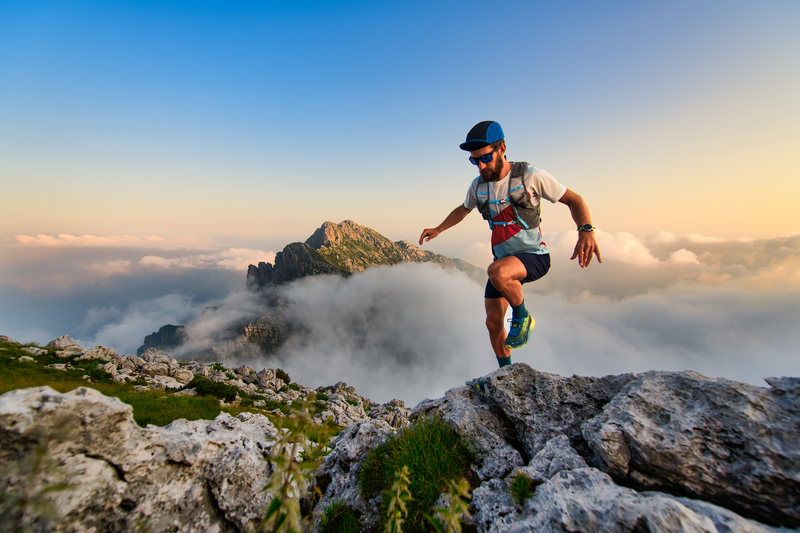
94% of researchers rate our articles as excellent or good
Learn more about the work of our research integrity team to safeguard the quality of each article we publish.
Find out more
ORIGINAL RESEARCH article
Front. Aging Neurosci. , 14 March 2023
Sec. Parkinson’s Disease and Aging-related Movement Disorders
Volume 15 - 2023 | https://doi.org/10.3389/fnagi.2023.1120615
This article is part of the Research Topic Insights in Parkinson’s Disease and Aging-related Movement Disorders: 2022 View all 12 articles
Introduction: Although the relationship between psychiatric disorders and Parkinson’s disease (PD) has attracted continuous research attention, the causal linkage between them has not reached a definite conclusion.
Methods: To identify the causal relationship between psychiatric disorders and PD, we used public summary-level data from the most recent and largest genome-wide association studies (GWASs) on psychiatric disorders and PD to conduct a bidirectional two-sample Mendelian randomization (MR). We applied stringent control steps in instrumental variable selection using the Mendelian randomization pleiotropy residual sum and outlier (MR-PRESSO) method to rule out pleiotropy. The inverse-variance weighted (IVW) method was used to identify the causal relationship between psychiatric disorders and PD. Multiple MR analysis methods, including MR-Egger, weighted-median, and leave-one-out analyses, were used for sensitivity analysis, followed by heterogeneity tests. Further validation and reverse MR analyses were conducted to strengthen the results of the forward MR analysis.
Results: The lack of sufficient estimation results could suggest a causal relationship between psychiatric disorders and PD in the forward MR analysis. However, the subsequent reverse MR analysis detected a causal relationship between PD and bipolar disorder (IVW: odds ratios [OR] =1.053, 95% confidence interval [CI] =1.02–1.09, p = 0.001). Further analysis demonstrated a causal relationship between genetically predicted PD and the risk of bipolar disorder subtype. No pleiotropy or heterogeneity was detected in the analyses.
Discussion: Our study suggested that while psychiatric disorders and traits might play various roles in the risk of developing PD, PD might also be involved in the risk of developing psychiatric disorders.
Parkinson’s disease (PD), the most common movement disorder and the second most common neurodegenerative disease, is characterised by a wide range of motor and non-motor symptoms (NMS) (Lees et al., 2009). PD affects 1% of the population over 60 years of age, and its prevalence generally ranges from 100 to 200 per 100,000 persons in unselected populations (von Campenhausen et al., 2005). Owing to PD aetiology, particularly in sporadic cases, distinguishing causal risk factors could be meaningful for treatment and prevention of this disease. PD diagnosis is mainly based on typical motor symptoms, including tremors, rigidity, bradykinesia, and motor impairment, which have also been increasingly considered an important component of PD (Armstrong and Okun, 2020). NMS, including sleep disorders, autonomic dysfunction, cognitive/neurobehavioural abnormalities, and sensory abnormalities (such as anosmia, pain, and paraesthesia) have an even higher negative impact on the quality of life than motor symptoms (Karlsen et al., 2000). In general, NMS are highly prevalent in PD patients; NMS can also develop at any phases of the disease and frequently precedes the onset of motor symptoms (Krishnan et al., 2011; Kim et al., 2013). Psychiatric disorders, including anxiety, depression, psychosis, sleep disturbances, and behavioural and cognitive changes, are widely recognised as common NMS in PD and are more frequent in PD patients than in the general population (Aarsland et al., 2009). Furthermore, numerous studies have shown that the morbidity of psychotic illnesses is higher in PD patients (Djamshidian and Friedman, 2014; Ghaddar et al., 2016; Ffytche et al., 2017).
Increasing evidence points to the multifactorial aetiology of PD, which involves ageing, genetic predisposition, environmental agents, trauma, and psychosocial impact (Perry et al., 2016). Additionally, psychiatric disorders have a significant influence on the quality of life of PD patients and may play a critical role in the rapid deterioration of clinical manifestations (Alvarado-Bolanos et al., 2015; Ng et al., 2015; Elefante et al., 2021). Therefore, further understanding of the association between psychiatric disorders and PD is valuable for research and clinical practice.
