- School of Basic Medical Sciences, Dali University, Dali, China
Background: Alzheimer’s disease (AD) is a common neurodegenerative disease. The pathogenesis is complex and has not been clearly elucidated, and there is no effective treatment. Recent studies have demonstrated that DNA methylation is closely associated with the pathogenesis of AD, which sheds light on investigating potential biomarkers for the diagnosis of early AD and related possible therapeutic approaches.
Methods: Alzheimer’s disease patients samples and healthy controls samples were collected from two datasets in the GEO database. Using LIMMA software package in R language to find differentially expressed genes (DEGs). Afterward, DEGs have been subjected to enrichment analysis of GO and KEGG pathways. The PPI networks and Hub genes were created and visualized based on the STRING database and Cytoscape. ROC curves were further constructed to analyze the accuracy of these genes for AD diagnosis.
Results: Analysis of the GSE109887 and GSE97760 datasets showed 477 significant DEGs. GO and KEGG enrichment analysis showed terms related to biological processes related to these genes. The top ten Hub genes were found on the basis of the PPI network using the CytoHubba plugin, and the AUC areas of these top ranked genes were all greater than 0.7, showing satisfactory diagnostic accuracy.
Conclusion: The study identified the top 10 Hub genes associated with AD-related DNA methylation, of which RPSA, RPS23, and RPLP0 have high diagnostic accuracy and excellent AD biomarker potential.
Introduction
Alzheimer’s disease is an age-related neurodegenerative disease that deteriorates with age and over time and often occurs in the elderly over 65 years. Currently, there are more than 55 million AD patients worldwide, and this number continues to grow, which represents a challenge to public health (Gauthier et al., 2021). According to data published by the World Health Organization (WHO) in 2020, AD and other dementias are the seventh greatest cause of mortality globally and one of the most socially costly chronic diseases (World Health Organization, 2021). As AD worsens, patients experience memory loss, mood swings, and even loss of self-care ability (Burns and Iliffe, 2009). It is predicted that the average life expectancy of patients with AD ranges from 3 to 9 years from diagnosis to further disease worsening (Querfurth and LaFerla, 2010; Todd et al., 2013). If diagnosed and intervened in a timely manner, the onset of AD will be delayed by 5–10 years (Ma et al., 2019). In the global pandemic of coronavirus disease 2019 (COVID-19), AD patients were reported to be more susceptible to coronavirus invasion (Mok et al., 2020), and for AD patients who have been infected with COVID-19, their mortality rate is significantly higher than that of healthy elderly (Hardan et al., 2021). Thus, the diagnosis of early AD can provide good help for both patient care and prognosis as well as global public health. Cognitive testing, magnetic resonance imaging (MRI), positron emission tomography (PET), and detection of cerebrospinal fluid (CSF) biomarkers are currently used clinically to diagnose AD, but according to ALZHEIMER’S DISEASE INTERNATIONAL (ADI) data, more than 75% of AD patients worldwide are not diagnosed, with 90% of patients in low and intermediate income (Nakamura et al., 2018; Sun et al., 2018; Gauthier et al., 2021; World Health Organization, 2021). Similarly, more than 77% of clinicians in an ADI and WHO survey intended to use new blood tests to improve the diagnostic accuracy of AD in clinical practice (Gauthier et al., 2021). Due to the ease of availability, high specificity, and economic advantages of blood marker performance, that is currently of great practical significance for exploring blood biomarkers for the diagnosis of AD.
In recent years, increasing studies have focused on AD-related DNA methylation, one of the key mechanisms of epigenetic research, which alters the expression of genes at the transcriptional level through the upregulation, downregulation, or silencing of genes without changing the DNA sequence (Mishra and Li, 2020; Sharma et al., 2020). DNA methylation regulates neuronal differentiation early in central nervous system development, whereas DNA methylation levels in the cerebral cortex change dynamically throughout life (Mohn et al., 2008). With the progression of aging, the degree of DNA methylation differences increases and can affect the function of learning and memory (Sharma et al., 2020; Li et al., 2021). Based on the specificity of different brain regions, neurons can express hypermethylation or hypomethylation at different sites during AD pathogenesis (Sharma et al., 2020). Hypomethylation of APP can lead to enhanced aggregation of amyloid plaques which is an important factor in AD pathogenesis (Gasparoni et al., 2018).
In addition to age, genes and family genetic history are also non-modifiable risk factors for AD. For AD cases other than early-onset familial AD (FEOAD), 70% of these risks can be attributed to genetic factors (Gatz et al., 2006; Ballard et al., 2011). Due to the continuous development and application of microarray and next-generation sequencing technology (NGS), genetic data-based research is growing, providing strong support for deciphering disease-related genetic factors (Mishra and Li, 2020). Some of these studies have achieved meaningful results for the diagnosis, prognosis and genetic analysis of AD based on genetic data. As early as 1993, Genome-wide association studies (GWAS) revealed APOE ε4 as the most important genetic risk factor for AD (Freudenberg-Hua et al., 2018). More recent risk gene loci including ABCA7, ACE, ADAM10, and ADAM17 have also been reported (Pimenova et al., 2018). Similarly, NGS also identified risk genes associated with AD pathogenesis such as NOTCH3, TREM2, and ARSA (Patel et al., 2019). Some studies on methylation of these genes have demonstrated a close relationship with the development of AD. In AD patients, hypomethylation at the TREM2 intron 1 CpG site results in higher expression of TREM2 mRNA in leukocytes than in healthy controls (Ozaki et al., 2017). Although CpG methylation in ABCA7 may have less of an impact on brain ABCA7 mRNA expression (Yu et al., 2015), the CpG islands of ABCA7 exhibit significant hypomethylation compared to healthy human brains (Humphries et al., 2015). In addition, 19 CpG island sites in the ABCA7 locus have been shown to be positively correlated with pathological AD diagnosis (Yu et al., 2015), including 12 CpG sites associated with Aβ content and 18 CpG sites associated with Tau tangle tightness (De Roeck et al., 2019). These results provide evidence that AD pathogenesis may involve methylation of associated risk genes. Since DNA methylation levels are more stable than mRNA levels (Paziewska et al., 2014), studies based on blood DNA methylation levels may provide insights into important biological pathways in AD development.
Therefore, in our study, differentially expressed genes (DEGs) correlated with DNA methylation in AD were analyzed in order to find blood biomarkers that can be used to diagnose early AD with potential therapeutic targets. The gene expression profiling data for GSE109887 and GSE97760 were obtained from the Gene Expression Omnibus (GEO) database. To elucidate the pathomechanism of AD-related DNA methylation, DEGs were subjected to enrichment analysis of the Gene Ontology (GO) and Kyoto Encyclopedia of Genes and Genomes (KEGG) pathways. We also used DEGs for constructing coexpression networks to obtain Hub genes with DNA methylation associated with AD, hoping to provide new directions and strategies for the diagnose and therapy of AD.
Materials and Methods
Collection of Gene Expression Profiling Data
Gene Expression Omnibus is a public genetic and genomic data source that accepts array- and sequence-based data submissions, and enables users to search and download experimental gene expression profiles (Edgar et al., 2002). GSE109887, a dataset containing data on DNA methylation and hydroxymethylation levels in the blood and middle temporal gyrus (MTG) regions of the brain in AD patients, was used to obtain DNA methylation levels of DEGs. The differential expression levels of DNA methylation vary in different brain regions and show an increase throughout the brain region with aging. Compared with other brain regions, the MTG shows a more significant difference in DNA methylation level (Coppieters et al., 2014). And according to research on AD patients AD-related pathological changes appeared first in the MTG regions (Ray and Zhang, 2010). In addition, the trend of differential DNA methylation levels in MTG regions of the brain was reported to be similar to that in blood (Lardenoije et al., 2019). To further investigate the effects of DNA methylation on the expression of genes, GSE97760 dataset was used to obtain the expression levels of DEGs in the blood of AD patients and normal controls, which then was used to compare the DNA methylation levels of DEGs obtained from the GSE109887 dataset.
The GSE109887 dataset was examined using the GPL10904 (Illumina HumanHT-12 V4.0 expression beadchip) platform and contained 78 samples derived from the MTG of the brain, of which 32 samples were derived from healthy individuals and 46 samples were derived from AD patients. The GSE97760 dataset was tested using the GPL16699 (Agilent-039494 SurePrint G3 Human GEv2 8 × 60K Microarray 039381) platform and contained 19 blood samples, 10 samples from healthy individuals and 9 samples from AD patients.
Data Processing and Identification of Differentially Expressed Genes
Limma (linear models for microarray data, doi: 10.1093/nar/gkv00), is a generalized linear model-based differential expression screening method. We used the R package Limma (version 3.40.6) to perform differential analysis of downloaded expression profile datasets to obtain differential genes between normal and AD groups. Specifically, we first set the P value threshold to 0.01, | log2FC | to 1.25, then log2 transform the data, further using Bayes’ function to compute moderated t-statistics, moderated F-statistic, and log-odds of differential empirical Bayes moderation values of the standard common toward expression errors, and finally obtain the differential significance of each gene.
