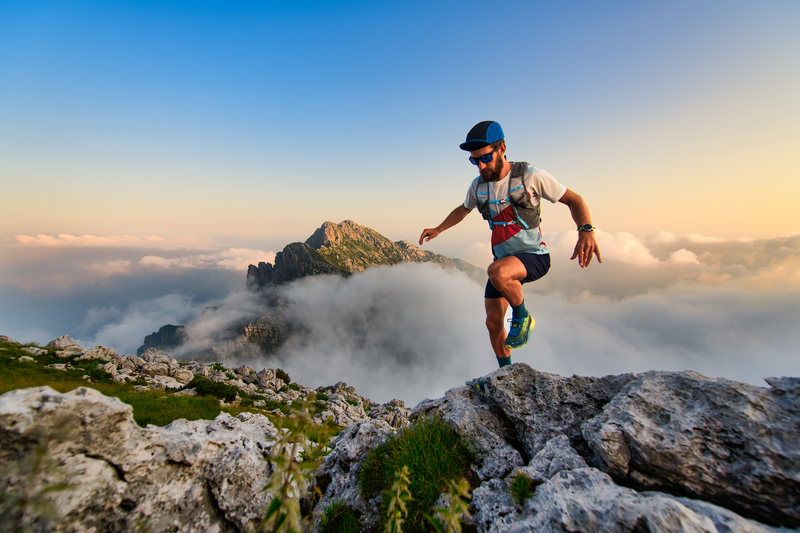
94% of researchers rate our articles as excellent or good
Learn more about the work of our research integrity team to safeguard the quality of each article we publish.
Find out more
SYSTEMATIC REVIEW article
Front. Aging Neurosci. , 01 September 2022
Sec. Neurocognitive Aging and Behavior
Volume 14 - 2022 | https://doi.org/10.3389/fnagi.2022.949763
Background: Cerebral small vessel disease (CSVD) is prevalent in the elderly and leads to an increased risk of cognitive impairment and dementia. The volume of white matter hyperintensities (WMHs) increases with age, which affects cognition.
Objective: To explore the relationship between WMH volume and cognitive decline in patients with CSVD.
Methods: We performed a systematic search of PubMed, Embase, and the Web of Science databases from their respective creation dates to the 5 May 2022 to identify all the clinical studies on either mild cognitive impairment (MCI) or dementia in regards to WMH volume in CSVD.
Results: White matter hyperintensities was associated with the risk of both the MCI and dementia, with a 35% increased risk [relative risk (RR) = 1.35; (95% CI: 1.01–1.81)] of progression from cognitively unimpaired (CU) to MCI (six studies, n = 2,278) and a 49% increased risk [RR = 1.49; (95% CI: 1.21–1.84)] of progression to dementia (six studies, n = 6,330). In a subgroup analysis, a follow-up period of over 5 years increased the risk of MCI by 40% [RR = 1.40; (95% CI: 1.07–1.82)] and dementia by 48% [RR = 1.48; (95% CI: 1.15–1.92)].
Conclusion: White matter hyperintensities was found to be substantially correlated with the risk of cognitive impairment. Furthermore, cognitive decline was found to be a chronic process, such that WMH predicted the rate of cognitive decline in CSVD beyond 5 years. The cognitive decline observed in patients with WMH may, therefore, be minimized by early intervention.
