- 1Department of Psychology, The University of Alabama, Tuscaloosa, AL, United States
- 2Alabama Research Institute on Aging, Tuscaloosa, AL, United States
- 3Department of Psychology, University of British Columbia, Vancouver, BC, Canada
Human-animal interactions that stem from pet ownership have a wide range of benefits for social, emotional, and physical health. These factors also tend to improve cognition. Following this logic, owning a pet could indirectly enhance cognitive and brain health through mechanisms like improvements in well-being, socialization, and decreased stress. In the present study, cross-sectional data were drawn from the Alabama Brain Study on Risk for Dementia in which 95 participants aged 20–74 were recruited. Specifically, 56 adults were pet-owners and 39 adults were not pet-owners. Multivariate analyses revealed that pet ownership was related to higher levels of cognition and larger brain structures, and these effects were largest in dog owners. The most consistent cognitive relationships were found with better processing speed, attentional orienting, and episodic memory for stories, and with dorsal attention, limbic, and default mode networks. Moreover, we show that owning a pet can reduce one’s brain age by up to 15 years. Pet ownership was not related to indirect factors including social, emotional, and physical health. We found also that older adults’ brain health benefited from owning more than one pet versus owning one or fewer pets. These findings indicate that pet ownership, especially dog ownership, may play a role in enhancing cognitive performance across the adult lifespan, which could in turn influence protection against age-related cognitive decline.
Introduction
According to the 2021–2022 National Pet Owners Survey, about 90.5 million families in the United States own a pet with nearly 70% of those being a dog (American Pet Products Association, 2021). Human-animal interactions that stem from pet ownership have a wide-range of benefits for social, emotional, and physical health (Hart, 1995; Wells, 2007; Perkins et al., 2008; Beetz et al., 2012; Beck, 2014; Ling et al., 2016). Pets enhance the social lives of older adults in many ways, including providing someone to talk to, presenting opportunities to meet new people on walks, and providing a topic of conversation (Beetz et al., 2012; Beck, 2014). All these factors can improve health by decreasing stress, increasing sleep quality, reducing blood pressure, and possibly improving one’s immune system functioning (Khan and Farrag, 2000; Headey and Grabka, 2006).
Behaviors that improve mental and physical functioning often improve cognition (Hertzog et al., 2008). Following this logic, owning a pet might indirectly confer protection against age-related declines in cognition through the aforementioned mechanisms. Researchers have also hypothesized that pet ownership might directly benefit cognition by enriching one’s environment and enhancing brain plasticity (Yorke, 2010). To take care of an animal, one needs to remember to feed, walk, and groom them. One must engage in critical thinking, plan for the future, and practice self-regulation when exerting patience with a pet (Ling et al., 2016). Because dogs tend to be more dependent than other pets like cats, some of these benefits may be more pronounced in dog owners than cat owners.
Despite these potential benefits of pet ownership, few studies have investigated the benefits of owning pets among community-dwelling older adults (Hughes et al., 2020; Albright et al., 2022). Most research investigating such relationships has focused on the therapeutic effects of animal-assisted therapy in patients with memory disorders (Kanamori et al., 2001; Bono et al., 2015) or those who need care in nursing homes (Stasi et al., 2004; Colombo et al., 2006). These studies investigated very coarse, global measures of cognition via measures like the Mini-Mental Status Exam (MMSE) and showed mixed results. Two notable studies that have investigated cognitive differences in community-dwelling older adults found small, but significant benefits for (1) executive function when comparing pet owners to non-pet owners (with no differences in dog versus cat owners; Branson et al., 2016) and (2) global cognition for those given an insect to care for compared to an older adult control group (Ko et al., 2016). This limited evidence for the benefits of pet ownership may be due to the focus on older adults after memory and health have already become sufficiently compromised or the use of insensitive, global tests of cognition.
An unexplored reason for the lack of strong evidence might be a relative insensitivity of pet ownership on distal outcomes like cognition because such changes in cognition might take a long time to manifest (i.e., the effects might be latent). Instead, more proximal outcomes like brain structure and function might be more sensitive to the impacts of owning a pet. As shown in Figure 1, the social, emotional, and physical health benefits described earlier should impact multiple brain regions (Bickart et al., 2014; Puce et al., 2015; Porcelli et al., 2019). To socialize (with humans or pets), one must understand and think about the others’ perspective—known as Theory of Mind (Gallagher and Frith, 2003). Studies have shown that engaging in theory of mind activates overlapping brain regions involved with memory, namely the default mode network (DMN; Spreng et al., 2009). Regulating one’s emotions also activates parts of the DMN (e.g., ventromedial prefrontal cortex; Hostinar and Gunnar, 2015). Physical exercise interventions increase blood flow and oxygen delivery to the brain, thereby improving brain function in older adults (Bherer et al., 2013). Some of these regions include the ventromedial prefrontal cortex and the hippocampus. Lastly, older adults engaging in complex learning environments show increases in brain activity in posterior brain regions that overlap with the DMN, including lateral parietal cortex and the precuneus (McDonough et al., 2015).
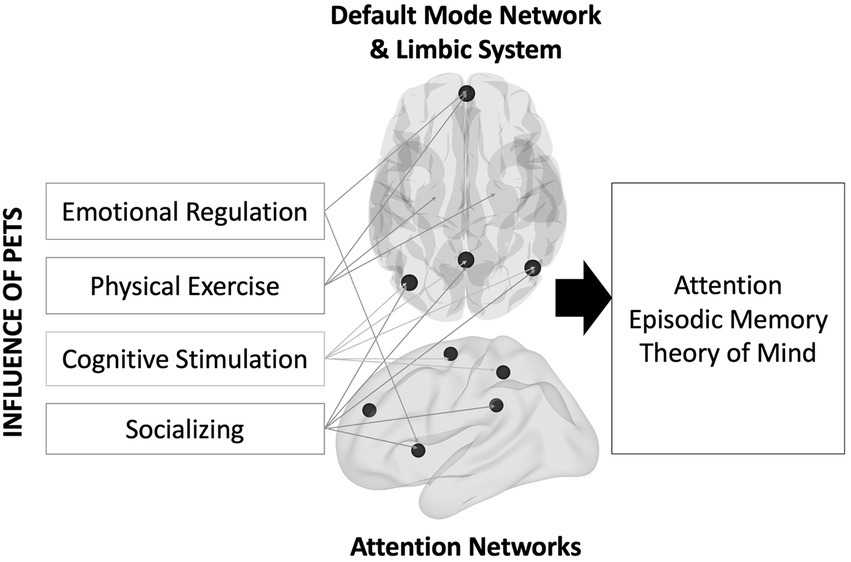
Figure 1. Theoretical model describing the influence of pet ownership on brain and cognitive health.
Only a handful of studies have compared brain activity between pet and non-pet owners and many of them have focused on the neural correlates of visual perception (Mormann et al., 2011; Stoeckel et al., 2014). When viewing photographs of companion animals, pet-owners exhibited more brain activation than non-pet owners in lateral frontal and occipital regions, which are thought to be involved in attention and visual processing (Hayama et al., 2016). This study also showed that greater bonds with their pets were associated with higher brain activity in the lateral parietal cortex and the precuneus (regions in the DMN). These regions might be related to inferring mental states in pets. Supporting this idea, Desmet et al. (2017) showed that interpreting dog behaviors activated the DMN to a greater extent than interpreting human behaviors, especially when the behaviors were harder to interpret. These findings suggest that pets clearly attract the interest and attention of humans, but these studies do not reveal whether pet-ownership confers long-term alterations within brain networks when not actively viewing pets or other animals.
As a group, older adults show widespread brain shrinkage, cortical thinning, and reduction in within-network brain activity (Grady, 2012). However, some neurocognitive theories of aging hypothesize that middle-aged and older adults who can maintain optimal cognitive and brain health often show a “youth-like” pattern of brain structure and function (Park and Reuter-Lorenz, 2009; Nyberg et al., 2012). Pet ownership might serve as a series of experiences that enhances cognitive and brain functioning.
Present study
First, we tested the extent that pet-ownership was associated with distal outcomes in specific cognitive domains (attention, episodic memory, and inductive reasoning). Additional proximal outcomes include brain structure (volume, surface area, and cortical thickness) and functional connectivity. Each neuroimaging modality was extracted from key cortical networks (DMN, limbic, and attention) to test targeted a priori hypotheses while reducing the number of multiple comparisons as would be done in a whole-brain approach. We hypothesized that owning a pet would be associated with (1) better cognitive functioning, (2) larger brain structures, and (3) higher within-network functional connectivity compared to not owning any pets. In sensitivity analyses, we also tested (a) whether the effects remained after removing non-dog owners from the analyses (i.e., directly comparing dog owners vs. non-pet owners), (b) whether dog-ownership differed from all others (both non-ownership and non-dog owners), and (c) whether the effects remained after controlling for global cognition/brain integrity.