Psychiatric disorders have been proposed to precede PD development and be a possible PD risk factor, which has been proven in observational studies (Ishihara-Paul et al., 2008; Jacob et al., 2010; Sanyal et al., 2010; Lin et al., 2014; Gustafsson et al., 2015; Schrag et al., 2015). A meta-analysis of prospective studies using a large United Kingdom biobank indicated that neuroticism was consistently associated with a higher risk of PD (Terracciano et al., 2021). Another analysis of four cohort studies and three cross-sectional studies, consisting of 4,374,211 individuals, also reported that patients with bipolar disorder (BD) might be at risk of having a subsequent idiopathic PD diagnosis (Dols and Lemstra, 2020). Additionally, sleep disturbances, including insomnia and rapid eye movement sleep behaviour disorder (RBD), are often considered early markers of PD pathology and an independent PD risk factor (Hsiao et al., 2017). Although specific underlying mechanism of this phenomenon remains unclear, recent studies have indicated that this process could be related to abnormal brainstem α-synucleinopathy, which caused RBD (Bohnen and Hu, 2019). These studies suggested that psychiatric disorders might potentially trigger the onset of PD. With the global escalation of the ageing process, the rapidly increasing incidence of PD is impacting the quality of life of more people. Therefore, clarifying the causality of these associations could be of practical value for improving the health of the elderly.
Mendelian randomization (MR) is an epidemiological technique that investigates the causal relationship between risk factors and outcomes. MR tends to avoid confounding issues and reverse causality by using genetic variants as instrumental variables (IVs). This technique has been extensively used to validate causal relationships discovered in observational studies (Plotnikov and Guggenheim, 2019). Owing to the development of genomic techniques and methodologies, an increasing number of disease-associated variants have been detected by genome-wide association studies (GWAS), which provide sufficient IV resources that could be used to increase the power of MR. Therefore, we used publicly available GWAS summary-level data of psychiatric disorders and PD for a bidirectional two-sample MR analysis to validate and explore the relationships among these traits.
In this two-sample MR analysis, we included publicly available GWAS results of eight psychiatric disorders/traits and PD conducted in European populations. The basic characteristics of GWASs, including exposures and outcomes, are listed in Table 1. Exposure to interest included anorexia nervosa (AN) (Watson et al., 2019), anxiety disorder (Purves et al., 2020), BD (Mullins et al., 2021), insomnia (Watanabe et al., 2022), major depressive disorder (MDD) (Howard et al., 2019), neuroticism (Nagel et al., 2018), obsessive–compulsive disorder (OCD) [International Obsessive Compulsive Disorder Foundation Genetics Collaborative (LOCDF-GC) and OCD Collaborative Genetics Association Studies (OCGAS), 2018], and schizophrenia (Trubetskoy et al., 2022). For PD, we used the largest GWAS conducted in the European population (Nalls et al., 2019) as a discovery study and a GWAS conducted in the Finnish population (Kurki et al., 2023) as a validation study. Detailed information regarding genotype platforms, statistical analysis protocols, and participants for each study is available in the corresponding papers (Data presentation).
Valid IVs need to meet the following three assumptions: (1) association with the risk exposure of interest (relevance); (2) no shared common cause with the outcome (independence); and (3) they affect the outcome only through the risk factor (exclusion restriction assumption) (Davies et al., 2018). To ensure that all included IVs were valid, we employed a series of stringent control steps (Figure 1). First, we extracted genome-wide significant (p < 5 × 10−8) single-nucleotide polymorphisms (SNPs) from exposure GWASs. Since no genome-wide significant SNPs were detected in the GWAS of OCD, we chose to use suggestive significant SNPs (p < 1 × 10−5) as IVs. We then performed linkage disequilibrium (LD) clumping (R2 < 0.001, window size = 10,000 kb) based on the European 1,000 Genomes Project reference panel to select independent significant SNPs. Those with minor allele frequency (MAF) >0.01 and the lowest value of p were retained. F and weak instrumental variables.
Using the selected SNPs, we extracted SNPs from the outcome/PD GWASs. For those absent in the outcome GWASs, a proxy SNP in LD (R2 > 0.8) with the requested SNP was searched according to 1,000 genomes of European samples. After harmonisation of the two aforementioned sets of SNPs, palindromic SNPs with intermediate allele frequencies were removed, and the remaining SNPs were kept as primary IVs. Next, we performed the Steiger test for each SNP to determine whether the R2 (variance of disease/trait explained by selected SNPs) of the exposure was larger than the R2 of the outcome. SNPs tested as “FALSE” direction (R2 of the outcome >R2 of the exposure) would be excluded.
The horizontal pleiotropy, which occurs when an IV affects the outcome outside of its effect on the exposure, is the main factor that violates the assumptions (Hemani et al., 2018). The MR pleiotropy residual sum and outlier (MR-PRESSO) test was developed to identify horizontal pleiotropy in multi-instrument summary-level MR testing (Verbanck et al., 2018). It is a sequential method comprising three components: a global test for horizontal pleiotropy, an outlier test for each genetic variant, and a distortion test of the difference in the causal estimates before and after outlier removal. At the stage of IV selection, we adopted a stringent filtering step by discarding SNPs with a value of p < 1 in the outlier test and repeated this step until no outliers were detected. Finally, the remaining SNPs were uploaded to PhenoScanner to examine whether any of them was previously associated with PD (p < 5 × 10−8 in published PD GWASs) and remove possible confounding SNPs (Kamat et al., 2019).
Utilizing R2 (variance of exposure explained by the selected IVs) generated from the Steiger test, we calculated the overall F statistics (F = R2 [n – k – 1]/k[1 − R2], n: sample size, k: number of IVs) to quantify the strength of the IVs, and F > 10 was considered sufficient to overcome the weak instrumental variable bias (Burgess et al., 2013).
The inverse variance weighted (IVW) fixed-effect method, which combines the ratio estimated using each variant in a fixed-effect meta-analysis mode, was adopted to estimate the causative effect of each exposure on the outcome (Burgess et al., 2013). Because the IVW method provides an unbiased causal estimation only when all IVs are valid, we adopted other methods of sensitive analysis when a violation of the assumptions inevitably existed. The weighted-median method can provide a consistent estimate when 50% of the IVs are invalid, at the cost of reduced statistical power (Bowden et al., 2016). MR-Egger regression allows all genetic variants to be invalid IVs under the Instrument Strength Independent of Direct Effect (InSIDE) assumption, which indicates that the pleiotropic effects of genetic variants on the outcome are direct (not through a confounder) (Bowden et al., 2015). The MR-Egger regression method also provides an intercept term for estimating the average pleiotropic effect across genetic variants. Even though we adopted stringent control steps, the MR-PRESSO method might still detect horizontal pleiotropy in the global test in the absence of detected outliers, which may be caused by a violation of the InSIDE assumption (Verbanck et al., 2018). Therefore, if pleiotropy was detected by the MR-PRESSO global test or the value of p of MR-Egger intercept was below 0.05, we used the results from the weighted-median method as the main method. The Cochran’s Q statistic was implemented to quantify heterogeneities, and a value of p < 0.05 was considered significant heterogeneity. We also performed a leave-one-out sensitivity analysis by sequential exclusion of each SNP at a time to determine whether particular variants were driving the association between the exposure and the outcome, and an IVW method was performed on the remaining SNPs. To ensure the stability of our results, we used another PD GWAS, conducted among the Finnish population, as the outcome as described above. Individual estimates were pooled using a fixed-effect meta-analysis. To adjust for multiple testing, a Bonferroni-corrected value of p was set as 0.05/8, meanwhile value of p < 0.05 was regarded as nominally significant.
