- 1Institute of Human Genetics, University Medical Center, Johannes Gutenberg University, Mainz, Germany
- 2Leibniz Institute for Resilience Research, Leibniz Association, Mainz, Germany
- 3Fraunhofer Institute for Industrial Mathematics (ITWM), Kaiserslautern, Germany
The common features of all neurodegenerative diseases, including Alzheimer's disease, Parkinson's disease, Amyotrophic Lateral Sclerosis (ALS), and Huntington's disease, are the accumulation of aggregated and misfolded proteins and the progressive loss of neurons, leading to cognitive decline and locomotive dysfunction. Still, they differ in their ultimate manifestation, the affected brain region, and the kind of proteinopathy. In the last decades, a vast number of processes have been described as associated with neurodegenerative diseases, making it increasingly harder to keep an overview of the big picture forming from all those data. In this meta-study, we analyzed genomic, transcriptomic, proteomic, and epigenomic data of the aforementioned diseases using the data of 234 studies in a network-based approach to study significant general coherences but also specific processes in individual diseases or omics levels. In the analysis part, we focus on only some of the emerging findings, but trust that the meta-study provided here will be a valuable resource for various other researchers focusing on specific processes or genes contributing to the development of neurodegeneration.
Introduction
The typical features of all neurodegenerative diseases (NDDs), including Alzheimer's disease (AD), Parkinson's disease (PD), Amyotrophic Lateral Sclerosis (ALS), and Huntington's disease (HD), are the accumulation of aggregated and misfolded proteins and the progressive loss of neurons, leading to cognitive decline and locomotive dysfunction. Still, they differ in their ultimate manifestation (e.g., dementia, Parkinsonism, motor neuron impairment), the affected brain region [e.g., hippocampus (AD), Substantia nigra (PD), striatum (HD), upper and lower motor neurons (ALS)], and the kind of proteinopathy [amyloidosis, tauopathies (AD)], a-synucleinopathy (PD), CAG triplet elongation (HD) and TAR DNA-binding protein 43 (TDP-43) aggregates (ALS) (Roos, 2010; Zarei et al., 2015; Rosenberg et al., 2016; Armstrong and Okun, 2020; Pathak et al., 2021).
Adult neurons maintain energy-intense functions, such as membrane excitability, neurotransmission, and plasticity. They cannot rejuvenate by dividing, so they are especially reliant on a high supply of energy, the maintenance of protein and organelle quality control, rapid delivery of molecules within and out of cells, and the trafficking of organelles and other factors over considerable distances within the cell (Franco-Iborra et al., 2018; Gan et al., 2018). There are myriads of biological processes involved in the well functioning of the brain, whose malfunction can lead to neurodegeneration. Hallmarks among others are an aberrant cell cycle regulation, mitochondrial dysfunction, ER stress, impaired protein folding and quality control, deregulated autophagy and apoptosis, oxidative stress and insufficient DNA damage repair, missing homeostasis, a malfunctioning extracellular matrix, excitotoxicity, aberrant axonal transport, and excessive neuroinflammation (Coppedè and Migliore, 2015; Lindberg et al., 2015; Nah et al., 2015; Briston and Hicks, 2018; Cabral-Miranda and Hetz, 2018; Sonbol, 2018; Guzman-Martinez et al., 2019; Armada-Moreira et al., 2020; Guo et al., 2020; Joseph et al., 2020; Iatrou et al., 2021). To disentangle the multitude of processes associated with neurodegenerative diseases, we collected data from four omics-layers in four neurodegenerative diseases from 234 studies. These data are analyzed in a network-based approach that provides structure regarding the most represented processes, hub genes, and differences in regulation between specific omics-layers and diseases by building communities within networks for each omics-layer in each condition.
To provide an overview of the central biological processes and the pathways involved, we have divided them into broader categories according to their primary location or mode of action, namely, intracellular mechanisms, local tissue environment influences, and systemic influences (Ramanan and Saykin, 2013). On the cellular level, a deregulated cell cycle and misguided autophagy and apoptosis lead to degenerated neurons. Within the local tissue environment, an intact extracellular matrix (ECM), an unimpaired cell development, signal transduction, and transport of vital molecules are of fundamental importance. Excessive immune response and a dysregulated metabolism lead to significant disturbances on the systemic level.
Within our analyses, we found thousands of biological processes (BP) as defined by the Gene Ontology and local network cluster terms given by the string DB database. To allow an understanding within this complexity, we assigned those multilayered and diverse terms to 6 hallmarks: “cell cycle,” “autophagy/apoptosis,” “ECM/development,” “signal transduction/transport,” “immune system,” and “metabolism” for each community in all created networks. This observation allows us to extract processes distributed across diseases and omics-layers, which are mainly present in specific omics-layers or conditions. We only address the most striking results but see this work as an opportunity for other researchers working primarily on the effect of particular processor genes on neurodegeneration to study the gene communities associated with those processes in more detail. For example, a specific gene cluster containing the gene or process of interest can be extracted from the Supplementary Data sources we provide (see Supplementary Table 1—Genes Per Community) and inserted, e.g., in string DB (https://string-db.org/). The resulting network can be selectively examined, and all publications, processes, pathways, etc., that are significantly associated with the respective candidates or networks can be analyzed. Consequently, we hope the big picture of neurodegeneration we draw here in our results will serve as an inspiring and rich source of data, providing a range of new insights and analysis perspectives for the scientific community. In the following, we introduce the six hallmarks, providing a preview of our associated low-level terms by showing them in italics.
Hallmark 1: Cell Cycle
Neuronal cells similar to muscle cells usually remain in a quiescent cell cycle state once they are differentiated due to their specialized functions. But cell cycle regulatory proteins like cyclins, CDKs, caspases, and p53 continue to be required for axonal migration, maturation, and survival (López-Sánchez et al., 2017; Joseph et al., 2020). One or more of these cell cycle proteins and pathways might get activated in response to various epigenetic or pathologic stimuli. Aberrant cell cycle reentry of neurons with duplication of the genetic material but without subsequent cell division is a well-known phenomenon. However, it is still unknown whether this is deleterious or beneficial. In the early stages of brain development, specific populations of neurons undergo incomplete mitosis and stay in a tetraploid state for the rest of their adult life. Still, a de novo neuronal tetraploidization in the adult brain seems to be an early indicator for neurodegeneration, with cells showing elevated molecular stress response and apoptotic marker proteins due to this aberrant regulation of cell cycle (Frade and Ovejero-Benito, 2015; López-Sánchez et al., 2017; Joseph et al., 2020). The correct assembly, positioning, and working of the mitotic spindle apparatus are essential for the development and differentiation of cells. Spindle orientation defects leading to an imbalance between symmetric and asymmetric divisions have been discussed as being linked to NDD. An asymmetric cell division leads to two daughter cells with different cell fates. An important example is the division of a stem cell, leading to a differentiated brain cell and another stem cell. The preconditions for an asymmetric cell division are the polarization of the content of the mother cell and the alignment of the mitotic spindle along the axis of polarity. If this process is disturbed by an impaired spindle orientation, this will have severe consequences in the development of the brain (Noatynska et al., 2012). During mitosis, motor proteins, together with Huntingtin, localize along spindle microtubules to segregate the chromosomes (Godin and Humbert, 2011). Thus, mutant Huntingtin could lead to defective neurogenesis during embryonic development leading to Huntington's disease later in life (Godin and Humbert, 2011; Wiatr et al., 2018). Defective DNA repair mechanism, together with elevated oxidative stress, is a typical hallmark for aging cells (Joseph et al., 2020). As the brain is the largest consumer of oxygen, reactive oxygen species (ROS) are the main inducers of DNA damage in neurons (Nissanka and Moraes, 2018). The accumulation of unrepaired damaged DNA might contribute to a cell cycle reentry of the neuron to increase polyploidy, thus compensating for the loss of information on some parts of the DNA. Also, general terms like transcription (mitochondrial) translation, RNA modification, spliceosome, and chromatin organization were defined as subprocesses integrated into the hallmark called the cell cycle.
Obviously, transcription, translation, and chromatin remodeling as major contributors to protein homeostasis have a considerable impact on all cellular functions, and genetically or epigenetically misregulated transcription factors can enhance or weaken all the processes that lead to or prevent neurodegeneration like neuroinflammation, oxidative stress, and protein homeostasis (Berson et al., 2018; Jin et al., 2019). Especially neurons face the problem that their locus of transcription is distant from the locus of translation as protein synthesis is decentralized to meet the rapidly changing metabolic needs of axons and dendrites. Nuclear mRNA is transported from the cell body to the periphery as RNA/protein granules linked to motor proteins of the cytoskeleton by adaptor complexes. Conditions, such as mislocalization of the mRNA, a defective cytoskeleton, and deleterious adaptor complexes, lead to synaptic dysfunction, loss of synapses, and, ultimately, to neuronal death (Liu et al., 2017).
Hallmark 2: Autophagy and Apoptosis
Accurate folding and control over levels of many proteins are tightly regulated in neuronal cells by a protein quality control network consisting of molecular chaperones and an intact degradation system of misfolded or discarded proteins, consisting of the ubiquitin-proteasome system and autophagic processes. Chaperone-mediated processes are involved in the promotion of de novo folding of nascent proteins or the refolding of misfolded polypeptide chains, thereby preventing their aggregation (Hartl et al., 2011; Chaplot et al., 2020).
Proteins that cannot be folded correctly or are no longer needed are tagged by the attachment of the small protein ubiquitin (ubiquitinylation) and degraded by a multi-subunit protein complex called the proteasome or by autophagy, which is a universal lysosome-dependent intracellular degradation process for not only protein aggregates but also cell organelles and cytoplasmic constituents to maintain cell homeostasis (Nah et al., 2015; Schmidt et al., 2021). Lysosome-dependent autophagy can either be carried out by lysosomes acting by themselves in a process called micro-autophagy, or in macro-autophagy where cell organelles with a lipid double layer derived from the ER called omegasomes fuse with lysosomes to form autophagosomes (Mizushima and Klionsky, 2007; Li et al., 2012; Nah et al., 2015). In neurons, these autophagosomes are generated constitutively in the cell periphery and mature on their way to the cell body (Xie and Klionsky, 2007). The proteins ensuring this axonal transport are Huntingtin and Huntingtin-associated protein 1. As huntingtin is predominantly cleared by autophagy, the deficiency of intact huntingtin in HD is a double stress factor leading to the accumulation of polyglutamine-expanded huntingtin polyQ-htt and other cytoplasmic constituents that need to be degraded (Wong and Holzbaur, 2014). Besides deficiencies in the protein quality control network leading to the typical protein aggregations in NDD, there are many more factors that impair homeostasis of the cell, causing stress to the cells. Heat, toxins, mechanical damage, infections, starvation, hypoxia, and oxidants all lead to cellular stress responses that aim to control the induced damage and, if possible, to confer resilience to the stressor. The integrated stress response (ISR) is an elaborate signaling cascade, where different stress signals activate different protein kinases that converge in phosphorylating the core of the ISR, the α-subunit of the translation initiation factor 2 (eIF2α). This leads to a reduction of global protein synthesis in favor of ISR-specific mRNAs, such as the activating transcription factor ATF4. ATF4 is the main effector of the ISR. It forms homo- and heterodimers that bind to DNA targets to control the expression of genes involved in cellular adaptation, mainly chaperones that refold denaturated proteins or tag them for immediate degradation (Harding et al., 2003; Fulda et al., 2010; Pakos-Zebrucka et al., 2016). To terminate the integrated stress response, dephosphorylation of eIF2α is required. However, dephosphorylation of eIF2α can also facilitate the production of death-inducing proteins in cases where the cell is so severely damaged that normal functioning and homeostasis cannot be restored (Pakos-Zebrucka et al., 2016). Highly regulated cascades of events will then lead to the decomposition of the cell in a process called apoptosis (Danial and Korsmeyer, 2004; Elmore, 2007; Fulda et al., 2010). Apoptosis is a caspase-mediated programmed cell death that can be triggered either by stress signals from within the cell or by signals that are released by the surrounding cells and that bind to cell-surface death-receptors (Danial and Korsmeyer, 2004; Elmore, 2007). Both intrinsic and extrinsic pathways ultimately induce cell death by activating caspases, enzymes that carry out the degradation of the cell, leading to cell shrinkage, plasma membrane blebbing, chromosome condensation and degradation, mitochondrial death with release of cytochrome C, cellular fragmentation, and formation of membranous apoptotic bodies. At the same time, phosphatidylserine residues are exposed at the cell surface to attract macrophages that phagocytize the cell fragments. The extrinsic pathway is activated by extracellular ligands (e.g., CD95 ligand, tumor necrosis factor α) binding to cell-surface death receptors, which either leads to the formation of the so-called DISC (death-inducing signaling complex) or the activation of nuclear factor kappa B (NFκB) (Wang and El-Deiry, 2003; Lavrik et al., 2005). Caspase 8, which serves as the initiator caspase, is activated within this complex. If the activating stimulus is sufficiently large, caspase 8 begins activating the effector Caspases 3, 6, and 7. They translocate to the mitochondria to trigger pore formation in the outer mitochondrial membrane (Fan et al., 2005), which ultimately leads to mitochondrial permeability and death. The release of cytochrome C promotes caspase-9 activation, which triggers a cascade of proteolytic events. The intrinsic signaling pathway is controlled by the Bcl2-family of proteins and is triggered by cell-internal factors, such as DNA damage, osmotic stress, or growth factor withdrawal. The triggering cell event activates the transcription factor p53, leading to the expression of proapoptotic Bad protein. The Bad protein, in turn, activates the multidomain proapoptotic Bax protein, which triggers permeabilization of the mitochondrial outer membrane. As a result, similar to the extrinsic pathway, cytochrome C escapes from the intermembrane space of the mitochondria and induces a proteolytic cascade (Mcilwain et al., 2013; Wang and Tjandra, 2013; Chen et al., 2018).
The NFkB signaling pathway is activated during apoptosis either to enforce the ongoing apoptotic processes or to navigate the cell back to the survival pathway by stimulating the expression of anti-apoptotic genes, especially TRAF1 and TRAF2, and thus overruling the activities of the caspases (Sheikh and Huang, 2003). NFκB is a family of transcription factors with multiple physiological and pathological functions. The NFκB complex exists in an inactive state in the cytoplasm. It consists of different NFkB dimers and inhibitory kB proteins (IκB) that prevent NFkB to enter the nucleus and bind to the DNA (Israël, 2010). NFkB can be activated by highly variable stimuli, including growth factors, cytokines like tumor necrosis factor alpha and interleukin 1-ß, bacterial and viral antigens like lipopolysaccharides or double-stranded RNA and physicochemical insults like ionizing radiation or free radicals. All these different stimuli activate different phosphorylation cascades that, in the end, lead to an interaction with the IκB kinase (IKK) complex, which then leads to the phosphorylation of IκB in the NFkB complex. Once phosphorylated, IκB gets ubiquitinylated and degraded by the proteasome. The released NFkB can now enter the nucleus to activate target gene expression that regulates immune responses, cell growth, and proliferation but also apoptosis (Qin et al., 2007). The selectivity of the NF-κB response is based on several factors, including dimer composition, timing, organization of chromatin, and cell type (Natoli, 2009; Sen and Smale, 2010). Besides the NFkB signaling pathway, an important low-level term in autophagy and apoptosis, was the mTOR signaling pathway. As mentioned above, an impaired autophagy in NDD leads to the accumulation of toxic protein aggregates that promote cellular stress and death. The kinase mammalian target of rapamycin (mTOR) is a central regulator of metabolism as it senses cellular nutrient, oxygen, and energy levels (Perluigi et al., 2015). It is a potent repressor of autophagy and reacts to food abundancy with cell growth and proliferation, whereas, in times of starvation, it is inhibited, thus allowing the cells to derive energy from autophagic and apoptotic processes (Heras-Sandoval et al., 2020).
Hallmark 3: Extracellular Matrix Organization and Development
The extracellular matrix (ECM) is a three-dimensional network that reaches all cells of the body to provide structural support, segregate tissues, and regulate intercellular communication by many different biochemical processes. It consists of minerals and macromolecules like collagen, elastin, enzymes, glycoproteins, and hyaluronan, which is most abundant in the brain (Bonnans et al., 2014). These compounds contribute to a varying degree of stiffness and elasticity of the ECM throughout the body from hard bone to soft brain tissues. The mechanical properties of the ECM environment can be sensed by the cells, which is the precondition for processes like cell migration, proliferation, differentiation and growth, apoptosis, and, on a higher level, the development of tissues and organs (Bonneh-Barkay and Wiley, 2009; Sethi and Zaia, 2017; Maguire, 2018). These processes also require a direct connection between cells and ECM. The ECM communicates with the cells by connecting to the cytoskeleton through cilia and (focal) adhesion complexes (Geiger and Bershadsky, 2002). The cytoskeleton is a functionally versatile filamental structure that transports and localizes the contents of the cell, it organizes the process of cell division, namely, chromosome segregation and cytokinesis, it connects the cell physically and biochemically to the external environment through interacting with the ECM (cell adhesion, focal adhesion, cell junction), and it enables the cell to move and change shape (cell migration, cell differentiation, growth, and localization) (Wozniak et al., 2004; Fletcher and Mullins, 2010). These functions are mediated by three filament types: microtubules, actin microfilaments, and intermediate filaments, which, in neurons, are called neurofilaments (Herrmann et al., 2007; Hohmann and Dehghani, 2019). Microtubules are hollow tubes consisting of tubulin dimers, which are stabilized by tau proteins. They grow out from the centrosome to the plasma membrane by constantly adding and subtracting tubulin dimers at both ends of the filament (Mitchison and Kirschner, 1984). They are responsible for the intracellular transport together with the motor proteins kinesin and dynein that transport, e.g., vesicles, nutrients, and essential organelles, but also broken-down neurotransmitters and misfolded proteins by extending along the axon and functioning as the cytoskeletal navigation pathways in the transporting process (Guo et al., 2020). The hyperphosphorylation of tau proteins as seen in AD results in microtubule destabilization and cytoskeleton abnormalities (Mandelkow et al., 1995; Barbier et al., 2019). Actin ß- and γ- monomers, together with formin homology proteins, form linear polymers called actin microfilaments, which can then be arranged as a network with the help of the proteins Arp2 and Arp3. The Arp2/3-complex forms dimers that resemble actin dimers, but are much more stable. They attach themselves in a 70° angle and serve as nucleation cores for actin filaments activated by the nucleation-promoting factor proteins of the WASP/WAVE-family (Kurisu and Takenawa, 2009). Both filaments and networks stabilize the plasma membrane of the cells and control the position of the nucleus and other cell organelles (Pfisterer et al., 2017). They maintain and adapt cell shape, thus allowing for cytokinesis, organization of cell junctions, and enhancement of cell adhesion. Cell migration is achieved by the polymerization of new actin filaments in the forward edge of a moving cell that pushes the cell membrane forward in protrusions called lamellipodia. These protrusions form mechanical links between the actin filaments and the ECM called focal adhesions. Once attached, the rear of the cell body contracts, squeezing its contents forward past the adhesion point. Once the adhesion point has moved to the rear of the cell, the cell disassembles it, allowing the rear of the cell to move forward (Ananthakrishnan and Ehrlicher, 2007). The actin filaments also provide the tracks for cellular transport, allowing actin-based motor proteins (myosins) to transport organelles over short distances.
The interactions of a-actin und myosin but also troponin and tropomyosin are most sophisticated in muscle cells. These highly specialized cells work together to contract and release muscles, thus leading to movement of the whole organism and to the cardiac muscle action potential (Cooper, 2000). Intermediate filaments are the major cytoskeletal proteins, which, in contrast to microtubules and microfilaments, are more heterogeneous, have tensile strength, and do not participate in cell motility (Herrmann et al., 2009). The intermediate filaments primarily provide structural support for the cell, especially for the long neuronal cells where they regulate the diameter of the axon and, thereby, the nerve conduction speed (Helfand et al., 2003).
All cells have intermediate filaments, but the protein subunits of these structures vary. They can be classified into six different types: Types I and II are the keratins, which are expressed in epithelia. Type III contains the proteins vimentin, which frequently colocalizes with microtubules, desmin, peripherin, and glial fibrillary acidic protein (GFAP). Type IV consists of the neurofilament proteins L, M, H, and internexin. Type V consists of the nuclear lamins, and Type VI consists of the protein nestin (Muñoz-Lasso et al., 2020). During embryonic development of the brain microtubules, microfilaments and neurofilaments work together to guarantee proper growth and guidance of axons; during adult life, they maintain neuronal homeostasis and plasticity. Given these fundamental processes rely on an intact cytoskeleton organization, it is not surprising that cytoskeleton defects, including alterations in microtubule stability, in axonal transport as well as in actin dynamics, have been characterized in several NDDs.
Hallmark 4: Signal Transduction and Transport
Neuronal signal transduction and transport of organelles and vital molecules along the axon maintain neuronal activity and health. In NDD, neuronal signaling is more and more impaired because of synaptic and cytoskeletal dysfunctions. The loss of synapses has detrimental consequences as they are critical to learning, memory, and behavior (Lepeta et al., 2016; Ardiles et al., 2017), and perturbations in axonal transport can lead to neuronal cell death (Perlson et al., 2010).
Cell communication in the brain is achieved by neuronal signaling via synapses. In this process, electrical activity is transferred from one neuron to another through neurotransmitters as chemical mediators. The most important neurotransmitters in the central nervous system are glutamate for the excitatory, and GABA and glycine for the inhibitory synapses, but also dopamine, serotonin, and acetylcholine (Fogarty et al., 2016). Action potentials that travel along axons induce an intracellular Ca2+ influx mediated by voltage-gated calcium channels at the presynaptic terminal. Increased Ca2+ levels are sensed by the synaptic vesicle protein synaptotagmin I, which induces the Soluble NSF Attachment Protein Receptor (SNARE) protein complex to mediate fusion of the neurotransmitter vesicles with the plasma membrane (SNARE binding), releasing them into the synaptic cleft (neurotransmitter secretion). The neurotransmitters then bind to and thus activate receptors of the postsynaptic cell, which can be either ion channels or G protein-coupled receptors. They transmit signals from the post-synaptic membrane to the cell body by transducing the chemical signal into an electrical signal that depolarizes the postsynaptic cell and is transmitted downstream to the next neuron (Lepeta et al., 2016; Taoufik et al., 2018).
Rather than being just a gap, the synaptic cleft is full of trans-synaptic adhesion molecules that not only physically connect the pre- and post-synaptic compartment but also mediate recognition and signaling processes that are essential for the establishment, specification, and plasticity of synapses. Such synapse-organizing adhesion molecules include neurexins and neuroligins, cadherins, integrins, receptor phosphotyrosine kinases, and phosphatases, such as ephrins and Rho GTPases (Missler et al., 2012; Jang et al., 2017). Deficiencies in the complex synaptic functioning are not only seen in neurodegenerative diseases but also in neuropsychiatric disorders, suggesting that the loss of synapses is not the endpoint incident of the disease but, rather, a triggering event (Taoufik et al., 2018).
Axonal transport, in general, is a process where the motor proteins kinesin and dynein loaded with diverse cargos navigate along the microtubules of the cytoskeleton. Anterograde axonal transport delivers proteins, lipids, mRNA, and mitochondria to the distal synapse, whereas retrograde transport clears misfolded or aggregated proteins and brings distal trophic signals to the soma (Millecamps and Julien, 2013; Sleigh et al., 2019).
Transmembranal transport systems in neurons are the anterograde ER to Golgi transport with COP II-coated vesicles and the retrograde trans-Golgi-Network transport with COP I-coated vesicles (Martínez-Menárguez et al., 2019; Wang L. et al., 2020).
Neurotransmitters or other secretory molecules leave the ER packed in COPII-coated vesicles to be further processed in the Golgi apparatus before being secreted into the synaptic cleft. After an action potential, neurotransmitter and receptors are recycled by the cell. They are protected from lysosomal degradation by COPI-coated vesicles of the retrograde trafficking and redirected to the ER where they are recycled for the next release (Lu and Hong, 2014).
Deficiencies in axonal transport through defects in the cytoskeletal organization, the impairment of motor protein attachment to the microtubules or the destabilization of motor-cargo binding but also aberrant transmembranal anterograde and retrograde transport between ER and Golgi apparatus can induce synaptic failure and ultimately lead to neurodegeneration, as shown in AD, PD, ALS, and HD (Perlson et al., 2010; Guo et al., 2020).
Hallmark 5: Immune System
A fundamental feature in NDD is the dysregulation of the innate immune response in the central nervous system by chronic inflammation. The innate immune response of the brain represents not only the first line of defense against invading microorganisms (defense response to other organisms) but also responds to endogenous stress like dying or damaged cells after cellular stress. It consists primarily of microglial cells and astrocytes. Microglia are resident tissue macrophages and the principal mediators of inflammation (Frank-Cannon et al., 2009), whereas astrocytes mainly supply neurons with nutrients and energy and form the blood–brain barrier (Blackburn et al., 2009). Both microglia and astrocytes express on their cell-surface pattern-recognition receptors that detect pathogen-associated molecular patterns like residues from bacteria, viruses, fungi but also danger-associated molecular patterns, which can be abnormal endogenous proteins, iron overload, antibodies, cytokines, and chemokines, including toll-like receptors (Akira et al., 2006; Medzhitov, 2007). Also, complement factors bind to these receptors, initiating the complement and coagulation cascade, which enhances the immune response of microglia and astrocytes.
Pathogen- and danger-associated molecular patterns induce neuroinflammation by activating the transcription factor NFkB in microglial cells. NFkB induces the synthesis of proinflammatory cytokines like Interleukin−1ß (IL-1β), IL-6, IL-12, interferon gamma (IFN-γ), chemokines, including the C-C motif chemokine ligand 2 and tumor necrosis factor alpha (TNF-α), but also prostaglandins (Schaefer, 2014; Guzman-Martinez et al., 2019) to promote efficient clearance of cell debris and plaques. Also, these factors, being released into the cell environment, activate the astrocytes to both increase the permeability of the blood–brain barrier for easier recruitment of B- and T-adaptive immune cells (leukocyte activation) in the brain parenchyma and to release intracellular signals like neurotrophic and growth factors and cytokines, promoting neuronal survival, neurite growth, and neurogenesis (Jha et al., 2019). At the same time, microglial cells synthesize and release short-lived cytotoxic factors, such as superoxide radicals, nitric oxide, and reactive oxygen species that help remove pathological agents (Harry and Kraft, 2008; Jurga et al., 2020).
Although an acute insult may trigger oxidative and nitrosative stress and a slightly more permeable blood-brain barrier, this reaction is typically short-lived and unlikely to be detrimental to long-term neuronal survival (Kurutas, 2015). But disease-induced protein aggregations like oligomers of tau and Aβ, aging, and other risk factors can lead to chronic neuroinflammation with a long-standing and, often, self-perpetuating neuroinflammatory response that persists long after the initial injury or insult. Permanently activated microglia may not be able to remove amyloid-beta deposits, which, in turn, contributes to further plaque accumulation as opposed to clearance (Frank-Cannon et al., 2009). Also, the permanently increased oxidative and nitrosative stress leads to damaged neurons, synaptic dysfunction, loss of synapses, and neuronal death (Schain and Kreisl, 2017; Shabab and Zorofchian, 2017; Kinney et al., 2018), aggravated by the fact that astrocytes lose their neurotrophic function during the progressive loss of neurons. This process called astrogliosis affects considerably the neurons and their integrity (Sofroniew and Vinters, 2010; Sofroniew, 2015). Chronic inflammation can also trigger signaling pathways that activate brain tau hyperphosphorylation in residues that are not modified under normal physiological conditions, again leading to degenerated neurons and, finally, the now permeable blood-brain barrier allows much more pathogens to enter the brain than the innate immune response can cope with (Gendelman, 2002).
Hallmark 6: Metabolic Processes
We associated the highest number of low-level terms across all six hallmarks to the metabolism hallmark as its pathways and biological processes are involved in all basic physiological processes, and the homeostasis of the compounds and their interactions is vital for the well-functioning of the organism. As neurons but also astrocytes, oligodendrocytes, and microglia are highly dependent on a continuous energy supply. One of the most significant hallmarks for NDD is the dysfunction of mitochondria. Mitochondrial metabolism, comprising the oxidation of pyruvate and fatty acids, the citric acid cycle, the oxidative phosphorylation within the respiratory chain, and the formation of antioxidants, was assigned to the hallmark metabolism, which also contains metabolic processes in the cytoplasm like fat metabolism, protein, carbohydrate, and nucleic acid metabolism, but also homeostasis of the cell. Lipid molecules are key components of the brain's complex structure and function, with lipids comprising around 50% of the brain's dry weight (O'brien et al., 1964). Many neurological diseases are caused by mutations in genes that are involved in lipid metabolism (Mesa-Herrera et al., 2019; Petit et al., 2020). As associated low-level terms, we included lipid-, cholesterol-, sphingolipid metabolism, lipoproteins, carboxylic acid, and fatty acid oxidation. Lipids function as key regulators of neurotransmission; cholesterol is a membrane stabilizer and organizer during synaptic vesicle exo- and endocytosis; sphingolipids and their metabolites are regulators of the fluidity of cell membranes and second messengers in signal transduction (Puchkov and Haucke, 2013; Barber and Raben, 2019). Being hydrophobic, lipids and cholesterol must be transported through the blood by lipoproteins. The term carboxylic acid was mostly connected to biological processes concerning the citric acid cycle and was thus assigned to mitochondrial metabolism, like fatty acids, which are degraded in the mitochondria in an energy-generating process called ß-oxidation (Petit et al., 2020).
