- 1Ethel Percy Andrus Gerontology Center, Leonard Davis School of Gerontology, University of Southern California, Los Angeles, CA, United States
- 2Corwin D. Denney Research Center, Department of Biomedical Engineering, Viterbi School of Engineering, University of Southern California, Los Angeles, CA, United States
Neural and cognitive deficits after mild traumatic brain injury (mTBI) are paralleled by changes in resting state functional correlation (FC) networks that mirror post-traumatic pathophysiology effects on functional outcomes. Using functional magnetic resonance images acquired both acutely and chronically after injury (∼1 week and ∼6 months post-injury, respectively), we map post-traumatic FC changes across 136 participants aged 19–79 (52 females), both within and between the brain’s seven canonical FC networks: default mode, dorsal attention, frontoparietal, limbic, somatomotor, ventral attention, and visual. Significant sex-dependent FC changes are identified between (A) visual and limbic, and between (B) default mode and somatomotor networks. These changes are significantly associated with specific functional recovery patterns across all cognitive domains (p < 0.05, corrected). Changes in FC between default mode, somatomotor, and ventral attention networks, on the one hand, and both temporal and occipital regions, on the other hand, differ significantly by age group (p < 0.05, corrected), and are paralleled by significant sex differences in cognitive recovery independently of age at injury (p < 0.05, corrected). Whereas females’ networks typically feature both significant (p < 0.036, corrected) and insignificant FC changes, males more often exhibit significant FC decreases between networks (e.g., between dorsal attention and limbic, visual and limbic, default-mode and somatomotor networks, p < 0.0001, corrected), all such changes being accompanied by significantly weaker recovery of cognitive function in males, particularly older ones (p < 0.05, corrected). No significant FC changes were found across 35 healthy controls aged 66–92 (20 females). Thus, male sex and older age at injury are risk factors for significant FC alterations whose patterns underlie post-traumatic cognitive deficits. This is the first study to map, systematically, how mTBI impacts FC between major human functional networks.
Introduction
Traumatic brain injury (TBI) ranks high among public health challenges in the United States partly due to its relatively high incidence (∼0.1% annually), to its potentially debilitating sequelae, and to its relatively long recovery periods (de la Plata et al., 2008). Recuperation and clinical outcomes after TBI vary substantially by age and sex (de la Plata et al., 2008). Age at injury and biological sex have been proposed to impact clinical outcome and pathophysiology significantly (LeBlanc et al., 2006; Gölz et al., 2019), although the available evidence on sex effects is equivocal (Caplan et al., 2017).
During wakeful rest, brain activity mapped by functional magnetic resonance imaging (fMRI) is organized within resting state networks (RSNs) of functionally coupled cortical regions (Irimia and Van Horn, 2015). Yeo et al. (2011) confirmed the existence of seven canonical RSNs: the visual, somatomotor, dorsal attention, ventral attention, limbic, frontoparietal, and default mode networks [visual network (VN), somatomotor network (SMN), dorsal attention network (DAN), ventral attention network (VAN), limbic network (LN), frontoparietal network (FPN), and default mode network (DMN), respectively]. These authors illustrated how RS brain activity patterns can further be classified into 17 more granular RSNs (N1 to N17 in our notation) that arise naturally as hierarchical subdivisions of the seven canonical RSNs. Specifically, Yeo et al. (2011) implemented a sophisticated and data-driven procedure to cluster RSNs hierarchically without a priori assumptions. This allowed these authors to provide extensive evidence on the hierarchical clustering of the RSNs. Across individuals, both RSN classification schemes are consistent in their neuroanatomy and in how conditions like TBI affect them (Yeo et al., 2011; Badhwar et al., 2017; Kawabata et al., 2018; Irimia et al., 2020).
The TBI-related abnormalities have been reported in all seven major RSNs. For example, abnormal DMN connectivity changes can inform models of cognitive impairment and of neurodegeneration along trajectories that can lead to neurodegenerative diseases (Irimia et al., 2020). fMRI studies of TBI (Stevens et al., 2012) have identified functional correlation (FC) changes involving the FPN (Scolari et al., 2015), whose post-traumatic connectomic abnormalities can be associated with specific cognitive deficits (Hillary et al., 2011; Kasahara et al., 2011). Post-traumatic FC abnormalities also include reduced connectivity of the VN to other brain regions, even in cognitively normal and in behaviorally unimpaired individuals (Slobounov et al., 2011). TBI-related disruptions of the DAN have also been reported, particularly involving long-range and interhemispheric information exchange (Han et al., 2016). Similarly, in mTBI-affected athletes, abnormal increases in FC in the VAN have been reported (Borich et al., 2015), and related to attention deficits (Kramer et al., 2008). Similarly, the LN may undergo significant post-traumatic FC increases (Stevens et al., 2012) paralleled by decreased WM integrity (Zhu et al., 2014; Santhanam et al., 2019). Finally, altered FC between the SMN to the supplementary motor area (N7), on the one hand, and to other brain regions, on the other hand, have been reported after TBI (Han et al., 2016). Thus, cross-sectional findings on TBI-related FC abnormalities have been explored extensively.
In this study, we extend the state of the art by considering the important topic of change in FC after mTBI as this pertains to cortical RSNs. Because post-traumatic degradation of cognitive functions is often paralleled by changes in FC between RSNs (Sharp et al., 2011), quantifying such changes can provide insight into the functional substrates and anatomic profiles of neural dysfunctions associated with post-traumatic deficits. Previous fMRI studies of TBI-related RSN changes describe how participants’ FC patterns differ from those of healthy controls (HCs) (Shumskaya et al., 2012; Vakhtin et al., 2013; Rigon et al., 2016a; Vergara et al., 2017). However, hardly any study has quantified either (A) post-traumatic FC changes, (B) how age and sex modulate these changes (de Souza et al., 2020), or (C) how the changes are related to cognitive function recovery. Such understanding could help to evaluate injury-related functional alterations occurring in parallel with structural changes involving white matter (WM) degradation, which frequently underlies cognitive degradation and/or recovery. This study leverages general linear models (GLMs) of blood oxygenation level-dependent (BOLD) fMRI signals to quantify (A) mTBI-related changes in FC between RSNs within the first ∼6 months post-injury, and (B) how age at injury and sex modulate these changes. Because studying functional interactions between cortical and non-cortical networks is sufficiently complex and difficult to warrant a separate study, we here limit ourselves to the investigation of cortical networks only. To our knowledge, this is the first systematic longitudinal study to map such post-traumatic changes occurring between the acute and chronic stages of TBI. It is also the first study to map how these changes are reflected by cognitive recovery. The acute and chronic stages of TBI overlap with the approximate timeframe of the most dynamic post-traumatic brain changes pertaining to functional reorganization, degradation, and recovery. For this reason, quantifying RSN alterations between these stages is relevant to the characterization of post-traumatic neuropathophysiology underlying cognitive function dynamics after TBI.
Materials and Methods
Participants
This study was approved by the Institutional Review Board at the University of Southern California and was carried out in accordance with the United States Code of Federal Regulations (45 C.F.R. 46) and with the Declaration of Helsinki. Participants were recruited through community outreach (e.g., using advertisements and flyers) and/or through healthcare professionals who had referred volunteers for neurocognitive assessments and neuroimaging. All subjects who satisfied the inclusion criteria and who could provide written informed consent were invited to participate. Participants included 136 individuals with mTBI [N = 136; 52 females; age range: 19–79 years (y), 72 participants below age 40 years; age mean μ = 42 years, standard deviation σ = 17 years, Table 1]. To reduce recruitment bias, all individuals satisfying the study’s inclusion criteria were invited to participate and all those who did also provided written informed consent. Inclusion criteria were (a) a TBI diagnosis due to a ground-level fall involving direct head trauma; (b) a Glasgow Coma Scale score above 12 upon initial clinical evaluation (μ ± σ = 14 ± 1); (c) loss of consciousness shorter than 30 min (μ ± σ ≃ 9 ± 4 min); (d) post-traumatic amnesia of less than 24 h (μ ± σ ≃ 3.6 ± 2.1 h); (e) availability of T1-weighted MRI scans acquired both acutely and chronically after the injury, i.e., within ∼7 days and ∼6 months (μ ± σ = 5.6 ± 0.3 months), respectively, and (f) no gross TBI pathology findings on clinical MRIs. Exclusion criteria were (a) a pre-traumatic history of cognitive impairment, neurological and/or psychiatric disease, and (b) a history of psychotropic substance abuse. This retrospective study did not involve any therapeutic intervention common across all mTBI participants. A group of 35 HCs whose fMRIs had been acquired at two timepoints were selected from the Alzheimer’s Disease Neuroimaging Initiative (ADNI), whose inclusion criteria are described elsewhere (Petersen et al., 2010).
Neuroimaging and Cognitive Assessments
T1-weighted MRIs were acquired from mTBI participants using a magnetization-prepared rapid acquisition gradient echo sequence with the following parameters: repetition time = 1.95 s; echo time = 2.98 ms; inversion time = 900 ms; voxel size = 1 mm × 1 mm × 1 mm. BOLD RS fMRI time series with at least 140 volumes per session were acquired (repetition time = 3 s, echo time = 30 ms, flip angle = 80 degrees, voxel size = 3.3125 mm × 3.3125 mm × 3.3 mm, acquisition matrix = 64 × 64 × 49). All data were anonymized and de-linked. HC subjects’ T1-weighted and functional MRIs were obtained from the ADNI database1. ADNI was launched in 2003 as a public-private partnership, led by Principal Investigator Michael W. Weiner, MD. The primary goal of ADNI has been to test whether serial magnetic resonance imaging (MRI), positron emission tomography (PET), other biological markers, and clinical and neuropsychological assessment can be combined to measure the progression of mild cognitive impairment (MCI) and early AD. Cognition was evaluated at the two timepoints using the Brief Test of Adult Cognition by Telephone (BTACT) (Tun and Lachman, 2006; Kliegel et al., 2007). BTACT measures episodic verbal memory [immediate recall (EVMI) and delayed recall (EVMD) of words on a 15-item list], working memory span (WMS, assessed using a backward digit span task), inductive reasoning (IR, assessed using a number series completion task), processing speed (PS, measured using a backward counting task) and verbal fluency (VF, assessed using a category fluency task). The BTACT is a phone-based cognitive assessment, so administering the BTACT is logistically convenient because participants do not have to be interviewed in person. The BTACT is also reliable, as it has been extensively validated against face-to-face assessments and against longer cognitive assessments, including the 90-min in-person Boston cognitive battery (Lachman et al., 2014). p-values for statistical tests involving cognitive assessments were corrected for multiple comparisons using the Benjamini–Hochberg procedure with a false discover rate (FDR) of 0.05 (Hochberg and Benjamini, 1990).
Preprocessing
T1 MRIs were segmented using FreeSurfer 6.0 (FS2) with default parameters (Dale et al., 1999; Fischl, 2012). FS (a) removes non-cerebral voxels, (b) transforms volumes into Talairach space, (c) normalizes signal intensities across voxels, (d) segments the gray matter, (e) tessellates the gray matter/white matter boundary, and (f) corrects surface topology. fMRI preprocessing was undertaken using the FreeSurfer Functional Analysis Stream (FS-FAST3) with default parameters. The stream includes frame-to-frame motion correction, frame censoring, frequency filtering for removal of scanner and physiological noise, brain masking, intensity normalization, co-registration between T1 and fMRI volumes, FS atlas surface sampling, smoothing using a kernel with a full width at half maximum of 5 mm, and volume resampling to the Montreal Neurological Institute’s regional parcellation space generated using data from 305 subjects. Nuisance timeseries due to motion, WM, and cerebrospinal fluid were regressed out and the first four volumes in each time series were removed for signal equilibration. Within a general linear model, FS-FAST was used to calculate the main effects of sex and age, as well as their interaction, on changes in FC between each of the 17 functional networks (seeds) and the rest of the cortex. Importantly, by default, FS-FAST implements a correction for multiple comparisons during the procedure for identifying statistically significant clusters. This default option for multiple comparison correction was used throughout this study.