Cerebrovascular small vessel disease (CSVD) is an age-related microvascular disease affecting the cerebral small arteries, small veins, and capillaries. The incidence of CSVD is related to the slow accumulation of tissue damage, which primarily manifests as static focal cerebrovascular diseases such as static lacunar infarction (LI), cerebral microbleeds (CMBs), local leukoaraiosis, and progressive cognitive dysfunction (Zhang et al., 2010), which are the leading causes of both the cognitive impairment and vascular dementia (VD) (Cipollini et al., 2019). The identification of CSVD most commonly relies on MRI markers, including white matter hyperintensities (WMHs), LI, enlarged perivascular spaces (EPVSs), CMBs, and brain atrophy (Pantoni, 2010), all of which have variably been associated with cognitive performance (Chojdak-Łukasiewicz et al., 2021). White matter is comprised of the structural connections between areas of the brain's gray matter. These white matter tracts carry information within the brain to sustain a variety of cognitive functions. Consequently, if white matter is disrupted, information transmission is disrupted. Studies have shown that a larger WMH volume is associated with slower local network transfer efficiency and information processing speed (Hilal et al., 2021). Furthermore, in the white matter tracts, which are important for information processing, having a greater WMH load was associated with slower cognitive speed (Vergoossen et al., 2021). Therefore, this study intended to investigate the effect of WMH volume on cognitive function. By systematically reviewing the existing literature, we sought to identify the short- and long-term incidence of WMH volume in subjects with CSVD and its association with cognitive impairment and dementia.
This was a quantitative analysis of the relationship between brain WMH volume and cognition in CSVD focusing on cohort studies that screened patients with CSVD (specifically nondementia, or other diseases that cause high signal changes in white matter, such as nonmajor vascular stroke or multiple sclerosis). We analyzed data from 14 studies on the impact of brain WMH volume on the risk of cognitive impairment to investigate whether there exists a link between WMH volume and cognitive impairment in CSVD.
This meta-analysis was conducted on literature obtained from the databases such as PubMed, Embase, and the Web of Science. To assess the interactions between WMH volume and cognition, the literature search was conducted using the keywords such as “cognition,” “dementias,” and “mild cognitive impairment” in combination with either “white matter hyperintensities volume” or “WMH.” We searched all the entries published up until 5 May 2022. All the searches were conducted by combining the Medical Subject Headings (MeSHs) terms and free text. We also screened any relevant references, including related review articles that were referred to in each publication.
We included both the observational cohort and case–control studies. The included studies determined brain WMH by MRI and white matter hyperintensity volume was calculated by FSL, MATLAB, or other computer segmentation software. Patients were diagnosed as either cognitively unimpaired (CU) or mild cognitive impairment (MCI) based on both the clinical assessment of their functional status in daily life and neuropsychological testing. Participants were excluded if they had a history of dementia, stroke, or other neurological problems. For the analysis of the prevalence of cognitive impairment and dementia in patients with a high white matter signal in the context of CSVD, articles with unclear baseline cognitive levels, i.e., studies without a clear “CU” or “MCI” at baseline, were excluded. There were no differences in either the gender distribution or education level between groups. Reported odds ratios (ORs), relative risks (RRs), and hazard ratios (HRs) were covered, and literature containing only the correlation coefficient values β and SE was excluded. Additionally, this study excluded case reports, cross-sectional studies, animal studies, and reviews that did not contain the original data.
The following data were extracted: authors, year of publication, sample size, study design, duration of follow-up, patient source, outcome measures, odds ratio (OR), relative risk (RR), hazards ratio (HR), and primary data. Effect estimates adjusted for covariates such as age, sex, and education were analyzed. If no OR, RR, or HR was reported, they were calculated from the original data. Studies were excluded if neither the raw data nor the OR/RR/HR was provided.
The RR and 95% CI were calculated using the R 4.2.0 “meta” package to summarize the RR/OR/HR. The total pooled effects were based on estimating cohort-specific effects. Heterogeneity across estimates was evaluated by the Cochran's Q statistic [a P-value assessed using I2 (P-value < 0.05 and I2 > 50% were considered to be indicative of statistically significant)]. If there was little evidence of heterogeneity between studies (i.e., from Cochran's I2 statistics < 50%), the fixed effects models were used to pool effect estimates. If there was heterogeneity between studies, we used the random effects models and probed for sources of heterogeneity through sensitivity analysis. Subgroup analyses were performed in accordance with the baseline cognitive level of subjects and their follow-up progression of cognitive impairment. We assessed publication bias using Egger's test. If statistically significant publication bias was found, the “trim-and-fill” method was utilized to adjust for the bias. The methods and results reported in this meta-analysis have been based on the Preferred Reporting Items for Systematic Reviews and Meta-Analyses (PRISMA) (Moher et al., 2009) guidelines. The nine star Newcastle–Ottawa Scale (NOS; accessed via http://www.ncbi.nlm.nih.gov/books/NBK35156/) (Stang, 2010) was used to appraise the quality of the cohort studies based on three dimensions: the selection of study subjects, the comparability of exposure groups, and the measurements of the outcomes of interest. Studies rated nine stars were considered high-quality studies. Studies rated either seven or eight stars were considered to be of moderately high quality. A rating below seven stars was deemed to be low quality.
