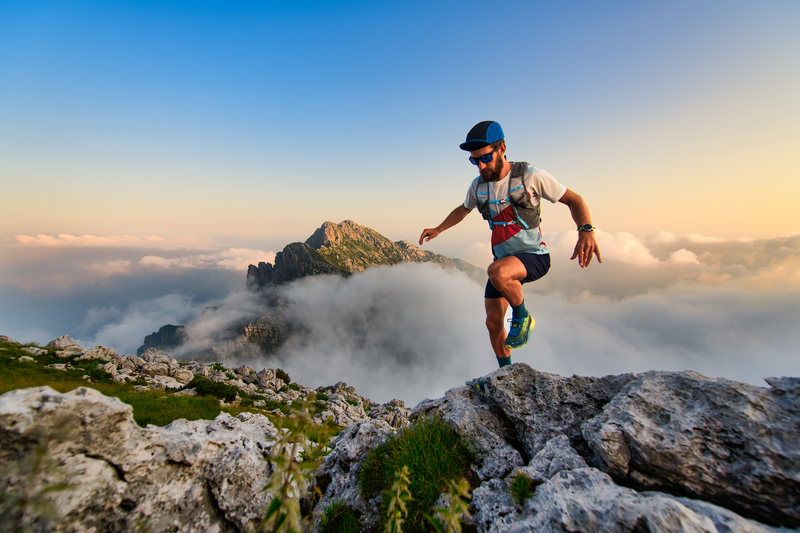
94% of researchers rate our articles as excellent or good
Learn more about the work of our research integrity team to safeguard the quality of each article we publish.
Find out more
METHODS article
Front. Aging Neurosci. , 13 June 2022
Sec. Parkinson’s Disease and Aging-related Movement Disorders
Volume 14 - 2022 | https://doi.org/10.3389/fnagi.2022.902169
This article is part of the Research Topic New Technologies for Detection, Monitoring and Treatment of Parkinson’s Disease, Volume II View all 7 articles
Objectives: [18F]9-fluoropropyl-(+)-dihydrotetrabenazine ([18F]-FP-DTBZ) positron emission tomography (PET) provides reliable information for the diagnosis of Parkinson’s disease (PD). In this study, we proposed a multi-atlas-based [18F]-FP-DTBZ PET image segmentation method for PD quantification assessment.
Methods: A total of 99 subjects from Xuanwu Hospital of Capital Medical University were included in this study, and both brain PET and magnetic resonance (MR) scans were conducted. Data from 20 subjects were used to generate atlases, based on which a multi-atlas-based [18F]-FP-DTBZ PET segmentation method was developed especially for striatum and its subregions. The proposed method was compared with the template-based method through striatal subregion parcellation performance and the standard uptake value ratio (SUVR) quantification accuracy. Discriminant analysis between healthy controls (HCs) and PD patients was further performed.
Results: Segmentation results of the multi-atlas-based method showed better consistency than the template-based method with the ground truth, yielding a dice coefficient of 0.81 over 0.73 on the full striatum. The SUVRs calculated by the multi-atlas-based method had an average interclass correlation coefficient (ICC) of 0.953 with the standardized result, whereas the template-based method only reached 0.815. The SUVRs of HCs were generally higher than that of patients with PD and showed significant differences in all of the striatal subregions (all p < 0.001). The median and posterior putamen performed best in discriminating patients with PD from HCs.
Conclusion: The proposed multi-atlas-based [18F]-FP-DTBZ PET image segmentation method achieved better performance than the template-based method, indicating great potential in improving accuracy and efficiency for PD diagnosis in clinical routine.
Parkinson’s disease (PD) is one of the most common age-related neurodegenerative disorders (Braak et al., 2003; Rai and Singh, 2020; Rai et al., 2021a). An increasing number of evidence suggests that positron emission tomography (PET) imaging aiming at the assessment of the dopaminergic function supports a more accurate diagnosis of PD (Frey et al., 1996; Ravina et al., 2005; Pirker et al., 2010). Vesicular monoamine transporter type 2 (VMAT2) is the transporter responsible for the uptake and storage of monoamines. As VMAT2 imaging by PET provides reliable information for the degeneration of nigrostriatal dopaminergic neurons, a novel radiotracer named [18F]9-fluoropropyl-(+)-dihydrotetrabenazine ([18F]-FP-DTBZ) has been developed (Gilman et al., 1998; Okamura et al., 2010). Previous studies have revealed that the [18F]-FP-DTBZ uptake in the striatum is significantly associated with the severity of PD (Braak et al., 2003; Hsiao, 2014). Therefore, improvement in the PET image quantification method is important for objective assessment of PD progression and diagnosis especially in the early stage (Hsiao, 2014; Liu et al., 2018).