Weighted Gene Coexpression Network Analysis
Weighted gene coexpression network analysis can identify gene sets with highly synergistic changes to recognize potential biomarker genes or therapeutic targets based on the correlation of phenotypes of gene sets (Wisniewski et al., 2013). Specifically, we calculated the Median Absolute Deviation (MAD) for each gene separately based on the previously downloaded gene expression profiling data and then eliminated the top 95% of the genes with the smallest MAD. Scale-free co-expression networks were further constructed by removing outlying genes and samples using the Good Samples expression method of the R software package WGCNA. Afterward, we analyzed all genes using Pearson’s correlation matrix and average linkage. The power function amn = | Cmn | ^β (set the soft threshold β to 6) is used to transform the adjacency matrix into a topological overlap matrix (TOM), and the corresponding difference (1-TOM) is calculated. To classify genes with similar expression profiles as gene modules, we performed hierarchical clustering based on the difference metric of TOM with at least 30 genes per module of the gene dendrogram. To further analyze the modules, we calculated the difference of module eigengenes, merging modules with a distance less than 0.25.
Enrichment Analysis of Differentially Expressed Genes
We performed enrichment analysis using the R package clusterProfiler (version 4.2.1) to obtain results for gene set enrichment. P-values less than 0.05 were judged to be statistically significant. The obtained results have been visualized using R and Enrichr.
Protein-Protein Interaction Network Analysis of Differentially Expressed Genes
We created the PPI network through the STRING database (Szklarczyk et al., 2021). Specifically, the results obtained in the STRING database used a total score greater than 0.4 as a requirement to obtain AD-related DNA methylation key genes. The final results have been visualized using Cytoscape software (version 3.7.0) (Shannon et al., 2003).
Acquisition of Hub Genes
We used the plugin CytoHubba in Cytoscape to find the key genes in the PPI network (Shannon et al., 2003). Since the degree value of a protein correlates with the importance of a gene and proteins with higher degrees values are more likely to be key proteins (Chin et al., 2014), the algorithm of Degree was employed in our study to assess those genes. Obtained the top ten ranked genes as hub genes using the Degree algorithm.
Establishment and Analysis of the Receiver Operating Characteristic Curve
We constructed receiver operating characteristic (ROC) curves based on the pROC package in R software and further assessed the value of Hub genes in the GSE109887 dataset for the early diagnosis of AD by calculating the area under the ROC curve (AUC). The Hub gene was considered to have good diagnostic accuracy when the AUC value was greater than 0.6. The results were visualized using the ggplot package.
Results
Differentially Expressed Genes
In this study, we compared two datasets, GSE109887 and GSE97760, and analyzed differentially expressed genes in a total of 42 normal control samples and 55 AD patient samples using the Limma package in R software. In the GSE109887 dataset, according to the set criteria (P < 0.01, | log2FC| = 1.25), we obtained 7,736 DEGs, including 4,230 upregulated DEGs and 3,506 downregulated DEGs (Figure 1). By using WGCNA analysis, we obtained two enriched modules containing 194 upregulated genes and 628 downregulated genes (Figure 2), which were intersected with DEGs after limma analysis, respectively, to obtain the final 477 DEGs (including 131 upregulated genes and 346 downregulated genes) (Figure 3). In addition, we analyzed the expression levels of the top 10 DEGs in the blood of the GSE97760 dataset. Figures 4A,B are the cluster analysis of the top 10 DEGs (differential expression level and DNA methylation level, respectively). Table 1 lists the basic information of the differential expression level and DNA methylation level of the top 10 DEGs.
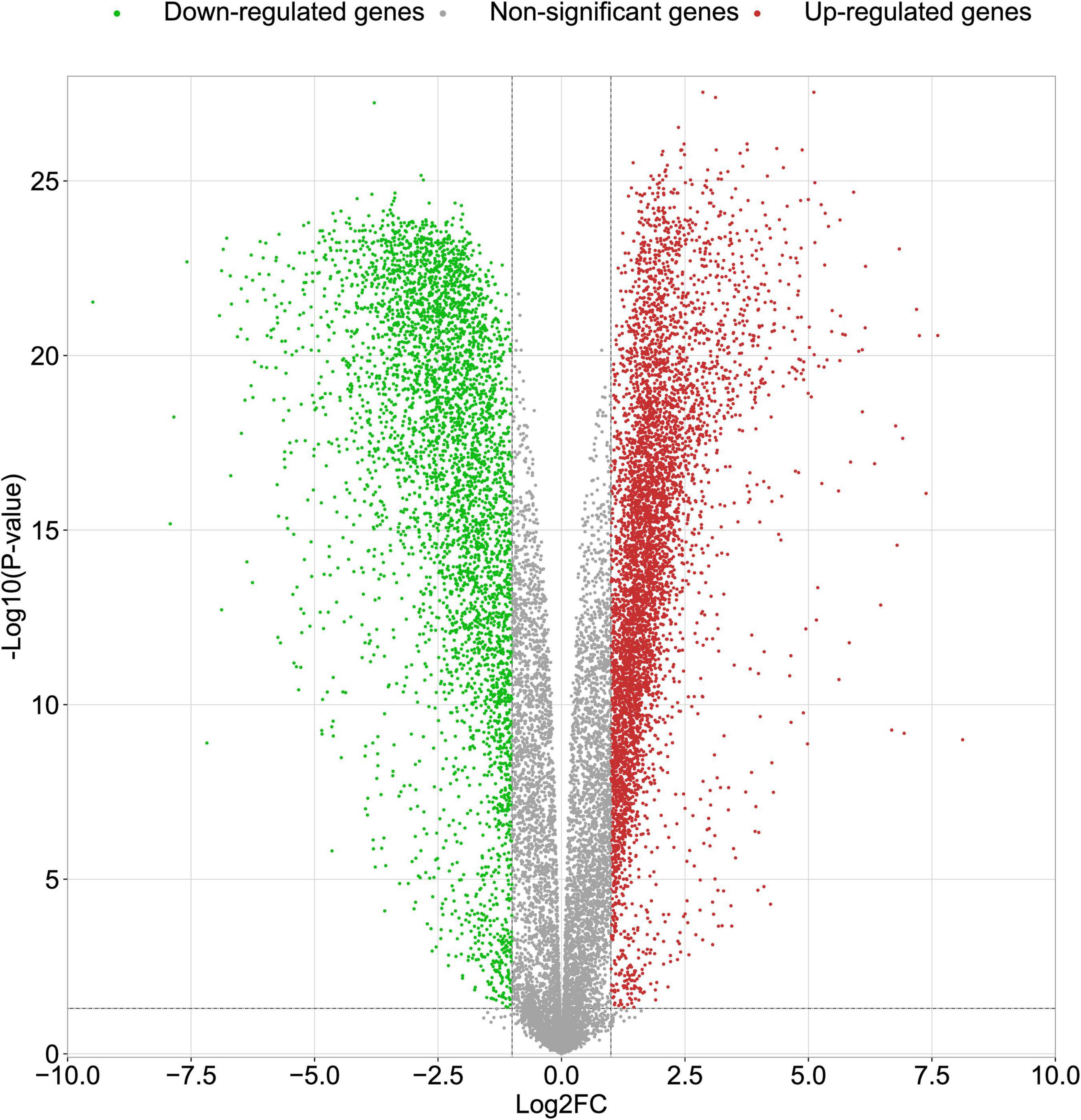
Figure 1. Volcano plots of DEGs in the GSE109887 datasets. The abscissa indicates fold change (Log2FC). The ordinate indicates –log10 (p value). Red dots indicate upregulated genes. Green dots indicate downregulated genes. Gray dots indicate non-significant genes.
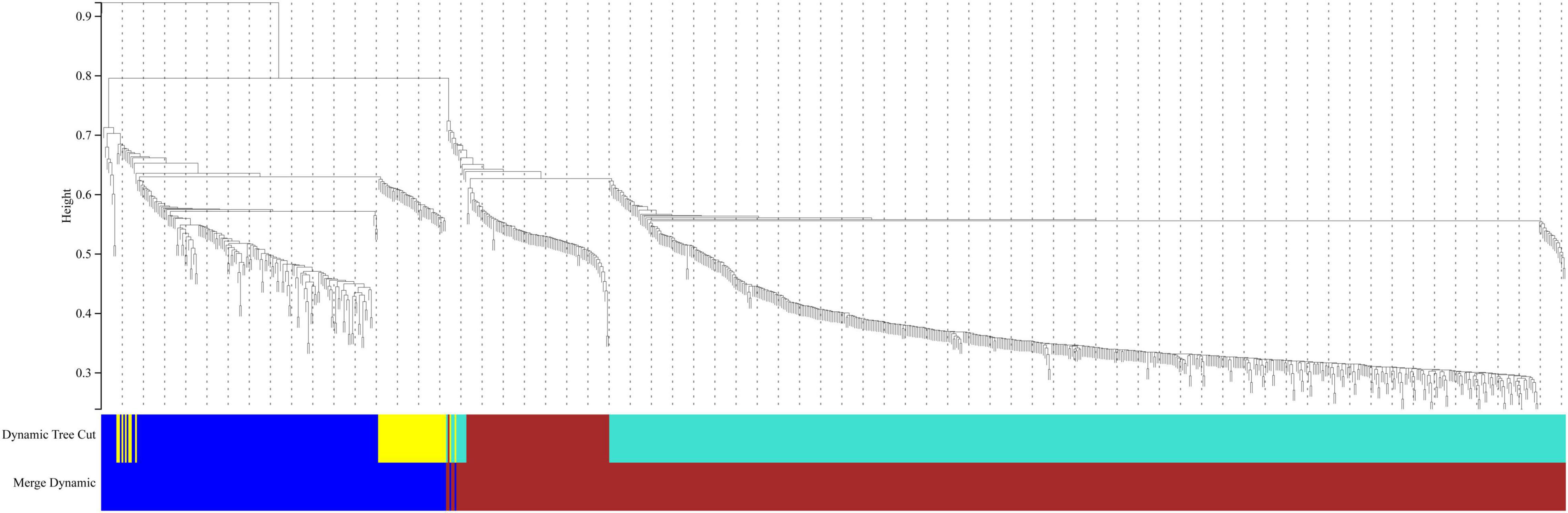
Figure 2. Cluster dendrogram of genes in the GSE109887 datasets. Gene dissimilarity based on 1-TOM. Different colors represent different modules of gene clustering.