A total of 6,177 literature entries from PubMed, Embase, and the Web of Science were retrieved. A total of 134 reports remained after the initial screening by removing duplicate literature items, old literature, reviews, animal experiments, etc., that failed to meet the inclusion criteria. Finally, leaving 14 items of literature are to be included for full-text reading and analysis (Figure 1). Six of these 14 studies were considered high quality, while six studies were considered medium-quality articles (Table 1).
A total of 10,444 enrolled patients participated. It was determined that 999 of the 10,444 (10%) participants developed a change in their cognitive diagnosis from baseline to follow-up. Of these patients, 7,474 patients were CU at baseline, 367 patients were patients with MCI, and 2,603 patients were CU- and MCI-promiscuous enrollees. Among them, 335 (4.48%) participants had incident MCI, while 368 (4.92%) participants had incident dementia (NAD = 211, AD = 157), the latter of which was comprised of 189 patients with CU, 103 patients with MCI, and 76 patients with CU + MCI.
The 14 cognition-related studies differed in their approaches used for cognitive testing. Nine studies used the Mini-Mental State Examination (MMSE), while four studies applied the Clinical Dementia Rating (CDR; Table 2). Cognitive function was assessed in all the participants at both the baseline and the end of follow-up.
According to the time of follow-up, we divided the included literature into two subgroups: more than 5 years and <5 years (Table 2). Studies with new-onset MCI had a mean follow-up time of 5.6 years. The mean follow-up time for the progression from baseline CU to dementia was 6.2 years, and the mean follow-up time for the conversion from baseline MCI to dementia was 4.5 years.
WMH was found to increase the risk of both the MCI and dementia. The cognitive score-based analysis conducted in the present study showed an association between WMH and both the MCI and dementia, where the risk of MCI was 21% greater [RR = 1.21; (95% CI: 1.01–1.45), P = 0.009], and that of dementia was 32% greater [RR = 1.32; (95% CI: 1.09–1.59), P < 0.001; Figure 2]. There was, however, marked heterogeneity between the studies (MCI: I2 = 63%; dementia: I2 = 72%). Subgroup analysis by the baseline level of cognition showed a 35% [RR = 1.35; (95% CI: 1.01–1.81)] increased risk of progression from CU to MCI and risk of dementia by 49% [RR = 1.49; (95% CI: 1.21–1.84)], but no increased risk of progression of dementia in patients with MCI [RR = 1.01; (95% CI: 0.99–1.04); Figure 3].
In a subgroup analysis by the duration of follow-up, patients with >5 years of follow-up showed a higher risk of developing MCI and dementia than patients with a follow-up period of fewer than 5 years. A follow-up period of over 5 years increased the risk of MCI by 40% [RR = 1.40; (95% CI: 1.07–1.82)] and dementia by 48% [RR = 1.48; (95% CI: 1.15–1.92)]. No association between MCI and dementia progression was observed in subgroups with less than a 5-year period of follow-up [MCI: RR = 1.03; (95% CI: 0.99–1.06); dementia: RR = 1.08; (95% CI: 0.92–1.28); Figure 4].