In addition, we performed reverse MR to examine for reverse causality of PD on psychiatric disorders/traits. Genetic variant selection steps and MR analysis were conducted in a similar manner to that of forward MR. All statistical analyses were conducted using R version 4.2.1, with the use of the ‘TwoSampleMR’, ‘MRPRESSO’ and ‘META’ packages for the MR analysis.
Details of the IVs used for each MR analysis (both primary and validation analyses) are presented in the Supplementary Tables S1–S9. The main results, including causal estimation, the pleiotropy test, and the heterogeneity test, are presented in Tables 2, 3.
For the primary analysis, instrumental variables from GWAS of psychiatric disorders collectively explained 0.18–4.40% of the variance. The F-statistic ranged from 22.40 to 109.40, suggesting no possible weak instrument bias. Under the IVW model, genetic predispositions to psychiatric disorders were not associated with PD (Table 2). Although we performed stringent control steps and no outliers were detected before we conducted MR analysis, the MR-PRESSO global test still indicated the existence of pleiotropy among IVs from schizophrenia GWAS, which indicated that the violation of the InSIDE assumption made causation estimates of IVW and MR-Egger unreliable. However, the estimates calculated using the weighted-median method were not significant. Horizontal pleiotropy in variants generated from MDD was detected by the MR-Egger regression intercept (p = 0.037), but not by the MR-PRESSO global test (p = 0.491). Therefore, it is more precise to adopt the estimation from MR-Egger calculation that genetically predicted MDD was negatively nominally associated with PD risk (OR = 0.278, 95%CI, 0.09–0.88, p = 0.035). However, this association was not confirmed by the weighted-median method. Leave-one-out analysis suggested no potential influence of a particular variant on the estimate (Supplementary Tables S2–S9).
In the validation analysis, no horizontal pleiotropy was detected using the MR-PRESSO global test, and the Cochran’s Q statistic value of p suggested no heterogeneity within each set of variants. Under the IVW model, genetically predicted AN was associated with PD risk (OR = 1.381, 95% CI = 1.02–1.87, p = 0.037). However, this association was not confirmed in the sensitivity analysis, which also showed a different association direction. Anxiety, insomnia, and MDD were predicted to be inversely associated with PD risk. Similar to AN, the causal estimation of anxiety in PD was not confirmed by other methods, which also showed a different association direction. However, estimation of the causal effect of insomnia and MDD on PD was validated by sensitivity analysis in the same direction, without observance of pleiotropy and heterogeneity. For neuroticism, the MR-Egger regression intercept indicated the presence of pleiotropy (p = 0.03), and MR-Egger showed an opposite causal estimate of PD risk (OR = 0.050, 95% CI = 0.00–0.62, p = 0.022). The sensitivity analysis also showed the same causal direction. Leave-one-out plots demonstrated that these associations were unlikely to be driven by extreme SNPs (Supplementary Tables S2–S9).
Due to the inconsistent results of the primary and validation analyses, we meta-analysed the results using a fixed-effect model. No significant results were detected, except for anxiety disorders (Pooled OR = 0.84, 95%CI, 0.71–0.99).
To clarify the precise causal relationship between psychiatric disorders and PD, we performed a reverse MR analysis, with PD as the exposure and psychiatric disorders as the outcome. Interestingly, we detected a causal relationship between genetically predicted PD and BD risk (Table 3). No pleiotropy or heterogeneity was detected in these results, and leave-one-out analysis demonstrated no potentially influential SNPs driving the causal link (Figure 2).
Figure 2. Causal effect estimation of PD on BD. (A–C) Scatterplots of potential effects of SNPs on BD (BD/BD I/BD II) and PD. Corresponding slope of each represents the estimated MR effect of each method. (D–F) Leave-one-out-sensitivity forest plots of PD-BD (BD/BD I/BD II) results.
Based on the potential relevance between psychiatric disorders and PD, our study appears to be the first research using bidirectional two-sample MR analysis to thoroughly investigate the causal relationship between genetically predicted psychiatric disorders/traits and PD. We found some evidence of different effects of psychiatric disorders/traits on the liabilities of PD. For MR detection of certain causal relationships, no reverse causal association was detected in the reverse MR analysis. Notably, we found evidence for the causality of PD on the risk of developing BD.
Although we found no statistical significance to support a causal association between AN and PD in the discovery cohort, the sensitivity analysis showed a consistent trend. Furthermore, a causal relationship was observed in the validation cohort. To our best knowledge, few studies have addressed the association between AN and PD. Although both diseases share some common clinical and pathological phenotypes, such as dopamine deficits, hyposmia, micrographia, and hypophonia (Roessner et al., 2005; Schreder et al., 2008; Sekar et al., 2010; Stievenard et al., 2017; Favier et al., 2020), the relationship between these diseases has yet to be firmly verified. Hence, this MR result needs to be interpreted cautiously and requires confirmation through additional relevant studies.
