- 1Department of Neurology, Division of Cognitive and Motor Aging, Albert Einstein College of Medicine, Bronx, NY, United States
- 2Department of Medicine, Division of Geriatrics, Albert Einstein College of Medicine, Bronx, NY, United States
- 3Department of Pediatrics, Albert Einstein College of Medicine, Bronx, NY, United States
- 4Gruss Magnetic Resonance Research Center, Albert Einstein College of Medicine, Bronx, NY, United States
- 5Division of Clinical Research, Nathan S. Kline Institute for Psychiatric Research, Orangeburg, NY, United States
- 6Department of Psychiatry, NYU Grossman School of Medicine, New York, NY, United States
- 7Department of Radiology, Montefiore Medical Center, Albert Einstein College of Medicine, Bronx, NY, United States
- 8The Dominick P. Purpura Department of Neuroscience, Albert Einstein College of Medicine, Bronx, NY, United States
- 9Department of Psychiatry and Behavioral Sciences, Montefiore Medical Center, Albert Einstein College of Medicine, Bronx, NY, United States
- 10Department of Radiology, Division of Nuclear Medicine, Montefiore Medical Center, Bronx, NY, United States
- 11Department of Epidemiology and Population Health, Albert Einstein College of Medicine, Bronx, NY, United States
- 12Center of Aging Brain, Montefiore Medical Center, Yonkers, NY, United States
Identification of novel, non-invasive, non-cognitive based markers of Alzheimer’s disease (AD) and related dementias are a global priority. Growing evidence suggests that Alzheimer’s pathology manifests in sensory association areas well before appearing in neural regions involved in higher-order cognitive functions, such as memory. Previous investigations have not comprehensively examined the interplay of sensory, cognitive, and motor dysfunction with relation to AD progression. The ability to successfully integrate multisensory information across multiple sensory modalities is a vital aspect of everyday functioning and mobility. Our research suggests that multisensory integration, specifically visual-somatosensory integration (VSI), could be used as a novel marker for preclinical AD given previously reported associations with important motor (balance, gait, and falls) and cognitive (attention) outcomes in aging. While the adverse effect of dementia and cognitive impairment on the relationship between multisensory functioning and motor outcomes has been highlighted, the underlying functional and neuroanatomical networks are still unknown. In what follows we detail the protocol for our study, named The VSI Study, which is strategically designed to determine whether preclinical AD is associated with neural disruptions in subcortical and cortical areas that concurrently modulate multisensory, cognitive, and motor functions resulting in mobility decline. In this longitudinal observational study, a total of 208 community-dwelling older adults with and without preclinical AD will be recruited and monitored yearly. Our experimental design affords assessment of multisensory integration as a new behavioral marker for preclinical AD; identification of functional neural networks involved in the intersection of sensory, motor, and cognitive functioning; and determination of the impact of early AD on future mobility declines, including incident falls. Results of The VSI Study will guide future development of innovative multisensory-based interventions aimed at preventing disability and optimizing independence in pathological aging.
Introduction
Alzheimer’s disease (AD) affects over 6 million Americans and is the most-common cause of dementia (Alzheimer’s Association, 2022). AD follows a prolonged, progressive disease course that begins with pathophysiological changes affecting individuals’ brains years before any clinical manifestations are observed (Jack et al., 2013). The notion that Alzheimer’s modifies sensory processing is in its very early stages (Albers et al., 2015). Yet, this supposition is supported by evidence demonstrating that amyloid-beta (Aβ) protein accumulates in sensory-association areas of the brain well before higher-order cognitive areas like the prefrontal cortex (PFC; Thal et al., 2002). While it is well known that mobility impairments are common in mild cognitive impairment and AD (Beauchet et al., 2008; Verghese et al., 2008a), the National Institute on Aging (NIA) has recognized that functional changes in sensory and motor systems also modulate the progression of AD. Thus, the NIA is supportive of new initiatives aimed at discovering novel, non-cognitive and non-invasive biomarkers for early detection of Alzheimer’s disease, and this is directly in line with the research priorities of our division.
There is a well-established association of higher-order cognitive processes including attention and executive functioning with balance (Woollacott and Shumway-Cook, 2002; Zettel-Watson et al., 2015), gait (Verghese et al., 2007b, 2008a; Holtzer et al., 2012; Groeger et al., 2022) and falls (Hausdorff and Yogev, 2006; Holtzer et al., 2007) in healthy, as well as cognitively impaired older adults. In fact, the PFC has been found to play a critical role in successful gait and cognition (Beauchet et al., 2016). Work from our division has linked gait to discrete brain structures such as cerebellar, precuneus, supplementary motor, insular, and PFC (Blumen et al., 2019). Additionally, we have found: (1) associations between walking performance and functional connectivity in sensory-motor and fronto-parietal resting-state networks (Yuan et al., 2015); (2) links between gray matter volume in areas involved in multisensory integration (including superior temporal sulcus and superior temporal gyrus) with aspects of gait and gait control (Tripathi et al., 2022); and (3) significant associations between gait and visual somatosensory integration (VSI) processes (Mahoney and Verghese, 2018, 2020). However, the interplay of multisensory, cognitive, and motor processes and the underlying functional neural networks involved remain largely undefined in healthy and pathological aging.
Sensory inputs emanating from a device like a cell phone (that simultaneously lights up, vibrates, and plays a ringtone) combine in the brain to yield faster responses than responses to individual unisensory components, thereby decreasing the time it takes to answer the phone. The magnitude of multisensory integration can be quantified using established probabilistic modeling procedures of behavioral performance, such as reaction time (RT) and accuracy (Mahoney and Verghese, 2019). Magnitude of multisensory integration is operationalized as the area-under-the-curve of the difference between actual and predicted cumulative probability distribution functions during a pre-identified portion of the difference waveform. For example, Figure 1 depicts cumulative probability difference values (y-axis) between actual and predicted distribution functions from our latest study for percentile binned RT responses ranging from 0.0 to 1.0 in 5% increments (Mahoney and Verghese, 2020).
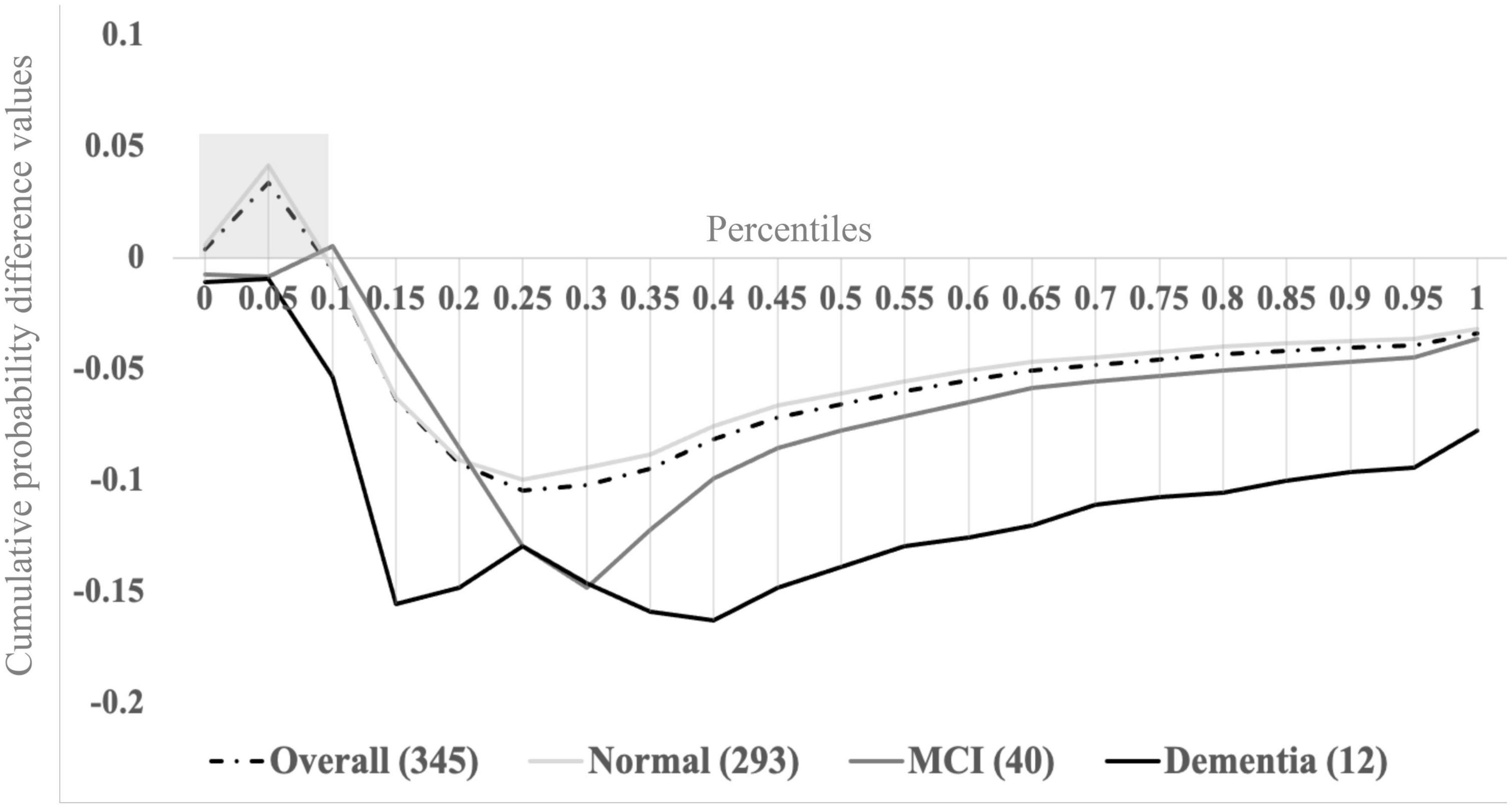
Figure 1. Visual-somatosensory integration (VSI) cumulative probability difference waves overall and by cognitive status (normal, mild cognitive impairment, or dementia). Adapted from Mahoney and Verghese (2022). Reprinted by permission of Oxford University Press on behalf of The Gerontological Society of America.