The result of Egger's test detected publication bias (t = 6.94, df = 16, P-value < 0.0001). This was corrected by using the “trim-and-fill” method (Figure 5), and the effect size after simple compliment was RR = 1.0475 (95% CI: 0.8742–1.2551). Observably, the funnel plot is approximately symmetrical, and there was a certain publication bias, but it had no substantial impact on the overall results. Sensitivity analysis found no outliers (Figure 6). However, the progression of the disease was protracted; only 16% (290/1,795) of participants progressed to an MCI diagnosis after a mean follow-up of 6 years, while 3% (143/4,629) of participants progressed from CU to dementia.
Figure 6. Sensitivity analysis of the included literature on the correlation between WMH volume and cognition.
The types of brain markers associated with CSVD include WMH, LI, EPVS, CMB, and brain atrophy (Heiland et al., 2021). CSVD is common in older adults (Kim et al., 2018) and is an important cause of cognitive and motor impairments (Willey et al., 2013). Studies have found that the overall volume of WMH increases with age (Franchetti et al., 2020), and the presumed mechanism behind this is that CSVD disrupts the structural connectivity of large-scale brain networks, thereby impairing the ability of the brain to efficiently integrate neural processes (Du et al., 2020). Some studies have suggested that WMH interferes with these brain networks by either directly or indirectly reducing brain region-specific functional connectivity (Langen et al., 2018). Various imaging and clinical studies have shown that white matter damage is closely associated with cognitive impairment and can increase the prevalence of neuropsychiatric disorders (Clancy et al., 2021), such as major depressive disorder, bipolar disorder, and schizophrenia. Studies have also shown that the effect of WMH on brain function varies according to its localization (Portet et al., 2012). For example, periventricular WMH (PVWMH) and deep WMH (DWMH) have distinct functional and clinical relevance. PVWMH is associated with impaired cognitive function, especially processing speed and executive function (Debette and Markus, 2010; Griffanti et al., 2018), while DWMH is associated with impairments in basic activities of daily living (Park et al., 2022).
This meta-analysis included 14 studies comprised of a total of 10,444 participants. Conjoint analysis showed that WMH volume was tied to the risk of cognitive impairment. To the best of our knowledge, this is the first meta-analysis conducted to investigate the association between WMH volume and the risk of cognitive impairment using solely longitudinal studies. Previous studies, including case–control studies and cohort studies, have explored the association of WMH with cognitive or neuropsychiatric symptoms, and in addition to inconsistent consequences, the underlying disease in patients with WMH has been uniform. For example, one study (Georgakis et al., 2019) reported the existence of a relationship between WMH and cognitive impairment after ischemic stroke. Clancy et al. (2021) reported the relationship between WMH and neuropsychiatric symptoms in CSVD. Through a further review of the existing cohort studies, most have suggested that WMH volume is associated with cognitive changes (Müller et al., 2020; Xia et al., 2020). In our study, the heterogeneity analysis showed high heterogeneity, so we combined an adjusted or a random effects model. In addition, both the cognitive decline and WMH in CSVD are age-related, and white matter loss accelerated with age (Smith et al., 2015). Therefore, longer follow-up time also represented an increase in patient age and white matter damage. However, follow-up time varied greatly among participants included in the study. To identify the sources of heterogeneity, we performed subgroup analyses of participants, cognitive levels at baseline, and the duration of follow-up across studies. Despite the presence of publication bias, by employing the “trim-fill” method, we determined that publication bias was unlikely to have had a significant effect on the results. The sensitivity analysis results were stable. Our meta-analysis did not include extra diseases that are known to cause WMH (such as Parkinson's disease, Alzheimer's disease, cerebrovascular disease, multiple sclerosis, and other neurological diseases) because they have a different clinical pathogenesis.
This article has some limitations. There exist different etiologies and pathogenic outcomes of WMH situated in different regions within the brain (Annweiler and Montero-Odasso, 2012); however, only two studies in the included literature dealt with WMH distribution. van den Heuvel et al. (2006) suggested that the PVWMH volume at baseline is longitudinally associated with reduced cognitive processing speed, which was shown in a cohort-based study. Another study by Godin et al. (2010) concluded that PVWMH was slightly more highly correlated with severe cognitive deterioration compared to DWMH. Unfortunately, we were unable to perform regional subgroup analysis on this topic due to a lack of sufficient data. Based on the available data, we could only perform a summary analysis of the total WMH volume. The relationship between different regions (DWMH, PVWMH) and cognition, therefore, requires more data for further analysis. Furthermore, the methods and software algorithms used for measuring the volume of WMH in our included studies were not consistent, which may have influenced the results. Future studies should, therefore, employ a consistent approach to determine the characteristic manifestations of WMH volume.