To date, many prospective historical cohorts and observational studies have proven epidemiological links between depression and PD (Wang et al., 2018). Many studies have attempted to demonstrate that depression and PD shared a similar pathophysiological brain dysfunction. Specifically, monoamine deficiency (i.e., dopamine, 5-hydroxytryptamine, and noradrenaline), one of the earliest suggested biological mechanisms of MDD, has also been observed in PD patients, particularly in early and prodromal patients with RBD (Hamon and Blier, 2013; Barber et al., 2018). In addition to monoaminergic neurotransmission and chronic inflammation, gamma-aminobutyric acid decline and cerebral atrophy are considered common pathophysiological characteristics underlying depression and PD (Gao and Bao, 2011; Saiki, 2014; Blaszczyk, 2016; Huang et al., 2016; D'Mello and Swain, 2017; Li et al., 2022). On one hand, since approximately 60% of PD patients have experienced psychosis, and many are taking or have taken antipsychotic medications (Weintraub et al., 2017), clinicians are more inclined to misdiagnose drug-induced parkinsonism as PD. On the other hand, as a frequently observed symptom of NMS, depression is more likely to be considered a common complication secondary to PD. First, in the discovery cohort, our analysis revealed a clear causal relationship between MDD and PD. This result was verified by subsequent duplicating assays with different GWAS. However, instead of increasing the risk of PD, genetically predicted MDD had a protective role against PD risk. Such opposing trends have also been observed in the association between insomnia and PD. However, the significant finding in the validation MR (IVW: OR = 0.563, 95%CI: 0.33–0.97, p = 0.037) was not supported by the sensitivity analysis. Moreover, these findings also contradicted those from the few current studies in this field, which considered that these diseases could predict an increased risk of PD (Weisskopf et al., 2003; Bower et al., 2010; Lin et al., 2015; Terracciano et al., 2021). This anomalous protective effect may be attributed to the survivor bias, caused by the reduced life expectancy of severe mental illness patients, and the increasing prevalence of PD in the older age group (Lees et al., 2009; Boef et al., 2015; Vansteelandt et al., 2018; Plana-Ripoll et al., 2019).
To clarify the exact causal relationship between psychiatric disorders and PD, we performed a reverse MR analysis, with PD as the exposure and psychiatric disorders as the outcomes. Interestingly, we detected a causal relationship between genetically predicted PD and BD risk. According to the Diagnostic and Statistical Manual Disorders, Fifth Edition (DSM-5), BD I and BD II are the major two clinical subtypes of BD, which are characterized by the classification of mania or hypomania. BD II is sometimes considered a milder form of BD I, because these two subtypes of BD share some phenomenological features (Liu et al., 2022). However, with increasing advances in BD epidemiology, clinical presentation (Zimmerman et al., 2013), and genetic basis (Charney et al., 2017), the important difference between the two subtypes is gradually being recognised. Further exploration of the specific role of BD subtypes in this association revealed that PD might be a risk factor for BD I rather than BD II. There is growing evidence that PD patients have a high prevalence rate of various psychiatric disorders. BD is generally considered a specific risk factor for PD, rather than an ensuing complication (Huang et al., 2019), which is contrary to our results. However, the diagnosis of PD is currently based on clinical manifestations which became evident when 50% of dopaminergic neurons were lost in the substantia nigra (Marsden, 1990). So it’s difficult to find out the exact beginning of the PD pathologic process. Taking the shared molecular mechanism into account, some may think it more appropriate that BD be viewed as an early symptom of PD (Pontone and Koch, 2019). As PD advances psychiatric complications emerge. 17% of patients with PD treated with dopaminergic therapy and 4% undergone DBS develop mania or hypomania, symptomatically similar to that of BD (Temel et al., 2006; Maier et al., 2014). In clinical terms, depression is not only a typical presentation of BD (Vieta et al., 2018), but also an important NMS of PD, which may hint to potential common clinical features between these diseases. However, similar to previous analyses, further related studies are needed to verify this finding.
Regarding other psychiatric disorders/traits, including OCD and schizophrenia, contrary to epidemiologic and other studies, we did not detect the causal association between these psychiatric conditions and PD. However, a close relationship exists between these two conditions. In the most updated nationwide longitudinal study published in 2022, OCD was reportedly an independent risk factor for PD (Liou et al., 2022). Another retrospective record-based case–control study demonstrated that schizophrenia spectrum disorder contributed to the incremental risk of PD in the elderly population (Kuusimaki et al., 2021). Understanding these potential association mechanisms may advance our knowledge of the underlying biology of these diseases and facilitate the early diagnosis of PD.
Our results should be viewed in the context of several limitations. Considering the existence of ethnicity-specific genomic heterogeneity, our findings must be interpreted with caution and may not be applicable to other racial/ethnic backgrounds, since all GWASs were conducted in European populations. Moreover, despite recent advances, existing GWAS methodology may still lack the ability to detect sufficient heritability of diseases, thus limiting the statistical power of the IVs used in this MR analysis. Owing to the lack of explicit measures and knowledge of potential confounders, pleiotropy may not be completely ruled out, and it is difficult to discuss the extent by which the results are influenced by this phenomenon. It is also difficult to determine the degree of sample overlap between the original GWASs of exposures and outcomes, which could cause weak instrument bias and violation of the independence assumption. However, bias from weak instruments in very large consortia may not be substantial and the F statistics was calculated to insure and minimize such bias (Burgess et al., 2016). For the violation of independence assumption, 2-sample MR methods can be safely applied to one-sample MR performed within large bio banks (Minelli et al., 2021). As presented above, many patients with psychiatric disorders have a history of antipsychotic use, which makes them more inclined to be diagnosed with drug-induced parkinsonism rather than PD. This possible misdiagnosis may have increased the effect of confounding factors on our results. Nevertheless, this study has several strengths. First, for each psychiatric exposure trait, we selected the latest and largest GWASs in European populations to ensure a reliable conclusion. Second, we applied a very stringent SNP quality threshold to reduce potential pleiotropic effects as much as possible. Moreover, this bidirectional MR analysis covered a wide variety of psychiatric disorders and traits, filling a gap in the observational literature and broadening our understanding of the relationship between psychosis and PD.
Collectively, in European populations, this 2-sample MR analysis provided evidence that genetically predicted psychiatric disorders may play various roles in the risk of PD. Concurrently, reverse MR analysis suggested that PD should be considered as a risk factor for BD subtype I. This significant result, supported by sensitive MR methods, showed no obvious heterogeneity or pleiotropy. Although these findings could be biased due to horizontal pleiotropy, we did not consider and detect hypotheses between psychiatric disorders and PD. As such, this topic merits further exploration.
