- 1Department of Orthopedics, The First Affiliated Hospital of Wenzhou Medical University, Wenzhou, China
- 2Department of Outpatient, The First Affiliated Hospital of Wenzhou Medical University, Wenzhou, China
Objective: The aim of this study was to examine whether osteoporosis (OP) is associated with Alzheimer’s disease-related cerebrospinal fluid (CSF) biomarkers and brain structures among older people.
Methods: From the Alzheimer’s disease Neuroimaging Initiative database, we grouped participants according to the OP status (OP+/OP−) and compared the Alzheimer’s disease (AD)-related CSF biomarker levels and the regional brain structural volumes between the two groups using multivariable models. These models were adjusted for covariates including age, education, gender, diagnosis of Alzheimer’s disease, and apolipoprotein E4 carrier status.
Results: In the cross-sectional analyses at baseline, OP was related to higher CSF t-tau (total tau) and p-tau181 (tau phosphorylated at threonine-181) but not to CSF amyloid-beta (1–42) or the volumes of entorhinal cortex and hippocampus. In the longitudinal analyses, OP was not associated with the change in the three CSF biomarkers over time but was linked to a faster decline in the size of the entorhinal cortex and hippocampus.
Conclusion: OP was associated with elevated levels of CSF t-tau and p-tau181 at baseline, and accelerated entorhinal cortex and hippocampal atrophies over time among older people.
Introduction
An increase in life expectancy causes the aging of the population. The growing older population is plagued by age-related diseases, typically Alzheimer’s disease (AD) and osteoporosis (OP). AD is a well-known neurodegenerative disease that causes cognitive dysfunction among older adults (Mangialasche et al., 2010). OP, on the contrary, is a skeletal disease characterized by low bone mineral density (BMD) and micro-architectural bone tissue deterioration (Black and Rosen, 2016). Although AD and OP appear to have nothing in common, we are not the first to suspect that they may have connections (Yaffe et al., 1999; Tan et al., 2005; Zhou et al., 2014; Sohrabi et al., 2015).
The potential connections have been implicated from a variety of perspectives. Experimental evidence supported that AD was linked to pathogenic changes in osteoporotic animal models (Li et al., 2013; Calabrese et al., 2016), while low BMD was a trait of certain AD mouse models (Xia et al., 2013; Dengler-Crish et al., 2017). According to epidemiological studies, low BMD increases the risk of AD, while OP affects nearly half of cognitively impaired patients (Ebrahimpur et al., 2020). Large-scale investigations focused on the relationship between the two chronic degenerative diseases were first reported by Yaffe et al. (1999) who found that women with osteoporosis have poorer cognitive functions and greater risks of cognitive deterioration. In Germany and South Korea, this relationship was further confirmed for both sexes (Kostev et al., 2018; Kwon et al., 2021). These studies indicated that AD and OP may share some central mechanisms. However, none of them have investigated the long-term pathological connections between OP and AD in living humans.
Pathological evidence has been widely valued in the diagnosis of AD. AD-related cerebrospinal fluid (CSF) biomarkers, including amyloid-beta (Aβ) protein, total tau (t-tau), and phosphorylated tau (p-tau), have been established as core indicators to define the progressive stage in the AD continuum (McKhann et al., 2011; Jack et al., 2016). The aberrant buildup of these pathological proteins will cause generalized atrophy of brain structures, typically affecting the entorhinal cortex and hippocampus (Callen et al., 2001; Killiany et al., 2002; Kril et al., 2002; Dengler-Crish et al., 2017). The degeneration of these brain structures, which are measurable on MRI, causes the progression of AD (Killiany et al., 2002).
Using the Alzheimer’s disease neuroimaging initiative (ADNI) database, this study aims to find out whether OP relates to AD-related CSF biomarkers and brain structures among older people.
Materials and methods
Alzheimer’s disease neuroimaging initiative
Raw data were pulled from the ADNI database.1 ADNI researchers collect, validate, and utilize data, including demographic characteristics, medical history, images, genetics, cognitive tests, CSF, and blood biomarkers, from participants who are adults aged 55–90 years with AD patients, mild cognitive impairment (MCI) subjects, and elderly normal controls (NC). The ADNI study was approved by the institutional review board at each ADNI center, and informed written consent was obtained from each participant. Further information is available at www.adni-info.org and in previous reports (Jack et al., 2010; Jagust et al., 2010; Petersen et al., 2010; Saykin et al., 2010; Weiner et al., 2010).
Participants
Alzheimer’s Disease Neuroimaging Initiative provides a systematic record of history for each participant (“RECMHIST.csv”), allowing researchers to extract terms using screening techniques. Our screening terms for OP included “OP” or “osteoporo (sis/tic),” which we used to group participants (OP+/OP−). Based on the information in ADNI data, 157 out of 2,292 participants were found to have OP at baseline, among whom, 102 out of 1,628 had complete covariate information and no history of depression, anxiety, malignant tumor, or stroke. Then, the data flow was split into two branches. One was CSF data (n = 717), which excluded participants with incomplete Aβ1–42, t-tau, and p-tau181 (tau phosphorylated at threonine-181) information. The other was MRI data (n = 992), which eliminated individuals with incomplete information about intracranial volume (ICV), entorhinal cortex volume (ECV), and hippocampal volume (HV). Besides, to conform the data to the demands of longitudinal analysis—that is, individuals must have at least one valid follow-up record in addition to the baseline one—345 participants were removed from the CSF data for the longitudinal analyses, whereas none were excluded from the MRI data. Figure 1 is the flowchart of the data processing.