A second problem with the data used in this study is the small number of studies included in our analysis. There were only two studies involving patients with MCI at baseline. This reduced the power of our statistical analysis on the risk of developing dementia from MCI, as well as introduced uncertainty to the results. In this meta-analysis, the necessary exclusion criteria exacerbated the results of the small number of studies; for example, our meta-analysis excluded other disorders that cause WMH (such as Parkinson's disease, Alzheimer's disease, cerebrovascular disease, and other neurological diseases such as multiple sclerosis) because they have different clinical pathogenesis. Additionally, different methods used for reporting results were utilized between studies; some studies provided raw data from which OR, RR, or HR could be calculated, while other studies provided adjusted or unadjusted OR/RR/HR or other forms of statistical measurements. This variability created analytical compatibility issues and prevented some studies from being enrolled in the analysis due to a lack of obtainable data. Additionally, some studies controlled for covariates, while other studies that performed multivariate analyses differed in the covariates included, thus affecting this meta-analysis. Moreover, patients with other imaging markers of CSVD and WMH should be examined separately to determine their respective specific impacts on cognitive changes. This meta-analysis suggests that WMH volume increases the likelihood of cognitive alterations. However, heterogeneity between the included studies and patient populations, the small number of studies analyzed, and the differences in measurement methods prevent any conclusive conclusions from being established.
This meta-analysis quantified a positive correlation between the WMH volume of CSVD and decreased cognition. WMH appears to increase the risk of cognitive impairment in patients with CSVD, and the cognitive decline is a chronic process, whereby WMH predicted the rate of cognitive decline in CSVD for at least 5 years. The results of this meta-analysis suggest that early intervention may prevent or delay the onset of clinical dementia, and minimize cognitive decline for at-risk patients with WMH.
The datasets presented in this study can be found in online repositories. The names of the repository/repositories and accession number(s) can be found in the article/supplementary material.
WG drew up the protocol, performed the search, extracted the data, did the meta-analysis, and drafted the manuscript. JS participated in data extraction, checking, editing and looking overall responsibility for the article, and supervised the management. All the authors were reviewed before the submission of the manuscript.
This study was supported in part by the National Key Research and Development Program of China (Grant No. 2018YFC1704100), the National Natural Science Foundation of China (Grant No. 82074362), and the Fundamental Research Funds for the Central Universities (Grant No. 2019-JYB-TD-007).
The authors declare that the research was conducted in the absence of any commercial or financial relationships that could be construed as a potential conflict of interest.
All claims expressed in this article are solely those of the authors and do not necessarily represent those of their affiliated organizations, or those of the publisher, the editors and the reviewers. Any product that may be evaluated in this article, or claim that may be made by its manufacturer, is not guaranteed or endorsed by the publisher.
Annweiler, C., and Montero-Odasso, M. (2012). Vascular burden as a substrate for higher-level gait disorders in older adults. A review of brain mapping literature. Panminerva Med. 54, 189–204.