The original contributions presented in the study are included in the article/Supplementary material, further inquiries can be directed to the corresponding author/s.
The studies involving human participants were reviewed and approved by The Ethics Committee of Xiangya Hospital of Central South University in China, the number of the approval is 202103191. The patients/participants provided their written informed consent to participate in this study.
QW and SL performed the majority of the analyses and wrote the manuscript. SL and YX conducted the systematic review. JG designed the study. All authors contributed to the article and approved the submitted version.
This study was supported by the national key plan for scientific research and development of China (2021YFC2501204), Technology Major Project of Hunan Provincial Science and Technology Department (grant no. 2021SK1010), the National Natural Science Foundation of China (grant no. 81873785, 82071439, 81974202, and U20A20355), Hunan Province Innovative Construction Project Science (grant no. 2019SK2335), and the Innovation-driven Team Project from Central South University (grant no. 2020CX016), the innovative team program from Department of Science & Technology of Hunan Province (grant no. 2019RS1010).
We want to acknowledge the participants and investigators of FinnGen study, Psychiatric Genomics Consortium, UK Biobank, Center for Neurogenomics and Cognitive Research and International Parkinson Disease Genomics Consortium for their generous donation of bio sample and making these data publicly available.
The authors declare that the research was conducted in the absence of any commercial or financial relationships that could be construed as a potential conflict of interest.
The reviewer ZH declared a shared affiliation with the authors to the handling editor at the time of review.
All claims expressed in this article are solely those of the authors and do not necessarily represent those of their affiliated organizations, or those of the publisher, the editors and the reviewers. Any product that may be evaluated in this article, or claim that may be made by its manufacturer, is not guaranteed or endorsed by the publisher.
The Supplementary material for this article can be found online at: https://www.frontiersin.org/articles/10.3389/fnagi.2023.1120615/full#supplementary-material
Aarsland, D., Marsh, L., and Schrag, A. (2009). Neuropsychiatric symptoms in Parkinson's disease. Mov. Disord. 24, 2175–2186. doi: 10.1002/mds.22589
Alvarado-Bolanos, A., Cervantes-Arriaga, A., Rodriguez-Violante, M., Llorens-Arenas, R., Calderon-Fajardo, H., Millan-Cepeda, R., et al. (2015). Impact of neuropsychiatric symptoms on the quality of life of subjects with Parkinson's disease. J. Parkinsons Dis. 5, 541–548. doi: 10.3233/JPD-150597
Armstrong, M. J., and Okun, M. S. (2020). Diagnosis and treatment of Parkinson disease: a review. JAMA 323, 548–560. doi: 10.1001/jama.2019.22360
Barber, T. R., Griffanti, L., Muhammed, K., Drew, D. S., Bradley, K. M., McGowan, D. R., et al. (2018). Apathy in rapid eye movement sleep behaviour disorder is associated with serotonin depletion in the dorsal raphe nucleus. Brain 141, 2848–2854. doi: 10.1093/brain/awy240
Blaszczyk, J. W. (2016). Parkinson's disease and neurodegeneration: gaba-collapse hypothesis. Front. Neurosci. 10:269. doi: 10.3389/fnins.2016.00269
Boef, A. G., le Cessie, S., and Dekkers, O. M. (2015). Mendelian randomization studies in the elderly. Epidemiology 26, e15–e16. doi: 10.1097/EDE.0000000000000243
Bohnen, N. I., and Hu, M. (2019). Sleep disturbance as potential risk and progression factor for Parkinson's disease. J. Parkinsons Dis. 9, 603–614. doi: 10.3233/JPD-191627
Bowden, J., Davey, S. G., and Burgess, S. (2015). Mendelian randomization with invalid instruments: effect estimation and bias detection through egger regression. Int. J. Epidemiol. 44, 512–525. doi: 10.1093/ije/dyv080
Bowden, J., Davey, S. G., Haycock, P. C., and Burgess, S. (2016). Consistent estimation in mendelian randomization with some invalid instruments using a weighted median estimator. Genet. Epidemiol. 40, 304–314. doi: 10.1002/gepi.21965
Bower, J. H., Grossardt, B. R., Maraganore, D. M., Ahlskog, J. E., Colligan, R. C., Geda, Y. E., et al. (2010). Anxious personality predicts an increased risk of Parkinson's disease. Mov. Disord. 25, 2105–2113. doi: 10.1002/mds.23230
Burgess, S., Butterworth, A., and Thompson, S. G. (2013). Mendelian randomization analysis with multiple genetic variants using summarized data. Genet. Epidemiol. 37, 658–665. doi: 10.1002/gepi.21758
Burgess, S., Davies, N. M., and Thompson, S. G. (2016). Bias due to participant overlap in two-sample mendelian randomization. Genet. Epidemiol. 40, 597–608. doi: 10.1002/gepi.21998
Charney, A. W., Ruderfer, D. M., Stahl, E. A., Moran, J. L., Chambert, K., Belliveau, R. A., et al. (2017). Evidence for genetic heterogeneity between clinical subtypes of bipolar disorder. Transl. Psychiatry 7:e993. doi: 10.1038/tp.2016.242
Davies, N. M., Holmes, M. V., and Davey, S. G. (2018). Reading mendelian randomisation studies: a guide, glossary, and checklist for clinicians. BMJ 362:k601. doi: 10.1136/bmj.k601