To quantify the PET images, the target-to-reference standard uptake value ratio (SUVR) is extensively used, by which the accuracy of identifying regions of interest (ROIs) directly affects the credibility of the quantification results (Clark et al., 2011; Fleisher et al., 2011; Wolk et al., 2012). Magnetic resonance image (MR)-based methods are widely applied to identify the target ROIs. With the assistance of structural MR images, segmentation results could achieve comparable accuracy with manual segmentation (Andersson et al., 1995; Kuhn et al., 2014). However, in clinical practice, the acquisition of extra MR images is expensive and inconvenient when patients take PET scans (Bailey et al., 2015). Template-based methods have been proposed to achieve ROI parcellation in the absence of MR data, by which target PET images are coregistered to a normalized PET template with predefined ROIs (Andersson et al., 1995; Chang et al., 2011; Dukart et al., 2011; Kuhn et al., 2014). However, in dopamine imaging, due to small ROI and heterogeneity of the tracer distribution, the single template strategy could not ensure the reliability of spatial normalization, which results in inevitable segmentation error and may introduce misestimate in the consequent quantitative analysis (van Rikxoort et al., 2010). In addition, most of the template-based methods have not reached quantification at the subregion level, though the distribution of dopamine receptors varies greatly among the striatal subregions, especially in the case of patients with PD (Staley and Mash, 1996; Martinez et al., 2003).
In this study, we proposed a multi-atlas-based [18F]-FP-DTBZ PET image segmentation method, which implements reliable MR-free ROI extraction for PET images. Comparisons between the multi-atlas-based, template-based method, and the gold standard were conducted on the segmentation and quantification level. Quantification results were further analyzed to reveal the most sensitive subregions in discriminating patients with PD. This would provide reliable information for PD diagnosis and facilitate efficient clinical workflow.
A total of 2 datasets with 99 subjects recruited from the Xuanwu Hospital of Capital Medical University were included in this study. All subjects underwent a PET and a high-resolution MR scan of the brain. UI dataset with PET/MR (scanned by United Imaging uPMR790) includes 30 HCs and 38 PDs. GE dataset with PET/MR (scanned by GE Healthcare) includes 11 HCs and 20 PDs. The PDs were diagnosed according to the MDS clinical diagnostic criteria for PD (Postuma and Berg, 2017). The study was approved by the Institutional Review Board of Xuanwu Hospital. Written informed consent was obtained from all participants prior to the study procedure.
Magnetic resonance imaging data acquisition was performed using a hybrid 3.0-T PET/MR scanner (uPMR790, UIH, Shanghai, China) with a 24-channel head/neck coil. A 3D T1-weighted imaging (T1WI) data were collected from all participants with the following parameters: TR/TE: 7.86/3.2 ms; flip angle: 10; FOV: 230 mm × 256 mm; voxel size: 0.5 mm × 0.5 mm × 0.67 mm. Scanning parameters of [18F]-FP-DTBZ PET imaging were as follows: field of view = 300 mm; voxel size = 1.17 mm × 1.17 mm × 1.4 mm. All patients with PD were scanned during their off-state condition (12 h after the last medication).
Positron emission tomography/MRI examinations were performed on an integrated simultaneous Signa PET/MR scanner (GE Healthcare). The scan began 90 min following an intravenous bolus injection of around 222 MBq (6 mCi) of [18F]-FP-DTBZ and lasted for 15 min. The PET bed position included a simultaneous 18-s 2-point Dixon scan for MRI-based attenuation correction as well as additional diagnostic 3D T1 BRAVO MR images with scanning parameters as follows: TR = 7.9 ms; TE = 3.6 ms; acquisition matrix = 232 × 224; acquired spatial voxel resolution 1.00 mm × 1.00 mm × 1.00 mm with a data acquisition time of 4 min and 17 s. Scanning parameters of PET imaging were as follows: field of view = 25 cm; matrix size = 192 × 192; voxel size = 1.82 mm × 1.82 mm × 2.78 mm; 89 slices. Attenuation correction, scatter correction, random correction, and dead-time correction were performed as well.
The multi-atlas-based [18F]-FP-DTBZ PET image segmentation and analysis method were developed by the procedure illustrated in Figure 1. A total of 20 subjects including 10 HCs and 10 patients with PD were selected randomly from the UI dataset as the atlas repository (9 men and 11 women). The remaining subjects in the UI dataset were used for testing, named cohort UI. GE dataset was used for reproducibility experiment, named cohort GE.
Figure 1. Schematic representation of the procedure for the automatic multi-atlas-based PET image segmentation method.
First, the rigid coregistration was performed on the MR images to the corresponding PET images for each individual in the atlas repository. A PET template was constructed by averaging all PET images using the multivariate template construction method by Advanced Normalization Tools (ANTs1) (Avants et al., 2009). An MR template was generated using the same method and coregistered to the PET template. Individual MR images, as well as the MR template, were segmented using Brain Label,2 which executes brain parcellation of T1 image based on pre-selection strategy and multiple atlas likelihood fusion algorithm (Tang et al., 2013; Wu et al., 2016). By applying the Brain Label, whole-brain label images consisting of 283 regions, including the caudate, putamen, nucleus accumbens, and cerebellum, were generated.