The combined study cohort (n = 345; dashed trace) reveals successful multisensory integration processes (i.e., positive cumulative probability difference values) during the fastest tenth (0.0–0.1) of RTs. Here, the area under the curve during the 0.0–0.1 percentiles (gray shaded box) is operationalized as the magnitude of multisensory integration (a continuous measure). Higher values indicate superior ability to integrate visual-somatosensory information (i.e., benefit from multisensory inputs), whereas lower and negative values indicate inability to integrate or to benefit from multisensory inputs. Stratifying the overall group based on cognitive status assigned during consensus case conference procedures [normal cognition (n = 293) – solid light gray trace; mild cognitive impairment (MCI; n = 40) – solid dark gray trace; and dementia (n = 12) – solid black trace] revealed that magnitude of multisensory integration is significantly reduced for individuals with MCI or dementia. Further, cognitive status significantly mediated the relationship between magnitude of multisensory integration and measures of mobility, such that older adults with cognitive impairments demonstrated impaired multisensory integration and significantly slower gait, as well as poorer balance compared to older adults without cognitive impairments (Mahoney and Verghese, 2020). Our findings further revealed that VSI is also correlated with attention-based performance measures (Mahoney et al., 2012; Mahoney and Verghese, 2020) that may target PFC regions known to be compromised in AD. Consequently, we argue that multisensory integration has potential utility in early AD detection, though further work is needed to uncover the exact structural and functional neural correlates of VSI.
Significance
Balance requires efficient interactions between musculoskeletal and sensory systems (Shumway-Cook and Woollacott, 2012), which are compromised in aging (Lord et al., 2007). Poor balance is a major predictor of falls, a leading cause of injury and death in older Americans. Our research reveals that better magnitude of VS integration, is associated with better balance and gait, as well as decreased risk of falls (Mahoney et al., 2019). Our previous investigations, however, did not determine the association of impaired VSI with early dementia stages, nor its contribution to mobility decline.
Impairments in cognition could adversely affect the association between magnitude of multisensory integration and mobility measures because: (1) multisensory processing appears to be regulated by PFC (Jones and Powell, 1970; Cao et al., 2019); (2) selective attention modulates multisensory integration in aging (Hugenschmidt et al., 2009; Mozolic et al., 2012); and (3) disruptions in executive attention and cognition in aging compromise multisensory integration and mobility processes (Yogev-Seligmann et al., 2008; Holtzer et al., 2012; Mahoney and Verghese, 2020). Although our preliminary findings are encouraging and of high public health significance, we believe that we are only scratching the surface for a much-needed larger multisensory investigation. The proposed study, from here on referred to as The VSI Study, is significant as it will identify the functional neural correlates of VSI, while also determining whether Alzheimer pathology concurrently impacts sensory integration and motor processes. The goal of The VSI Study is to determine the combined influence of multisensory, cognitive and motor changes in early Alzheimer’s disease in an effort to shape the development of future innovative multisensory-based interventions, prognostic tools, and new research-driven therapies aimed at preventing disability and optimizing independence in pathological aging.
Specific aims
The VSI Study seeks to achieve three main specific aims denoted as stars in Figure 2. In this conceptual model, cognitive, motor, and (multi) sensory functioning are depicted as individual gears that must work together to transmit a behavioral response. However, the impact of preclinical AD on each of the individual gears, as well as on the overall system (requiring successful interactions across all functions) requires systematic examination. Thus, our three main study aims are as follows:
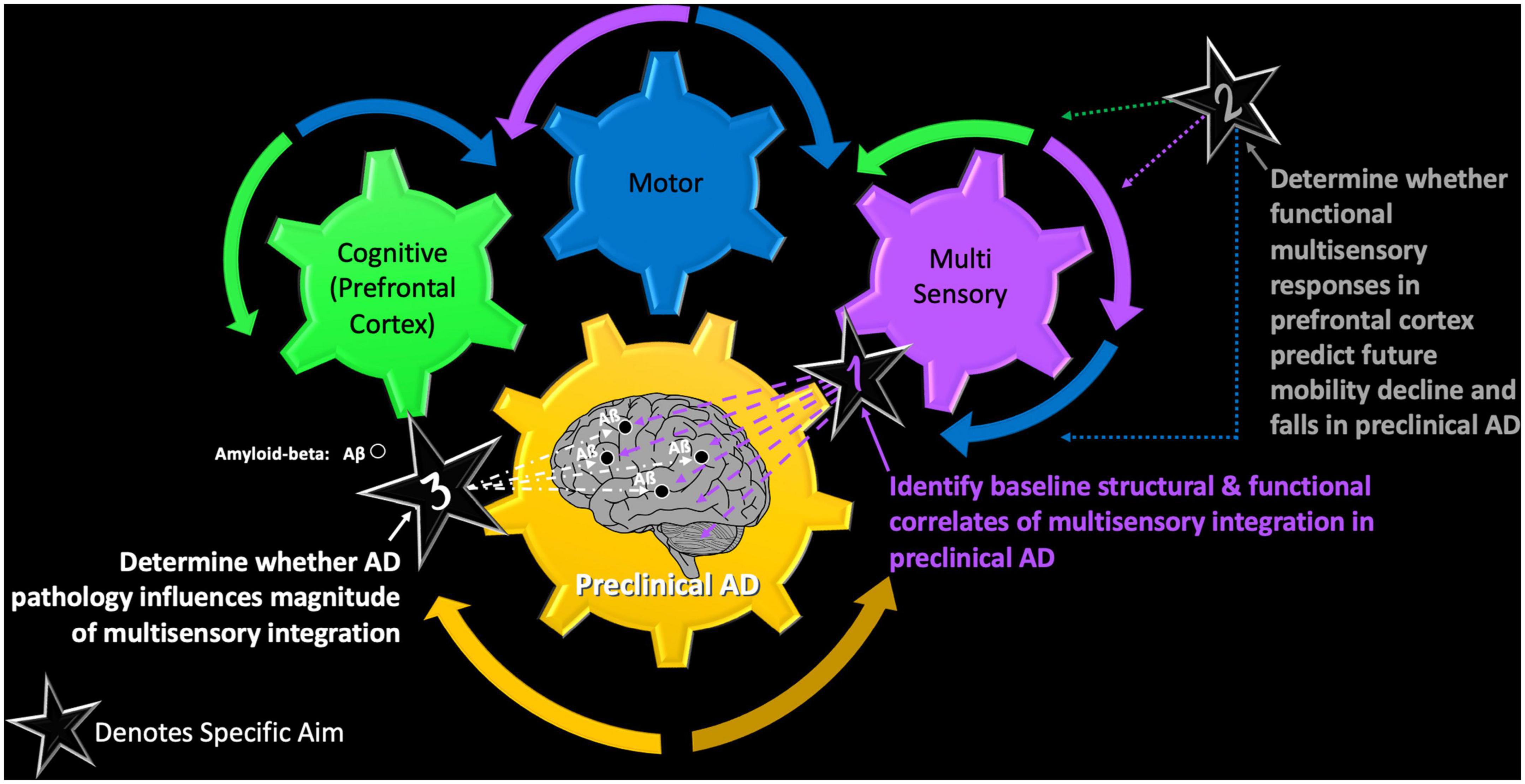
Figure 2. The VSI Study – a conceptual model of the main objectives of the VSI Study and how each afford examination of the intersection of cognitive, motor, and multisensory functioning in healthy and pathological aging.
Identify baseline structural and functional neural correlates of VSI in preclinical AD
Results from The VSI Study employing multimodal neuroimaging procedures will provide a deeper understanding of the structural and functional neural correlates of VSI in older adults with normal and preclinical AD. Here, preclinical AD will be defined as manifesting impaired cognitive performance [performance worse than 1.5 standard deviations from the mean on standardized neuropsychological tests] and presence of elevated Aß in plasma at baseline using established cut scores (Bateman et al., 2019). We hypothesize that the magnitude of VSI will be correlated with gray matter volume, cortical thickness, and blood-oxygen-level-dependent (BOLD) signal activation in subcortical and cortical regions of interest including dorsal lateral prefrontal cortex (DLPFC), rostral middle frontal, and superior frontal gyrus at study baseline (Year 1). We predict that older adults with preclinical AD will manifest reduced magnitude of VSI (worse), decreased cortical volumetrics, decreased functional connectivity, and lower BOLD responses when compared to older adults with normal cognition.
Determine whether VSI task-related BOLD activation in prefrontal cortex predicts future mobility decline and falls
Individuals with Alzheimer’s disease are at greater risks for falls and mobility disability, but specific causes of AD and the temporal onset of functional changes across systems are currently not known. We have shown a mediating effect of dementia and mild cognitive impairment on the relationship between VSI and motor outcomes (Mahoney and Verghese, 2018; Mahoney et al., 2019). These results suggested that individuals with cognitive impairments manifested poor VSI and poor balance/slow gait. Using an fMRI task where participants are asked to respond as quickly as possible to unisensory visual, unisensory somatosensory and combined visual-somatosensory stimuli in a 3-Tesla (3T) magnet, our second specific aim will determine whether visual-somatosensory task-related BOLD activation in the prefrontal cortex at baseline predicts future mobility (gait) declines and risk of incident falls. We hypothesize that preclinical AD causes disruptions in subcortical and cortical (multisensory, motor, and cognitive) regions that modulate multisensory, motor, and cognitive functions necessary for efficient mobility.