Functional Correlation Network Parcellation and Seeds
Functional correlation seeds were identical to those defined by Yeo et al. (2011), who had assigned each cortical region to one of seven canonical RSNs (DMN, DAN, VAN, FPN, LN, SMN, or VN, Figure 1A). Although these networks consist of non-contiguous cortical parcels, most such parcels within each RSN exhibit significantly intercorrelated RS fMRI signals, whence their grouping into RSNs. The seven canonical RSNs can be hierarchically subdivided into 17 more granular networks Ni where i = 1, …, 17 (Figure 1B). These 17 networks map onto the seven canonical RSNs approximately as follows: N1 and N2 map onto the VN, N3 and N4 onto the SMN, N5 and N6 onto the DAN, N7 and N8 onto the VAN, N9 and N10 onto the LN, N8, N12 and N13 onto the FPN, N16 and N17 onto the DMN (Figure 2). Because the 17-network parcellation scheme is more anatomically granular, each of its RSNs was used as a seed in the FC analysis. In the remainder of this subsection, each RSN and its functions are described to facilitate conceptual interpretation.
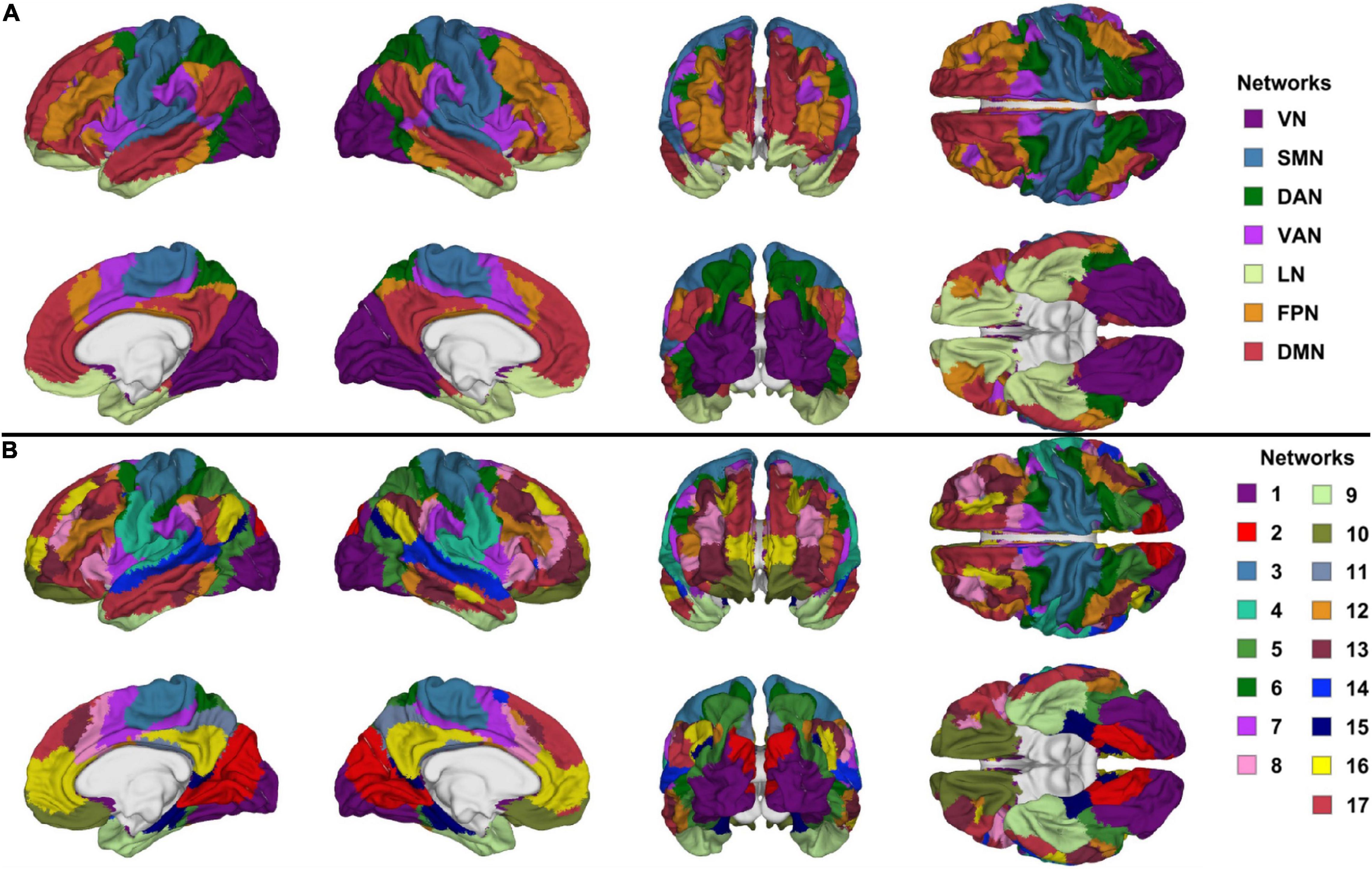
Figure 1. Canonical views (left, right, anterior, posterior, dorsal, ventral, and medial) of (A) the seven canonical RSNs and (B) the 17-network RSNs delineated by Yeo et al. (2011). The color scheme and nomenclature are those used in the original publication (Yeo et al., 2011). RSN, resting state network.
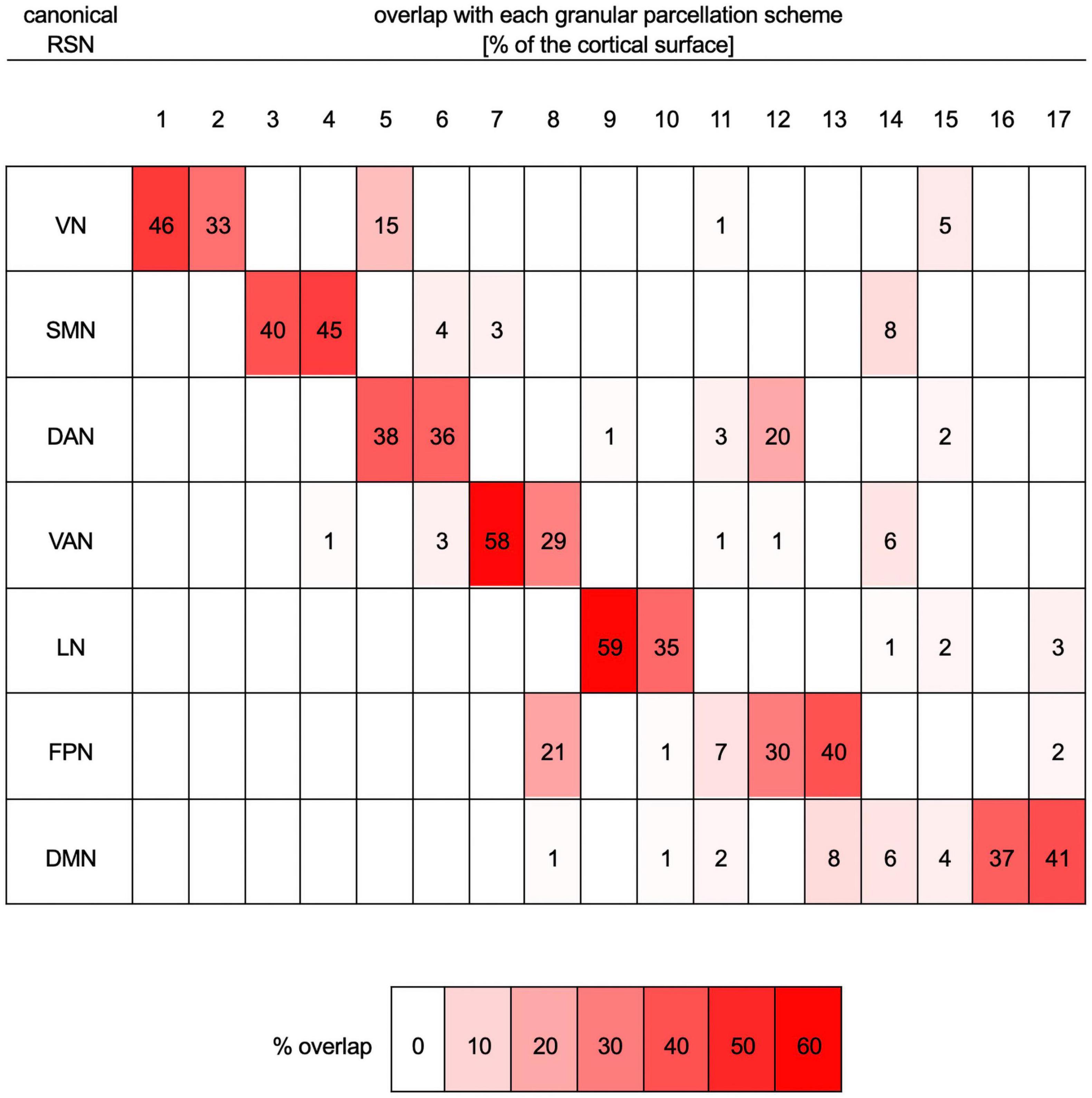
Figure 2. Quantitative mapping of overlaps between the parcellation scheme with seven RSNs onto the more granular parcellation scheme with 17 RSNs. Both schemes are those of Yeo et al. (2011). Each row lists data pertaining to one of the seven canonical networks (VN, SMN, DAN, VAN, LN, FPN, and DMN). Columns list data corresponding to one of the networks (N1 to N17) in the more granular 17-network cortical parcellation scheme. For each row (i.e., RSN), the table indicates the percentage of that RSN’s cortical surface area, in the seven-network scheme, that overlaps with each of the more granular networks in the 17-network scheme. For example, 46% of the VN overlaps with N1, 33% of it overlaps with N2, 15% with N5, 1% with N11, and 5% with N15. For convenience, percentages are also color-coded, where white indicates 0% overlap and bright red indicates maximum overlap (59%). DAN, dorsal attention network; DMN, default mode network; FPN, frontoparietal network; LN, limbic network; RSN, resting state network; SMN, somatomotor network; VAN, ventral attention network; VN, visual network.
The VN’s primary role is in processing visual information (DeAngelis et al., 1995; Culham et al., 2001), and TBI can lead to visual deficits underpinned by VN dysfunction (Palacios et al., 2017). The VN fractionates into two subnetworks comprising central (N1) and peripheral visual (N2) areas, respectively, divided across by the calcarine fissure. N1 spans the lateral and the posterior part of the medial occipital lobe (i.e., most of the primary visual cortex, V1), whereas N2 comprises other visual areas (i.e., V2–V5). Thus, the VN contains neuronal populations attuned to detecting visual features (across both N1 and N2), object motion (N2), as well as the speed and direction of such motion (e.g., visual area V5, which is part of N2) (Dubner and Zeki, 1971; Maunsell and Van Essen, 1983). The superior temporal gyrus/auditory cortex is a part of the visuospatial attentional system which, along with the VN, is engaged in exogenous orientation to verbal instructions to perform a visual task (Mayer et al., 2004; Halgren et al., 2011).
The SMN is subdivided by separating the superior and inferior portions of the precentral gyrus (PCG) and postcentral gyrus (PoCG) by a line proceeding, in anteroposterior fashion, along the crown of the middle frontal gyrus (MFG). This produces one dorsal and one ventral network (N3 and N4, respectively), reflecting a functional subdivision of somatomotor representation areas. Insights facilitated by the 17-network parcellation help to conceptualize post-traumatic somatomotor deficits as reflecting changes in the FCs of highly specialized somatomotor representation areas. Specifically, N3 (which includes the superior dorsolateral aspects of the PCG and PoCG), is responsible for proprioceptive sensation and for the control of hands and fingers, whereas N4 (which includes the inferior aspects of the PCG and PoCG, the posterior aspect of the insula and the temporal plane), processes sensory information from the face, eyes, nose, mouth, tongue, and jaws (Grodd et al., 2001). Wernicke’s area (N4) is associated with both visual and auditory language comprehension (Binder et al., 1997). FC between the SMN (N3) and the superior frontal gyrus (SFG) is implicated in inhibitory control during no-go tasks (Nakata et al., 2009). This may partly explain why TBI patients often experience behavioral impulsivity and reduced inhibitory control (Dimoska-Di Marco et al., 2011).