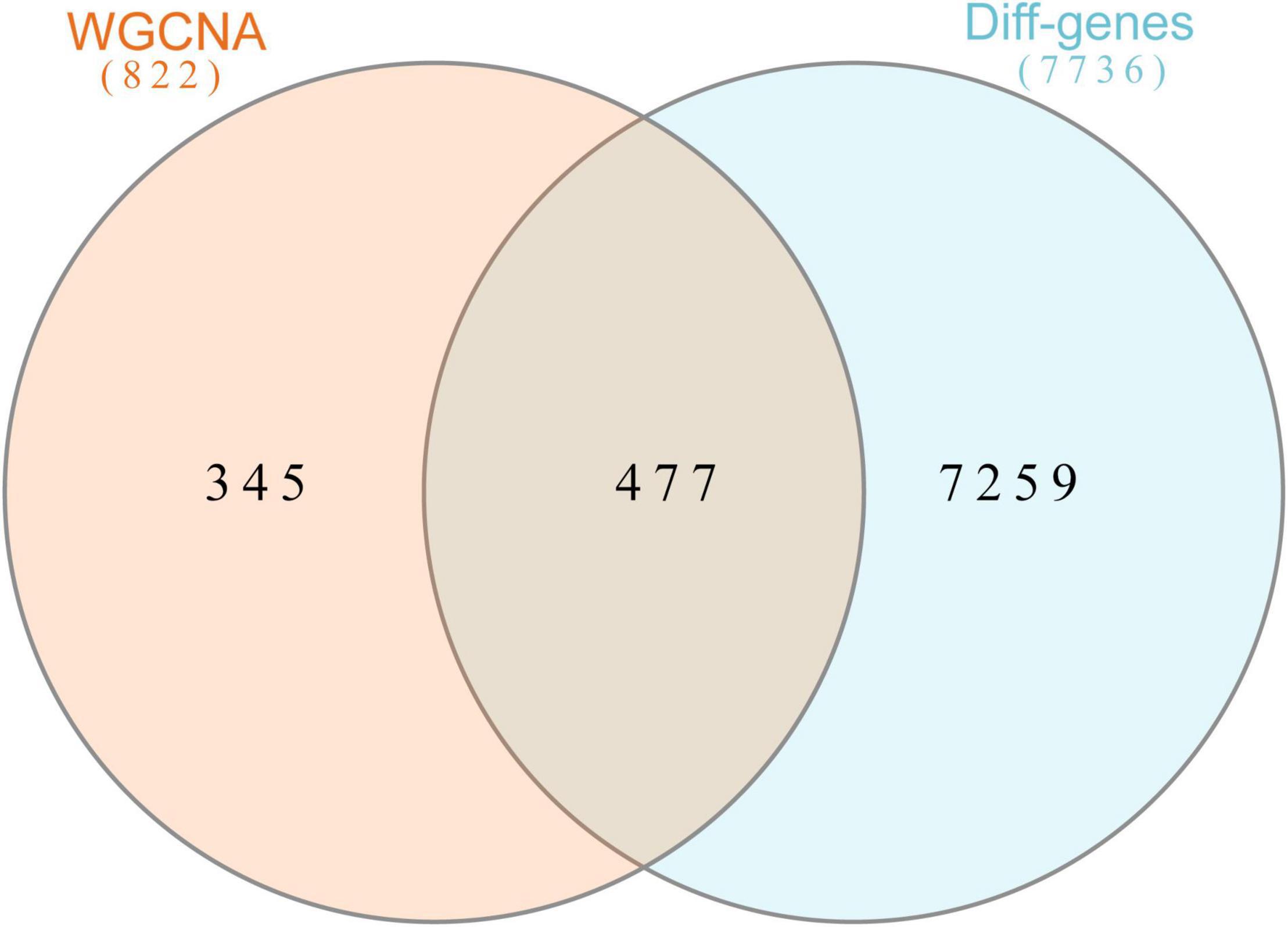
Figure 3. Overlapping genes in WGCNA versus DEGs were analyzed with Venn. Orange circle represents genes in WGCNA. Blue circle represents differential genes.
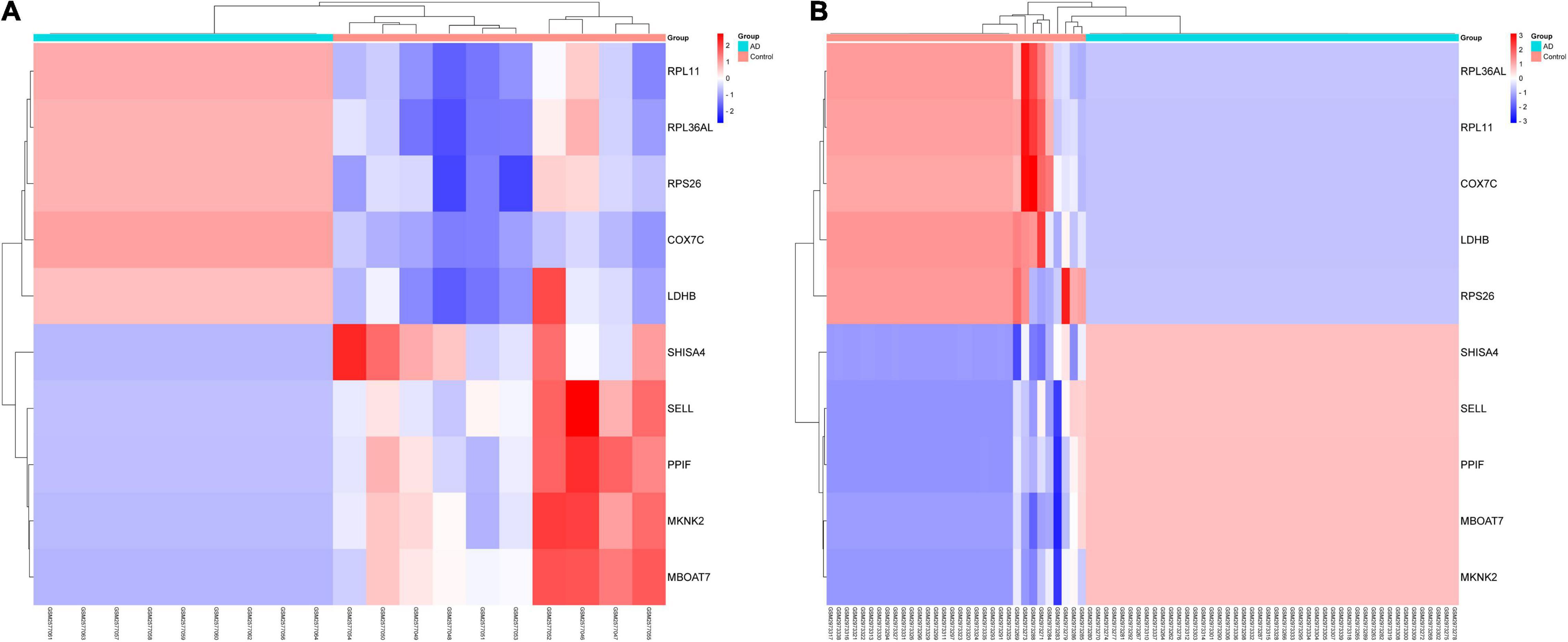
Figure 4. By analyzing two datasets, GSE97760 and GSE109887, a cluster heatmap of the top 10 DEGs between AD and normal controls was obtained. (A) Expression levels of DEGs. (B) DNA methylation of DEGs.
Gene Ontology and Pathway Enrichment Analysis of Differentially Expressed Genes
Gene Ontology (GO) consists of three aspects in the biological field: molecular function, cellular composition, and biological process (Ashburner et al., 2000; Gene Ontology Consortium, 2021). The Kyoto Encyclopedia of Genes and Genomes (KEGG) is a database that understands the advanced functions and utilities of biological systems (Kanehisa et al., 2022). Mastering the potential biological functions of genes can help to explore the role of DEGs in the pathogenesis of AD. Based on this, we performed enrichment analysis of DEGs in DNA methylation associated with AD. The results of GO analysis are presented in Table 2, which shows that these DEGs are significantly enriched in functions related to ribosomes and cytoplasm, involving biological processes including cytoplasmic translation, T cell activation, proliferation and cell-cell adhesion of leukocytes, and ribosomal biological processes (Figures 5A,B). KEGG pathway enrichment analysis indicated that these genes were significantly enriched in Coronavirus disease-COVID-19, Ribosome, Pathways of neurodegeneration-multiple diseases, Alzheimer’s disease, and Epstein-Barr virus infection (Figure 5C). Table 3 presents information on these pathways.