Assess the validity of VSI as a novel Alzheimer’s behavioral marker
The validity of VSI as a novel marker for AD will be established by correlating the magnitude of VSI with presence of Aβ using plasma-based measures at baseline. In Year 2, positron emission tomography (PET) measures affording localization of Aβ deposits (Piramal Imaging) to estimate Aβ neuritic plaque density will also be examined in relation to magnitude of VSI. Aβ protein deposition has been documented in both sensory and cognitive areas (Thal et al., 2002; Jack et al., 2018, 2019; Bateman et al., 2019). Therefore, we hypothesize that increased Aβ accumulation in sensory and cognitive areas, areas related to increased AD pathology, will be associated with decreased magnitude of VSI.
In keeping with the NIA-AA research framework (Jack et al., 2018), our innovative and timely project will distinguish AD symptomology (presence of mild cognitive impairment) from AD pathology (Aβ accumulation), while also applying the AT (N) classification system [Aβ (A), tau (T), and neurodegeneration (N)] to attain more direct assessment of neuropathologic changes. More specifically, and in keeping with the goals of establishing whether magnitude of VSI is a novel and early biomarker of AD, associations of VSI with plasma-based total and phosphorylated Tau, neurofilament (NfL), ApoE, and multimodal neuroimaging measures of Neurodegeneration will also be examined for study completeness.
Innovation
Multisensory integration is not well-understood in aging and its relation to cognitive and motor functioning is recognized as a major knowledge gap in the field (Meyer and Noppeney, 2011; Wallace, 2012; Mahoney and Barnett-Cowan, 2019; Campos et al., 2022). The NIA recognizes that functional changes in sensory and motor (i.e., non-cognitive) systems have an impact on the development and progression of AD and requests identification of novel, non-cognitive non-invasive predictors to aid in early AD detection. Our multisensory integration research meets this request, while also addressing the knowledge gap and providing significant public health implications. We are recognized as the first group to have established the clinical utility of magnitude of VSI in aging by linking it to poor motor outcomes including loss of balance, falls, and gait decline.
Additional innovation highlights of the VSI Study include: (1) access to established research infrastructure and existing collaborations; (2) cost and time-efficient design affording access to a priori identified participants with and without preclinical AD; (3) longitudinal design affording comprehensive examination of systemic changes (and their interactions) over time on the progression of AD and its subsequent link to mobility declines; (4) novel project with comprehensive multimodal neuroimaging approach providing clear clinical application of results; and (5) identification of a novel, non-cognitive behavioral marker that simultaneously taps multiple integrative systems that have not been systematically examined in previous AD investigations.
The current study also provides innovation beyond its specific aims as it affords: (1) a deeper investigation of the onset of functional systemic changes over time; (2) comprehensive investigation of the neurobiological consequences of AD and its links to medical co-morbidities in relation to VSI processes given previously reported diminished multisensory integration in older adults with diabetes (Mahoney et al., 2021); (3) enhancement of multisensory digital health tools like CatchU® used to screen and prevent falls for older adults in clinical settings (Mahoney et al., 2022); and (4) development of future multisensory-based interventions that will further enhance quality of life for seniors.
Methods
Study design
We propose a longitudinal study of older adults with (n = 104) and without (n = 104) preclinical AD; participants meeting criteria for dementia or AD will be excluded. In accordance with the NIA-AA research framework (Jack et al., 2018) and as stated earlier, our innovative project will allow us to disentangle differences in outcomes related to Alzheimer’s symptomatology [mere presence of mild cognitive impairment syndrome at established clinical case conference (Holtzer et al., 2008)] from those related to Alzheimer’s pathology (Aβ accumulation). Based on our previous studies (Mahoney and Verghese, 2020), we expect our preclinical AD group will include older adults with varying levels of cognitive impairment, ranging from amnestic and mixed MCI to preclinical AD. Sub-groupings of MCI and mild stage AD will afford post hoc analyses aimed at examining the impact of cognitive impairment syndromes on multisensory integration processes.
Interested participants will undergo extensive neuropsychological, sensory, physical functioning (mobility), neuroimaging, and blood testing, though we recognize that participants may decline participation in some procedures. The VSI Study includes three study sessions in Year 1 with subsequent follow-up calls every 2 months (to monitor falls) and yearly in-house visits in study Years 2 and 3. Initial enrollment of all 208 participants will be staggered across study Years 1–3, with follow-up visits conducted during study Years 2–5. Baseline sessions, designed using established divisional research studies as a model, aim to minimize fatigue and maximize effort by spreading test procedures out over three study sessions, each lasting about 3–4 h in duration (see Figure 3 for overview of study procedures by session). Based on our experience with previous and currently NIH-funded divisional studies that have similar protocols, we estimate a 90% completion rate for this protocol.
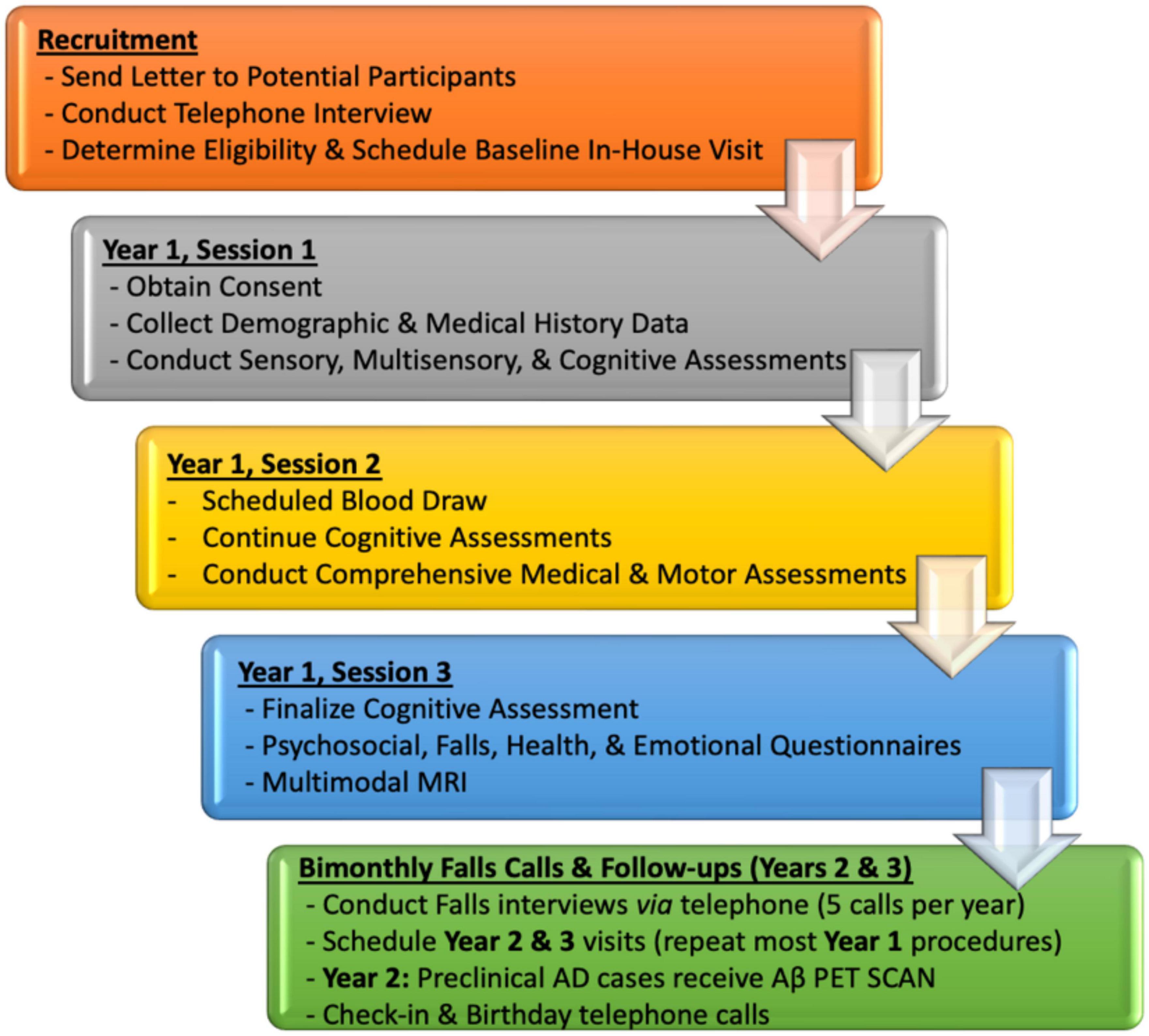
Figure 3. VSI Study flow by Year with high-level overview of targeted domains by baseline (Year 1) and follow-up sessions.
Recruitment and study criteria
Participant recruitment for this project will be strategic. We will utilize existing infrastructure, recruitment methods, and available registration lists over 600 eligible and interested participants from previously funded divisional studies (R01AG036921, R01AG044007, and 1R01AG050448; and K01AG049813) for enrollment in the VSI Study. Adults aged 65 and older living in the NY metropolitan area may also be contacted using a commercially available third-party list. We have used these and other lists to recruit over 1,000 participants for various aging studies over the past 12 years. Identification of older adults with preclinical Alzheimer’s disease will be supplemented by clinical recommendations from neurologists and neuropsychologists (Drs. Verghese, Weiss, and Zwerling), as well as clinical patient lists from Montefiore’s Center of Excellence for Alzheimer’s disease (CEAD), including both the Center for the Aging Brain (CAB) and the Memory Disorders Center. Since the VSI Study builds on existing research infrastructure, we will ensure similar distributions of age, gender, and ethnicity for older adults with and without preclinical Alzheimer’s disease by monitoring demographic and clinical parameters and adjusting as needed as we accrue our sample.