Second, we tested a novel hypothesis that owning multiple pets might increase one’s daily cognitive load. Cognitive load might be elevated due to the different behaviors and needs of each pet. Accordingly, we predicted that owning more than one pet, regardless of type, will be associated with greater cognitive functioning and brain integrity than owning one or no pet. In sensitivity analyses, we tested whether the relationships remained after controlling for global cognition/brain integrity.
In exploratory analyses, we tested for interactions with age to examine whether the benefits of owning a pet differ depending on age group. Another way to investigate this question is to calculate a “brain age” score for each participant and test whether owning a pet leads to decelerated brain aging or accelerated brain aging. Brain age scores are created by comparing one’s predicted age based on brain measures and one’s chronological age as a summary score of brain health (Cole and Franke, 2017). Finally, we tested for evidence that any significant relationships can be accounted for by indirect effects of social, emotional, and physical health.
Materials and methods
The hypotheses and planned analyses were pre-registered on the Open Science Framework at: https://osf.io/e7up3 to improve the integrity of research by preventing practices that allow for too many researcher degrees of freedom, thereby creating false positives (Simmons et al., 2021).
Participants
Data were used from the Alabama Brain Study on Risk for Dementia (McDonough et al., 2019). One of the goals of the study was to uncover evidence for new potential protective factors for cognitive and brain health in middle-aged and older adults at varying risk for dementia along with a comparative sample of young adults. Participants were recruited from the Tuscaloosa and Birmingham areas through word of mouth, flyers, online ads, and newsletters. Participants were initially screened over the phone to assess study eligibility. Participants were included if they fell in the young adult age range (20–30) or were between 50 and 75 years old. Participants were excluded if they had contra-indicators for magnetic resonance imaging (MRI), were left-handed, had a prior diagnosis of any neurological condition, stroke, traumatic brain injury, claustrophobia, or history of substance abuse. Participants were then scheduled for a neuropsychological/physical health assessment and an MRI assessment. In between the two assessments, an online survey was given to assess sociodemographic information and various lifestyle factors including subjective well-being, social well-being, and psychological distress. After the neuropsychological assessment, participants were further excluded if they had a St. Louis University Mental Status (SLUMS; Tariq et al., 2006) score less than 20 (after adjusting for education level). All participants gave informed consent as approved by the institutional review board at The University of Alabama.
A power analysis using G*Power indicated that a total sample size of 78 participants would be needed to detect a large effect size (d = 0.80) with 80% power for an independent t-test (e.g., pet owner vs. non-owner) at an alpha level of 0.01. Ninety-five participants completed the neuropsychological assessment and 86 completed the MRI session. Participants’ vision was normal or corrected to normal using MR-compatible glasses or contact lenses. One participant was missing their years of education which was used as a covariate in all analyses. Regression-based imputation was used to estimate this value to maximize the sample size. The parameter estimates from this regression equation were then used to predict the participant’s education level.
Cognitive assessments
Six cognitive domains were assessed: attention, processing speed, episodic memory, executive function, reasoning, and crystallized ability (Table 1). Composite scores were created when at least two tests represented a construct. For our primary hypotheses, we only tested whether pet ownership would be related specifically to attention/speed, episodic memory, and reasoning. The other cognitive domains were included in an analysis controlling for global cognition to investigate differential associations.
Three different types of attention were assessed using the Attention Network Test (ANT; Fan et al., 2002): alerting, orienting, and executive attention. Response times derived from difference scores were used as outcome variables. Processing speed was assessed using the Digit Comparison Task (Salthouse and Babcock, 1991; Hedden et al., 2002), Trail Making Test A (Reitan, 1979), and mean response times from the Attention Network Test (Fan et al., 2002). Response times were square root transformed and reversed. Episodic memory was assessed using the Wechsler Memory Scale IV (Drozdick et al., 2018) and tested verbal, story, and spatial memory. Each of the three memory tests consisted of an immediate recall test, a delayed recall test, and a delayed recognition test, which were standardized and averaged to create composite scores. Preliminary correlations between each test were low, suggesting that separate composite scores would be most appropriate. Reasoning was assessed using Raven’s Progressive Matrices (Raven, 1938). Accuracy was used as the primary outcome variable. The additional cognitive tasks were used to form a global cognition score. These included a word reading and sentence completion task from the Wide Range Achievement Test-Fourth Edition (WRAT-4; Wilkinson and Robertson, 2006) to form a crystallized ability score. Executive function was measured using the Trail Making Test B and the executive attention score from the ANT.
Physical health assessments
Several health assessments were administered as a break in between the cognitive sessions (Table 1). Height was self-reported by participants. Weight was assessed using an OMRON body composition BF511 scale (Omron, Japan). Body mass index (BMI) was calculated as weight (kg)/height (m)2. Waist circumference was assessed by trained study staff who measured circumference at the level of the navel. A HealthSmart semi-automatic blood pressure monitor was used to estimate systolic and diastolic blood pressure after being seated for at least 20 min. When possible, two measures were taken at the separate assessment sessions and averaged together. Lastly, in an online survey, subjects were asked several questions that were used in the Jackson Heart Study (Taylor et al., 2005) regarding their health habits. The Physical Activity Form was used to measure the frequency that participants engage in physical activity during their leisure time; each item used a five-point scale: less than once a month, once a month, 2–3 times a month, once a week, and more than once a week.
Psychosocial assessments
Several questionnaires were administered in an online survey between the cognitive and MRI sessions. Several were chosen to represent a priori constructs related to subjective well-being, social well-being, and psychological distress (Table 1). Pet ownership has been previously associated with higher well-being and reduced psychological distress. For each construct, each scale was standardized, reversed if appropriate, and averaged together. Two questionnaires were used to assess subjective well-being: the Positive and Negative Affect Schedule (PANAS; Watson et al., 1988) and the Satisfaction with Life Scale (SWLS; Diener et al., 1985). Higher scores indicated greater subjective well-being. Two questionnaires were used to assess social well-being: the Revised UCLA Loneliness Scale (Russell, 1996) and a short form of the Interpersonal Support Evaluation List (ISEL; Cohen and Hoberman, 1983; Payne et al., 2012). Higher scores indicated greater social well-being. Three questionnaires were used to assess psychological distress: Center for Epidemiological Studies–Depression (CES-D) Scale (Radloff, 1977), the short-Perceived Stress Scale (PSS-4; Cohen et al., 1983; Warttig et al., 2013), and three questions assessing feelings of anxiety or depression from the Short-Form Health Survey (SF-36; Ware and Sherbourne, 1992). Higher scores represented greater distress.
MRI assessments
Participants underwent one structural MRI, task-related fMRI (not reported here) and two 5-min resting state scans. The present analysis focused on structural and resting-state scans.
Structural MRI acquisition and processing
MRI data were collected using a 3T Siemens PRISMA scanner at the UAB Civitan International Neuroimaging Laboratory. High resolution T1-weighted structural MPRAGE scans were acquired using (parallel acquisition acceleration type = GRAPPA; acceleration factor = 3, TR = 5,000 ms, TE = 2.93 ms, TI 1 = 700 ms, TI 2 = 2,030 ms, flip angle 1 = 4°, flip angle 2 = 5°, FOV = 256 mm, matrix = 240 × 256 mm2, in-plane resolution = 1.0 × 1.0 mm2).
T1 structural images were visually inspected for signs of movement or artifact. Structural images were then preprocessed in FreeSurfer version 6.0 (Fischl, 2012). The Freesurfer recon-all pipeline transformed the raw imaging data with a series of processes, including affine registration onto Talairach atlas, bias correction, skull stripping, and removing brain stem and cerebellum. Mindcontrol (Keshavan et al., 2018) was used to detect outlying regions and manual corrections were implemented to correct for any errors. Volumes transformed to the default surface-based map and network templates from Yeo et al. (2011) were used to extract mean gray matter volume, surface area, and cortical thickness values for each network. Intracranial volume was regressed out of all gray matter volume and area estimates as recommended by Buckner et al. (2004) before entering them into our main analyses.
Functional MRI acquisition and processing
Functional scans used T2*-weighted EPI sequences (56 interleaved axial slices, 2.5 mm thickness, TR = 1,720 ms, TE = 35.8 ms, flip angle = 73°, FOV = 260 mm, matrix = 104 × 104 mm, in-plane resolution = 2.5 × 2.5 mm2, multi-band acceleration factor = 4). Functional data were unwarped, realigned, and spatially smoothed using an 8 mm FWHM kernel using the CONN toolbox (Whitfield-Gabrieli and Nieto-Castanon, 2012). These smoothed images were then denoised using Multivariate Exploratory Linear Optimized Decomposition into Independent Components (MELODIC). Specifically, in-house scripts were used to flag spatiotemporal components that applied machine learning to frequency and temporal elements indicative of potential artifacts. The flagged components were then regressed from the BOLD signal using MELODIC. The denoised data were warped into a study template using Advanced Normalization Tools (ANTS; Avants et al., 2009). The CONN Toolbox was used to regress out white matter and CSF BOLD time series, motion parameters and their first derivatives, and outlying time points and their first derivatives (CONN’s version of scrubbing). Bandpass filtering was implemented with a lowpass of 0.008 Hz and a highpass of 0.09 Hz. Quadratic detrending and despiking were also implemented. Finally, the first scan was removed from all sessions and the two scans were concatenated before analysis.
