., Panchal, M., Desbenoit, N., Petit, V., Touboul, D., et al. (2013). Time-of-flight secondary ion mass spectrometry (TOF-SIMS) imaging reveals cholesterol overload in the cerebral cortex of Alzheimer disease patients. Acta Neuropathol. 125, 133–144. doi: 10.1007/s00401-012-1041-1
Lazova, R., Seeley, E., Keenan, M., Gueorguieva, R., and Caprioli, R. (2012). Imaging mass spectrometry–a new and promising method to differentiate Spitz nevi from Spitzoid malignant melanomas. Am. J. Dermatopathol. 34, 82–90. doi: 10.1097/DAD.0b013e31823df1e2
Lebrigand, K., Bergenstråhle, J., Thrane, K., Mollbrink, A., Meletis, K., Barbry, P., et al. (2023). The spatial landscape of gene expression isoforms in tissue sections. Nucleic Acids Res. 51:e47. doi: 10.1093/nar/gkad169
Lee, S., Zimmerman, M., Narkhede, A., Nasrabady, S., Tosto, G., Meier, I., et al. (2018). White matter hyperintensities and the mediating role of cerebral amyloid angiopathy in dominantly-inherited Alzheimer’s disease. PLoS One 13:e0195838. doi: 10.1371/journal.pone.0195838
Long, J. M., and Holtzman, D. M. (2019). Alzheimer Disease: An Update on Pathobiology and Treatment Strategies. Cell 179, 312–339. doi: 10.1016/j.cell.2019.09.001
Lovatt, D., Ruble, B., Lee, J., Dueck, H., Kim, T., Fisher, S., et al. (2014). Transcriptome in vivo analysis (TIVA) of spatially defined single cells in live tissue. Nat. Methods 11, 190–196. doi: 10.1038/nmeth.2804
McDonnell, L. A., Heeren, R. M. A., de Lange, R. P. J., and Fletcher, I. W. (2006). Higher Sensitivity Secondary Ion Mass Spectrometry of Biological Molecules for High Resolution, Chemically Specific Imaging. J. Am. Soc. Mass Spectrom. 17, 1195–1202. doi: 10.1016/j.jasms.2006.05.003
Meding, S., Nitsche, U., Balluff, B., Elsner, M., Rauser, S., Schöne, C., et al. (2012). Tumor classification of six common cancer types based on proteomic profiling by MALDI imaging. J. Proteome Res. 11, 1996–2003. doi: 10.1021/pr200784p
Merritt, C., Ong, G., Church, S., Barker, K., Danaher, P., Geiss, G., et al. (2020). Multiplex digital spatial profiling of proteins and RNA in fixed tissue. Nat. Biotechnol. 38, 586–599. doi: 10.1038/s41587-020-0472-9
Michno, W., Kaya, I., Nyström, S., Guerard, L., Nilsson, K., Hammarström, P., et al. (2018). Multimodal Chemical Imaging of Amyloid Plaque Polymorphism Reveals Aβ Aggregation Dependent Anionic Lipid Accumulations and Metabolism. Anal. Chem. 90, 8130–8138. doi: 10.1021/acs.analchem.8b01361
Michno, W., Stringer, K., Enzlein, T., Passarelli, M., Escrig, S., Vitanova, K., et al. (2021). Following spatial Aβ aggregation dynamics in evolving Alzheimer’s disease pathology by imaging stable isotope labeling kinetics. Sci. Adv. 7, eabg4855. doi: 10.1126/sciadv.abg4855
Michno, W., Wehrli, P., Koutarapu, S., Marsching, C., Minta, K., Ge, J., et al. (2022). Structural amyloid plaque polymorphism is associated with distinct lipid accumulations revealed by trapped ion mobility mass spectrometry imaging. J. Neurochem. 160, 482–498. doi: 10.1111/jnc.15557
Moffitt, J., Lundberg, E., and Heyn, H. (2022). The emerging landscape of spatial profiling technologies. Nat. Rev. Genet. 23, 741–759. doi: 10.1038/s41576-022-00515-3
Moggridge, S., Sorensen, P. H., Morin, G. B., and Hughes, C. S. (2018). Extending the Compatibility of the SP3 Paramagnetic Bead Processing Approach for Proteomics. J. Proteome Res. 17, 1730–1740. doi: 10.1021/acs.jproteome.7b00913
Mrdjen, D., Fox, E., Bukhari, S., Montine, K., Bendall, S., and Montine, T. (2019). The basis of cellular and regional vulnerability in Alzheimer’s disease. Acta Neuropathol. 138, 729–749. doi: 10.1007/s00401-019-02054-4