Djamshidian, A., and Friedman, J. H. (2014). Anxiety and depression in parkinson's disease. Curr. Treat. Options Neurol. 16:285. doi: 10.1007/s11940-014-0285-6
D'Mello, C., and Swain, M. G. (2017). Immune-to-brain communication pathways in inflammation-associated sickness and depression. Curr. Top. Behav. Neurosci. 31, 73–94. doi: 10.1007/7854_2016_37
Dols, A., and Lemstra, A. W. (2020). Parkinsonism and bipolar disorder. Bipolar Disord. 22, 413–415. doi: 10.1111/bdi.12888
Elefante, C., Brancati, G. E., Bacciardi, S., Mazzucchi, S., Del, P. E., Palermo, G., et al. (2021). Prevalence and clinical correlates of comorbid anxiety and panic disorders in patients with Parkinson's disease. J. Clin. Med. 10:2302. doi: 10.3390/jcm10112302
Favier, M., Janickova, H., Justo, D., Kljakic, O., Runtz, L., Natsheh, J. Y., et al. (2020). Cholinergic dysfunction in the dorsal striatum promotes habit formation and maladaptive eating. J. Clin. Invest. 130, 6616–6630. doi: 10.1172/JCI138532
Ffytche, D. H., Creese, B., Politis, M., Chaudhuri, K. R., Weintraub, D., Ballard, C., et al. (2017). The psychosis spectrum in Parkinson disease. Nat. Rev. Neurol. 13, 81–95. doi: 10.1038/nrneurol.2016.200
Gao, S. F., and Bao, A. M. (2011). Corticotropin-releasing hormone, glutamate, and gamma-aminobutyric acid in depression. Neuroscientist 17, 124–144. doi: 10.1177/1073858410361780
Ghaddar, A., Fawaz, M., Khazen, G., Abdallah, J., and Milane, A. (2016). Prevalence of depression in Parkinson's disease in a Lebanese tertiary clinic. J. Clin. Exp. Neuropsychol. 38, 51–58. doi: 10.1080/13803395.2015.1087466
Gustafsson, H., Nordstrom, A., and Nordstrom, P. (2015). Depression and subsequent risk of Parkinson disease: a nationwide cohort study. Neurology 84, 2422–2429. doi: 10.1212/WNL.0000000000001684
Hamon, M., and Blier, P. (2013). Monoamine neurocircuitry in depression and strategies for new treatments. Prog. Neuro-Psychopharmacol. Biol. Psychiatry 45, 54–63. doi: 10.1016/j.pnpbp.2013.04.009
Hemani, G., Bowden, J., and Davey, S. G. (2018). Evaluating the potential role of pleiotropy in mendelian randomization studies. Hum. Mol. Genet. 27, R195–R208. doi: 10.1093/hmg/ddy163
Howard, D. M., Adams, M. J., Clarke, T. K., Hafferty, J. D., Gibson, J., Shirali, M., et al. (2019). Genome-wide meta-analysis of depression identifies 102 independent variants and highlights the importance of the prefrontal brain regions. Nat. Neurosci. 22, 343–352. doi: 10.1038/s41593-018-0326-7
Hsiao, Y. H., Chen, Y. T., Tseng, C. M., Wu, L. A., Perng, D. W., Chen, Y. M., et al. (2017). Sleep disorders and an increased risk of parkinson's disease in individuals with non-apnea sleep disorders: a population-based cohort study. J. Sleep Res. 26, 623–628. doi: 10.1111/jsr.12545
Huang, M. H., Cheng, C. M., Huang, K. L., Hsu, J. W., Bai, Y. M., Su, T. P., et al. (2019). Bipolar disorder and risk of Parkinson disease: a nationwide longitudinal study. Neurology 92, e2735–e2742. doi: 10.1212/WNL.0000000000007649
Huang, P., Lou, Y., Xuan, M., Gu, Q., Guan, X., Xu, X., et al. (2016). Cortical abnormalities in Parkinson's disease patients and relationship to depression: a surface-based morphometry study. Psychiatry Res. Neuroimaging 250, 24–28. doi: 10.1016/j.pscychresns.2016.03.002
International Obsessive Compulsive Disorder Foundation Genetics Collaborative (LOCDF-GC) and OCD Collaborative Genetics Association Studies (OCGAS) (2018). Revealing the complex genetic architecture of obsessive-compulsive disorder using meta-analysis. Mol. Psychiatry 23, 1181–1188. doi: 10.1038/mp.2017.154
Ishihara-Paul, L., Wainwright, N. W., Khaw, K. T., Luben, R. N., Welch, A. A., Day, N. E., et al. (2008). Prospective association between emotional health and clinical evidence of Parkinson's disease. Eur. J. Neurol. 15, 1148–1154. doi: 10.1111/j.1468-1331.2008.02299.x
Jacob, E. L., Gatto, N. M., Thompson, A., Bordelon, Y., and Ritz, B. (2010). Occurrence of depression and anxiety prior to Parkinson's disease. Parkinsonism Relat. Disord. 16, 576–581. doi: 10.1016/j.parkreldis.2010.06.014
Kamat, M. A., Blackshaw, J. A., Young, R., Surendran, P., Burgess, S., Danesh, J., et al. (2019). Phenoscanner v2: an expanded tool for searching human genotype-phenotype associations. Bioinformatics 35, 4851–4853. doi: 10.1093/bioinformatics/btz469
Karlsen, K. H., Tandberg, E., Arsland, D., and Larsen, J. P. (2000). Health related quality of life in Parkinson's disease: a prospective longitudinal study. J. Neurol. Neurosurg. Psychiatry 69, 584–589. doi: 10.1136/jnnp.69.5.584
Kim, H. S., Cheon, S. M., Seo, J. W., Ryu, H. J., Park, K. W., and Kim, J. W. (2013). Nonmotor symptoms more closely related to Parkinson's disease: comparison with normal elderly. J. Neurol. Sci. 324, 70–73. doi: 10.1016/j.jns.2012.10.004
Krishnan, S., Sarma, G., Sarma, S., and Kishore, A. (2011). Do nonmotor symptoms in Parkinson's disease differ from normal aging? Mov. Disord. 26, 2110–2113. doi: 10.1002/mds.23826
Kurki, M. I., Karjalainen, J., Palta, P., Sipilä, T. P., Kristiansson, K., Donner, K., et al. (2023). Author correction: Finngen provides genetic insights from a well-phenotyped isolated population. Nature. doi: 10.1038/s41586-023-05837-8