Within-network functional connectivity was computed using the Dual_Regression function in FSL to extract the time series and spatial correlation maps that best fit a volume-based atlas of the 17 networks from Yeo et al. (2011). The dual regression resulted in Z-scored subject-specific maps for each network. To summarize connectivity, the average Z-score was computed for each subject’s map using each network template as a mask. Higher scores represent stronger within-network connectivity. Although we calculated within-network connectivity as defined by the original templates, many of these networks also were highly correlated with subcortical regions. The average connectivity values in subcortical brain region for each network tested can be found in Supplementary material.
Statistical analysis
Cognition and brain health were assessed using multivariate analyses of covariate (MANCOVAs) separately for each modality (cognition, brain volume, surface area, cortical thickness, and resting-state functional connectivity). The first family of tests investigated the effects of pet ownership (the independent variable) while controlling for a priori variables chronological age, years of education, ethnoracial group (1 = non-Hispanic White, 0 = others), and sex (1 = Male, 0 = Female), implementing Bonferroni corrections for five comparisons. If the multivariate statistic was significant, follow-up analyses of covariance (ANCOVAs) were conducted for each individual measure.
These analyses were followed by sensitivity analyses to test whether similar effects would be found for (a) dog owners compared with non-dog owners (pet owners and non-pet owners), (b) dog owners with non-pet owners, and (c) pet ownership with non-pet ownership after controlling for a global measure of the current metric (cognition, brain volume, etc.). Global scores were calculated by standardizing all cognitive measures or brain networks and averaging them together. These global scores were regressed out of each measure and the MANCOVA was recalculated. Given the goal of the sensitivity analyses were to understand general trends of variations in analyses, we report uncorrected p-values for the MANOVA and only correct for multiple comparisons in follow-up ANOVAs.
The second family of tests used a series of MANCOVAs to investigate whether owning more than one pet was associated with better cognitive or brain health compared with owning one or no pets, implementing Bonferroni corrections for five comparisons. The only sensitivity analysis that was appropriate was investigating the effects of owning multiple pets while also controlling for global cognitive or brain health.
Lastly, exploratory analyses were conducted to test whether chronological age moderated any of the effects, whether owning a pet was associated with a younger “brain age,” and whether previously found health and psychosocial variables indirectly related pet ownership to cognitive or brain health. Even though these analyses were exploratory, they were all planned exploratory analyses except for the BrainAGE analysis (for review, see Franke and Gaser, 2019). For this analysis, we created a matrix of all cognitive and brain network scores and regressed out the covariates for participants that had each score (N = 83). We then centered and scaled this matrix and used linear support vector regression with default parameters to assess a brain age score for each participant. A leave-one-participant-out method was used such that all the available data was used to create an age model. The left-out participant then served as the test item to apply that model in an unbiased way and to determine a predicted brain age score. This process was applied repeatedly leaving out a new participant each time, resulting in 83 models. A brain age correction factor was then implemented (as discussed in de Lange and Cole, 2020) to correct for extreme estimations that often occur at the lowest and highest ends of the age continuum. The gap between the predicted age and chronological age represents an accelerated or decelerated biological age (i.e., BrainAGE) relative to one’s actual age.
To test for indirect effects, four a priori indirect constructs were assessed: physical health, subjective well-being, social well-being, and psychological distress. All analyses were carried out in R (R Core Team, 2021).
Results
Sociodemographic descriptive statistics
Compared with non-pet owners, pet owners rated themselves higher on perceived social class (t (76.34) = 2.50, p = 0.014) and were more likely to be non-Hispanic White (χ2(1) = 6.05, p = 0.014). Compared with owning one or fewer pets, owning multiple pets was more likely for females than males (χ2(1) = 5.51, p = 0.019) and for non-Hispanic White Americans than ethnoracial minorities (χ2(1) = 9.77, p = 0.0018). No other variables were significant (ps > 0.065; Table 2).1 Mean values of brain metrics can be found in Supplementary material.
Effects of pet ownership on cognitive and brain health
Cognitive health
A MANCOVA was conducted to investigate the effect of pet ownership on seven measures of cognition, controlling for chronological age, years of education, racial category, and biological sex (Table 3). This analysis resulted in a significant multivariate effect of pet ownership, Pillai’s Trace = 0.27, F(7, 81) = 4.25, padj = 0.0024. Follow-up univariate analyses showed that pet ownership was associated with greater attentional orienting (β = 0.30, 95% CI [0.10, 0.47], padj = 0.029), processing speed (β = 0.31, 95% CI [0.11, 0.47], padj = 0.024), verbal memory (β = 0.31, 95% CI [0.11, 0.48], padj = 0.0098), and story memory (β = 0.34, 95% CI [0.15, 0.50], padj = 0.0026). Details can be found in Figure 2 (Panel A).
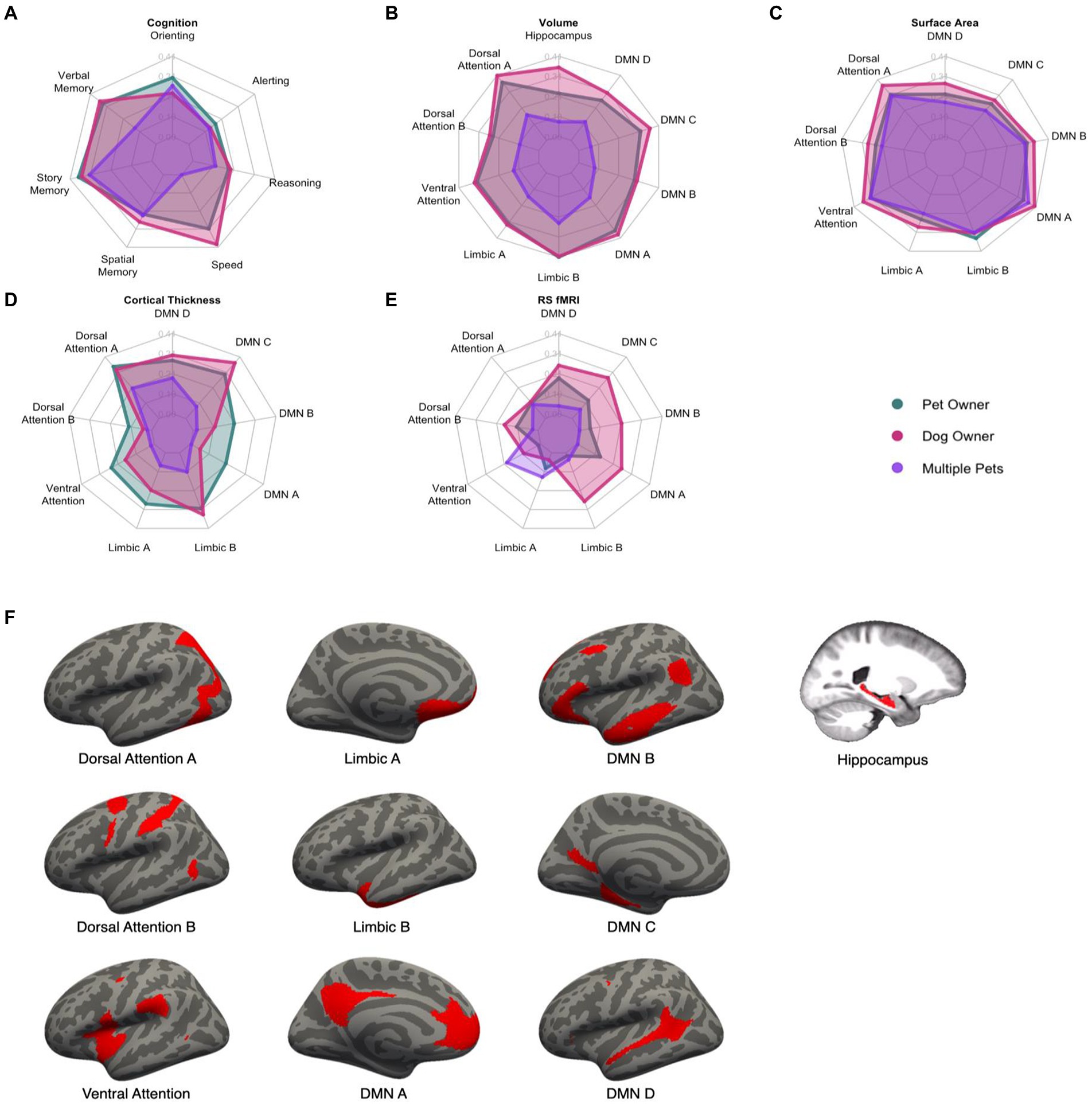
Figure 2. Radar plots of the standardized effect sizes of pet ownership (green), dog ownership (red), and owning multiple pets (purple) for cognitive and brain outcomes (A–E). Higher values indicate a greater difference between the three groups and non-pet owners. Visual depiction of the networks used in the analyses (F). DMN, Default Mode Network.