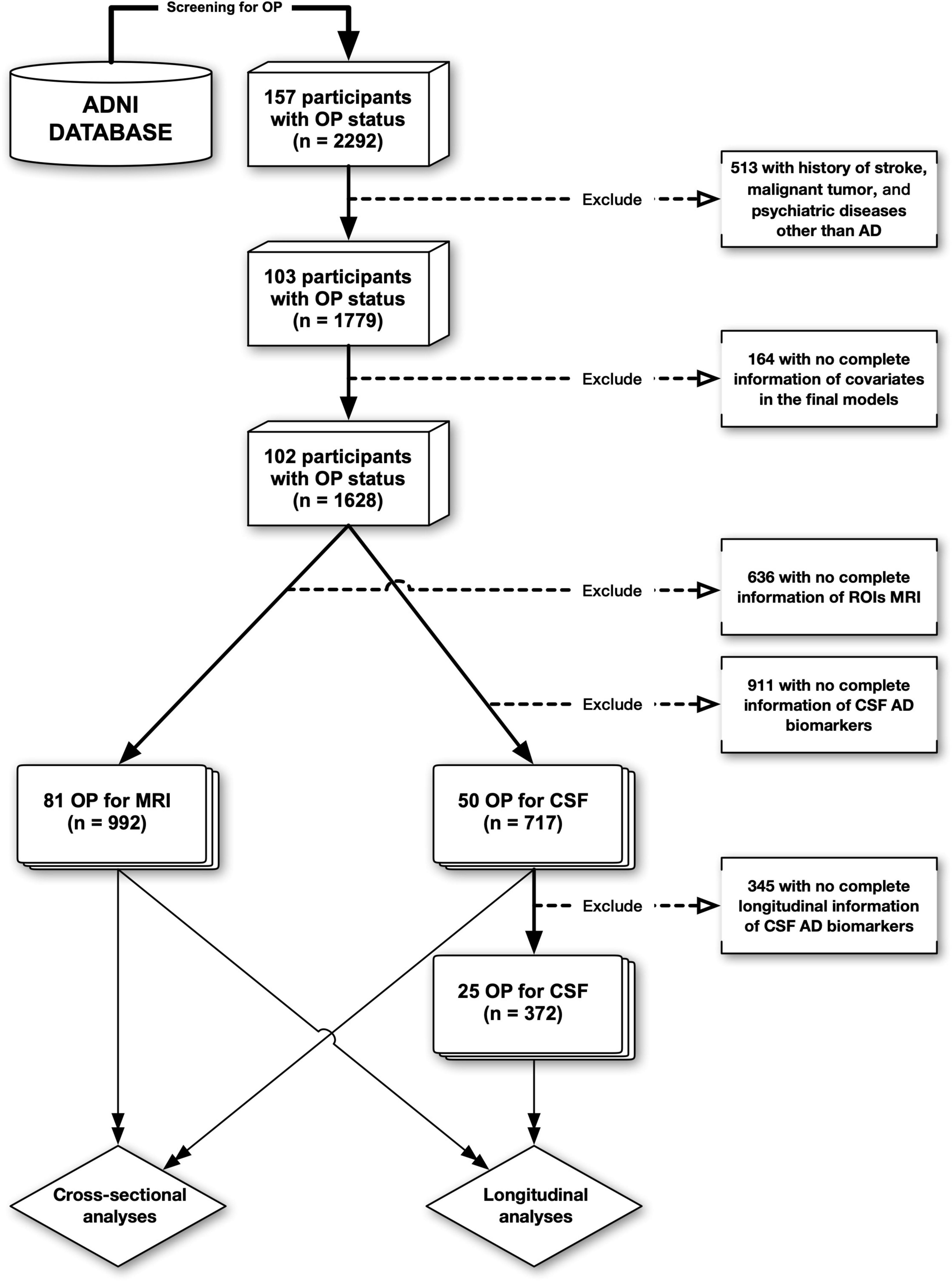
Figure 1. The flowchart of data processing. ADNI, Alzheimer’s disease neuroimaging initiative; OP, osteoporosis; AD, Alzheimer’s disease; CSF, cerebrospinal fluid.
Cerebrospinal fluid measurements
Cerebrospinal fluid Aβ1–42, t-tau, and p-tau181 were measured using the INNOBIA AlzBio3 immunoassay (Fujirebio, Belgium, pg/ml). To keep the within-batch precision values under 10% (5.1–7.8% for Aβ1–42, 4.4–9.8% for t-tau, and 5.1–8.8% for p-tau181), ADNI provided unified CSF collection and procedural protocols (Shaw et al., 2009). From the ADNI file (“ADNIMERGE.csv”), we set the measurements of these CSF biomarkers as response variables in our models of CSF data.
Magnetic resonance imaging measurements
Alzheimer’s disease neuroimaging initiative provides a set of standardized processes for MRI acquisition to reduce systematic error (Christensen et al., 1997; Hsu et al., 2002). The ECV, HV, and ICV were also extracted from the ADNI file (“ADNIMERGE.csv”). Both ECV and HV were normalized by ICV using a residual method to remove head size differences (Voevodskaya et al., 2014; Pintzka et al., 2015). In our models of MRI data, the response variables were ICV normalized ECV and HV (ECVn and HVn, mm3).
Statistical analyses
Demographic, cross-sectional, and longitudinal analyses were conducted on CSF and MRI data. Baseline demographic variables were compared between the OP− and OP+ groups (Chi-square tests for categorical variables; Student’s t-tests for normally distributed variables; and Mann–Whitney U-tests for non-normally distributed variables). The results of descriptive statistics are presented for the normally distributed variables as “mean [SD]” and for the non-normally distributed variables as “median [P25, P75]” (P25: 25th percentile; P75: 75th percentile).
In the cross-sectional analyses at baseline, we first investigated the associations between OP and CSF biomarkers (Aβ1–42, t-tau, and p-tau181) after adjusting for covariates including age, sex, education, apolipoprotein E4 (APOE4) carrier status, and diagnosis of AD. Next, we tested the differences in brain structures (ECVn and HVn) between the OP− and OP+ groups via linear regression models adjusted for identical covariates.
In the longitudinal analyses, we fitted linear mixed-effects models to characterize individual paths of change. These models had random intercepts and slopes for time and an unstructured covariance matrix for the random effects and included the interaction between (continuous) time and OP status as the predictor. These models were adjusted for the same covariates, including age, sex, education, APOE4 carrier status, and diagnosis of AD.
P-values <0.05 were considered to reject the null hypothesis. P-values in the linear regression models and linear mixed-effects models were calculated using the Satterthwaite’s degrees of freedom method (“lmerTest” R package), and the Holm’s method (also called the Holm–Bonferroni method) is used to counteract the problem of multiple comparisons. R software (version 4.2.0) and GraphPad Prism (version 9.3.1) were used for statistical analysis and visualization.
Results
Demographic variables at baseline
Cerebrospinal fluid biomarkers
In the CSF data, at baseline, the measurements of Aβ1–42, t-tau, and p-tau181 were available for 717 participants, of whom 50 had OP (154 [23.1%] NC, 383 [57.4%] MCI, and 130 [19.5%] AD in OP− group; 8 [16.0%] NC, 28 [56.0%] MCI, and 14 [28.0%] AD in OP + group; P = 0.256). Participants without OP were about 1 year younger than those with OP (73.9 [7.2] for OP−, 74.9 [7.0] for OP+; P = 0.382). Participants with OP were more likely to be female (42 [84.0%]) than those without OP (female 233 [34.9%]; P < 0.001). A similar APOE4 carrier rate was found between the two groups (345 [51.7%] for OP−; 26 [52.0%] for OP+; P = 1.000). Education years were approximately 6 months shorter for participants with OP (16.0 [14.0, 18.0] for OP−; 15.5 [13.0, 18.0] for OP+; P = 0.108). In short, only “sex” was found to be significantly different between the two groups.
We further compared the CSF Aβ1–42, t-tau, and p-tau181 between the two groups at baseline. The OP− group had a lower Aβ1–42 (763.1 [566.4, 1112.8], pg/ml) than the OP + group (792.2 [619.5, 989.8], pg/ml; P = 0.841). Both t-tau and p-tau181 were significantly lower in OP− group than OP + group (253.6 [186.6, 347.6] vs. 324.8 [237.2, 438.2] for t-tau, pg/ml; P = 0.003; 24.4 [16.6, 35.4] vs. 31.8 [21.8, 44.1] for p-tau181, pg/ml; P = 0.005).