The DAN is a task-positive network partly responsible for visuospatial attention (Umarova et al., 2010). The DAN contains one anterior and one posterior subnetwork (N5 and N6, respectively, Figure 2). The anterior subnetwork of the DAN (N5) comprises the anterior aspects of the inferior occipital gyrus and sulcus, the lateral occipito-temporal gyrus, the lingual part of the medial occipito-temporal gyrus, and the superior parietal lobule. The posterior subnetwork of the DAN (N6) includes posterior portions of the MFG, as well as the supramarginal gyrus, the superior parietal lobe, the precentral sulci, and the precuneus (PCun). The DAN can act in concert with the VAN, which is involved in redirecting attention to novel stimuli of behavioral relevance (Corbetta and Shulman, 2002; La et al., 2014). The fusiform gyrus (N5) is involved in high-level visual computations undertaken during face perception, object recognition, and reading (Weiner and Zilles, 2016). Worse performance on tasks related to these cognitive functions has been reported after TBI (Alnawmasi et al., 2019; Turkstra et al., 2020).
The VAN consists of two subnetworks (N7 and N8) extending across the anterior insula and parts of the frontal lobe. N7 areas are adjacent and posterior to N8 and include the SFG, portions of middle cingulate and supramarginal cortices, as well as the anterior opercular part of the inferior frontal gyrus. N8 encompasses the orbital and triangular parts of the inferior frontal gyrus, as well as the MFG, SFG, and supramarginal gyri. Specifically, the VAN specializes in detecting (A) unexpected and unattended stimuli, and (B) selecting a stimulus upon which the DAN should focus its neural processing capabilities related to sustained attention (Vossel et al., 2014). In right-handed individuals, the opercular and triangular parts of the inferior frontal gyrus together form the right-hemisphere homolog of Broca’s area (N7), which is activated by relatively complex tasks involving speech processing and word retrieval (Just et al., 1996; Drager et al., 2004; Papoutsi et al., 2009; Schremm et al., 2018). One year after TBI, patients often exhibit deficits in their voluntary control of attention, and these deficits are correlated with reductions in FC between the VAN and other regions, relative to HCs (Richard et al., 2018).
The LN consists of two subnetworks, one temporal and another frontal (N9 and N10, respectively). Deficits pertaining to the recognition of faces and facial expressions, both of which involve the LN, are more prevalent after TBI than after brain tumors or stroke by 20 and 77%, respectively (Prigatano and Pribram, 1982; Valentine et al., 2006; Knox and Douglas, 2009).
The FPN is partitioned into N12 [comprising the inferior frontal sulcus, inferior frontal gyrus, MFG, and posterior aspect of the middle temporal gyrus (MTG)] and N13 (comprising the inferior temporal sulcus, MTG, medial aspect of the SFG, superior frontal and lateral orbital sulci, as well as parts of the angular and supramarginal gyri). The cortical regions belonging to N12 and N13 are typically adjacent. Thus, N12 includes the MFG, parts of the postcentral sulci, and parts of the inferior temporal gyri (posterior aspects). N13 includes parts of the MFG, lateral orbital sulci, postcentral sulci, inferior temporal gyri, and small parts of the SFG (medial aspects). N16 overlaps with the anterior cingulate cortex, PCun, the ventral aspects of supramarginal gyri, and with part of the anterior aspect of the MTG. FC degradation in the MTG is associated with cognitive decline after injury in males (Konstantinou et al., 2018).
The DMN includes N16,which overlaps with the anterior cingulate cortex, PCun, the ventral aspects of supramarginal gyri, and part of the anterior aspect of the MTG; and N17, which contains the SFG, MTG, superior frontal sulcus, and the angular gyrus.
Theoretical Framework for Sex and Age Differences
Our methodological approach relies on a theoretical framework for age- and sex-related differences in mTBI-related changes in FC that emerges from the functional neuroanatomy literature. This framework has not been expounded adequately before, yet its specifications are important for understanding the rationale of our approach and for the interpretations of our results. For these reasons, this theoretical framework is synthesized in what follows. Thus, age-related decline in cognitive performance is related to cortical thinning and related functional plasticity to compensate for this thinning (Greenwood, 2007). Older age has been associated with reduction in FC; for example, in older adults, Wang et al. (2010) found both a decline in frontotemporal and temporoparietal FC, as well as an increase in DMN FC during memory encoding and recognition.
The relationship between cognitive functions and FC is also modulated by sex, although a comprehensive theoretical framework explaining these differences has not been established. Male mTBI patients have been found to exhibit decreased FC in the VN compared to females, as well as increased FC across multiple networks, including an executive function-related network associated with insomnia severity (Wang et al., 2018). Functions of the PCun that involve the VN pertain to the processing of visuospatial imagery and to episodic memory retrieval (Cavanna and Trimble, 2006), both of which are often affected by TBI (Kwon and Jang, 2011; Gillis and Hampstead, 2015; Gilmore et al., 2016). PCun (N2) involvement in these functions is also modulated by sex (Butler et al., 2006; Zilles et al., 2016).
In terms of the SMN, meta-analytic findings on sex-related differences pertaining to deficits of motor function and working memory are inconclusive (Gupte et al., 2019). HC females typically achieve better inhibitory control during no-go tasks (Li et al., 2006), and males’ exhibit reduced inhibitory control manifested as willful hand stillness during motor tasks (Nakata et al., 2009). The intrinsic FC of areas recruited by motor function is affected by TBI in a sex-dependent manner (Wang et al., 2018). The supplementary motor area (N3) is likely involved in motor performance, particularly motor control (Goldberg, 1985), and TBI patients often suffer from motor deficits (Kuhtz-Buschbeck et al., 2003; Choi et al., 2012). Post-TBI, females tend to score worse than men on motor skill tests (Moen et al., 2014). Females typically demonstrate fewer somatosensory deficits following mTBI compared to males indicating sex differences in somatosensory deficit severity (Covassin et al., 2006; Bay et al., 2009). Some of these clinical observations (Irimia and Bradshaw, 2005a,b) have been attributed to females’ greater neuroprotective immune responses after TBI, which may be due to sex differences in hormone levels and endocrine function after injury (Stein, 2008). Sex-related differences in somatosensation (whose processing is localized to the PoCG) are modulated by the estrous cycle, which also predicates sex differences in neural plasticity (Alexander et al., 2018).
One of the clusters whose post-traumatic FC to the DAN (N5) changes in a sex-dependent manner overlaps, to a large extent, with the fusiform gyrus. Poorer performance during face perception, object recognition, and reading has been reported in male TBI patients (Moore et al., 2010; Wang et al., 2018). Hypoconnectivity between the VAN and occipital cortex underlies attention deficits (Farrant and Uddin, 2016), and females’ ability to sustain attention is typically greater in the chronic stages of TBI (Ratcliff et al., 2007).
Functional correlation between the LN (N9) and the lingual gyrus is directly involved in face recognition (Kanwisher et al., 1997; Rotshtein et al., 2001), which is involved in the Diagnostic Analysis of Nonverbal Accuracy task (on which males perform significantly better) (Nowicki and Carton, 1993; Babbage et al., 2018). The superior parietal lobule, which overlaps with N3 and is involved in language processing, has altered FC with the FPN after TBI in a sex-dependent manner (Segal and Petrides, 2012; Banaszkiewicz et al., 2021). After injury, this structure undergoes BOLD signal reductions, which translate into altered FC between the superior parietal lobule and other regions (Sanchez-Carrion et al., 2008).
The inferior parietal lobule (IPL) deserves individual treatment here due to its well-documented and important sexual dimorphism (Frederikse et al., 1999), which may be responsible for sex differences in visuospatial ability (Culham and Kanwisher, 2001; Zago and Tzourio-Mazoyer, 2002). Emotion perception is among the primary functions of the IPL, which overlaps with N1, N5, N15, and N16 (Engelen et al., 2015). Following injury, the ability to perceive emotions evoked by facial and by other stimuli tends to degrade (Green et al., 2004; Bornhofen and McDonald, 2008a,b,c). Furthermore, females exhibit weaker deficits in emotion perception after injury (Rigon et al., 2016b). The connectome hub localized within the IPL is among the largest in the connectome; aside from emotion processing, the IPL is also involved in language production (Barbeau et al., 2017; Southwell et al., 2017; Jiao et al., 2020), which can be affected by TBI (DePompei and Hotz, 2001; Steel et al., 2017; Koebli et al., 2020) in a sex-dependent manner. Specifically, after injury, females typically preserve their overall language abilities better than males (Covassin and Bay, 2012; Eramudugolla et al., 2014).
Functional Correlation Analysis
Within FS-FAST, a weighted least-squares GLM implemented by the FS mri_glmfit function was used to identify cortical areas exhibiting significant FC to each seed RSN. Such areas consisted of one or more contiguous cortical surface clusters whose overall BOLD signals had statistically significant partial correlations ρ with the BOLD signal of the seed RSN, both at the acute (ρA) and chronic (ρC) timepoints (null hypothesis: ρA = ρC). The correlation values were compared across timepoints over the entire cortical surface, rather than only across clusters of significant correlations identified by the BOLD signal analysis. The FS-FAST mri_glmfit-sim function was used to evaluate cluster-wise statistical significance using the –cwp flag. Multiple comparison correction was implemented using –3 spaces flag. We chose the partial correlation coefficient ρ rather than the standard contrast effect size as a measure of FC because ρ ranges from −1 to 1, thus making interpretations and comparisons more straightforward for our purposes. This choice does not affect the results of the study because FS-FAST takes into account both when calculating statistical significance. Here and throughout, the effect sizes discussed are those pertaining to partial correlations ρ, i.e., to FCs. Let ρF and ρM denote the partial correlation coefficients of females and males, respectively. Similarly, let ρO and ρY denote the partial correlation coefficients of older adults (OAs) and younger adults (YAs), i.e., between adults younger vs. older than 40 years, respectively. There are three effect size types considered in this study: (A) the difference ΔρT = ρC−ρA, (quantifying FC changes with time), (B) the difference ΔρS = ρF−ρM (quantifying differences between sexes), and (C) the difference ΔρA = ρO−ρY (quantifying FC differences between age groups). Effect sizes were calculated for each subject, and all subjects’ timeseries were then concatenated for group-level statistical analysis. Significant group differences between YAs and OAs and group differences between sexes were identified within the same GLM. The age cutoff of 40 years was selected partly for convenience, as this cutoff splits our sample roughly into half. Testing null hypotheses of group contrasts allowed us to identify ρ values that differed significantly across groups. Δp-values were computed clusterwise (i.e., at the cluster rather than vertex level). To facilitate reporting and tabulation, group-wise averages of partial correlations were calculated across clusters and effect sizes were reported using Cohen’s d multiplied by either −1 or +1, depending on whether the effect was associated with a decrease or increase in FC, respectively. Thus, for example, d = −0.2 indicates an FC decrease with an effect size of 0.2; analogously, d = +0.5 indicates an FC increase with an effect size of 0.5. To relate FCs to cognitive measures, the maximum absolute value of Δρ was first identified for each subject within each of that subject’s spatial cluster of statistical significance. In other words, for each cluster of significance that had been identified across all subjects, the peak effect size Δρmax was first identified within each cluster and for each subject. Pearson’s product moment correlation coefficient between each maximum effect size Δρmax and each cognitive score (EVMI, EVMD, WMS, IR, and PS) was then calculated, and its statistical significance was tested using Student’s t-test with N-2 degrees of freedom, N being the sample size. Figures visualizing statistical results were generated in Mathematica (Wolfram Research, Urbana-Champaign, IL, United States); cortical maps of significant statistical findings were generated in MATLAB (Mathworks, Natick, MA, United States).
Tabulation and Reporting
To improve anatomo-functional localization, our FC analysis was implemented using the 17-network parcellation of Yeo et al. (2011) to define FC seeds, rather than the coarser seven-network (canonical) RSN parcellation. Because the 17-network parcellation is more granular, this allows one to localize age- and sex-specific effects on the cortex at relatively higher spatial resolution. Additionally, this reduces both (A) spatial filtering effects due to time series averaging and (B) cancellation effects whereby the anatomical patterns and distributions of correlation coefficients with opposite signs are lost (canceled out) when the coefficients are averaged over across relatively small cortical patches. Nevertheless, because the seven RSNs are more frequently studied, better understood, and easier to interpret, we choose to report, tabulate, and discuss results using the nomenclature of the seven-network parcellation scheme. For completeness, however, we also highlight notable anatomic and functional insights facilitated by our use of the 17-network RSN parcellation scheme. To summarize results obtained from our 17-RSN FC analysis, we leveraged the information in Figure 2, which maps the 17-RSN scheme onto the 7-RSN scheme, to map results obtained within the former onto the latter.