After that, the PET images were coregistered to the PET template using the non-linear registration method by ANTs. The transform matrix and normalization parameters generated by these two steps were also applied to the label images. A manual check on segmentation accuracy for each participant was performed by a senior neurologist with 7-year experience in nuclear image processing.
Several image preprocessing methods were applied to the PET images in the template space, including skull stripping, partial volume effect correction, smoothing, and histogram specification. Each individual skull stripped PET image was corrected for partial volume effect by PETPVC, with kernel size = 6.0 mm × 6.0 mm × 6.0 mm, number of iterations = 10, number of deconvolution iterations = 10, alpha value = 1.5, stopping criterion = 0.01 (Thomas et al., 2016; Alavi et al., 2018). The VOI mask required by PETPVC was extracted from the label of the template. An isotropic Gaussian kernel of 3.7 mm × 3.7 mm × 6.6 mm full width at half maximum was applied for smoothing (Tournier et al., 2019). Global histogram specification was applied to transform the histogram of the individual PET images to the histogram of the template, followed by local histogram specification on the ROI of the striatum. Through histogram specification, PET image intensity was normalized to facilitate further comparison. Supplementary Figure 1 shows the examples of histogram specifications.
Finally, an atlas database including a PET template and 20 atlases was obtained to provide segmentation reference for target PET images. Each atlas contained a PET image in the template space with a corresponding label image and its global as well as local histogram-specified results.
Target PET images in cohort UI were coregistered to the template space and followed a similar preprocessing procedure as PET atlases (skull stripping, smoothing, and global and local histogram specification) for further evaluation. Mean square error (MSE) was applied to measure the similarity between target PET images and atlases. For each target, five best-matched atlases from the atlas database were selected by following two steps. In the first step, MSE between each atlas’ global-specified PET image and the target’s global-specified PET image was calculated. A total of 10 atlases with the least MSE values were selected as the candidates for the second step. In the second step, five best-matched atlases were selected based on MSE calculated between local-specified regions (i.e., the striatum) on PET images.
The segmentation results were produced by merging the label images of the best-matched atlases. The joint label fusion (JLF, operated by ANTs) was performed to fuse the obtained multiple labels of the PET image for each target participant (Avants et al., 2009). First, the chosen PET images were coregistered to the target PET image. Using the warping parameters, label images of the chosen atlases were warped to the target PET space and subsequently fused into one label image. Comparisons were conducted between the multi-atlas-based method and the conventional template-based method. The template-based method shared the same PET template as well as its label generated in section “Atlas Creation,” VOI was selected from the whole-brain label image, and the target PET image was coregistered to the template directly for VOI extraction.
A detailed subregion segmentation criterion based on the topographic structural information was adopted to find prominent parts of the striatum for discriminating patients with PD from HCs. The putamen and the caudate were divided into anterior, median, and posterior parts, respectively. Using the median point of the whole putamen pixels under the coronal axis, a sagittal plane was established to separate the striatum into left and right parts. Then, the bilateral putamen and caudate were further segmented into three equal parts along the line determined by their front-end and back-end points under the sagittal axis. Finally, the striatum was segmented into seven subregions (bilaterally, including the anterior putamen, median putamen, posterior putamen, anterior caudate, median caudate, posterior caudate, and nucleus accumbens). Figure 2 shows a sample of subregion segmentation result in 2D and 3D images to illustrate our subregion segmentation criterion. The total time required to segment a target PET image was about 20 min [CPU: Intel X(R) CPU E5-2620 v4 @ 2.10 GHz].
Figure 2. An example of a subregion segmentation result. Bilateral putamen and caudate were divided into three equal parts in length. (A) 3D. (B) Transverse. (C) Sagittal. (D) Coronal.
Standardized uptake value ratios of all seven subregions of the striatum on each PET image were calculated by dividing the mean counts per voxel in the target subregions by the mean counts per voxel in the reference region (Cerebellum):
The result of the MR-based method was applied as the ground truth. MR images for cohort UI subjects were segmented by Brain Label and coregistered to PET images. The subregion segmentation criterion was the same as mentioned before, and the final results were manually checked by the experienced neurologist.
Dice coefficient was applied to measure the similarity of segmentation results between the multi-atlas-based method and the MR-based method (Dice, 1945). It is computed by:
where A and B are two different segmentation results, respectively, and |⋅| represents the number of voxels within the segmentation result. A larger dice coefficient means better overlap. For larger structures, values above 0.8 are usually accepted as successful results while for smaller structures, values greater than 0.7 are preferred (Xiao et al., 2015).
The two-way mixed effect interclass correlation coefficients (ICCs) were calculated to identify the consistency between the SUVRs obtained by the two segmentation methods (Shrout and Fleiss, 1979). ICC is calculated by:
where σs denotes variance caused by differences between the segmentation methods and σs denotes variance caused by differences between the values in the segmentation results.