Main low-level terms of protein metabolism were branched amino acid degradation and proteoglycans. Valine, isoleucine, and leucine are essential branched-chain amino acids (BCAAs). Their catabolism starts in muscle mitochondria and yields NADH and FADH2, which can be utilized for ATP generation. In the brain, BCAAs contribute to the synthesis of excitatory glutamate and inhibitory gamma-aminobutyric acid (GABA) and, at the same time, can reduce the production of serotonin and the catecholamines because they compete for transport across the blood-brain barrier with the aromatic amino acids tryptophan, tyrosine, and phenylalanine, which are the precursors of these neurotransmitters (Fernstrom, 2005). AD metabolomics studies demonstrate that altered branched-chain amino acids metabolism accompanies Alzheimer's disease development. Lower plasma valine levels are correlated with accelerated cognitive decline in AD and the severity of motor dysfunction in HD (Polis and Samson, 2020; Xu et al., 2020). No clear correlation between the levels of BCAA and ALS or PD could be found (Bjornevik et al., 2019; Xu et al., 2020).
Carbohydrates are the main source for energy production in the brain and are broken down by glycolysis, the tricarboxylic acid cycle, and oxidative phosphorylation (Attwell and Laughlin, 2001). The main causes for defective glucose usage in NDD are deleterious mitochondria and a decreased secretion of insulin, coupled with resistance to its actions, problems that link NDD to type II diabetes (Ristow, 2004; Haan, 2006; Seneff et al., 2011; Schaeffer et al., 2021).
During nutritional uptake, the insulin pathway secures the release of the hormone insulin into the blood, which then binds to insulin receptors on the cell surface and thus regulates the uptake of glucose into the cells. But insulin also controls divergent signaling pathways like the Act-pathway or the MAP-kinase pathway, which are involved in cell growth and differentiation (Saltiel and Pessin, 2002). Studies with mouse models have shown that insulin is also involved in the excitability of (hippocampal) neurons (Dai et al., 2014). AD neurons show a reduced action potential due to soluble Aß, an effect that is aggravated by missing insulin and that can be partially reversed by the anti-cancer agent bexarotene (Cramer et al., 2012; Dai et al., 2014).
Another important task of carbohydrates is the glycosylation of proteins and lipids in the ER. In the brain, glycosylated proteins participate in a myriad of processes, from electrical gradients to neurotransmission (Conroy et al., 2021), and glycolipids are crucial for the interaction of cells and for signal transduction (Reily et al., 2019). Thus, an intact N-glycosylation and O-glycosylation of lipids and proteins are important for the well functioning of the brain, and disrupted glycosylation can lead to NDD (Moll et al., 2020), e.g., O-GlcNAcylation of CNS proteins important for axonal and synaptic function is significantly reduced in AD, ALS, HD, and PD patients' tissue (Liu et al., 2004; Lüdemann et al., 2005; Kumar et al., 2014).
Nucleotides are not only the building blocks of DNA and RNA but also are involved in cellular metabolism, with, especially, ATP and NADH as energy carriers and cAMP, a second messenger molecule, as a translator of signals from outside to cellular processes. Thus, these molecules are involved in all basic physiological processes critical to the proper function of the organism (Huang et al., 2021). Nucleotides are either built de novo or, as this process takes much energy, recovered during the degradation of DNA and RNA in a nucleotide salvage pathway. A disbalance in the synthesis pathways again disturbs energy homeostasis and many cellular processes like proliferation, differentiation, migration, and apoptosis. The respiratory chain complex in the mitochondria not only efficiently produces energy but also reactive oxygen species that can harm the mitochondrial DNA. Antioxidants like glutathione protect mitochondria and cells against damage, but, if this homeostasis is disturbed, oxidative stress induces the destruction of mitochondria and apoptosis of neuronal and other brain cells (Kausar et al., 2018).
Materials and Methods
To make all steps from data acquisition via data management to data analysis comprehensible, we will outline these steps following that order. The data analysis is composed of several steps, the analysis of the intersections of the individual omics-layers per NDD, the analysis of the conformity of the mean regulatory direction across omics-layers per NDD, as well as the core of the data analysis: the generation and evaluation of the protein-protein interaction networks, and the generation and evaluation of the communities.
Data Acquisition
In this meta-study, we used datasets of four different omics-layers: Genomics (SNP data), Transcriptomics, Proteomics, and Methylomics. In the following, we describe where these data were acquired from.
The SNP data are based on the genome-wide association studies (GWAS) Catalog (Buniello et al., 2019), which has been updated on November 18, 2021. The experimental factor ontology (EFO) numbers for the exact search pattern were EFO_0000249 (Alzheimer's disease), EFO_0002508 (Parkinson's disease), Orphanet_399 (Huntington's disease), and EFO_0000253 (Amyotrophic Lateral Sclerosis). For all NDD but HD, a child term was given, which was also included in the data acquisition step. In total, 153 studies with genomic data were used for the analyses (Maraganore et al., 2005; Fung et al., 2006; Coon et al., 2007; Reiman et al., 2007; Schymick et al., 2007; van Es et al., 2007; Van Es et al., 2008, 2009; Abraham et al., 2008; Bertram et al., 2008; Cronin et al., 2008, 2009; Li et al., 2008, 2021; Webster et al., 2008; Beecham et al., 2009, 2015; Carrasquillo et al., 2009; Harold et al., 2009; Lambert et al., 2009, 2013a,b; Landers et al., 2009; Latourelle et al., 2009; Pankratz et al., 2009, 2012; Satake et al., 2009; Simón-Sánchez et al., 2009; Edwards et al., 2010; Feulner et al., 2010; Hamza et al., 2010; Heinzen et al., 2010; Laaksovirta et al., 2010; Naj et al., 2010, 2011; Seshadri et al., 2010; Shatunov et al., 2010; Stein et al., 2010; Antúnez et al., 2011; Do et al., 2011; Furney et al., 2011; Hollingworth et al., 2011, 2012; Hu et al., 2011, 2016; Kim et al., 2011; Kramer et al., 2011; Liu et al., 2011, 2021; Logue et al., 2011; Nalls et al., 2011, 2014, 2019; Saad et al., 2011; Spencer et al., 2011; Wijsman et al., 2011; Chung et al., 2012, 2018; Cummings et al., 2012; Kamboh et al., 2012a,b; Kwee et al., 2012; Lill et al., 2012; Meda et al., 2012; Cruchaga et al., 2013; Davis et al., 2013; Deng et al., 2013; Jonsson et al., 2013; Langefeld, 2013; Martinelli-Boneschi et al., 2013; Miyashita et al., 2013; Reitz et al., 2013; Diekstra et al., 2014; Fogh et al., 2014, 2016; Goris et al., 2014; Hill-Burns et al., 2014, 2016; Kauwe et al., 2014; Nelson et al., 2014; Pérez-Palma et al., 2014; Ramanan et al., 2014; Ramirez et al., 2014; Sherva et al., 2014, 2020; Vacic et al., 2014; Xie et al., 2014; Hirano et al., 2015; McLaughlin et al., 2015; Tosto et al., 2015; Wang et al., 2015, 2021; Biernacka et al., 2016; Chen et al., 2016; Deming et al., 2016; Herold et al., 2016; Jun et al., 2016, 2017; Pickrell et al., 2016; Schott et al., 2016; Traylor et al., 2016; Van Rheenen et al., 2016; Watanabe et al., 2016; Benyamin et al., 2017; Chang et al., 2017; Foo et al., 2017, 2020; Lee et al., 2017; Mez et al., 2017; Moss et al., 2017; Siitonen et al., 2017; Sims et al., 2017; Chao et al., 2018; Gusareva et al., 2018; Marioni et al., 2018; Miron et al., 2018; Mukherjee et al., 2018; Nicolas et al., 2018; Pottier et al., 2018; Wallen et al., 2018; Yashin et al., 2018; Bandres-Ciga et al., 2019; Blauwendraat et al., 2019, 2020; Dekker et al., 2019; Jansen et al., 2019; Kunkle et al., 2019, 2021; Lo et al., 2019; Moreno-Grau et al., 2019; Nazarian et al., 2019a,b; Wei et al., 2019; Zhu et al., 2019; Cha et al., 2020; Hong et al., 2020; Nakamura et al., 2020; Ryu et al., 2020; Alfradique-Dunham et al., 2021; Bone et al., 2021; DeMichele-Sweet et al., 2021; de Rojas et al., 2021; Kang et al., 2021; Loesch et al., 2021; Park et al., 2021; Reddy et al., 2021; Rodrigo and Nyholt, 2021; Sakaue et al., 2021; Schwartzentruber et al., 2021; Shigemizu et al., 2021; Smeland et al., 2021; Tan et al., 2021; Wightman et al., 2021).
For the transcriptomic and proteomic layer, we used the studies that we have identified previously (Ruffini et al., 2020), using the Gene Expression Omnibus (GEO) (Barrett et al., 2013), the Expression Atlas (Papatheodorou et al., 2018) databases, and doing literature research (Ruffini et al., 2020). For the transcriptomic data, the keywords used in the GEO database were <name of disease> AND (“microarray” OR “RNAseq”) AND “human,” The Expression Atlas was used in Release 35 (May 2020, https://www.ebi.ac.uk/gxa/home) and scanned for Alzheimer's, Parkinson's, Huntington, and Amyotrophic Lateral Sclerosis, using the filter “Homo sapiens” in the section “Differential Experiments.”
For the proteomic data, literature search was conducted in PubMed and Google Scholar, with the keywords: (“neurodegenerative diseases” OR “Alzheimer's* disease” OR “Parkinson's* disease” OR “Huntington* disease” OR “Amyotrophic Lateral Sclerosis”) AND (proteomics OR “quantitative proteomics” OR “differentially expressed proteins” OR biomarkers) AND human NOT mice for publications from 2010 to 2020.
Of the included experiments, 63% of the transcriptomic experiments were performed with brain material, while 67% of the proteomic experiments were conducted with brain material. Among the non-control patients, 84% were classified as having a moderate or severe condition in the transcriptome experiments and 90% in the proteome experiments. In total, transcriptomic data of 39 studies (Blalock et al., 2004, 2011; Zhang et al., 2005; Dunckley et al., 2006; Lesnick et al., 2007; Liang et al., 2007; Scherzer et al., 2007; Simunovic et al., 2009; Cox et al., 2010; Elstner et al., 2011; Dumitriu et al., 2012, 2016; Feyeux et al., 2012; Berchtold et al., 2013; Hokama et al., 2014; Riley et al., 2014; Calligaris et al., 2015; Dijkstra et al., 2015; Labadorf et al., 2015; Magistri et al., 2015; Prudencio et al., 2015; Raman et al., 2015; Ring et al., 2015; Kapeli et al., 2016; Lin et al., 2016; Scheckel et al., 2016; Lim et al., 2017a,b; Gagliardi et al., 2018; Mehta et al., 2018; Stopa et al., 2018; Mathys et al., 2019; Meyer et al., 2019; Otake et al., 2019; Swindell et al., 2019; Switońska et al., 2019; Al-Dalahmah et al., 2020; Dols-Icardo et al., 2020; Higginbotham et al., 2020) and proteomic data of 22 studies were acquired (Fang et al., 2009; van Dijk et al., 2012; Varghese et al., 2013; McQuade et al., 2014; Riley et al., 2014; Collins et al., 2015; Dumitriu et al., 2016; Hondius et al., 2016; Ratovitski et al., 2016; Lachén-Montes et al., 2017, 2019; Seyfried et al., 2017; Umoh et al., 2018; Zhang et al., 2018; Higginbotham et al., 2019, 2020; Iridoy et al., 2019; Bader et al., 2020; Johnson et al., 2020; Oeckl et al., 2020; Rotunno et al., 2020; Wingo et al., 2020) (see also Table 1). A table giving the number of samples per condition, the severity state of the included samples, and the used technology is given in Supplementary Table 4.
For the methylomic data, we used all data from the PDMethDB (Wang C. et al., 2020) (last accessed December 13, 2021), the EWAS Atlas (Li M. et al., 2019) (accessed 01.02.21) for the four respective diseases, and other data we found through literature research using PubMed and Google Scholar. Queries for “epigenome-wide” OR “epigenome wide” OR “EWAS” OR “genome-wide” AND “methylation” OR “genome wide” AND “methylation,” as well as “metastudy” AND “neurodegenerative disease” AND “epigenetic,” were used to find studies published after 2010. Combined, the databases and literature research resulted in the inclusion of data of 20 studies (Bakulski et al., 2012; Kaut et al., 2012; Masliah et al., 2013; de Jager et al., 2014; Lunnon et al., 2014; Sanchez-Mut et al., 2016; Watson et al., 2016; Zhang et al., 2016; Young et al., 2017, 2019; Gasparoni et al., 2018; Smith et al., 2018, 2019; Zadel et al., 2018; Altuna et al., 2019; Li P. et al., 2019; Tarr et al., 2019; Go et al., 2020; Lu et al., 2020; Marshall et al., 2020), one of which contained data for both AD and PD. As the higher availability of methylome data for Alzheimer's and Parkinson's diseases made it possible, we chose to include exclusively brain tissue-derived data for those two diseases. However, as the data availability for HD and ALS were much poorer, only blood tissue data were included for those NDDs. In total, we gathered data from 234 different studies for the four respective diseases and omics-layers (see Table 1).
Data Management
The raw gene lists obtained from the transcriptomic, proteomic, and methylomic data were filtered for a false discovery ratio (FDR) < = 0.05, the proteomic data were mapped to gene names, and all the obtained gene names (from genomic, transcriptomic, proteomic, and methylomic data) were then mapped to the list of current protein-coding gene symbols (22 March 2021) from the HUGO Gene Nomenclature Committee (Tweedie et al., 2021) by mapping all alias names to the original gene symbol. Those names that were not found as any gene symbol or alias were discarded. The following data management step consisted of removing those genes that appeared as significantly altered only once in our transcriptomic, proteomic, and methylomic data. However, since the database for our methylomic and proteomic data in ALS and for the proteomic data in HD was very sparse, we decided to retain all significant genes for these gene lists to interpret a potentially noisy signal instead of hardly any signal at all.
Mean Direction of Regulation
The transcriptomic, proteomic, and methylomic data also contained information about the regulation of these significant genes/proteins. This information was contained as G-fold change (FC), log2 FC or other in transcriptomic and proteomic experiments, and as beta values in some methylomic experiments. To make this information from the different data sources as comparable as possible, we derived the mean direction of regulation (MeanRegDir) for all significantly conspicuous genes in each of the three omics levels, which equals to 1 if a gene/protein was overexpressed/hypomethylated in the disease group in every instance in which it occurred. Conversely, MeanRegDir indicates a value of −1 if a gene in the disease group was downregulated/hypomethylated in all experiments in which it appeared as significantly striking. If the signal is ambiguous, MeanRegDir will give a value between −1 and 1, as indicated in Equation (1).
Equation 1: Calculation of mean regulation of direction
with
n = number of appearances for gene with FDR ≤ 0.05
geneifoldChange = fold change of genei
Method 1: Omics-Layer Intersections per Disease
By intersecting the four analyzed omics-layers per disease, we were able to test whether the number of shared genes between each pair of omics-layers per disease was significantly increased. We used a hypergeometric test for the overlapping sets, with the total amount of 19,207 gene symbols of protein-coding genes (HUGO Gene Nomenclature Committee, 22 March 2021) (Braschi et al., 2019) as the total population. To also have a measure for the effect size of the significantly high numbers of genes in the overlapping sets and thus being able to differentiate between the differently significantly found large gene sets, we calculated the effect size of the hypergeometric test results by setting the difference between observed and expected value in relation to the standard deviation of the hypergeometric distribution. This led to the following formula and the value effsize that gives the number of standard deviations by which our expected value is beyond/below the expected value:
Equation 2: Effect size of the hypergeometric test results
with:
with:
Intersections were performed and visualized using the R (version 4.0.2) (Urbanek et al., 2014) package venn (version 1.10) (Dusa, 2016).
Method 2: Multi-Omics Conformity
Stacked Bar Plots
In order to visualize those genes, which are appearing as altered/differentially expressed in multiple omics-layers, stacked bar plots showing conformity in genomics, transcriptomics, proteomics, and methylomics data (Supplementary Presentation 2—StackedBarPlots) were created using the python package matplotlib (Hunter, 2007). As the overlap between genomics and the other three omics-layers was rather low, stacked bar plots were also created for those genes appearing only in transcriptomic, proteomic, and methylomic levels. Plots were only created if there was any overlap. To gain insights into the direction of regulation (up- or downregulation) for the overlapping genes, the stacked bars are also color-coded. The color represents the ratio of studies in which a gene was significantly up- or downregulated (see Equation 1 Calculation of mean regulation of direction). This data representation shows information about the certainty of the direction of differential regulation as well as coherency between transcriptomic, proteomic, and methylomic over- or underrepresentation or hyper-/hypomethylation, respectively.
Correlation Test
The pearson function that is available in the scipy.stats module (Virtanen et al., 2020) was used to compute the correlation and its two-sided p-value for the relation between transcriptomic and proteomic direction of regulation. The correlation analysis showed if there was a significant correlation between the direction of regulation in the pairwise intersection between the transcriptomic, proteomic, and methylomic levels. The value of the pairwise correlation and information about the p-value of the correlation test was calculated for each pairwise intersection of the transcriptomic, proteomic, and methylomic data layers for each disease. The results are shown as a heatmap in Figure 2.
Method 3: Protein-Protein Interaction Networks
The significantly distinctive genes and proteins that emerged in our analysis represented a variety of processes connected to the respected NDD. Consequently, the functional analysis of all genes that emerged for an individual omics-layer for a particular NDD would lead to results showing a combination of different related processes and would, therefore, lower the statistical power of the hypergeometric test and interpretability of the results.
To better separate the distinct processes related to our gene sets, we constructed protein-protein interaction (PPI) graphs for each omics-layer of each NDD and isolated communities of high modularity within that graph with the louvain algorithm (Blondel et al., 2008). These communities of high modularity were then used for the functional analyses and showed clearly distinct processes for our different gene sets.
Network Construction
PPI networks were constructed to visualize and utilize protein-protein interaction data for each omics layer per NDD, which were subsequently used to identify communities of high modularity and associated hub genes within each network. The networks were constructed using the Python module louvain (2.6.3) (Hagberg et al., 2008). The PPI data were based on the louvain dataset for human PPI enrichment (species = 9,606, accessed on October 18, 2021) and were filtered for those PPI with a combined score > = 400 (default value). The combined score in string DB consists of a combination of the individual scores (annotated pathways, gene neighborhood, …), emerging from the underlying databases and is typically higher than the individual scores, as it expresses increased confidence if a connection between two genes is supported by different types of evidence (von Mering et al., 2005). This resulted in a graph that consisted of nodes representing the genes that are connected by weighted edges, representing the combined interaction score of the involved genes. These weights were later used in the hub gene identification to represent the importance of an edge or to calculate a distance between edges as distance = 1/weight, depending on each centrality algorithms input requirements.
Furthermore, the modularity of the networks was computed using the Python module louvain, with the louvain.algorithms.community.quality.modularity function with a resolution parameter set to 1. For this function, modularity is defined as described by Newman (2010) and shown below:
Equation 3: Modularity
with
m = number of edges
A = adjacency matrix of Graph G
ki = degree of node ui
γ = a resolution parameter (for this analysis, set to 1)
Community Creation
The community search was carried out using the Python module community (1.0.0b1) with the community.best_partition function, which computes a partition of the graph, maximizing the modularity using the louvain heuristics (Blondel et al., 2008). As the louvain algorithm's result varies with the order in which the input is given, we applicated the louvain algorithm 100 times with permutated input of the whole network for each omics-layer in each disease and calculated the whole graph's modularity, picking the best result in terms of network modularity as our final community output.
Functional Analysis and Hallmark Definition
For each of the resulting communities, we performed the functional annotation using the stringDB API (stringDB Version 11.5) and further focused on all communities with at least one biological process with an FDR < = 0.05, as we considered those communities as most helpful for creating a meaningful redefinition of the neurodegenerative hallmarks. We also visualized these communities of the networks in Arcplots and Matrixplots using the Python module nxviz (0.7.2).
Derived from the functional analysis results, we defined six hallmarks that resemble the most meaningful categorization of our results, given prior knowledge and the most recurring processes throughout our multi-omics analysis of the four NDDs. When interpreting the functional analysis results and defining not only the six hallmarks but also low-level terms for each hallmark, we especially focused on the GO terms, pathways, and stringDB local network clusters that were associated with our communities. The stringDB local network clusters are precomputed protein clusters from stringDB that are derived from hierarchical clustering of the whole stringDB PPI network and further reduced by excluding all child clusters that are too redundant and only vary from the parent cluster by less than five proteins. These clusters' names are then derived from the proteins' annotation given in databases from Gene Ontology (GO) (Carbon et al., 2021), KEGG (Kanehisa et al., 2021), Reactome (Fabregat et al., 2016), UniProt (Bateman et al., 2021), Pfam (Mistry et al., 2021), SMART (Letunic et al., 2021), and InterPro (Blum et al., 2021).
The actual analysis of the associations between our communities and the low-level terms was performed by comparing the found number of associations with the expected number of associations per NDD and omics-layer based on the overall distribution. Additionally, we performed an X2-Test across all NDDs and, also, across all omics-layers to see if there was a statistically significant difference between the expected and observed numbers of associations across the four NDDs or across the four omics-layers for all six defined hallmarks.
Hub Gene Identification
To find out if, for some of our extracted communities, there were key players clearly playing the most prominent role in their respective communities, we aimed to identify the hub genes in our communities, meaning the most central node in our community networks. In network theory, there are different concepts for determining the centrality of a node, meaning the node's dominance within the network. Three widely used centrality measures were originally described by Freeman in the late '70s, namely, degree centrality, betweenness centrality, and closeness centrality (Freeman, 1977, 1978; Freeman et al., 1979), based on the number of direct links between a node and other nodes, the mediation role of a node in a network and on the sum of the shortest paths to all other nodes in the network, respectively. For closeness centrality, the Wassermann- and Faust-improved formula was used (Wasserman and Faust, 1994). These three centrality measures were computed with the Python module networkx and were based on the following formulas:
Equation 4: Degree centrality of node ui
with:
i ≠ j;
N = number of nodes in the graph;
Equation 5: Betweenness centrality of node ui
with:
s, t ≠ ui; s ≠ t;
V = set of all nodes in the graph;
σ(s, t) = the number of shortest s, t paths
σ(s, t|ui) = the number of shortest s, t paths going through ui
Equation 6: Closeness centrality of node ui (Wasserman- and Faust-improved formula)
with:
n = number of reachable nodes from ui;
N = number of nodes in the graph;
In addition to these three centrality measures originally proposed by Freeman, we also computed the eigenvector centrality of each node (Newman, 2010; Bonacich, 2015), which gives a centrality score to each node that is proportional to the sum of the centrality scores of each node's neighbors.
Equation 7: Eigenvector centrality of node ui
Ce(ui) is the i-th element of the vector x defined by:
with A = adjacency matrix of the graph with eigenvalue λ.
The current literature on finding hub genes in biological networks differs but often uses the node degree (Li et al., 2018; Zhou et al., 2018) or weighted gene coexpression network analysis (Zhang and Horvath, 2005) as a basis for identification of hub genes. Here, we combined the four mentioned centrality measurements that give information for each node ui about:
• The number of other nodes in the network connected to ui
• The number of shortest paths between two other nodes in which ui is involved
• The accumulated distance to all other nodes in the network from ui
• The importance of nodes connected to ui
We considered hub genes in our communities as those that are among the most important nodes in several of these centrality measures. Accordingly, we defined hub genes as being among the top three nodes in at least three of the four centrality measures mentioned. Genes that appeared among the three most central nodes in the network on all four calculated measures of centrality, or that were the single most central nodes according to at least three measures of centrality, were given special consideration for subsequent interpretation of hub genes in our community networks.
Results
Omics-Layer Intersections per Disease
The intersection of the four analyzed omics-layers per disease showed significantly high numbers of shared genes between the transcriptomic and proteomic levels for all four analyzed NDDs (Figure 1, Table 2). All other pairwise intersections show a significantly high number of shared genes in two to three of the analyzed NDDs, while AD shows significantly high numbers of shared genes for all pairwise intersections of omics-layers. AD also showed the highest number of genes in total across all omics-layers with 14,970 genes. PD followed with 6,026, and then HD with 5,795 and ALS with 2,725 genes (Figure 1). The mean direction of regulation was close to 0 for all omics-layers in AD (0.13, −0.1, −0.06 for transcriptomic, proteomic, and methylomic data, respectively); in PD, the mean direction of regulation was −0.59 for the transcriptomic level, 0.42 for the proteomic level, and 0.33 for the methylomic level. For HD, the methylomic layer showed a mean direction of regulation of −0.8, while the other omics-layers were closer to 0 (0.04 transcriptomic, −0.18 proteomic). In ALS, the transcriptomic and proteomic layers showed a positive mean direction of regulation (0.12 and 0.16), while the methylomic layer showed a strong negative mean direction of regulation (−0.75). For the genomic level, there are no mean directions of regulation as these data show an association between the presence of specific SNPs and the respective NDDs. The calculated effect sizes for the hypergeometric test results show the highest values in the transcriptomic-proteomic intersection for all NDDs, with differences between expected and observed numbers of genes of more than 10 standard deviations (stds) except for PD (second highest value with 9.9 stds). In PD, the highest effect size was achieved in the transcriptomic-methylomic intersection with 13 stds difference. All other comparisons showed much weaker effect sizes, with values below 5 stds.
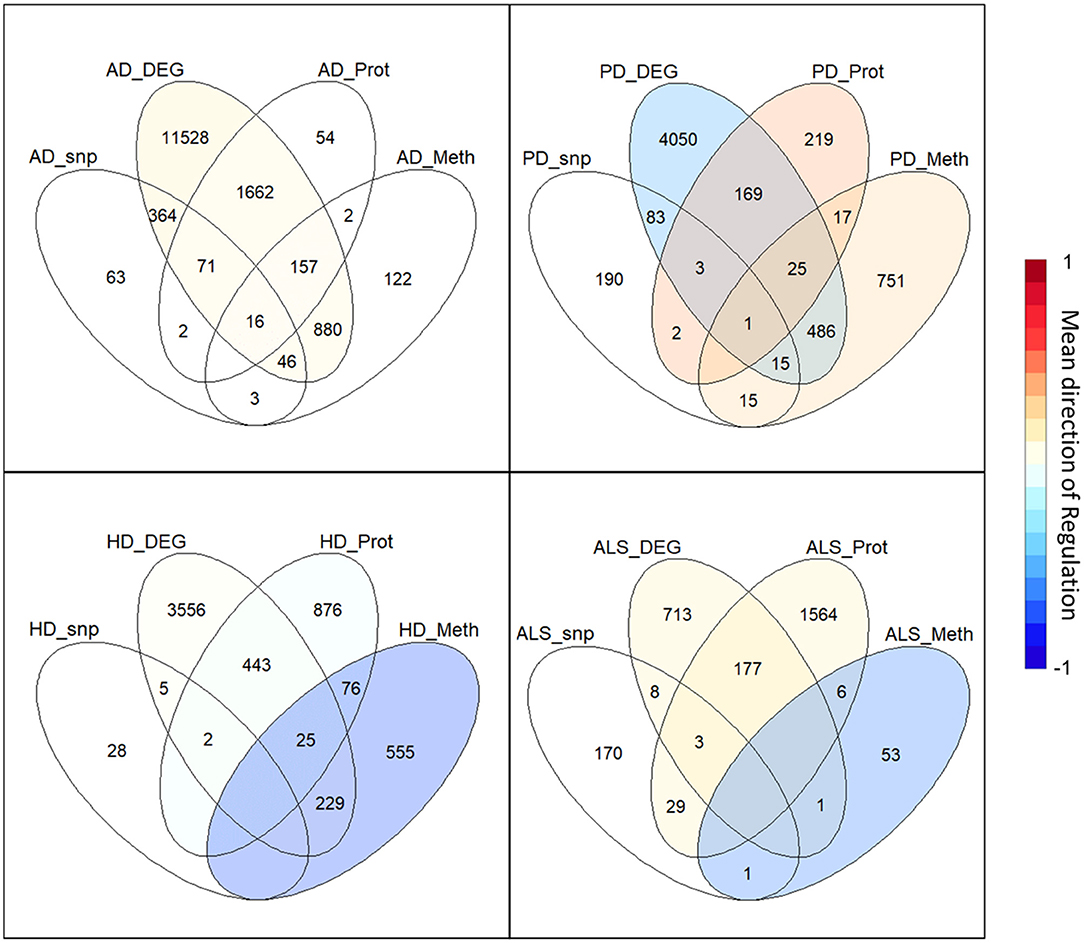
Figure 1. Omics-layer intersections per disease: The Venn diagrams for the four NDDs, AD, PD, HD, and ALS are shown with the numbers of shared genes in the intersections of the specific omics-layers. Additionally, the transcriptomic, proteomic, and methylomic layers of each NDD are colored to give each layer's mean direction of regulation. This color represents the color of only the respective layer, not of the individual intersections, as those have a specific regulation for each omics-layer.