Results
In this section, age- and sex-related effects on FC degradation after mTBI are reported. The text makes frequent reference to Table 2, which lists significant correlations between cognitive measures and FC changes. Because this table reports test statistics and related p-values, these quantities are not reported in the text; instead, the row of the table where these values can be found are reported there.
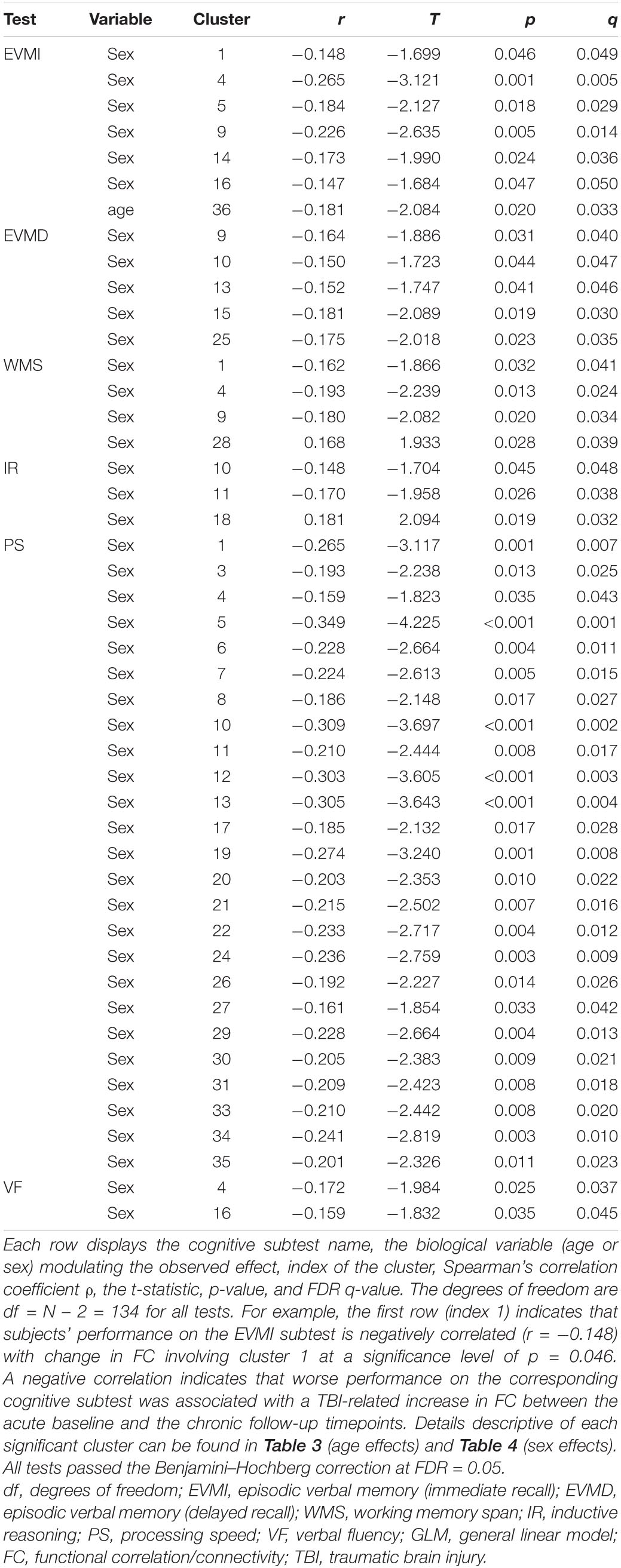
Table 2. Statistically significant correlations between FC changes involving significant cortical clusters identified through GLM and cognitive task performance.
Age Effects
The YAs and OAs differ significantly in how the SMN, VAN, and DMN are functionally coupled to various cortical clusters (Figure 3 and Table 3). Here and throughout, dY and dO stand for Cohen’s d (the effect sizes for YAs and OAs, respectively) multiplied by the sign of the change in FC associated with d. We find a significant age group difference in FC changes (dY = 0.05, dO = −0.19, ΔdA = −0.24, p = 0.0027) between the SMN and a cortical cluster spanning a patch of lateral temporal cortex overlapping with the LN and DMN (Figure 3A). This cluster’s FC changes are significantly and negatively associated with changes in EVMI (r = −0.18, t134 = −2.09, p = 0.02, q = 0.03, see cluster 36 in Table 2). Another significant age difference in FC change (dY = −0.04; dO = −0.21, ΔdA = −0.25, p = 0.0305) is found between the VAN and a cluster spanning lateral occipital and inferior parietal regions (Figure 3B). Thirdly, a significant difference in FC change (dY = 0.10, dO = −0.11, ΔdA = −0.21, p = 0.0078) is found between the DMN and a cluster spanning parts of the superior temporal, middle temporal, and anterior cingulate cortices (Figure 3C). Notably, OAs exhibit relatively larger FC decreases than YAs (Figure 4). In OAs, FC decreases across all three clusters whereas, in YAs, FC is relatively unchanged for the YAs, such that |dY| < |dO|.
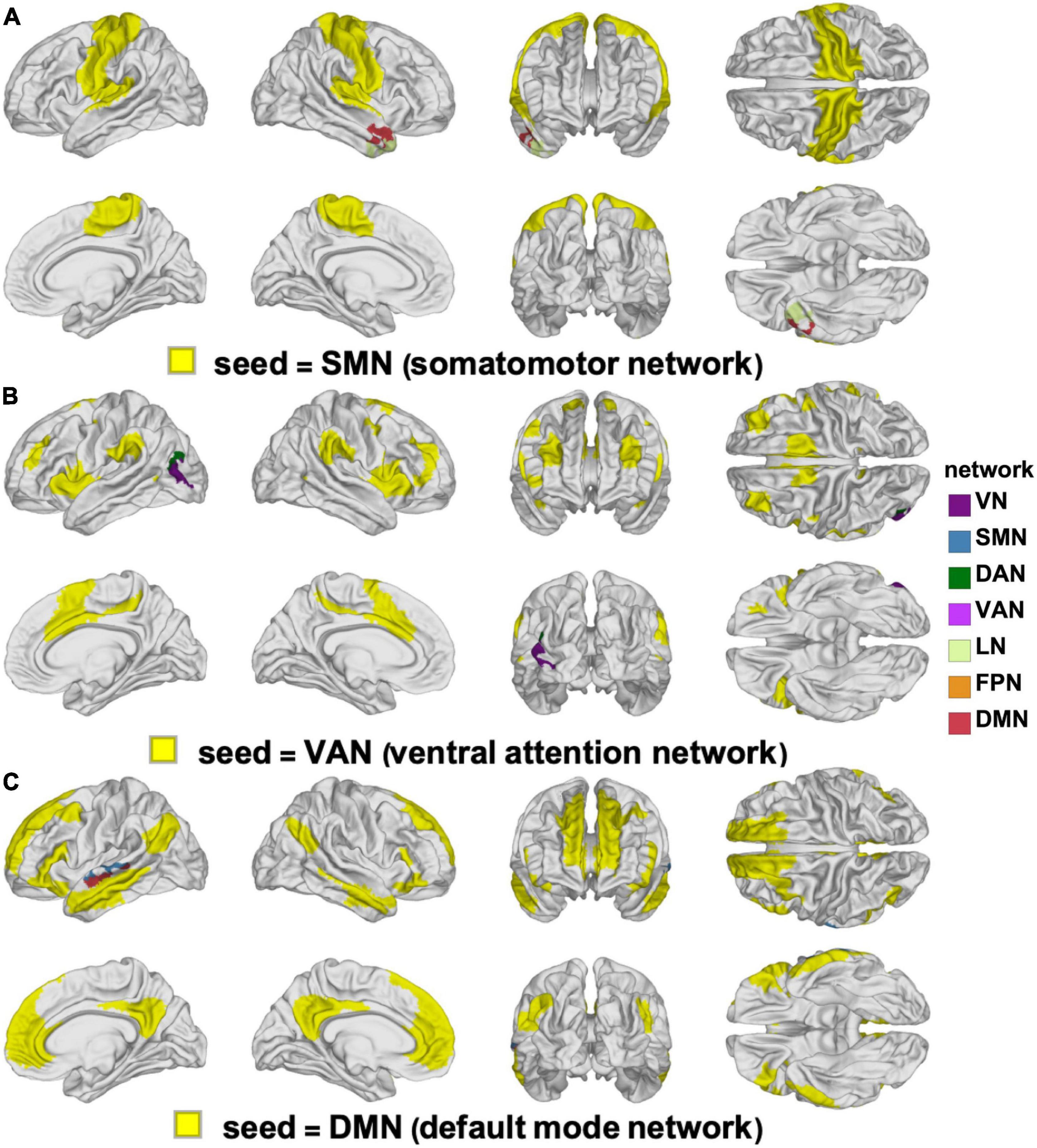
Figure 3. Clusters of statistically significant FC changes that differ significantly between YAs and OAs. In each panel, the seed RSN is painted in yellow and the significant clusters are painted using the color(s) of the RSN(s) with which they overlap, following the color scheme of Yeo et al. (2011), see also Figure 2. The seed networks are the SMN (A), VAN (B), and DMN (C). No significant clusters were found for the DAN, FPN, LN, or VN. DAN, dorsal attention network; DMN, default mode network; FC, functional correlation; FPN, frontoparietal network; LN, limbic network; OA, older adult; RSN, resting state network; SMN, somatomotor network; VAN, ventral attention network; VN, visual network; YA, young adult.
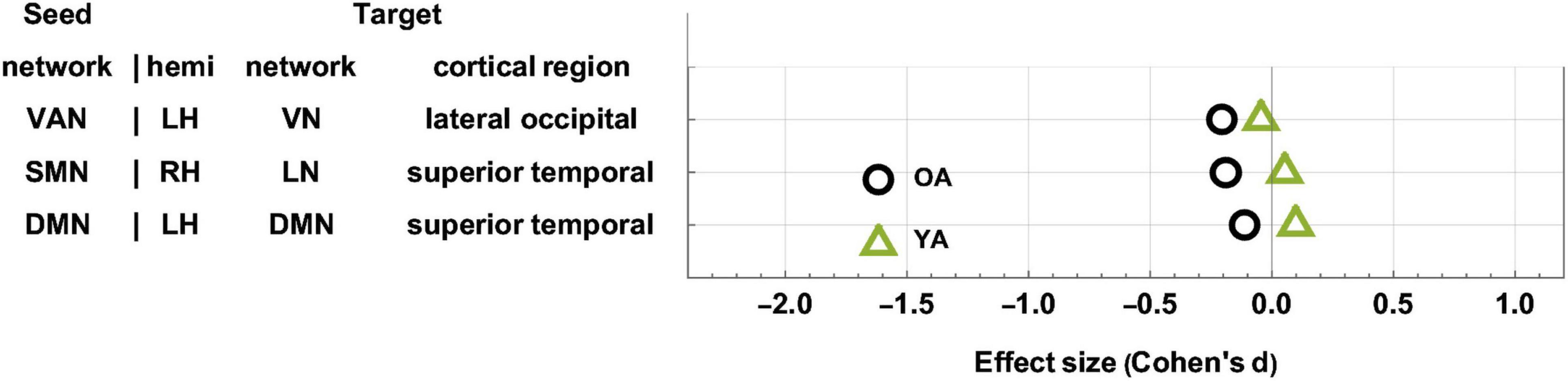
Figure 4. Age-related differences in FC change after TBI. OAs exhibit FC decreases across all statistically significant target clusters, whereas YAs exhibit relatively fewer significant changes across the ∼6-month follow-up period. The horizontal axis encodes effect size (Cohen’s d), and seed-target cluster pairs are listed in ascending order according to males’ effect size d. FC, functional correlation; OA, older adult; TBI, traumatic brain injury; YA, young adult.