Bangen, K. J., Preis, S. R., Delano-Wood, L., Wolf, P. A., Libon, D. J., Bondi, M. W., et al. (2018). Baseline white matter hyperintensities and hippocampal volume are associated with conversion from normal cognition to mild cognitive impairment in the Framingham Offspring Study. Alzheimer Dis. Assoc. Disord. 32, 50–56. doi: 10.1097/WAD.0000000000000215
Boyle, P. A., Yu, L., Fleischman, D. A., Leurgans, S., Yang, J., Wilson, R. S., et al. (2016). White matter hyperintensities, incident mild cognitive impairment, and cognitive decline in old age. Ann Clin Transl Neurol. 3, 791–800. doi: 10.1002/acn3.343
Burke, S. L., Hu, T., Fava, N. M., Li, T., Rodriguez, M. J., Schuldiner, K. L., et al. (2019). Sex differences in the development of mild cognitive impairment and probable Alzheimer's disease as predicted by hippocampal volume or white matter hyperintensities. J. Women Aging. 31, 140–164. doi: 10.1080/08952841.2018.1419476
Chojdak-Łukasiewicz, J., Dziadkowiak, E., Zimny, A., and Paradowski, B. (2021). Cerebral small vessel disease: a review. Adv. Clin. Exp. Med. 30, 349–356. doi: 10.17219/acem/131216
Cipollini, V., Troili, F., and Giubilei, F. (2019). Emerging biomarkers in vascular cognitive impairment and dementia: from pathophysiological pathways to clinical application. Int. J. Mol. Sci. 20, 2812. doi: 10.3390/ijms20112812
Clancy, U., Gilmartin, D., Jochems, A. C. C., Knox, L., Doubal, F. N., Wardlaw, J. M., et al. (2021). Neuropsychiatric symptoms associated with cerebral small vessel disease: a systematic review and meta-analysis. Lancet Psychiatry. 8, 225–236. doi: 10.1016/S2215-0366(20)30431-4
Debette, S., Beiser, A., DeCarli, C., Au, R., Himali, J. J., Kelly-Hayes, M., et al. (2010). Association of MRI markers of vascular brain injury with incident stroke, mild cognitive impairment, dementia, and mortality: the Framingham Offspring Study. Stroke. 41, 600–606. doi: 10.1161/STROKEAHA.109.570044
Debette, S., and Markus, H. S. (2010). The clinical importance of white matter hyperintensities on brain magnetic resonance imaging: systematic review and meta-analysis. BMJ. 341, c3666. doi: 10.1136/bmj.c3666
Du, J., Zhu, H., Zhou, J., Lu, P., Qiu, Y., Yu, L., et al. (2020). Structural brain network disruption at preclinical stage of cognitive impairment due to cerebral small vessel disease. Neuroscience. 449, 99–115. doi: 10.1016/j.neuroscience.2020.08.037
Franchetti, M. K., Bharadwaj, P. K., Nguyen, L. A., Van Etten, E. J., Klimentidis, Y. C., Hishaw, G. A., et al. (2020). Interaction of age and self-reported physical sports activity on white matter hyperintensity volume in healthy older adults. Front. Aging Neurosci. 12, 576025. doi: 10.3389/fnagi.2020.576025
Georgakis, M. K., Duering, M., Wardlaw, J. M., and Dichgans, M. (2019). WMH and long-term outcomes in ischemic stroke: a systematic review and meta-analysis. Neurology. 92, e1298–e1308. doi: 10.1212/WNL.0000000000007142
Godin, O., Tzourio, C., Rouaud, O., Zhu, Y., Maillard, P., Pasquier, F., et al. (2010). Joint effect of white matter lesions and hippocampal volumes on severity of cognitive decline: the 3C-Dijon MRI study. J. Alzheimers. Dis. 20, 453–463. doi: 10.3233/JAD-2010-1389
Griffanti, L., Jenkinson, M., Suri, S., Zsoldos, E., Mahmood, A., Filippini, N., et al. (2018). Classification and characterization of periventricular and deep white matter hyperintensities on MRI: a study in older adults. Neuroimage. 170, 174–181. doi: 10.1016/j.neuroimage.2017.03.024