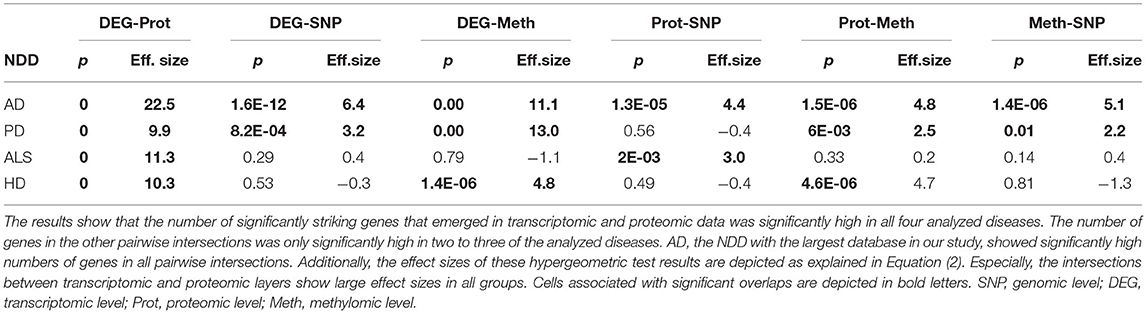
Table 2. Omics-layers intersections per disease: the number of resulting genes in each pairwise intersection of omics-layers per disease was tested for enrichment in a hypergeometric test.
Multi-Omics Conformity
The correlation between the transcriptomic and proteomic mean direction of regulation of the genes in the transcriptomic and proteomic layers' intersection in AD, HD, and ALS was significantly high (all p < 10−5) (see Figure 2). The correlation for this layer intersection in PD was not significantly high. However, the correlation was slightly positive, even though the mean direction of regulation for the whole transcriptomic PD data was highly negative (−0.59), while it was highly positive (0.42) for the whole proteomic PD data. All other correlations of mean direction of regulation in the pairwise intersections between transcriptomic, proteomic, and methylomic layers per disease were neither significantly high nor low (p ≥ 0.05).
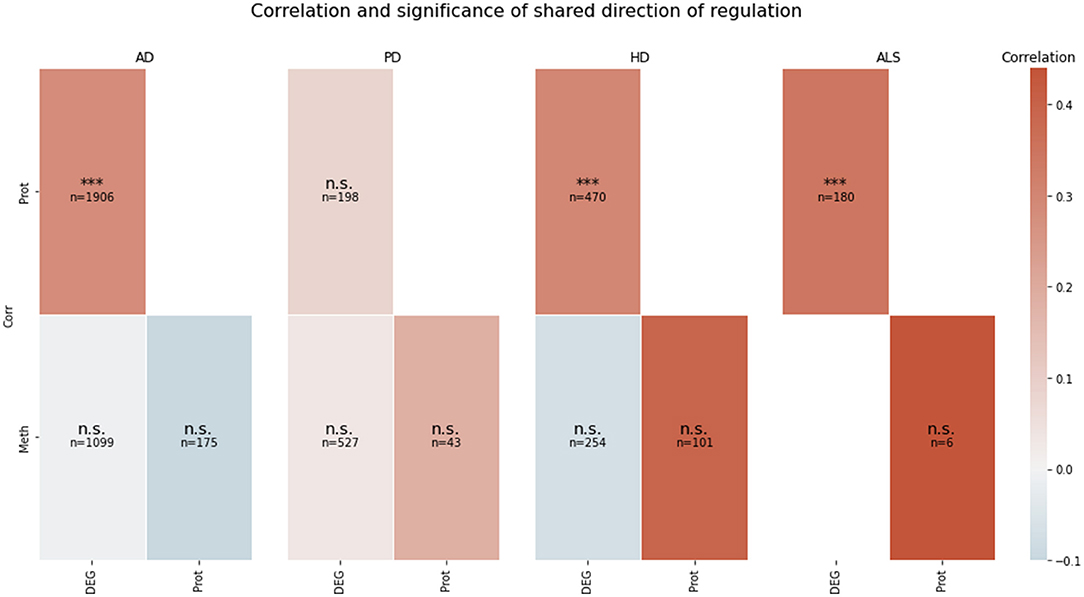
Figure 2. Pairwise correlation of the omics-layers per disease. For each of the four NDDs, a heatmap is showing the pairwise correlation value of the mean direction of regulation between each pair of omics-layers, which shows a direction of regulation (transcriptomics, proteomics, methylomics). Additionally, the significance of the correlation is given in each tile (n.s., not significant, ***p < 0.001). SNP, genomic level; DEG, transcriptomic level; Prot, proteomic level; Meth, methylomic level.
Protein-Protein Interaction Networks
The network construction step led to a PPI network for each analyzed omics-layer in all four analyzed NDDs. However, the constructed communities of the networks based on the genomic layer in HD and ALS, as well as the methylomic layer in ALS, showed a little functional signal. Consequently, we focused on the remaining 13 PPI networks for the analysis.
These remaining networks varied between 7 (PD proteome) and 15 (PD methylome) communities with sufficient functional annotation (any biological process with FDR < = 0.05) to be further considered in our analysis. The modularity of these 13 PPI networks varied after community creation between 0.484 (PD proteome) and 0.588 (ALS proteome), thus showing a small deviation in modularity values across the different NDDs and omics-layers. The modularity value is positive if the communities in the network show higher interconnectedness than randomly expected; negative values if there are less connections within the communities than randomly expected are strictly lower than 1 (Equation 3: Modularity). Thus, the 13 networks that we further consider show more connections within their communities than expected by chance (as all have positive modularity values). Examples of those network plots for PD are given in Figures 3–6. The networks for AD, HD, and ALS can be found in the Supplementary Presentation 1—Network Figures.
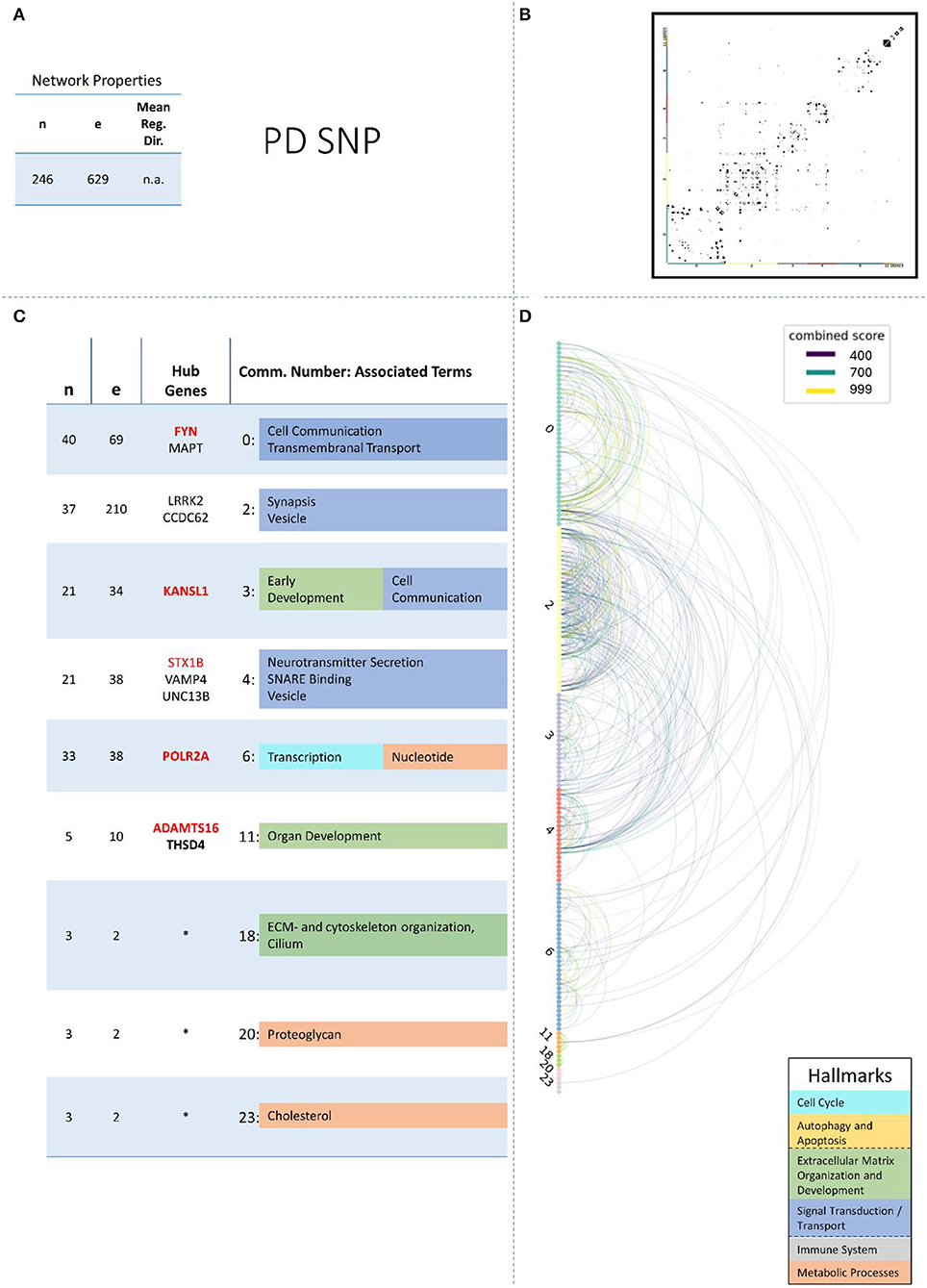
Figure 3. Summarizing figure of the network analysis results of the genomic layer of PD. The general properties, such as number of nodes (genes) and edges (a combined score > 400 between two nodes) of the PPI, network constructed with all PD genomic genes are given in Subfigure (A). The individual connections with a combined score > = 400 between each two genes are also given in a matrix plot (B) and an Arcplot (D) that are ordered by community. The latter shows the confidence of the connection between two genes additionally by the color of the arc. The general properties of each individual community, as well as their hub genes and the categorization of their functional annotation, are given in the table in Subfigure (C). The connected processes are colored by the associated hallmarks that are also displayed in the bottom right corner.
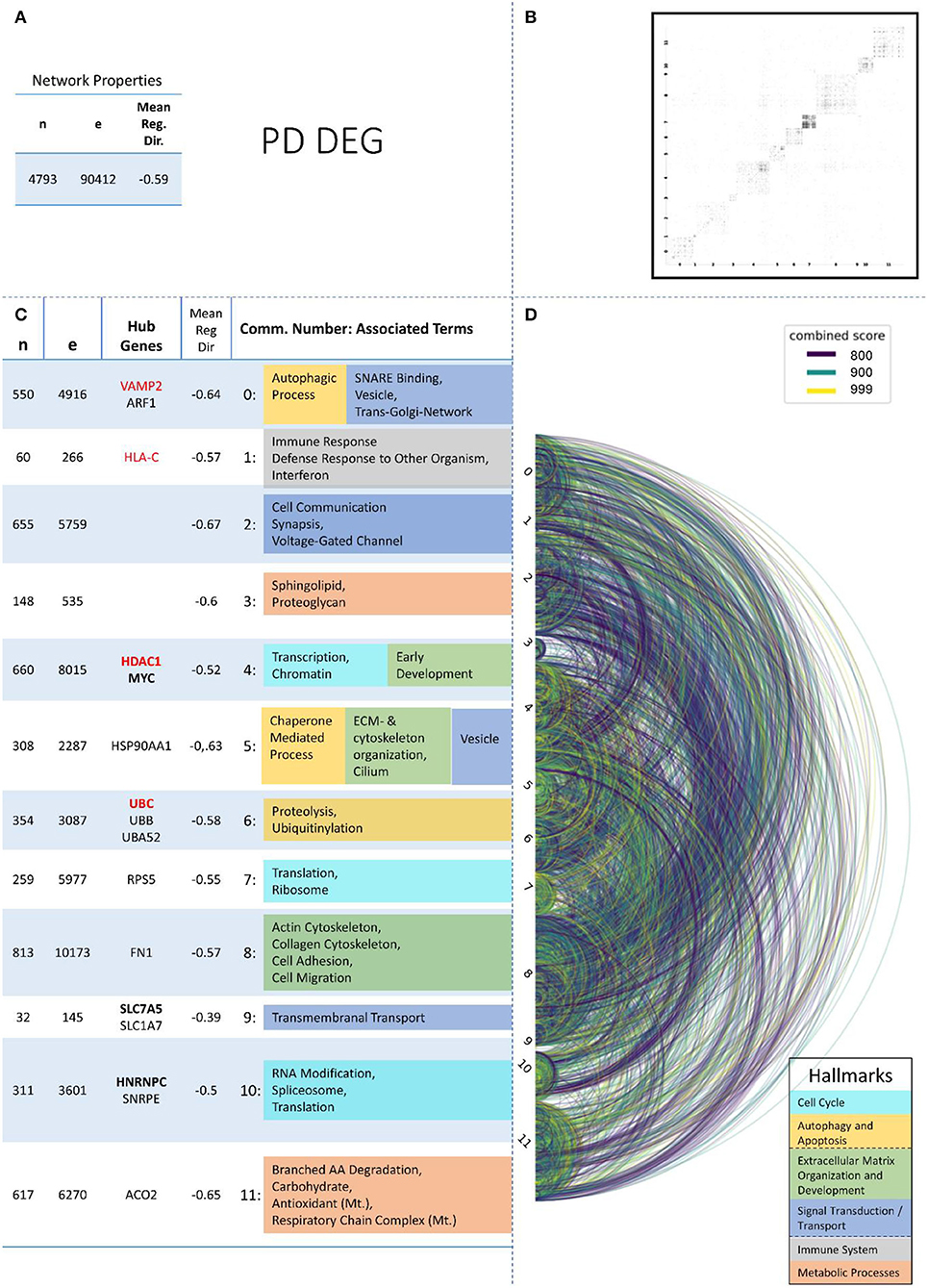
Figure 4. Summarizing figure of the network analysis results of the transcriptomic layer of PD. The general properties, such as number of nodes (genes) and edges (a combined score > 400 between two nodes) of the PPI network constructed with all PD transcriptomic genes, are given in Subfigure (A). The individual connections with a combined score > = 800 between each two genes are also given in a matrix plot (B) and an Arcplot (D) that are ordered by community. The latter shows the confidence of the connection between two genes additionally by the color of the arc. The general properties of each individual community, as well as their hub genes and the categorization of their functional annotation, are given in the table in Subfigure (C). The connected processes are colored by the associated hallmarks that are also displayed in the bottom right corner.
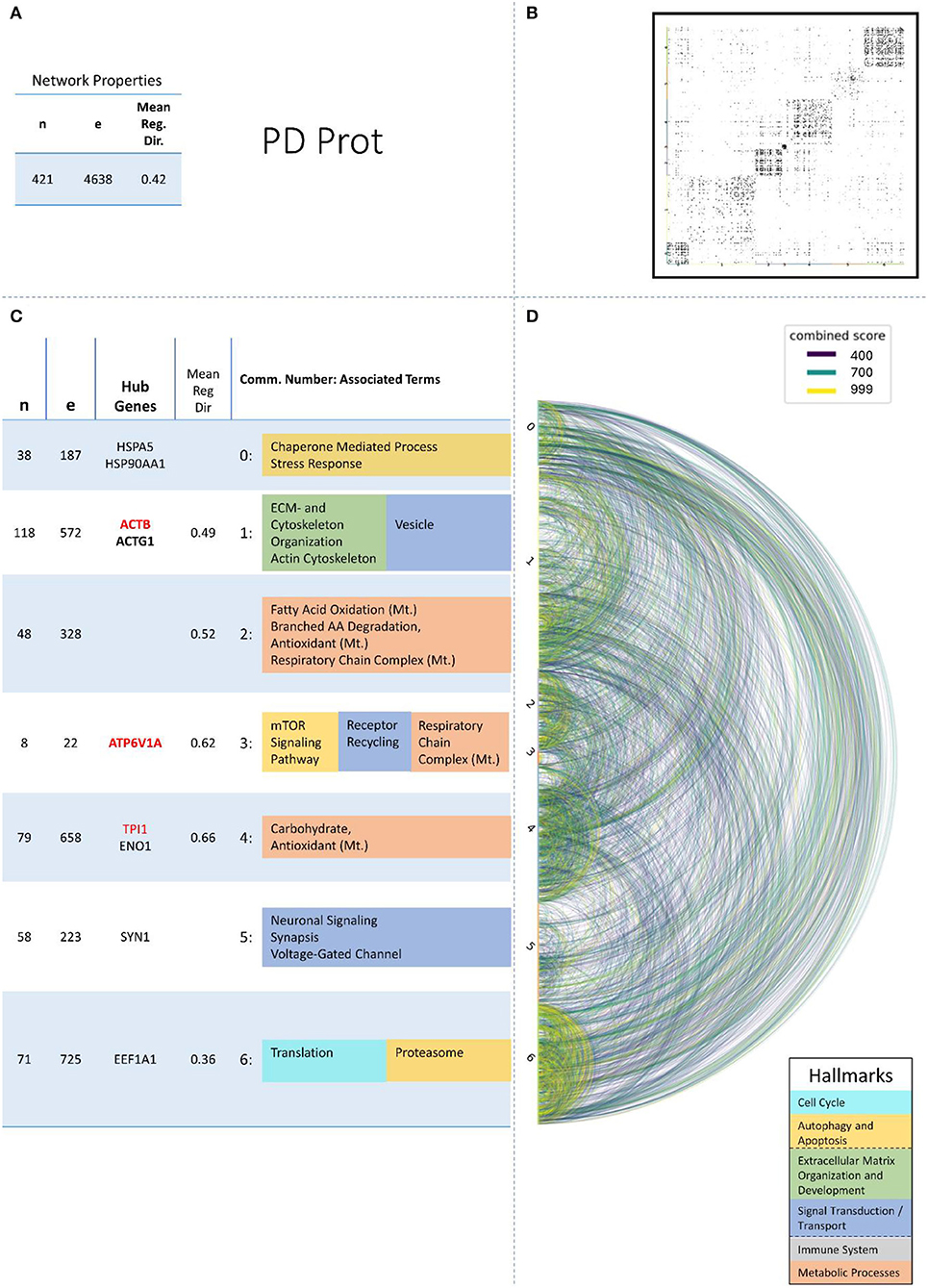
Figure 5. Summarizing figure of the network analysis results of the proteomic layer of PD. The general properties. such as number of nodes (genes) and edges (a combined score > 400 between two nodes) of the PPI network constructed with all PD proteomic genes, are given in Subfigure (A). The individual connections with a combined score > = 400 between each two genes are also given in a matrix plot (B) and an Arcplot (D) that are ordered by community. The latter shows the confidence of the connection between two genes additionally by the color of the arc. The general properties of each individual community, as well as their hub genes and the categorization of their functional annotation, are given in the table in Subfigure (C). The connected processes are colored by the associated hallmarks that are also displayed in the bottom right corner.
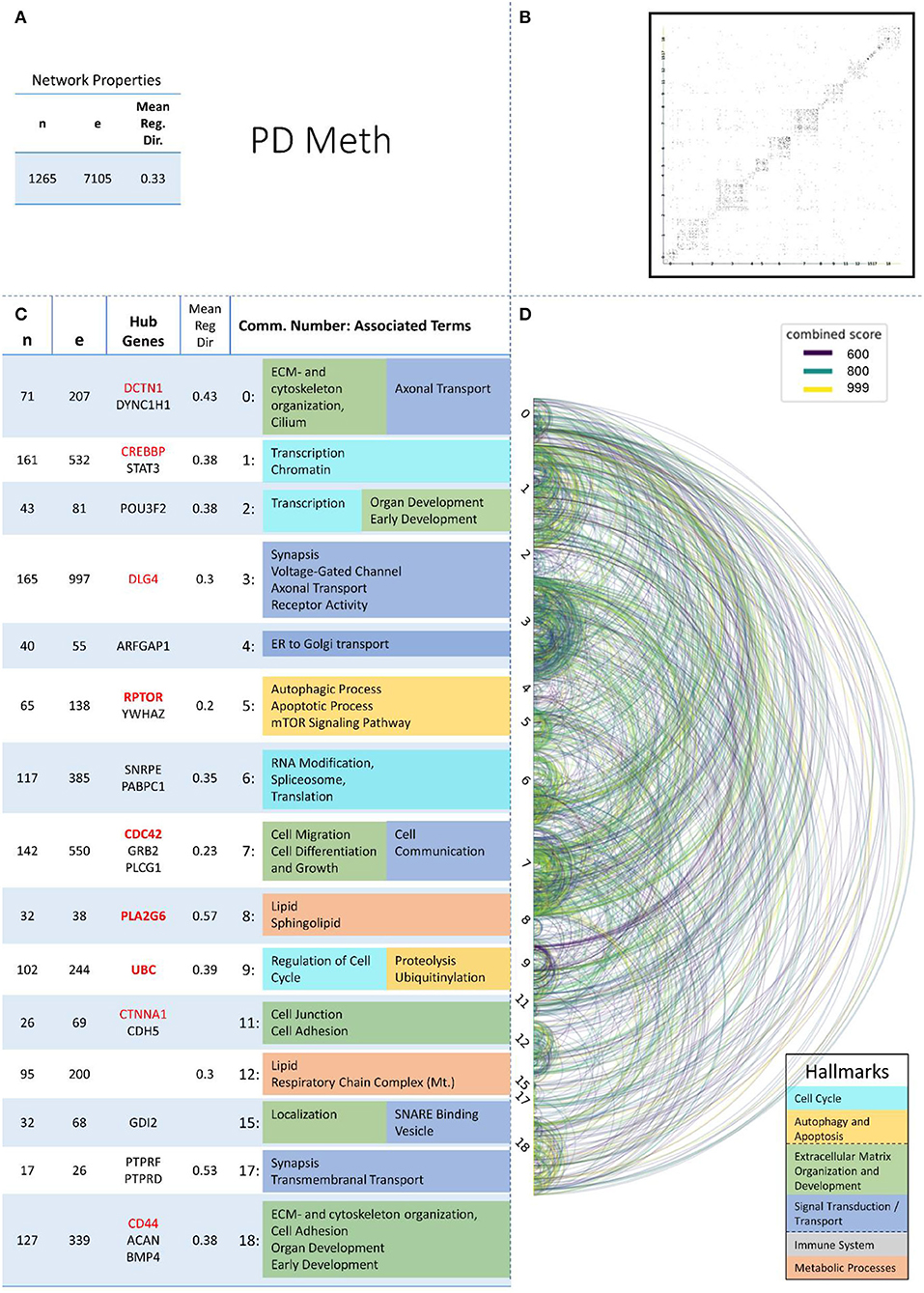
Figure 6. Summarizing figure of the network analysis results of the methylomic layer of PD. The general properties, such as number of nodes (genes) and edges (a combined score > 400 between two nodes) of the PPI network constructed with all PD methylomic genes, are given in Subfigure (A). The individual connections with a combined score > = 600 between each two genes are also given in a matrix plot (B) and an Arcplot (D) that are ordered by community. The latter shows the confidence of the connection between two genes additionally by the color of the arc. The general properties of each individual community, as well as their hub genes and the categorization of their functional annotation, are given in the table in Subfigure (C). The connected processes are colored by the associated hallmarks that are also displayed in the bottom right corner.
Hallmark Definition
The hallmarks of neurodegenerative diseases that we defined based on the functional analyses of the communities are a deregulated Cell Cycle, defects in autophagy and apoptosis on the cellular level, a defective Extracellular Matrix Organization and misguided cell and organ development, the impaired Signal Transduction/Transport of vital molecules within and between cells within the local tissue environment, an excessive Immune System response, and defective Metabolic Processes in the whole systemic environment.
The results of the X2-test showed that there is a significant difference between the expected and observed values for the four omics-layers across all six hallmarks (p < 0.05), but not for the four NDDs across all six hallmarks (see Supplementary Table 3—Low-Level Term Associations).
The most striking results we found when analyzing the accumulated numbers of associations between our communities and low-level terms across the four NDDs and also across the four omics-layers were the low number of associations found in PD for the Immune System hallmark and the low number of associations in the methylomic layer in the Metabolic Processes hallmark while having a high number of associations in the proteomic layer.
A more detailed description of the results is presented in the following;
Cell Cycle
In the category of Cell Cycle 56 communities could be assigned to the low-level terms Regulation of Cell Cycle, Spindle Apparatus, DNA repair, Transcription, RNA Modification, Spliceosome, Translation, Mitochondrial Translation, and Chromatin.
AD showed the most low-level terms (21), followed by PD, HD, and ALS (15, 13, 7), while the transcriptomic and methylomic layers showed a higher number of low-level terms (21, 20) than the proteomic and genomic layers (11 and 4) (see Table 3). In general, for the hallmark Cell Cycle, the numbers of associations between communities and low-level terms neither strongly differed across NDDs nor across omics-layers from the expected values, given the overall distribution of associations across all hallmarks, NDDS, and omics-layers.
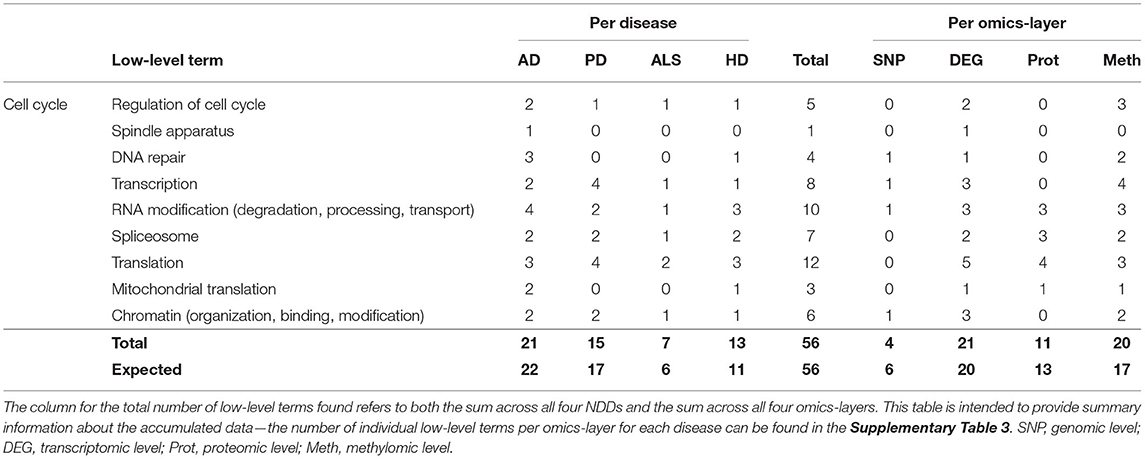
Table 3. Accumulated number of low-level terms per NDD and per omics-layer for the hallmark Cell Cycle.
Autophagy and Apoptosis
Of all NDDs and omics-layers, 46 communities were involved in autophagy and apoptosis specified by low-level terms like Chaperone-Mediated Process, Proteolysis, Ubiquitinylation, Proteasome, Stress Response, Apoptotic Process, and the mTOR and NfkB Signaling Pathways.
Again, AD showed the most associations (16), followed by PD, HD, and ALS (13, 9, and 8), as shown in Table 4. The expected number for ALS is 5, in contrast to the observed 8 associations, but, overall, the deviations between observation and expectation for the NDDs in this hallmark were quite low.
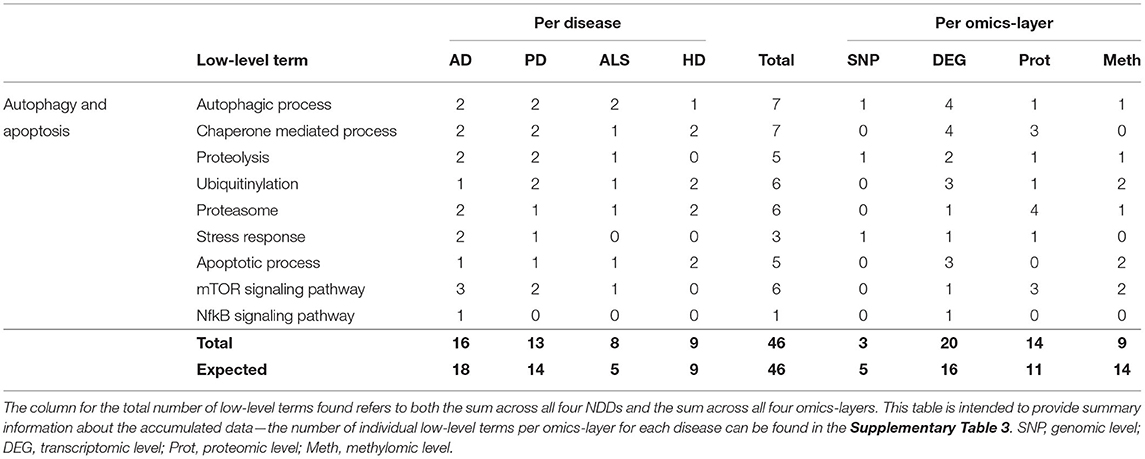
Table 4. Accumulated number of low-level terms per NDD and per omics-layer for the hallmark autophagy and apoptosis.
The distribution of associations across the four omics-layers shows that the transcriptomic data had the most associations (20), followed by the proteomic, methylomic, and genomic layers (14, 9, and 3). Here, the discrepancy between observation and expectation is larger, with the methylomic data showing only 9 associations, whereas 14 are expected, and the transcriptomic and proteomic layers showing 4 and 3 more associations, respectively, than expected.
Extracellular Matrix Organization and Development
In total, 80 associations were found between communities and the low-level terms for Extracellular Matrix (ECM) and Cytoskeleton Organization, with the different fibril components Actin -, Collagen -, and Keratin Cytoskeleton, Cilium as the bridge between ECM and cytoskeleton, Cell Adhesion, Focal Adhesion, Cell Junction, Cell Migration along microtubules, Cell Differentiation and Growth (e.g., neuron, axon, dendrite, organelle), Localization of cells or proteins, Organ Development (brain, muscle, heart, bone, blood vessels), and Early Development (embryogenesis and cell fate commitment).
The distribution of the number of associated low-level terms across the four NDDs is according to the expectation that can be derived from the summed number of associations across all low-level terms, with AD showing the highest number of associations (33), followed by PD (26), HD (14), and ALS (7), as given in Table 5, with having the highest deviation between observation and expectation for HD.