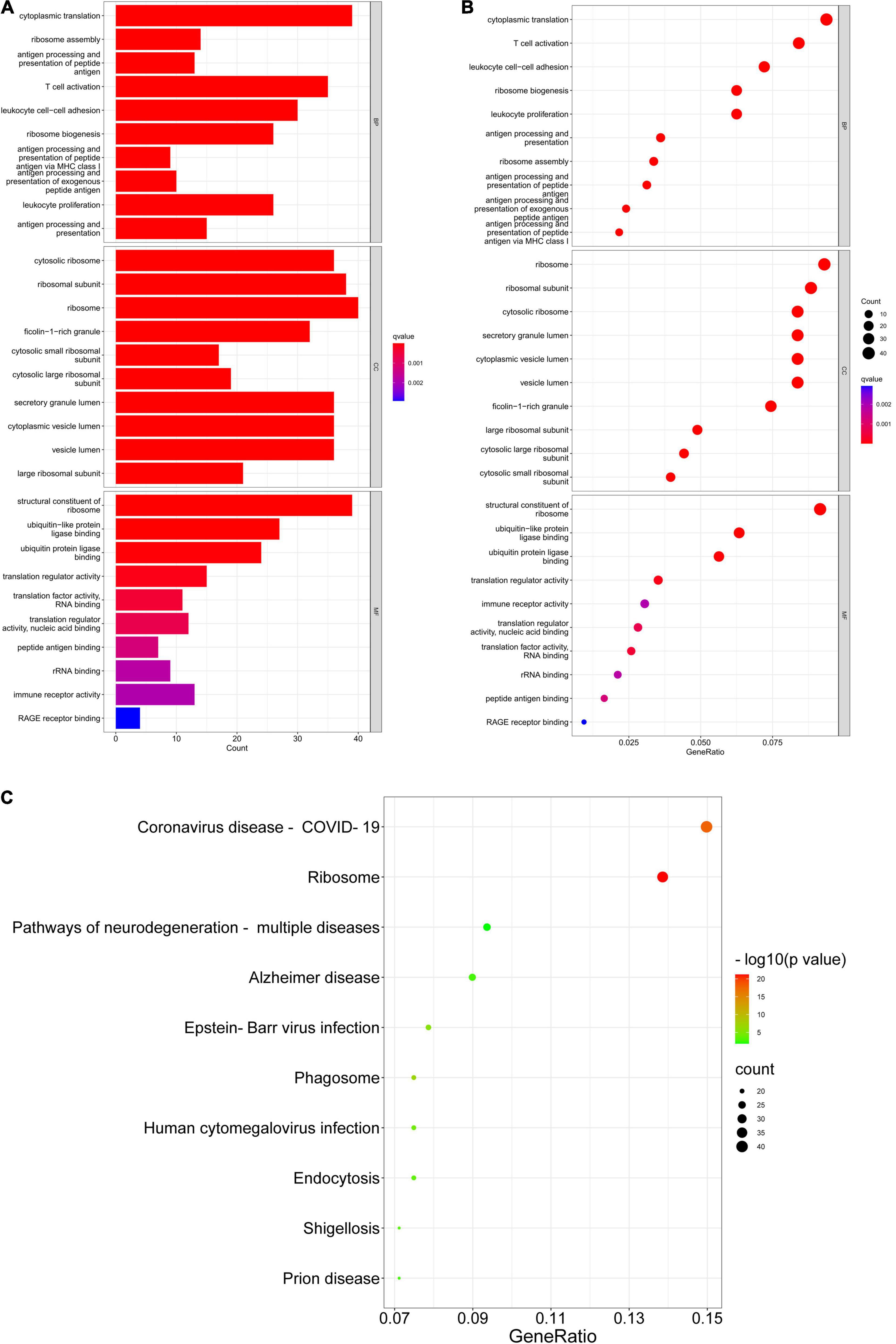
Figure 5. GO enrichment and KEGG pathway. (A,B) GO enrichment analysis of DEGs. (C) KEGG pathway analysis of DEGs.
The Protein-Protein Interaction Network and Hub Genes Analysis
We built a PPI network through the STRING database, and in addition to the nodes with interrupted networks, Figure 6A presents 308 nodes and 3,244 edges in the PPI network, where edges indicate interactions among genes. The top 10 Hub genes (RPL5, RPLP0, RPS15A, RPS18, RPS23, RPS27A, RPS29, RPS3, RPS6, and RPSA) were obtained based on Degree algorithm in CytoHubba (Figure 6B). Afterward we obtained the differential expression of these Hub genes without DNA methylation modification from the GSE97760 dataset and found that the expression of these genes was downregulated, contrary to the level of DNA methylation. Information about these Hub genes is listed in Table 4.
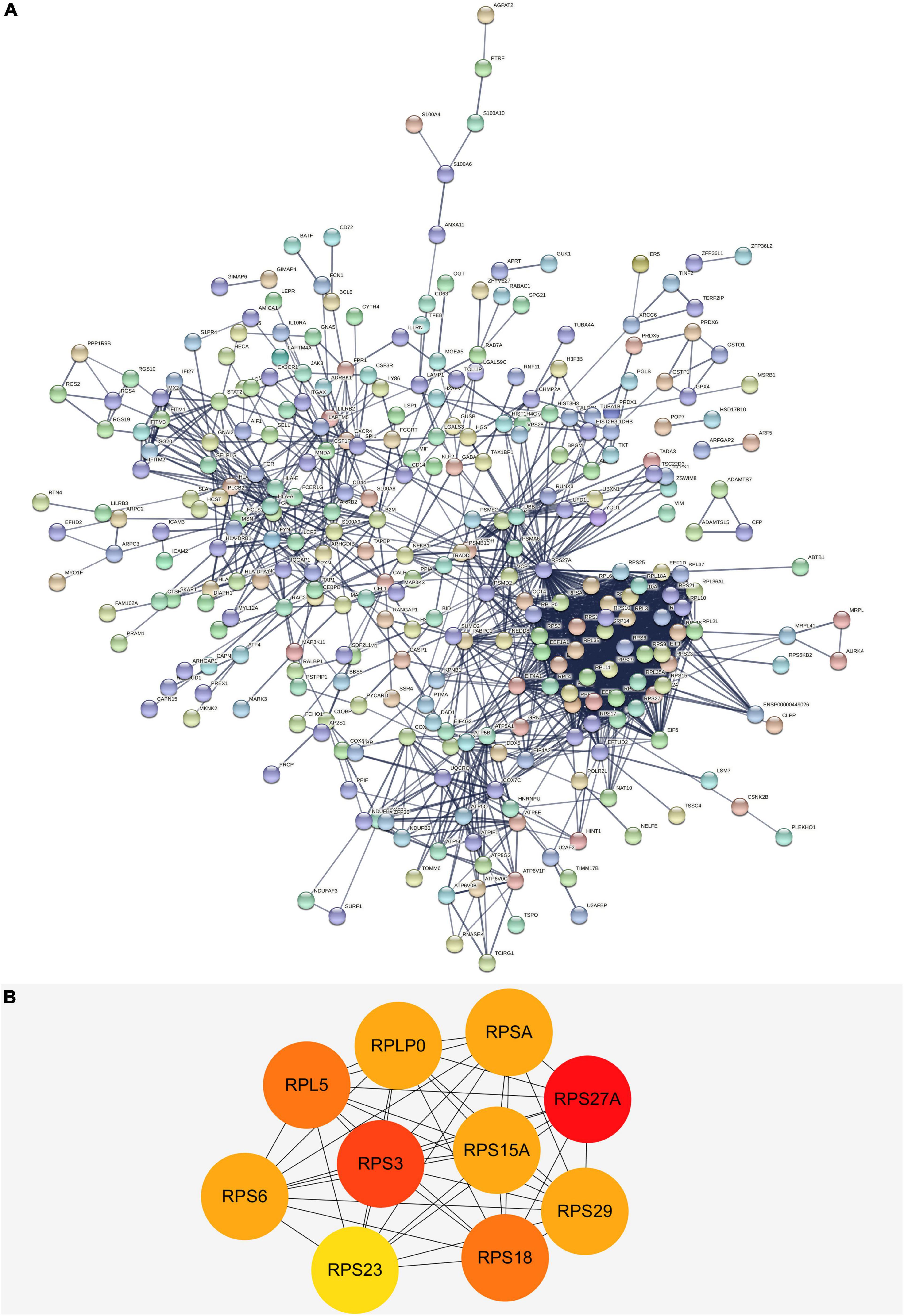
Figure 6. (A) The PPI network of DEGs in AD. There are a total of 308 nodes and 3,244 edges. Nodes represent proteins and edges represent interactions between proteins. (B) The top 10 hub genes with higher degree screened from a PPI network according to the Degree algorithm. Darker color indicates degree. PPI, protein-protein interaction; DEGs, differentially expressed genes.