Statistical analysis of demographic details between groups was performed using a two-sample t-test and effect size. The t-test was further applied to compare SUVRs between HCs and patients with PD. Additionally, p < 0.05 was determined significant in our statistical results.
Table 1 summarizes the demographic details of participants from UI scanner. Descriptive data are presented as the mean ± standard deviation (SD) for continuous variables and percentage for dichotomous variables. No significant group differences were found in sex and age. In the PD group, mean UPDRS-III score was 21.5 (SD = 11.8) and the median of Hoehn and Yahr stage was 2 (range 1–5). The demographic details of participants from GE scanner are shown in Supplementary Table 1.
We compared the segmentation accuracy of the striatum subregions between the multi-atlas-based and template-based methods on cohort UI. As shown in Table 2, the multi-atlas-based method showed better segmentation performance. The mean dice coefficients of the whole striatum, caudate, and putamen all showed excellent accuracy (dice >0.8). The nucleus accumbens had the worst performance due to its smaller size. Compared to the multi-atlas-based method, the template-based method had a general performance degradation of 8%, which indicated the multi-atlas strategy held better performance than the single template strategy.
Figure 3 presents the subregions obtained by the multi-atlas-based method and the MR-based method. For each case, the result of the multi-atlas-based method was shown on the left whereas the result of the MR-based was shown on the right. The backgrounds were the native PET images. Segmentation results of the multi-atlas-based method showed good agreement with the MR-based method in varied conditions and slices.
Figure 3. Segmentation results comparison. Each subgraph consists of a subject’s original PET image, superimposed with seven subregion labels obtained by two segmentation methods, the multi-atlas-based method (left) and the MR-based method (right). Both PDs and HCs from cohort UI and GE were selected to demonstrate their segmentation effects at different cross-sectional slices. (A) A 46-year-old HC (male) in cohort UI case 4. (B) A 56-year-old PD (female) in cohort UI case 20. (C) A 48-year-old PD (female) in cohort GE case 3. (D) A 59-year-old HC (female) in cohort UI case 12. (E) A 61-year-old PD (male) in cohort UI case 32. (F) A 75-year-old PD (male) in cohort GE case 31.
The subregion SUVR values were calculated according to the segmentation results generated by the multi-atlas-based method and the MR-based method, respectively. The correlation of results obtained by these two analyses was calculated and visualized in Figure 4. As demonstrated, SUVR of all subregions showed great consistency with an average ICC of 0.953. In contrast, the average ICC between the template-based method and the MR-based method was 0.815 (Supplementary Figure 2).
Figure 4. Subregion SUVR correlations. Subregion SUVR correlations between the multi-atlas-based PET segmentation and the MR-based segmentation in cohort UI. The horizontal axis is the SUVR of each subregion separated by the multi-atlas-based PET segmentation, whereas the vertical axis is the SUVR of each subregion separated by the MR-based segmentation.
Figure 5 and Table 3 show the SUVRs of [18F]-FP-DTBZ in the subregions of PD and HC groups from cohort UI. The SUVRs of HCs were generally higher than that of patients with PD and showed significant differences in all of the subregions (all p < 0.001). The SUVRs of the median putamen and posterior putamen in patients with PD could be separated clearly from HCs without any overlap between the two groups. The t-test demonstrated SUVR values to be significantly different in all of the subregions for HCs and patients with PD (p < 0.001). Supplementary Table 2 shows the effect size of the multi-atlas-based method and the template-based method. In most subregions, the multi-atlas-based method obtained better divergence in discriminating HC and patients with PD.
Figure 5. Subregion [18F]-FP-DTBZ SUVRs in cohort UI. In the violin plot, the central mark indicates the median, and the bottom and top edges indicate the 25th and 75th percentiles. HC_UI, healthy control from UI scanner; PD_UI, Parkinson’s disease from UI scanner. Significance: ***p < 0.001 t-test analysis.
Table 3. [18F]9-fluoropropyl-(+)-dihydrotetrabenazine SUVRs in seven subregions in PDs and HCs from cohort UI.
Reproducibility experiments were performed on cohort GE. The multi-atlas-based PET image segmentation method and the MR-based method were applied to extract the ROI subregions. The dice coefficient of the two methods reached 0.792 on the whole striatum and 0.816, 0.788, and 0.652 on the putamen, caudate, and nucleus accumbens, respectively. SUVR of all subregions calculated by the multi-atlas-based method and the MR-based method is compared in Supplementary Figure 3 having an average ICC of 0.969. Supplementary Figure 4 and Supplementary Table 3 demonstrated the SUVR comparison between HCs and PDs in cohort GE and showed a similar pattern as cohort UI, indicating that the multi-atlas-based method could be applied for multi-center use.