In terms of our recruitment procedures, we will first mail letters to participants explaining the VSI Study. Then, the research team will follow-up by telephone and inquire whether the letter was received. If the participant received the letter, the research assistant will conduct standardized telephone recruitment interview procedures. If a letter was not received, the participant’s name and mailing address will be verified by the research team and a new letter will be mailed out, which will be followed by a telephone interview call. Interested participants meeting study eligibility criteria (see Table 1 for detailed inclusion and exclusion criteria) will be scheduled to come to the Albert Einstein College of Medicine, Division of Cognitive and Motor Aging, Sensorimotor Integration in Aging Lab for all in-house study sessions. After baseline study procedures are completed, the VSI Study case consensus team will convene and provide clinical diagnoses based on neuropsychological performance, neurological exam, medical history, and Aβ plasma results. Study group assignment will be determined during Year 1 case-conferences, and monitored every study year.
Study measures
A comprehensive list of established assessment measures is delineated in Table 2 by domain and session. The variables of interest and their applications will be explained in detail below as they relate to each specific aim. Note that additional measures (i.e., pilot measures) unrelated to the study’s specific aims may be included in the protocol but are not listed here. Variables and test measures labeled in green will be used as covariates in certain statistical models, depending on the specific research aim. As noted earlier, our central hypothesis is that preclinical AD is associated with neural disruptions in subcortical and cortical areas that concurrently modulate sensory, motor, and cognitive functions, resulting in mobility decline. Therefore, our study strategically includes a wide array of test measures in each domain.
Our independent variable is magnitude of VSI derived from our established VSI test (Mahoney and Verghese, 2019), and our dependent variables include neuroimaging measures of functional integrity (BOLD signal and resting state functional connectivity), motor outcomes (balance, gait, and falls), and Alzheimer pathology (Aß presence and accumulation). Comprehensive screening measures, neuropsychological and neurological/medical history assessments will be used to ensure study appropriateness, characterize our cohort, as well as aid in determination of cognitive status and study group enrollment. Additional psychosocial, social, emotional, and personality measures are included for study completeness as they will foster future research initiatives.
Primary research outcomes and statistical plan by aim
As stated earlier, our group has linked the magnitude of VSI to important cognitive and motor outcomes (Mahoney et al., 2014; Mahoney and Verghese, 2018, 2020; Mahoney et al., 2019). Furthermore, we highlighted the adverse effect of dementia and mild cognitive impairments on these outcomes (Mahoney and Verghese, 2020). However, the functional neural substrates of VSI have not been identified in healthy or cognitively impaired adults. The justification for identifying associated functional neural networks of multisensory integration will allow us to design novel multisensory-based interventions to complement existing interventions that demonstrate some fall reduction. The VSI Study will employ a theoretical and empirical approach to determine whether VSI is indeed a novel non-cognitive, non-invasive predictor of early Alzheimer’s disease and specifically, address the following research aims:
Identify baseline structural and functional neural correlates of VSI in preclinical AD
Participants will complete a simple reaction time (RT) test employing three bilaterally presented conditions (visual, somatosensory, and multisensory visual-somatosensory) and a control (i.e., “catch”) condition where no stimulation is presented, and no response is expected. The four stimulus conditions will be randomly presented with equal frequency (15 trials per condition per block, 3 blocks, yielding a total of 180 trials). The addition of “catch” trials and variable inter-stimulus-interval (ranging from 1–3 s) impedes anticipatory effects (see Mahoney and Verghese, 2018; Mahoney and Verghese, 2019, 2020; Mahoney et al., 2019; for details). Participants will be instructed to respond to all stimuli as quickly as possible. Performance accuracy will be defined as the number of accurate stimulus detections divided by 45 trials per condition. Using our established methodology, robust probability (P) models that compare the cumulative distribution function (CDF) of combined unisensory visual (V) and unisensory somatosensory (S) reaction times with an upper limit of 1 min [P (RTV ≤ t) + P (RTS ≤ t), 1] to the CDF of multisensory visual-somatosensory (VS) reaction times [P (RTVS ≤ t)] will be implemented. For any latency t, the inequality holds when the CDF of the actual multisensory VS condition [P (RTVS ≤ t)] is less than or equal to the predicted CDF {min [P (RTV ≤ t) + P (RTS ≤ t), 1]}. When the actual CDF is greater than the predicted CDF (i.e., positive value), the model is violated, and the RT facilitation is the result of multisensory interactions that allow signals from redundant information to integrate or combine non-linearly. Predicted CDF will be subtracted from the actual CDF to form a difference curve. The area-under-the-curve of the group-level violated portion of the difference curve will serve as the continuous measure of magnitude of VSI.
All neuroimaging procedures will be conducted at the Gruss Magnetic Resonance Research (MRRC) Center at Albert Einstein College of Medicine under the direction of Dr. Lipton. The MRRC offers state-of-the-art multimodal neuroimaging on Philips whole-body Ingenia Elition 3.0 Tesla Magnetic Resonance Imaging (MRI) scanner equipped with 32-channel head coil. Multimodal MRIs will be captured at baseline (i.e., study Year 1) and processed by our neuroimaging team consisting of Drs. Blumen, Fleysher, and Hoptman. Our non-invasive multimodal MRI imaging techniques are reliable and have been used extensively in both healthy aging and dementia studies in our division/department. For the VSI Study, specific MRI outcome measures are listed by modality in Table 3. Structural MRI (sMRI; ∼5 min) will be acquired using high-resolution T1-weighted whole head structural imaging using axial 3D-MP-RAGE acquisition over a 240 mm field of view (FOV) with 1.0 mm isotropic resolution. TE = 4.6 ms, TR = 9.9 ms, α = 8°, and SENSE factor = 2.6 (left-right) x 2 (head-foot). Functional MRI (∼10 min total) will be acquired using whole brain T2* weighted images with echo planar weighted images with echo planar imaging over a 224 mm FOV on a 112 × 112 acquisition matrix, 3 mm slice thickness (no gap); TE = 30 ms, TR = 2,000 ms, flip angle = 90°, SENSE factor = 2 and 42 trans-axial slices per volume. The fMRI procedures will measure BOLD activation (outcome measure) during the VSI task. This event-related design emulates our established psychophysical protocol where 60 trials of visual alone, somatosensory alone, multisensory visual-somatosensory (VS; Mahoney and Verghese, 2019) will be presented in the scanner, but will also include the above-mentioned “catch” trials (60 trials). The visual (V) stimulus will be bilateral asterisks presented for 100 ms on a VisuaStim digital visor (Resonance Technology, Inc., Northridge, CA, USA). The somatosensory (S) stimulus will be bilateral pneumatic pulses presented for 100 ms through the Somatosensory Stimulus Generator system (4-D Neuroimaging) which is compatible in the MRI scanner. These stimuli will be presented alone and concurrently in the case of the concurrent VS stimulus. The critical contrast here will examine differences in BOLD activation between the multisensory VS condition vs. the sum of the two unisensory conditions (V + S). Resting-state (rs)-fMRI (10 min) will also be captured while participant lay still and relax (i.e., a passive no-task condition) with their eyes open. Fluid Attenuated Inversion Recovery (FLAIR; ∼5 min total) will account for white matter hyperintensities (WHI) indicative of small vessel disease. FLAIR will be acquired using whole head imaging sagittal 3D-TSE-IR acquisition over a 250 mm FOV with 1 mm isotropic resolution. TE = 338 ms, TR = 4,800 ms, TI = 1,650 ms TSE Factor = 182, compressed SENSE acceleration factor 3.5. FLAIR results will account for presence/absence of small vessel disease and will be considered as a covariate. Additional multimodal neuroimaging procedures to be included for study completeness, beyond the scope of the specific aims include: Susceptibility Weighting Imaging (SWI); Pseudo-Continuous Arterial spin labeling (pc-ASL); and Neurite orientation and dispersion density imaging (NODDI) – see Table 3 for details.
In terms of our statistical approach for Aim 1, the magnitude of VSI (independent variable) will be analyzed and quantified using established probabilistic modeling procedures (Mahoney and Verghese, 2019). The dependent measures of structural and functional neural integrity include: (1) cortical thickness: (2) volumetric measures for regions of interest extracted from structural MRI; (3) Beta-weights for the multisensory contrasts for each region of interest extracted from task-based fMRI; and (4) Fisher z-transformed resting-state functional connectivities between pairs of regions extracted from resting-state fMRI.
Covariates identified in our prior studies (Holtzer et al., 2007; Verghese et al., 2007b,2008a; Mahoney and Verghese, 2018, 2019, 2020), including but not limited to age, gender, ethnicity, medical comorbidities (including cardiovascular disease), total intracranial volume, and attentional capacity will be selected to account for their influence on VSI and association with outcomes. Participants will be categorized into two groups based on preclinical AD diagnosis at baseline. All statistical approaches will be supervised by our study statistician, Dr. Wang.