Receiver Operating Characteristic Curve Analysis
We performed ROC curve analysis to validate the accuracy of Hub gene for the early diagnosis of AD. The closer the value of AUC is to 1, representing a greater diagnostic value. Our results showed that the top ten genes all had good diagnostic value. As shown in Figures 7A,B, the maximum AUC was 0.848 and the minimum AUC was 0.776. Among them, the AUC of RPS23, RPSA, and RPLP0 was greater than 0.8, indicating that these three genes may play a good accuracy in the early diagnosis of AD pathogenesis.
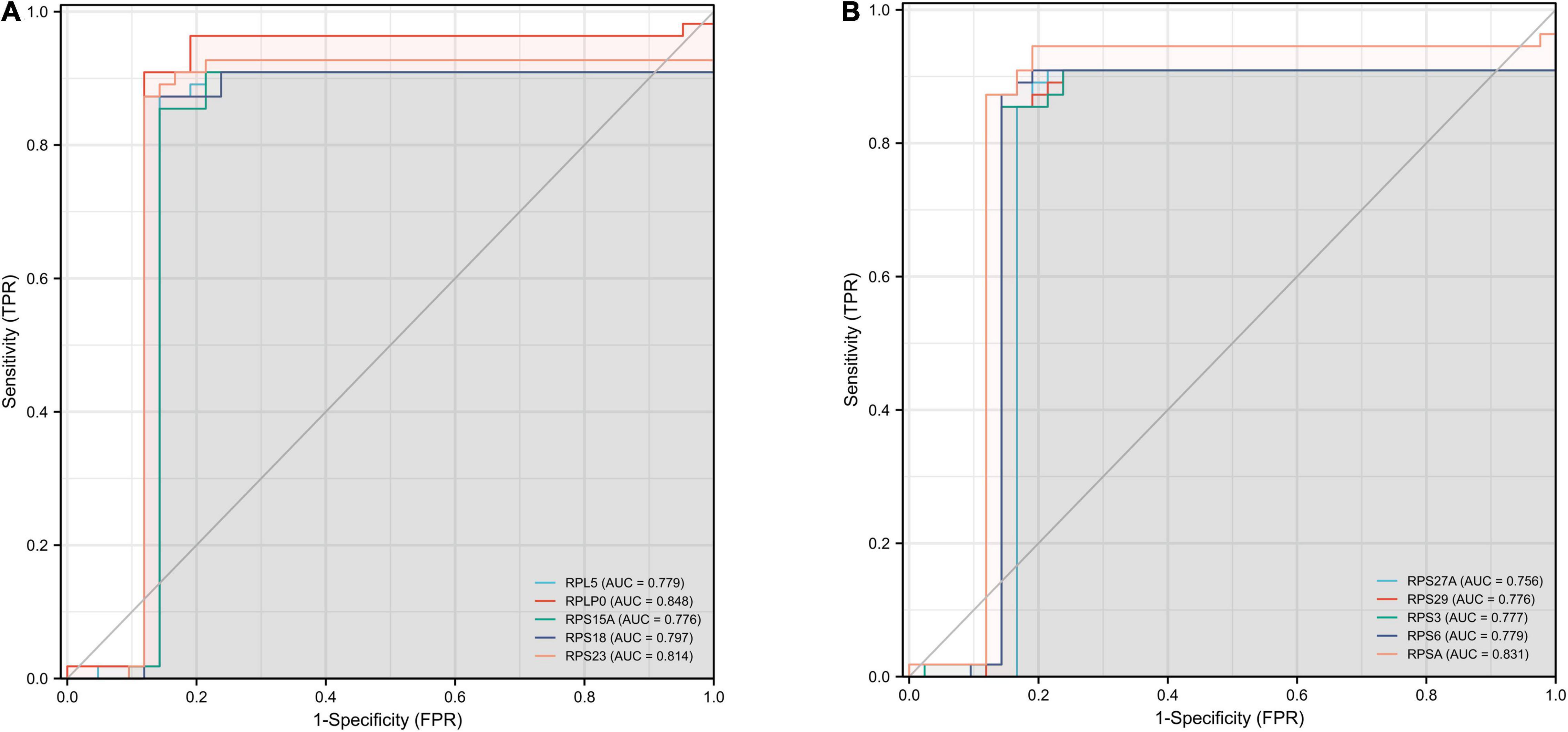
Figure 7. ROC curves for the top ten hub genes. The abscissa represents the FPR and the ordinate represents the TPR. The value of the AUC represents the accuracy of the diagnostic value, with values closer to 1 indicating better accuracy. ROC, operating receiver characteristic; FPR, false positive rate; TPR, true positive rate; AUC, area under the curve. (A) RPL5, RPLP0, RPS15A, RPS18, and RPS23. (B) RPS27A, RPS29, RPS3, RPS6, and RPSA.
Discussion
Over the past century, people have not stopped exploring AD. Many hypotheses have attempted to elucidate the pathogenesis of AD, such as oxidative stress, neuronal excitotoxicity, and tau hyperphosphorylation (Ju and Tam, 2022). In recent years, it has been demonstrated that epigenetic mechanisms play a significant part in the formation and development of memory during growth under physiological and pathological conditions, and are likely to participate in the pathogenesis and progression of AD (Sananbenesi and Fischer, 2009; Fischer et al., 2010; Day and Sweatt, 2011). DNA methylation, as one of the epigenetically important mechanisms, has been demonstrated to have a place in AD pathogenesis (Mohn et al., 2008; Qazi et al., 2018; Sharma et al., 2020), and genes and proteins associated with DNA methylation are potential biomarkers in AD (Qazi et al., 2018). However, the specific mechanisms underlying need to be further explored.
DNA methylation is the process of adding a methyl group to DNA mediated by DNA methyltransferases (DNMTs). The process does not involve changes in DNA sequence, but rather influences DNA activity and work ability (Coppedè, 2021). It is required for neuronal differentiation, embryonic development and regulation of gene expression levels, and is also involved in regulation of biological processes such as brain development and memory formation (Jones, 2012). Impairment of DNA methylation has already been shown to have a relationship with neural development and neurodegenerative diseases, in studies in primates and rodents, lack of dietary B-vitamins or excessive exposure to metals (lead) early in development can affect DNA methylation levels in vivo, resulting in changes in Aβ peptide gene expression associated with the pathogenesis of AD, such as BACE1, PSEN1, and APP genes (Fuso et al., 2005, 2011; Bihaqi et al., 2011). Impaired expression and activity of enzymes involved in DNA methylation and demethylation can also be detected in the brains of these animals (Fuso et al., 2011). Alterations in genes associated with DNA methylation and Aβ can also be detected in the brains of AD patients after death (Wang et al., 2008). This is consistent with a previous study concluding that alterations in DNA methylation is one of the causes of decreased neural function in the brain during aging (Liu et al., 2009). Reduced DNA methylation levels in mitochondria-associated regions can also be detected in peripheral blood of patients with delayed AD (De Jager et al., 2014). A study has shown that increased DNA methylation levels of the brain-derived neurotrophic factor (BDNF) promoter result in reduced BDNF mRNA protein expression and add a higher risk of AD development (Xie et al., 2017). In terms of AD treatment, some progress and success has been made in adding DNA methyl donors (folic acid and vitamin B12) to the diet of AD patients (Durga et al., 2007; Haan et al., 2007).
Based on the close link between DNA methylation and the development of AD, we downloaded datasets related to DNA methylation from the GEO data repository for bioinformatics analysis to obtain key genes implicated in AD pathogenesis. In our work, the DEGs in the GSE109887 dataset were first screened and additionally the expression of these DEGs when not DNA methylated was analyzed from the GSE97760 dataset. Afterward, these genes were subjected to GO and KEGG enrichment analysis. The results of GO enrichment analysis showed that cytoplasmic translation, ribosome production and assembly, and ubiquitination of proteins were engaged in the production and progression of AD. This is in line with prior studies indicating that DNA methylation plays an essential role in the advancement of AD. This indicates that the results of our biological information analysis are acceptable.
In our work, the top 10 DEGs of DNA methylation associated with AD were selected: RPL5, RPLP0, RPS15A, RPS18, RPS23, RPS27A, RPS29, RPS3, RPS6, and RPSA. Through the analysis results of the ROC curve, RPS23, RPSA, and RPLP0 all had very excellent diagnostic accuracy.
A recent experiment analyzing cell and molecular markers in donor brain samples by a combination of Fluoro deoxy glucose positron emission tomography (FDG-PET) and Allen Human Brain Atlas revealed that genes related to cytoplasmic ribosomes including RPLP0 showed high enrichment (Patel et al., 2020). Notably, studies on the synthesis of ribosomes and proteins in the cytosol have been demonstrated many years ago to be associated with mild cognitive impairment and AD pathogenesis (Albert et al., 2011). These results are in high agreement with our analysis of DEGs that the top ranked Hub genes have surprisingly high correlations with ribosomal proteins and indicate that ribosomal protein genes associated with DNA methylation may be a new risk locus for AD. Ribosomes, as organelles that regulate intracellular protein biosynthesis and translation, are closely related to cell development and the lifespan of organisms (Nosrati et al., 2014). There is no doubt that AD itself, as a disease with Tau protein hyperphosphorylation and Aβ misfolding, is inseparable from abnormalities in intracellular biological processes related to protein synthesis and DNA transcription.