In this study, we proposed a multi-atlas-based PET segmentation method that achieved reliable segmentation performance in an MR-free situation. Analysis on [18F]-FP-DTBZ PET quantification showed great potential for our method in PD diagnosis. The current PET image analysis methods mainly include the manual method, MR-based method, and MR-free template-based method. Our multi-atlas-based method was fully automatic and did not rely on MR images. Thus, it was operator-independent and had great reproducibility with high processing efficiency. As compared to the template-based method, our proposed method used an atlas database rather than a single template, which provided a higher parcellation accuracy. In the previous studies, the PET template was usually generated from HCs or adapted from existing results such as Montreal Neurological Institute (MNI) standard space, and target PET images were coregistered to the PET template directly (Evans et al., 1993). Given that the binding of [18F]-FP-DTBZ was affected by VMAT2 distribution, PET images of different disease severity would present different intensity distributions according to VMAT2 density (Lin et al., 2011). However, the coregistration method could not work well when the image intensity did not reflect the real structure information. So, coregistration error would be introduced if only one template is referenced. The multi-atlas selection strategy could automatically select the most matched atlases using both global and local information, thus avoiding potential segmentation degeneration caused by coregistration with inappropriate images. By comparison, the multi-atlas-based method showed better accuracy than the template-based method (Table 2).
Our method further segmented the striatum into seven subregions. The putamen and the caudate were divided into three parts, respectively. Our study showed that the reduction of VMAT2 integrity among patients with PD varies in different parts of the striatum. The improvement in the segmentation method helped to further distinguish patients with PD from HCs. Analysis of SUVR showed that all subregions of patients with PD exhibited different levels of lower VMAT2 densities than HCs, suggesting presynaptic nigrostriatal dysfunction in PD (Lung et al., 2018). The most affected subregion was the posterior putamen, followed by the rest parts of the putamen and subregions in the caudate, which was in line with the previous study (Okamura et al., 2010; Hsiao, 2014). SUVRs in the median putamen and posterior putamen had the best performance to discriminate patients with PD from HCs.
The multi-atlas-based method also showed potential in processing datasets from other institutions and was expected to be applicable to other PET modalities (Bui et al., 2020). Reproducibility experiments indicated that our method could achieve guaranteed segmentation and quantification results on a different scanner. In this study, [18F]-FP-DTBZ PET was selected as our imaging modality because VMAT2 has a high density in striatal regions and shows low sensitivity to drug effects (Lee et al., 2000; Sun et al., 2012). But the multi-atlas-based method could be applied to other PETs targeting DAT or AADC as well (de Natale et al., 2018). Since the generation of PET images followed the same principle, our method could also be utilized for some other neurodegenerative diseases which could be examined by PET such as AD.
Several limitations in this study should be mentioned. First, a large number of subjects, as well as samples from other imaging centers for further validation, are warranted. Due to the limited sample size, our current atlas database only included 20 cases. Although a significant segmentation performance improvement has been achieved, more atlases were expected to provide better segmentation accuracy since more PET distribution patterns might be included. Second, our subregion segmentation criterion was restricted to topographic structural information. Through significant difference has been found in some of the subregions, the combination of structural connectivity and functional information might provide more insights into dopamine function and contribute to better subdivision (Tziortzi et al., 2014; Rai et al., 2020, 2021b). Finally, future work to establish the correlation between the analysis results and the clinical disease severity is needed. As we did not include H–Y stage information of subjects in the study, further studies focusing on evaluating the performances of our proposed method in different severities of PD are warranted.
In this study, a multi-atlas-based [18F]-FP-DTBZ PET image segmentation method was proposed for PD quantification and showed better performance than the template-based method. The application analysis in patients with PD suggests that the proposed method has potential value for improving the accuracy and efficiency of PD diagnostic in clinical routine.
The datasets presented in this article are not readily available because it is for institutional use only. Requests to access the datasets should be directed to TM, dG1hQGhpdC5lZHUuY24=.
The studies involving human participants were reviewed and approved by the Institutional Review Board of Xuanwu Hospital. The patients/participants provided their written informed consent to participate in this study. Written informed consent was obtained from the individual(s) for the publication of any potentially identifiable images or data included in this article.
TM conceived and supervised the project. HQ and TS helped the data acquisition. YP, SL, YZ, and CY analyzed the data. YP drafted the manuscript. YZ created the figures. CY and HL revised the manuscript. SL, PC, and JL provided the insight on clinical aspects. TM, JL, and PC gave final approval to the manuscript. All authors read and approved the final manuscript.
This study was supported by grants from the National Key Research and Development Program of China (nos. 2018YFC1312000, 2017YFC0840105, and 2017ZX09304018), China Postdoctoral Science Foundation funded project (no. 2021M691686), Basic Research Foundation of Shenzhen Science and Technology Stable Support Plan (no. GXWD20201230155427003–20200822115709001), National Natural Science Foundation of China (nos. 62106113, 81901285, 81701726, and 81522021), Beijing Municipal Administration of Hospitals (nos. SML20150803 and DFL20180802), and Beijing Municipal Science and Technology Commission (no. Z171100000117013).