Brain structures
In the MRI data, at baseline, the measurements of ECV, HV, and ICV were available for 992 participants, of whom 81 had OP (274 [30.1%] NC, 492 [54.0%] MCI, and 145 [15.9%] AD in OP− group; 20 [24.7%] NC, 44 [54.3%] MCI, and 17 [21.0%] AD in OP + group; P = 0.387). Participants with OP were roughly 2 years older than those without OP (73.7 [6.9] for OP−, 75.7 [7.0] for OP+; P = 0.013). Sex composition differed significantly between the two groups (female, OP−: 337 [37.0%] vs. OP+: 69 [85.2%]; P < 0.001). There was no significant difference in the APOE4 carrier rate between the two groups (423 [46.4%] for OP−; 36 [44.4%] for OP+; P = 0.820). Education years between the two groups were very close (OP−: 16.0 [14.0, 18.0] vs. OP+: 16.0 [14.0, 18.0]; P = 0.651). To sum up, “age” and “sex” were found to be significantly different between the two groups.
We further examined the ECVn and HVn between the two groups at baseline. Both ECVn and HVn were higher in the OP− group than in the OP + group (3,537.1 [756.2] vs. 3,375.8 [746.9] for ECVn, mm3; P = 0.066; 6,898.1 [1,145.8] vs. 6,558.3 [949.9] for HVn, mm3; P = 0.010). However, the difference was only significant for HVn.
Table 1 provides an overview of the baseline CSF and MRI data, in which the differences between the two groups are summarized.
Cross-sectional analyses at baseline
To evaluate the relationship between OP and AD at baseline, CSF biomarkers and brain structural volumes were adjusted for five covariates, including age, sex, education, APOE4 carrier status, and AD diagnosis via linear regression models. Briefly speaking, OP was significantly linked to a higher estimated average effect of CSF t-tau and p-tau181 at baseline (estimate = 39.4 pg/ml, 95% CI: 3.2–75.5 pg/ml, P = 0.033 for t-tau; estimate = 4.5 pg/ml, 95% CI: 0.4–8.5 pg/ml, P = 0.030 for p-tau181). ECVn was almost irrelevant with OP (estimate = 0.7 mm3, 95% CI: −156.2–157.6 mm3, P = 0.993; mm3). Baseline estimated average effect of CSF Aβ1–42 and HVn were slightly lower in OP + group, but not statistically significant (estimate = –5.9 pg/ml, 95% CI: −104.4–92.7 pg/ml, P = 0.907 for Aβ1–42; estimate = −77.1 mm3, 95% CI: −293.8–139.6 mm3, P = 0.485 for HVn). The simplified outcomes of these models are presented in Table 2 and are visualized in Figure 2. The detailed results (including covariates) are available in Supplementary Table 1.
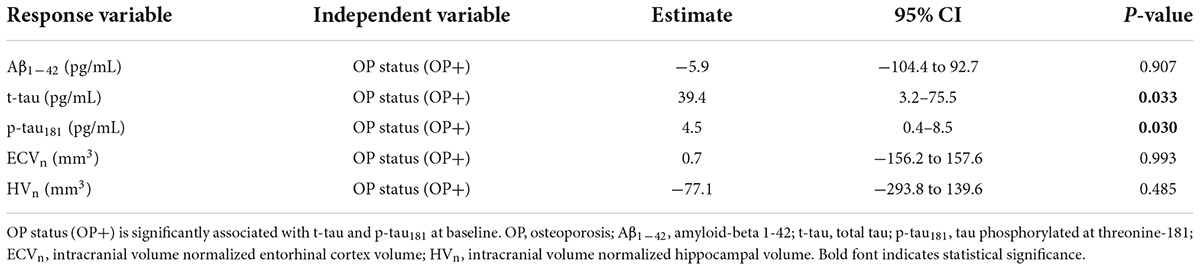
Table 2. Summary of linear regression models examining the association of OP status with the response variables.
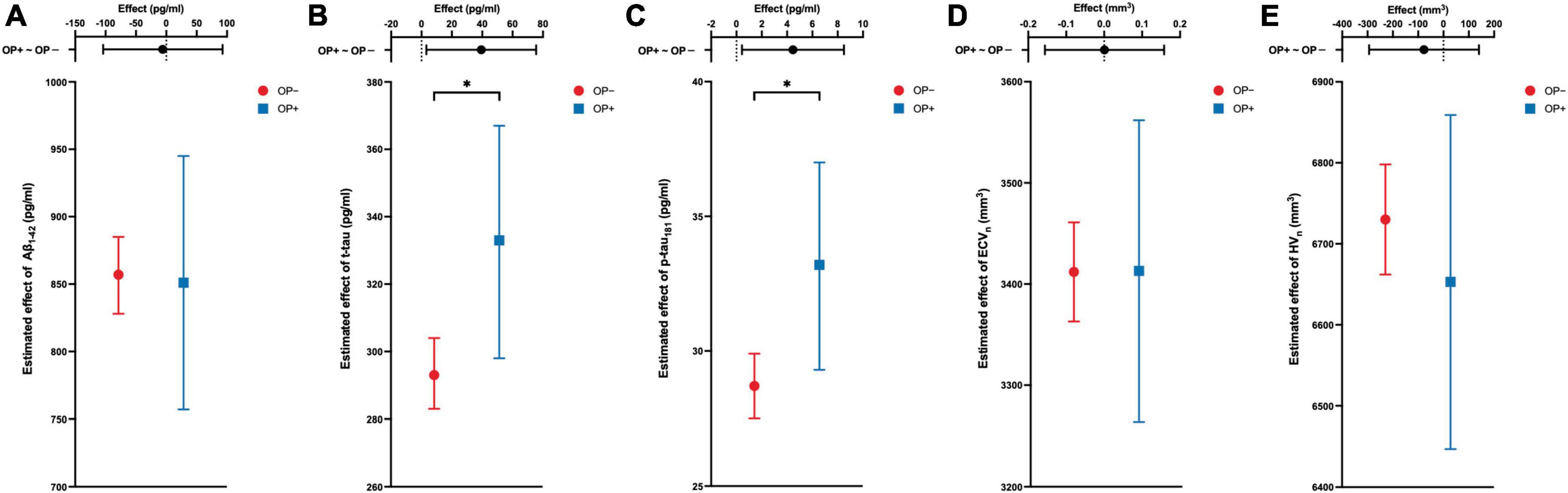
Figure 2. The cross-sectional associations between OP and the response variable of the model. Estimated average effects with 95% CI error bars are demonstrated on each panel. The upper part of each panel represents the contrast (mean of OP + group minus mean of OP– group) with a 95% CI error bar. OP is associated with cerebrospinal fluid levels of t-tau and p-tau181 (B,C), but not associated with the Aβ1–42 (A), ECVn (D), and HVn (E). All analyses are adjusted for age, gender, education, APOE4 carrier status, and diagnosis of Alzheimer’s disease (AD). *P-value <0.05. OP, osteoporosis; Aβ1–42, amyloid-beta 1-42; t-tau, total tau; p-tau181, tau phosphorylated at threonine-181; ECVn, intracranial volume normalized entorhinal cortex volume; HVn, intracranial volume normalized hippocampal volume.