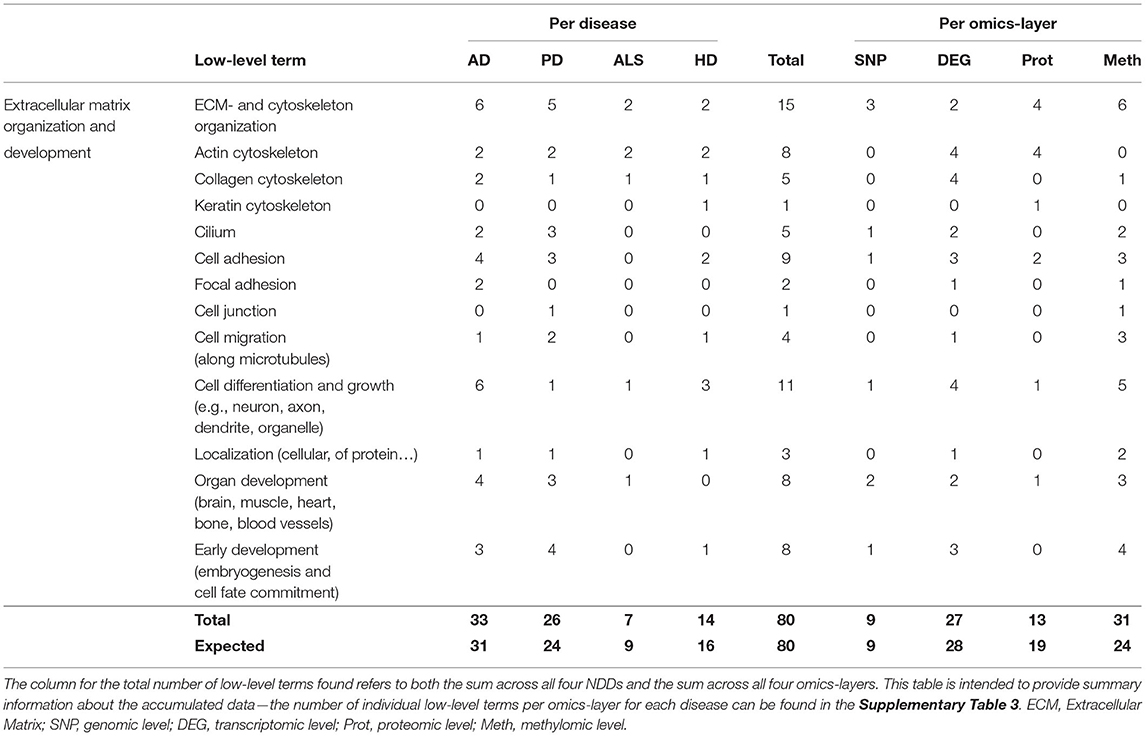
Table 5. Accumulated number of low-level terms per NDD and per omics-layer for the hallmark Extracellular Matrix Organization and Development.
However, the distribution of associations across the different omics-layers for the hallmark ECM Organization and Development deviates from the expectation for the proteomic and methylomic layers. The highest number of associations can be observed on the methylomic layer, closely followed by the transcriptomic (31, 27) and then by the proteomic and genomic layers (13, 9). Here, the methylomic layer shows a nearly 25% higher number of associations than expected, while the number for the proteomic layer is nearly 30% lower than expected.
Signal Transduction/Transport
This hallmark consisted of the low-level terms Cell Communication, Neuronal Signaling, Synapsis, Ion- and Voltage-Gated Channel, Neurotransmitter Secretion, SNARE Binding, Receptor Activity and Recycling, Axonal Transport, Vesicle (exo- and endocytosis, clathrin coat, extracellular exosome), Transmembranal Transport (small molecules, e.g., AA), Trans-Golgi-Network (retrograde), ER to Golgi transport (anterograde), and (Cardiac) Muscle Action Potential.
The hallmark Signal Transduction/Transport united the most associations between communities and low-level terms (88), having the most associations in AD (34), followed by PD (31), HD (17), and ALS (6). Compared to the number of expected associations, ALS showed a reduction of 4 associations (−40%) and PD, an increase of 5 associations (approximately + 20%) compared to the expectation.
Observing the results per omics-layer, it becomes apparent that not the transcriptomic but the methylomic layer showed the most associations for this hallmark (31). The number of associations in the transcriptomic layer is lower than the methylomic layer's associations (24), followed by the proteomic (18) and genomic layers (15), as given in Table 6. While the transcriptomic layer shows surprisingly little associations (– 22%), the genomic layer, on opposite, shows a surprisingly high number of associations (+50%), in contrast to the expectation.
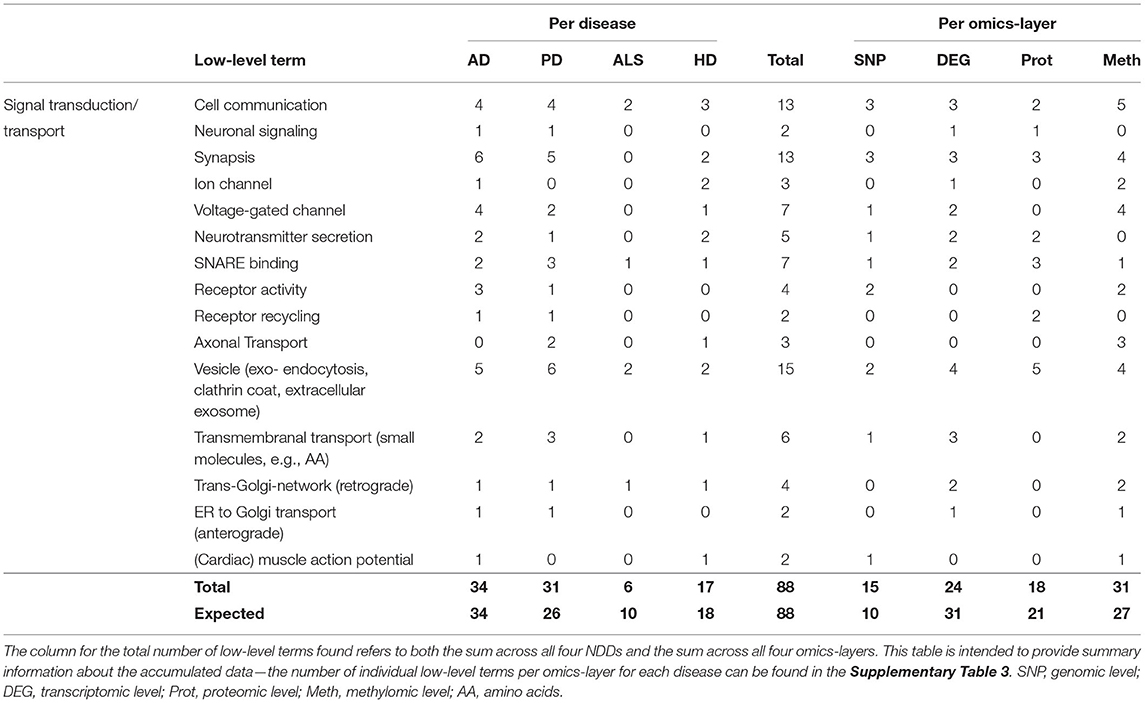
Table 6. Accumulated number of low-level terms per NDD and per omics-layer for the hallmark Signal Transduction/Transport.
A closer look at all low-level terms in relation to the individual NDD's omics-layers (see Supplementary Table 3—Low-Level Term Associations) also shows that two out of the three associations for Axonal Transport are found in the methylomic PD network, and two out of the four Receptor Activity associations are found in the AD genomic network.
Looking at the aggregated data per layer in each omics-layer also shows a high number of genomic PD associations (8 out of 88 for Signal Transduction/Transport), far exceeding the overall 4% proportion of low-level terms associated with the PD genomic layer.
At the same time, the proportion of transcriptomic ALS communities associated with the low-level terms of these hallmarks (2 of 88) is only half the proportion that might be expected based on the overall distribution of low-level terms associated with the transcriptomic ALS layer (>5%).
Immune System
Only 28 associations between communities and the low-level terms related to Immune System were found, making it the hallmark with the lowest number of associations. Low-level terms, which were referred to by these communities, were Immune Response, Defense Response to Other Organisms, Cytokine, Interferon, Complement and Coagulation Cascade, Inflammation, and Leukocyte Activation.
The highest number of associations was found in AD (13), followed by HD (7), ALS (5), and PD (3), making this the only hallmark in which PD showed the lowest number of associations (see Table 7). This number of associations found across all omics-layers in PD is more than 60% lower than expected.
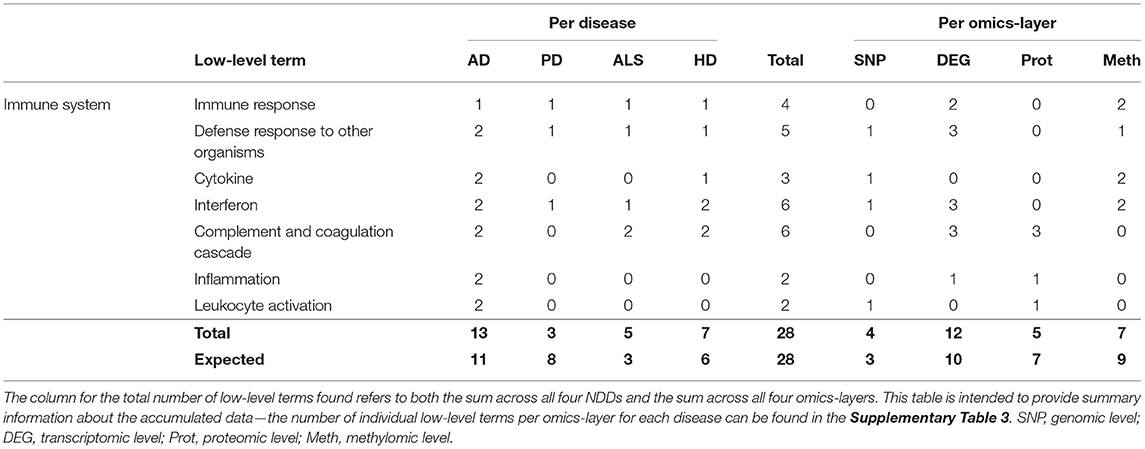
Table 7. Accumulated number of low-level terms per NDD and per omics-layer for the hallmark Immune System.
The findings in the aggregation per omics-layers seem less surprising, with the transcriptomic layer showing the most associations (12), followed by the methylomic (7), proteomic (5), and genomic layers (4).
Metabolic Processes
The hallmark Metabolic Processes consisted of the low-level terms Lipid, Cholesterol, Sphingolipid, Fatty acid oxidation [mitochondrial (Mt.)], Carboxylic Acid (Mt.), Lipoprotein, Protein, Carbohydrate, and Nucleotide, most of which referred to the respective metabolic processes and of terms like Glycosylation, NADH (Mt.) Homeostasis, Respiratory Chain Complex (Mt.), and Antioxidant (Mt.), which were tagged with the “Mt.” term if they represent processes that occur in the mitochondrion rather than in the cytoplasm.
Looking at the accumulated associations between communities and low-level terms across the four different NDDs (see Table 8), the most associations can be found across the communities of AD (26), followed by PD (20), HD (14), and ALS (7). This distribution across NDDs perfectly matches the expected distribution of associations.
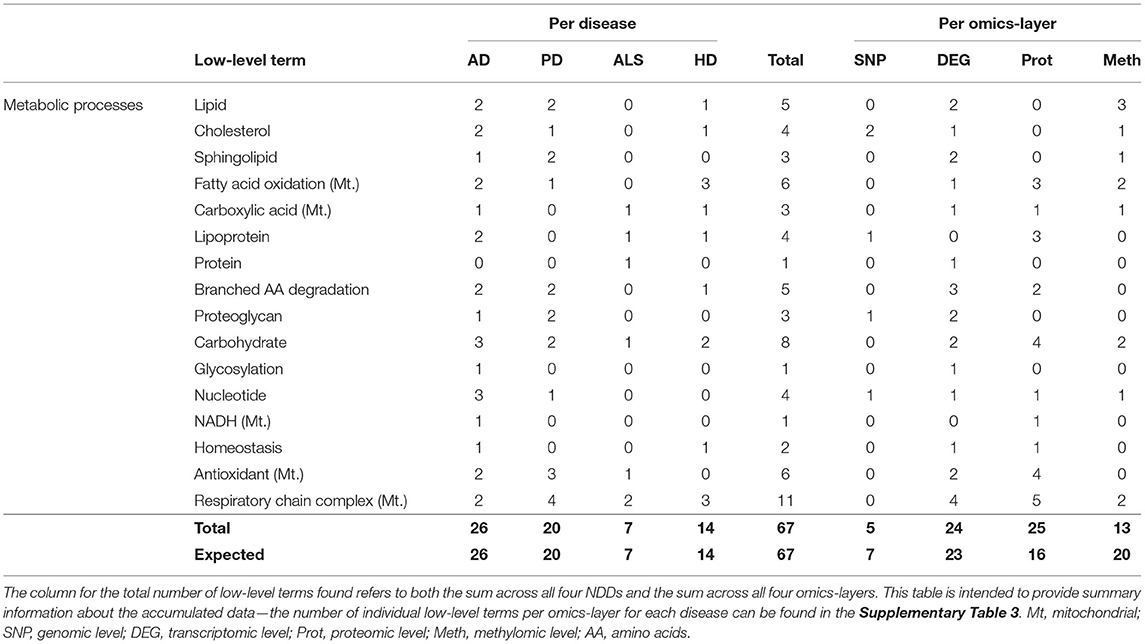
Table 8. Accumulated number of low-level terms per NDD and per omics-layer for the hallmark Metabolic Processes.
However, the distribution across the four omics-layers is more conspicuous, as Metabolic Processes are the only hallmark having the majority of the associations in the proteomic layer (25). This is closely followed by the transcriptomic (24) and then the methylomic and genomic layers (13 and 5). Following the overall distribution of associations across the omics-layers, only 16, instead of 25, associations were expected across the proteomic layers (+ 56%) of all NDDs, but 20, instead of 13, associations were expected for the methylomic layers of all NDDs (−35%).
The individual omics-layers per NDD showed that, especially, the proteomic layer of PD and AD had enriched numbers of associations for this hallmark, both with having more than 33% of their community associations to the low-level term in Metabolic Processes, while, overall, only ~18% were expected.
Discussion
Omics-Layer Intersections per Disease
The intersection of genes found in genomic, transcriptomic, proteomic, and methylomic data per disease showed that, while the number of shared genes between the transcriptomic and proteomic level was significantly high in all four investigated NDDs, the significance of the intersections was more heterogenous in the other comparisons.
Only AD showed a significant number of shared genes in all pairwise intersections of the four analyzed omics-layers. The fact that ALS methylomic and HD genomic data consisted of only two studies and thus had a very small database (61 and 35 genes) may have contributed to the non-significance of pairwise comparisons that considered the methylomic level for ALS and the genomic layer for HD. However, even though the database was much broader for HD methylomic data (885 genes), these genes also stem from only two studies, but showed significant overlaps in all pairwise intersections, except for the one with genomic data. Summarizing, especially those pairwise comparisons with a low number of expected overlapping genes, seemed to not show a significantly large pairwise overlap. All pairwise intersections that are based on two large groups of genes and, therefore, showed an expected number of shared genes > = 10 also showed a significantly high number of overlapping genes in these hypergeometric tests (Supplementary Table 2—Hypergeom Test Per Disease).
The fact that the effect size was highest for the transcriptomic-proteomic intersection in AD, ALS, and HD and, also, very high in PD (9.9) seems as if these layers shared the most striking relation in terms of overlapping genes. However, in PD, the effect size is even higher between the transcriptomic and methylomic layers. PD is also the only disease in which not proteomic and transcriptomic layers showed the largest database in terms of including the most genes but the transcriptomic and methylomic data did. Thus, in all NDDs, especially those intersections, showed the largest effect sizes that were based on the omics-layers, with the most genes per NDD. The statement that the transcriptomic and proteomic layers of our data showed high significantly enriched numbers of genes in their overlap in all analyzed NDDs holds true; however, it is, to this point, unclear if the high effect sizes in contrast to the other intersections are due to biological facts or to the broader database for these omics-layers in our data.
A more surprising point is the fact that the mean direction of regulation was vastly unequal to zero for both, the transcriptomic and the proteomic data in PD. This was also the case for the methylomic layer of HD and ALS, but, given that the transcriptomic and proteomic layers of PD are based on much more studies (11 and 7, while 2 studies for HD and ALS methylome), this high absolute mean direction of regulation, in combination with the dichotomy of these values, constitutes an interesting finding. The finding of a mainly (70%) negative direction of regulation for PD transcriptomic differentially expressed genes was already described in a meta-study, focusing only on microarray data (Kelly et al., 2019). However, the reason for this phenomenon remains unclear.
Multi-Omics Conformity
The finding of significant overlaps between the transcriptomic and proteomic data for only three out of four NDDs seems to be surprising and to hint at an extraordinary phenomenon in the posttranscriptional or translational processes in PD. However, given that the mean direction of regulation for the PD transcriptomic data was highly negative (−0.59), while the PD proteomic data were, overall, highly positive (0.42), it is striking that the genes in the intersection of these two omics-layers are still positively correlated, even though not significantly. Thus, this result might show a signal for some altered posttranscriptional behavior in PD in contrast to the other NDDs, but this could also be an artifact of the striking negative mean direction of regulation in the transcriptomic and the similarly strong positive mean direction of regulation in the proteomic data that were discussed earlier.
However, even the highly significant correlations between the transcriptomic and proteomic data in AD, HD, and ALS reached values of r = 0.283 (AD) to r = 0.344 (ALS), which might seem surprisingly small, following James Watson's simplified version of the central dogma of molecular biology (Watson et al., 1988).
Even though proteins are produced from mRNA, the correlation between mRNA levels and protein abundances is moderate. This non-trivial mRNA–protein relation is a general phenomenon, ranging from yeast to humans (De Sousa Abreu et al., 2009), and can have biological or, simply, technical reasons. Biological reasons can be found both on the posttranscriptional and on the translational levels, where many different mechanisms enhance or repress the synthesis of proteins from a certain copy number of mRNA molecules (Crick, 1958; Varshavsky, 1997; Fire et al., 1998; Kozak, 1999; Glickman and Ciechanover, 2002; Pillai et al., 2007; Kurreck, 2009; Maier et al., 2009; Chursov et al., 2013; Komar, 2016; Liu et al., 2016; McShane et al., 2016; Lau et al., 2018).
Protein-Protein Interaction Networks
The Hub Gene HDAC1
On the transcriptomic level of all 4 diseases communities were found, that showed Histone Deacetylase 1 (HDAC1) as one of their hub genes. HDAC1 deacetylates lysine residues on the N-terminal part of the core histones H2A, H2B, H3, and H4, thereby contributing to epigenetic silencing of active chromatin (Carroll et al., 2018). HDAC1 plays an important role in transcriptional regulation, cell cycle progression, and developmental events. This was also reflected in the low-level terms of the associated communities of all four diseases: In AD and PD, they referred to transcription, chromatin, and early development, together with the hubgenes TP53, EP300, and MYC, respectively. In ALS, the low-level terms referred to regulation of cell cycle, transcription, and chromatin, together with the hub gene CCND1. HD showed low-level terms about cell differentiation, cell and early development, together with the hubgenes MYC and TP53.
All the hubgenes that were related to HDAC1 are involved in similar processes like regulation of cell cycle, cell growth and migration, and development by influencing the chromatin formation (EP300), binding to DNA as transcription factors (TP53, MYC) or regulating other proteins (CCND1). Beyond the aforementioned similarities, researchers can, maybe, find differences in how the diseases developed by comparing the functions of the hub genes and their StringDB protein networks per disease.
The Hub Genes BIN1, ABCA7, and PICALM
BIN1, ABCA7, and PICALM were found to be among the three most central nodes in, at least, three of our four centrality measures and were, therefore, defined as hub genes in our analysis for the AD genomic community that was associated with the two processes, proteolysis and ECM/cytoskeleton. Approximately, 38 genes were involved in this network, showing 284 interactions giving an average node degree (Nd) of 14.9. Mutations in these three genes are well-known risk factors in AD, and all the three of them are tightly connected to amyloid ß metabolism.
BIN1 or Bridging Integrator-1 is a key player in the control of plasma membrane shaping. The depletion of BIN1 increases cellular β-site APP-cleaving enzyme 1 (BACE1) levels through impaired endosomal trafficking and reduced lysosomal degradation, resulting in an increased Aβ production.
ABCA7 is a member of the superfamily of ATP-binding cassette (ABC) transporters, which transport various molecules across extra- and intra-cellular membranes. As with BIN1, ABCA7 could be shown to be involved in the generation and processing of amyloid ß peptides (Satoh et al., 2015), but also in the lipid metabolism and in phagocytosis and immune response (Dib et al., 2021).
PICALM, the phosphatidylinositol-binding clathrin assembly protein, also affects AD risk primarily by modulating production, transportation, and clearance of β-amyloid (Aβ) peptide, but other Aβ-independent pathways are discussed, including tauopathy (Dean and Shaw, 2010), synaptic dysfunction (Jahn and Scheller, 2006), disorganized lipid metabolism (Eisenstein, 2011), immune disorder (Carter, 2010), and disrupted iron homeostasis (Xu et al., 2014).
These genes, together with the other 35 genes found to interact closely with the two low-level terms proteolysis and ECM/cytoskeleton, can now be studied in more detail by specialists in the field with respect to the relationship between the known functions of the genes and the processes in which they are involved. This combination of all three genes was only found in AD, and any of these three was found as a hub gene only in the AD genomic network.
However, BIN1 was also present in the transcriptomic [community 0 (C0)], proteomic (C1), and methylomic (C7) levels of PD, representing mainly ECM and Development and Signal Transduction/Transport processes but also one for Autophagy and Apoptosis. PICALM was also part of C0 in the PD transcriptomic network. In HD, the proteomic network showed both, BIN1 and PICALM in C0, which represent Signal Transduction/Transport processes like SNARE Binding and Vesicle. In ALS, none of these genes were involved in any considered community.
The Hub Gene APOE
APOE, the well-known risk gene for late onset AD, was found on the genomic level in AD in a community associated with the low-level terms cholesterol and lipoprotein, but also on the transcriptomic level of ALS associated with the low-level terms complement/coagulation cascade and immune response.
The complement cascade stimulates phagocytes to clear microbes and damaged cell materials, it promotes inflammation, and attacks the pathogen's cell membrane. It, thus, combines innate and adaptive immune responses and additionally integrates the coagulation system into the defense response against other organisms (Kenawy et al., 2015). In addition, some of the early components of this cascade play a beneficial role in synapse elimination during the development of the nervous system, although excessive complement-mediated synaptic pruning in the adult or injured brain may be detrimental in multiple neurogenerative disorders (Schartz and Tenner, 2020). A correlation between ALS and the complement system has been frequently described (Carpanini et al., 2019; Kjældgaard et al., 2021), but a connection to APOE still remains elusive.
Here, our study may provide a good starting point for exploring the community to which APOE belongs and the genes to which it is linked in this network. The fact that APOE is among the top three nodes according to all four measures of centrality implies that it plays an important role in this network, as it is among the three most interconnected genes, has direct links to other very important genes in the network, and plays an important role in mediating between pairwise-linked genes in the network, while it is also among the three nodes that have the shortest paths to all other nodes in the network. Although APOE has not been previously reported to be associated with the complement and coagulation cascades, it is strongly associated with a subnetwork of genes that are significantly overrepresented in this very process. About one third of the directly linked 19 genes are directly involved in the complement cascade (C1QB, C1QC, C3, C4A, C4B, CFH); another third is involved in cholesterol synthesis and transport (ABCA1, ABCB1, HMGCR, LDLR, MSR1, NPC2); A2M disrupts inflammatory cascades but also is involved in the lipoprotein metabolism; BACE2, CTSB, and PSEN are involved in the proteolytic processing of the amyloid precursor protein; CP is a metalloprotein that binds most of the copper in plasma and is involved in the peroxidation of Fe (II) transferrin to Fe (III) transferrin. PCYOX1 cleaves the thioether bond of prenyl-L-cysteines, and PSRC1 may participate in p53-mediated growth suppression.
Discussion of Methodological Approach
We attempted to limit the data included in this study to those derived from brain tissue whenever possible. For this reason, and because of the generally greater availability of data, we analyzed data in this study mainly from patients in the late stages of their respective NDD. This results in better comparability and less noise within the data but should be considered in the analysis and conclusions drawn from these studies, as effects occurring mainly in early stages of disease progression may not be apparent in this analysis.
It should also be noted that we included only data of studies that were published and, furthermore, available in PubMed, Google Scholar, the GWAS Catalog (Buniello et al., 2019), the GEO database (Barrett et al., 2013), the Expression Atlas (Papatheodorou et al., 2018), PDMethDB (Wang C. et al., 2020) or the EWAS Atlas (Li M. et al., 2019). Therefore, as pointed out in Pan et al. (2005), this introduces some language bias, as our methodology did not include searching for results published in other than the English language in, e.g., local journals, which was shown to influence the strength of p-values between, e.g., Chinese and non-Chinese studies (Pan et al., 2005; Tang, 2005). Also, we did not consider unpublished as well as unsignificant data. However, as our approach was mainly aimed for giving a comprehensive and broad overview and, therefore, mainly focused on those genes that were found as significant in, at least, two experiments, regardless of the exact strength of the actual p-value, we consider this problem of publication bias as a minor one for our approach as the number of overexaggerated and false-positive disease associations should be strongly reduced by this methodology.
For some approaches used in this study, it was necessary to choose between different possible approaches, e.g., for the hallmarks to be used or the definition of hub genes. In addition, the network creation of the louvain algorithm is non-deterministic and, therefore, subject to a random influence in the creation of the networks. To minimize this random influence, we applied the louvain algorithm with the input shuffled 100 times anew for each of the analyzed networks, and then chose the iteration that produced the highest modularity. With this approach, we found that the modularity between the different iterations did not change much; however, taking the best of 100 runs makes the results of the louvain community creation more reliable.
The definition of hallmarks and hub genes involves some flexibility, so we have attempted to define hallmarks in light of previous definitions of hallmarks for neurodegeneration (e.g., Ramanan and Saykin, 2013) and of our actual observed subprocesses in the functional analyses. As there are myriads of centrality measurements for nodes in a network, we took centrality measurements into account that were connected to the number of direct links between a node and other nodes, the mediation role of a node in a network, and to the sum of the shortest paths to all other nodes in the network, respectively (Freeman, 1977, 1978; Freeman et al., 1979). In addition, we chose to include the eigenvector centrality of each node (Newman, 2010; Bonacich, 2015), which gives a centrality score to each node that is proportional to the sum of the centrality scores of each node's neighbors to cover some varieties of ways for defining centrality of a node in a network.
Conclusion
The results of this study present a comprehensive picture drawn from the results of the analysis of 234 studies across four neurodegenerative disorders and four omics layers. Roughly summarizing, the 69 low-level terms we chose to categorize into 6 hallmarks show a significantly different distribution across the different omics layers, but not across the four diseases, which might be due to the inclusion of mainly late-stage disease data for broadening the database. The number of genes in the intersection of two diseases and the correlation of their regulatory direction was mainly distinctive between the transcriptomic and proteomic levels.
However, the possibilities for further analysis arising from this study are, at least, as important as the results just mentioned. For example, the communities found, and the processes associated with them can be compared with regard to the direction of regulation of the communities, the presence and absence of processes at certain levels/diseases, or, also, with regard to the hub genes found, as we have already exemplified with three examples in the discussion. Consequently, we not only provide an overview—the big picture of neurodegeneration—but also many new starting points for further research going deeper into individual communities or even genes within these communities. We achieve this by providing comprehensive illustrations as well as all relevant tables for exploring specific communities and their associated genes. Ultimately, identifying the hub genes and the other genes involved in the different clusters may make it easier to develop therapeutics by both getting a better idea of which protein can be targeted in a chosen process but, at the same time, whether too many other important processes could be disturbed by the potential inhibition or amplification.
Data Availability Statement
The original contributions presented in the study are included in the article/Supplementary Materials, further inquiries can be directed to the corresponding author.
Author Contributions
NR and SK wrote the manuscript, designed the research question, performed the analysis, wrote code, interpreted the data, and visualized the results. SS and RH contributed to writing, interpreting the data, and editing the manuscript. SG conceptualized the research, contributed to writing and interpretation, edited the manuscript, supervised the study, and acquired the funding. All authors have read and agreed to the published version of the manuscript. All authors contributed to the article and approved the submitted version.
Funding
This research was partially funded by the ReALity initiative—Resilience, Adaptation, and Longevity (SG and SS). NR and RH acknowledge funding of the Leibniz Institute for Resilience Research (LIR) gGmbH and the IDSAIR initiative.
Conflict of Interest
The authors declare that the research was conducted in the absence of any commercial or financial relationships that could be construed as a potential conflict of interest.
Publisher's Note
All claims expressed in this article are solely those of the authors and do not necessarily represent those of their affiliated organizations, or those of the publisher, the editors and the reviewers. Any product that may be evaluated in this article, or claim that may be made by its manufacturer, is not guaranteed or endorsed by the publisher.