SAS 9.4 (Cary, NC) will be used for the analyses. We will conduct multivariate mixed effects models for imaging outcomes for the following a priori selected regions of interest including: dorsal lateral PFC, rostral middle frontal, and superior frontal gyrus regions, superior temporal sulcus, motor cortex, thalamus, basal ganglia, hippocampal, and cerebellum (one per outcome, with group factors as necessary). These regions are selected based on preliminary findings in 100 older adults (unpublished data) which reveal significant (p < 0.05) associations between magnitude of VSI and measures of structural integrity (defined here as either volume or cortical thickness) in the following regions: parahippocampal (memory); caudal middle-frontal dorsal lateral prefrontal cortex (DLPFC: cognitive functions - especially executive & attention); superior temporal sulcus (STS; multisensory); precentral (motor), postcentral (somatosensory), and lateral occipital (visual). Preliminary fMRI findings in 56 healthy older adults (ages 65–92; unpublished data) further supports inclusion of these regions given significant associations between VSI magnitude and blood-oxygen-level-dependent (BOLD) responses in known multisensory (middle temporal), motor (basal ganglia), and cognitive areas (PFC including DLPFC). The outcomes in these models include measures of neural integrity, structural (volume and thickness), and/or functional (BOLD) activation isolated by region, and the predictor of interest is the magnitude of VSI. Additional models will further include cognitive status (normal or preclinical AD) and its interaction with magnitude of VSI. The effect of cognitive status (normal or preclinical AD) on magnitude of VSI will be evaluated using similar mixed effects models. The hypothesis-driven analyses will be limited to BOLD activation in the aforementioned regions of interest. Models will be run unadjusted and then adjusted for confounders.
Determine whether VSI task-related BOLD activation in prefrontal cortex predicts future mobility decline and falls in preclinical AD
Here, we propose that reduced VSI activation in specific regions of PFC (fMRI BOLD responses), will be associated with worse balance (unipedal stance), slower gait (worse Pace scores), and increased risk of incident falls. Additionally, we propose that preclinical AD will reduce VSI activation in PFC regions of interest, which will in turn adversely affect mobility measures. Our longitudinal design will allow us to identify the impact of functional changes in (multi)sensory, motor, and cognitive processes (and their interactions) on the progression of AD that result in mobility decline.
The VSI task and multimodal neuroimaging procedures for this aim have been described above in Aim 1. For Aim 2, the VSI task will be run in the magnet to obtain fMRI task-related BOLD responses with concurrent psychophysical data. The following aim-related mobility procedures [established tests that have been validated and utilized in our center for over two decades (Verghese et al., 2002a,2007b,2008a,2009, 2012; Holtzer et al., 2007, 2012, 2014; Verghese and Xue, 2010, 2011; Ayers and Verghese, 2014; Mahoney et al., 2014, 2017, Holtzer et al., 2014; Mahoney and Verghese, 2018, 2020)] will be included in Aim 2:
Balance (∼2 min) will be assessed using unipedal stance time, which requires individuals to balance their body weight with foot on the ground for a maximum of 30 s (Hurvitz et al., 2000, 2001). Unipedal stance time is a widely used clinical test that is listed under NIH’s toolbox. Poor scores on this test have been associated with presence of neuropathy (Hurvitz et al., 2001), and predict falls in older adults (Hurvitz et al., 2000). This test will be administered twice during each study visit and maximum unipedal stance time (sec) will serve as the outcome measure.
Quantitative Gait (∼5 min) will be assessed on a 28-foot instrumented walkway (PKMAS system; Zenometrics LLC) with embedded pressure sensors that provides spatial and temporal gait parameters including: gait velocity, stride length, percentage of double support, stride time, stance time, cadence, stride length variability, and swing time variability. Participants will be assessed twice while walking on the mat at their everyday pace. Gait velocity, as well as the Pace Factor score comprised of gait velocity, stride length and percentage of double support, will serve as dependent measures.
History of falls (∼5 min) in the past 1 year, number of incident falls over a 3 year longitudinal study-period, and fall information such as type, injury and location will be tracked at yearly in-house interviews and during bimonthly telephone interviews using established criteria and standardized questionnaires (Tinetti et al., 1994). Falls are defined as sudden, unintentional, unprovoked changes in body posture, not due to a major intrinsic event (stroke) or overwhelming hazard. Dichotomous ratings of fall-history over the past 1 year (0, 1), presence of incident fall over study period (0, 1) and time to fall/censor will serve as outcome measures.
The association of functional VSI activation in specific PFC regions of interest with mobility measures of balance and quantitative gait will be examined cross-sectionally at baseline (Year 1), using linear regression models. Linear mixed effects models (LMEM) will be used to examine the association of baseline functional VSI activation in the PFC on the changes in the longitudinal balance and quantitative gait performance. The predictors will be examined as continuous variables to facilitate clinical translation of results. Adjustments for multiple comparisons will be made. Additional LMEMs will be employed to examine interplay and time course of multisensory, cognitive, and motor functioning. Cox proportional hazard model will be used to evaluate the association of magnitude of VSI with the risk of incident falls (Mahoney et al., 2019) and hazard ratios (HR) with 95% confidence intervals (CI) will be reported. Time to fall will be recorded as number of days from baseline study date to the interview date when the fall was recorded. If the participant does not report a fall, the follow-up time will be defined as the number of days from the baseline in-house visit to the last date of contact. Repeated incident falls will be examined using Andersen-Gil extension of Cox model (Anderson and Gill, 1982) and Poisson models. Robust sandwich covariance estimates account for correlations among multiple events within the same participant. Cox models will be adjusted for potential confounders. Proportional hazards assumptions of all models will be tested graphically and analytically. We will also apply mediation analysis using product of coefficients methods to evaluate whether cognitive status (normal vs. preclinical AD; independent variable) causes variation in PFC-related VSI activation (mediator), which in turn causes variation in specific mobility measures (dependent variables) using separate mediation models (balance and pace). Mediation analyses will be run using IBM’s Statistical Package for the Social Sciences (SPSS-28) and Hayes’ PROCESS package (Hayes, 2018). Confidence intervals that do not include 0 for the mediator will be defined as mediation.
Assess the validity of VSI as a novel Alzheimer’s behavioral marker
Alzheimer’s disease is associated with build-up of specific proteins (i.e., biological markers) in the brain, namely Amyloid-βeta (Aβ) in the form of plaques (Thal et al., 2002) and tau (T) in the form of neurofibrillary tangles. Common brain imaging techniques such as MRI or Computerized Tomography (CT) do not afford assessment of amyloid plaques and neurofibrillary tangles. However, molecular imaging procedures like Positron Emission Tomography (PET) imaging directly visualize these characteristic features of Alzheimer’s disease. Aβ accumulates in sensory association areas well before higher-order cognitive areas like the PFC (Thal et al., 2002). In Aim 3, we predict that AD pathology (i.e., accumulation of Aβ) will be associated with decreased magnitude of VSI in preclinical Alzheimer’s disease participants. Here, presence of Aβ will be measured in blood at baseline using plasma-based assays and established cut-scores (Bateman et al., 2019). Individuals that are Aβ+ on plasma-based tests at baseline, will receive amyloid PET imaging in study year 2. The combined use of conventional MRI with these techniques will also contribute to the early identification of Alzheimer’s disease.
The experimental design for this aim has been described above. Beyond the VSI task the following specific Aβ procedures will be implemented to determine its association with magnitude of VSI, and ultimately its use as a novel and early biomarker for preclinical AD.
Plasma-Based Blood Testing will be conducted during baseline visits for each participant. Aβ (40 and 42) and Apolipoprotein E (ApoE) will be assessed by C2N Diagnostics lab using novel multiplexed assays (Jack et al., 2018). PrecivityAD™ accuracy for determining amyloid positive versus negative status was 86%. Blood samples will be collected and placed in Einstein’s biorepository. Frozen samples will be subsequently shipped to C2N Diagnostics to be processed. Results from plasma-based testing conducted on bloods drawn at baseline, in conjunction with neuropsychological performance, will be critical for study group assignment, as well as disentangling AD symptomology from AD pathology.
Amyloid PET Imaging will only be conducted in study Year 2 for participants enrolled in the preclinical AD group (n = 104). All PET scans will be conducted at Montefiore Medical Center by Dr. Valdivia and her team. Positron emission tomography (PET) is expensive and involves the use of an imaging device (scanner) and a radiotracer that is injected into the patient’s bloodstream. The radiotracer used to estimate Aβ neuritic plaque density for this specific aim is called Neuraceq (florbetaben F-18) and is manufactured by Piramal Imaging. PET imaging after the radiotracer is injected, will afford quantification of the distribution of Aβ in the brain, where affected brain regions containing Aβ will be tabulated.
The association of magnitude of VSI with presence of Aβ protein levels in both plasma-based Aβ levels (continuous measure) and amyloid PET scans (dichotomous ± rating by brain region) will be examined using logistic and linear regression models, respectively, while adjusting for potential confounders.
Discussion
In summary, we propose to recruit 208 community-dwelling older adults with and without preclinical AD for a three-year longitudinal study. Our central hypothesis is that preclinical Alzheimer’s disease is associated with neural disruptions in subcortical and cortical areas that concurrently modulate sensory, cognitive, and motor functions, resulting in mobility decline. Our project seeks to address a NIH-identified high-priority research topic, where the interplay of multisensory integration with cognitive and mobility outcomes will be extensively studied in individuals with and without preclinical Alzheimer’s disease. A deeper understanding of the underlying neural correlates of VSI and their association with cognitive and motor outcomes will support the advance of novel, non-invasive, and non-cognitive AD markers, as well as foster the development of novel multisensory interventions designed to target specific neural derailments, while significantly augmenting existing interventions to prevent disability and optimize independence.