Heiland, E. G., Welmer, A. K., Kalpouzos, G., Laveskog, A., Wang, R., Qiu, C., et al. (2021). Cerebral small vessel disease, cardiovascular risk factors, and future walking speed in old age: a population-based cohort study. BMC Neurol. 21, 496. doi: 10.1186/s12883-021-02529-6
Hilal, S., Liu, S., Wong, T. Y., Vrooman, H., Cheng, C. Y., Venketasubramanian, N., et al. (2021). White matter network damage mediates association between cerebrovascular disease and cognition. J. Cereb. Blood Flow Metab. 41, 1858–1872. doi: 10.1177/0271678X21990980
Ikram, M. A., Vrooman, H. A., Vernooij, M. W., den Heijer, T., Hofman, A., Niessen, W. J., et al. (2010). Brain tissue volumes in relation to cognitive function and risk of dementia. Neurobiol. Aging. 31, 378–386. doi: 10.1016/j.neurobiolaging.2008.04.008
Kim, H. J., Park, S., Cho, H., Jang, Y. K., San Lee, J., Jang, H., et al. (2018). Assessment of extent and role of tau in subcortical vascular cognitive impairment using 18F-AV1451 positron emission tomography imaging. JAMA Neurol. 75, 999–1007. doi: 10.1001/jamaneurol.2018.0975
Langen, C. D., Cremers, L. G. M., de Groot, M., White, T., Ikram, M. A., Niessen, W. J., et al. (2018). Disconnection due to white matter hyperintensities is associated with lower cognitive scores. Neuroimage. 183, 745–756. doi: 10.1016/j.neuroimage.2018.08.037
Lopez, O. L., Becker, J. T., Chang, Y., Klunk, W. E., Mathis, C., Price, J., et al. (2018). Amyloid deposition and brain structure as long-term predictors of MCI, dementia, and mortality. Neurology. 90, e1920–e1928. doi: 10.1212/WNL.0000000000005549
Moher, D., Liberati, A., Tetzlaff, J., Altman, D. G., and Group PRISMA. (2009). Preferred reporting items for systematic reviews and meta-analyses: the PRISMA statement. PLoS Med. 6, e1000097. doi: 10.1371/journal.pmed.1000097
Müller, T., Payton, N. M., Kalpouzos, G., Jessen, F., Grande, G., Bäckman, L., et al. (2020). Cognitive, genetic, brain volume, and diffusion tensor imaging markers as early indicators of dementia. J. Alzheimers. Dis. 77, 1443–1453. doi: 10.3233/JAD-200445
Pantoni, L. (2010). Cerebral small vessel disease: from pathogenesis and clinical characteristics to therapeutic challenges. Lancet Neurol. 9, 689–701. doi: 10.1016/S1474-4422(10)70104-6
Park, D. G., Kang, S. Y., Hong, J. Y., Sunwoo, M. K., and Yoon, J. H. (2022). Factors associated with motor severity in vascularparkinsonism with normal dopamine transporter imaging. Parkinsonism Relat. Disord. 94, 99–103. doi: 10.1016/j.parkreldis.2021.11.033
Portet, F., Brickman, A. M., Stern, Y., Scarmeas, N., Muraskin, J., Provenzano, F. A., et al. (2012). Metabolic syndrome and localization of white matter hyperintensities in the elderly population. Alzheimers Dement. 8, S88–95.e1. doi: 10.1016/j.jalz.2011.11.007
Silbert, L. C., Howieson, D. B., Dodge, H., and Kaye, J. A. (2009). Cognitive impairment risk: white matter hyperintensity progression matters. Neurology. 73, 120–125. doi: 10.1212/WNL.0b013e3181ad53fd
Smith, E. E., Egorova, S., Blacker, D., Killiany, R. J., Muzikansky, A., Dickerson, B. C., et al. (2008). Magnetic resonance imaging white matter hyperintensities and brain volume in the prediction of mild cognitive impairment and dementia. Arch. Neurol. 65, 94–100. doi: 10.1001/archneurol.2007.23
Smith, E. E., O'Donnell, M., Dagenais, G., Lear, S. A., Wielgosz, A., Sharma, M., et al. (2015). Early cerebral small vessel disease and brain volume, cognition, and gait. Ann. Neurol. 77, 251–261. doi: 10.1002/ana.24320
Stang, A. (2010). Critical evaluation of the Newcastle - Ottawa scale for the assessment of the quality of nonrandomized studies in meta-analyses. Eur. J. Epidemiol. 25, 603–605. doi: 10.1007/s10654-010-9491-z