Longitudinal analyses
After completing the cross-sectional analyses, we investigated how OP affected the changes in CSF biomarkers and brain structural volumes over time. Unlike the cross-sectional results, CSF biomarkers were not associated with OP status over time (Table 3). By contrast, compared with individuals without OP, those with OP showed significantly faster declines in both ECVn and HVn (estimate = −37 mm3, 95% CI: –68.3 to –5.6 mm3, P = 0.021 for ECVn on interaction of OP and time [OP+: time], Figure 3A; estimate = −42.5 mm3, 95% CI: −71.1 to –13.8 mm3, P = 0.004 for HVn on interaction of OP and time [OP+: time], Figure 3B). Detailed longitudinal results (including covariates) are provided in Supplementary Table 2.
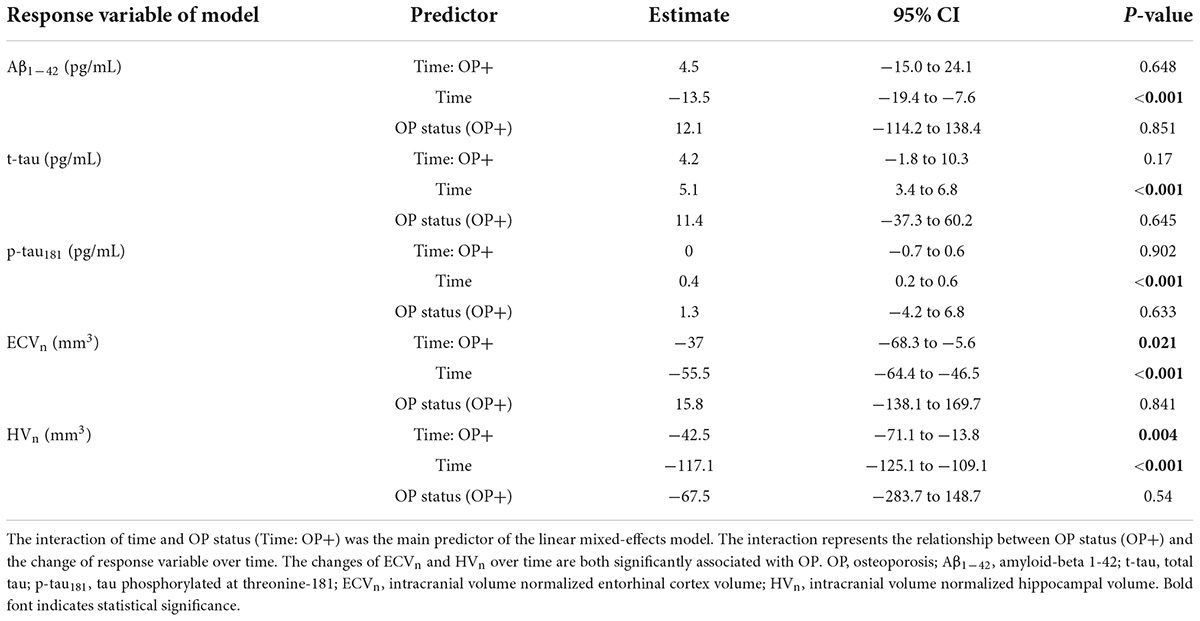
Table 3. Summary of linear mixed-effects models examining the association of OP status with changes in response variables over time.
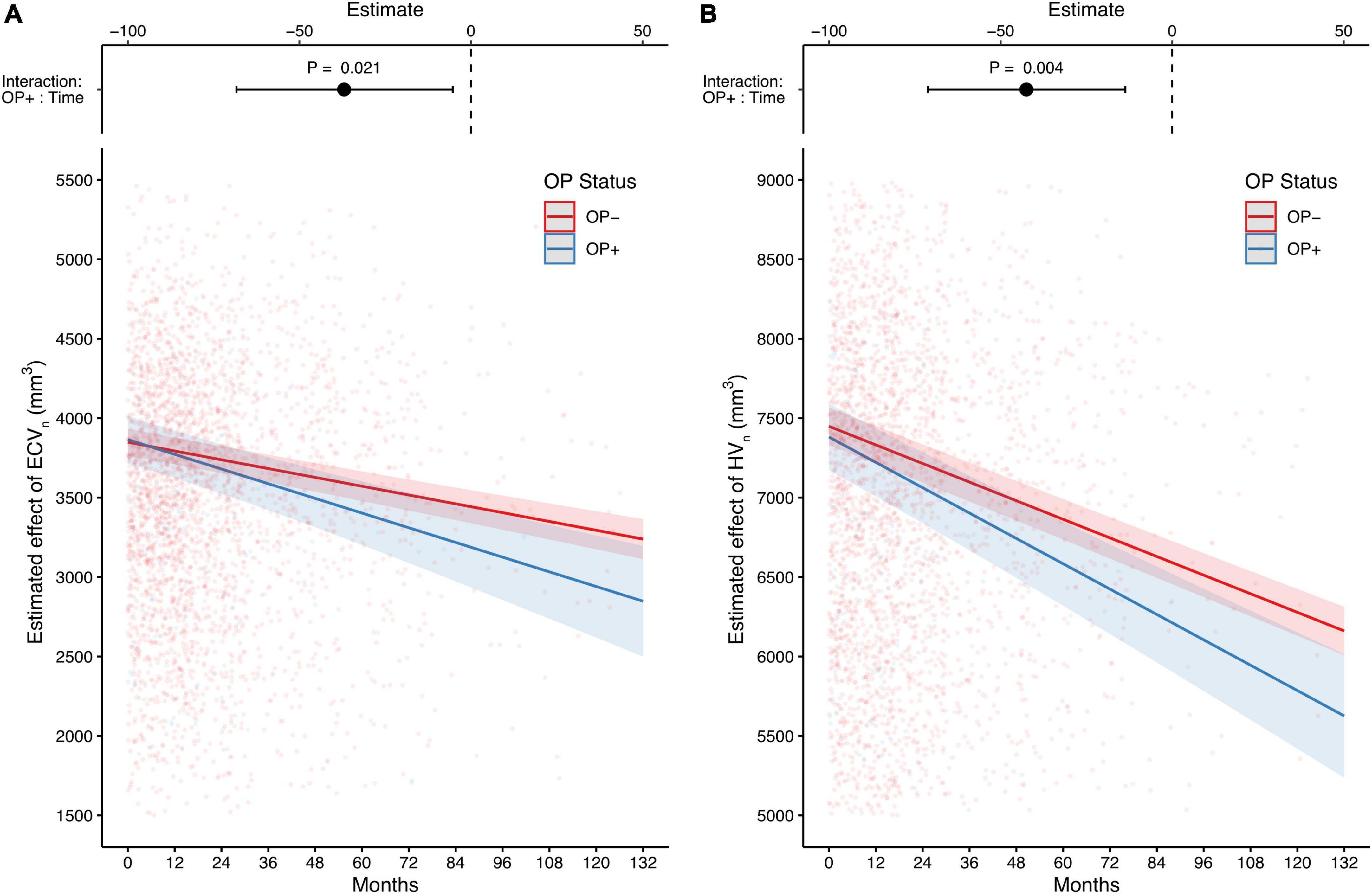
Figure 3. The long-term effects of OP on entorhinal cortex and hippocampus MRI-based measurements. Compared with individuals without OP, those with OP show a faster decline in both the estimated effect of ECVn and HVn (A,B). The upper part of each panel represents the contrast of the predictor (the interaction of OP+ and time; mean of OP + group minus mean of OP– group) with a 95% CI error bar. Both analyses are adjusted for age, gender, education, APOE4 carrier status, and diagnosis of Alzheimer’s disease (AD). OP, osteoporosis; ECVn, intracranial volume normalized entorhinal cortex volume; HVn, intracranial volume normalized hippocampal volume.