As with any study, there are potential pitfalls and limitations that should be discussed, along with strategies to mitigate any potential shortcomings. Missing data is a concern of any longitudinal study; to reduce the likelihood of missing data, we will reschedule study visits that are missed and update participant contact information annually. Though not a main objective, diffusion tensor imaging data will be collected and analyzed using FSL software to provide a measure of functional anisotropy. Further examination of multimodal neuroimaging relationships will be computed using probabilistic tractography between regions of interest (ROIs). Neuroimaging data access can enable investigations of additional cortical pathways not identified in our proposed neural circuit; our approach here, however, is to focus on theory-based predictions. We recognize that biomarkers, including the AT (N) classification system [Aβ (A), tau (T), and neurodegeneration (N)] recently developed the NIA-AA task-force, affords a more direct assessment of neuropathologic changes (Jack et al., 2019). In an effort to determine whether magnitude of VSI is a novel and early biomarker of mild stage Alzheimer’s disease, the current study will also assess plasma-based total and phosphorylated Tau, neurofilament (NfL) and (ApoE).
In line with current preventative approaches, results from The VSI Study will provide insight into the neurobiology of early AD and aid the development of novel prognostic tools and therapeutic interventions. The primary focus of this project will guide strategic design of new multisensory-based interventions for non-cognitive outcomes like falls. Although not a specific aim of the current study, development of future multisensory-based interventions in high-risk patients requires identification of the structural and functional neural networks involved in multisensory integration processes, as well as understanding of the impact of early AD on these networks and systems. Such knowledge will be essential to designing future remediation trials that target key PFC and other regions involved in multisensory integration to induce neural plasticity that will be associated with improvements in sensory, cognitive, and mobility outcomes for older adults with and without pre-existing cognitive impairments.
Ethics statement
The studies involving human participants were reviewed and approved by the Institutional Review Board at the Albert Einstein College of Medicine. All participants provided written informed consent to participate in this study.
Author contributions
JRM wrote the manuscript. All authors reviewed, revised, and approved the final manuscript before submission.
Funding
This work was supported by the National Institutes on Aging at the National Institute of Health (R01AG075679 to JRM).
Conflict of interest
JRM has a financial interest in JET Worldwide Enterprises Inc., a digital health startup spun out of research conducted at Albert Einstein College of Medicine. MH reports financial support was provided by American Foundation for Suicide Prevention and also relationship with Kessler Research Foundation that includes consulting or advisory and serves on the Editorial board of Brain Sciences and is an Associate Editor for Frontiers of Psychiatry - Schizophrenia Section.
The remaining authors declare that the research was conducted in the absence of any commercial or financial relationships that could be construed as a potential conflict of interest.
Publisher’s note
All claims expressed in this article are solely those of the authors and do not necessarily represent those of their affiliated organizations, or those of the publisher, the editors and the reviewers. Any product that may be evaluated in this article, or claim that may be made by its manufacturer, is not guaranteed or endorsed by the publisher.
References
Albers, M. W., Gilmore, G. C., Kaye, J., Murphy, C., Wingfield, A., Bennett, D. A., et al. (2015). At the interface of sensory and motor dysfunctions and Alzheimer’s disease. Alzheimers Dement. 11, 70–98. doi: 10.1016/j.jalz.2014.04.514
Alzheimer’s Association (2022). 2022 Alzheimer’s disease facts and figures. Alzheimers Dement. 18, 700–789. doi: 10.1002/alz.12638
Anderson, P., and Gill, R. (1982). Cox’s regression model for counting processes: A large sample study. Ann. Stat. 10, 1100–1120. doi: 10.1214/aos/1176345976
Ashendorf, L., Jefferson, A. L., Green, R. C., and Stern, R. A. (2009). Test-retest stability on the WRAT-3 reading subtest in geriatric cognitive evaluations. J. Clin. Exp. Neuropsychol. 31, 605–610. doi: 10.1080/13803390802375557
Ayers, E., and Verghese, J. (2014). Gait speed reflects cognitive function. Todays Geriatr. Med. 7:26.
Baker, P. S., Bodner, E. V., and Allman, R. M. (2003). Measuring life-space mobility in community-dwelling older adults. J. Am. Geriatr. Soc. 51, 1610–1614. doi: 10.1046/j.1532-5415.2003.51512.x
Barrick, M. R., and Mount, M. K. (1991). The big five personality dimensions and job performance: A meta-analysis. Pers. Psychol. 44, 1–26. doi: 10.1111/j.1744-6570.1991.tb00688.x
Bateman, R. J., Blennow, K., Doody, R., Hendrix, S., Lovestone, S., Salloway, S., et al. (2019). Plasma biomarkers of AD emerging as essential tools for drug development: An EU/US CTAD task force report. J. Prev. Alzheimers Dis. 6, 169–173. doi: 10.14283/jpad.2019.21
Beauchet, O., Allali, G., Annweiler, C., and Verghese, J. (2016). Association of motoric cognitive risk syndrome with brain volumes: Results from the GAIT study. J. Gerontol. A Biol. Sci. Med. Sci. 71, 1081–1088. doi: 10.1093/gerona/glw012
Beauchet, O., Allali, G., Berrut, G., Hommet, C., Dubost, V., and Assal, F. (2008). Gait analysis in demented subjects: Interests and perspectives. Neuropsychiatr. Dis. Treat. 4, 155–160. doi: 10.2147/NDT.S2070
Beck, A. T., Epstein, N., Brown, G., and Steer, R. A. (1988). An inventory for measuring clinical anxiety: Psychometric properties. J. Consult. Clin. Psychol. 56, 893–897. doi: 10.1037/0022-006X.56.6.893
Benton, A. L. (1968). Differential behavioral effects in frontal lobe disease. Neuropsychologia 6, 53–60. doi: 10.1016/0028-3932(68)90038-9
Berg, K. O., Wood-Dauphinee, S. L., Williams, J. I., and Maki, B. (1992). Measuring balance in the elderly: Validation of an instrument. Can. J. Public Health 83(Suppl. 2), S7–S11.
Blumen, H. M., Brown, L. L., Habeck, C., Allali, G., Ayers, E., Beauchet, O., et al. (2019). Gray matter volume covariance patterns associated with gait speed in older adults: A multi-cohort MRI study. Brain Imaging Behav. 13, 446–460. doi: 10.1007/s11682-018-9871-7
Brink, T. L., Yesavage, J. A., Lum, O., Heersema, P. H., Adey, M., and Rose, T. L. (1982). Screening tests for depression. Clin. Gerontol. 1, 37–43. doi: 10.1300/J018v01n01_06
Buysse, D. J., Reynolds, C. F. III, Monk, T. H., Berman, S. R., and Kupfer, D. J. (1989). The pittsburgh sleep quality index: A new instrument for psychiatric practice and research. Psychiatry Res. 28, 193–213. doi: 10.1016/0165-1781(89)90047-4
Campos, J. L., Marusic, U., and Mahoney, J. R. (2022). Editorial: The intersection of cognitive, motor, and sensory processing in aging: Links to functional outcomes, Volume I. Front. Aging Neurosci. 14:1009532. doi: 10.3389/fnagi.2022.1009532
Cao, Y., Summerfield, C., Park, H., Giordano, B. L., and Kayser, C. (2019). Causal inference in the multisensory brain. Neuron 102, 1076–1087.e8. doi: 10.1016/j.neuron.2019.03.043
Chung, F., Yegneswaran, B., Liao, P., Chung, S. A., Vairavanathan, S., Islam, S., et al. (2008). STOP questionnaire: A tool to screen patients for obstructive sleep apnea. J. Am. Soc. Anesthesiol. 108, 812–821. doi: 10.1097/ALN.0b013e31816d83e4
Conners, C. K. (2014). Conners continuous performance test manual, 3rd Edn. Toronto: Multi-Health Systems.
Craft, S., Newcomer, J., Kanne, S., Dagogo-Jack, S., Cryer, P., Sheline, Y., et al. (1996). Memory improvement following induced hyperinsulinemia in Alzheimer’s disease. Neurobiol. Aging 17, 123–130. doi: 10.1016/0197-4580(95)02002-0
de Mos, M., De Bruijn, A., Huygen, F., Dieleman, J., Stricker, B. C., and Sturkenboom, M. (2007). The incidence of complex regional pain syndrome: A population-based study. Pain 129, 12–20. doi: 10.1016/j.pain.2006.09.008
Duncan, P. W., Weiner, D. K., Chandler, J., and Studenski, S. (1990). Functional reach: A new clinical measure of balance. J. Gerontol. 45, M192–M197. doi: 10.1093/geronj/45.6.M192
Fan, J., McCandliss, B., Sommer, T., Raz, A., and Posner, M. (2002). Testing the efficiency and independence of attentional networks. J. Cogn. Neurosci. 14, 340–347. doi: 10.1162/089892902317361886
Feldman, E. L., Stevens, M. J., Thomas, P. K., Brown, M. B., Canal, N., and Greene, D. A. (1994). A practical two-step quantitative clinical and electrophysiological assessment for the diagnosis and staging of diabetic neuropathy. Diabetes Care 17, 1281–1289. doi: 10.2337/diacare.17.11.1281
Galvin, J. E., Roe, C. M., Powlishta, K. K., Coats, M. A., Muich, S. J., Grant, E., et al. (2005). The AD8: A brief informant interview to detect dementia. Neurology 65, 559–564. doi: 10.1212/01.wnl.0000172958.95282.2a
Golden, C. J. (1978). Stroop color and word test: A manual for clinical and experimental uses. Chicago, IL: Stoelting Co.