HL was employed by Mindsgo Life Science Shenzhen Co. Ltd.
The remaining authors declare that the research was conducted in the absence of any commercial or financial relationships that could be construed as a potential conflict of interest.
All claims expressed in this article are solely those of the authors and do not necessarily represent those of their affiliated organizations, or those of the publisher, the editors and the reviewers. Any product that may be evaluated in this article, or claim that may be made by its manufacturer, is not guaranteed or endorsed by the publisher.
The Supplementary Material for this article can be found online at: https://www.frontiersin.org/articles/10.3389/fnagi.2022.902169/full#supplementary-material
ANTs, Advanced Normalization Tools; [18F]-FP-DTBZ, [18F]9-fluoropropyl-(+)-dihydrotetrabenazine; HC, healthy control; ICC, interclass correlation coefficient; JLF, joint label fusion; MSE, mean square error; PD, Parkinson’s disease; SUVR, standard uptake value ratio; VMAT2, vesicular monoamine transporter type 2.
Alavi, A., Werner, T. J., Høilund-Carlsen, P. F., and Zaidi, H. (2018). Correction for partial volume effect is a must, not a luxury, to fully exploit the potential of quantitative PET imaging in clinical oncology. Mol. Imaging Biol. 20, 1–3. doi: 10.1007/s11307-017-1146-y
Andersson, J. L., Sundin, A., and Valind, S. (1995). A method for coregistration of PET and MR brain images. J. Nucl. Med. 36, 1307–1315.
Avants, B. B., Tustison, N., and Song, G. (2009). Advanced normalization tools (ANTS). Insight J. 2, 1–35. doi: 10.1007/s11682-020-00319-1
Bailey, D., Pichler, B., Gückel, B., Barthel, H., Beer, A., Bremerich, J., et al. (2015). Combined PET/MRI: multi-modality multi-parametric imaging is here. Mol. Imaging Biol. 17, 595–608. doi: 10.1007/s11307-015-0886-9
Braak, H., Rüb, U., Gai, W., and Del Tredici, K. (2003). Idiopathic Parkinson’s disease: possible routes by which vulnerable neuronal types may be subject to neuroinvasion by an unknown pathogen. J. Neural Transm. 110, 517–536. doi: 10.1007/s00702-002-0808-2
Bui, V., Hsu, L.-Y., Shanbhag, S. M., Tran, L., Bandettini, W. P., Chang, L.-C., et al. (2020). Improving multi-atlas cardiac structure segmentation of computed tomography angiography: a performance evaluation based on a heterogeneous dataset. Comput. Biol. Med. 125:104019. doi: 10.1016/j.compbiomed.2020.104019
Chang, I. C., Lue, K. H., Hsieh, H. J., Liu, S. H., and Kao, C. H. (2011). Automated striatal uptake analysis of (1)(8)F-FDOPA PET images applied to Parkinson’s disease patients. Ann. Nucl. Med. 25, 796–803. doi: 10.1007/s12149-011-0533-8
Clark, C. M., Schneider, J. A., Bedell, B. J., Beach, T. G., Bilker, W. B., Mintun, M. A., et al. (2011). Use of florbetapir-PET for imaging β-amyloid pathology. J. Am. Med. Assoc. 305, 275–283.
de Natale, E. R., Niccolini, F., Wilson, H., and Politis, M. (2018). Molecular imaging of the dopaminergic system in idiopathic Parkinson’s Disease. Int. Rev. Neurobiol. 141, 131–172. doi: 10.1016/bs.irn.2018.08.003
Dice, L. R. (1945). Measures of the amount of ecologic association between species. Ecology 26, 297–302.
Dukart, J., Mueller, K., Horstmann, A., Barthel, H., Möller, H. E., Villringer, A., et al. (2011). Combined evaluation of FDG-PET and MRI improves detection and differentiation of dementia. PLoS One 6:e18111. doi: 10.1371/journal.pone.0018111
Evans, A. C., Collins, D. L., Mills, S., Brown, E. D., Kelly, R. L., and Peters, T. M. (1993). “3D statistical neuroanatomical models from 305 MRI volumes,” in Proceeding of the 1993 IEEE Conference Record Nuclear Science Symposium and Medical Imaging Conference, (San Francisco, CA: IEEE), 1813–1817.
Fleisher, A. S., Chen, K., Liu, X., Roontiva, A., Thiyyagura, P., Ayutyanont, N., et al. (2011). Using positron emission tomography and florbetapir F 18 to image cortical amyloid in patients with mild cognitive impairment or dementia due to Alzheimer Disease. Arch. Neurol. 68, 1404–1411.