Supplementary Material
The Supplementary Material for this article can be found online at: https://www.frontiersin.org/articles/10.3389/fnagi.2022.866886/full#supplementary-material
References
Abraham, R., Moskvina, V., Sims, R., Hollingworth, P., Morgan, A., Georgieva, L., et al. (2008). A genome-wide association study for late-onset Alzheimer's disease using DNA pooling. BMC Med. Genom. 1, 44. doi: 10.1186/1755-8794-1-44
Akira, S., Uematsu, S., and Takeuchi, O. (2006). Pathogen recognition and innate immunity. Cell 124, 783–801. doi: 10.1016/J.CELL.2006.02.015
Al-Dalahmah, O., Sosunov, A. A., Shaik, A., Ofori, K., Liu, Y., Vonsattel, J. P., et al. (2020). Single-nucleus RNA-Seq identifies huntington disease astrocyte states. Acta Neuropathol. Commun. 8, 1–21. doi: 10.1186/s40478-020-0880-6
Alfradique-Dunham, I., Al-Ouran, R., von Coelln, R., Blauwendraat, C., Hill, E., Luo, L., et al. (2021). Genome-wide association study meta-analysis for parkinson disease motor subtypes. Neurol Genet. 7, e557. doi: 10.1212/NXG.0000000000000557
Altuna, M., Urdánoz-Casado, A., Sánchez-Ruiz De Gordoa, J., Zelaya, M. v., Labarga, A., Lepesant, J. M. J., et al. (2019). DNA methylation signature of human hippocampus in Alzheimer's disease is linked to neurogenesis. Clin. Epigenet. 11, 1–16. doi: 10.1186/S13148-019-0672-7
Ananthakrishnan, R., and Ehrlicher, A. (2007). The forces behind cell movement. Int. J. Biol. Sci. 3, 303. doi: 10.7150/IJBS.3.303
Antúnez, C., Boada, M., González-Pérez, A., Gayán, J., Ramírez-Lorca, R., Marín, J., et al. (2011). The membrane-spanning 4-domains, subfamily A (MS4A) gene cluster contains a common variant associated with Alzheimer's disease. Genome Med. 3, 33. doi: 10.1186/gm249
Ardiles, A. O., Grabrucker, A. M., Scholl, F. G., Rudenko, G., and Borsello, T. (2017). Molecular and cellular mechanisms of synaptopathies. Neural Plast [Preprint]. doi: 10.1155/2017/2643943
Armada-Moreira, A., Gomes, J. I., Pina, C. C., Savchak, O. K., Gonçalves-Ribeiro, J., Rei, N., et al. (2020). Going the extra (synaptic) mile: excitotoxicity as the road toward neurodegenerative diseases. Front. Cell. Neurosci. 14, 90. doi: 10.3389/FNCEL.2020.00090/BIBTEX
Armstrong, M. J., and Okun, M. S. (2020). Diagnosis and treatment of Parkinson disease: a review. JAMA 323, 548–560. doi: 10.1001/JAMA.2019.22360
Attwell, D., and Laughlin, S. B. (2001). An energy budget for signaling in the grey matter of the brain. J. Cereb. Blood Flow Metab. 21, 1133–1145. doi: 10.1097/00004647-200110000-00001
Bader, J. M., Geyer, P. E., Müller, J. B., Strauss, M. T., Koch, M., Leypoldt, F., et al. (2020). Proteome profiling in cerebrospinal fluid reveals novel biomarkers of Alzheimer's disease. Mol. Syst. Biol. 16, e9356. doi: 10.15252/msb.20199356
Bakulski, K. M., Dolinoy, D. C., Sartor, M. A., Paulson, H. L., Konen, J. R., Lieberman, A. P., et al. (2012). Genome-wide DNA methylation differences between late-onset Alzheimer's disease and cognitively normal controls in human frontal cortex. J. Alzheimer's Dis. 29, 571–588. doi: 10.3233/JAD-2012-111223
Bandres-Ciga, S., Ahmed, S., Sabir, M. S., Blauwendraat, C., Adarmes-Gómez, A. D., Bernal-Bernal, I., et al. (2019). The genetic architecture of Parkinson disease in Spain: characterizing population-specific risk, differential haplotype structures, and providing etiologic insight. Mov Disord. 34, 1851–1863. doi: 10.1002/mds.27864
Barber, C. N., and Raben, D. M. (2019). Lipid metabolism crosstalk in the brain: glia and neurons. Front. Cell. Neurosci. 13, 212. doi: 10.3389/FNCEL.2019.00212/BIBTEX
Barbier, P., Zejneli, O., Martinho, M., Lasorsa, A., Belle, V., Smet-Nocca, C., et al. (2019). Role of tau as a microtubule-associated protein: structural and functional aspects. Front. Aging Neurosci. 11, 204. doi: 10.3389/FNAGI.2019.00204
Barrett, T., Wilhite, S. E., Ledoux, P., Evangelista, C., Kim, I. F., Tomashevsky, M., et al. (2013). NCBI GEO: archive for functional genomics data sets - update. Nucleic Acids Res. 41, D991–D995. doi: 10.1093/nar/gks1193
Bateman, A., Martin, M. J., Orchard, S., Magrane, M., Agivetova, R., Ahmad, S., et al. (2021). UniProt: the universal protein knowledgebase in 2021. Nucleic Acids Res. 49, D480–D489. doi: 10.1093/NAR/GKAA1100
Beecham, G. W., Dickson, D. W., Scott, W. K., Martin, E. R., Schellenberg, G., Nuytemans, K., et al. (2015). PARK10 is a major locus for sporadic neuropathologically confirmed Parkinson disease. Neurology 84, 972–980. doi: 10.1212/WNL.0000000000001332
Beecham, G. W., Martin, E. R., Li, Y. J., Slifer, M. A., Gilbert, J. R., Haines, J. L., et al. (2009). Genome-wide association study implicates a chromosome 12 risk locus for late-onset Alzheimer disease. Am. J. Hum. Genet. 84, 35–43. doi: 10.1016/J.AJHG.2008.12.008
Benyamin, B., He, J., Zhao, Q., Gratten, J., Garton, F., Leo, P. J., et al. (2017). Cross-ethnic meta-analysis identifies association of the GPX3-TNIP1 locus with amyotrophic lateral sclerosis. Nat. Commun. 8, 1–7. doi: 10.1038/S41467-017-00471-1
Berchtold, N. C., Coleman, P. D., Cribbs, D. H., Rogers, J., Gillen, D. L., and Cotman, C. W. (2013). Synaptic genes are extensively downregulated across multiple brain regions in normal human aging and Alzheimer's disease. Neurobiol. Aging 34, 1653–1661. doi: 10.1016/j.neurobiolaging.2012.11.024
Berson, A., Nativio, R., Berger, S. L., and Bonini, N. M. (2018). Epigenetic regulation in neurodegenerative diseases. Trends Neurosci. 41, 587–598. doi: 10.1016/J.TINS.2018.05.005
Bertram, L., Lange, C., Mullin, K., Parkinson, M., Hsiao, M., Hogan, M. F., et al. (2008). Genome-wide association analysis reveals putative Alzheimer's disease susceptibility loci in addition to APOE. Am. J. Hum. Genet. 83, 623–632. doi: 10.1016/j.ajhg.2008.10.008
Biernacka, J. M., Chung, S. J., Armasu, S. M., Anderson, K. S., Lill, C. M., Bertram, L., et al. (2016). Genome-wide gene-environment interaction analysis of pesticide exposure and risk of Parkinson's disease. Parkinsonism Related Disord. 32, 25–30. doi: 10.1016/j.parkreldis.2016.08.002
Bjornevik, K., O'Reilly, É. J., Berry, J. D., Clish, C. B., Jeanfavre, S., Kato, I., et al. (2019). Prediagnostic plasma branched-chain amino acids and the risk of amyotrophic lateral sclerosis. Neurology 92, e2081–e2088. doi: 10.1212/WNL.0000000000006669
Blackburn, D., Sargsyan, S., Monk, P. N., and Shaw, P. J. (2009). Astrocyte function and role in motor neuron disease: a future therapeutic target? Glia 57, 1251–1264. doi: 10.1002/GLIA.20848
Blalock, E. M., Buechel, H. M., Popovic, J., Geddes, J. W., and Landfield, P. W. (2011). Microarray analyses of laser-captured hippocampus reveal distinct gray and white matter signatures associated with incipient Alzheimer's disease. J. Chem. Neuroanat. 42, 118–126. doi: 10.1016/j.jchemneu.2011.06.007
Blalock, E. M., Geddes, J. W., Chen, K. C., Porter, N. M., Markesbery, W. R., and Landfield, P. W. (2004). Incipient Alzheimer's disease: microarray correlation analyses reveal major transcriptional and tumor suppressor responses. Proc. Natl. Acad. Sci. U.S.A. 101, 2173–2178. doi: 10.1073/pnas.0308512100
Blauwendraat, C., Heilbron, K., Vallerga, C. L., Bandres-Ciga, S., von Coelln, R., Pihlstrøm, L., et al. (2019). Parkinson's disease age at onset genome-wide association study: defining heritability, genetic loci, and A-synuclein mechanisms. Mov. Disord. 34, 866–875. doi: 10.1002/mds.27659
Blauwendraat, C., Reed, X., Krohn, L., Heilbron, K., Bandres-Ciga, S., Tan, M., et al. (2020). Genetic modifiers of risk and age at onset in GBA associated Parkinson's disease and lewy body dementia. Brain 143, 234–248. doi: 10.1093/brain/awz350
Blondel, V. D., Guillaume, J. L., Lambiotte, R., and Lefebvre, E. (2008). Fast unfolding of communities in large networks. J. Statistical Mech. Theory Exp. 2008, P10008. doi: 10.1088/1742-5468/2008/10/p10008
Blum, M., Chang, H. Y., Chuguransky, S., Grego, T., Kandasaamy, S., Mitchell, A., et al. (2021). The interpro protein families and domains database: 20 years on. Nucleic Acids Res. 49, D344–D354. doi: 10.1093/NAR/GKAA977
Bonacich, P. (2015). Power and centrality: a family of measures. Am. J. Sociol. 92, 1170–1182. doi: 10.1086/228631
Bone, W. P., Siewert, K. M., Jha, A., Klarin, D., Damrauer, S. M., Chang, K. M., et al. (2021). Multi-trait association studies discover pleiotropic loci between Alzheimer's disease and cardiometabolic traits. Alzheimers. Res. Ther. 13, 34. doi: 10.1186/S13195-021-00773-Z
Bonnans, C., Chou, J., and Werb, Z. (2014). Remodelling the extracellular matrix in development and disease. Nat. Rev. Mol. Cell Biol. 15, 786. doi: 10.1038/NRM3904
Bonneh-Barkay, D., and Wiley, C. A. (2009). Brain extracellular matrix in neurodegeneration. Brain Pathol. 19, 573. doi: 10.1111/J.1750-3639.2008.00195.X
Braschi, B., Denny, P., Gray, K., Jones, T., Seal, R., Tweedie, S., et al. (2019). Genenames.Org: the HGNC and VGNC resources in 2019. Nucleic Acids Res. 47, D786–D792. doi: 10.1093/nar/gky930
Briston, T., and Hicks, A. R. (2018). Mitochondrial dysfunction and neurodegenerative proteinopathies: mechanisms and prospects for therapeutic intervention. Biochem. Soc. Trans. 46, 829–842. doi: 10.1042/BST20180025
Buniello, A., Macarthur, J. A. L., Cerezo, M., Harris, L. W., Hayhurst, J., Malangone, C., et al. (2019). The NHGRI-EBI GWAS catalog of published genome-wide association studies, targeted arrays and summary statistics 2019. Nucleic Acids Res. 47, D1005–D1012. doi: 10.1093/NAR/GKY1120
Cabral-Miranda, F., and Hetz, C. (2018). “ER stress and neurodegenerative disease: a cause or effect relationship?,” in Current Topics in Microbiology and Immunology, Vol. 414, eds R. L. Wiseman and C. M. Haynes (Heidelberg: Springer Verlag), 131–157.
Calligaris, R., Banica, M., Roncaglia, P., Robotti, E., Finaurini, S., Vlachouli, C., et al. (2015). Blood transcriptomics of drug-naïve sporadic Parkinson's disease patients. BMC Genom. 16, 1–14. doi: 10.1186/s12864-015-2058-3
Carbon, S., Douglass, E., Good, B. M., Unni, D. R., Harris, N. L., Mungall, C. J., et al. (2021). The gene ontology resource: enriching a gold mine. Nucleic Acids Res. 49, D325–D334. doi: 10.1093/NAR/GKAA1113
Carpanini, S. M., Torvell, M., and Morgan, B. P. (2019). Therapeutic inhibition of the complement system in diseases of the central nervous system. Front. Immunol. 10, 362. doi: 10.3389/FIMMU.2019.00362/BIBTEX
Carrasquillo, M. M., Zou, F., Pankratz, V. S., Wilcox, S. L., Ma, L., Walker, L. P., et al. (2009). Genetic variation in PCDH11X is associated with susceptibility to late-onset Alzheimer's disease. Nat. Genet. 41, 192–198. doi: 10.1038/ng.305
Carroll, P. A., Freie, B. W., Mathsyaraja, H., and Eisenman, R. N. (2018). The MYC transcription factor network: balancing metabolism, proliferation and oncogenesis. Front. Med. 12, 412–425. doi: 10.1007/S11684-018-0650-Z
Carter, C. J. (2010). APP, APOE, complement receptor 1, clusterin and picalm and their involvement in the herpes simplex life cycle. Neurosci. Lett. 483, 96–100. doi: 10.1016/J.NEULET.2010.07.066
Cha, P. C., Satake, W., Ando-Kanagawa, Y., Yamamoto, K., Murata, M., and Toda, T. (2020). Genome-wide association study identifies zonisamide responsive gene in Parkinson's disease patients. J. Hum. Genet. 65, 693–704. doi: 10.1038/S10038-020-0760-8
Chang, D., Nalls, M. A., Hallgrímsdóttir, I. B., Hunkapiller, J., Brug, M., van der Cai, F., et al. (2017). A meta-analysis of genome-wide association studies identifies 17 new Parkinson's disease risk loci. Nat. Genet. 49, 1511–1516. doi: 10.1038/ng.3955
Chao, M. J., Kim, K.-H., Shin, J. W., Lucente, D., Wheeler, V. C., Li, H., et al. (2018). Population-specific genetic modification of Huntington's disease in venezuela. PLoS Genet. 14, e1007274. doi: 10.1371/journal.pgen.1007274
Chaplot, K., Jarvela, T. S., and Lindberg, I. (2020). Secreted chaperones in neurodegeneration. Front. Aging Neurosci. 12, 268. doi: 10.3389/FNAGI.2020.00268/BIBTEX
Chen, C. J., Chen, C. M., Pai, T. W., Chang, H. T., and Hwang, C. S. (2016). A genome-wide association study on amyotrophic lateral sclerosis in the Taiwanese han population. Biomark. Med. 10, 597–611. doi: 10.2217/bmm.15.115
Chen, Q., Kang, J., and Fu, C. (2018). The independence of and associations among apoptosis, autophagy, and necrosis. Signal Transduction Targeted Ther. 3, 1–11. doi: 10.1038/s41392-018-0018-5
Chung, J., Wang, X., Maruyama, T., Ma, Y., Zhang, X., Mez, J., et al. (2018). Genome-wide association study of Alzheimer's disease endophenotypes at prediagnosis stages. Alzheimer's Dement. 14, 623–633. doi: 10.1016/j.jalz.2017.11.006
Chung, S. J., Armasu, S. M., Biernacka, J. M., Anderson, K. J., Lesnick, T. G., Rider, D. N., et al. (2012). Genomic determinants of motor and cognitive outcomes in Parkinson's disease. Parkinsonism Related Disord. 18, 881–886. doi: 10.1016/j.parkreldis.2012.04.025
Chursov, A., Kopetzky, S. J., Bocharov, G., Frishman, D., and Shneider, A. (2013). RNAtips: analysis of temperature-induced changes of RNA secondary structure. Nucleic Acids Res. 41, W486. doi: 10.1093/nar/gkt486
Collins, M. A., An, J., Hood, B. L., Conrads, T. P., and Bowser, R. P. (2015). Label-free LC-MS/MS proteomic analysis of cerebrospinal fluid identifies protein/pathway alterations and candidate biomarkers for amyotrophic lateral sclerosis. J. Proteome Res. 14, 4486–4501. doi: 10.1021/acs.jproteome.5b00804
Conroy, L. R., Hawkinson, T. R., Young, L. E. A., Gentry, M. S., and Sun, R. C. (2021). Emerging roles of N-linked glycosylation in brain physiology and disorders. Trends Endocrinol. Metab. 32, 980–993. doi: 10.1016/J.TEM.2021.09.006
Coon, K. D., Myers, A. J., Craig, D. W., Webster, J. A., Pearson, J. V., Lince, D. H., et al. (2007). A high-density whole-genome association study reveals that APOE is the major susceptibility gene for sporadic late-onset Alzheimer's disease. J. Clin. Psychiatry 68, 613–618. doi: 10.4088/JCP.v68n0419
Cooper, G. M. (2000). Actin, Myosin, and Cell Movement - The Cell - NCBI Bookshelf. Available online: https://www.ncbi.nlm.nih.gov/books/NBK9961/ (accessed March 25, 2022).
Coppedè, F., and Migliore, L. (2015). DNA damage in neurodegenerative diseases. Mutat. Res. 776, 84–97. doi: 10.1016/J.MRFMMM.2014.11.010
Cox, L. E., Ferraiuolo, L., Goodall, E. F., Heath, P. R., Higginbottom, A., Mortiboys, H., et al. (2010). Mutations in CHMP2B in lower motor neuron predominant amyotrophic lateral sclerosis (ALS). PLoS ONE 5, e9872. doi: 10.1371/journal.pone.0009872
Cramer, P. E., Cirrito, J. R., Wesson, D. W., Lee, C. Y. D., Karlo, J. C., Zinn, A. E., et al. (2012). ApoE-directed therapeutics rapidly clear β-amyloid and reverse deficits in AD mouse models. Science 335, 1503–1506. doi: 10.1126/SCIENCE.1217697/SUPPL_FILE/CRAMER.SOM.V2.PDF
Cronin, S., Berger, S., Ding, J., Schymick, J. C., Washecka, N., Hernandez, D. G., et al. (2008). A genome-wide association study of sporadic ALS in a homogenous Irish population. Hum. Mol. Genet. 17, 768–774. doi: 10.1093/hmg/ddm361
Cronin, S., Tomik, B., Bradley, D. G., Slowik, A., and Hardiman, O. (2009). Screening for replication of genome-wide SNP associations in sporadic ALS. Euro. J. Human Genet. 17, 213–218. doi: 10.1038/ejhg.2008.194
Cruchaga, C., Kauwe, J. S. K., Harari, O., Jin, S. C., Cai, Y., Karch, C. M., et al. (2013). GWAS of cerebrospinal fluid tau levels identifies risk variants for Alzheimer's disease. Neuron 78, 256–268. doi: 10.1016/j.neuron.2013.02.026
Cummings, A. C., Jiang, L., Velez Edwards, D. R., McCauley, J. L., Laux, R., McFarland, L. L., et al. (2012). Genome-wide association and linkage study in the amish detects a novel candidate late-onset Alzheimer disease gene. Ann. Hum. Genet. 76, 342–351. doi: 10.1111/j.1469-1809.2012.00721.x
Dai, W., Yang, J., Chen, T., and Yang, Z. (2014). Protective effects of bexarotene against amyloid-B25-35-induced dysfunction in hippocampal neurons through the insulin signaling pathway. Neurodegener. Dis. 14, 77–84. doi: 10.1159/000358397
Danial, N. N., and Korsmeyer, S. J. (2004). Cell death: critical control points. Cell 116, 205–219. doi: 10.1016/S0092-8674(04)00046-7
Davis, M. F., Cummings, A. C., D'Aoust, L. N., Jiang, L., Velez Edwards, D. R., Laux, R., et al. (2013). Parkinson disease loci in the mid-western amish. Hum. Genet. 132, 1213–1221. doi: 10.1007/s00439-013-1316-1
de Jager, P. L., Srivastava, G., Lunnon, K., Burgess, J., Schalkwyk, L. C., Yu, L., et al. (2014). Alzheimer's disease: early alterations in brain DNA methylation at ANK1, BIN1, RHBDF2 and other loci. Nat. Neurosci. 17, 1156–1163. doi: 10.1038/NN.3786
de Rojas, I., Moreno-Grau, S., Tesi, N., Grenier-Boley, B., Andrade, V., Jansen, I. E., et al. (2021). Common variants in Alzheimer's disease and risk stratification by polygenic risk scores. Nat. Commun. 12, 1–16. doi: 10.1038/S41467-021-22491-8
De Sousa Abreu, R., Penalva, L. O., Marcotte, E. M., and Vogel, C. (2009). Global signatures of protein and MRNA expression levels. Mol. Biosyst. 5, 1512–1526. doi: 10.1039/b908315d
Dean, R. A., and Shaw, L. M. (2010). Use of cerebrospinal fluid biomarkers for diagnosis of incipient Alzheimer disease in patients with mild cognitive impairment. Clin. Chem. 56, 7–9. doi: 10.1373/CLINCHEM.2009.134692
Dekker, A. M., Diekstra, F. P., Pulit, S. L., Tazelaar, G. H. P., van der Spek, R. A., van Rheenen, W., et al. (2019). Exome array analysis of rare and low frequency variants in amyotrophic lateral sclerosis. Sci. Rep. 9, 1–8. doi: 10.1038/s41598-019-42091-3
DeMichele-Sweet, M. A. A., Klei, L., Creese, B., Harwood, J. C., Weamer, E. A., McClain, L., et al. (2021). Genome-wide association identifies the first risk loci for psychosis in Alzheimer disease. Mol. Psychiatry, 26, 5797–5811. doi: 10.1038/S41380-021-01152-8
Deming, Y., Xia, J., Cai, Y., Lord, J., Holmans, P., Bertelsen, S., et al. (2016). A potential endophenotype for Alzheimer's disease: cerebrospinal fluid clusterin. Neurobiol. Aging 37, 208.e1–e9. doi: 10.1016/j.neurobiolaging.2015.09.009
Deng, M., Wei, L., Zuo, X., Tian, Y., Xie, F., Hu, P., et al. (2013). Genome-wide association analyses in han chinese identify two new susceptibility loci for amyotrophic lateral sclerosis. Nat. Genet. 45, 697–700. doi: 10.1038/ng.2627
Dib, S., Pahnke, J., and Gosselet, F. (2021). Role of ABCA7 in human health and in Alzheimer's disease. Int. J. Mol. Sci. 22, 4603. doi: 10.3390/IJMS22094603
Diekstra, F. P., Van Deerlin, V. M., van Swieten, J. C., Al-Chalabi, A., Ludolph, A. C., Weishaupt, J. H., et al. (2014). C9orf72 and UNC13A are shared risk loci for amyotrophic lateral sclerosis and frontotemporal dementia: a genome-wide meta-analysis. Ann. Neurol. 76, 120–133. doi: 10.1002/ana.24198
Dijkstra, A. A., Ingrassia, A., De Menezes, R. X., Van Kesteren, R. E., Rozemuller, A. J. M., Heutink, P., et al. (2015). Evidence for immune response, axonal dysfunction and reduced endocytosis in the substantia nigra in early stage Parkinson's disease. PLoS ONE 10, e128651. doi: 10.1371/journal.pone.0128651
Do, C. B., Tung, J. Y., Dorfman, E., Kiefer, A. K., Drabant, E. M., Francke, U., et al. (2011). Web-based genome-wide association study identifies two novel loci and a substantial genetic component for Parkinson's disease. PLoS Genet. 7, e1002141. doi: 10.1371/journal.pgen.1002141
Dols-Icardo, O., Montal, V., Sirisi, S. S., López-pernas, G., Cervera, L., Lopez-Pernas, G., et al. (2020). Characterization of the motor cortex transcriptome supports microgial-related key events in amyotrophic lateral sclerosis. bioRxiv. 2020.02.07.938662. doi: 10.1101/2020.02.07.938662
Dumitriu, A., Golji, J., Labadorf, A. T., Gao, B., Beach, T. G., Myers, R. H., et al. (2016). Integrative analyses of proteomics and RNA transcriptomics implicate mitochondrial processes, protein folding pathways and GWAS loci in Parkinson disease. BMC Med. Genom. 9, 5. doi: 10.1186/s12920-016-0164-y
Dumitriu, A., Latourelle, J. C., Hadzi, T. C., Pankratz, N., Garza, D., Miller, J. P., et al. (2012). Gene expression profiles in Parkinson disease prefrontal cortex implicate FOXO1 and genes under its transcriptional regulation. PLoS Genet. 8, e1002794. doi: 10.1371/journal.pgen.1002794
Dunckley, T., Beach, T. G., Ramsey, K. E., Grover, A., Mastroeni, D., Walker, D. G., et al. (2006). Gene expression correlates of neurofibrillary tangles in Alzheimer's disease. Neurobiol. Aging 27, 1359–1371. doi: 10.1016/j.neurobiolaging.2005.08.013
Dusa, A. (2016). Package “Venn.” Available online at: https://en.wikipedia.org/wiki/Centroid (accessed August 14, 2020).
Edwards, T. L., Scott, W. K., Almonte, C., Burt, A., Powell, E. H., Beecham, G. W., et al. (2010). Genome-wide association study confirms SNPs in SNCA and the MAPT region as common risk factors for Parkinson disease. Ann. Hum. Genet. 74, 97–109. doi: 10.1111/j.1469-1809.2009.00560.x
Elmore, S. (2007). Apoptosis: a review of programmed cell death. Toxicol. Pathol. 35, 495. doi: 10.1080/01926230701320337
Elstner, M., Morris, C. M., Heim, K., Bender, A., Mehta, D., Jaros, E., et al. (2011). Expression analysis of dopaminergic neurons in parkinson's disease and aging links transcriptional dysregulation of energy metabolism to cell death. Acta Neuropathol. 122, 75–86. doi: 10.1007/s00401-011-0828-9
Fabregat, A., Sidiropoulos, K., Garapati, P., Gillespie, M., Hausmann, K., Haw, R., et al. (2016). The reactome pathway knowledgebase. Nucleic Acids Res. 44, D481–D487. doi: 10.1093/NAR/GKV1351
Fan, T.-J., Han, L.-H., Cong, R.-S., and Liang, J. (2005). Caspase family proteases and apoptosis. Acta Biochimica et Biophysica Sinica. 37, 719–727. doi: 10.1111/J.1745-7270.2005.00108.X
Fang, Q., Strand, A., Law, W., Faca, V. M., Fitzgibbon, M. P., Hamel, N., et al. (2009). Brain-specific proteins decline in the cerebrospinal fluid of humans with Huntington disease. Mol. Cell. Proteomics 8, 451–466. doi: 10.1074/mcp.M800231-MCP200
Fernstrom, J. D. (2005). Branched-chain amino acids and brain function. J. Nutr. 135, 1539–1546. doi: 10.1093/JN/135.6.1539S
Feulner, T. M., Laws, S. M., Friedrich, P., Wagenpfeil, S., Wurst, S. H. R., Riehle, C., et al. (2010). Examination of the current top candidate genes for AD in a genome-wide association study. Mol. Psychiatry 15, 756–766. doi: 10.1038/mp.2008.141
Feyeux, M., Bourgois-Rocha, F., Redfern, A., Giles, P., Lefort, N., Aubert, S., et al. (2012). Early transcriptional changes linked to naturally occurring Huntington's disease mutations in neural derivatives of human embryonic stem cells. Hum. Mol. Genet. 21, 3883–3895. doi: 10.1093/hmg/dds216
Fire, A., Xu, S., Montgomery, M. K., Kostas, S. A., Driver, S. E., and Mello, C. C. (1998). Potent and specific genetic interference by double-stranded RNA in caenorhabditis elegans. Nature 391, 806–811. doi: 10.1038/35888
Fletcher, D. A., and Mullins, R. D. (2010). Cell mechanics and the cytoskeleton. Nature 463, 485. doi: 10.1038/NATURE08908
Fogarty, M. J., Kanjhan, R., Bellingham, M. C., and Noakes, P. G. (2016). Glycinergic neurotransmission: a potent regulator of embryonic motor neuron dendritic morphology and synaptic plasticity. J. Neurosci. 36, 80. doi: 10.1523/JNEUROSCI.1576-15.2016
Fogh, I., Lin, K., Tiloca, C., Rooney, J., Gellera, C., Diekstra, F. P., et al. (2016). Association of a locus in the CAMTA1 gene with survival in patients with sporadic amyotrophic lateral sclerosis. JAMA Neurol. 73, 812–820. doi: 10.1001/JAMANEUROL.2016.1114
Fogh, I., Ratti, A., Gellera, C., Lin, K., Tiloca, C., Moskvina, V., et al. (2014). A genome-wide association meta-analysis identifies a novel locus at 17q11.2 associated with sporadic amyotrophic lateral sclerosis. Hum. Mol. Genet. 23, 2220–2231. doi: 10.1093/HMG/DDT587
Foo, J. N., Chew, E. G. Y., Chung, S. J., Peng, R., Blauwendraat, C., Nalls, M. A., et al. (2020). Identification of risk loci for parkinson disease in asians and comparison of risk between asians and europeans: a genome-wide association study. JAMA Neurol. 77, 746–754. doi: 10.1001/JAMANEUROL.2020.0428
Foo, J. N., Tan, L. C., Irwan, I. D., Au, W. L., Low, H. Q., Prakash, K. M., et al. (2017). Genome-wide association study of Parkinson's disease in east asians. Hum. Mol. Genet. 26, 226–232. doi: 10.1093/hmg/ddw379
Frade, J. M., and Ovejero-Benito, M. C. (2015). Neuronal cell cycle: the neuron itself and its circumstances. Cell Cycle 14, 712. doi: 10.1080/15384101.2015.1004937
Franco-Iborra, S., Vila, M., and Perier, C. (2018). Mitochondrial quality control in neurodegenerative diseases: focus on Parkinson's disease and Huntington's disease. Front. Neurosci. 12, 342. doi: 10.3389/FNINS.2018.00342/BIBTEX
Frank-Cannon, T. C., Alto, L. T., McAlpine, F. E., and Tansey, M. G. (2009). Does neuroinflammation fan the flame in neurodegenerative diseases? Mol. Neurodegener. 4, 47. doi: 10.1186/1750-1326-4-47
Freeman, L. C. (1977). A set of measures of centrality based on betweenness. Sociometry 40, 35. doi: 10.2307/3033543
Freeman, L. C. (1978). Elsevier Sequoia S.A., Lausanne-Printed in the Netherlands. Soc. Netw. 1, 35–41.