In addition, as “loss to follow-up” aggravated with time in both groups, a series of sensitivity tests were conducted on the data with follow-up cutoffs from 24 to 132 (maximum) months. We found no decisive differences in outcomes when the follow-up cutoff time exceeded 48 months. For each time point, the numbers of follow-up visits are listed, along with the P-values of sensitivity tests (Supplementary Table 3, full results not shown).
Discussion
Alzheimer’s Disease Neuroimaging Initiative is a historic study of brain aging that aims to accelerate discovery in the race to prevent, treat, and eventually cure AD. For more than a decade, ADNI researchers have been working to better understand AD. In addition to studying AD itself, many researchers, including ourselves, are interested in investigating the links between AD and other diseases, such as osteoarthritis (Li et al., 2020), Parkinson’s disease (Wang et al., 2018), hearing loss (Xu et al., 2019), hypertension (Scott et al., 2015; Zhou et al., 2020), and hypercholesterolemia (Varma et al., 2021), from the ADNI database. To the best of our knowledge, this is the first study to demonstrate the links between OP and higher levels of CSF t-tau and p-tau181 at baseline, and the faster volumetric declines of enterhinal cortex and hippocampus over time among older people.
Our findings are consistent with previous studies on tau pathology. Histopathological data showed a significantly reduced BMD phenotype was found in the htau mouse models (Dengler-Crish et al., 2017). Dengler-Crish et al. (2018) further reported that the BMD reduction occurred before the presence of significant tauopathy in the hippocampus, which can be implied that OP status may risk neurodegeneration via promoting tau pathology. Tauopathy could induce cognitive impairment across the AD spectrum via synaptic dysfunction and neuronal loss (Andorfer et al., 2003; Polydoro et al., 2009; Di et al., 2016), independent of amyloid pathology (Bejanin et al., 2017). Notably, the weak links we found between OP and the buildup of CSF t-tau and p-tau181 over time were inconsistent with Dengler-Crish’s study (Dengler-Crish et al., 2018). These conflicts may be partially explained by sample removal for longitudinal CSF data analyses, which is reasonable given that the CSF collection is invasive and the subjects are real humans. Still, it is hoped that future research will provide more comprehensive longitudinal data to validate our results.
Interestingly, subjects with OP began with comparable baseline volumes as those without OP and experienced a significantly faster decline in both ECVn and HVn over time. In addition, sensitivity tests’ outcomes barely changed when they were tracked for more than 48 months. The possible explanations are as follows: (1) OP might play different roles in different stages of the AD continuum; (2) the individual variability of ECVn and HVn at baseline was low; and (3) the process of brain structural atrophy caused by abnormal accumulation of CSF pathological proteins takes a long time (Killiany et al., 2002; Kril et al., 2002; Dengler-Crish et al., 2018). These explanations merit further studies.
There are several possible molecular biological explanations for our findings. Currently known, pathways that OP and AD may share include RANK-RANKL (Luckhaus et al., 2009; Stapledon et al., 2021), vitamin D receptor (Morello et al., 2018; Jia et al., 2019), C/EBPβ-δ-secretase (Xiong et al., 2022), PI3K-Akt (Fehsel and Christl, 2022), and Wnt/β-catenin (Li et al., 2013; Dengler-Crish et al., 2018; Folke et al., 2019), among which Wnt/β-catenin had the most attention. Wnt/β-catenin signaling is known to facilitate bone formation in bone tissue and to promote synaptogenesis in the brain. Likewise, pathological inhibition of this pathway has been implicated in both OP and AD pathogenesis, albeit in separate contexts (Dengler-Crish and Elefteriou, 2019). More importantly, Wnt deficiency was detectable in bone prior to the brain in the htau mouse model (Dengler-Crish et al., 2018), which may help explain our findings and emphasize the necessity of OP screening in AD-susceptible populations. Aside from Wnt/β-catenin signaling, RANK-RANKL signaling is another pathway shared by both OP and AD. According to Li et al. (2016) Aβ enhances RANKL-induced osteoclast activation and function, implying that Aβ is involved in the pathogenesis of OP at molecular levels in osteoclasts. Furthermore, the link between vitamin D deficiency and AD is gaining attention. Vitamin D supplementation improved cognitive function in both the AD mouse model (Morello et al., 2018) and in a randomized, double-blind, and placebo-controlled trial in which Aβ-related biomarkers were found to be lower in elderly patients with AD (Jia et al., 2019). Our findings, however, did not support these Aβ-related pathophysiological explanations. These discrepancies may be partially explained by differences in Aβ distribution and accumulation between the central nervous system and peripheral tissues (Li et al., 2014; Roberts et al., 2014; Ristori et al., 2020). More studies are needed to elucidate the potential mechanisms through which OP acts in AD.
Our study has certain limitations. First, the attrition bias due to loss to follow-up is not corrected in the analyses. Furthermore, the sample size is not balanced between the two groups. Future studies with larger sample size, longer follow-up duration, and lower attrition rates might provide more powerful evidence to support our findings. Second, the ADNI database does not contain adequate information about OP, such as the time OP started, lab evidence, or imaging evidence, for every participant with OP or without OP. This could lead to some wrong classifications. Even so, this is a common limitation of database-based research (Norton et al., 2014; Frain et al., 2017; Xu et al., 2019; Harshfield et al., 2020; Li et al., 2020; Kwon et al., 2021). Future studies are encouraged to extend our work to other databases to verify our results. Third, the associations we discovered can reflect but not represent causal relationships. Direct evidence of causal relationships may be provided by future research focusing on the protective effect of anti-osteoporosis drugs, such as bisphosphonates, on AD development. Fourth, although we excluded individuals with psychiatric conditions other than AD in the present analyses, we still cannot exclude the influence of other potential confounders, such as social isolation, eating disorder, or addictive behavior. Moreover, our models included five covariates. Other potential covariates (e.g., hypertension and hyperlipemia) are not included since they are recorded either binomially (without specific values) or merely once. Adding them to our models may not improve accuracy but will increase complexity and inefficiency (Zhang, 2014). Future research can be devoted to adding more valuable covariates, but this will need better database support.
Conclusion
This study identified the cross-sectional and longitudinal association between OP and the known pathological features of AD. Our findings suggest that OP’s neurodegenerative effects may be driven by elevated baseline CSF t-tau and p-tau levels, and the accelerated entorhinal cortex and hippocampal atrophy among older adults, providing critical insight into the neuropathological mechanisms by which OP increases the risk of developing AD. Furthermore, our results suggest that preventing or managing OP during the preclinical and prodromal stages of AD may be effective in combating neurodegeneration among older people.