Sex Effects
Table 4 lists (A) Cohen’s dM and dF, and (B) Cohen’s dS for differences in FC change between sexes (ΔρS). These data are tabulated for each seed-target pair; the seed is an RSN, and the target is a significant cortical cluster to which the RSN is coupled. Also listed is the overlap of each target cortical cluster with various RSNs. Here and throughout, dF and dM stand for Cohen’s d (the effect size for females and males, respectively) multiplied by the sign of the respective change in FC associated with d. RSN-cluster pairs are sorted in ascending order of males’ effect sizes, which range from dM = −2.14 (FC decrease) to dM = 0.77 (FC increase); females’ effect sizes range from dF = −1.89 to dF = 1.03. The clusters listed in Table 4 are also displayed on the cortical surface in Figure 5. The reason for which males—rather than females—were selected to determine the order of displays in Table 4 and Figure 5 is that males had been found to exhibit a wider range of FC changes. This made the comparison of their FCs relative to those of females easier because females’ relatively smaller average FC changes provided a pseudo-baseline, relative to which males’ FCs could be compared. To assist the reader in comparing females’ effect sizes relative to men’s, Supplementary Table 1 lists the data in Table 4 in ascending order of females’ effect sizes. A statistical comparison of the sexes across HCs identified no statistically significant results. Thus, across all seed networks, no significant difference in FC change across timepoints was found between sexes.
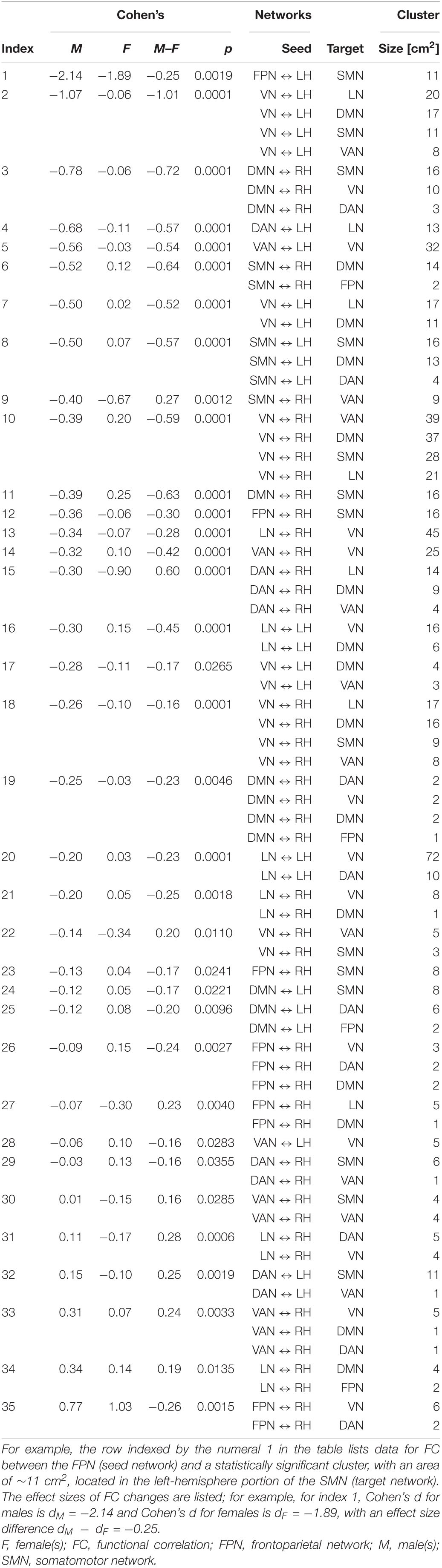
Table 4. Like Table 3, for FC changes that differ significantly across males (M) and females (F).
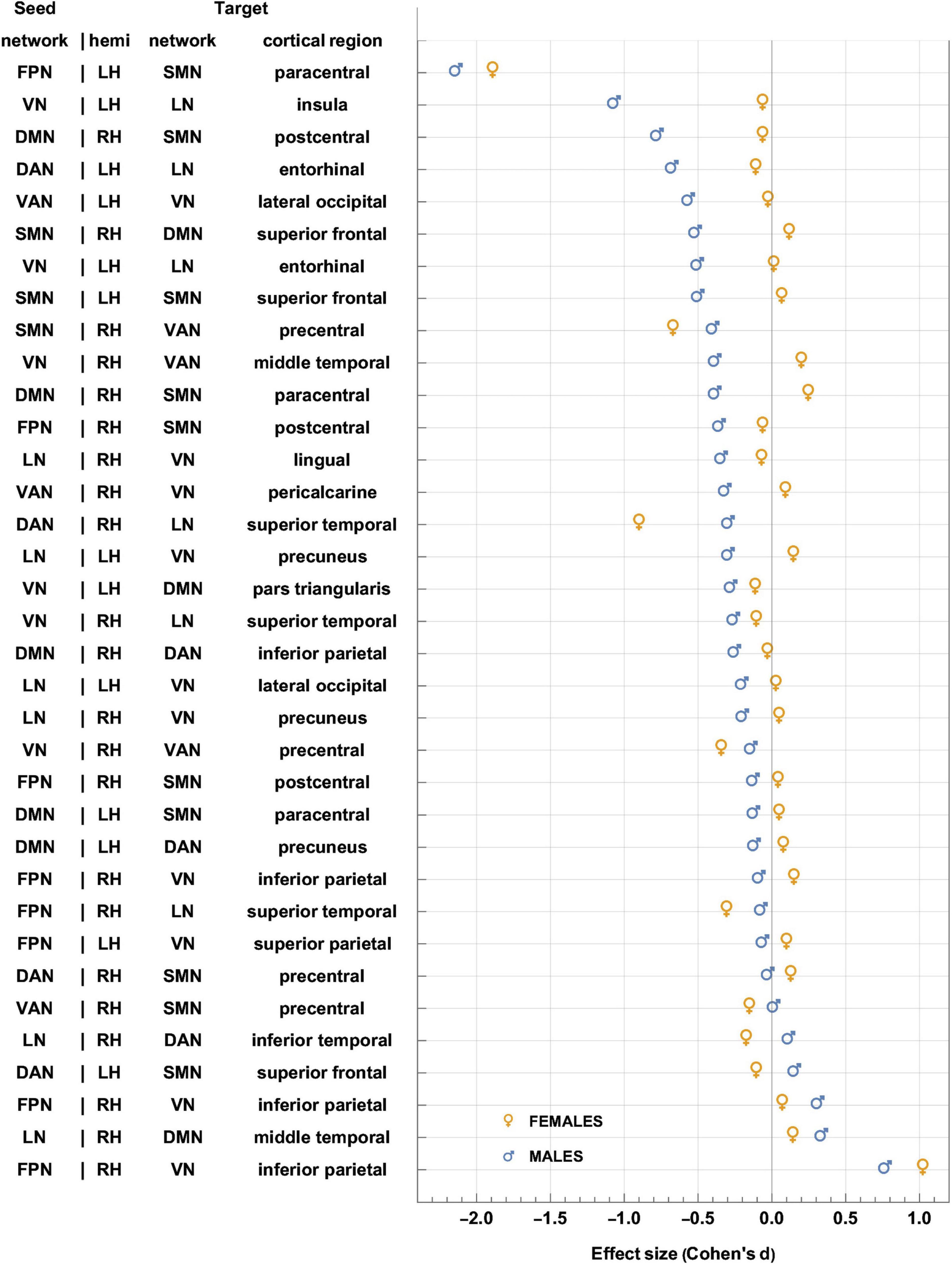
Figure 5. Like Figure 4, for sex-related effects. Clusters are sorted in ascending order of FC change in males. Females and males are indicated by gold ♀ and by blue ♂ symbols, respectively. Most significant findings are associated with greater FC decreases in males, whereas females’ typical significant FC changes are relatively smaller. FC, functional correlation.
Each inset of Figure 5 displays a single seed RSN and the cortical clusters whose FCs to that RSN change in a way that is predicated significantly upon sex. Thus, as already stated, Figure 5 provides cortical displays of the results in Table 4. As Figure 5 and Table 4 indicate, males exhibit greater variability, across both source RSNs and target clusters, in the magnitudes of their FC changes. All seven RSNs exhibit sex-related differences in how their RS FCs to other areas change after injury (Figure 5 and Table 4). The magnitudes of most such changes are relatively smaller in females; for example, the effect size of the change in FC between the VN and the portion of the LN overlapping the insula is dF = −0.06. By contrast, males’ FC changes are relatively larger in magnitude than females’ and most are negative (i.e., they involve FC decreases). Thus, FC between the VN and portion of the LN overlapping the insula changes significantly post-injury (dM = −0.78, p < 0.0001). Males’ largest FC increases are between (A) the LN and the middle temporal portion of the DMN (dM = 0.34, p < 0.0001), and (B) the FPN and the inferior parietal portion of the VN (dM = 0.77, p < 0.0001, Figure 5).
Let ΔdS=dF−dM, where dF and dM are the effect sizes of females and males, respectively. Thus, Δds is the difference in effect sizes between sexes. Because |dF| < |dM| in most cases, most sex differences in FC change occur in males. Thus, Figure 5 indicates that the largest sex differences observed pertain to FC changes between portions of the VN and LN that overlap with the insula, entorhinal cortex, and other temporal regions. For example, in the insula and entorhinal cortex, males’ FCs decrease more than in females by |Δds| = 1.01 and by |Δds| = 0.52, respectively (p < 0.0001). The second-largest, sex-related difference pertains to changes in FC between the DMN and SMN, for which |Δds| = 0.72 (DMN to the SMN portion in the postcentral gyrus) and |Δds| = 0.64 (SMN to the DMN portion in the SFG), respectively. Larger effects are found in males (p < 0.0001 for both clusters, Figure 5 and Table 4).
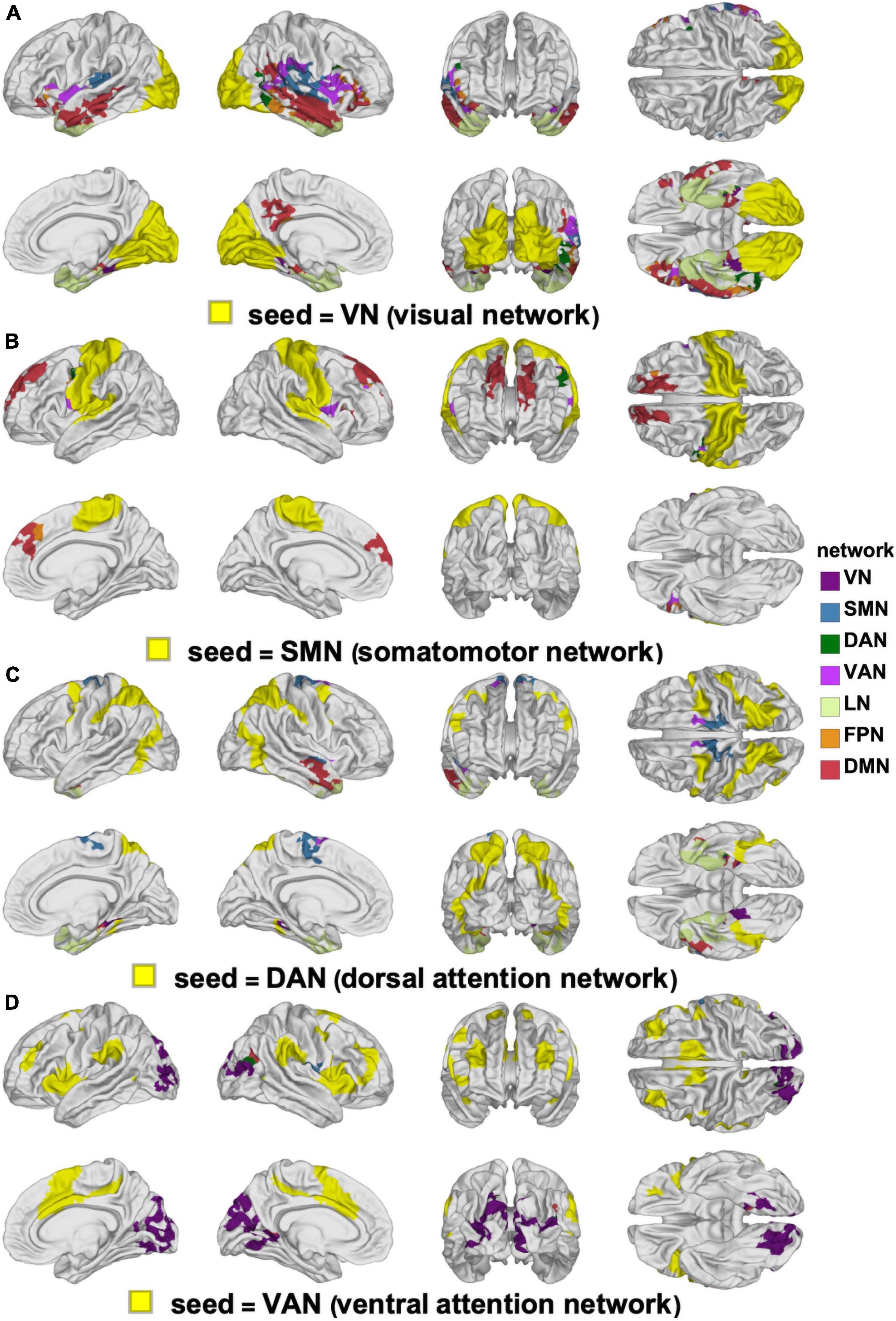
Figure 6. Canonical RSNs (seeds, yellow) and corresponding cortical clusters whose FC with the corresponding seed network changes significantly according to sex. The seed RSNs are the VN (A), SMN (B), DAN (C), and VAN (D). DAN, dorsal attention network; FC, functional correlation; RSN, resting state network; SMN, somatomotor network; VAN, ventral attention network; VN, visual network.