van den Heuvel, D. M., ten Dam, V H., de Craen, A. J., Admiraal-Behloul, F., Olofsen, H., Bollen, E. L., et al. (2006). Increase in periventricular white matter hyperintensities parallels decline in mental processing speed in a non-demented elderly population. J. Neurol. Neurosurg. Psychiatry 77, 149–153. doi: 10.1136/jnnp.2005.070193
van Uden, I. W., van der Holst, H. M., Tuladhar, A. M., van Norden, A. G., de Laat, K. F., Rutten-Jacobs, L. C., et al. (2016). White matter and hippocampal volume predict the risk of dementia in patients with cerebral small vessel disease: the RUN DMC Study. J. Alzheimers. Dis. 49, 863–873. doi: 10.3233/JAD-150573
Vergoossen, L. W. M., Jansen, J. F. A., van Sloten, T. T., Stehouwer, C. D. A., Schaper, N. C., Wesselius, A., et al. (2021). Interplay of white matter hyperintensities, cerebral networks, and cognitive function in an adult population: diffusion-tensor imaging in the Maastricht Study. Radiology. 298, 384–392. doi: 10.1148/radiol.2021202634
Weinstein, G., Beiser, A. S., Decarli, C., Au, R., Wolf, P. A., Seshadri, S., et al. (2013). Brain imaging and cognitive predictors of stroke and Alzheimer disease in the Framingham Heart Study. Stroke. 44, 2787–2794. doi: 10.1161/STROKEAHA.113.000947
Willey, J. Z., Scarmeas, N., Provenzano, F. A., Luchsinger, J. A., Mayeux, R., Brickman, A. M., et al. (2013). White matter hyperintensity volume and impaired mobility among older adults. J. Neurol. 260, 884–890. doi: 10.1007/s00415-012-6731-z
Xia, Y., Shen, Y., Wang, Y., Yang, L., Wang, Y., Li, Y., et al. (2020). White matter hyperintensities associated with progression of cerebral small vessel disease: a 7-year Chinese urban community study. Aging. 12, 8506–8522. doi: 10.18632/aging.103154
Xiong, L., Boulouis, G., Charidimou, A., Roongpiboonsopit, D., Jessel, M. J., Pasi, M., et al. (2018). Dementia incidence and predictors in cerebral amyloid angiopathy patients without intracerebral hemorrhage. J. Cereb. Blood Flow Metab. 38, 241–249. doi: 10.1177/0271678X17700435
Keywords: WMH, cognition, dementia, cerebral small vessel disease, meta
Citation: Guo W and Shi J (2022) White matter hyperintensities volume and cognition: A meta-analysis. Front. Aging Neurosci. 14:949763. doi: 10.3389/fnagi.2022.949763
Received: 21 May 2022; Accepted: 08 August 2022;
Published: 01 September 2022.
Edited by:
Julie Suhr, Ohio University, United StatesReviewed by:
Michael Malek-Ahmadi, Banner Alzheimer's Institute, United StatesCopyright © 2022 Guo and Shi. This is an open-access article distributed under the terms of the Creative Commons Attribution License (CC BY). The use, distribution or reproduction in other forums is permitted, provided the original author(s) and the copyright owner(s) are credited and that the original publication in this journal is cited, in accordance with accepted academic practice. No use, distribution or reproduction is permitted which does not comply with these terms.
*Correspondence: Jing Shi, c3NoaWppbmc4N0AxNjMuY29t
Disclaimer: All claims expressed in this article are solely those of the authors and do not necessarily represent those of their affiliated organizations, or those of the publisher, the editors and the reviewers. Any product that may be evaluated in this article or claim that may be made by its manufacturer is not guaranteed or endorsed by the publisher.
Research integrity at Frontiers
Learn more about the work of our research integrity team to safeguard the quality of each article we publish.