Data availability statement
The original contributions presented in this study are included in the article/Supplementary material, further inquiries can be directed to the corresponding author/s.
Ethics statement
The studies involving human participants were reviewed and approved by Alzheimer’s Disease Neuroimaging Initiative. The patients/participants provided their written informed consent to participate in this study.
Author contributions
YL designed and supervised the study. HP, JC, CW, PW, JL, and FH performed the research, analyzed the data, and wrote the manuscript. All authors contributed to the article and approved the submitted version.
Funding
This study was partially supported by the Natural Science Foundation of Zhejiang Province (LGF19H070004) and the Wenzhou Science and Technology Bureau (Y20210418, Y20210435, and Y20190275). Data collection and sharing for this project was funded by the Alzheimer’s Disease Neuroimaging Initiative (ADNI) (National Institutes of Health Grant U01 AG024904) and DOD ADNI (Department of Defense award number: W81XWH-12-2-0012). ADNI was funded by the National Institute on Aging, the National Institute of Biomedical Imaging and Bioengineering, and through generous contributions from the following: AbbVie, Alzheimer’s Association; Alzheimer’s Drug Discovery Foundation; Araclon Biotech; BioClinica, Inc.; Biogen; Bristol-Myers Squibb Company; CereSpir, Inc.; Cogstate; Eisai Inc.; Elan Pharmaceuticals, Inc.; Eli Lilly and Company; Euroimmun; F. Hoffmann-La Roche Ltd. and its affiliated company Genentech, Inc.; Fujirebio; GE Healthcare; IXICO Ltd.; Janssen Alzheimer Immunotherapy Research & Development, LLC.; Johnson & Johnson Pharmaceutical Research & Development LLC.; Lumosity; Lundbeck; Merck & Co., Inc.; Meso Scale Diagnostics, LLC.; NeuroRx Research; Neurotrack Technologies; Novartis Pharmaceuticals Corporation; Pfizer Inc.; Piramal Imaging; Servier; Takeda Pharmaceutical Company; and Transition Therapeutics. The Canadian Institutes of Health Research is providing funds to support ADNI clinical sites in Canada. Private sector contributions are facilitated by the Foundation for the National Institutes of Health (www.fnih.org). The grantee organization is the Northern California Institute for Research and Education, and the study is coordinated by the Alzheimer’s Therapeutic Research Institute at the University of Southern California. ADNI data are disseminated by the Laboratory for Neuro Imaging at the University of Southern California.
Conflict of interest
The authors declare that the research was conducted in the absence of any commercial or financial relationships that could be construed as a potential conflict of interest.
Publisher’s note
All claims expressed in this article are solely those of the authors and do not necessarily represent those of their affiliated organizations, or those of the publisher, the editors and the reviewers. Any product that may be evaluated in this article, or claim that may be made by its manufacturer, is not guaranteed or endorsed by the publisher.
Supplementary Material
The Supplementary Material for this article can be found online at: https://www.frontiersin.org/articles/10.3389/fnagi.2022.958050/full#supplementary-material
Footnotes
References
Andorfer, C., Kress, Y., Espinoza, M., De Silva, R., Tucker, K. L., Barde, Y.-A., et al. (2003). Hyperphosphorylation and aggregation of tau in mice expressing normal human tau isoforms. J. Neurochem. 86, 582–590. doi: 10.1046/j.1471-4159.2003.01879.x
Bejanin, A., Schonhaut, D. R., La Joie, R., Kramer, J. H., Baker, S. L., Sosa, N., et al. (2017). Tau pathology and neurodegeneration contribute to cognitive impairment in Alzheimer’s disease. Brain 140, 3286–3300. doi: 10.1093/brain/awx243
Black, D. M., and Rosen, C. J. (2016). Postmenopausal osteoporosis. N. Engl. J. Med. 374, 254–262. doi: 10.1056/NEJMcp1513724
Calabrese, G., Mesner, L. D., Foley, P. L., Rosen, C. J., and Farber, C. R. (2016). Network analysis implicates alpha-synuclein (Snca) in the regulation of ovariectomy-induced bone loss. Sci. Rep. 6:29475. doi: 10.1038/srep29475
Callen, D. J. A., Black, S. E., Gao, F., Caldwell, C. B., and Szalai, J. P. (2001). Beyond the hippocampus: MRI volumetry confirms widespread limbic atrophy in AD. Neurology 57, 1669–1674. doi: 10.1212/WNL.57.9.1669
Christensen, G. E., Joshi, S. C., and Miller, M. I. (1997). Volumetric transformation of brain anatomy. IEEE Trans. Med. Imaging 16, 864–877. doi: 10.1109/42.650882
Dengler-Crish, C. M., and Elefteriou, F. (2019). Shared mechanisms: Osteoporosis and Alzheimer’s disease? Aging 11, 1317–1318. doi: 10.18632/aging.101828
Dengler-Crish, C. M., Ball, H. C., Lin, L., Novak, K. M., and Cooper, L. N. (2018). Evidence of Wnt/β-catenin alterations in brain and bone of a tauopathy mouse model of Alzheimer’s disease. Neurobiol. Aging 67, 148–158. doi: 10.1016/j.neurobiolaging.2018.03.021
Dengler-Crish, C. M., Smith, M. A., and Wilson, G. N. (2017). Early evidence of low bone density and decreased serotonergic synthesis in the dorsal raphe of a tauopathy model of Alzheimer’s disease. J. Alzheimers Dis. 55, 1605–1619. doi: 10.3233/jad-160658
Di, J., Cohen, L. S., Corbo, C. P., Phillips, G. R., El Idrissi, A., and Alonso, A. D. (2016). Abnormal tau induces cognitive impairment through two different mechanisms: Synaptic dysfunction and neuronal loss. Sci. Rep. 6:20833. doi: 10.1038/srep20833
Ebrahimpur, M., Sharifi, F., Shadman, Z., Payab, M., Mehraban, S., Shafiee, G., et al. (2020). Osteoporosis and cognitive impairment interwoven warning signs: Community-based study on older adults—Bushehr Elderly Health (BEH) Program. Arch. Osteoporos. 15:140. doi: 10.1007/s11657-020-00817-1
Fehsel, K., and Christl, J. (2022). Comorbidity of osteoporosis and Alzheimer’s disease: Is ‘AKT ‘-ing on cellular glucose uptake the missing link? Ageing Res. Rev. 76:101592. doi: 10.1016/j.arr.2022.101592
Folke, J., Pakkenberg, B., and Brudek, T. (2019). Impaired Wnt signaling in the prefrontal cortex of Alzheimer’s disease. Mol. Neurobiol. 56, 873–891. doi: 10.1007/s12035-018-1103-z