Brain health
For brain volume, the MANCOVA resulted in a significant effect of pet ownership, Pillai’s Trace = 0.32, F(10, 71) = 3.30, padj = 0.0075. Pet ownership was associated with larger volumes in the dorsal attention A (β = 0.37, 95% CI [0.16, 0.53], padj = 0.0068), ventral attention (β = 0.32, 95% CI [0.11, 0.49], padj = 0.035), limbic A (β = 0.32, 95% CI [0.11, 0.49], padj = 0.035), limbic B (β = 0.41, 95% CI [0.22, 0.56], padj = 0.0012), default mode A (β = 0.37, 95% CI [0.16, 0.53], padj = 0.0072), and default mode C (β = 0.32, 95% CI [0.11, 0.49], padj = 0.035) networks. The MANCOVA did not reach the criterion for significance for pet ownership on surface area (Pillai’s Trace = 0.24, F(9, 72) = 2.51, padj = 0.075), cortical thickness (Pillai’s Trace = 0.23, F(9, 72) = 2.37, padj = 0.11), or resting-state connectivity (Pillai’s Trace = 0.086, F(9, 72) = 0.76, padj = 1.00). Standardized effect sizes for each of the brain measures are shown in Figure 2.
Sensitivity analyses for effects of pet ownership
We conducted three additional planned analyses to assess the robustness of the effects by testing (a) dog-owners vs. non-dog owners (but could include other pets), (b) dog-owners vs. non-pet owners, and (c) pet-owners vs. non-pet owners after controlling for global cognition and brain integrity.
Cognitive health
The first compared dog owners with non-dog owners and yielded a significant effect of dog ownership (Pillai’s Trace = 0.28, F(7, 81) = 4.56, puncorr = 0.00025). In follow-up ANOVAs by measure, processing speed, verbal memory, and story memory reached significance (psadj < 0.005; Figure 2). The second analysis compared dog owners with non-pet owners and yielded a significant effect of dog owners (Pillai’s Trace = 0.35, F(7, 69) = 5.20, puncorr = 0.000086). Attentional orienting and story memory reached significance (psadj < 0.037). The last analysis intended to test whether pet ownership would be significant after controlling for global cognition. This analysis yielded a significant effect of pet ownership (Pillai’s Trace = 0.20, F(7, 81) = 2.86, puncorr = 0.010). Only attentional orienting (padj = 0.0083) and story memory (padj = 0.037) were significant. These analyses suggest that the effects of pet ownership on cognition are quite robust and even stronger for dog owners than other pet owners. Moreover, pet ownership has differentially strong effects on story memory even after accounting for global cognition.
Brain health
For brain volume, dog owners showed a significant multivariate effect compared with non-dog owners (Pillai’s Trace = 0.33, F(10, 71) = 4.22, puncorr = 0.00079). The same networks were significant as in the primary pet owner analysis (psadj < 0.026) and bilateral hippocampal volume also was significant (β = 0.35, 95% CI [0.15, 0.52], padj = 0.011). Dog owners also showed a significant multivariate effect compared with non-pet owners (Pillai’s Trace = 0.33, F(10, 71) = 4.22, puncorr = 0.00079) with the same significant networks (psadj < 0.017) but not the hippocampus (padj = 0.060). After controlling for global brain volume, pet ownership continued to be significant (Pillai’s Trace = 0.27, F(10, 71) = 2.63, puncorr = 0.0088) for the dorsal attention A, limbic B, and default mode A networks (psadj < 0.022).
For surface area, dog owners showed a significant multivariate effect compared with non-dog owners (Pillai’s Trace = 0.28, F(9, 72) = 3.16, puncorr = 0.0029) with significant effects in the dorsal attention A, ventral attention, limbic B, default mode A, and default mode B networks (psadj < 0.037). Dog owners also showed a significant multivariate effect compared with non-pet owners (Pillai’s Trace = 0.32, F(9, 61) = 3.14, puncorr = 0.0036) for the same significant networks (psadj < 0.022). After controlling for global surface area, pet ownership was now significant (Pillai’s Trace = 0.25, F(9, 72) = 2.68, p = 0.0096) for the same significant networks as for dog-owners (psadj < 0.048).
For cortical thickness, dog owners showed a significant multivariate effect compared with non-dog owners (Pillai’s Trace = 0.33, F(9, 72) = 3.79, puncorr = 0.00038) with significant effects in the dorsal attention A, limbic B, and default mode C networks (psadj < 0.024). Dog owners also showed a significant multivariate effect compared with non-pet owners (Pillai’s Trace = 0.33, F(9, 61) = 3.31, puncorr = 0.0024) for the same significant networks (psadj < 0.016). After controlling for global cortical thickness, pet ownership was not significant (Pillai’s Trace = 0.18, F(9, 72) = 1.62, puncorr = 0.13). For resting-state connectivity, no multivariate effects were found for the subsequent sensitivity analyses (psuncorr > 0.22).
Effects of owning multiple pets on cognitive and brain health
Cognitive health
The same MANCOVA was conducted as in the previous analyses. This resulted in a significant multivariate effect of owning multiple pets compared with owning one or no pets, Pillai’s Trace = 0.23, F(7, 81) = 3.52, padj = 0.012 (Figure 2). Multiple pet ownership was associated with better story memory (β = 0.32, 95% CI [0.11, 0.48], padj = 0.019).
Brain health
No multivariate effect of owning multiple pets was found on brain volume compared with owning one or no pets (Pillai’s Trace = 0.17, F(10, 71) = 1.42, padj = 0.95), surface area (Pillai’s Trace = 0.13, F(9, 72) = 1.15, padj = 1.00), cortical thickness (Pillai’s Trace = 0.13, F(9, 72) = 1.18, padj = 1.00), nor resting-state connectivity (Pillai’s Trace = 0.11, F(9, 72) = 1.02, padj = 1.00).
Sensitivity analyses for effects of owning multiple pets
Cognitive health
After controlling for global cognition, this analysis yielded a significant effect of owning multiple pets (Pillai’s Trace = 0.24, F(7, 81) = 3.71, padj = 0.008). In follow-up ANOVAs, story memory and spatial memory were significant (psadj < 0.034).
Brain health
After controlling for the relevant global brain integrity score, no multivariate effect of owning multiple pets was found on brain volume (Pillai’s Trace = 0.18, F(10, 71) = 1.50, padj = 0.80), surface area (Pillai’s Trace = 0.15, F(9, 72) = 1.35, padj = 1.00), cortical thickness (Pillai’s Trace = 0.14, F(9, 72) = 1.34, padj = 1.00), nor resting-state connectivity (Pillai’s Trace = 0.11, F(9, 72) = 0.98, padj = 1.00).
Exploratory analyses
Interactions with age
In these analyses, age was treated as a continuous variable. Across cognitive and brain health measures, only cortical thickness revealed interactions with age when owning multiple pets (Pillai’s Trace = 0.23, F(9, 71) = 2.29, puncorr = 0.026). Follow-up univariate tests were not significant (psuncorr > 0.17). Thus, to better understand the nature of the results, a linear discriminant analysis was conducted on the cortical thickness measures after regressing out education, race, and sex. The largest coefficients were the dorsal attention A (12.90), default mode D (8.50), and limbic A (4.95) networks. Figure 3 breaks down age into categorial groups to show the nature of the interaction. The general trend was that owning multiple pets was associated with greater cortical thickness more so in older adults than middle-aged or young adults (Figure 3). No other interactions with age were found for pet ownership (ps > 0.10), dog ownership (ps > 0.15), or owning multiple pets (ps > 0.39).
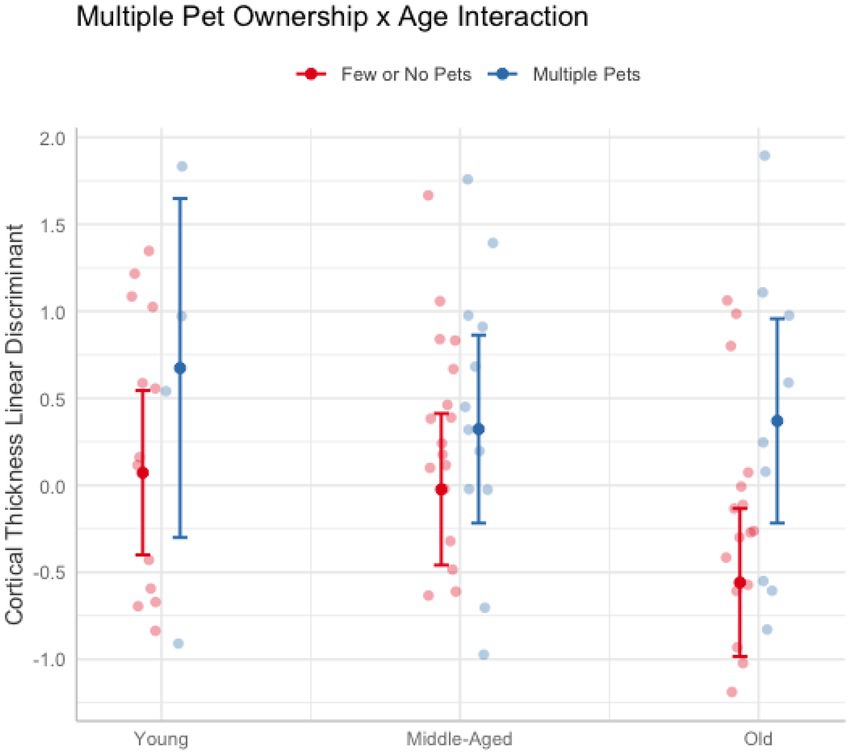
Figure 3. Plot illustrating the significant interaction between owning multiple pets and age on cortical thickness. Older adults (62 and older) who owned more than one pet (blue) had greater cortical thickness than older adults who owned one or no pets (red). The effect of multiple pet ownership was smaller in younger (aged 20–30) and middle-aged adults (aged 50–61). Error bars represent 95% confidence intervals.