Freeman, L. C., Roeder, D., and Mulholland, R. R. (1979). Centrality in social networks: II. Experimental results. Soc. Netw. 2, 119–141. doi: 10.1016/0378-8733(79)90002-9
Fulda, S., Gorman, A. M., Hori, O., and Samali, A. (2010). Cellular stress responses: cell survival and cell death. Int. J. Cell Biol. 2010. doi: 10.1155/2010/214074
Fung, H. C., Scholz, S., Matarin, M., Simón-Sánchez, J., Hernandez, D., Britton, A., et al. (2006). Genome-wide genotyping in Parkinson's disease and neurologically normal controls: first stage analysis and public release of data. Lancet Neurol. 5, 911–916. doi: 10.1016/S1474-4422(06)70578-6
Furney, S. J., Simmons, A., Breen, G., Pedroso, I., Lunnon, K., Proitsi, P., et al. (2011). Genome-wide association with MRI atrophy measures as a quantitative trait locus for Alzheimer's disease. Mol. Psychiatry 16, 1130–1138. doi: 10.1038/mp.2010.123
Gagliardi, S., Zucca, S., Pandini, C., Diamanti, L., Bordoni, M., Sproviero, D., et al. (2018). Long non-coding and coding RNAs characterization in peripheral blood mononuclear cells and spinal cord from amyotrophic lateral sclerosis patients. Sci. Rep. 8, 1–11. doi: 10.1038/s41598-018-20679-5
Gan, L., Cookson, M. R., Petrucelli, L., and la Spada, A. R. (2018). Converging pathways in neurodegeneration, from genetics to mechanisms. Nat. Neurosci. 21, 1300–1309. doi: 10.1038/s41593-018-0237-7
Gasparoni, G., Bultmann, S., Lutsik, P., Kraus, T. F. J., Sordon, S., Vlcek, J., et al. (2018). DNA methylation analysis on purified neurons and glia dissects age and Alzheimer's disease-specific changes in the human cortex. Epigenetics Chromatin. 11, 1–19. doi: 10.1186/S13072-018-0211-3
Geiger, B., and Bershadsky, A. (2002). Exploring the neighborhood: adhesion-coupled cell mechanosensors. Cell 110, 139–142. doi: 10.1016/S0092-8674(02)00831-0
Gendelman, H. E. (2002). Neural immunity: friend or foe? J. Neurovirol. 8, 474–479. doi: 10.1080/13550280290168631
Glickman, M. H., and Ciechanover, A. (2002). The ubiquitin-proteasome proteolytic pathway: destruction for the sake of construction. Physiol. Rev. 82, 373–428. doi: 10.1152/physrev.00027.2001
Go, R. C. P., Corley, M. J., Ross, G. W., Petrovitch, H., Masaki, K. H., Maunakea, A. K., et al. (2020). Genome-wide epigenetic analyses in Japanese immigrant plantation workers with Parkinson's disease and exposure to organochlorines reveal possible involvement of glial genes and pathways involved in neurotoxicity. BMC Neurosci. 21, 1–18. doi: 10.1186/S12868-020-00582-4
Godin, J. D., and Humbert, S. (2011). Mitotic spindle: focus on the function of huntingtin. Int. J. Biochem. Cell Biol. 43, 852–856. doi: 10.1016/J.BIOCEL.2011.03.009
Goris, A., van Setten, J., Diekstra, F., Ripke, S., Patsopoulos, N. A., Sawcer, S. J., et al. (2014). No evidence for shared genetic basis of common variants in multiple sclerosis and amyotrophic lateral sclerosis. Hum. Mol. Genet. 23, 1916–1922. doi: 10.1093/hmg/ddt574
Guo, W., StoklundDittlau, K., and van den Bosch, L. (2020). Axonal transport defects and neurodegeneration: molecular mechanisms and therapeutic implications. Semin. Cell Dev. Biol. 99, 133–150. doi: 10.1016/J.SEMCDB.2019.07.010
Gusareva, E. S., Twizere, J. C., Sleegers, K., Dourlen, P., Abisambra, J. F., Meier, S., et al. (2018). Male-specific epistasis between WWC1 and TLN2 genes is associated with Alzheimer's disease. Neurobiol. Aging 72, 188.e3–e12. doi: 10.1016/j.neurobiolaging.2018.08.001
Guzman-Martinez, L., Maccioni, R. B., Andrade, V., Navarrete, L. P., Pastor, M. G., and Ramos-Escobar, N. (2019). Neuroinflammation as a common feature of neurodegenerative disorders. Front. Pharmacol. 10, 1008. doi: 10.3389/FPHAR.2019.01008/BIBTEX
Haan, M. N. (2006). Therapy insight: type 2 diabetes mellitus and the risk of late-onset Alzheimer's disease. Nat. Clin. Prac. Neurol. 2, 159–166. doi: 10.1038/ncpneuro0124
Hagberg, A., Swart, P., and Chult, D. (2008). Exploring Network Structure, Dynamics, and Function Using NetworkX. Available online at: https://www.osti.gov/biblio/960616 (accessed December 14, 2021).
Hamza, T. H., Zabetian, C. P., Tenesa, A., Laederach, A., Montimurro, J., Yearout, D., et al. (2010). Common genetic variation in the HLA region is associated with late-onset sporadic Parkinson's disease. Nat. Genet. 42, 781. doi: 10.1038/ng.642
Harding, H. P., Zhang, Y., Zeng, H., Novoa, I., Lu, P. D., Calfon, M., et al. (2003). An integrated stress response regulates amino acid metabolism and resistance to oxidative stress. Mol. Cell 11, 619–633. doi: 10.1016/S1097-2765(03)00105-9
Harold, D., Abraham, R., Hollingworth, P., Sims, R., Gerrish, A., Hamshere, M. L., et al. (2009). Genome-wide association study identifies variants at CLU and PICALM associated with Alzheimer's disease. Nat. Genet. 41, 1088–1093. doi: 10.1038/ng.440
Harry, G. J., and Kraft, A. D. (2008). Neuroinflammation and microglia: considerations and approaches for neurotoxicity assessment. Expert Opin. Drug Metab. Toxicol. 4, 1265. doi: 10.1517/17425255.4.10.1265
Hartl, F. U., Bracher, A., and Hayer-Hartl, M. (2011). Molecular chaperones in protein folding and proteostasis. Nature 475, 324–332. doi: 10.1038/nature10317
Heinzen, E. L., Need, A. C., Hayden, K. M., Chiba-Falek, O., Roses, A. D., Strittmatter, W. J., et al. (2010). Genome-wide scan of copy number variation in late-onset Alzheimer's disease. J. Alzheimer's Dis. 19, 69–77. doi: 10.3233/JAD-2010-1212
Helfand, B. T., Mendez, M. G., Pugh, J., Delsert, C., and Goldman, R. D. (2003). A role for intermediate filaments in determining and maintaining the shape of nerve cells. Mol. Biol. Cell 14, 5069. doi: 10.1091/MBC.E03-06-0376
Heras-Sandoval, D., Pérez-Rojas, J. M., and Pedraza-Chaverri, J. (2020). Novel compounds for the modulation of MTOR and autophagy to treat neurodegenerative diseases. Cell. Signal. 65, 109442. doi: 10.1016/J.CELLSIG.2019.109442
Herold, C., Hooli, B. V., Mullin, K., Liu, T., Roehr, J. T., Mattheisen, M., et al. (2016). Family-based association analyses of imputed genotypes reveal genome-wide significant association of Alzheimer's disease with OSBPL6, PTPRG, and PDCL3. Mol. Psychiatry 21, 1608–1612. doi: 10.1038/mp.2015.218
Herrmann, H., Bär, H., Kreplak, L., Strelkov, S. v., and Aebi, U. (2007). Intermediate filaments: from cell architecture to nanomechanics. Nat. Rev. Mol. Cell Biol. 8, 562–573. doi: 10.1038/nrm2197
Herrmann, H., Strelkov, S. v., Burkhard, P., and Aebi, U. (2009). Intermediate filaments: primary determinants of cell architecture and plasticity. J. Clin. Investig. 119, 1772–1783. doi: 10.1172/JCI38214
Higginbotham, L., Dammer, E., Duong, D., Modeste, E., Montine, T., Lah, J., et al. (2019). Network analysis of a membrane-enriched brain proteome across stages of Alzheimer's disease. Proteomes 7, 30. doi: 10.3390/proteomes7030030
Higginbotham, L., Ping, L., Dammer, E. B., Duong, D. M., Zhou, M., Gearing, M., et al. (2020). Integrated proteomics reveals brain-based cerebrospinal fluid biomarkers in asymptomatic and symptomatic Alzheimer's disease. Sci. Adv. 6, eaaz9360. doi: 10.1126/sciadv.aaz9360
Hill-Burns, E. M., Ross, O. A., Wissemann, W. T., Soto-Ortolaza, A. I., Zareparsi, S., Siuda, J., et al. (2016). Identification of genetic modifiers of age-at-onset for familial Parkinson's disease. Hum. Mol. Genet. 25, 3849–3862. doi: 10.1093/hmg/ddw206
Hill-Burns, E. M., Wissemann, W. T., Hamza, T. H., Factor, S. A., Zabetian, C. P., and Payami, H. (2014). Identification of a novel Parkinson's disease locus via stratified genome-wide association study. BMC Genom. 15, 118. doi: 10.1186/1471-2164-15-118
Hirano, A., Ohara, T., Takahashi, A., Aoki, M., Fuyuno, Y., Ashikawa, K., et al. (2015). A genome-wide association study of late-onset Alzheimer's disease in a Japanese population. Psychiatr. Genet. 25, 139–146. doi: 10.1097/YPG.0000000000000090
Hohmann, T., and Dehghani, F. (2019). The cytoskeleton—a complex interacting meshwork. Cells 8, 362. doi: 10.3390/CELLS8040362
Hokama, M., Oka, S., Leon, J., Ninomiya, T., Honda, H., Sasaki, K., et al. (2014). Altered expression of diabetes-related genes in Alzheimer's disease brains: the hisayama study. Cereb. Cortex 24, 2476–2488. doi: 10.1093/cercor/bht101
Hollingworth, P., Harold, D., Sims, R., Gerrish, A., Lambert, J. C., Carrasquillo, M. M., et al. (2011). Common variants at ABCA7, MS4A6A/MS4A4E, EPHA1, CD33 and CD2AP are associated with Alzheimer's disease. Nat. Genet. 43, 429–436. doi: 10.1038/ng.803
Hollingworth, P., Sweet, R., Sims, R., Harold, D., Russo, G., Abraham, R., et al. (2012). Genome-wide association study of Alzheimer's disease with psychotic symptoms. Mol. Psychiatry 17, 1316–1327. doi: 10.1038/mp.2011.125
Hondius, D. C., van Nierop, P., Li, K. W., Hoozemans, J. J. M., van der Schors, R. C., van Haastert, E. S., et al. (2016). Profiling the human hippocampal proteome at all pathologic stages of Alzheimer's disease. Alzheimer Dementia 12, 654–668. doi: 10.1016/j.jalz.2015.11.002
Hong, S., Prokopenko, D., Dobricic, V., Kilpert, F., Bos, I., Vos, S. J. B., et al. (2020). Genome-wide association study of Alzheimer's disease CSF biomarkers in the EMIF-AD multimodal biomarker discovery dataset. Transl. Psychiatry 10, 1–12. doi: 10.1038/S41398-020-01074-Z
Hu, X., Pickering, E., Liu, Y. C., Hall, S., Fournier, H., Katz, E., et al. (2011). Meta-analysis for genome-wide association study identifies multiple variants at the BIN1 locus associated with late-onset Alzheimer's disease. PLoS ONE 6, e16616. doi: 10.1371/journal.pone.0016616
Hu, Y., Deng, L., Zhang, J., Fang, X., Mei, P., Cao, X., et al. (2016). A pooling genome-wide association study combining a pathway analysis for typical sporadic Parkinson's disease in the han population of Chinese Mainland. Mol. Neurobiol. 53, 4302–4318. doi: 10.1007/s12035-015-9331-y
Huang, Z., Xie, N., Illes, P., di Virgilio, F., Ulrich, H., Semyanov, A., et al. (2021). From purines to purinergic signalling: molecular functions and human diseases. Signal Trans. Targeted Ther. 6, 1–20. doi: 10.1038/s41392-021-00553-z
Hunter, J. D. (2007). Matplotlib: a 2D graphics environment. Comput. Sci. Eng. 9, 90–95. doi: 10.1109/MCSE.2007.55
Iatrou, A., Clark, E. M., and Wang, Y. (2021). Nuclear dynamics and stress responses in Alzheimer's disease. Mol. Neurodegener. 16, 1–15. doi: 10.1186/S13024-021-00489-6
Iridoy, M. O., Zubiri, I., Zelaya, M. V., Martinez, L., Ausín, K., Lachen-Montes, M., et al. (2019). Neuroanatomical quantitative proteomics reveals common pathogenic biological routes between amyotrophic lateral sclerosis (ALS) and frontotemporal dementia (FTD). Int. J. Mol. Sci. 20. doi: 10.3390/ijms20010004
Israël, A. (2010). The IKK complex, a central regulator of NF-KB activation. Cold Spring Harb. Perspect. Biol. 2, a000158. doi: 10.1101/CSHPERSPECT.A000158
Jahn, R., and Scheller, R. H. (2006). SNAREs — engines for membrane fusion. Nat. Rev. Mol. Cell Biol. 7, 631–643. doi: 10.1038/nrm2002
Jang, S., Lee, H., and Kim, E. (2017). Synaptic adhesion molecules and excitatory synaptic transmission. Curr. Opin. Neurobiol. 45, 45–50. doi: 10.1016/J.CONB.2017.03.005
Jansen, I. E., Savage, J. E., Watanabe, K., Bryois, J., Williams, D. M., Steinberg, S., et al. (2019). Genome-wide meta-analysis identifies new loci and functional pathways influencing Alzheimer's disease risk. Nat. Genet. 51, 404–413. doi: 10.1038/s41588-018-0311-9
Jha, M. K., Jo, M., Kim, J. H., and Suk, K. (2019). Microglia-astrocyte crosstalk: an intimate molecular conversation. Neuroscientist 25, 227–240. doi: 10.1177/1073858418783959
Jin, W., Qazi, T. J., Quan, Z., Li, N., and Qing, H. (2019). Dysregulation of transcription factors: a key culprit behind neurodegenerative disorders. Neuroscientist 25, 548–565. doi: 10.1177/1073858418811787
Johnson, E. C. B., Dammer, E. B., Duong, D. M., Ping, L., Zhou, M., Yin, L., et al. (2020). Large-scale proteomic analysis of Alzheimer's disease brain and cerebrospinal fluid reveals early changes in energy metabolism associated with microglia and astrocyte activation. Nat. Med. 26, 769–780. doi: 10.1038/s41591-020-0815-6
Jonsson, T., Stefansson, H., Steinberg, S., Jonsdottir, I., Jonsson, P. V., Snaedal, J., et al. (2013). Variant of TREM2 associated with the risk of Alzheimer's disease. N. Engl. J. Med. 368, 107–116. doi: 10.1056/NEJMoa1211103
Joseph, C., Mangani, A. S., Gupta, V., Chitranshi, N., Shen, T., Dheer, Y., et al. (2020). Cell cycle deficits in neurodegenerative disorders: uncovering molecular mechanisms to drive innovative therapeutic development. Aging Dis. 11, 946. doi: 10.14336/AD.2019.0923
Jun, G., Ibrahim-Verbaas, C. A., Vronskaya, M., Lambert, J. C., Chung, J., Naj, A. C., et al. (2016). A novel Alzheimer disease locus located near the gene encoding tau protein. Mol. Psychiatry 21, 108–117. doi: 10.1038/mp.2015.23
Jun, G. R., Chung, J., Logue, M. W., Sherva, R., Farrer, L. A., Mez, J., et al. (2017). Transethnic genome-wide scan identifies novel Alzheimer's disease loci. Alzheimer. Demen. 13, 727–738. doi: 10.1016/j.jalz.2016.12.012
Jurga, A. M., Paleczna, M., and Kuter, K. Z. (2020). Overview of general and discriminating markers of differential microglia phenotypes. Front. Cell. Neurosci. 14, 198. doi: 10.3389/FNCEL.2020.00198/BIBTEX
Kamboh, M. I., Barmada, M. M., Demirci, F. Y., Minster, R. L., Carrasquillo, M. M., Pankratz, V. S., et al. (2012a). Genome-wide association analysis of age-at-onset in Alzheimer's disease. Mol. Psychiatry 17, 1340–1346. doi: 10.1038/mp.2011.135
Kamboh, M. I., Demirci, F. Y., Wang, X., Minster, R. L., Carrasquillo, M. M., Pankratz, V. S., et al. (2012b). Genome-wide association study of Alzheimer's disease. Transl. Psychiatry 2, e117. doi: 10.1038/tp.2012.45
Kanehisa, M., Sato, Y., and Kawashima, M. (2021). KEGG mapping tools for uncovering hidden features in biological data. Protein Sci. 31, 47–53. doi: 10.1002/PRO.4172
Kang, S., Gim, J., Lee, J., Gunasekaran, T. I., Choi, K. Y., Lee, J. J., et al. (2021). Potential novel genes for late-onset Alzheimer's disease in east-asian descent identified by APOE-stratified genome-wide association study. J. Alzheimers. Dis. 82, 1451–1460. doi: 10.3233/JAD-210145
Kapeli, K., Pratt, G. A., Vu, A. Q., Hutt, K. R., Martinez, F. J., Sundararaman, B., et al. (2016). Distinct and shared functions of ALS-associated proteins TDP-43, FUS and TAF15 revealed by multisystem analyses. Nat. Commun. 7, 1–14. doi: 10.1038/ncomms12143
Kausar, S., Wang, F., and Cui, H. (2018). The role of mitochondria in reactive oxygen species generation and its implications for neurodegenerative diseases. Cells 7, 274. doi: 10.3390/CELLS7120274
Kaut, O., Schmitt, I., and Wüllner, U. (2012). Genome-scale methylation analysis of Parkinson's disease patients' brains reveals DNA hypomethylation and increased MRNA expression of cytochrome P450 2E1. Neurogenetics 13, 87–91. doi: 10.1007/S10048-011-0308-3
Kauwe, J. S. K., Bailey, M. H., Ridge, P. G., Perry, R., Wadsworth, M. E., Hoyt, K. L., et al. (2014). Genome-wide association study of CSF levels of 59 Alzheimer's disease candidate proteins: significant associations with proteins involved in amyloid processing and inflammation. PLoS Genet. 10, e1004758. doi: 10.1371/journal.pgen.1004758
Kelly, J., Moyeed, R., Carroll, C., Albani, D., and Li, X. (2019). Gene expression meta-analysis of Parkinson's disease and its relationship with Alzheimer's disease. Mol. Brain 12, 1–10. doi: 10.1186/S13041-019-0436-5
Kenawy, H. I., Boral, I., and Bevington, A. (2015). Complement-coagulation cross-talk: a potential mediator of the physiological activation of complement by low PH. Front. Immunol. 6, 215. doi: 10.3389/FIMMU.2015.00215/BIBTEX
Kim, S., Swaminathan, S., Shen, L., Risacher, S. L., Nho, K., Foroud, T., et al. (2011). Genome-wide association study of CSF biomarkers Aβ1-42, t-tau, and p-Tau181p in the ADNI cohort. Neurology 76, 69–79. doi: 10.1212/WNL.0b013e318204a397
Kinney, J. W., Bemiller, S. M., Murtishaw, A. S., Leisgang, A. M., Salazar, A. M., and Lamb, B. T. (2018). Inflammation as a central mechanism in Alzheimer's disease. Alzheimer's Dement. Transl. Res. Clin. Interv. 4, 575–590. doi: 10.1016/j.trci.2018.06.014
Kjældgaard, A. L., Pilely, K., Olsen, K. S., Lauritsen, A. Ø., Pedersen, S. W., Svenstrup, K., et al. (2021). Complement profiles in patients with amyotrophic lateral sclerosis: a prospective observational cohort study. J. Inflamm. Res. 14, 1043–1053. doi: 10.2147/JIR.S298307
Komar, A. A. (2016). The Yin and Yang of codon usage. Hum. Mol. Genet. 25, R77–R85. doi: 10.1093/hmg/ddw207
Kramer, P. L., Xu, H., Woltjer, R. L., Westaway, S. K., Clark, D., Erten-Lyons, D., et al. (2011). Alzheimer disease pathology in cognitively healthy elderly: a genome-wide study. Neurobiol. Aging 32, 2113–2122. doi: 10.1016/j.neurobiolaging.2010.01.010
Kumar, A., Singh, P. K., Parihar, R., Dwivedi, V., Lakhotia, S. C., and Ganesh, S. (2014). Decreased O-linked GlcNAcylation protects from cytotoxicity mediated by Huntingtin exon1 protein fragment. J. Biol. Chem. 289, 13543. doi: 10.1074/JBC.M114.553321
Kunkle, B. W., Grenier-Boley, B., Sims, R., Bis, J. C., Damotte, V., Naj, A. C., et al. (2019). Genetic meta-analysis of diagnosed Alzheimer's disease identifies new risk loci and implicates Aβ, tau, immunity and lipid processing. Nat. Genet. 51, 414–430. doi: 10.1038/S41588-019-0358-2
Kunkle, B. W., Schmidt, M., Klein, H. U., Naj, A. C., Hamilton-Nelson, K. L., Larson, E. B., et al. (2021). Novel Alzheimer disease risk loci and pathways in African American individuals using the African genome resources panel: a meta-analysis. JAMA Neurol. 78, 102–113. doi: 10.1001/JAMANEUROL.2020.3536
Kurisu, S., and Takenawa, T. (2009). The WASP and WAVE family proteins. Genome Biol. 10, 226. doi: 10.1186/GB-2009-10-6-226/FIGURES/3
Kurreck, J. (2009). RNA interference: from basic research to therapeutic applications. Angew. Chem. Int. Ed. 48, 1378–1398. doi: 10.1002/anie.200802092
Kurutas, E. B. (2015). The importance of antioxidants which play the role in cellular response against oxidative/nitrosative stress: current state. Nutr. J. 15, 1–22. doi: 10.1186/S12937-016-0186-5
Kwee, L. C., Liu, Y., Haynes, C., Gibson, J. R., Stone, A., Schichman, S. A., et al. (2012). A high-density genome-wide association screen of sporadic Als in US veterans. PLoS ONE 7, e32768. doi: 10.1371/journal.pone.0032768
Laaksovirta, H., Peuralinna, T., Schymick, J. C., Scholz, S. W., Lai, S. L., Myllykangas, L., et al. (2010). Chromosome 9p21 in amyotrophic lateral sclerosis in Finland: a genome-wide association study. Lancet Neurol. 9, 978–985. doi: 10.1016/S1474-4422(10)70184-8
Labadorf, A., Hoss, A. G., Lagomarsino, V., Latourelle, J. C., Hadzi, T. C., Bregu, J., et al. (2015). RNA sequence analysis of human huntington disease brain reveals an extensive increase in inflammatory and developmental gene expression. PLoS ONE 10, e143563. doi: 10.1371/journal.pone.0143563
Lachén-Montes, M., González-Morales, A., Iloro, I., Elortza, F., Ferrer, I., Gveric, D., et al. (2019). Unveiling the olfactory proteostatic disarrangement in Parkinson's disease by proteome-wide profiling. Neurobiol. Aging 73, 123–134. doi: 10.1016/j.neurobiolaging.2018.09.018
Lachén-Montes, M., González-Morales, A., Zelaya, M. V., Pérez-Valderrama, E., Ausín, K., Ferrer, I., et al. (2017). Olfactory bulb neuroproteomics reveals a chronological perturbation of survival routes and a disruption of prohibitin complex during Alzheimer's disease progression. Sci. Rep. 7, 1–15. doi: 10.1038/s41598-017-09481-x
Lambert, J. C., Grenier-Boley, B., Harold, D., Zelenika, D., Chouraki, V., Kamatani, Y., et al. (2013a). Genome-wide haplotype association study identifies the FRMD4A gene as a risk locus for Alzheimer's disease. Mol. Psychiatry 18, 461–470. doi: 10.1038/mp.2012.14
Lambert, J. C., Heath, S., Even, G., Campion, D., Sleegers, K., Hiltunen, M., et al. (2009). Genome-wide association study identifies variants at CLU and CR1 associated with Alzheimer's disease. Nat. Genet. 41, 1094–1099. doi: 10.1038/ng.439
Lambert, J. C., Ibrahim-Verbaas, C. A., Harold, D., Naj, A. C., Sims, R., Bellenguez, C., et al. (2013b). Meta-analysis of 74,046 individuals identifies 11 new susceptibility loci for Alzheimer's disease. Nat. Genet. 45, 1452–1458. doi: 10.1038/ng.2802
Landers, J. E., Melki, J., Meininger, V., Glass, J. D., Van Den Berg, L. H., Van Es, M. A., et al. (2009). Reduced expression of the kinesin-associated protein 3 (KIFAP3) gene increases survival in sporadic amyotrophic lateral sclerosis. Proc. Natl. Acad. Sci. U.S.A. 106, 9004–9009. doi: 10.1073/pnas.0812937106
Langefeld, C. D. (2013). Age of onset of amyotrophic lateral sclerosis is modulated by a locus on 1p34.1. Neurobiol. Aging 34, 357.e7–e19. doi: 10.1016/j.neurobiolaging.2012.07.017
Latourelle, J. C., Pankratz, N., Dumitriu, A., Wilk, J. B., Goldwurm, S., Pezzoli, G., et al. (2009). Genomewide association study for onset age in Parkinson disease. BMC Med. Genet. 10, 98. doi: 10.1186/1471-2350-10-98
Lau, E., Cao, Q., Lam, M. P. Y., Wang, J., Ng, D. C. M., Bleakley, B. J., et al. (2018). Integrated omics dissection of proteome dynamics during cardiac remodeling. Nat. Commun. 9, 1–14. doi: 10.1038/s41467-017-02467-3
Lavrik, I., Golks, A., and Krammer, P. H. (2005). Death receptor signaling. J. Cell Sci. 118, 265–267. doi: 10.1242/JCS.01610
Lee, E., Giovanello, K. S., Saykin, A. J., Xie, F., Kong, D., Wang, Y., et al. (2017). Single-nucleotide polymorphisms are associated with cognitive decline at Alzheimer's disease conversion within mild cognitive impairment patients. Alzheimer. Dement. Diagnosis Assessment Dis. Monitor. 8, 86–95. doi: 10.1016/j.dadm.2017.04.004
Lepeta, K., Lourenco, M. v., Schweitzer, B. C., Martino Adami, P. v., Banerjee, P., Catuara-Solarz, S., et al. (2016). Synaptopathies: synaptic dysfunction in neurological disorders – a review from students to students. J. Neurochem. 138, 785. doi: 10.1111/JNC.13713
Lesnick, T. G., Papapetropoulos, S., Mash, D. C., Ffrench-Mullen, J., Shehadeh, L., De Andrade, M., et al. (2007). A genomic pathway approach to a complex disease: axon guidance and Parkinson disease. PLoS Genet. 3, 0984–0995. doi: 10.1371/journal.pgen.0030098
Letunic, I., Khedkar, S., and Bork, P. (2021). SMART: recent updates, new developments and status in 2020. Nucleic Acids Res. 49, D458–D460. doi: 10.1093/NAR/GKAA937
Li, C., Ou, R., Chen, Y., Gu, X., Wei, Q., Cao, B., et al. (2021). Genetic modifiers of age at onset for Parkinson's disease in asians: a genome-wide association study. Mov. Disord. 36, 2077–2084. doi: 10.1002/MDS.28621
Li, H., Wetten, S., Li, L., St. Jean, P. L., Upmanyu, R., Surh, L., et al. (2008). Candidate single-nucleotide polymorphisms from a genomewide association study of Alzheimer disease. Arch. Neurol. 65, 45–53. doi: 10.1001/archneurol.2007.3
Li, M., Zou, D., Li, Z., Gao, R., Sang, J., Zhang, Y., et al. (2019). EWAS atlas: a curated knowledgebase of epigenome-wide association studies. Nucleic Acids Res. 47, D983–D988. doi: 10.1093/NAR/GKY1027
Li, P., Marshall, L., Oh, G., Jakubowski, J. L., Groot, D., He, Y., et al. (2019). Epigenetic dysregulation of enhancers in neurons is associated with Alzheimer's disease pathology and cognitive symptoms. Nat. Commun. 10, 1–14. doi: 10.1038/s41467-019-10101-7
Li, T., Gao, X., Han, L., Yu, J., and Li, H. (2018). Identification of hub genes with prognostic values in gastric cancer by bioinformatics analysis. World J. Surg. Oncol. 16, 1–12. doi: 10.1186/S12957-018-1409-3/FIGURES/5
Li, W. W., Li, J., and Bao, J. K. (2012). Microautophagy: lesser-known self-eating. Cell. Mol. Life Sci. 69, 1125–1136. doi: 10.1007/S00018-011-0865-5
Liang, W. S., Dunckley, T., Beach, T. G., Grover, A., Mastroeni, D., Walker, D. G., et al. (2007). Gene expression profiles in anatomically and functionally distinct regions of the normal aged human brain. Physiol. Genom. 28, 311–322. doi: 10.1152/physiolgenomics.00208.2006