Frey, K. A., Koeppe, R. A., Kilbourn, M. R., Vander Borght, T. M., Albin, R. L., Gilman, S., et al. (1996). Presynaptic monoaminergic vesicles in Parkinson’s disease and normal aging. Ann. Neurol. 40, 873–884. doi: 10.1002/ana.410400609
Gilman, S., Koeppe, R. A., Adams, K. M., Junck, L., Kluin, K. J., Johnson-Reene, D., et al. (1998). Decreased striatal monoaminergic terminals in severe chronic alcoholism demonstrated with (+)[11C]Dihydrotetrabenazine and positron emission tomography. Ann. Neurol. 44, 326–333. doi: 10.1002/ana.410440307
Hsiao, I. T. (2014). Correlation of parkinson disease severity and F-18-DTBZ positron emission tomography. JAMA Neurol. 71, 803–803.
Kuhn, F. P., Warnock, G. I., Burger, C., Ledermann, K., Martin-Soelch, C., and Buck, A. (2014). Comparison of PET template-based and MRI-based image processing in the quantitative analysis of C 11-raclopride PET. EJNMMI Res. 4:7.
Lee, C. S., Samii, A., Sossi, V., Ruth, T. J., Schulzer, M., Holden, J. E., et al. (2000). In vivo positron emission tomographic evidence for compensatory changes in presynaptic dopaminergic nerve terminals in Parkinson’s disease. Ann. Neurol. 47, 493–503.
Lin, K.-J., Lin, W.-Y., Hsieh, C.-J., Weng, Y.-H., Wey, S.-P., Lu, C.-S., et al. (2011). Optimal scanning time window for 18F-FP-(+)-DTBZ (18F-AV-133) summed uptake measurements. Nucl. Med. Biol. 38, 1149–1155. doi: 10.1016/j.nucmedbio.2011.05.010
Liu, Z. Y., Liu, F. T., Zuo, C. T., Koprich, J. B., and Wang, J. (2018). Update on molecular imaging in Parkinson’s Disease. Neurosci. Bull. 34, 330–340. doi: 10.1007/s12264-017-0202-6
Lung, H. J., Weng, Y.-H., Wen, M.-C., Hsiao, T., and Lin, K.-J. (2018). Quantitative study of 18 F-(+) DTBZ image: comparison of PET template-based and MRI based image analysis. Sci. Rep. 8:16027. doi: 10.1038/s41598-018-34388-6
Martinez, D., Slifstein, M., Broft, A., Mawlawi, O., Hwang, D.-R., Huang, Y., et al. (2003). Imaging human mesolimbic dopamine transmission with positron emission tomography. Part II: amphetamine-induced dopamine release in the functional subdivisions of the striatum. J. Cereb. Blood Flow Metab. 23, 285–300. doi: 10.1097/01.WCB.0000048520.34839.1A
Okamura, N., Villemagne, V. L., Drago, J., Pejoska, S., and Rowe, C. C. (2010). In vivo measurement of vesicular monoamine transporter type 2 density in Parkinson disease with F-18-AV-133. J. Nucl. Med. 51, 223–228.
Pirker, W., Djamshidian, S., Asenbaum, S., Gerschlager, W., Tribl, G., Hoffmann, M., et al. (2010). Progression of dopaminergic degeneration in Parkinson’s disease and atypical parkinsonism: a longitudinal beta-CIT SPECT study. Mov. Disord. 17, 45–53. doi: 10.1002/mds.1265
Postuma, R. B., and Berg, D. (2017). The new diagnostic criteria for Parkinson’s disease. Int. Rev. Neurobiol. 132, 55–78.
Rai, S. N., Chaturvedi, V. K., Singh, P., Singh, B. K., and Singh, M. P. (2020). Mucuna pruriens in Parkinson’s and in some other diseases: recent advancement and future prospective. 3 Biotech 10:522. doi: 10.1007/s13205-020-02532-7
Rai, S. N., and Singh, P. (2020). Advancement in the modelling and therapeutics of Parkinson’s disease. J. Chem. Neuroanat. 104:101752. doi: 10.1016/j.jchemneu.2020.101752
Rai, S. N., Singh, P., Varshney, R., Chaturvedi, V. K., Vamanu, E., Singh, M. P., et al. (2021a). Promising drug targets and associated therapeutic interventions in Parkinson’s disease. Neural Regen. Res. 16, 1730–1739. doi: 10.4103/1673-5374.306066
Rai, S. N., Tiwari, N., Singh, P., Mishra, D., Singh, A. K., Hooshmandi, E., et al. (2021b). Therapeutic potential of vital transcription factors in Alzheimer’s and Parkinson’s disease with particular emphasis on transcription factor eb mediated autophagy. Front. Neurosci. 15:777347. doi: 10.3389/fnins.2021.777347
Ravina, B., Eidelberg, D., Ahlskog, J., Albin, R., Brooks, D., Carbon, M., et al. (2005). The role of radiotracer imaging in Parkinson disease. Neurology 64, 208–215.