Additionally, we analyzed the expression levels of Hub genes by GSE97760 dataset (Table 4), consistent with previous findings that differential expression of DNA methylation can affect gene activity and is usually associated with repression of gene expression (Coppieters et al., 2014). RPS23 is a highly conserved component of the 40S subunit in eukaryotes (Ma et al., 2020). It is involved in physiological and pathological processes such as tumorigenesis, immune signaling and growth and development (Zhou et al., 2015). Disturbed protein balance is a key factor leading to aging and age-related diseases, and translation is one of its key determinants (Hipp et al., 2019). And one of the most important roles of RPS23 is to maintain the fidelity of protein translational synthesis. Recently, studies have been conducted to explore how the fidelity of protein synthesis can be regulated to prolong the life span of organisms starting from RPS23 (Martinez-Miguel et al., 2021). Like genes encoding ribosomal proteins, RPS23 has multiple processed pseudogenes dispersed in the genome, of which closely related to AD is the RPS23RG family produced by the inversion of RPS23 (Huang et al., 2010). It has been shown that RPS23RG1 and RPS23RG2 can interact with adenylyl cyclase and upregulate cAMP levels, which further increases PKA activity, thereby limiting the activity of GSK-3, reducing Aβ production and tau hyperphosphorylation to resist the development of AD (Zhang et al., 2009; Hipp et al., 2019). Among them, RPS23RG1 has also been shown to be a gene essential for maintaining synaptic integrity and resisting AD-related cognitive deficits (Zhao et al., 2019). In our study, it was found that the DNA methylation level of RPS23 was highly upregulated in AD patients, which may downregulate the gene expression (Table 4). Based on the important function of RPS23 and our results, we hypothesized that the expression of RPS23 can be downregulated by DNA methylation and thus participate in the development of AD.
RPSA is a non-integrin laminin receptor, also known as 37/67 kDa high-affinity laminin receptor precursor/laminin receptor (LRP/LR; Jovanovic et al., 2015). Studies have shown that knockdown of RPSA can lead to neurodegenerative diseases through apoptosis (Lowe and Lin, 2000), which is consistent with our findings that the expression of RPSA in AD patients was downregulated through DNA methylation (Table 4). While some other studies have pointed out new insights of RPSA in AD. Da Costa Dias et al. (2013) found that RPSA co-localizes with Aβ42 on the cell surface, and can physically bind to peptides that synthesize Aβ42, and low activity of RPSA can lead to enhanced Aβ42 toxicity. Research on targeting RPSA for prion diseases has been reported (Jovanovic et al., 2015), and the development of RPSA-targeted drugs can provide a new perspective for the treatment of AD.
RPLP0 is an acidic ribosomal protein capable of mediating cell cycle arrest and apoptosis induced by phospholipase A and acyltransferase 4 (PLAAT4; Ban et al., 2014). It has been shown that RPLP0 can interact with PLAAT4, and a significant reduction in cell survival has been observed in cells that overexpress PLAAT4 or knockdown RPLP0 (Wang et al., 2019). RPLP0 was essential for maintaining ribosome activity (Remacha et al., 1995), and downregulation of RPLP0 mRNA can mediate Endoplasmic reticulum stress response to induce abnormal autophagy (Artero-Castro et al., 2015). In K369I tau transgenic mice and rTg4510 tau transgenic mice, it was found that tau hyperphosphorylation resulted in decreased expression of RPLP0 (Evans et al., 2019). Similarly, in our study, the upregulated DNA methylation level of RPLP0 in AD patients could lead to a decrease in the expression level of genes (Table 4).
Although our study found some genes with DNA methylation associated with AD development, and on this basis, the accuracy of Hub gene for the early diagnosis of AD was analyzed. However, the conclusions of our study still have some deficiencies. All of our research results are based on GEO public database and already published data, and due to sample size and platform limitations, further biological or clinical experiments are needed to confirm our conclusions in the future. But, in general, these hub genes provide new insights and directions for explaining AD pathogenesis and may become potential biomarkers and targeted therapies for accurate diagnosis and therapy of early AD in the near future.
Conclusion
In summary, our study identified the top 10 hub genes associated with AD-related DNA methylation. These findings indicate that these genes may have participated in the pathological process of AD. Among them, RPS23, RPSA, and RPLP0 have high diagnostic accuracy and excellent AD biomarker potential, which is worthy of further attention and research.
Data Availability Statement
The datasets presented in this study can be found in online repositories. The names of the repository/repositories and accession number(s) can be found below: https://www.ncbi.nlm.nih.gov/, GSE109887; https://www.ncbi.nlm.nih.gov/, GSE97760.
Author Contributions
XH provided the experimental ideas of this study and reviewed and revised the first draft of the manuscript. FC and NW did the data collection and verified by XH. FC did the data analysis, visualization of results, and wrote the first draft of the manuscript. All authors contributed to the article and approved the submitted version.
Funding
This project was supported by grants from the High-Level Talent Research Start-up Fund Project of Dali University (KY1916103740).
Conflict of Interest
The authors declare that the research was conducted in the absence of any commercial or financial relationships that could be construed as a potential conflict of interest.
Publisher’s Note
All claims expressed in this article are solely those of the authors and do not necessarily represent those of their affiliated organizations, or those of the publisher, the editors and the reviewers. Any product that may be evaluated in this article, or claim that may be made by its manufacturer, is not guaranteed or endorsed by the publisher.
Acknowledgments
FC would like to thank XH for her guidance and assistance, and in addition, the GEO database for providing information on the datasets used in this study.
References
Albert, M. S., DeKosky, S. T., Dickson, D., Dubois, B., Feldman, H. H., Fox, N. C., et al. (2011). The diagnosis of mild cognitive impairment due to Alzheimer’s disease: recommendations from the National institute on aging-Alzheimer’s association workgroups on diagnostic guidelines for Alzheimer’s disease. Alzheimers Dement 7, 270–279. doi: 10.1016/j.jalz.2011.03.008
Artero-Castro, A., Perez-Alea, M., Feliciano, A., Leal, J. A., Genestar, M., Castellvi, J., et al. (2015). Disruption of the ribosomal P complex leads to stress-induced autophagy. Autophagy 11, 1499–1519. doi: 10.1080/15548627.2015.1063764
Ashburner, M., Ball, C. A., Blake, J. A., Botstein, D., Butler, H., Cherry, J. M., et al. (2000). Gene ontology: tool for the unification of biology. The Gene Ontology Consortium. Nat Genet 25, 25–29. doi: 10.1038/75556
Ballard, C., Gauthier, S., Corbett, A., Brayne, C., Aarsland, D., and Jones, E. (2011). Alzheimer’s disease. Lancet(Lond Engl) 377, 1019–1031. doi: 10.1016/S0140-6736(10)61349-9
Ban, N., Beckmann, R., Cate, J. H., Dinman, J. D., Dragon, F., Ellis, S. R., et al. (2014). A new system for naming ribosomal proteins. Curr. Opin. Struct. Biol. 24, 165–169. doi: 10.1016/j.sbi.2014.01.002
Bihaqi, S. W., Huang, H., Wu, J., and Zawia, N. H. (2011). Infant exposure to lead (Pb) and epigenetic modifications in the aging primate brain: implications for Alzheimer’s disease. J. Alzheimers Dis. 27, 819–833. doi: 10.3233/JAD-2011-111013
Chin, C. H., Chen, S. H., Wu, H. H., Ho, C. W., Ko, M. T., and Lin, C. Y. (2014). cytoHubba: identifying hub objects and sub-networks from complex interactome. BMC Syst. Biol. 8 (Suppl. 4):S11. doi: 10.1186/1752-0509-8-S4-S11