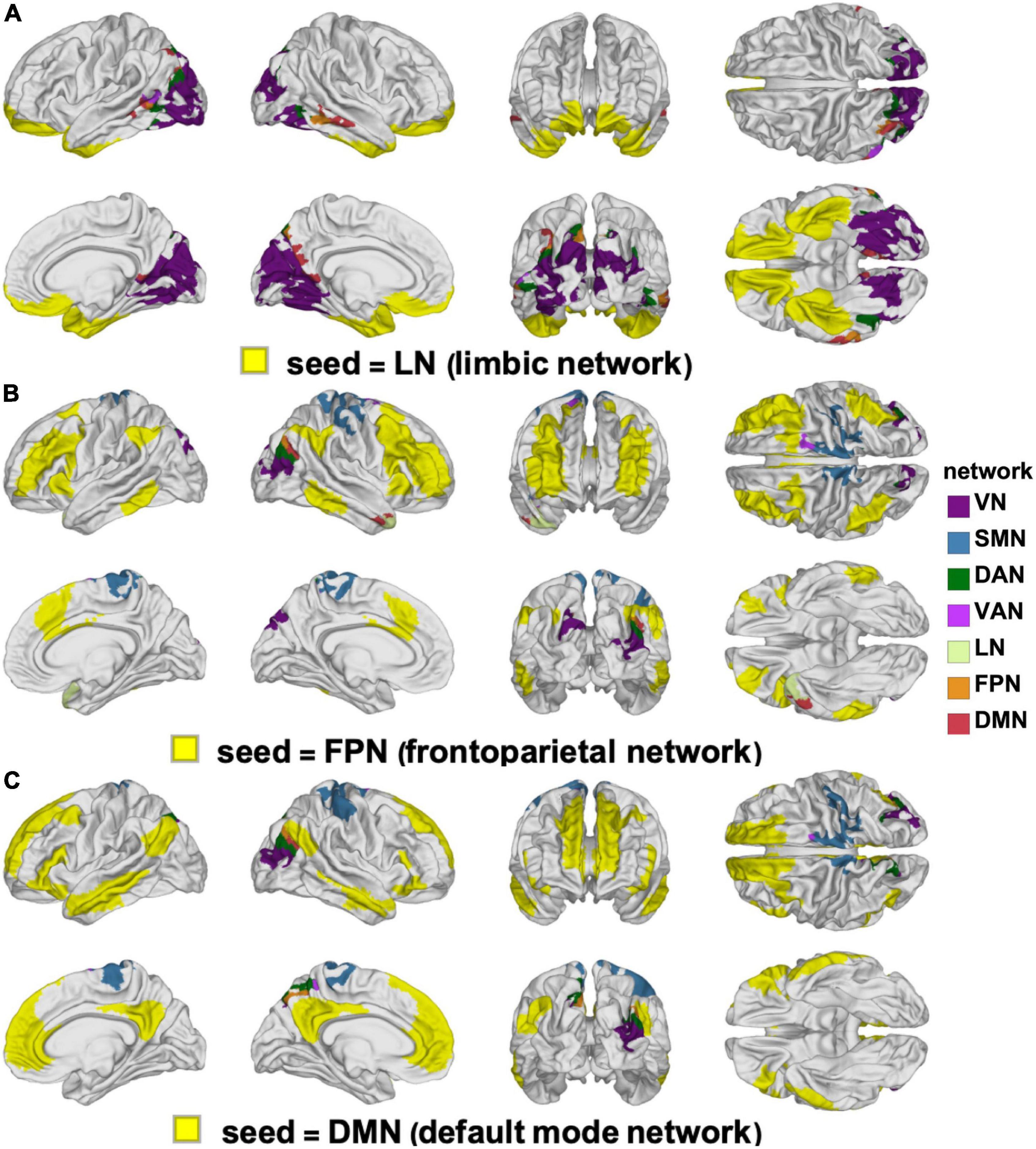
Figure 7. Like Figure 6, for the LN (A), FPN (B), and DMN (C). DMN, default mode network; FPN, frontoparietal network; LN, limbic network.
Only a handful of seed-target pairs exhibit FC changes that are smaller in females (i.e., where females’ FC decreases more than males’ after mTBI), and most of these involve FCs between the DAN and other cortical regions, and between the VAN and other areas (Figures 5, 6C,D and Table 4). Examples include sex differences in FC between (A) the DAN and the superior frontal portion of the SMN (|Δds| = 0.25, p = 0.0019), (B) the DAN and the superior temporal portion of the LN (|Δds| = 0.60, p < 0.0001), and (C) the LN and the inferior temporal lobe portion of the DAN (|Δds| = 0.28, p = 0.0006). Other RSN-cluster pairs whose FC decreases are significantly larger in males include (A) the DAN and the entorhinal portion of the LN (|Δds| = 0.57, p < 0.0001), and (B) the VAN and the lateral occipital portion of the VN (|Δds| = 0.54, p < 0.0001) as shown in Figures 5, 6C,D and Table 4.
The clusters whose FCs to various RSNs change in ways that differ significantly by sex are mapped on the cortical surface in Figure 6 (for the VN, SMN, DAN, and VAN) and in Figure 7 (for the LN, FPN, and DMN). For each of the RSNs, there is at least one cluster whose FC to the seed RSN changes significantly in a sex-dependent manner. The pairs of regions exhibiting sex-dependent FC changes include:
(A) The VN (N2) and the MTG portion (N16) of the DMN (|Δds| = 0.59, p < 0.0001).
(B) The DMN (N16) and the paracentral lobule portion (N3) of the SMN (|Δds| = 0.63, p < 0.0001).
(C) The SMN (N4) and the PCG portion (N7) of the VAN (|Δds| = 0.27, p < 0.0012).
(D) The DAN (N6) and the PCG portion (N3) of the SMN (|Δds| = 0.16, p < 0.0355).
(E) The FPN (N13) and the superior temporal gyrus portion (N9) of the LN (|Δds| = 0.23, p < 0.0040).
Most clusters occur bilaterally, but do not typically overlap with a single RSN (Figures 6, 7).
Interaction of Age and Sex
The interaction between our main variables of sex and age were limited to small cortical clusters where significant differences were found in how FC changes depended on the sex × age interaction (Figure 8). The largest and most significant cluster is in the left supramarginal gyrus (p < 0.0010) and involves FC with the VN. The next most prominent clusters of interaction involve the VN seed and overlap with left rostral anterior cingulate cortex (p < 0.0010) and with the IPL (p < 0.0010).
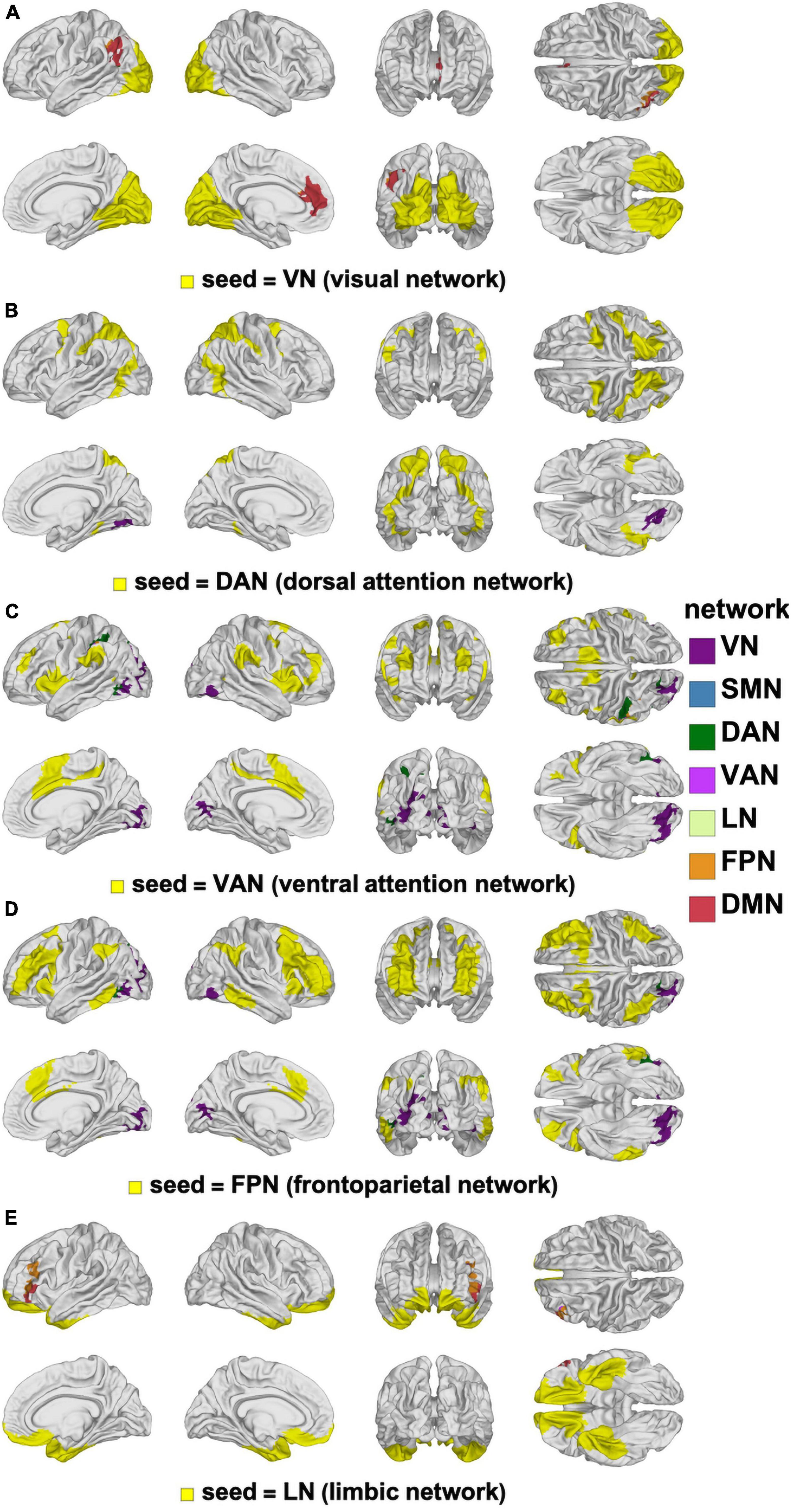
Figure 8. Canonical RSNs (seeds, yellow) and corresponding cortical clusters whose FC with the corresponding seed network changes significantly according to the age-by-sex interaction. The seed RSNs are the VN (A), DAN (B), VAN (C), FPN (D), and LN (E). DAN, dorsal attention network; FPN, frontal parietal network; FC, functional correlation; LN, limbic network; RSN, resting state network; VAN, ventral attention network; VN, visual network.
Discussion
In what follows, we interpret and discuss our findings by RSN, both for sex- and age-related effects. Where cognitive measures were significantly correlated with an FC change, plausible explanations, hypotheses, and postulations are provided for the change in question, along with references to similar findings in previous studies. It is important to note that these do not imply causation, given that analyses were restricted to correlations. Instead, our reasoned explanations merely convey the potential neuropsychological basis for sex- or age-related changes in FC following mTBI.