Frain, L., Swanson, D., Cho, K., Gagnon, D., Lu, K. P., Betensky, R. A., et al. (2017). Association of cancer and Alzheimer’s disease risk in a national cohort of veterans. Alzheimers Dement. 13, 1364–1370. doi: 10.1016/j.jalz.2017.04.012
Harshfield, E. L., Pennells, L., Schwartz, J. E., Willeit, P., Kaptoge, S., Bell, S., et al. (2020). Association between depressive symptoms and incident cardiovascular diseases. JAMA 24, 2396–2405. doi: 10.1001/jama.2020.23068
Hsu, Y.-Y., Schuff, N., Du, A.-T., Mark, K., Zhu, X., Hardin, D., et al. (2002). Comparison of automated and manual MRI volumetry of hippocampus in normal aging and dementia. J. Magn. Reson. Imaging 16, 305–310. doi: 10.1002/jmri.10163
Jack, C. R. Jr., Bernstein, M. A., Borowski, B. J., Gunter, J. L., Fox, N. C., Thompson, P. M., et al. (2010). Update on the magnetic resonance imaging core of the Alzheimer’s disease neuroimaging initiative. Alzheimers Dement. 6, 212–220. doi: 10.1016/j.jalz.2010.03.004
Jack, C. R., Bennett, D. A., Blennow, K., Carrillo, M. C., Feldman, H. H., Frisoni, G. B., et al. (2016). A/T/N: An unbiased descriptive classification scheme for Alzheimer disease biomarkers. Neurology 87, 539–547. doi: 10.1212/wnl.0000000000002923
Jagust, W. J., Bandy, D., Chen, K., Foster, N. L., Landau, S. M., Mathis, C. A., et al. (2010). The Alzheimer’s disease neuroimaging initiative positron emission tomography core. Alzheimers Dement. 6, 221–229. doi: 10.1016/j.jalz.2010.03.003
Jia, J., Hu, J., Huo, X., Miao, R., Zhang, Y., and Ma, F. (2019). Effects of vitamin D supplementation on cognitive function and blood Aβ-related biomarkers in older adults with Alzheimer’s disease: A randomised, double-blind, placebo-controlled trial. J. Neurol. Neurosurg. Psychiatry 90, 1347. doi: 10.1136/jnnp-2018-320199
Killiany, R. J., Hyman, B. T., Gomez-Isla, T., Moss, M. B., Kikinis, R., Jolesz, F., et al. (2002). MRI measures of entorhinal cortex vs hippocampus in preclinical AD. Neurology 58, 1188–1196. doi: 10.1212/WNL.58.8.1188
Kostev, K., Hadji, P., and Jacob, L. (2018). Impact of osteoporosis on the risk of dementia in almost 60,000 patients followed in general practices in Germany. J. Alzheimers Dis. 65, 401–407. doi: 10.3233/jad-180569
Kril, J. J., Patel, S., Harding, A. J., and Halliday, G. M. (2002). Neuron loss from the hippocampus of Alzheimer’s disease exceeds extracellular neurofibrillary tangle formation. Acta Neuropathol. (Berl.) 103, 370–376. doi: 10.1007/s00401-001-0477-5
Kwon, M. J., Kim, J.-H., Kim, J. H., Cho, S. J., Nam, E. S., and Choi, H. G. (2021). The occurrence of Alzheimer’s disease and Parkinson’s disease in individuals with osteoporosis: A longitudinal follow-up study using a national health screening database in Korea. Front. Aging Neurosci. 13:786337. doi: 10.3389/fnagi.2021.786337
Li, S., Liu, B., Zhang, L., and Rong, L. (2014). Amyloid beta peptide is elevated in osteoporotic bone tissues and enhances osteoclast function. Bone 61, 164–175. doi: 10.1016/j.bone.2014.01.010
Li, S., Yang, B., Teguh, D., Zhou, L., Xu, J., and Rong, L. (2016). Amyloid β peptide enhances RANKL-induced osteoclast activation through NF-κB, ERK, and calcium oscillation signaling. Int. J. Mol. Sci. 17, E1683. doi: 10.3390/ijms17101683
Li, X., Tong, Q., Gao, J., Liu, C., Alzheimer’s Disease Neuroimaging Initiative, and Liu, Y. (2020). Osteoarthritis was associated with a faster decline in hippocampal volumes in cognitively normal older people. Front. Aging Neurosci. 12:190. doi: 10.3389/fnagi.2020.00190
Li, X.-F., Xu, H., Zhao, Y.-J., Tang, D.-Z., Xu, G.-H., Holz, J., et al. (2013). Icariin augments bone formation and reverses the phenotypes of osteoprotegerin-deficient mice through the activation of Wnt/β-Catenin-BMP signaling. J. Evid. Based Complement. Altern. Med. 2013:e652317. doi: 10.1155/2013/652317
Luckhaus, C., Mahabadi, B., Grass-Kapanke, B., Jänner, M., Willenberg, H., Jäger, M., et al. (2009). Blood biomarkers of osteoporosis in mild cognitive impairment and Alzheimer’s disease. J. Neural Transm. Vienna 116, 905–911. doi: 10.1007/s00702-009-0241-x
Mangialasche, F., Solomon, A., Winblad, B., Mecocci, P., and Kivipelto, M. (2010). Alzheimer’s disease: Clinical trials and drug development. Lancet Neurol. 9, 702–716. doi: 10.1016/S1474-4422(10)70119-8
McKhann, G. M., Knopman, D. S., Chertkow, H., Hyman, B. T., Jack, C. R. Jr., Kawas, C. H., et al. (2011). The diagnosis of dementia due to Alzheimer’s disease: Recommendations from the national institute on aging-Alzheimer’s association workgroups on diagnostic guidelines for Alzheimer’s disease. Alzheimers Dement. 7, 263–269. doi: 10.1016/j.jalz.2011.03.005
Morello, M., Landel, V., Lacassagne, E., Baranger, K., Annweiler, C., Féron, F., et al. (2018). Vitamin D improves neurogenesis and cognition in a mouse model of Alzheimer’s disease. Mol. Neurobiol. 55, 6463–6479. doi: 10.1007/s12035-017-0839-1
Norton, S., Matthews, F. E., Barnes, D. E., Yaffe, K., and Brayne, C. (2014). Potential for primary prevention of Alzheimer’s disease: An analysis of population-based data. Lancet Neurol. 13, 788–794. doi: 10.1016/S1474-4422(14)70136-X
Petersen, R. C., Aisen, P. S., Beckett, L. A., Donohue, M. C., Gamst, A. C., Harvey, D. J., et al. (2010). Alzheimer’s disease neuroimaging initiative (ADNI). Neurology 74, 201–209. doi: 10.1212/wnl.0b013e3181cb3e25
Pintzka, C., Hansen, T. I., Evensmoen, H., and Håberg, A. (2015). Marked effects of intracranial volume correction methods on sex differences in neuroanatomical structures: A HUNT MRI study. Front. Neurosci. 9:238. doi: 10.3389/fnins.2015.00238
Polydoro, M., Acker, C. M., Duff, K., Castillo, P. E., and Davies, P. (2009). Age-dependent impairment of cognitive and synaptic function in the htau mouse model of tau pathology. J. Neurosci. 29, 10741–10749. doi: 10.1523/JNEUROSCI.1065-09.2009
Ristori, E., Donnini, S., and Ziche, M. (2020). New insights into blood-brain barrier maintenance: The homeostatic role of β-amyloid precursor protein in cerebral vasculature. Front. Physiol. 11:1056. doi: 10.3389/fphys.2020.01056