Muñoz-Castro, C., Noori, A., Magdamo, C., Li, Z., Marks, J., Frosch, M., et al. (2022). Cyclic multiplex fluorescent immunohistochemistry and machine learning reveal distinct states of astrocytes and microglia in normal aging and Alzheimer’s disease. J. Neuroinflammation 19:30. doi: 10.1186/s12974-022-02383-4
Murray, H., Johnson, K., Sedlock, A., Highet, B., Dieriks, B., Anekal, P., et al. (2022). Lamina-specific immunohistochemical signatures in the olfactory bulb of healthy, Alzheimer’s and Parkinson’s disease patients. Commun. Biol. 5:88. doi: 10.1038/s42003-022-03032-5
Nativio, R., Lan, Y., Donahue, G., Sidoli, S., Berson, A., Srinivasan, A. R., et al. (2020). An integrated multi-omics approach identifies epigenetic alterations associated with Alzheimer’s disease. Nat. Genetics 52, 1024–1035. doi: 10.1038/s41588-020-0696-0
Neumann, E., Patterson, N., Rivera, E., Allen, J., Brewer, M., deCaestecker, M., et al. (2022). Highly multiplexed immunofluorescence of the human kidney using co-detection by indexing. Kidney Int. 101, 137–143. doi: 10.1016/j.kint.2021.08.033
Nichterwitz, S., Benitez, J., Hoogstraaten, R., Deng, Q., and Hedlund, E. L. C. M. - (2018). Seq: A method for spatial transcriptomic profiling using laser capture microdissection coupled with PolyA-Based RNA Sequencing. Methods Mol. Biol. 1649, 95–110. doi: 10.1007/978-1-4939-7213-5_6
Nilsson, A., Goodwin, R., Shariatgorji, M., Vallianatou, T., Webborn, P., and Andrén, P. (2015). Mass spectrometry imaging in drug development. Anal. Chem. 87, 1437–1455. doi: 10.1021/ac504734s
Norris, J. L., and Caprioli, R. M. (2013). Analysis of tissue specimens by matrix-assisted laser desorption/ionization imaging mass spectrometry in biological and clinical research. Chem. Rev. 113, 2309–2342. doi: 10.1021/cr3004295
Qi, K., Wu, L., Liu, C., and Pan, Y. (2021). Recent advances of ambient mass spectrometry imaging and its applications in lipid and metabolite analysis. Metabolites 11:10780. doi: 10.3390/metabo11110780
Qian, X., Harris, K., Hauling, T., Nicoloutsopoulos, D., Muñoz-Manchado, A., Skene, N., et al. (2020). Probabilistic cell typing enables fine mapping of closely related cell types in situ. Nat. Methods 17, 101–106. doi: 10.1038/s41592-019-0631-4
Rajan, K. B., Weuve, J., Barnes, L. L., McAninch, E. A., Wilson, R. S., and Evans, D. A. (2021). Population estimate of people with clinical Alzheimer’s disease and mild cognitive impairment in the United States (2020–2060). Alzheimers Dement. 17, 1966–1975. doi: 10.1002/alz.12362
Rao, A., Barkley, D., França, G. S., and Yanai, I. (2021). Exploring tissue architecture using spatial transcriptomics. Nature 596, 211–220. doi: 10.1038/s41586-021-03634-9
Roach, P. J., Laskin, J., and Laskin, A. (2010). Nanospray desorption electrospray ionization: An ambient method for liquid-extraction surface sampling in mass spectrometry. Analyst 135, 2233–2236. doi: 10.1039/c0an00312c
Rodriques, S., Stickels, R., Goeva, A., Martin, C., Murray, E., Vanderburg, C., et al. (2019). Slide-seq: A scalable technology for measuring genome-wide expression at high spatial resolution. Science 363, 1463–1467. doi: 10.1126/science.aaw1219
Ryan, D., Patterson, N., Putnam, N., Wilde, A., Weiss, A., Perry, W., et al. (2019). MicroLESA: integrating autofluorescence microscopy, in situ micro-digestions, and liquid extraction surface analysis for high spatial resolution targeted proteomic studies. Anal. Chem. 91, 7578–7585. doi: 10.1021/acs.analchem.8b05889