Shrout, P. E., and Fleiss, J. L. (1979). Intraclass correlations: uses in assessing rater reliability. Psychol. Bull. 86:420. doi: 10.1037//0033-2909.86.2.420
Staley, J. K., and Mash, D. C. (1996). Adaptive increase in D3 dopamine receptors in the brain reward circuits of human cocaine fatalities. J. Neurosci. 16, 6100–6106. doi: 10.1523/JNEUROSCI.16-19-06100.1996
Sun, J., Xu, J., Cairns, N. J., Perlmutter, J. S., and Mach, R. H. (2012). Dopamine D1, D2, D3 receptors, vesicular monoamine transporter type-2 (VMAT2) and dopamine transporter (DAT) densities in aged human brain. PLoS One 7:e49483. doi: 10.1371/journal.pone.0049483
Tang, X., Oishi, K., Faria, A. V., Hillis, A. E., Albert, M. S., Mori, S., et al. (2013). Bayesian parameter estimation and segmentation in the multi-atlas random orbit model. PLoS One 8:e65591. doi: 10.1371/journal.pone.0065591
Thomas, B. A., Cuplov, V., Bousse, A., Mendes, A., Thielemans, K., Hutton, B. F., et al. (2016). PETPVC: a toolbox for performing partial volume correction techniques in positron emission tomography. Phys. Med. Biol. 61, 7975–7993. doi: 10.1088/0031-9155/61/22/7975
Tournier, J. D., Smith, R., Raffelt, D., Tabbara, R., Dhollander, T., Pietsch, M., et al. (2019). MRtrix3: a fast, flexible and open software framework for medical image processing and visualisation. Neuroimage 202:116137. doi: 10.1016/j.neuroimage.2019.116137
Tziortzi, A. C., Haber, S. N., Searle, G. E., Tsoumpas, C., Long, C. J., Shotbolt, P., et al. (2014). Connectivity-based functional analysis of dopamine release in the striatum using diffusion-weighted MRI and positron emission tomography. Cereb. Cortex 24, 1165–1177. doi: 10.1093/cercor/bhs397
van Rikxoort, E. M., Isgum, I., Arzhaeva, Y., Staring, M., Klein, S., Viergever, M. A., et al. (2010). Adaptive local multi-atlas segmentation: application to the heart and the caudate nucleus. Med. Image Anal. 14, 39–49. doi: 10.1016/j.media.2009.10.001
Wolk, D. A., Zhang, Z., Boudhar, S., Clark, C. M., Pontecorvo, M. J., and Arnold, S. E. (2012). Amyloid imaging in Alzheimer’s disease: comparison of florbetapir and Pittsburgh compound-B positron emission tomography. J. Neurol. Neurosurg. Psychiatry 83, 923–926. doi: 10.1136/jnnp-2012-302548
Wu, D., Ma, T., Ceritoglu, C., Li, Y., Chotiyanonta, J., Hou, Z., et al. (2016). Resource atlases for multi-atlas brain segmentations with multiple ontology levels based on T1-weighted MRI. Neuroimage 125, 120–130. doi: 10.1016/j.neuroimage.2015.10.042
Keywords: Parkinson’s disease, [18F]-FP-DTBZ, image segmentation, striatum subregion, SUVR quantification
Citation: Pan Y, Liu S, Zeng Y, Ye C, Qiao H, Song T, Lv H, Chan P, Lu J and Ma T (2022) A Multi-Atlas-Based [18F]9-Fluoropropyl-(+)-Dihydrotetrabenazine Positron Emission Tomography Image Segmentation Method for Parkinson’s Disease Quantification. Front. Aging Neurosci. 14:902169. doi: 10.3389/fnagi.2022.902169
Received: 22 March 2022; Accepted: 23 May 2022;
Published: 13 June 2022.
Edited by:
Soojin Lee, University of British Columbia, CanadaReviewed by:
Sachchida Nand Rai, University of Allahabad, IndiaCopyright © 2022 Pan, Liu, Zeng, Ye, Qiao, Song, Lv, Chan, Lu and Ma. This is an open-access article distributed under the terms of the Creative Commons Attribution License (CC BY). The use, distribution or reproduction in other forums is permitted, provided the original author(s) and the copyright owner(s) are credited and that the original publication in this journal is cited, in accordance with accepted academic practice. No use, distribution or reproduction is permitted which does not comply with these terms.
*Correspondence: Jie Lu, aW1hZ2luZ2x1QGhvdG1haWwuY29t; Ting Ma, dG1hQGhpdC5lZHUuY24=
†These authors have contributed equally to this work and share first authorship
Disclaimer: All claims expressed in this article are solely those of the authors and do not necessarily represent those of their affiliated organizations, or those of the publisher, the editors and the reviewers. Any product that may be evaluated in this article or claim that may be made by its manufacturer is not guaranteed or endorsed by the publisher.
Research integrity at Frontiers
Learn more about the work of our research integrity team to safeguard the quality of each article we publish.