Roberts, K. F., Elbert, D. L., Kasten, T. P., Patterson, B. W., Sigurdson, W. C., Connors, R. E., et al. (2014). Amyloid-β efflux from the central nervous system into the plasma. Ann. Neurol. 76, 837–844. doi: 10.1002/ana.24270
Saykin, A. J., Shen, L., Foroud, T. M., Potkin, S. G., Swaminathan, S., Kim, S., et al. (2010). Alzheimer’s disease neuroimaging Initiative biomarkers as quantitative phenotypes: Genetics core aims, progress, and plans. Alzheimers Dement. 6, 265–273. doi: 10.1016/j.jalz.2010.03.013
Scott, J. A., Braskie, M. N., Tosun, D., Thompson, P. M., Weiner, M., DeCarli, C., et al. (2015). Cerebral amyloid and hypertension are independently associated with white matter lesions in elderly. Front. Aging Neurosci. 7:221. doi: 10.3389/fnagi.2015.00221
Shaw, L. M., Vanderstichele, H., Knapik-Czajka, M., Clark, C. M., Aisen, P. S., Petersen, R. C., et al. (2009). Cerebrospinal fluid biomarker signature in Alzheimer’s disease neuroimaging initiative subjects. Ann. Neurol. 65, 403–413. doi: 10.1002/ana.21610
Sohrabi, H. R., Bates, K. A., Weinborn, M., Bucks, R. S., Rainey-Smith, S. R., Rodrigues, M. A., et al. (2015). Bone mineral density, adiposity, and cognitive functions. Front. Aging Neurosci. 7:16. doi: 10.3389/fnagi.2015.00016
Stapledon, C. J. M., Stamenkov, R., Cappai, R., Clark, J. M., Bourke, A., Bogdan Solomon, L., et al. (2021). Relationships between the bone expression of Alzheimer’s disease-related genes, bone remodelling genes and cortical bone structure in neck of femur fracture. Calcif. Tissue Int. 108, 610–621. doi: 10.1007/s00223-020-00796-y
Tan, Z. S., Seshadri, S., Beiser, A., Zhang, Y., Felson, D., Hannan, M. T., et al. (2005). Bone mineral density and the risk of Alzheimer disease. Ann. Neurol. 62, 107–111. doi: 10.1001/archneur.62.1.107
Varma, V. R., Wang, Y., An, Y., Varma, S., Bilgel, M., Doshi, J., et al. (2021). Bile acid synthesis, modulation, and dementia: A metabolomic, transcriptomic, and pharmacoepidemiologic study. PLoS Med. 18:e1003615. doi: 10.1371/journal.pmed.1003615
Voevodskaya, O., Simmons, A., Nordenskjöld, R., Kullberg, J., Ahlström, H., Lind, L., et al. (2014). The effects of intracranial volume adjustment approaches on multiple regional MRI volumes in healthy aging and Alzheimer’s disease. Front. Aging Neurosci. 6:264. doi: 10.3389/fnagi.2014.00264
Wang, H., Stewart, T., Toledo, J. B., Ginghina, C., Tang, L., Atik, A., et al. (2018). A longitudinal study of total and phosphorylated alpha-synuclein with other biomarkers in cerebrospinal fluid of Alzheimer’s disease and mild cognitive impairment. J. Alzheimers Dis. 61, 1541–1553. doi: 10.3233/JAD-171013
Weiner, M. W., Aisen, P. S., Jack, C. R. Jr., Jagust, W. J., Trojanowski, J. Q., Shaw, L., et al. (2010). The Alzheimer’s disease neuroimaging initiative: Progress report and future plans. Alzheimers Dement. 6, 202–211.e7. doi: 10.1016/j.jalz.2010.03.007
Xia, W.-F., Jung, J.-U., Shun, C., Xiong, S., Xiong, L., Shi, X.-M., et al. (2013). Swedish mutant APP suppresses osteoblast differentiation and causes osteoporotic deficit, which are ameliorated by N-acetyl-L-cysteine. J. Bone Miner. Res. 28, 2122–2135. doi: 10.1002/jbmr.1954
Xiong, J., Kang, S. S., Wang, Z., Liu, X., Kuo, T.-C., Korkmaz, F., et al. (2022). FSH blockade improves cognition in mice with Alzheimer’s disease. Nature 603, 470–476. doi: 10.1038/s41586-022-04463-0
Xu, W., Zhang, C., Li, J.-Q., Tan, C.-C., Cao, X.-P., Tan, L., et al. (2019). Age-related hearing loss accelerates cerebrospinal fluid tau levels and brain atrophy: A longitudinal study. Aging 11, 3156–3169. doi: 10.18632/aging.101971
Yaffe, K., Browner, W., Cauley, J., Launer, L., and Harris, T. (1999). Association between bone mineral density and cognitive decline in older women. J. Am. Geriatr. Soc. 47, 1176–1182. doi: 10.1111/j.1532-5415.1999.tb05196.x
Zhang, Z. (2014). Too much covariates in a multivariable model may cause the problem of overfitting. J. Thorac. Dis. 6, E196–E197. doi: 10.3978/j.issn.2072-1439.2014.08.33
Zhou, R., Chen, H., Ye, F., Huang, S., and Zhang, J. (2020). Influence of hypertension on longitudinal changes in brain glucose metabolism was modified by the apoe4 allele among cognitively normal older individuals. Front. Aging Neurosci. 12:85. doi: 10.3389/fnagi.2020.00085
Keywords: Alzheimer’s disease, cerebrospinal fluid, biomarker, entorhinal cortex, hippocampus, osteoporosis, cross-sectional study, longitudinal study
Citation: Pan H, Cao J, Wu C, Huang F, Wu P, Lang J and Liu Y (2022) Osteoporosis is associated with elevated baseline cerebrospinal fluid biomarkers and accelerated brain structural atrophy among older people. Front. Aging Neurosci. 14:958050. doi: 10.3389/fnagi.2022.958050
Received: 31 May 2022; Accepted: 23 August 2022;
Published: 16 September 2022.
Edited by:
Hans J. Grabe, University of Greifswald, GermanyReviewed by:
Feng Han, The Pennsylvania State University (PSU), United StatesWen-Cheng Xiong, Case Western Reserve University, United States
Lijun Wang, Shanghai Jiao Tong University, China
Copyright © 2022 Pan, Cao, Wu, Huang, Wu, Lang and Liu. This is an open-access article distributed under the terms of the Creative Commons Attribution License (CC BY). The use, distribution or reproduction in other forums is permitted, provided the original author(s) and the copyright owner(s) are credited and that the original publication in this journal is cited, in accordance with accepted academic practice. No use, distribution or reproduction is permitted which does not comply with these terms.
*Correspondence: Yangbo Liu,, liuyb@wmu.edu.cn
†Data used in the preparation of this article were obtained from the Alzheimer’s Disease Neuroimaging Initiative (ADNI) database (adni.loni.usc.edu). As such, the investigators within the ADNI contributed to the design and implementation of ADNI and/or provided data but did not participate in the analysis or writing of this report. A complete listing of ADNI investigators can be found at: http://adni.loni.usc.edu/wp-content/uploads/how_to_apply/ADNI_Acknowledgement_List.pdf