BrainAGE gap
We also tested the extent that owning a pet was associated with a younger “brain age.” Chronological age was correlated with predicted age, r (81) = 0.41, p = 0.00015, 95% CI [0.21, 0.57] (Figure 4A). As in prior work (Smith et al., 2019), the BrainAGE score (i.e., the corrected difference between chronological age and predicted age) was not correlated with chronological age, r (81) = 0.00, p = 1.00 (Figure 4B). Independent t-tests were conducted to test for differential brain aging as a function of pet ownership. As can be seen in Figure 5, pet owners had a lower BrainAGE score than non-pet owners (Mean Difference = 15.10 years, t (73.28) = 3.22, puncorr = 0.0019, padj = 0058), dog owners had a lower BrainAGE score than non-pet owners (Mean Difference = 12.95 years, t (76.76) = 2.72, puncorr = 0.0082, padj = 0.025), and multiple pet owners than participants with one pet or no pets (Mean Difference = 12.56 years, t (56.69) = 2.60, puncorr = 0.012, padj = 0.035). The top 20 features that contributed to the BrainAGE score can be found in Figure 6. More than half of the top 20 consisted of cortical thickness measures in occipital, default mode, and attention networks. Thus, consistent with the notion of pet ownership as a protective factor of biological aging, owning a pet reduces one’s brain age up to 15 years, according to this metric.2
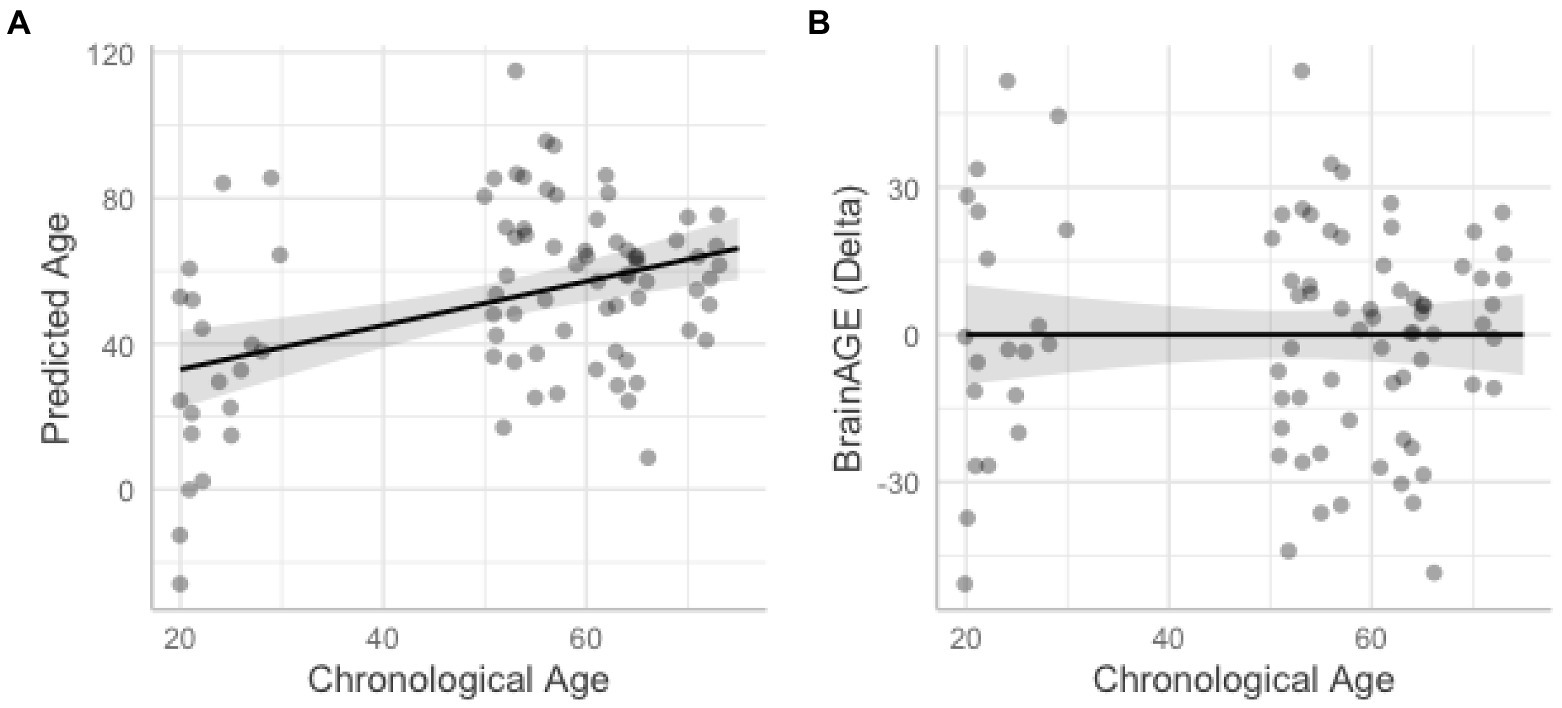
Figure 4. Scatterplots show correlations between chronological age and predicted age from the support vector machine model (A) and BrainAGE with higher difference scores representing accelerated brain aging (B). Shaded areas represent 95% confidence intervals.
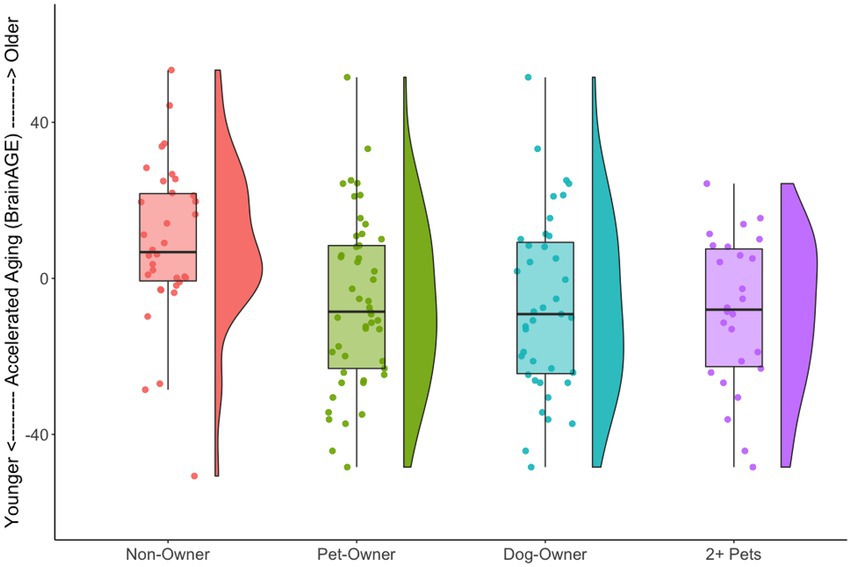
Figure 5. Raincloud plots illustrating BrainAGE scores as a function of pet ownership group. For each group, the left side shows a box plot and jittered instances of each participant and the right side shows a density plot. Non-pet owners had a higher BrainAGE score than the various pet owner groups.
Indirect effects of pet ownership relationships on cognitive and brain health
We next investigated indirect effects through physical health, subjective well-being, social well-being, and psychological distress. None were related to pet ownership (ps > 0.31), dog ownership (ps > 0.32), or owning multiple pets (ps > 0.06). Thus, we did not conduct further analyses.
Discussion
This study is the first to investigate a comprehensive analysis of the associations between pet ownership and both cognitive and brain health. In overarching multivariate analyses, we found clear support for the association between pet ownership and cognitive/brain health in an adult sample ranging up to 74 years. These effects were strongest for dog owners, followed by all pet-owners, and some effects were found for those owning multiple pets. Establishing these strong links are critical to inform neurocognitive interventions and to better understand potential brain mechanisms underlying the benefits of pet ownership.