Age Effects
Three FC clusters were found to be significantly different between OAs and YAs with mTBI (one in the left lateral part of the occipital lobe, and one in each hemisphere’s superior temporal lobe, Figures 3, 4). These clusters feature FC decreases that are significantly greater in OAs (Table 3), potentially reflecting their poorer outcomes, whether neurological, cognitive, or affective (Mosenthal et al., 2002; Mushkudiani et al., 2007; Senathi-Raja et al., 2010). In particular, the lateral occipital gyrus is recruited by visual memory, a cognitive process that is more severely affected in OAs than in YAs (Larsson and Heeger, 2006; Senathi-Raja et al., 2010; Nagy et al., 2012; Byom et al., 2019). The lateral occipital gyrus also exhibits reduced FC to the VAN after mTBI, a phenomenon we found to be associated with poorer immediate recall (EVMI) in OAs (see Figures 3, 4, and row 1 in Table 3). This abnormal connectivity between the lateral occipital gyrus and the VAN may underlie impairments of visual memory, since visual mnemonic aids supported by the lateral occipital gyrus can be employed as encoding strategies on verbal memory tasks to improve recall (Unsworth et al., 2019). Two other clusters were identified here whose FC changes differ significantly by age group, and both overlap substantially with the superior temporal gyri. These bilateral clusters exhibit FCs to the SMN and DMN, respectively, whose post-traumatic FC decreases are significantly larger in older adults (Table 3). The superior temporal lobe is involved in language processing and in emotion perception, both of which frequently degrade after TBI (Green et al., 2004; Bornhofen and McDonald, 2008a,c). Thus, our findings may provide insight on how age modulates the extent of cognitive impairments after mTBI.
Sex Effects
The sex effects identified here involve all seven canonical RSNs. Typically, males demonstrated FC decreases compared to females, who either showed smaller decreases, no difference, or increases (Figures 5, 9, and Table 4). We also observed that the typical magnitude of FC changes in males is larger than in females (Figure 9 and Supplementary Figure 1). For these reasons, we highlight male sex as an independent risk factor for functional network degradation after mTBI, in agreement with previous work (Gupte et al., 2019; Robles et al., 2021). Tables 5–11 provide systematic interpretations for each result, cluster by cluster. Typically, across all seed networks, we found evidence for greater FC decreases in males; in many cases, decreases were correlated with poorer cognitive performance.
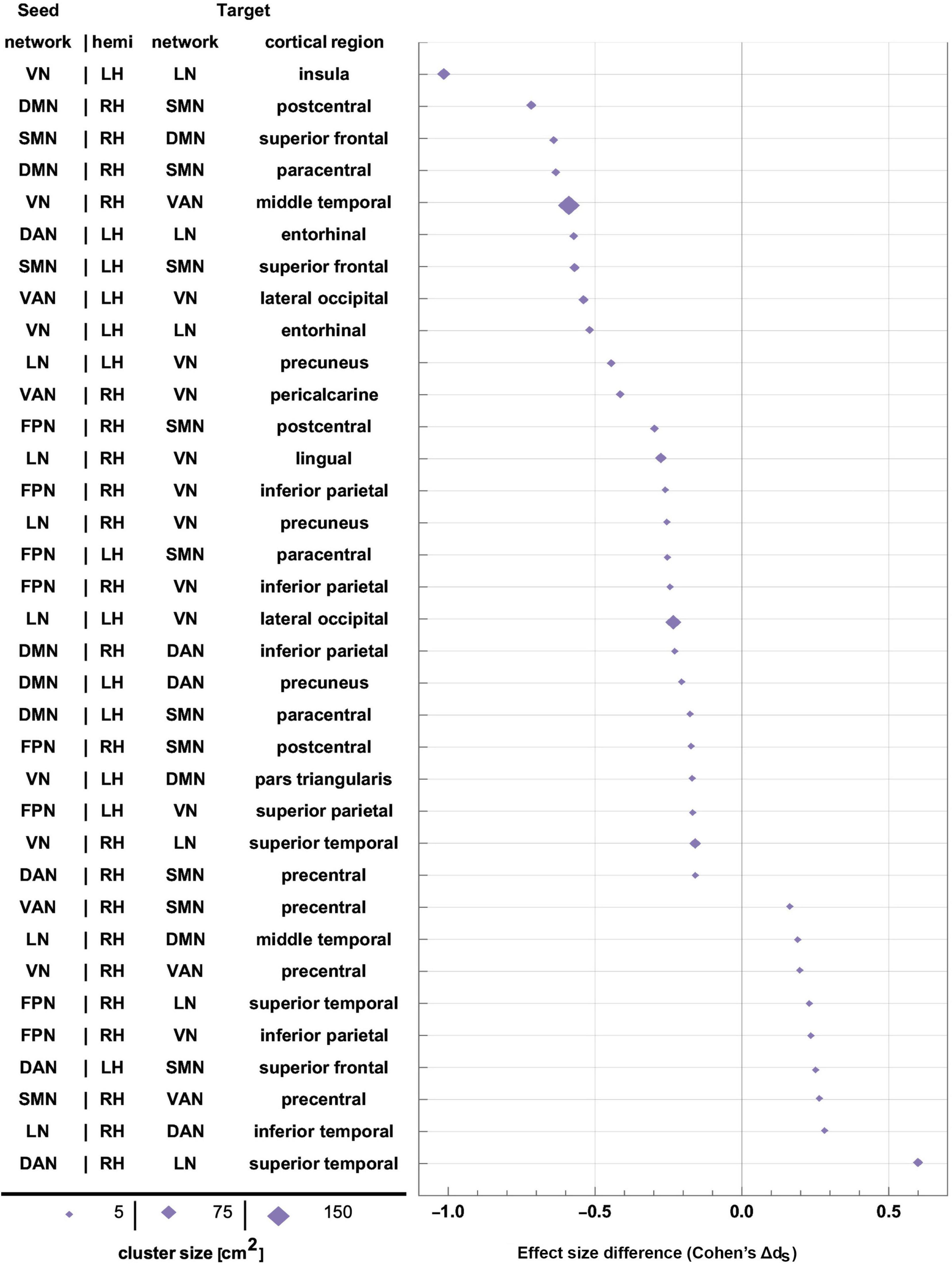
Figure 9. Effect sizes of FC changes predicated significantly upon sex. Pairs of RSNs (seeds) and corresponding statistically significant clusters (targets) are listed according to sex differences in effect size (Cohen’s dS=dM-dF, where d_M and d_F are Cohen’s d values for males and females, respectively). Values of d_S are plotted on the horizontal axis, sorted in ascending order. Marker size is proportional to cortical cluster size. FC, functional correlation; RSN, resting state network.
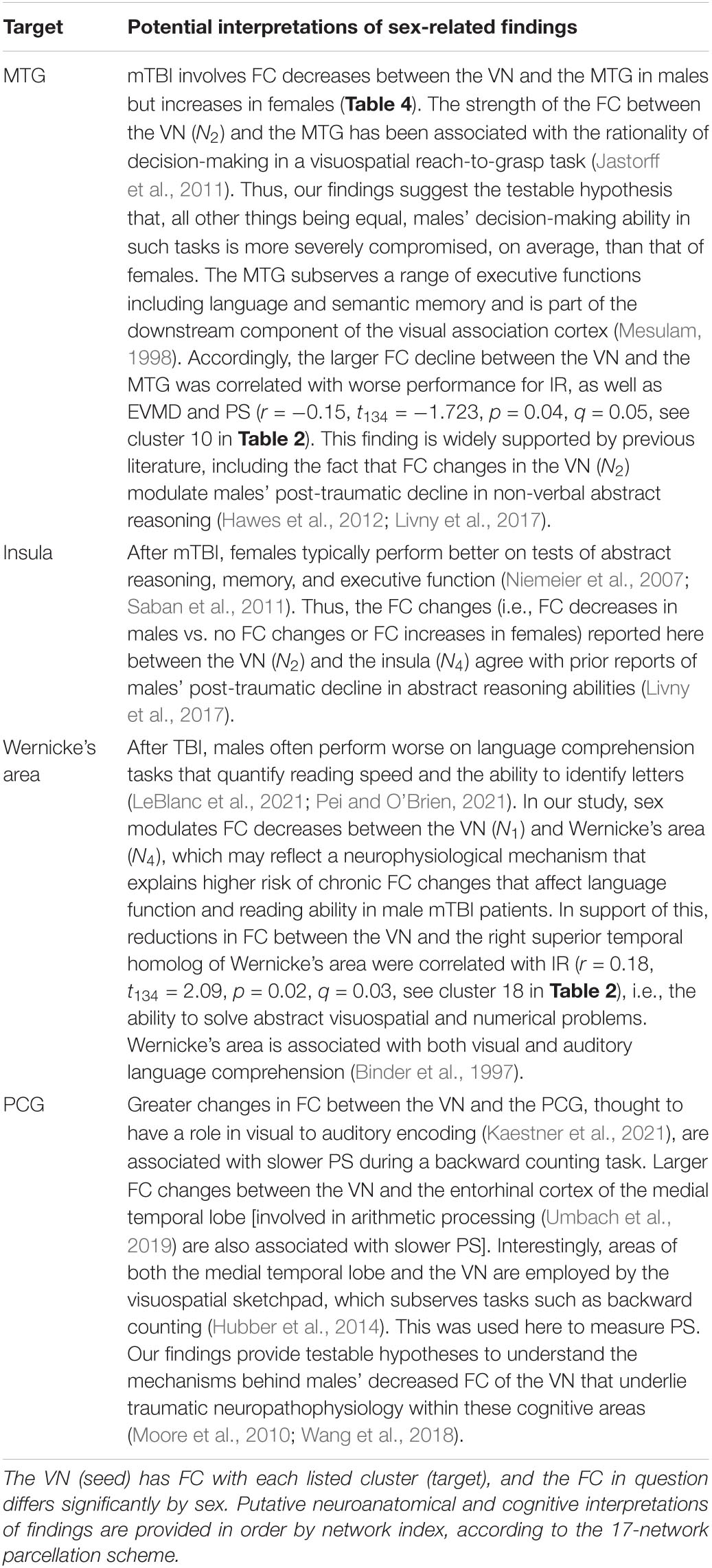
Table 5. Functional neuroanatomy localizations and putative interpretation of sex-related findings pertaining to the visual network (VN).
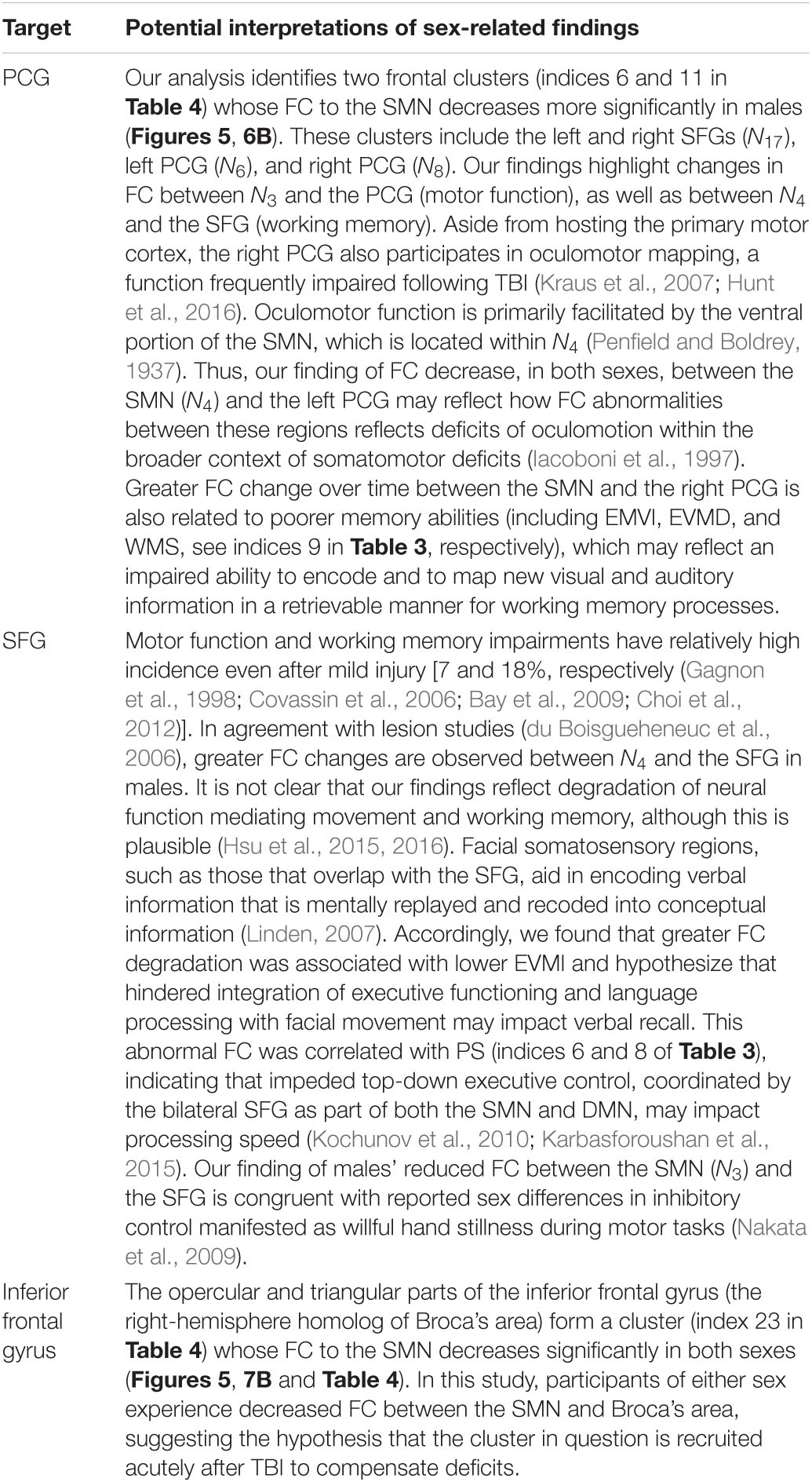
Table 6. Like Table 5, for the SMN.