Saka, S., Wang, Y., Kishi, J., Zhu, A., Zeng, Y., Xie, W., et al. (2019). Immuno-SABER enables highly multiplexed and amplified protein imaging in tissues. Nat. Biotechnol. 37, 1080–1090. doi: 10.1038/s41587-019-0207-y
Schrag, M., McAuley, G., Pomakian, J., Jiffry, A., Tung, S., Mueller, C., et al. (2010). Correlation of hypointensities in susceptibility-weighted images to tissue histology in dementia patients with cerebral amyloid angiopathy: a postmortem MRI study. Acta Neuropathol. 119, 291–302. doi: 10.1007/s00401-009-0615-z
Schrag, M. S., Mahoney, E. R., Shostak, A., Nackenoff, A., Dumitrescu, L., Akers, C., et al. (2020). Granulovacuolar degenerating body markers accumulate alongside dysfunctional lysosomes in dystrophic neurites and correlate with cognition in Alzheimer’s disease. Alzheimers Dementia 16:S3. doi: 10.1002/alz.047657
Spengler, B., and Hubert, M. (2002). Scanning microprobe matrix-assisted laser desorption ionization (SMALDI) mass spectrometry: Instrumentation for sub-micrometer resolved LDI and MALDI surface analysis. J. Am. Soc. Mass Spectrom. 13, 735–748. doi: 10.1016/S1044-0305(02)00376-8
Spraggins, J., Djambazova, K., Rivera, E., Migas, L., Neumann, E., Fuetterer, A., et al. (2019). High-Performance Molecular Imaging with MALDI Trapped Ion-Mobility Time-of-Flight (timsTOF) Mass Spectrometry. Anal. Chem. 91, 14552–14560. doi: 10.1021/acs.analchem.9b03612
Stickels, R., Murray, E., Kumar, P., Li, J., Marshall, J., Di Bella, D., et al. (2021). Highly sensitive spatial transcriptomics at near-cellular resolution with Slide-seqV2. Nat. Biotechnol. 39, 313–319. doi: 10.1038/s41587-020-0739-1
Taga, M., Petyuk, V., White, C., Marsh, G., Ma, Y., Klein, H., et al. (2020). BIN1 protein isoforms are differentially expressed in astrocytes, neurons, and microglia: neuronal and astrocyte BIN1 are implicated in tau pathology. Mol. Neurodegener. 15:44. doi: 10.1186/s13024-020-00387-3
Tideman, L., Migas, L., Djambazova, K., Patterson, N., Caprioli, R., Spraggins, J., et al. (2021). Automated biomarker candidate discovery in imaging mass spectrometry data through spatially localized Shapley additive explanations. Anal. Chim. Acta 1177:338522. doi: 10.1016/j.aca.2021.338522
Tsurui, H., Nishimura, H., Hattori, S., Hirose, S., Okumura, K., and Shirai, T. (2000). Seven-color fluorescence imaging of tissue samples based on Fourier spectroscopy and singular value decomposition. J. Histochem. Cytochem. 48, 653–662. doi: 10.1177/002215540004800509
Van de Plas, R., Yang, J., Spraggins, J., and Caprioli, R. (2015). Image fusion of mass spectrometry and microscopy: a multimodality paradigm for molecular tissue mapping. Nat. Methods 12, 366–372. doi: 10.1038/nmeth.3296
van Dyck, C., Swanson, C., Aisen, P., Bateman, R., Chen, C., Gee, M., et al. (2022). Lecanemab in Early Alzheimer’s Disease. N. Engl. J. Med. 388, 9–21. doi: 10.1056/NEJMoa2212948
van Etten, E. S., Auriel, E., Haley, K. E., Ayres, A. M., Vashkevich, A., Schwab, K. M., et al. (2014). Incidence of Symptomatic Hemorrhage in Patients With Lobar Microbleeds. Stroke 45, 2280–2285. doi: 10.1161/STROKEAHA.114.005151
Verbeeck, N., Caprioli, R. M., and van de Plas, R. (2020). Unsupervised machine learning for exploratory data analysis in imaging mass spectrometry. Mass Spectrom. Rev. 39, 245–291. doi: 10.1002/mas.21602
Veselkov, K., Mirnezami, R., Strittmatter, N., Goldin, R., Kinross, J., Speller, A., et al. (2014). Chemo-informatic strategy for imaging mass spectrometry-based hyperspectral profiling of lipid signatures in colorectal cancer. Proc. Natl. Acad. Sci. U. S. A. 111, 1216–1221. doi: 10.1073/pnas.1310524111