Kuusimaki, T., Al-Abdulrasul, H., Kurki, S., Hietala, J., Hartikainen, S., Koponen, M., et al. (2021). Increased risk of Parkinson's disease in patients with schizophrenia spectrum disorders. Mov. Disord. 36, 1353–1361. doi: 10.1002/mds.28484
Lees, A. J., Hardy, J., and Revesz, T. (2009). Parkinson's disease. Lancet 373, 2055–2066. doi: 10.1016/S0140-6736(09)60492-X
Li, K. L., Huang, H. Y., Ren, H., and Yang, X. L. (2022). Role of exosomes in the pathogenesis of inflammation in Parkinson's disease. Neural Regen. Res. 17, 1898–1906. doi: 10.4103/1673-5374.335143
Lin, H. L., Lin, H. C., and Chen, Y. H. (2014). Psychiatric diseases predated the occurrence of Parkinson disease: a retrospective cohort study. Ann. Epidemiol. 24, 206–213. doi: 10.1016/j.annepidem.2013.12.010
Lin, C. H., Lin, J. W., Liu, Y. C., Chang, C. H., and Wu, R. M. (2015). Risk of Parkinson's disease following anxiety disorders: a nationwide population-based cohort study. Eur. J. Neurol. 22, 1280–1287. doi: 10.1111/ene.12740
Liou, Y. J., Bai, Y. M., Chen, M. H., Cheng, C. M., Chen, T. J., and Tsai, S. J. (2022). Obsessive-compulsive disorder and risk of Parkinson disease: a nationwide longitudinal study. Psychosom. Med. 84, 1096–1102. doi: 10.1097/PSY.0000000000001120
Liu, W., Jiang, X., Deng, Z., Jia, L., Sun, Q., Kong, L., et al. (2022). Altered dynamic amplitude of low-frequency fluctuation between bipolar type i and type ii in the depressive state. Neuroimage Clin 36:103184. doi: 10.1016/j.nicl.2022.103184
Maier, F., Merkl, J., Ellereit, A. L., Lewis, C. J., Eggers, C., Pedrosa, D. J., et al. (2014). Hypomania and mania related to dopamine replacement therapy in Parkinson's disease. Parkinsonism Relat. Disord. 20, 421–427. doi: 10.1016/j.parkreldis.2014.01.001
Minelli, C., Del, G. M. F., van der Plaat, D. A., Bowden, J., Sheehan, N. A., and Thompson, J. (2021). The use of two-sample methods for mendelian randomization analyses on single large datasets. Int. J. Epidemiol. 50, 1651–1659. doi: 10.1093/ije/dyab084
Mullins, N., Forstner, A. J., O'Connell, K. S., Coombes, B., Coleman, J., Qiao, Z., et al. (2021). Genome-wide association study of more than 40,000 bipolar disorder cases provides new insights into the underlying biology. Nat. Genet. 53, 817–829. doi: 10.1038/s41588-021-00857-4
Nagel, M., Jansen, P. R., Stringer, S., Watanabe, K., de Leeuw, C. A., Bryois, J., et al. (2018). Meta-analysis of genome-wide association studies for neuroticism in 449,484 individuals identifies novel genetic loci and pathways. Nat. Genet. 50, 920–927. doi: 10.1038/s41588-018-0151-7
Nalls, M. A., Blauwendraat, C., Vallerga, C. L., Heilbron, K., Bandres-Ciga, S., Chang, D., et al. (2019). Identification of novel risk loci, causal insights, and heritable risk for Parkinson's disease: a meta-analysis of genome-wide association studies. Lancet Neurol. 18, 1091–1102. doi: 10.1016/S1474-4422(19)30320-5
Ng, A., Chander, R. J., Tan, L. C., and Kandiah, N. (2015). Influence of depression in mild Parkinson's disease on longitudinal motor and cognitive function. Parkinsonism Relat. Disord. 21, 1056–1060. doi: 10.1016/j.parkreldis.2015.06.014
Perry, D. C., Sturm, V. E., Peterson, M. J., Pieper, C. F., Bullock, T., Boeve, B. F., et al. (2016). Association of traumatic brain injury with subsequent neurological and psychiatric disease: a meta-analysis. J. Neurosurg. 124, 511–526. doi: 10.3171/2015.2.JNS14503
Plana-Ripoll, O., Pedersen, C. B., Agerbo, E., Holtz, Y., Erlangsen, A., Canudas-Romo, V., et al. (2019). A comprehensive analysis of mortality-related health metrics associated with mental disorders: a nationwide, register-based cohort study. Lancet 394, 1827–1835. doi: 10.1016/S0140-6736(19)32316-5
Plotnikov, D., and Guggenheim, J. A. (2019). Mendelian randomisation and the goal of inferring causation from observational studies in the vision sciences. Ophthalmic Physiol. Opt. 39, 11–25. doi: 10.1111/opo.12596
Pontone, G. M., and Koch, G. (2019). An association between bipolar disorder and Parkinson disease: when mood makes you move. Neurology 92, 1125–1126. doi: 10.1212/WNL.0000000000007641
Purves, K. L., Coleman, J., Meier, S. M., Rayner, C., Davis, K., Cheesman, R., et al. (2020). A major role for common genetic variation in anxiety disorders. Mol. Psychiatry 25, 3292–3303. doi: 10.1038/s41380-019-0559-1
Roessner, V., Bleich, S., Banaschewski, T., and Rothenberger, A. (2005). Olfactory deficits in anorexia nervosa. Eur. Arch. Psychiatry Clin. Neurosci. 255, 6–9. doi: 10.1007/s00406-004-0525-y
Saiki, S. (2014). The association of Parkinson's disease pathogenesis with inflammation. Rinsho Shinkeigaku 54, 1125–1127. doi: 10.5692/clinicalneurol.54.1125
Sanyal, J., Chakraborty, D. P., Sarkar, B., Banerjee, T. K., Mukherjee, S. C., Ray, B. C., et al. (2010). Environmental and familial risk factors of Parkinsons disease: case-control study. Can. J. Neurol. Sci. 37, 637–642. doi: 10.1017/s0317167100010829
Schrag, A., Horsfall, L., Walters, K., Noyce, A., and Petersen, I. (2015). Prediagnostic presentations of Parkinson's disease in primary care: a case-control study. Lancet Neurol. 14, 57–64. doi: 10.1016/S1474-4422(14)70287-X
Schreder, T., Albrecht, J., Kleemann, A. M., Schopf, V., Kopietz, R., Anzinger, A., et al. (2008). Olfactory performance of patients with anorexia nervosa and healthy subjects in hunger and satiety. Rhinology 46, 175–183.