Specific cognitive relationships with pet ownership
Although many of the cognitive domains exhibited moderate effect sizes, the most consistent relationships were found with better processing speed, attentional orienting, and episodic memory for stories. Processing speed was measured through multiple reaction time tests. In the context of pet ownership, one might need to react quickly to alter a pet’s behavior (e.g., preventing ingestion of poisonous foods or clawing at furniture). We note that these processing speed tasks tap into both cognitive and motor speed–both of which may be needed when interacting with pets.
Attentional orienting occurs when a cue in one’s environment helps guide where attention should be directed next. In the case of pet ownership, owners often need to read the cues of their pets or the environment to predict how their pet might behave and adjust their actions accordingly (e.g., tightening their grip on a leash upon seeing a nearby cat). Stories often include an ordered and contextualized sequence of events. Memory for such stories might be needed for a variety of purposes in the context of pet ownership. When communicating to others about their pets, people often do so through storytelling of interesting (or comical) events, thus requiring remembering the original event in story form. When communicating to veterinarians, stories about recent unusual behaviors and context might help to better understand the underlying medical issue. Remembering previous sequences of events with one’s pet also aids in putting ourselves in their shoes (i.e., theory of mind) to consider their point of view and predict their future actions in similar situations. For these cognitive domains, repeated and sustained use might lead to long term benefits. Moreover, attentional orienting and story memory continued to be significant even after controlling for global cognitive performance, suggesting a differentially strong and specific effect for these domains.
Specific brain relationships with pet ownership
The brain associations with pet ownership strongly align with the cognitive associations. The most robust brain networks that were associated with larger brain structures include the dorsal attention A (largely the posterior parietal), limbic B (temporal), and default mode A (medial prefrontal and parietal) networks. Whereas pet ownership showed moderately strong relationships only with brain volume, the same networks also were found for surface area and cortical thickness in the analysis comparing dog owners to non-dog owners and non-pet owners.
Collectively, these networks resemble two core brain systems involved in attention and episodic memory: the anterior temporal (AT) system and the posterior medial (PM) system (Ranganath and Ritchey, 2012). Specifically, the anterior portion of the default mode A network and the lateral temporal lobe from the limbic B network make up the AT system. This system is purportedly involved in processing familiarity signals of memory, emotional processing, and social cognition to aid in making inferences about the relevance or intentions of another person, animal, or other object (Ranganath and Ritchey, 2012). In contrast, the PM system has been implicated in retrieving past recollections and simulating possible future events (Ritchey and Cooper, 2020). Both processes involve creating a type of situation model that binds temporal, spatial, and sensory details (like in a story). Thus, the PM system is thought to create such mental situation models and apply those models to the current situation. Through functional interactions with the AT system, a person can create situation models of their own potential actions and those of others. Pet owners, especially dog owners, often attempt to read signals from their pets, create a mental model through anthropomorphism, and predict pets’ current and future needs.
Effects of owning more than one pet
The present study proposed a novel hypothesis that owning more than one pet may confer additional benefits than owning one pet. Specifically, we proposed that owning more than one pet requires additional cognitive resources to juggle the responsibilities and understand the unique needs of each pet. Although we found a significant multivariate effect on cognition, the effect sizes were much smaller and only story memory survived multiple comparisons correction at the single-domain level. None of the main effects between owning multiple pets and the brain measures were significant. However, we did find a multivariate effect for an interaction between chronological age and owning multiple pets on cortical thickness overall. This interaction appeared to be due to greater effects of owning multiple pets as one gets older. Older pet owners might have more or longer opportunities to interact with pets than younger pet owners, potentially magnifying the effects. Older adults tend to have fewer social roles compared to younger adults and therefore may benefit more from the social, emotional, and physical aspects of pet ownership.
Pets as protective or selected by the cognitively able?
One criticism of the human-animal interaction literature when using cross-sectional data is the inability to distinguish the direction of the causal relationships having to do with pet ownership. For example, we show here that pet owners (and dog owners, specifically) have better cognitive and brain health than non-pet owners. Our theoretical framework is based on the notion that owning pets leads to repeated and sustained use of specific cognitive processes, thereby strengthening those processes across the adult lifespan. This argument assumes that pet ownership, in part, causes better cognitive and brain health. However, another interpretation is that people who have better cognitive and brain health are more likely to adopt pets. Perhaps only people that have awareness that they can handle caring for a pet choose to do so whereas some people know that the responsibility is too great for them.
The evidence shown here supports both possibilities. On the one hand, most of the associations were not specific to older adults but were also found in younger adults who have not had as long to benefit from sustained pet ownership and might be at their peak cognitive abilities (Hartshorne and Germine, 2015). These main effects might be interpreted as evidence for the “cognitively able select pets” argument. On the other hand, cognitive training tasks and physical exercise have shown that even young adults’ cognition can be improved (Jaeggi et al., 2008; Stroth et al., 2009), suggesting that pet ownership has potential to still serve as a real-world cognitive training scenario. The decelerated brain aging (i.e., lower BrainAGE score) in pet owners might be interpreted as favoring the “enhancement” argument because people do not have direct access to information about their biological age. Interestingly, a study investigating the relationship between one’s subjective age (i.e., how old one feels they are) explained some small variance in BrainAGE (R2 = 0.10; Kwak et al., 2018). The aforementioned interaction between multiple pet ownership and cortical thickness also might be interpreted as favoring the “enhancement” argument because the effects were specific to older adults and not to all age groups that consist of adults with equally high or higher cognitive abilities. Lastly, and perhaps the strongest evidence for enhancement effects, is that many of the specific cognitive and brain effects were significant after controlling for global cognition and global brain health. Thus, while the direction of the causal effects remain limited in this cross-sectional study, we feel the balance of evidence favors an argument that pet ownership enhances cognitive and brain health.
Limitations
In addition to being unable to fully tease out the causal role that pet ownership has on cognitive and brain health, other limitations are inherent in these analyses. First, we did not have information about attachment to pets or whether the participant was the primary caretaker, both of which might serve as moderating factors that influence dedication and attention to the needs of one’s pet. Second, we did not have information on the age of the pets. Caring for a younger animal versus an older animal would entail very different behaviors and, thus, brain benefits. Lastly, we did not have information about the length of time participants have owned pets. Our theoretical framework assumes that pet ownership would have to be long enough to see sustained changes in cognition and brain health but the time needed to show such effects remain unknown.
Conclusion and implications
The present study provides strong evidence that pet ownership is associated with better cognitive and brain health with the strongest effects for dog owners. Such evidence is needed to support the rationale and motivation for lifespan interventions to improve brain health before overt decline is present. Currently, nearly every cognitive intervention study using pets or trained animals is aimed at older adults with cognitive decline. If such interventions also show benefits in cognitively normal older adults, it would reveal a type of far-transfer effect to multiple domains of cognition and multiple brain networks, including those involved in processing speed, attention, and episodic memory. It may be that community-based interventions to involve healthy older adults with pets (fostering pets, volunteering at shelters, or pet-assisted exercise such as dog walking) would enhance lifespan cognitive health and meet community-based needs. Notably, while similar life experiences such as raising a child or grandchild appear to have some overlapping qualities as pet ownership, some research suggests that raising children costs rather than aids cognitive health and mortality (Burn and Szoeke, 2015; Bonsang and Skirbekk, 2022). Thus, pet ownership might have multiple unique benefits for adults of all ages.
Data availability statement
The datasets presented in this article are not readily available because the data collected in this study are owned by The University of Alabama. Interested parties must fill out a data use agreement with UA to access the data or collaborate with the lead author. Requests to access the datasets should be directed to aW1tY2Rvbm91Z2hAdWEuZWR1.
Ethics statement
The studies involving human participants were reviewed and approved by the institutional review board at The University of Alabama. The patients/participants provided their written informed consent to participate in this study.
Author contributions
IM: conceptualization, data curation, formal analysis, investigation, methodology, project administration, visualization, writing–original draft, reviewing, and editing. HE: writing–original draft, reviewing, and editing. NS and RA: conceptualization, writing–reviewing, and editing. All authors contributed to the article and approved the submitted version.
Funding
This research was funded by The University of Alabama through the College Academy of Research, Scholarship, and Creative Activity to IM and the University of Alabama, Birmingham (#A18-0284-001) to IM. No funds were received for open access publication fees.
Acknowledgments
We are grateful to our own pets for clearly enhancing our lives and quite possibly also our cognitive and brain health: Gordon Shumway and Cosmo (IM), Tiger Lily and Tink (HE), Muffet and Luke (NS), and Chloe Dog (RA).
Conflict of interest
The authors declare that the research was conducted in the absence of any commercial or financial relationships that could be construed as a potential conflict of interest.
Publisher’s note
All claims expressed in this article are solely those of the authors and do not necessarily represent those of their affiliated organizations, or those of the publisher, the editors and the reviewers. Any product that may be evaluated in this article, or claim that may be made by its manufacturer, is not guaranteed or endorsed by the publisher.
Supplementary material
The Supplementary material for this article can be found online at: https://www.frontiersin.org/articles/10.3389/fnagi.2022.953889/full#supplementary-material
Footnotes
1. ^Because perceived SES (measured via the MacArthur Scale of Subjective Socioeconomic Status) significantly differed between groups, analyses were re-conducted using this measure as a post-hoc covariate. Perceived SES was not related to the cognitive or brain variables and controlling for it did not alter the results.