Vijayaragavan, K., Cannon, B., Tebaykin, D., Bossé, M., Baranski, A., Oliveria, J., et al. (2022). Single-cell spatial proteomic imaging for human neuropathology. Acta Neuropathol. Commun. 10:158. doi: 10.1186/s40478-022-01465-x
Walker, J., Kazempour Dehkordi, S., Fracassi, A., Vanschoiack, A., Pavenko, A., Taglialatela, G., et al. (2022). Differential protein expression in the hippocampi of resilient individuals identified by digital spatial profiling. Acta Neuropathol. Commun. 10:23. doi: 10.1186/s40478-022-01324-9
Wang, Y., Xu, B., and Xue, L. (2022). Applications of CyTOF in Brain Immune Component Studies. Engineering 16, 187–197. doi: 10.1016/j.eng.2021.06.022
Weissleder, R. (2002). Scaling down imaging: molecular mapping of cancer in mice. Nat. Rev. Cancer 2, 11–18. doi: 10.1038/nrc701
Wu, C., Dill, A., Eberlin, L., Cooks, R., and Ifa, D. (2013). Mass spectrometry imaging under ambient conditions. Mass Spectrom. Rev. 32, 218–243. doi: 10.1002/mas.21360
Wu, K. J., and Odom, R. W. (1996). Matrix-Enhanced Secondary Ion Mass Spectrometry: A Method for Molecular Analysis of Solid Surfaces. Anal. Chem. 68, 873–882. doi: 10.1021/ac950717i
Xu, K., Liang, Y., Piehowski, P., Dou, M., Schwarz, K., Zhao, R., et al. (2019). Benchtop-compatible sample processing workflow for proteome profiling of < 100 mammalian cells. Anal. Bioanal. Chem. 411, 4587–4596. doi: 10.1007/s00216-018-1493-9
Zhang, X., Wu, C., and Tan, W. (2021). Brain lipid dynamics in amyloid precursor protein/presenilin 1 mouse model of early Alzheimer’s Disease by desorption electrospray ionization and matrix assisted laser desorption ionization-mass spectrometry imaging techniques. J. Proteome Res. 20, 2643–2650. doi: 10.1021/acs.jproteome.0c01050
Keywords: Alzheimer’s disease, spatial multi-omics, mass spectrometry, neuroscience, imaging mass spectrometry (IMS), spatial transcriptomics (ST), microscopy, immunoflorescence
Citation: Marshall CR, Farrow MA, Djambazova KV and Spraggins JM (2023) Untangling Alzheimer’s disease with spatial multi-omics: a brief review. Front. Aging Neurosci. 15:1150512. doi: 10.3389/fnagi.2023.1150512
Received: 24 January 2023; Accepted: 13 June 2023;
Published: 17 July 2023.
Edited by:
Christophe Hirtz, Université de Montpellier, FranceReviewed by:
Paolo Inglese, Imperial College London, United KingdomCopyright © 2023 Marshall, Farrow, Djambazova and Spraggins. This is an open-access article distributed under the terms of the Creative Commons Attribution License (CC BY). The use, distribution or reproduction in other forums is permitted, provided the original author(s) and the copyright owner(s) are credited and that the original publication in this journal is cited, in accordance with accepted academic practice. No use, distribution or reproduction is permitted which does not comply with these terms.
*Correspondence: Jeffrey M. Spraggins, amVmZi5zcHJhZ2dpbnNAdmFuZGVyYmlsdC5lZHU=
Disclaimer: All claims expressed in this article are solely those of the authors and do not necessarily represent those of their affiliated organizations, or those of the publisher, the editors and the reviewers. Any product that may be evaluated in this article or claim that may be made by its manufacturer is not guaranteed or endorsed by the publisher.
Research integrity at Frontiers
Learn more about the work of our research integrity team to safeguard the quality of each article we publish.