Sekar, M. K., Arcelus, J., and Palmer, R. L. (2010). Micrographia and hypophonia in anorexia nervosa. Int. J. Eat. Disord. 43, 762–765. doi: 10.1002/eat.20768
Stievenard, A., Mequinion, M., Andrews, Z. B., Destee, A., Chartier-Harlin, M. C., Viltart, O., et al. (2017). Is there a role for ghrelin in central dopaminergic systems? Focus on nigrostriatal and mesocorticolimbic pathways. Neurosci. Biobehav. Rev. 73, 255–275. doi: 10.1016/j.neubiorev.2016.11.021
Temel, Y., Kessels, A., Tan, S., Topdag, A., Boon, P., and Visser-Vandewalle, V. (2006). Behavioural changes after bilateral subthalamic stimulation in advanced Parkinson disease: a systematic review. Parkinsonism Relat. Disord. 12, 265–272. doi: 10.1016/j.parkreldis.2006.01.004
Terracciano, A., Aschwanden, D., Stephan, Y., Cerasa, A., Passamonti, L., Toschi, N., et al. (2021). Neuroticism and risk of Parkinson's disease: a meta-analysis. Mov. Disord. 36, 1863–1870. doi: 10.1002/mds.28575
Trubetskoy, V., Pardinas, A. F., Qi, T., Panagiotaropoulou, G., Awasthi, S., Bigdeli, T. B., et al. (2022). Mapping genomic loci implicates genes and synaptic biology in schizophrenia. Nature 604, 502–508. doi: 10.1038/s41586-022-04434-5
Vansteelandt, S., Dukes, O., and Martinussen, T. (2018). Survivor bias in mendelian randomization analysis. Biostatistics 19, 426–443. doi: 10.1093/biostatistics/kxx050
Verbanck, M., Chen, C. Y., Neale, B., and Do, R. (2018). Detection of widespread horizontal pleiotropy in causal relationships inferred from mendelian randomization between complex traits and diseases. Nat. Genet. 50, 693–698. doi: 10.1038/s41588-018-0099-7
Vieta, E., Berk, M., Schulze, T. G., Carvalho, A. F., Suppes, T., Calabrese, J. R., et al. (2018). Bipolar disorders. Nat. Rev. Dis. Primers. 4:18008. doi: 10.1038/nrdp.2018.8
von Campenhausen, S., Bornschein, B., Wick, R., Botzel, K., Sampaio, C., Poewe, W., et al. (2005). Prevalence and incidence of parkinson's disease in europe. Eur. Neuropsychopharmacol. 15, 473–490. doi: 10.1016/j.euroneuro.2005.04.007
Wang, S., Mao, S., Xiang, D., and Fang, C. (2018). Association between depression and the subsequent risk of parkinson's disease: a meta-analysis. Prog. Neuro-Psychopharmacol. Biol. Psychiatry 86, 186–192. doi: 10.1016/j.pnpbp.2018.05.025
Watanabe, K., Jansen, P. R., Savage, J. E., Nandakumar, P., Wang, X., Hinds, D. A., et al. (2022). Genome-wide meta-analysis of insomnia prioritizes genes associated with metabolic and psychiatric pathways. Nat. Genet. 54, 1125–1132. doi: 10.1038/s41588-022-01124-w
Watson, H. J., Yilmaz, Z., Thornton, L. M., Hubel, C., Coleman, J., Gaspar, H. A., et al. (2019). Genome-wide association study identifies eight risk loci and implicates metabo-psychiatric origins for anorexia nervosa. Nat. Genet. 51, 1207–1214. doi: 10.1038/s41588-019-0439-2
Weintraub, D., Chiang, C., Kim, H. M., Wilkinson, J., Marras, C., Stanislawski, B., et al. (2017). Antipsychotic use and physical morbidity in Parkinson disease. Am. J. Geriatr. Psychiatry 25, 697–705. doi: 10.1016/j.jagp.2017.01.076
Weisskopf, M. G., Chen, H., Schwarzschild, M. A., Kawachi, I., and Ascherio, A. (2003). Prospective study of phobic anxiety and risk of Parkinson's disease. Mov. Disord. 18, 646–651. doi: 10.1002/mds.10425
Zimmerman, M., Martinez, J. H., Morgan, T. A., Young, D., Chelminski, I., and Dalrymple, K. (2013). Distinguishing bipolar ii depression from major depressive disorder with comorbid borderline personality disorder: demographic, clinical, and family history differences. J. Clin. Psychiatry 74, 880–886. doi: 10.4088/JCP.13m08428
Keywords: Parkinson’s disease, psychiatric disorders, Mendelian randomization, genome-wide association studies, causal relationship
Citation: Wu Q, Liu S, Huang X, Liu J, Wang Y, Xiang Y, Tang X, Xu Q, Yan X, Tang B and Guo J (2023) Bidirectional Mendelian randomization study of psychiatric disorders and Parkinson’s disease. Front. Aging Neurosci. 15:1120615. doi: 10.3389/fnagi.2023.1120615
Received: 10 December 2022; Accepted: 21 February 2023;
Published: 14 March 2023.
Edited by:
Huaibin Cai, National Institute on Aging (NIH), United StatesReviewed by:
Zhonghua Hu, Central South University, ChinaCopyright © 2023 Wu, Liu, Huang, Liu, Wang, Xiang, Tang, Xu, Yan, Tang and Guo. This is an open-access article distributed under the terms of the Creative Commons Attribution License (CC BY). The use, distribution or reproduction in other forums is permitted, provided the original author(s) and the copyright owner(s) are credited and that the original publication in this journal is cited, in accordance with accepted academic practice. No use, distribution or reproduction is permitted which does not comply with these terms.
*Correspondence: Jifeng Guo, Z3VvamlmZW5nMjAwM0AxNjMuY29t
†These authors have contributed equally to this work
Disclaimer: All claims expressed in this article are solely those of the authors and do not necessarily represent those of their affiliated organizations, or those of the publisher, the editors and the reviewers. Any product that may be evaluated in this article or claim that may be made by its manufacturer is not guaranteed or endorsed by the publisher.
Research integrity at Frontiers
Learn more about the work of our research integrity team to safeguard the quality of each article we publish.