Gollan, T. H., Weissberger, G. H., Runnqvist, E., Montoya, R. I., and Cera, C. M. (2012). Self-ratings of spoken language dominance: A multilingual naming test (MINT) and preliminary norms for young and aging Spanish–English bilinguals. Bilingualism 15, 594–615. doi: 10.1017/S1366728911000332
Greve, K. W. (2001). The WCST-64: A standardized short-form of the wisconsin card sorting test. Clin. Neuropsychol. 15, 228–234. doi: 10.1076/clin.15.2.228.1901
Grober, E., Buschke, H., Crystal, H., Bang, S., and Dresner, R. (1988). Screening for dementia by memory testing. Neurology 38, 900–903. doi: 10.1212/WNL.38.6.900
Groeger, J. L., Ayers, E., Barzilai, N., Beauchet, O., Callisaya, M., Torossian, M. R., et al. (2022). Inflammatory biomarkers and motoric cognitive risk syndrome: Multicohort survey. Cereb. Circ. Cogn. Behav. 3:100151. doi: 10.1016/j.cccb.2022.100151
Guralnik, J. M., Simonsick, E. M., Ferrucci, L., Glynn, R. J., Berkman, L. F., Blazer, D. G., et al. (1994). A short physical performance battery assessing lower extremity function: Association with self-reported disability and prediction of mortality and nursing home admission. J. Gerontol. 49, M85–M94. doi: 10.1093/geronj/49.2.M85
Hausdorff, J. M., and Yogev, G. (2006). Cognitive function may be important for fall injury prevention trials. J. Am. Geriatr. Soc. 54, 865; author reply 865–866. doi: 10.1111/j.1532-5415.2006.00718.x
Hayes, A. (2018). Introduction to mediation, moderation, and conditional process analysis: A regression-based approach. New York, NY: The Guilford Press.
Heaton, R. K. (1981). A manual for the wisconsin card sorting task. Odessa: Psychological Assessment Resources, Inc.
Holtzer, R., Friedman, R., Lipton, R. B., Katz, M., Xue, X., and Verghese, J. (2007). The relationship between specific cognitive functions and falls in aging. Neuropsychology 21, 540–548. doi: 10.1037/0894-4105.21.5.540
Holtzer, R., Mahoney, J. R., Izzetoglu, M., Izzetoglu, K., Onaral, B., and Verghese, J. (2011). fNIRS study of walking and walking while talking in young and old individuals. J. Gerontol. A Biol. Sci. Med. Sci. 66, 879–887. doi: 10.1093/gerona/glr068
Holtzer, R., Mahoney, J., and Verghese, J. (2014). Intraindividual variability in executive functions but not speed of processing or conflict resolution predicts performance differences in gait speed in older adults. J. Gerontol. A Biol. Sci. Med. Sci. 69, 980–986. doi: 10.1093/gerona/glt180
Holtzer, R., Verghese, J., Wang, C., Hall, C. B., and Lipton, R. B. (2008). Within-person across-neuropsychological test variability and incident dementia. JAMA 300, 823–830. doi: 10.1001/jama.300.7.823
Holtzer, R., Wang, C., and Verghese, J. (2012). The relationship between attention and gait in aging: Facts and fallacies. Motor Control 16, 64–80. doi: 10.1123/mcj.16.1.64
Hugenschmidt, C. E., Mozolic, J. L., and Laurienti, P. J. (2009). Suppression of multisensory integration by modality-specific attention in aging. Neuroreport 20, 349–353. doi: 10.1097/WNR.0b013e328323ab07
Hurvitz, E. A., Richardson, J. K., and Werner, R. A. (2001). Unipedal stance testing in the assessment of peripheral neuropathy. Arch. Phys. Med. Rehabil. 82, 198–204. doi: 10.1053/apmr.2001.17830
Hurvitz, E. A., Richardson, J. K., Werner, R. A., Ruhl, A. M., and Dixon, M. R. (2000). Unipedal stance testing as an indicator of fall risk among older outpatients. Arch. Phys. Med. Rehabil. 81, 587–591. doi: 10.1016/S0003-9993(00)90039-X
Jack, C. R. Jr., Bennett, D. A., Blennow, K., Carrillo, M. C., Dunn, B., Haeberlein, S. B., et al. (2018). NIA-AA research framework: Toward a biological definition of Alzheimer’s disease. Alzheimers Dement. 14, 535–562. doi: 10.1016/j.jalz.2018.02.018
Jack, C. R. Jr., Knopman, D. S., Jagust, W. J., Petersen, R. C., Weiner, M. W., Aisen, P. S., et al. (2013). Tracking pathophysiological processes in Alzheimer’s disease: An updated hypothetical model of dynamic biomarkers. Lancet Neurol. 12, 207–216. doi: 10.1016/S1474-4422(12)70291-0
Jack, C. R. Jr., Wiste, H. J., Therneau, T. M., Weigand, S. D., Knopman, D. S., Mielke, M. M., et al. (2019). Associations of amyloid, tau, and neurodegeneration biomarker profiles with rates of memory decline among individuals without dementia. JAMA 321, 2316–2325. doi: 10.1001/jama.2019.7437
Jones, E. G., and Powell, T. P. (1970). An anatomical study of converging sensory pathways within the cerebral cortex of the monkey. Brain 93, 793–820. doi: 10.1093/brain/93.4.793
Kaplan, E., Goodglass, H., and Weintraub, S. (1983). The Boston naming test. Philadelphia, PA: Lea & Febiger.
Lawton, M., and Brody, E. M. (1970). Assessment of older people: Self-maintaining and instrumental activities of daily living. Nurs. Res. 19:278. doi: 10.1097/00006199-197005000-00029
Lezak, M. D., Diane, B. H., and Loring, D. W. (2004). Neuropsychological assessment. New York, NY: Oxford University Press.
Lipton, R. B., Katz, M. J., Kuslansky, G., Sliwinski, M. J., Stewart, W. F., Verghese, J., et al. (2003). Screening for dementia by telephone using the memory impairment screen. J. Am. Geriatr. Soc. 51, 1382–1390. doi: 10.1046/j.1532-5415.2003.51455.x
Lord, S., Sherrington, C., Menz, H., and Close, J. C. (2007). Falls in older people: Risk factors and strategies for prevention. Cambridge: Cambridge University Press. doi: 10.1017/CBO9780511722233
Lunetta, M., Le Moli, R., Grasso, G., and Sangiorgio, L. (1998). A simplified diagnostic test for ambulatory screening of peripheral diabetic neuropathy. Diabetes Res. Clin. Pract. 39, 165–172. doi: 10.1016/S0168-8227(98)00005-9
Mahoney, J. R., and Barnett-Cowan, M. (2019). Introduction to the special issue on multisensory processing and aging (part II): Links to clinically meaningful outcomes. Multisens. Res. 32, 665–670. doi: 10.1163/22134808-20191509
Mahoney, J. R., and Verghese, J. (2018). Visual-somatosensory integration and quantitative gait performance in aging. Front. Aging Neurosci. 10:377. doi: 10.3389/fnagi.2018.00377
Mahoney, J. R., and Verghese, J. (2019). Using the race model inequality to quantify behavioral multisensory integration effects. J. Vis. Exp. 147:59575. doi: 10.3791/59575-v
Mahoney, J. R., and Verghese, J. (2020). Does cognitive impairment influence visual-somatosensory integration and mobility in older adults? J. Gerontol. A Biol. Sci. Med. Sci. 75, 581–588. doi: 10.1093/gerona/glz117
Mahoney, J. R., and Verghese, J. (2022). Does cognitive impairment influence visual-somatosensory integration and mobility in older adults? J. Gerontol. A Biol. Sci. Med. Sci. 75, 581–588. Reprinted by permission of Oxford University Press on behalf of The Gerontological Society of America.
Mahoney, J. R., Cotton, K., and Verghese, J. (2019). Multisensory integration predicts balance and falls in older adults. J. Gerontol. A Biol. Sci. Med. Sci. 74, 1429–1435. doi: 10.1093/gerona/gly245
Mahoney, J. R., George, C. J., and Verghese, J. (2022). Introducing CatchU™: A novel multisensory tool for assessing patients’ risk of falling. J. Percept. Imaging 5, 407–401. doi: 10.2352/J.Percept.Imaging.2022.5.000407
Mahoney, J. R., Holtzer, R., and Verghese, J. (2014). Visual-somatosensory integration and balance: Evidence for psychophysical integrative differences in aging. Multisens. Res. 27, 17–42. doi: 10.1163/22134808-00002444
Mahoney, J. R., Oh-Park, M., Ayers, E., and Verghese, J. (2017). Quantitative trunk sway and prediction of incident falls in older adults. Gait Posture 58, 183–187. doi: 10.1016/j.gaitpost.2017.07.115
Mahoney, J. R., Verghese, J., and George, C. J. (2021). The influence of diabetes on multisensory integration and mobility in aging. Brain Sci. 11:285. doi: 10.3390/brainsci11030285
Mahoney, J. R., Verghese, J., Dumas, K., Wang, C., and Holtzer, R. (2012). The effect of multisensory cues on attention in aging. Brain Res. 1472, 63–73. doi: 10.1016/j.brainres.2012.07.014
Meyer, G. F., and Noppeney, U. (2011). Multisensory integration: From fundamental principles to translational research. Exp. Brain Res. 213, 163–166. doi: 10.1007/s00221-011-2803-z
Mozolic, J. L., Hugenschmidt, C. E., Peiffer, A. M., and Laurienti, P. J. (2012). “Multisensory integration and aging,” in The neural bases of multisensory processes, eds M. M. Murray and M. T. Wallace (Boca Raton, FL: CRC Press). doi: 10.1201/9781439812174-25
Nasreddine, Z. S., Phillips, N. A., Bédirian, V., Charbonneau, S., Whitehead, V., Collin, I., et al. (2005). The montreal cognitive assessment, MoCA: A brief screening tool for mild cognitive impairment. J. Am. Geriatr. Soc. 53, 695–699. doi: 10.1111/j.1532-5415.2005.53221.x
Pillemer, S., Holtzer, R., and Blumen, H. M. (2017). Functional connectivity associated with social networks in older adults: A resting-state fMRI study. Soc. Neurosci. 12, 242–252. doi: 10.1080/17470919.2016.1176599
Possin, K. L., Laluz, V. R., Alcantar, O. Z., Miller, B. L., and Kramer, J. H. (2011). Distinct neuroanatomical substrates and cognitive mechanisms of figure copy performance in Alzheimer’s disease and behavioral variant frontotemporal dementia. Neuropsychologia 49, 43–48. doi: 10.1016/j.neuropsychologia.2010.10.026
Powell, L. E., and Myers, A. M. (1995). The activities-specific balance confidence (ABC) scale. J. Gerontol. A Biol. Sci. Med. Sci. 50, M28–M34. doi: 10.1093/gerona/50A.1.M28
Russell, D. (1996). UCLA loneliness scale (version 3): Reliability, validity, and factor structure. J. Pers. Assess. 66, 20–40. doi: 10.1207/s15327752jpa6601_2
Sanders, A. E., Holtzer, R., Lipton, R. B., Hall, C., and Verghese, J. (2008). Egocentric and exocentric navigation skills in older adults. J. Gerontol. A Biol. Sci. Med. Sci. 63, 1356–1363. doi: 10.1093/gerona/63.12.1356
Shahid, A., Wilkinson, K., Marcu, S., and Shapiro, C. M. (2011). “Brief fatigue inventory,” in STOP, THAT and one hundred other sleep scales, eds A. Shahid, K. Wilkinson, S. Marcu, and C. Shapiro (New York, NY: Springer), 75–77. doi: 10.1007/978-1-4419-9893-4_11
Sherbourne, C. D., and Stewart, A. L. (1991). The MOS social support survey. Soc. Sci. Med. 32, 705–714. doi: 10.1016/0277-9536(91)90150-B
Shumway-Cook, A., and Woollacott, M. (2012). Motor control. Philadelphia, PA: Lippincott Williams & Wilkins.