Coppedè, F. (2021). Epigenetic regulation in Alzheimer’s disease: is it a potential therapeutic target? Expert Opin. Ther. Targets 25, 283–298. doi: 10.1080/14728222.2021.1916469
Coppieters, N., Dieriks, B. V., Lill, C., Faull, R. L., Curtis, M. A., and Dragunow, M. (2014). Global changes in DNA methylation and hydroxymethylation in Alzheimer’s disease human brain. Neurobiol. Aging 35, 1334–1344. doi: 10.1016/j.neurobiolaging.2013.11.031
Da Costa Dias, B., Jovanovic, K., Gonsalves, D., Moodley, K., Reusch, U., Knackmuss, S., et al. (2013). Anti-LRP/LR specific antibody IgG1-iS18 and knock-down of LRP/LR by shRNAs rescue cells from Aβ42 induced cytotoxicit. Sci. Rep. 3:2702. doi: 10.1038/srep02702
Day, J. J., and Sweatt, J. D. (2011). Epigenetic mechanisms in cognition. Neuron 70, 813–829. doi: 10.1016/j.neuron.2011.05.019
De Jager, P. L., Srivastava, G., Lunnon, K., Burgess, J., Schalkwyk, L. C., Yu, L., et al. (2014). Alzheimer’s disease: early alterations in brain DNA methylation at ANK1, BIN1, RHBDF2 and other loci. Nat. Neurosci. 17, 1156–1163. doi: 10.1038/nn.3786
De Roeck, A., Van Broeckhoven, C., and Sleegers, K. (2019). The role of ABCA7 in Alzheimer’s disease: evidence from genomics, transcriptomics and methylomics. Acta Neuropathol. 138, 201–220. doi: 10.1007/s00401-019-01994-1
Durga, J., van Boxtel, M. P., Schouten, E. G., Kok, F. J., Jolles, J., Katan, M. B., et al. (2007). Effect of 3-year folic acid supplementation on cognitive function in older adults in the FACIT trial: a randomised, double blind, controlled trial. Lancet 369, 208–216. doi: 10.1016/S0140-6736(07)60109-3
Edgar, R., Domrachev, M., and Lash, A. E. (2002). Gene expression Omnibus: NCBI gene expression and hybridization array data repository. Nucleic Acids Res. 30, 207–210. doi: 10.1093/nar/30.1.207
Evans, H. T., Benetatos, J., van Roijen, M., Bodea, L. G., and Götz, J. (2019). Decreased synthesis of ribosomal proteins in tauopathy revealed by non-canonical amino acid labelling. EMBO J. 38:e101174. doi: 10.15252/embj.2018101174
Fischer, A., Sananbenesi, F., Mungenast, A., and Tsai, L.-H. (2010). Targeting the correct HDAC(s) to treat cognitive disorders. Trends Pharmacol. Sci. 31, 605–617. doi: 10.1016/j.tips.2010.09.003
Freudenberg-Hua, Y., Li, W., and Davies, P. (2018). The role of genetics in advancing precision medicine for Alzheime’s Disease-a narrative review. Front. Med. (Lausanne) 5:108. doi: 10.3389/fmed.2018.00108
Fuso, A., Nicolia, V., Cavallaro, R. A., and Scarpa, S. (2011). DNA methylase and demethylase activities are modulated by one-carbon metabolism in Alzheimer’s disease models. J. Nutr. Biochem. 22, 242–251. doi: 10.1016/j.jnutbio.2010.01.010
Fuso, A., Seminara, L., Cavallaro, R. A., D’Anselmi, F., and Scarpa, S. (2005). S-adenosylmethionine/homocysteine cycle alterations modify DNA methylation status with consequent deregulation of PS1 and BACE and beta-amyloid production. Mol. Cell Neurosci. 28, 195–204. doi: 10.1016/j.mcn.2004.09.007
Gasparoni, G., Bultmann, S., Lutsik, P., Kraus, T. F. J., Sordon, S., Vlcek, J., et al. (2018). DNA methylation analysis on purified neurons and glia dissects age and Alzheimer’s disease-specific changes in the human cortex. Epigenetics Chromatin 11:41. doi: 10.1186/s13072-018-0211-3
Gatz, M., Reynolds, C. A., Fratiglioni, L., Johansson, B., Mortimer, J. A., Berg, S., et al. (2006). Role of genes and environments for explaining Alzheimer disease. Arch. Gen. Psychiatry 63, 168–174. doi: 10.1001/archpsyc.63.2.168
Gauthier, S., Rosa-Neto, P., Morais, J. A., and Webster, C. (2021)). World Alzheimer Report 2021: Journey through the Diagnosis of Dementia. London: Alzheimer’s Disease International.
Gene Ontology Consortium (2021). The gene ontology resource: enriching a GOld mine. Nucleic Acids Res. 49, D325–D334. doi: 10.1093/nar/gkaa1113
Haan, M. N., Miller, J. W., Aiello, A. E., Whitmer, R. A., Jagust, W. J., Mungas, D. M., et al. (2007). Homocysteine, B vitamins, and the incidence of dementia and cognitive impairment: results from the sacramento area latino study on aging. Am. J. Clin. Nutr. 85, 511–517. doi: 10.1093/ajcn/85.2.511
Hardan, L., Filtchev, D., Kassem, R., Bourgi, R., Lukomska-Szymanska, M., Tarhini, H., et al. (2021). COVID-19 and Alzheimer’s Disease: a literature review. Medicina (Kaunas) 57:1159. doi: 10.3390/medicina57111159
Hipp, M. S., Kasturi, P., and Hartl, F. U. (2019). The proteostasis network and its decline in ageing. Nat. Rev. Mol. Cell Biol. 20, 421–435. doi: 10.1038/s41580-019-0101-y
Huang, X., Chen, Y., Li, W. B., Cohen, S. N., Liao, F. F., Li, L., et al. (2010). The Rps23rg gene family originated through retroposition of the ribosomal protein s23 mRNA and encodes proteins that decrease Alzheimer’s beta-amyloid level and tau phosphorylation. Hum. Mol. Genet. 19, 3835–3843. doi: 10.1093/hmg/ddq302
Humphries, C., Kohli, M. A., Whitehead, P., Mash, D. C., Pericak-Vance, M. A., and Gilbert, J. (2015). Alzheimer disease (AD) specific transcription, DNA methylation and splicing in twenty AD associated loci. Mol. Cell Neurosci. 67, 37–45. doi: 10.1016/j.mcn.2015.05.003
Jones, P. A. (2012). Functions of DNA methylation: islands, start sites, gene bodies and beyond. Nat. Rev. Genet. 13, 484–492. doi: 10.1038/nrg3230
Jovanovic, K., Chetty, C. J., Khumalo, T., Da Costa Dias, B., Ferreira, E., Malindisa, S. T., et al. (2015). Novel patented therapeutic approaches targeting the 37/67 kDa laminin receptor for treatment of cancer and Alzheimer’s disease. Expert Opin. Ther. Pat. 25, 567–582. doi: 10.1517/13543776.2015.1014802
Ju, Y., and Tam, K. Y. (2022). Pathological mechanisms and therapeutic strategies for Alzheimer’s disease. Neural Regen. Res. 17, 543–549. doi: 10.4103/1673-5374.320970
Kanehisa, M., Sato, Y., and Kawashima, M. (2022). KEGG mapping tools for uncovering hidden features in biological data. Protein Sci. 31, 47–53. doi: 10.1002/pro.4172
Khayer, N., Mirzaie, M., Marashi, S. A., and Jalessi, M. (2020). Rps27a might act as a controller of microglia activation in triggering neurodegenerative diseases. PLoS One 15:e0239219. doi: 10.1371/journal.pone.0239219
Kim, W., Youn, H., Lee, S., Kim, E., Kim, D., Sub Lee, J., et al. (2018). RNF138-mediated ubiquitination of rpS3 is required for resistance of glioblastoma cells to radiation-induced apoptosis. Exp. Mol. Med. 50:e434. doi: 10.1038/emm.2017.247
Lardenoije, R., Roubroeks, J. A. Y., Pishva, E., Leber, M., Wagner, H., Iatrou, A., et al. (2019). Alzheimer’s disease-associated (hydroxy)methylomic changes in the brain and blood. Clin. Epigenetics 11:164. doi: 10.1186/s13148-019-0755-5
Li, T., Wei, Y., Qu, M., Mou, L., Miao, J., Xi, M., et al. (2021). Formaldehyde and De/methylation in age-related cognitive impairment. Genes (Basel) 12:913. doi: 10.3390/genes12060913
Liu, L., van Groen, T., Kadish, I., and Tollefsbol, T. O. (2009). DNA methylation impacts on learning and memory in aging. Neurobiol. Aging 30, 549–560. doi: 10.1016/j.neurobiolaging.2007.07.020
Lowe, S. W., and Lin, A. W. (2000). Apoptosis in cancer. Carcinogenesis 21, 485–495. doi: 10.1093/carcin/21.3.485
Ma, G., Liu, M., Du, K., Zhong, X., Gong, S., Jiao, L., et al. (2019). Differential expression of mRNAs in the brain tissues of patients with Alzheimer’s disease based on GEO expression profile and its clinical significance. Biomed. Res. Int. 2019:8179145. doi: 10.1155/2019/8179145
Ma, Z., Qu, B., Yao, L., Gao, Z., and Zhang, S. (2020). Identification and functional characterization of ribosomal protein S23 as a new member of antimicrobial protein. Dev. Comp. Immunol. 110:103730. doi: 10.1016/j.dci.2020.103730
Martinez-Miguel, V. E., Lujan, C., Espie-Caullet, T., Martinez-Martinez, D., Moore, S., Backes, C., et al. (2021). Increased fidelity of protein synthesis extends lifespan. Cell Metab. 33, 2288–2300.e12. doi: 10.1016/j.cmet.2021.08.017