Lill, C. M., Roehr, J. T., McQueen, M. B., Kavvoura, F. K., Bagade, S., Schjeide, B. M. M., et al. (2012). Comprehensive research synopsis and systematic meta-analyses in Parkinson's disease genetics: the PDgene database. PLoS Genet. 8, e1002548. doi: 10.1371/journal.pgen.1002548
Lim, R. G., Quan, C., Reyes-Ortiz, A. M., Lutz, S. E., Kedaigle, A. J., Gipson, T. A., et al. (2017b). Huntington's disease IPSC-derived brain microvascular endothelial cells reveal WNT-mediated angiogenic and blood-brain barrier deficits. Cell Rep. 19, 1365–1377. doi: 10.1016/j.celrep.2017.04.021
Lim, R. G., Salazar, L. L., Wilton, D. K., King, A. R., Stocksdale, J. T., Sharifabad, D., et al. (2017a). Developmental alterations in Huntington's disease neural cells and pharmacological rescue in cells and mice. Nat. Neurosci. 20, 648–660. doi: 10.1038/nn.4532
Lin, L., Park, J. W., Ramachandran, S., Zhang, Y., Tseng, Y. T., Shen, S., et al. (2016). Transcriptome sequencing reveals aberrant alternative splicing in Huntington's disease. Hum. Mol. Genet. 25, 3454–3466. doi: 10.1093/hmg/ddw187
Lindberg, I., Shorter, J., Wiseman, R. L., Chiti, F., Dickey, C. A., and McLean, P. J. (2015). Chaperones in neurodegeneration. J. Neurosci. 35, 13853–13859. doi: 10.1523/JNEUROSCI.2600-15.2015
Liu, E. Y., Cali, C. P., and Lee, E. B. (2017). RNA metabolism in neurodegenerative disease. DMM Dis. Models Mech. 10, 509–518. doi: 10.1242/dmm.028613
Liu, F., Iqbal, K., Grundke-Iqbal, I., Hart, G. W., and Gong, C. X. (2004). O-GlcNAcylation regulates phosphorylation of tau: a mechanism involved in Alzheimer's disease. Proc. Natl. Acad. Sci. U.S.A. 101, 10804. doi: 10.1073/PNAS.0400348101
Liu, G., Peng, J., Liao, Z., Locascio, J. J., Corvol, J. C., Zhu, F., et al. (2021). Genome-wide survival study identifies a novel synaptic locus and polygenic score for cognitive progression in Parkinson's disease. Nat. Genet. 53, 787–793. doi: 10.1038/S41588-021-00847-6
Liu, X., Cheng, R., Verbitsky, M., Kisselev, S., Browne, A., Mejia-Sanatana, H., et al. (2011). Genome-wide association study identifies candidate genes for Parkinson's disease in an Ashkenazi Jewish population. BMC Med. Genet. 12, 104. doi: 10.1186/1471-2350-12-104
Liu, Y., Beyer, A., and Aebersold, R. (2016). On the dependency of cellular protein levels on MRNA abundance. Cell 165, 535–550. doi: 10.1016/j.cell.2016.03.014
Lo, M. T., Kauppi, K., Fan, C. C., Sanyal, N., Reas, E. T., Sundar, V. S., et al. (2019). Identification of genetic heterogeneity of Alzheimer's disease across age. Neurobiol. Aging 84, 243.e1–e9. doi: 10.1016/j.neurobiolaging.2019.02.022
Loesch, D. P., Horimoto, A. R. V. R., Heilbron, K., Sarihan, E. I., Inca-Martinez, M., Mason, E., et al. (2021). Characterizing the genetic architecture of Parkinson's disease in latinos. Ann. Neurol. 90, 353–365. doi: 10.1002/ANA.26153
Logue, M. W., Schu, M., Vardarajan, B. N., Buros, J., Green, R. C., Go, R. C. P., et al. (2011). A comprehensive genetic association study of Alzheimer disease in African Americans. Arch. Neurol. 68, 1569–1579. doi: 10.1001/archneurol.2011.646
López-Sánchez, N., Fontán-Lozano, Á., Pallé, A., González-Álvarez, V., Rábano, A., Trejo, J. L., et al. (2017). Neuronal tetraploidization in the cerebral cortex correlates with reduced cognition in mice and precedes and recapitulates Alzheimer's-associated neuropathology. Neurobiol. Aging 56, 50–66. doi: 10.1016/J.NEUROBIOLAGING.2017.04.008
Lu, A. T., Narayan, P., Grant, M. J., Langfelder, P., Wang, N., Kwak, S., et al. (2020). DNA methylation study of Huntington's disease and motor progression in patients and in animal models. Nat. Commun. 11, 1–15. doi: 10.1038/S41467-020-18255-5
Lu, L., and Hong, W. (2014). From endosomes to the trans-golgi network. Semin. Cell Dev. Biol. 31, 30–39. doi: 10.1016/J.SEMCDB.2014.04.024
Lüdemann, N., Clement, A., Hans, V. H., Leschik, J., Behl, C., and Brandt, R. (2005). O-glycosylation of the tail domain of neurofilament protein M in human neurons and in spinal cord tissue of a rat model of amyotrophic lateral sclerosis (ALS) *. J. Biol. Chem. 280, 31648–31658. doi: 10.1074/JBC.M504395200
Lunnon, K., Smith, R., Hannon, E., de Jager, P. L., Srivastava, G., Volta, M., et al. (2014). Methylomic profiling implicates cortical deregulation of ANK1 in Alzheimer's disease. Nat. Neurosci. 17, 1164–1170. doi: 10.1038/NN.3782
Magistri, M., Velmeshev, D., Makhmutova, M., and Faghihi, M. A. (2015). Transcriptomics profiling of Alzheimer's disease reveal neurovascular defects, altered amyloid-β homeostasis, and deregulated expression of long noncoding RNAs. J. Alzheimer's Dis. 48, 647–665. doi: 10.3233/JAD-150398
Maguire, G. (2018). Neurodegenerative diseases are a function of matrix breakdown: how to rebuild extracellular matrix and intracellular matrix. Neural Regener. Res. 13, 1185. doi: 10.4103/1673-5374.235026
Maier, T., Güell, M., and Serrano, L. (2009). Correlation of mRNA and protein in complex biological samples. FEBS Lett. 583, 3966–3973. doi: 10.1016/j.febslet.2009.10.036
Mandelkow, E. M., Biernat, J., Drewes, G., Gustke, N., Trinczek, B., and Mandelkow, E. (1995). Tau domains, phosphorylation, and interactions with microtubules. Neurobiol. Aging 16, 355–362. doi: 10.1016/0197-4580(95)00025-A
Maraganore, D. M., De Andrade, M., Lesnick, T. C., Strain, K. J., Farrer, M. J., Rocca, W. A., et al. (2005). High-resolution whole-genome association study of Parkinson disease. Am. J. Hum. Genet. 77, 685–693. doi: 10.1086/496902
Marioni, R. E., Harris, S. E., Zhang, Q., McRae, A. F., Hagenaars, S. P., Hill, W. D., et al. (2018). GWAS on family history of Alzheimer's DISEASE. Transl. Psychiatry 8, 99. doi: 10.1038/s41398-018-0150-6
Marshall, L. L., Killinger, B. A., Ensink, E., Li, P., Li, K. X., Cui, W., et al. (2020). Epigenomic analysis of Parkinson's disease neurons identifies Tet2 loss as neuroprotective. Nat. Neurosci. 23, 1203–1214. doi: 10.1038/S41593-020-0690-Y
Martinelli-Boneschi, F., Giacalone, G., Magnani, G., Biella, G., Coppi, E., Santangelo, R., et al. (2013). Pharmacogenomics in Alzheimer's disease: a genome-wide association study of response to cholinesterase inhibitors. Neurobiol. Aging 34, 1711.e7–e13. doi: 10.1016/j.neurobiolaging.2012.12.008
Martínez-Menárguez, J. Á., Tomás, M., Martínez-Martínez, N., and Martínez-Alonso, E. (2019). Golgi fragmentation in neurodegenerative diseases: is there a common cause? Cells 8, 748. doi: 10.3390/CELLS8070748
Masliah, E., Dumaop, W., Galasko, D., and Desplats, P. (2013). Distinctive patterns of DNA methylation associated with parkinson disease: identification of concordant epigenetic changes in brain and peripheral blood leukocytes. Epigenetics 8, 1030–1038. doi: 10.4161/EPI.25865
Mathys, H., Davila-Velderrain, J., Peng, Z., Gao, F., Mohammadi, S., Young, J. Z., et al. (2019). Single-cell transcriptomic analysis of Alzheimer's disease. Nature 570, 332–337. doi: 10.1038/s41586-019-1195-2
Mcilwain, D. R., Berger, T., Mak, T. W., Baehrecke, E. H., Green, D. R., Kornbluth, S., et al. (2013). Caspase functions in cell death and disease. Cold Spring Harb. Perspect. Biol. 5, a008656. doi: 10.1101/CSHPERSPECT.A008656
McLaughlin, R. L., Kenna, K. P., Vajda, A., Bede, P., Elamin, M., Cronin, S., et al. (2015). A second-generation irish genome-wide association study for amyotrophic lateral sclerosis. Neurobiol. Aging 36, 1221.e7–e13. doi: 10.1016/j.neurobiolaging.2014.08.030
McQuade, L. R., Balachandran, A., Scott, H. A., Khaira, S., Baker, M. S., and Schmidt, U. (2014). Proteomics of Huntington's disease-affected human embryonic stem cells reveals an evolving pathology involving mitochondrial dysfunction and metabolic disturbances. J. Proteome Res. 13, 5648–5659. doi: 10.1021/pr500649m
McShane, E., Sin, C., Zauber, H., Wells, J. N., Donnelly, N., Wang, X., et al. (2016). Kinetic analysis of protein stability reveals age-dependent degradation. Cell 167, 803–815.e21. doi: 10.1016/j.cell.2016.09.015
Meda, S. A., Narayanan, B., Liu, J., Perrone-Bizzozero, N. I., Stevens, M. C., Calhoun, V. D., et al. (2012). A large scale multivariate parallel ICA method reveals novel imaging-genetic relationships for Alzheimer's disease in the ADNI Cohort. Neuroimage 60, 1608–1621. doi: 10.1016/j.neuroimage.2011.12.076
Medzhitov, R. (2007). Recognition of microorganisms and activation of the immune response. Nature 449, 819–826. doi: 10.1038/nature06246
Mehta, S. R., Tom, C. M., Wang, Y., Bresee, C., Rushton, D., Mathkar, P. P., et al. (2018). Human Huntington's disease IPSC-derived cortical neurons display altered transcriptomics, morphology, and maturation. Cell Rep. 25, 1081–1096.e6. doi: 10.1016/j.celrep.2018.09.076
Mesa-Herrera, F., Taoro-González, L., Valdés-Baizabal, C., Diaz, M., and Marín, R. (2019). Lipid and lipid Raft alteration in aging and neurodegenerative diseases: a window for the development of new biomarkers. Int. J. Mol. Sci. 20, 3810. doi: 10.3390/IJMS20153810
Meyer, K., Feldman, H. M., Lu, T., Drake, D., Lim, E. T., Ling, K. H., et al. (2019). REST and neural gene network dysregulation in IPSC models of Alzheimer's disease. Cell Rep. 26, 1112–1127.e9. doi: 10.1016/j.celrep.2019.01.023
Mez, J., Chung, J., Jun, G., Kriegel, J., Bourlas, A. P., Sherva, R., et al. (2017). Two novel loci, COBL and SLC10A2, for Alzheimer's disease in African Americans. Alzheimers Dement. 13, 119–129. doi: 10.1016/J.JALZ.2016.09.002
Millecamps, S., and Julien, J. P. (2013). Axonal transport deficits and neurodegenerative diseases. Nat. Rev. Neurosci. 14, 161–176. doi: 10.1038/nrn3380
Miron, J., Picard, C., Nilsson, N., Frappier, J., Dea, D., Théroux, L., et al. (2018). CDK5RAP2 gene and tau pathophysiology in late-onset sporadic Alzheimer's disease. Alzheimers Dement. 14, 787–796. doi: 10.1016/J.JALZ.2017.12.004
Missler, M., Südhof, T. C., and Biederer, T. (2012). Synaptic cell adhesion. Cold Spring Harb. Perspect. Biol. 4, a005694. doi: 10.1101/CSHPERSPECT.A005694
Mistry, J., Chuguransky, S., Williams, L., Qureshi, M., Salazar, G. A., Sonnhammer, E. L. L., et al. (2021). Pfam: the protein families database in 2021. Nucleic Acids Res. 49, D412. doi: 10.1093/NAR/GKAA913
Mitchison, T., and Kirschner, M. (1984). Dynamic instability of microtubule growth. Nature 312, 237–242. doi: 10.1038/312237a0
Miyashita, A., Koike, A., Jun, G., Wang, L. S., Takahashi, S., Matsubara, E., et al. (2013). SORL1 is genetically associated with late-onset Alzheimer's disease in Japanese, Koreans and Caucasians. PLoS ONE 8, e0058618. doi: 10.1371/journal.pone.0058618
Mizushima, N., and Klionsky, D. J. (2007). Protein turnover via autophagy: implications for metabolism. Annu. Rev. Nutr. 27, 19–40. doi: 10.1146/ANNUREV.NUTR.27.061406.093749
Moll, T., Shaw, P. J., and Cooper-Knock, J. (2020). Disrupted glycosylation of lipids and proteins is a cause of neurodegeneration. Brain 143, 1332. doi: 10.1093/BRAIN/AWZ358
Moreno-Grau, S., de Rojas, I., Hernández, I., Quintela, I., Montrreal, L., Alegret, M., et al. (2019). Genome-wide association analysis of dementia and its clinical endophenotypes reveal novel loci associated with Alzheimer's disease and three causality networks: the GR@ACE project. Alzheimer's Dementia 15, 1333–1347. doi: 10.1016/j.jalz.2019.06.4950
Moss, D. J. H., Tabrizi, S. J., Mead, S., Lo, K., Pardiñas, A. F., Holmans, P., et al. (2017). Identification of genetic variants associated with huntington's disease progression: a genome-wide association study. Lancet Neurol. 16, 701–711. doi: 10.1016/S1474-4422(17)30161-8
Mukherjee, S., Mez, J., Trittschuh, E. H., Saykin, A. J., Gibbons, L. E., Fardo, D. W., et al. (2018). Genetic data and cognitively defined late-onset Alzheimer's disease subgroups. Mol. Psychiatry. 25, 2942–2951. doi: 10.1038/s41380-018-0298-8
Muñoz-Lasso, D. C., Romá-Mateo, C., Pallardó, F. v., and Gonzalez-Cabo, P. (2020). Much more than a scaffold: cytoskeletal proteins in neurological disorders. Cells 9, 358. doi: 10.3390/CELLS9020358
Nah, J., Yuan, J., and Jung, Y. K. (2015). Autophagy in neurodegenerative diseases: from mechanism to therapeutic approach. Mol. Cells 38, 381. doi: 10.14348/MOLCELLS.2015.0034
Naj, A. C., Beecham, G. W., Martin, E. R., Gallins, P. J., Powell, E. H., Konidari, I., et al. (2010). Dementia revealed: novel chromosome 6 locus for late-onset Alzheimer disease provides genetic evidence for folate-pathway abnormalities. PLoS Genet. 6, e1001130. doi: 10.1371/journal.pgen.1001130
Naj, A. C., Jun, G., Beecham, G. W., Wang, L. S., Vardarajan, B. N., Buros, J., et al. (2011). Common variants at MS4A4/MS4A6E, CD2AP, CD33 and EPHA1 are associated with late-onset Alzheimer's disease. Nat. Genet. 43, 436–443. doi: 10.1038/ng.801
Nakamura, R., Misawa, K., Tohnai, G., Nakatochi, M., Furuhashi, S., Atsuta, N., et al. (2020). A multi-ethnic meta-analysis identifies novel genes, including ACSL5, associated with amyotrophic lateral sclerosis. Commun. Biol. 3, 1–9. doi: 10.1038/S42003-020-01251-2
Nalls, M. A., Blauwendraat, C., Vallerga, C. L., Heilbron, K., Bandres-Ciga, S., Chang, D., et al. (2019). Identification of novel risk loci, causal insights, and heritable risk for Parkinson's disease: a meta-analysis of genome-wide association studies. Lancet Neurol. 18, 1091–1102. doi: 10.1016/S1474-4422(19)30320-5
Nalls, M. A., Pankratz, N., Lill, C. M., Do, C. B., Hernandez, D. G., Saad, M., et al. (2014). Large-scale meta-analysis of genome-wide association data identifies six new risk loci for Parkinson's disease. Nat. Genet. 46, 989–993. doi: 10.1038/ng.3043
Nalls, M. A., Plagnol, V., Hernandez, D. G., Sharma, M., Sheerin, U. M., Saad, M., et al. (2011). Imputation of sequence variants for identification of genetic risks for Parkinson's disease: a meta-analysis of genome-wide association studies. Lancet 377, 641–649. doi: 10.1016/S0140-6736(10)62345-8
Natoli, G. (2009). Control of NF-KB-dependent transcriptional responses by chromatin organization. Cold Spring Harb. Perspect. Biol. 1, a000224. doi: 10.1101/CSHPERSPECT.A000224
Nazarian, A., Arbeev, K. G., Yashkin, A. P., and Kulminski, A. M. (2019a). Genetic heterogeneity of Alzheimer's disease in subjects with and without hypertension. Geroscience 41, 137–154. doi: 10.1007/S11357-019-00071-5/TABLES/5
Nazarian, A., Yashin, A. I., and Kulminski, A. M. (2019b). Genome-wide analysis of genetic predisposition to Alzheimer's disease and related sex disparities. Alzheimer's Res. Ther. 11, 1–21. doi: 10.1186/s13195-018-0458-8
Nelson, P. T., Estus, S., Abner, E. L., Parikh, I., Malik, M., Neltner, J. H., et al. (2014). ABCC9 gene polymorphism is associated with hippocampal sclerosis of aging pathology. Acta Neuropathol. 127, 825–843. doi: 10.1007/s00401-014-1282-2
Nicolas, A., Kenna, K., Renton, A. E., Ticozzi, N., Faghri, F., Chia, R., et al. (2018). Genome-wide analyses identify KIF5A as a novel ALS gene. Neuron 97, 1268–1283.e6. doi: 10.1016/j.neuron.2018.02.027
Nissanka, N., and Moraes, C. T. (2018). Mitochondrial DNA damage and reactive oxygen species in neurodegenerative disease. FEBS Lett. 592, 728–742. doi: 10.1002/1873-3468.12956
Noatynska, A., Gotta, M., and Meraldi, P. (2012). Mitotic spindle (DIS)orientation and disease: cause or consequence? J. Cell Biol. 199, 1025. doi: 10.1083/JCB.201209015
O'brien, J. S., Sampson, E. L., Brien, O., Fillerup, D. L., Mead, J. F., and Lz, J. (1964). Lipid composition of the normal human brain: gray matter, white matter, and myelin. J. Lipid Res. 5, 329.
Oeckl, P., Weydt, P., Thal, D. R., Weishaupt, J. H., Ludolph, A. C., and Otto, M. (2020). Proteomics in cerebrospinal fluid and spinal cord suggests UCHL1, MAP2 and GPNMB as biomarkers and underpins importance of transcriptional pathways in amyotrophic lateral sclerosis. Acta Neuropathol. 139, 119–134. doi: 10.1007/s00401-019-02093-x
Otake, K., Kamiguchi, H., and Hirozane, Y. (2019). Identification of biomarkers for amyotrophic lateral sclerosis by comprehensive analysis of exosomal MRNAs in human cerebrospinal fluid. BMC Med. Genom. 12, 7. doi: 10.1186/s12920-019-0473-z
Pakos-Zebrucka, K., Koryga, I., Mnich, K., Ljujic, M., Samali, A., and Gorman, A. M. (2016). The integrated stress response. EMBO Rep. 17, 1374. doi: 10.15252/EMBR.201642195
Pan, Z., Trikalinos, T. A., Kavvoura, F. K., Lau, J., and Ioannidis, J. P. A. (2005). Local literature bias in genetic epidemiology: an empirical evaluation of the Chinese literature. PLoS Med. 2, 1309–1317. doi: 10.1371/JOURNAL.PMED.0020334
Pankratz, N., Beecham, G. W., Destefano, A. L., Dawson, T. M., Doheny, K. F., Factor, S. A., et al. (2012). Meta-analysis of Parkinson's disease: identification of a novel locus, RIT2. Ann. Neurol. 71, 370–384. doi: 10.1002/ana.22687
Pankratz, N., Wilk, J. B., Latourelle, J. C., DeStefano, A. L., Halter, C., Pugh, E. W., et al. (2009). Genomewide association study for susceptibility genes contributing to familial Parkinson disease. Hum. Genet. 124, 593–605. doi: 10.1007/s00439-008-0582-9
Papatheodorou, I., Fonseca, N. A., Keays, M., Tang, Y. A., Barrera, E., Bazant, W., et al. (2018). Expression atlas: gene and protein expression across multiple studies and organisms. Nucleic Acids Res. 46, D246–D251. doi: 10.1093/nar/gkx1158
Park, K. W., Jo, S., Kim, M. S., Jeon, S. R., Ryu, H. S., Kim, J., et al. (2021). Genomic association study for cognitive impairment in Parkinson's disease. Front. Neurol. 11, 579268. doi: 10.3389/FNEUR.2020.579268
Pathak, N., Vimal, S. K., Tandon, I., Agrawal, L., Hongyi, C., and Bhattacharyya, S. (2021). Neurodegenerative disorders of Alzheimer, Parkinsonism, Amyotrophic lateral sclerosis and multiple sclerosis: an early diagnostic approach for precision treatment. Metab. Brain Dis. 37, 67–104. doi: 10.1007/S11011-021-00800-W/FIGURES/8
Pérez-Palma, E., Bustos, B. I., Villamán, C. F., Alarcón, M. A., Avila, M. E., Ugarte, G. D. V., et al. (2014). Overrepresentation of glutamate signaling in Alzheimer's disease: network-based pathway enrichment using meta-analysis of genome-wide association studies. PLoS ONE 9, e95413. doi: 10.1371/journal.pone.0095413
Perlson, E., Maday, S., Fu, M. m., Moughamian, A. J., and Holzbaur, E. L. F. (2010). Retrograde axonal transport: pathways to cell death? Trends Neurosci. 33, 335. doi: 10.1016/J.TINS.2010.03.006
Perluigi, M., di Domenico, F., and Butterfield, D. A. (2015). MTOR signaling in aging and neurodegeneration: at the crossroad between metabolism dysfunction and impairment of autophagy. Neurobiol. Dis. 84, 39–49. doi: 10.1016/J.NBD.2015.03.014
Petit, C. S., Lee, J. J., Boland, S., Swarup, S., Christiano, R., Lai, Z. W., et al. (2020). Inhibition of sphingolipid synthesis improves outcomes and survival in GARP mutant wobbler mice, a model of motor neuron degeneration. Proc. Natl. Acad. Sci. U.S.A. 117, 10565–10574. doi: 10.1073/PNAS.1913956117
Pfisterer, K., Jayo, A., and Parsons, M. (2017). Control of nuclear organization by F-actin binding proteins. Nucleus 8, 126–133. doi: 10.1080/19491034.2016.1267093
Pickrell, J. K., Berisa, T., Liu, J. Z., Ségurel, L., Tung, J. Y., and Hinds, D. A. (2016). Detection and interpretation of shared genetic influences on 42 human traits. Nat. Genet. 48, 709–717. doi: 10.1038/ng.3570
Pillai, R. S., Bhattacharyya, S. N., and Filipowicz, W. (2007). Repression of protein synthesis by MiRNAs: how many mechanisms? Trends Cell Biol. 17, 118–126. doi: 10.1016/j.tcb.2006.12.007
Polis, B., and Samson, A. O. (2020). Role of the metabolism of branched-chain amino acids in the development of Alzheimer's disease and other metabolic disorders. Neural Regener. Res. 15, 1460. doi: 10.4103/1673-5374.274328
Pottier, C., Zhou, X., Perkerson, R. B., Baker, M., Jenkins, G. D., Serie, D. J., et al. (2018). Potential genetic modifiers of disease risk and age at onset in patients with frontotemporal lobar degeneration and GRN mutations: a genome-wide association study. Lancet Neurol. 17, 548–558. doi: 10.1016/S1474-4422(18)30126-1
Prudencio, M., Belzil, V. V., Batra, R., Ross, C. A., Gendron, T. F., Pregent, L. J., et al. (2015). Distinct brain transcriptome profiles in C9orf72-associated and sporadic ALS. Nat. Neurosci. 18, 1175–1182. doi: 10.1038/nn.4065
Puchkov, D., and Haucke, V. (2013). Greasing the synaptic vesicle cycle by membrane lipids. Trends Cell Biol. 23, 493–503. doi: 10.1016/J.TCB.2013.05.002
Qin, Z. H., Tao, L. Y., and Chen, X. (2007). Dual roles of NF-KB in cell survival and implications of NF-KB inhibitors in neuroprotective therapy. Acta Pharmacol. Sin. 28, 1859–1872. doi: 10.1111/j.1745-7254.2007.00741.x
Raman, R., Allen, S. P., Goodall, E. F., Kramer, S., Ponger, L. L., Heath, P. R., et al. (2015). Gene expression signatures in motor neurone disease fibroblasts reveal dysregulation of metabolism, hypoxia-response and RNA processing functions. Neuropathol. Appl. Neurobiol. 41, 201–226. doi: 10.1111/nan.12147
Ramanan, V. K., Risacher, S. L., Nho, K., Kim, S., Swaminathan, S., Shen, L., et al. (2014). APOE and BCHE as modulators of cerebral amyloid deposition: a florbetapir PET genome-wide association study. Mol. Psychiatry 19, 351–357. doi: 10.1038/mp.2013.19
Ramanan, V. K., and Saykin, A. J. (2013). Pathways to neurodegeneration: mechanistic insights from GWAS in Alzheimer's disease, Parkinson's disease, and Related Disorders. Am. J. Neurodegener. Dis. 2, 145–175. Available online at: www.AJND.us
Ramirez, A., van der Flier, W. M., Herold, C., Ramonet, D., Heilmann, S., Lewczuk, P., et al. (2014). SUCLG2 identified as both a determinator of CSF Aβ1-42 levels and an attenuator of cognitive decline in Alzheimer's disease. Hum. Mol. Genet. 23, 6644–6658. doi: 10.1093/hmg/ddu372
Ratovitski, T., Chaerkady, R., Kammers, K., Stewart, J. C., Zavala, A., Pletnikova, O., et al. (2016). Quantitative proteomic analysis reveals similarities between Huntington's disease (HD) and Huntington's disease-like 2 (HDL2) human brains. J. Proteome Res. 15, 3266–3283. doi: 10.1021/acs.jproteome.6b00448
Reddy, J. S., Allen, M., Ho, C. C. G., Oatman, S. R., Iş, Ö., Quicksall, Z. S., et al. (2021). Genome-wide analysis identifies a novel LINC-PINT splice variant associated with vascular amyloid pathology in Alzheimer's disease. Acta Neuropathol. Commun. 9, 1–15. doi: 10.1186/S40478-021-01199-2
Reily, C., Stewart, T. J., Renfrow, M. B., and Novak, J. (2019). Glycosylation in health and disease. Nat. Rev. Nephrol. 15, 346–366. doi: 10.1038/s41581-019-0129-4
Reiman, E. M., Webster, J. A., Myers, A. J., Hardy, J., Dunckley, T., Zismann, V. L., et al. (2007). GAB2 alleles modify Alzheimer's risk in APOE E4 carriers. Neuron 54, 713–720. doi: 10.1016/j.neuron.2007.05.022
Reitz, C., Jun, G., Naj, A., Rajbhandary, R., Vardarajan, B. N., Wang, L. S., et al. (2013). Variants in the ATP-binding cassette transporter (ABCA7), apolipoprotein e E4, and the risk of late-onset Alzheimer disease in African Americans. JAMA 309, 1483–1492. doi: 10.1001/jama.2013.2973
Riley, B. E., Gardai, S. J., Emig-Agius, D., Bessarabova, M., Ivliev, A. E., Schüle, B., et al. (2014). Systems-based analyses of brain regions functionally impacted in Parkinson's disease reveals underlying causal mechanisms. PLoS ONE 9, e102909. doi: 10.1371/journal.pone.0102909
Ring, K. L., An, M. C., Zhang, N., O'Brien, R. N., Ramos, E. M., Gao, F., et al. (2015). Genomic analysis reveals disruption of striatal neuronal development and therapeutic targets in human Huntington's disease neural stem cells. Stem Cell Rep. 5, 1023–1038. doi: 10.1016/j.stemcr.2015.11.005
Ristow, M. (2004). Neurodegenetive disorders associated with diabetes mellitus. J. Mol. Med. 82, 510–529. doi: 10.1007/S00109-004-0552-1/TABLES/1
Rodrigo, L. M., and Nyholt, D. R. (2021). Imputation and reanalysis of exome chip data identifies novel, conditional and joint genetic effects on Parkinson's disease risk. Genes 12, 689. doi: 10.3390/GENES12050689
Roos, R. A. C. (2010). Huntington's disease: a clinical review. Orphanet J. Rare Dis. 5, 40. doi: 10.1186/1750-1172-5-40
Rosenberg, R. N., Lambracht-Washington, D., Yu, G., and Xia, W. (2016). Genomics of Alzheimer disease: a review. JAMA Neurol. 73, 867–874. doi: 10.1001/JAMANEUROL.2016.0301