2. ^BrainAGE analyses often are created using only brain metrics rather than a composite of cognitive and brain metrics. Although brain functioning underlies cognitive performance, a one-to-one mapping of our brain metrics to cognition does not exist. Thus, cognitive performance ultimately reveals additional brain functioning. Nonetheless, re-calculating the BrainAGE scores continued to reveal group differences in pet ownership (psadj < 0.040).
References
Albright, A. E., Cui, R., and Allen, R. S. (2022). Pet ownership and mental and physical health in older White and black males and females. Int. J. Environ. Res. Public Health 19:5655. doi: 10.3390/ijerph19095655
American Pet Products Association (2021). Pet Industry Market Size, Trends & Ownership Statistics. Available at: https://www.americanpetproducts.org/press_industrytrends.asp
Avants, B., Tustison, N. J., and Song, G. (2009). Advanced normalization tools: V1.0. Insight J. 2, 1–35. doi: 10.54294/uvnhin
Beck, A. M. (2014). The biology of the human–animal bond. Anim. Front. 4, 32–36. doi: 10.2527/af.2014-0019
Beetz, A., Uvnäs-Moberg, K., Julius, H., and Kotrschal, K. (2012). Psychosocial and psychophysiological effects of human-animal interactions: the possible role of oxytocin. Front. Psychol. 3:234. doi: 10.3389/fpsyg.2012.00234
Bherer, L., Erickson, K. I., and Liu-Ambrose, T. (2013). A review of the effects of physical activity and exercise on cognitive and brain functions in older adults. J. Aging Res. 2013, 1–8. doi: 10.1155/2013/657508
Bickart, K. C., Dickerson, B. C., and Barrett, L. F. (2014). The amygdala as a hub in brain networks that support social life. Neuropsychologia 63, 235–248. doi: 10.1016/j.neuropsychologia.2014.08.013
Bono, A. V., Benvenuti, C., Buzzi, M., Ciatti, R., Chiarelli, V., Chiambretto, P., et al. (2015). Effects of animal assisted therapy (AAT) carried out with dogs on the evolution of mild cognitive impairment. J. Gerontol. Geriatr. 63, 32–36.
Bonsang, E., and Skirbekk, V. (2022). Does childbearing affect cognitive health in later life? Evidence from an instrumental variable approach. Demography 59, 975–994. doi: 10.1215/00703370-9930490
Branson, S., Boss, L., Cron, S., and Kang, D.-H. (2016). Examining differences between homebound older adult pet owners and non-pet owners in depression, systemic inflammation, and executive function. Anthrozoös 29, 323–334. doi: 10.1080/08927936.2016.1152764
Buckner, R. L., Head, D., Parker, J., Fotenos, A. F., Marcus, D., Morris, J. C., et al. (2004). A unified approach for morphometric and functional data analysis in young, old, and demented adults using automated atlas-based head size normalization: reliability and validation against manual measurement of total intracranial volume. NeuroImage 23, 724–738. doi: 10.1016/j.neuroimage.2004.06.018
Burn, K., and Szoeke, C. (2015). Is grandparenting a form of social engagement that benefits cognition in ageing? Maturitas 80, 122–125. doi: 10.1016/j.maturitas.2014.10.017
Cohen, S., Kamarck, T., and Mermelstein, R. (1983). A global measure of perceived stress. J. Health Soc. Behav. 24:385. doi: 10.2307/2136404
Cole, J. H., and Franke, K. (2017). Predicting age using neuroimaging: innovative brain ageing biomarkers. Trends Neurosci. 40, 681–690. doi: 10.1016/j.tins.2017.10.001
Colombo, G., Buono, M. D., Smania, K., Raviola, R., and De Leo, D. (2006). Pet therapy and institutionalized elderly: a study on 144 cognitively unimpaired subjects. Arch. Gerontol. Geriatr. 42, 207–216. doi: 10.1016/j.archger.2005.06.011
de Lange, A. M. G., and Cole, J. H. (2020). Commentary: correction procedures in brain-age prediction. NeuroImage: Clinical 26:102229. doi: 10.1016/j.nicl.2020.102229
Desmet, C., van der Wiel, A., and Brass, M. (2017). Brain regions involved in observing and trying to interpret dog behaviour. PLoS One 12:e0182721. doi: 10.1371/journal.pone.0182721
Diener, E., Emmons, R. A., Larsen, R. J., and Griffin, S. (1985). The satisfaction with life scale. J. Pers. Assess. 49, 71–75. doi: 10.1207/s15327752jpa4901_13
Drozdick, L. W., Raiford, S. E., Wahlstrom, D., and Weiss, L. G. (2018). “The Wechsler adult intelligence scale—fourth edition and the Wechsler memory scale—fourth edition,” in Contemporary Intellectual Assessment: Theories, Tests, and Issues. eds. D. P. Flanagan and E. M. McDonough (New York, NY: The Guilford Press), 486–511.
Fan, J., McCandliss, B. D., Sommer, T., Raz, A., and Posner, M. I. (2002). Testing the efficiency and independence of attentional networks. J. Cogn. Neurosci. 14, 340–347. doi: 10.1162/089892902317361886
Franke, K., and Gaser, C. (2019). Ten years of brain age as a neuroimaging biomarker of brain aging: what insights have we gained? Front. Neuro 10:789. doi: 10.3389/fneur.2019.00789
Gallagher, H. L., and Frith, C. D. (2003). Functional imaging of theory of mind. Trends Cogn. Sci. 7, 77–83. doi: 10.1016/S1364-6613(02)00025-6
Grady, C. (2012). The cognitive neuroscience of ageing. Nat. Rev. Neuroci. 13, 491–505. doi: 10.1038/nrn3256
Hart, L. A. (1995). “The role of pets in enhancing human well-being: effects for older people,” in The Waltham Book of Human–Animal Interaction. ed. I. Robinson (Exeter: Pergamon), 19–31. doi: 10.1016/B978-0-08-042284-8.50009-8
Hartshorne, J. K., and Germine, L. T. (2015). When does cognitive functioning peak? The asynchronous rise and fall of different cognitive abilities across the life span. Psychol. Sci. 26, 433–443. doi: 10.1177/0956797614567339
Hayama, S., Chang, L., Gumus, K., King, G. R., and Ernst, T. (2016). Neural correlates for perception of companion animal photographs. Neuropsychologia 85, 278–286. doi: 10.1016/j.neuropsychologia.2016.03.018
Headey, B., and Grabka, M. M. (2006). Pets and human health in Germany and Australia: national longitudinal results. Soc. Indic. Res. 80, 297–311. doi: 10.1007/s11205-005-5072-z
Hedden, T., Park, D. C., Nisbett, R., Ji, L. J., Jing, Q., and Jiao, S. (2002). Cultural variation in verbal versus spatial neuropsychological function across the life span. Neuropsychology 16, 65–73. doi: 10.1037/0894-4105.16.1.65
Hertzog, C., Kramer, A. F., Wilson, R. S., and Lindenberger, U. (2008). Enrichment effects on adult cognitive development. Psychol. Sci. Public Interest 9, 1–65. doi: 10.1111/j.1539-6053.2009.01034.x
Hostinar, C. E., and Gunnar, M. R. (2015). Social support can buffer against stress and shape brain activity. AJOB Neurosci. 6, 34–42. doi: 10.1080/21507740.2015.1047054
Hughes, M. J., Verreynne, M. L., Harpur, P., and Pachana, N. A. (2020). Companion animals and health in older populations: a systematic review. Clin. Gerontol. 43, 365–377. doi: 10.1080/07317115.2019.1650863
Jaeggi, S. M., Buschkuehl, M., Jonides, J., and Perrig, W. J. (2008). Improving fluid intelligence with training on working memory. Proc. Natl. Acad. Sci. U. S. A. 105, 6829–6833. doi: 10.1073/pnas.0801268105
Kanamori, M., Suzuki, M., Yamamoto, K., Kanda, M., Matsui, Y., Kojima, E., et al. (2001). A day care program and evaluation of animal-assisted therapy (AAT) for the elderly with senile dementia. Am. J. Alzheimers Dis. 16, 234–239. doi: 10.1177/153331750101600409
Keshavan, A., Datta, E., McDonough, I. M., Madan, C. R., Jordan, K., and Henry, R. G. (2018). Mindcontrol: a web application for brain segmentation quality control. NeuroImage 170, 365–372. doi: 10.1016/j.neuroimage.2017.03.055
Khan, M., and Farrag, N. (2000). Animal-assisted activity and infection control implications in a healthcare setting. J. Hosp. Infect. 46, 4–11. doi: 10.1053/jhin.2000.0785
Ko, H.-J., Youn, C.-H., Kim, S.-H., and Kim, S.-Y. (2016). Effect of pet insects on the psychological health of community-dwelling elderly people: a single-blinded, randomized, controlled trial. Gerontol. 62, 200–209. doi: 10.1159/000439129
Kwak, S., Kim, H., Chey, J., and Youm, Y. (2018). Feeling how old I am: Subjective age is associated with estimated brain age. Front. Aging Neurosci. 10:168. doi: 10.3389/fnagi.2018.00168
Ling, D. S., Kelly, M., and Diamond, A. (2016). “Human-animal interaction and the development of executive functions”, in The Social Neuroscience of Human-Animal Interaction. eds. L. S. Freund, S. McCune, L. Esposito, N. R. Gee, and P. McCardle (Washington, DC, United States: American Psychological Association).