Shy, M. E., Frohman, E. M., So, Y. T., Arezzo, J. C., Cornblath, D. R., Giuliani, M. J., et al. (2003). Quantitative sensory testing: Report of the therapeutics and technology assessment subcommittee of the American academy of neurology. Neurology 60, 898–904. doi: 10.1212/01.WNL.0000058546.16985.11
Thal, D. R., Rub, U., Orantes, M., and Braak, H. (2002). Phases of A beta-deposition in the human brain and its relevance for the development of AD. Neurology 58, 1791–1800. doi: 10.1212/WNL.58.12.1791
Tiffin, J., and Asher, E. J. (1948). The purdue pegboard: Norms and studies of reliability and validity. J. Appl. Psychol. 32, 234–247. doi: 10.1037/h0061266
Tinetti, M. E., Baker, D. I., Mcavay, G., Claus, E. B., Garrett, P., Gottschalk, M., et al. (1994). A multifactorial intervention to reduce the risk of falling among elderly people living in the community. N. Engl. J. Med. 331, 821–827. doi: 10.1056/NEJM199409293311301
Tinetti, M. E., Richman, D., and Powell, L. (1990). Falls efficacy as a measure of fear of falling. J. Gerontol. 45, 239–243. doi: 10.1093/geronj/45.6.P239
Tripathi, S., Verghese, J., Callisaya, M., Mahoney, J. R., Srikanth, V., and Blumen, H. M. (2022). Brain patterns of pace – but not rhythm – are associated with vascular disease in older adults. Cereb. Circ. Cogn. Behav. 3:100154. doi: 10.1016/j.cccb.2022.100154
Verghese, J., and Xue, X. (2010). Identifying frailty in high functioning older adults with normal mobility. Age Ageing 39, 382–385. doi: 10.1093/ageing/afp226
Verghese, J., and Xue, X. (2011). Predisability and gait patterns in older adults. Gait Posture 33, 98–101. doi: 10.1016/j.gaitpost.2010.10.004
Verghese, J., Buschke, H., Viola, L., Katz, M., Hall, C., Kuslansky, G., et al. (2002a). Validity of divided attention tasks in predicting falls in older individuals: A preliminary study. J. Am. Geriatr. Soc. 50, 1572–1576. doi: 10.1046/j.1532-5415.2002.50415.x
Verghese, J., Cuiling, W., Katz, M. J., Sanders, A., and Lipton, R. B. (2003). Leisure activities and risk of vascular cognitive impairment in older adults. J. Geriatr. Psychiatry Neurol. 22, 110–118. doi: 10.1177/0891988709332938
Verghese, J., Holtzer, R., Lipton, R. B., and Wang, C. (2012). Mobility stress test approach to predicting frailty, disability, and mortality in high-functioning older adults. J. Am. Geriatr. Soc. 60, 1901–1905. doi: 10.1111/j.1532-5415.2012.04145.x
Verghese, J., Holtzer, R., Lipton, R., and Wang, C. (2009). Quantitative gait markers and incident fall risk in older adults. J. Gerontol. A Biol. Sci. Med. Sci. 64, 896–901. doi: 10.1093/gerona/glp033
Verghese, J., Katz, M. J., Derby, C. A., Kuslansky, G., Hall, C. B., and Lipton, R. B. (2004). Reliability and validity of a telephone-based mobility assessment questionnaire. Age Ageing 33, 628–632. doi: 10.1093/ageing/afh210
Verghese, J., Kuslansky, G., Holtzer, R., Katz, M., Xue, X., Buschke, H., et al. (2007a). Walking while talking: Effect of task prioritization in the elderly. Arch. Phys. Med. Rehabil. 88, 50–53. doi: 10.1016/j.apmr.2006.10.007
Verghese, J., Lipton, R. B., Hall, C. B., Kuslansky, G., Katz, M. J., and Buschke, H. (2002b). Abnormality of gait as a predictor of non-Alzheimer’s dementia. N. Engl. J. Med. 347, 1761–1768. doi: 10.1056/NEJMoa020441
Verghese, J., Robbins, M., Holtzer, R., Zimmerman, M., Wang, C., Xue, X., et al. (2008a). Gait dysfunction in mild cognitive impairment syndromes. J. Am. Geriatr. Soc. 56, 1244–1251. doi: 10.1111/j.1532-5415.2008.01758.x
Verghese, J., Wang, C., Lipton, R. B., Holtzer, R., and Xue, X. (2007b). Quantitative gait dysfunction and risk of cognitive decline and dementia. J. Neurol. Neurosurg. Psychiatry 78, 929–935. doi: 10.1136/jnnp.2006.106914
Verghese, J., Wang, C., Xue, X., and Holtzer, R. (2008b). Self-reported difficulty in climbing up or down stairs in nondisabled elderly. Arch. Phys. Med. Rehabil. 89, 100–104. doi: 10.1016/j.apmr.2007.08.129
Wallace, M. T. (2012). “The impact of multisensory alterations in human developmental disabilities and disease: The tip of the iceberg?,” in The new handbook of multisensory processing, ed. B. E. Stein (Cambridge, MA: The MIT Press), 645–656. doi: 10.7551/mitpress/8466.003.0057
Ware, J. E. Jr., Kosinski, M., and Keller, S. D. (1996). A 12-Item short-form health survey: Construction of scales and preliminary tests of reliability and validity. Med. Care 34, 220–233. doi: 10.1097/00005650-199603000-00003
Wechsler, D. (2008). Wechsler adult intelligence scale, 4th Edn. San Antonio, TX: Pearson. doi: 10.1037/t15169-000
Woollacott, M., and Shumway-Cook, A. (2002). Attention and the control of posture and gait: A review of an emerging area of research. Gait Posture 16, 1–14. doi: 10.1016/S0966-6362(01)00156-4
Yogev-Seligmann, G., Hausdorff, J. M., and Giladi, N. (2008). The role of executive function and attention in gait. Mov. Disord. 23, 329–342. doi: 10.1002/mds.21720
Yuan, J., Blumen, H. M., Verghese, J., and Holtzer, R. (2015). Functional connectivity associated with gait velocity during walking and walking-while-talking in aging: A resting-state fMRI study. Hum. Brain Mapp. 36, 1484–1493. doi: 10.1002/hbm.22717
Keywords: multisensory integration, sensory processing, mobility, cognition, Alzheimer’s disease
Citation: Mahoney JR, Blumen HM, De Sanctis P, Fleysher R, Frankini C, Hoang A, Hoptman MJ, Jin R, Lipton M, Nunez V, Twizer L, Uy N, Valdivia A, Verghese T, Wang C, Weiss EF, Zwerling J and Verghese J (2023) Visual-somatosensory integration (VSI) as a novel marker of Alzheimer’s disease: A comprehensive overview of the VSI study. Front. Aging Neurosci. 15:1125114. doi: 10.3389/fnagi.2023.1125114
Received: 15 December 2022; Accepted: 03 March 2023;
Published: 30 March 2023.
Edited by:
Jean-Jacques Temprado, Aix-Marseille Université, FranceReviewed by:
Hadrien Ceyte, Aix-Marseille Université, FranceKimberly Albert, Vanderbilt University Medical Center, United States
Copyright © 2023 Mahoney, Blumen, De Sanctis, Fleysher, Frankini, Hoang, Hoptman, Jin, Lipton, Nunez, Twizer, Uy, Valdivia, Verghese, Wang, Weiss, Zwerling and Verghese. This is an open-access article distributed under the terms of the Creative Commons Attribution License (CC BY). The use, distribution or reproduction in other forums is permitted, provided the original author(s) and the copyright owner(s) are credited and that the original publication in this journal is cited, in accordance with accepted academic practice. No use, distribution or reproduction is permitted which does not comply with these terms.
*Correspondence: Jeannette R. Mahoney, amVhbm5ldHRlLm1haG9uZXlAZWluc3RlaW5tZWQuZWR1