Rotunno, M. S., Lane, M., Zhang, W., Wolf, P., Oliva, P., Viel, C., et al. (2020). Cerebrospinal fluid proteomics implicates the granin family in Parkinson's disease. Sci. Rep. 10, 1–11. doi: 10.1038/s41598-020-59414-4
Ruffini, N., Klingenberg, S., Schweiger, S., and Gerber, S. (2020). Common factors in neurodegeneration: a meta-study revealing shared patterns on a multi-omics scale. Cells 9, 2642. doi: 10.3390/CELLS9122642
Ryu, H. S., Park, K. W., Choi, N., Kim, J., Park, Y. M., Jo, S., et al. (2020). Genomic analysis identifies new loci associated with motor complications in Parkinson's disease. Front. Neurol. 11, 570. doi: 10.3389/FNEUR.2020.00570
Saad, M., Lesage, S., Saint-Pierre, A., Corvol, J. C., Zelenika, D., Lambert, J. C., et al. (2011). Genome-wide association study confirms BST1 and suggests a locus on 12q24 as the risk loci for Parkinson's disease in the European population. Hum. Mol. Genet. 20, 615–627. doi: 10.1093/hmg/ddq497
Sakaue, S., Kanai, M., Tanigawa, Y., Karjalainen, J., Kurki, M., Koshiba, S., et al. (2021). A cross-population atlas of genetic associations for 220 human phenotypes. Nat. Genet. 53, 1415–1424. doi: 10.1038/S41588-021-00931-X
Saltiel, A. R., and Pessin, J. E. (2002). Insulin signaling pathways in time and space. Trends Cell Biol. 12, 65–71. doi: 10.1016/S0962-8924(01)02207-3
Sanchez-Mut, J. v., Heyn, H., Vidal, E., Moran, S., Sayols, S., Delgado-Morales, R., et al. (2016). Human DNA methylomes of neurodegenerative diseases show common epigenomic patterns. Transl. Psychiatry 6, e718. doi: 10.1038/TP.2015.214
Satake, W., Nakabayashi, Y., Mizuta, I., Hirota, Y., Ito, C., Kubo, M., et al. (2009). Genome-wide association study identifies common variants at four loci as genetic risk factors for Parkinson's disease. Nat. Genet. 41, 1303–1307. doi: 10.1038/ng.485
Satoh, K., Abe-Dohmae, S., Yokoyama, S., st. George-Hyslop, P., and Fraser, P. E. (2015). ATP-binding cassette transporter A7 (ABCA7) loss of function alters Alzheimer amyloid processing. J. Biol. Chem. 290, 24152–24165. doi: 10.1074/JBC.M115.655076
Schaefer, L. (2014). Complexity of danger: the diverse nature of damage-associated molecular patterns *. J. Biol. Chem. 289, 35237–35245. doi: 10.1074/jbc.R114.619304
Schaeffer, E., Schermann, A., Zirbs, F., and Berg, D. (2021). Increased intake of fast-acting carbohydrates in patients with Parkinson's disease. Neurodegener. Dis. 21, 103–108. doi: 10.1159/000520594
Schain, M., and Kreisl, W. C. (2017). Neuroinflammation in neurodegenerative disorders—a review. Curr. Neurol. Neurosci. Rep. 17, 1–11. doi: 10.1007/s11910-017-0733-2
Schartz, N. D., and Tenner, A. J. (2020). The good, the bad, and the opportunities of the complement system in neurodegenerative disease. J. Neuroinflamm. 17, 1–25. doi: 10.1186/S12974-020-02024-8
Scheckel, C., Drapeau, E., Frias, M. A., Park, C. Y., Fak, J., Zucker-Scharff, I., et al. (2016). Regulatory consequences of neuronal ELAV-like protein binding to coding and non-coding RNAs in human brain. Elife 5, e10421. doi: 10.7554/eLife.10421
Scherzer, C. R., Eklund, A. C., Morse, L. J., Liao, Z., Locascio, J. J., Fefer, D., et al. (2007). Molecular markers of early Parkinson's disease based on gene expression in blood. Proc. Natl. Acad. Sci. U.S.A. 104, 955–960. doi: 10.1073/pnas.0610204104
Schmidt, M. F., Gan, Z. Y., Komander, D., and Dewson, G. (2021). Ubiquitin signalling in neurodegeneration: mechanisms and therapeutic opportunities. Cell Death Different. 28, 570–590. doi: 10.1038/s41418-020-00706-7
Schott, J. M., Crutch, S. J., Carrasquillo, M. M., Uphill, J., Shakespeare, T. J., Ryan, N. S., et al. (2016). Genetic risk factors for the posterior cortical atrophy variant of Alzheimer's disease. Alzheimer's Dementia 12, 862–871. doi: 10.1016/j.jalz.2016.01.010
Schwartzentruber, J., Cooper, S., Liu, J. Z., Barrio-Hernandez, I., Bello, E., Kumasaka, N., et al. (2021). Genome-wide meta-analysis, fine-mapping and integrative prioritization implicate new Alzheimer's disease risk genes. Nat. Genet. 53, 392–402. doi: 10.1038/S41588-020-00776-W
Schymick, J. C., Scholz, S. W., Fung, H. C., Britton, A., Arepalli, S., Gibbs, J. R., et al. (2007). Genome-wide genotyping in amyotrophic lateral sclerosis and neurologically normal controls: first stage analysis and public release of data. Lancet Neurol. 6, 322–328. doi: 10.1016/S1474-4422(07)70037-6
Sen, R., and Smale, S. T. (2010). Selectivity of the NF-KB response. Cold Spring Harb. Perspect. Biol. 2, a000257. doi: 10.1101/CSHPERSPECT.A000257
Seneff, S., Wainwright, G., and Mascitelli, L. (2011). Nutrition and Alzheimer's disease: the detrimental role of a high carbohydrate diet. Eur. J. Intern. Med. 22, 134–140. doi: 10.1016/J.EJIM.2010.12.017
Seshadri, S., Fitzpatrick, A. L., Ikram, M. A., DeStefano, A. L., Gudnason, V., Boada, M., et al. (2010). Genome-wide analysis of genetic loci associated with Alzheimer disease. JAMA 303, 1832–1840. doi: 10.1001/jama.2010.574
Sethi, M. K., and Zaia, J. (2017). Extracellular matrix proteomics in Schizophrenia and Alzheimer's disease. Anal. Bioanal. Chem. 409, 379. doi: 10.1007/S00216-016-9900-6
Seyfried, N. T., Dammer, E. B., Swarup, V., Nandakumar, D., Duong, D. M., Yin, L., et al. (2017). A multi-network approach identifies protein-specific co-expression in asymptomatic and symptomatic Alzheimer's disease. Cell Syst. 4, 60–72.e4. doi: 10.1016/j.cels.2016.11.006
Shabab, T., and Zorofchian, S. (2017). Neuroinflammation pathways: a general review. Int. J. Neurosci. 217, 624–633. doi: 10.1080/00207454.2016.1212854
Shatunov, A., Mok, K., Newhouse, S., Weale, M. E., Smith, B., Vance, C., et al. (2010). Chromosome 9p21 in sporadic amyotrophic lateral sclerosis in the UK and seven other countries: a genome-wide association study. Lancet Neurol. 9, 986–994. doi: 10.1016/S1474-4422(10)70197-6
Sheikh, M. S., and Huang, Y. (2003). Cell cycle death receptor activation complexes: it takes two to activate TNF receptor. Cell Cycle 1, 549–551. doi: 10.4161/cc.2.6.566
Sherva, R., Gross, A., Mukherjee, S., Koesterer, R., Amouyel, P., Bellenguez, C., et al. (2020). Genome-wide association study of rate of cognitive decline in Alzheimer's disease patients identifies novel genes and pathways. Alzheimers Dement 16, 1134–1145. doi: 10.1002/ALZ.12106
Sherva, R., Tripodis, Y., Bennett, D. A., Chibnik, L. B., Crane, P. K., De Jager, P. L., et al. (2014). Genome-wide association study of the rate of cognitive decline in Alzheimer's disease. Alzheimer's Dementia 10, 45–52. doi: 10.1016/j.jalz.2013.01.008
Shigemizu, D., Mitsumori, R., Akiyama, S., Miyashita, A., Morizono, T., Higaki, S., et al. (2021). Ethnic and trans-ethnic genome-wide association studies identify new loci influencing Japanese Alzheimer's disease risk. Transl. Psychiatry 11, 1–10. doi: 10.1038/S41398-021-01272-3
Siitonen, A., Nalls, M. A., Hernández, D., Gibbs, J. R., Ding, J., Ylikotila, P., et al. (2017). Genetics of early-onset Parkinson's disease in Finland: exome sequencing and genome-wide association study. Neurobiol Aging 53, 195.e7–e10. doi: 10.1016/J.NEUROBIOLAGING.2017.01.019
Simón-Sánchez, J., Schulte, C., Bras, J. M., Sharma, M., Gibbs, J. R., Berg, D., et al. (2009). Genome-wide association study reveals genetic risk underlying Parkinson's disease. Nat. Genet. 41, 1308–1312. doi: 10.1038/ng.487
Sims, R., van der Lee, S. J., Naj, A. C., Bellenguez, C., Badarinarayan, N., Jakobsdottir, J., et al. (2017). Rare coding variants in PLCG2, ABI3, and TREM2 implicate microglial-mediated innate immunity in Alzheimer's disease. Nat. Genet. 49, 1373–1384. doi: 10.1038/NG.3916
Simunovic, F., Yi, M., Wang, Y., Macey, L., Brown, L. T., Krichevsky, A. M., et al. (2009). Gene expression profiling of substantia nigra dopamine neurons: further insights into Parkinson's disease pathology. Brain 132, 1795–1809. doi: 10.1093/brain/awn323
Sleigh, J. N., Rossor, A. M., Fellows, A. D., Tosolini, A. P., and Schiavo, G. (2019). Axonal transport and neurological disease. Nat. Rev. Neurol. 15, 691–703. doi: 10.1038/s41582-019-0257-2
Smeland, O. B., Shadrin, A., Bahrami, S., Broce, I., Tesli, M., Frei, O., et al. (2021). Genome-wide association analysis of Parkinson's disease and schizophrenia reveals shared genetic architecture and identifies novel risk loci. Biol. Psychiatry 89, 227–235. doi: 10.1016/J.BIOPSYCH.2020.01.026
Smith, A. R., Smith, R. G., Pishva, E., Hannon, E., Roubroeks, J. A. Y., Burrage, J., et al. (2019). Parallel profiling of DNA methylation and hydroxymethylation highlights neuropathology-associated epigenetic variation in Alzheimer's disease. Clin. Epigenet. 11, 1–13. doi: 10.1186/S13148-019-0636-Y/FIGURES/5
Smith, R. G., Hannon, E., de Jager, P. L., Chibnik, L., Lott, S. J., Condliffe, D., et al. (2018). Elevated DNA methylation across a 48-Kb region spanning the HOXA gene cluster is associated with Alzheimer's disease neuropathology. Alzheimers Dement. 14, 1580–1588. doi: 10.1016/J.JALZ.2018.01.017
Sofroniew, M. v. (2015). Astrocyte barriers to neurotoxic inflammation. Nat. Rev. Neurosci. 16, 249–263. doi: 10.1038/NRN3898
Sofroniew, M. v., and Vinters, H. v. (2010). Astrocytes: biology and pathology. Acta Neuropathol. 119, 7–35. doi: 10.1007/S00401-009-0619-8
Sonbol, H. S. (2018). Extracellular matrix remodeling in human disease. J. Microscopy Ultrastruct. 6, 123. doi: 10.4103/JMAU.JMAU_4_18
Spencer, C. C. A., Plagnol, V., Strange, A., Gardner, M., Paisan-Ruiz, C., Band, G., et al. (2011). Dissection of the genetics of Parkinson's disease identifies an additional association 5' of SNCA and multiple associated haplotypes at 17q21. Hum. Mol. Genet. 20, 345–353. doi: 10.1093/hmg/ddq469
Stein, J. L., Hua, X., Morra, J. H., Lee, S., Hibar, D. P., Ho, A. J., et al. (2010). Genome-wide analysis reveals novel genes influencing temporal lobe structure with relevance to neurodegeneration in Alzheimer's disease. Neuroimage 51, 542–554. doi: 10.1016/j.neuroimage.2010.02.068
Stopa, E. G., Tanis, K. Q., Miller, M. C., Nikonova, E. V., Podtelezhnikov, A. A., Finney, E. M., et al. (2018). Comparative transcriptomics of choroid plexus in Alzheimer's disease, frontotemporal dementia and Huntington's disease: implications for CSF homeostasis. Fluids Barriers CNS 15, 1–10. doi: 10.1186/s12987-018-0102-9
Swindell, W. R., Kruse, C. P. S., List, E. O., Berryman, D. E., and Kopchick, J. J. (2019). ALS blood expression profiling identifies new biomarkers, patient subgroups, and evidence for neutrophilia and hypoxia. J. Transl. Med. 17, 170. doi: 10.1186/s12967-019-1909-0
Switońska, K., Szlachcic, W. J., Handschuh, L., Wojciechowski, P., Marczak, Ł., Stelmaszczuk, M., et al. (2019). Identification of altered developmental pathways in human juvenile HD IPSC with 71Q and 109Q using transcriptome profiling. Front. Cell. Neurosci. 12, 528. doi: 10.3389/fncel.2018.00528
Tan, M. M. X., Lawton, M. A., Jabbari, E., Reynolds, R. H., Iwaki, H., Blauwendraat, C., et al. (2021). Genome-wide association studies of cognitive and motor progression in Parkinson's disease. Mov. Disord. 36, 424–433. doi: 10.1002/MDS.28342
Tang, J. L. (2005). Selection bias in meta-analyses of gene-disease associations. PLoS Med. 2, 1226–1227. doi: 10.1371/JOURNAL.PMED.0020409
Taoufik, E., Kouroupi, G., Zygogianni, O., and Matsas, R. (2018). Synaptic dysfunction in neurodegenerative and neurodevelopmental diseases: an overview of induced pluripotent stem-cell-based disease models. Open Biol. 8, 180138. doi: 10.1098/RSOB.180138
Tarr, I. S., McCann, E. P., Benyamin, B., Peters, T. J., Twine, N. A., Zhang, K. Y., et al. (2019). Monozygotic twins and triplets discordant for amyotrophic lateral sclerosis display differential methylation and gene expression. Sci. Rep. 9, 1–17. doi: 10.1038/S41598-019-44765-4
Tosto, G., Fu, H., Vardarajan, B. N., Lee, J. H., Cheng, R., Reyes-Dumeyer, D., et al. (2015). F-Box/LRR-repeat protein 7 is genetically associated with Alzheimer's disease. Ann. Clin. Transl. Neurol. 2, 810–820. doi: 10.1002/acn3.223
Traylor, M., Adib-Samii, P., Harold, D., Dichgans, M., Williams, J., Lewis, C. M., et al. (2016). Shared genetic contribution to ischemic stroke and Alzheimer's disease. Ann. Neurol. 79, 739–747. doi: 10.1002/ana.24621
Tweedie, S., Braschi, B., Gray, K., Jones, T. E. M., Seal, R. L., Yates, B., et al. (2021). Genenames.Org: the HGNC and VGNC resources in 2021. Nucleic Acids Res. 49, D939–D946. doi: 10.1093/NAR/GKAA980
Umoh, M. E., Dammer, E. B., Dai, J., Duong, D. M., Lah, J. J., Levey, A. I., et al. (2018). A proteomic network approach across the ALS - FTD disease spectrum resolves clinical phenotypes and genetic vulnerability in human brain. EMBO Mol. Med. 10, 48–62. doi: 10.15252/emmm.201708202
Urbanek, S., Bibiko, H.-J., and Stefano, M. L. (2014). R: A Language and Environment for Statistical Computing. Vienna: The R Foundation for Statistical Computing.
Vacic, V., Ozelius, L. J., Clark, L. N., Bar-Shira, A., Gana-Weisz, M., Gurevich, T., et al. (2014). Genome-wide mapping of IBD segments in an Ashkenazi PD cohort identifies associated haplotypes. Hum. Mol. Genet. 23, 4693–4702. doi: 10.1093/hmg/ddu158
van Dijk, K. D., Berendse, H. W., Drukarch, B., Fratantoni, S. A., Pham, T. V., Piersma, S. R., et al. (2012). The proteome of the locus ceruleus in Parkinson's disease: relevance to pathogenesis. Brain Pathol. 22, 485–498. doi: 10.1111/j.1750-3639.2011.00540.x
van Es, M. A., Van Vught, P. W., Blauw, H. M., Franke, L., Saris, C. G., Andersen, P. M., et al. (2007). ITPR2 as a susceptibility gene in sporadic amyotrophic lateral sclerosis: a genome-wide association study. Lancet Neurol. 6, 869–877. doi: 10.1016/S1474-4422(07)70222-3
Van Es, M. A., Van Vught, P. W. J., Blauw, H. M., Franke, L., Saris, C. G. J., Van Den Bosch, L., et al. (2008). Genetic variation in DPP6 is associated with susceptibility to amyotrophic lateral sclerosis. Nat. Genet. 40, 29–31. doi: 10.1038/ng.2007.52
Van Es, M. A., Veldink, J. H., Saris, C. G. J., Blauw, H. M., Van Vught, P. W. J., Birve, A., et al. (2009). Genome-wide association study identifies 19p13.3 (UNC13A) and 9p21.2 as susceptibility loci for sporadic amyotrophic lateral sclerosis. Nat. Genet. 41, 1083–1087. doi: 10.1038/ng.442
Van Rheenen, W., Shatunov, A., Dekker, A. M., McLaughlin, R. L., Diekstra, F. P., Pulit, S. L., et al. (2016). Genome-wide association analyses identify new risk variants and the genetic architecture of amyotrophic lateral sclerosis. Nat. Genet. 48, 1043–1048. doi: 10.1038/ng.3622
Varghese, A. M., Sharma, A., Mishra, P., Vijayalakshmi, K., Harsha, H. C., Sathyaprabha, T. N., et al. (2013). Chitotriosidase - a putative biomarker for sporadic amyotrophic lateral sclerosis. Clin. Proteomics. 10, 1–9. doi: 10.1186/1559-0275-10-19
Varshavsky, A. (1997). The N-end rule pathway of protein degradation. Genes Cells 2, 13–28. doi: 10.1046/j.1365-2443.1997.1020301.x
Virtanen, P., Gommers, R., Oliphant, T. E., Haberland, M., Reddy, T., Cournapeau, D., et al. (2020). SciPy 1.0: fundamental algorithms for scientific computing in python. Nat. Methods 17, 261–272. doi: 10.1038/s41592-019-0686-2
von Mering, C., Jensen, L. J., Snel, B., Hooper, S. D., Krupp, M., Foglierini, M., et al. (2005). STRING: known and predicted protein–protein associations, integrated and transferred across organisms. Nucleic Acids Res. 33, D433. doi: 10.1093/NAR/GKI005
Wallen, Z. D., Chen, H., Hill-Burns, E. M., Factor, S. A., Zabetian, C. P., and Payami, H. (2018). Plasticity-related gene 3 (LPPR1) and age at diagnosis of Parkinson disease. Neurol. Genet. 4, e271. doi: 10.1212/nxg.0000000000000271
Wang, C., Chen, L., Zhang, M., Yang, Y., and Wong, G. (2020). PDmethDB: a curated Parkinson's disease associated methylation information database. Comput. Struct. Biotechnol. J. 18, 3745–3749. doi: 10.1016/J.CSBJ.2020.11.015
Wang, H., Lo, M. T., Rosenthal, S. B., Makowski, C., Andreassen, O. A., Salem, R. M., et al. (2021). Similar genetic architecture of Alzheimer's disease and differential APOE effect between sexes. Front. Aging Neurosci. 13, 674318. doi: 10.3389/FNAGI.2021.674318
Wang, L., Yin, Y. L., Liu, X. Z., Shen, P., Zheng, Y. G., Lan, X. R., et al. (2020). Current understanding of metal ions in the pathogenesis of Alzheimer's disease. Transl. Neurodegener. 9, 1–13. doi: 10.1186/S40035-020-00189-Z
Wang, S., and El-Deiry, W. S. (2003). TRAIL and apoptosis induction by TNF-family death receptors. Oncogene 22, 8628–8633. doi: 10.1038/SJ.ONC.1207232
Wang, X., Lopez, O., Sweet, R. A., Becker, J. T., Dekosky, S. T., Barmada, M. M., et al. (2015). Genetic determinants of survival in patients with Alzheimer's disease. J. Alzheimer's Dis. 45, 651–658. doi: 10.3233/JAD-142442
Wang, Y., and Tjandra, N. (2013). Structural insights of TBid, the caspase-8-activated Bid, and its BH3 domain. J. Biol. Chem. 288, 35840–35851. doi: 10.1074/JBC.M113.503680
Watanabe, H., Atsuta, N., Hirakawa, A., Nakamura, R., Nakatochi, M., Ishigaki, S., et al. (2016). A rapid functional decline type of amyotrophic lateral sclerosis is linked to low expression of TTN. J. Neurol. Neurosurg. Psychiatry 87, 851–858. doi: 10.1136/JNNP-2015-311541
Watson, C. T., Roussos, P., Garg, P., Ho, D. J., Azam, N., Katsel, P. L., et al. (2016). Genome-wide12 DNA methylation profiling in the superior temporal gyrus reveals epigenetic signatures associated with Alzheimer's disease. Genome Med. 8, 1–14. doi: 10.1186/S13073-015-0258-8/FIGURES/1
Watson, J., Hopkins, N., Roberts, J., and Steitz, J. (1988). Molecular biology of the gene. Int. J. Biochem. 20, 1457. doi: 10.1016/s0020-711x(98)90017-9
Webster, J. A., Myers, A. J., Pearson, J. V., Craig, D. W., Hu-Lince, D., Coon, K. D., et al. (2008). Sorl1 as an Alzheimer's disease predisposition gene? Neurodegener. Dis. 5, 60–64. doi: 10.1159/000110789
Wei, L., Tian, Y., Chen, Y., Wei, Q., Chen, F., Cao, B., et al. (2019). Identification of TYW3/CRYZ and FGD4 as susceptibility genes for amyotrophic lateral sclerosis. Neurol. Genet. 5. doi: 10.1212/NXG.0000000000000375
Wiatr, K., Szlachcic, W. J., Trzeciak, M., Figlerowicz, M., and Figiel, M. (2018). Huntington disease as a neurodevelopmental disorder and early signs of the disease in stem cells. Mol. Neurobiol. 55, 3351. doi: 10.1007/S12035-017-0477-7
Wightman, D. P., Jansen, I. E., Savage, J. E., Shadrin, A. A., Bahrami, S., Holland, D., et al. (2021). A genome-wide association study with 1,126,563 individuals identifies new risk loci for Alzheimer's disease. Nat. Genet. 53, 1276–1282. doi: 10.1038/S41588-021-00921-Z
Wijsman, E. M., Pankratz, N. D., Choi, Y., Rothstein, J. H., Faber, K. M., Cheng, R., et al. (2011). Genome-wide association of familial late-onset Alzheimer's disease replicates BIN1 and CLU and nominates CUGBP2 in interaction with APOE. PLoS Genet. 7, e1001308. doi: 10.1371/journal.pgen.1001308
Wingo, A. P., Fan, W., Duong, D. M., Gerasimov, E. S., Dammer, E. B., Liu, Y., et al. (2020). Shared proteomic effects of cerebral atherosclerosis and Alzheimer's disease on the human brain. Nat. Neurosci. 23, 696–700. doi: 10.1038/s41593-020-0635-5
Wong, Y. C., and Holzbaur, E. L. F. (2014). The regulation of autophagosome dynamics by Huntingtin and HAP1 is disrupted by expression of mutant Huntingtin, leading to defective cargo degradation. J. Neurosci. 34, 1293. doi: 10.1523/JNEUROSCI.1870-13.2014
Wozniak, M. A., Modzelewska, K., Kwong, L., and Keely, P. J. (2004). Focal adhesion regulation of cell behavior. Biochim. Biophys. Acta Mol. Cell Res. 1692, 103–119. doi: 10.1016/J.BBAMCR.2004.04.007
Xie, T., Deng, L., Mei, P., Zhou, Y., Wang, B., Zhang, J., et al. (2014). A genome-wide association study combining pathway analysis for typical sporadic amyotrophic lateral sclerosis in Chinese Han populations. Neurobiol. Aging 35, 1778.e9–e23. doi: 10.1016/J.NEUROBIOLAGING.2014.01.014
Xie, Z., and Klionsky, D. J. (2007). Autophagosome formation: core machinery and adaptations. Nat. Cell Biol. 9, 1102–1109. doi: 10.1038/NCB1007-1102
Xu, J., Jakher, Y., and Ahrens-Nicklas, R. C. (2020). Brain branched-chain amino acids in maple syrup urine disease: implications for neurological disorders. Int. J. Mol. Sci. 21, 7490. doi: 10.3390/IJMS21207490
Xu, W., Tan, L., and Yu, J. T. (2014). The role of PICALM in Alzheimer's disease. Mol. Neurobiol. 52, 399–413. doi: 10.1007/S12035-014-8878-3
Yashin, A. I., Fang, F., Kovtun, M., Wu, D., Duan, M., Arbeev, K., et al. (2018). Hidden heterogeneity in Alzheimer's disease: insights from genetic association studies and other analyses. Exp. Gerontol. 107, 148–160. doi: 10.1016/j.exger.2017.10.020
Young, J. I., Sivasankaran, S. K., Wang, L., Ali, A., Mehta, A., Davis, D. A., et al. (2019). Genome-wide brain DNA methylation analysis suggests epigenetic reprogramming in Parkinson disease. Neurol Genet. 5. doi: 10.1212/NXG.0000000000000342
Young, P. E., Jew, S. K., Buckland, M. E., Pamphlett, R., and Suter, C. M. (2017). Epigenetic differences between monozygotic twins discordant for amyotrophic lateral sclerosis (ALS) provide clues to disease pathogenesis. PLoS ONE 12, e0182638. doi: 10.1371/JOURNAL.PONE.0182638
Zadel, M., Maver, A., Kovanda, A., and Peterlin, B. (2018). DNA methylation profiles in whole blood of Huntington's disease patients. Front. Neurol. 9, 655. doi: 10.3389/FNEUR.2018.00655
Zarei, S., Carr, K., Reiley, L., Diaz, K., Guerra, O., Altamirano, P. F., et al. (2015). A comprehensive review of amyotrophic lateral sclerosis. Surg. Neurol. Int. 6. doi: 10.4103/2152-7806.169561
Zhang, B., and Horvath, S. (2005). A general framework for weighted gene co-expression network analysis. Stat. Appl. Genet. Mol. Biol. 4. doi: 10.2202/1544-6115.1128
Zhang, L., Deng, J., Pan, Q., Zhan, Y., Fan, J. B., Zhang, K., et al. (2016). Targeted Methylation sequencing reveals dysregulated Wnt signaling in Parkinson disease. J. Genet. Genom. 43, 587–592. doi: 10.1016/J.JGG.2016.05.002
Zhang, Q., Ma, C., Gearing, M., Wang, P. G., Chin, L. S., and Li, L. (2018). Integrated proteomics and network analysis identifies protein hubs and network alterations in Alzheimer's disease. Acta Neuropathol. Commun. 6, 19. doi: 10.1186/s40478-018-0524-2
Zhang, Y., James, M., Middleton, F. A., and Davis, R. L. (2005). Transcriptional analysis of multiple brain regions in Parkinson's disease supports the involvement of specific protein processing, energy metabolism, and signaling pathways, and suggests novel disease mechanisms. Am. J. Med. Genet. Neuropsychiatric Genet. 137 B, 5–16. doi: 10.1002/ajmg.b.30195
Zhou, Z., Cheng, Y., Jiang, Y., Liu, S., Zhang, M., Liu, J., et al. (2018). Ten hub genes associated with progression and prognosis of pancreatic carcinoma identified by co-expression analysis. Int. J. Biol. Sci. 14, 124. doi: 10.7150/IJBS.22619
Keywords: neurodegenerative diseases, metastudy, multi-omic analyses, bioinformatics, hub genes and pathways
Citation: Ruffini N, Klingenberg S, Heese R, Schweiger S and Gerber S (2022) The Big Picture of Neurodegeneration: A Meta Study to Extract the Essential Evidence on Neurodegenerative Diseases in a Network-Based Approach. Front. Aging Neurosci. 14:866886. doi: 10.3389/fnagi.2022.866886
Received: 31 January 2022; Accepted: 13 May 2022;
Published: 27 June 2022.
Edited by:
Shu Wen, Baylor College of Medicine, United StatesReviewed by:
Weiwei Dai, Johns Hopkins Medicine, United StatesXuan Chen, Tufts University, United States
Copyright © 2022 Ruffini, Klingenberg, Heese, Schweiger and Gerber. This is an open-access article distributed under the terms of the Creative Commons Attribution License (CC BY). The use, distribution or reproduction in other forums is permitted, provided the original author(s) and the copyright owner(s) are credited and that the original publication in this journal is cited, in accordance with accepted academic practice. No use, distribution or reproduction is permitted which does not comply with these terms.
*Correspondence: Susanne Gerber, c3VnZXJiZXImI3gwMDA0MDt1bmktbWFpbnouZGU=
†These authors have contributed equally to this work