McDonough, I. M., Haber, S., Bischof, G. N., and Park, D. C. (2015). The synapse project: engagement in mentally challenging activities enhances neural efficiency. Restor. Neurol. Neurosci. 33, 865–882. doi: 10.3233/RNN-150533
McDonough, I. M., Letang, S. K., and Stinson, E. A. (2019). Dementia risk elevates brain activity during memory retrieval: a functional MRI analysis of middle aged and older adults. J. Alzheimers Dis. 70, 1005–1023. doi: 10.3233/JAD-190035
Mormann, F., Dubois, J., Kornblith, S., Milosavljevic, M., Cerf, M., Ison, M., et al. (2011). A category-specific response to animals in the right human amygdala. Nat. Neurosci. 14, 1247–1249. doi: 10.1038/nn.2899
Nyberg, L., Lövdén, M., Riklund, K., Lindenberger, U., and Bäckman, L. (2012). Memory aging and brain maintenance. Trends Cogn. Sci. 16, 292–305. doi: 10.1016/j.tics.2012.04.005
Park, D. C., and Reuter-Lorenz, P. (2009). The adaptive brain: aging and neurocognitive scaffolding. Ann. Rev. Psychol. 60, 173–196. doi: 10.1146/annurev.psych.59.103006.093656
Payne, T. J., Andrew, M., Butler, K. R., Wyatt, S. B., Dubbert, P. M., and Mosley, T. H. (2012). Psychometric evaluation of the interpersonal support evaluation list–short form in the ARIC study cohort. SAGE Open 2:215824401246192. doi: 10.1177/2158244012461923
Perkins, J., Bartlett, H., Travers, C., and Rand, J. (2008). Dog-assisted therapy for older people with dementia: a review. Aust. J. Ageing 27, 177–182. doi: 10.1111/j.1741-6612.2008.00317.x
Porcelli, S., Van Der Wee, N., van der Werff, S., Aghajani, M., Glennon, J. C., van Heukelum, S., et al. (2019). Social brain, social dysfunction and social withdrawal. Neurosci. Biobehav. Rev. 97, 10–33. doi: 10.1016/j.neubiorev.2018.09.012
Puce, A., Latinus, M., Rossi, A., Dasilva, E., Parada, F., Love, S., et al. (2015). “Neural bases for social attention in healthy humans,” in The Many Faces of Social Attention (Cham: Springer), 93–127.
R Core Team (2021). R: A Language and Environment for Statistical Computing. R Foundation for Statistical Computing, Vienna, Austria. Available at: https://www.R-project.org/
Radloff, L. S. (1977). The CES-D scale: a self-report depression scale for research in the general population. Appl. Psychol. Meas. 1, 385–401. doi: 10.1177/014662167700100306
Ranganath, C., and Ritchey, M. (2012). Two cortical systems for memory-guided behaviour. Nat. Rev. Neurosci. 13, 713–726. doi: 10.1038/nrn3338
Reitan, R., (1979). Manual for Administration of Neuropsychological Test Batteries for Adults and Children. Tucson, AZ: Reitan Neuropsychology Laboratories.
Ritchey, M., and Cooper, R. A. (2020). Deconstructing the posterior medial episodic network. Trends Cogn. Sci. 24, 451–465. doi: 10.1016/j.tics.2020.03.006
Russell, D. W. (1996). UCLA loneliness scale (version 3): reliability, validity, and factor structure. J. Pers. Assess. 66, 20–40. doi: 10.1207/s15327752jpa6601_2
Salthouse, T. A., and Babcock, R. L. (1991). Decomposing adult age differences in working memory. Dev. Psychol. 27, 763–776.
Simmons, J. P., Nelson, L. D., and Simonsohn, U. (2021). Pre-registration: why and how. J. Cons. Psychol. 31, 151–162. doi: 10.1002/jcpy.1208
Smith, S. M., Vidaurre, D., Alfaro-Almagro, F., Nichols, T. E., and Miller, K. L. (2019). Estimation of brain age delta from brain imaging. NeuroImage 200, 528–539. doi: 10.1016/j.neuroimage.2019.06.017
Spreng, R. N., Mar, R. A., and Kim, A. S. (2009). The common neural basis of autobiographical memory, prospection, navigation, theory of mind, and the default mode: a quantitative meta-analysis. J. Cogn. Neurosci. 21, 489–510. doi: 10.1162/jocn.2008.21029
Stasi, M. F., Amati, D., Costa, C., Resta, D., Senepa, G., Scarafioti, C., et al. (2004). Pet-therapy: a trial for institutionalized frail elderly patients. Arch. Gerontol. Geriatr. 38, 407–412. doi: 10.1016/j.archger.2004.04.052
Stoeckel, L. E., Palley, L. S., Gollub, R. L., Niemi, S. M., and Evins, A. E. (2014). Patterns of brain activation when mothers view their own child and dog: an fMRI study. PLoS One 9:e107205. doi: 10.1371/journal.pone.0107205
Stroth, S., Hille, K., Spitzer, M., and Reinhardt, R. (2009). Aerobic endurance exercise benefits memory and affect in young adults. Neuropsychol. Rehabil. 19, 223–243. doi: 10.1080/09602010802091183
Tariq, S. H., Tumosa, N., Chibnall, J. T., Perry, M. H. III, and Morley, J. E. (2006). Comparison of the Saint Louis university mental status examination and the mini-mental state examination for detecting dementia and mild neurocognitive disorder—a pilot study. The Am. J. Geriatr. Psychiatry. 14, 900–910. doi: 10.1097/01.JGP.0000221510.33817.86
Taylor, H. A. Jr., Wilson, J. G., Jones, D. W., Sarpong, D. F., Srinivasan, A., Garrison, R. J., et al. (2005). Toward resolution of cardiovascular health disparities in African Americans: design and methods of the Jackson heart study. Ethn. Dis. 15, S6–S4.
Ware, J. E., and Sherbourne, C. D. (1992). The MOS 36-ltem short-form health survey (SF-36). Med. Care 30, 473–483. doi: 10.1097/00005650-199206000-00002
Warttig, S. L., Forshaw, M. J., South, J., and White, A. K. (2013). New, normative, English-sample data for the short form perceived stress scale (PSS-4). J. Health Psychol. 18, 1617–1628. doi: 10.1177/1359105313508346
Watson, D., Clark, L. A., and Tellegen, A. (1988). Development and validation of brief measures of positive and negative affect: the PANAS scales. J. Pers. Soc. Psychol. 54, 1063–1070. doi: 10.1037/0022-3514.54.6.1063
Wells, D. L. (2007). Domestic dogs and human health: an overview. Brit. J. Health Psychol. 12, 145–156. doi: 10.1348/135910706X103284
Whitfield-Gabrieli, S., and Nieto-Castanon, A. (2012). CONN: a functional connectivity toolbox for correlated and Anticorrelated brain networks. Brain Connect. 2, 125–141. doi: 10.1089/brain.2012.0073
Wilkinson, G. S., and Robertson, G. J., (2006). Wide Range Achievement Test (WRAT4). Lutz, FL: Psychological Assessment Resources.
Yeo, B. T., Krienen, F. M., Sepulcre, J., Sabuncu, M. R., Lashkari, D., Hollinshead, M., et al. (2011). The organization of the human cerebral cortex estimated by intrinsic functional connectivity. J. Neurophysiol. 106, 1125–1165. doi: 10.1152/jn.00338.2011
Keywords: brain age gap, independent component analysis, aging, protective factors (resilience), magnetic resonance imaging, resting state-fMRI
Citation: McDonough IM, Erwin HB, Sin NL and Allen RS (2022) Pet ownership is associated with greater cognitive and brain health in a cross-sectional sample across the adult lifespan. Front. Aging Neurosci. 14:953889. doi: 10.3389/fnagi.2022.953889
Edited by:
Mary Ann Ottinger, University of Houston, United StatesReviewed by:
Suwei Wang, Duke University, United StatesLinda Chang, University of Maryland, Baltimore, United States
Copyright © 2022 McDonough, Erwin, Sin and Allen. This is an open-access article distributed under the terms of the Creative Commons Attribution License (CC BY). The use, distribution or reproduction in other forums is permitted, provided the original author(s) and the copyright owner(s) are credited and that the original publication in this journal is cited, in accordance with accepted academic practice. No use, distribution or reproduction is permitted which does not comply with these terms.
*Correspondence: Ian M. McDonough, aW1tY2Rvbm91Z2hAdWEuZWR1