Mishra, R., and Li, B. (2020). The application of artificial intelligence in the genetic study of Alzheimer’s Disease. Aging Dis. 11, 1567–1584. doi: 10.14336/AD.2020.0312
Mohn, F., Weber, M., Rebhan, M., Roloff, T. C., Richter, J., Stadler, M. B., et al. (2008). Lineage-specific polycomb targets and de novo DNA methylation define restriction and potential of neuronal progenitors. Mol. Cell 30, 755–766. doi: 10.1016/j.molcel.2008.05.007
Mok, V. C. T., Pendlebury, S., Wong, A., Alladi, S., Au, L., Bath, P. M., et al. (2020). Tackling challenges in care of Alzheimer’s disease and other dementias amid the COVID-19 pandemic, now and in the future. Alzheimers Dement 16, 1571–1581. doi: 10.1002/alz.12143
Nakamura, A., Kaneko, N., Villemagne, V. L., Kato, T., Doecke, J., Doré, V., et al. (2018). High performance plasma amyloid-β biomarkers for Alzheimer’s disease. Nature 554, 249–254. doi: 10.1038/nature25456
Nosrati, N., Kapoor, N. R., and Kumar, V. (2014). Combinatorial action of transcription factors orchestrates cell cycle-dependent expression of the ribosomal protein genes and ribosome biogenesis. FEBS J. 281, 2339–2352. doi: 10.1111/febs.12786
Ozaki, Y., Yoshino, Y., Yamazaki, K., Sao, T., Mori, Y., Ochi, S., et al. (2017). DNA methylation changes at TREM2 intron 1 and TREM2 mRNA expression in patients with Alzheimer’s disease. J. Psychiatr. Res. 92, 74–80. doi: 10.1016/j.jpsychires.2017.04.003
Patel, D., Mez, J., Vardarajan, B. N., Staley, L., Chung, J., Zhang, X., et al. (2019). Association of rare coding mutations with Alzheimer disease and other dementias among adults of European ancestry. JAMA Netw. Open 2:e191350. doi: 10.1001/jamanetworkopen.2019.1350
Patel, S., Howard, D., Man, A., Schwartz, D., Jee, J., Felsky, D., et al. (2020). Donor-specific transcriptomic analysis of Alzheimer’s disease-associated hypometabolism highlights a unique donor, ribosomal proteins and microglia. eNeuro 7, ENEURO.255–ENEURO.220. doi: 10.1523/ENEURO.0255-20.2020
Paziewska, A., Dabrowska, M., Goryca, K., Antoniewicz, A., Dobruch, J., Mikula, M., et al. (2014). DNA methylation status is more reliable than gene expression at detecting cancer in prostate biopsy. Br. J. Cancer 111, 781–789. doi: 10.1038/bjc.2014.337
Pimenova, A. A., Raj, T., and Goate, A. M. (2018). Untangling genetic risk for Alzheimer’s disease. Biol. Psychiatry 83, 300–310. doi: 10.1016/j.biopsych.2017.05.014
Qazi, T. J., Quan, Z., Mir, A., and Qing, H. (2018). Epigenetics in Alzheimer’s disease: perspective of DNA methylation. Mol. Neurobiol. 55, 1026–1044. doi: 10.1007/s12035-016-0357-6
Querfurth, H. W., and LaFerla, F. M. (2010). Alzheimer’s disease. N. Engl. J. Med. 362, 329–344. doi: 10.1056/NEJMra0909142
Ray, M., and Zhang, W. (2010). Analysis of Alzheimer’s disease severity across brain regions by topological analysis of gene co-expression networks. BMC Syst. Biol. 4:136. doi: 10.1186/1752-0509-4-136
Remacha, M., Jimenez-Diaz, A., Santos, C., Briones, E., Zambrano, R., Rodriguez Gabriel, M. A., et al. (1995). Proteins P1, P2, and P0, components of the eukaryotic ribosome stalk. new structural and functional aspects. Biochem. Cell Biol. 73, 959–968. doi: 10.1139/o95-103
Safran, M., Dalah, I., Alexander, J., Rosen, N., Iny Stein, T., Shmoish, M., et al. (2010). GeneCards version 3: the human gene integrator. Database (Oxford) 2010:baq020. doi: 10.1093/database/baq020
Sananbenesi, F., and Fischer, A. (2009). The epigenetic bottleneck of neurodegenerative and psychiatric diseases. Biol. Chem. 90, 1145–1153. doi: 10.1515/BC.2009.131
Shannon, P., Markiel, A., Ozier, O., Baliga, N. S., Wang, J. T., Ramage, D., et al. (2003). Cytoscape: a software environment for integrated models of biomolecular interaction networks. Genome Res. 13, 2498–2504. doi: 10.1101/gr.1239303
Sharma, V. K., Mehta, V., and Singh, T. G. (2020). Alzheimer’s disorder: epigenetic connection and associated risk factors. Curr. Neuropharmacol. 18, 740–753. doi: 10.2174/1570159X18666200128125641
Sun, B. L., Li, W. W., Zhu, C., Jin, W. S., Zeng, F., Liu, Y. H., et al. (2018). Clinical research on Alzheimer’s Disease: progress and perspectives. Neurosci. Bull. 34, 1111–1118. doi: 10.1007/s12264-018-0249-z
Szklarczyk, D., Gable, A. L., Nastou, K. C., Lyon, D., Kirsch, R., Pyysalo, S., et al. (2021). The STRING database in 2021: customizable protein–protein networks, and functional characterization of user-uploaded gene/measurement sets. Nucleic Acids Res. 49, D605–D612.
Todd, S., Barr, S., Roberts, M., and Passmore, A. P. (2013). Survival in dementia and predictors of mortality: a review. Int. J. Geriatr. Psychiatry 28, 1109–1124. doi: 10.1002/gps.3946
Wang, C. H., Wang, L. K., Wu, C. C., Chen, M. L., Lee, M. C., Lin, Y. Y., et al. (2019). The ribosomal protein RPLP0 mediates PLAAT4-induced cell cycle arrest and cell apoptosis. Cell Biochem. Biophys. 77, 253–260. doi: 10.1007/s12013-019-00876-3
Wang, S. C., Oelze, B., and Schumacher, A. (2008). Age-specific epigenetic drift in late-onset Alzheimer’s disease. PLoS One 3:e2698. doi: 10.1371/journal.pone.0002698
Wisniewski, N., Cadeiras, M., Bondar, G., Cheng, R. K., Shahzad, K., Onat, D., et al. (2013). Weighted gene coexpression network analysis (WGCNA) modeling of multiorgan dysfunction syndrome after mechanical cir-culatory support therapy. J. Heart Lung Transpl. 32:S223. doi: 10.1016/j.healun.2013.01.565
World Health Organization (2021). Global Status Report on the Public Health Response to Dementia. Geneva: World Health Organization.
Xie, B., Liu, Z., Liu, W., Jiang, L., Zhang, R., Cui, D., et al. (2017). DNA methylation and Tag SNPs of the BDNF gene in conversion of amnestic mild cognitive impairment into Alzheimer’s disease: a cross-sectional cohort study. J. Alzheimers Dis. 58, 263–274. doi: 10.3233/JAD-170007
Yu, L., Chibnik, L. B., Srivastava, G. P., Pochet, N., Yang, J., Xu, J., et al. (2015). Association of brain DNA methylation in SORL1, ABCA7, HLA-DRB5, SLC24A4, and BIN1 with pathological diagnosis of Alzheimer disease. JAMA Neurol. 72, 15–24. doi: 10.1001/jamaneurol.2014.3049
Zhang, C., Fu, J., Xue, F., Ryu, B., Zhang, T., Zhang, S., et al. (2016). Knockdown of ribosomal protein S15A induces human glioblastoma cell apoptosis. World J. Surg. Oncol. 14:129. doi: 10.1186/s12957-016-0891-8
Zhang, Y. W., Liu, S., Zhang, X., Li, W. B., Chen, Y., Huang, X., et al. (2009). A functional mouse retroposed gene Rps23r1 reduces Alzheimer’s beta-amyloid levels and tau phosphorylation. Neuron 64, 328–340. doi: 10.1016/j.neuron.2009.08.036
Zhao, D., Meng, J., Zhao, Y., Huo, Y., Liu, Y., Zheng, N., et al. (2019). RPS23RG1 is required for synaptic integrity and rescues Alzheimer’s disease-associated cognitive deficits. Biol. Psychiatry 86, 171–184. doi: 10.1016/j.biopsych.2018.08.009
Keywords: Alzheimer’s disease, differentially expressed genes (DEGs), hub genes, DNA methylation, bioinformatics
Citation: Chen F, Wang N and He X (2022) Identification of Differential Genes of DNA Methylation Associated With Alzheimer’s Disease Based on Integrated Bioinformatics and Its Diagnostic Significance. Front. Aging Neurosci. 14:884367. doi: 10.3389/fnagi.2022.884367
Received: 26 February 2022; Accepted: 19 April 2022;
Published: 09 May 2022.
Edited by:
Vivek Swarup, University of California, Irvine, United StatesReviewed by:
Carlos J. Rodriguez-Ortiz, University of California, Irvine, United StatesMuthuswamy Anusuyadevi, Bharathidasan University, India
Copyright © 2022 Chen, Wang and He. This is an open-access article distributed under the terms of the Creative Commons Attribution License (CC BY). The use, distribution or reproduction in other forums is permitted, provided the original author(s) and the copyright owner(s) are credited and that the original publication in this journal is cited, in accordance with accepted academic practice. No use, distribution or reproduction is permitted which does not comply with these terms.
*Correspondence: Xiaping He, aHhwQGRhbGkuZWR1LmNu