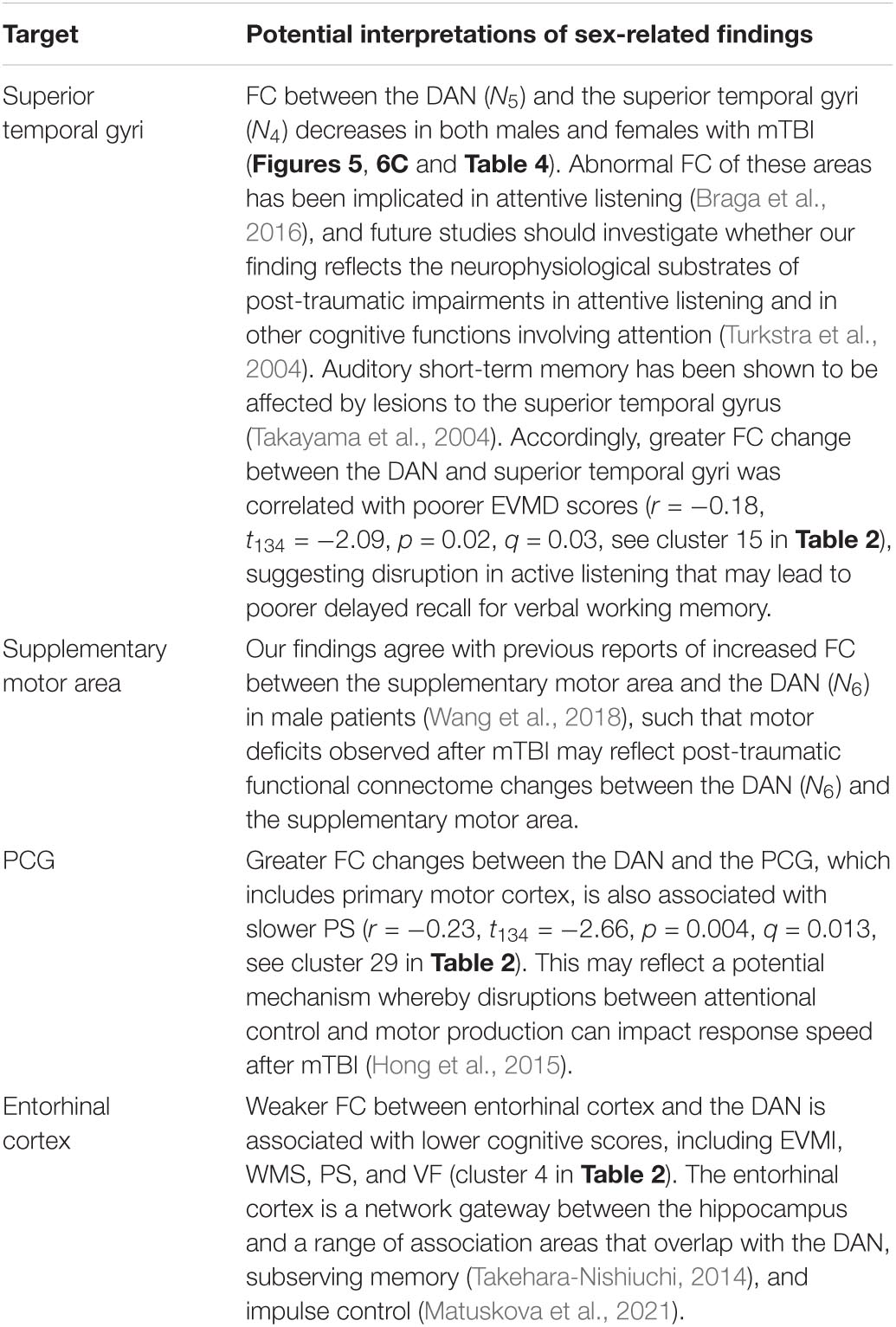
Table 7. Like Table 5, for the DAN.
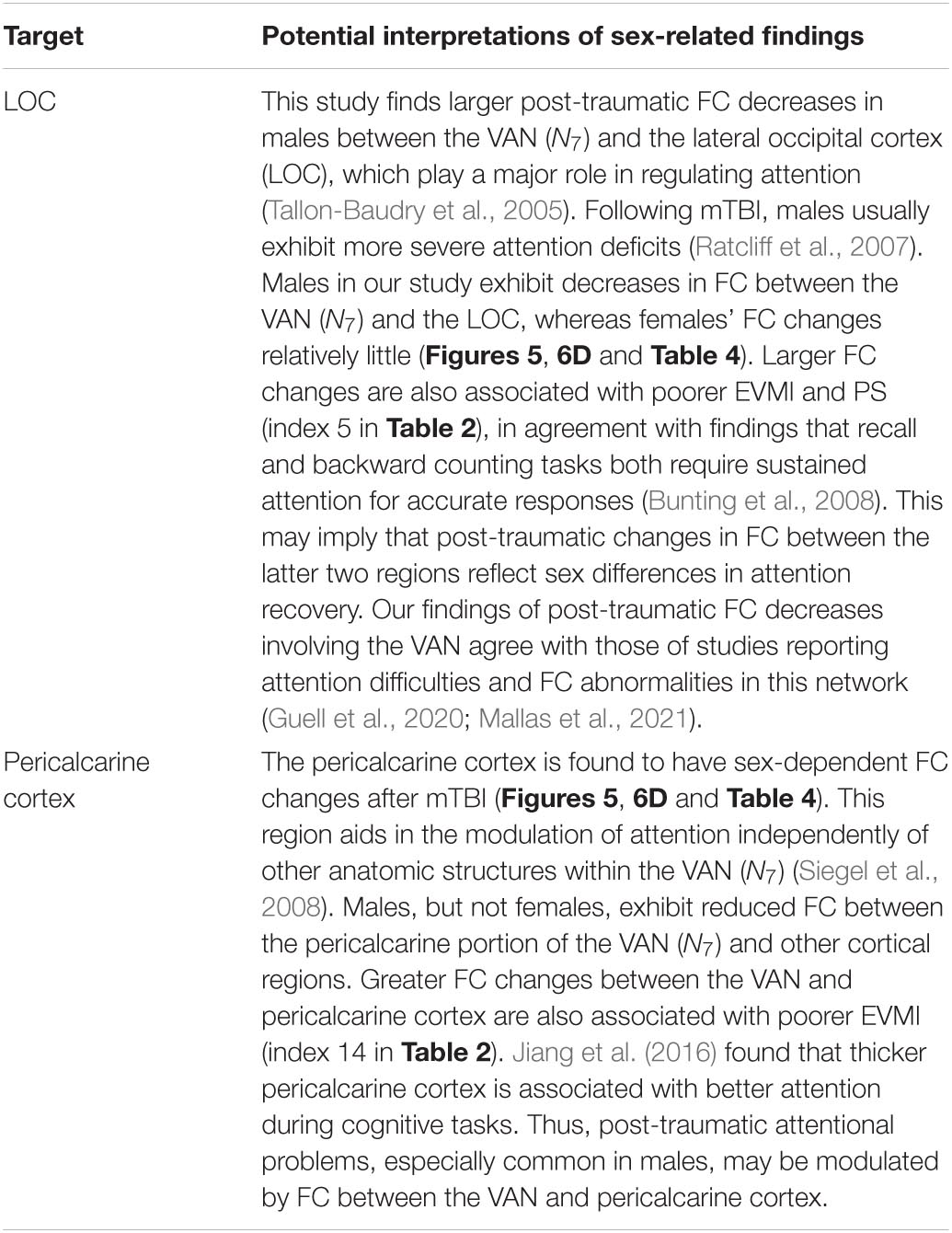
Table 8. Like Table 5, for the VAN.
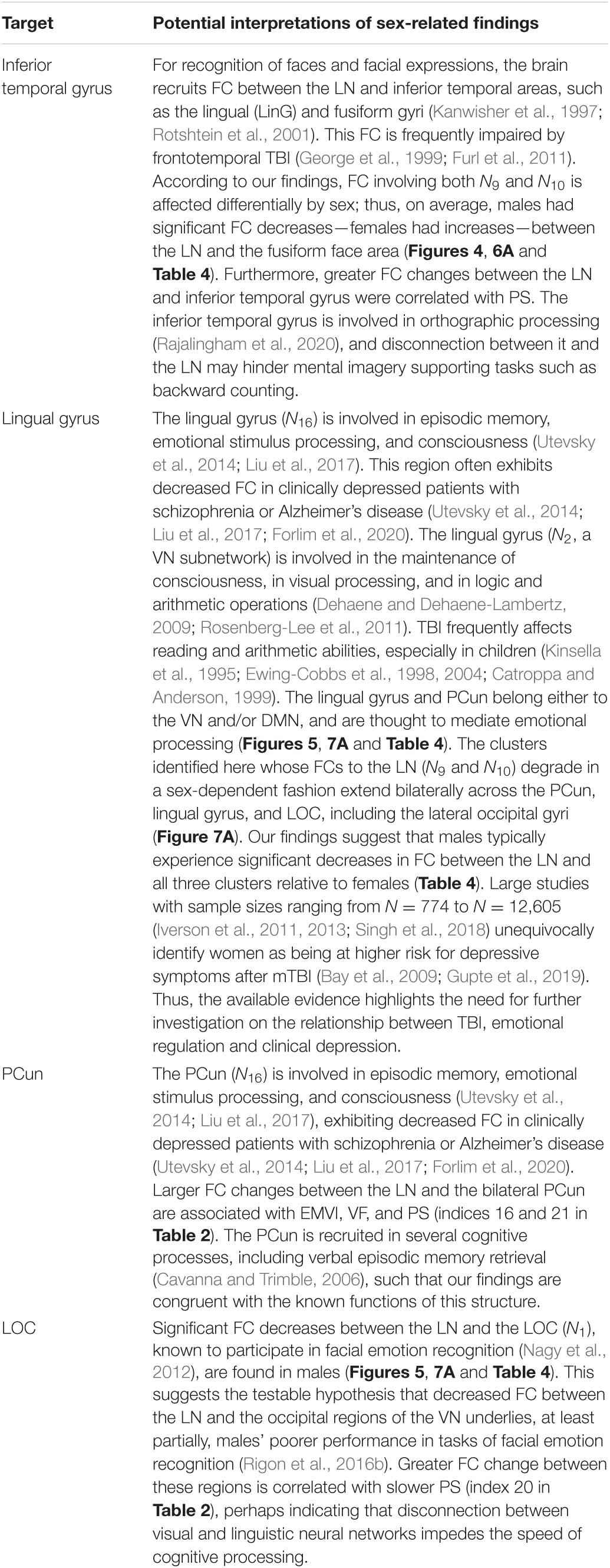
Table 9. Like Table 5, for the LN.
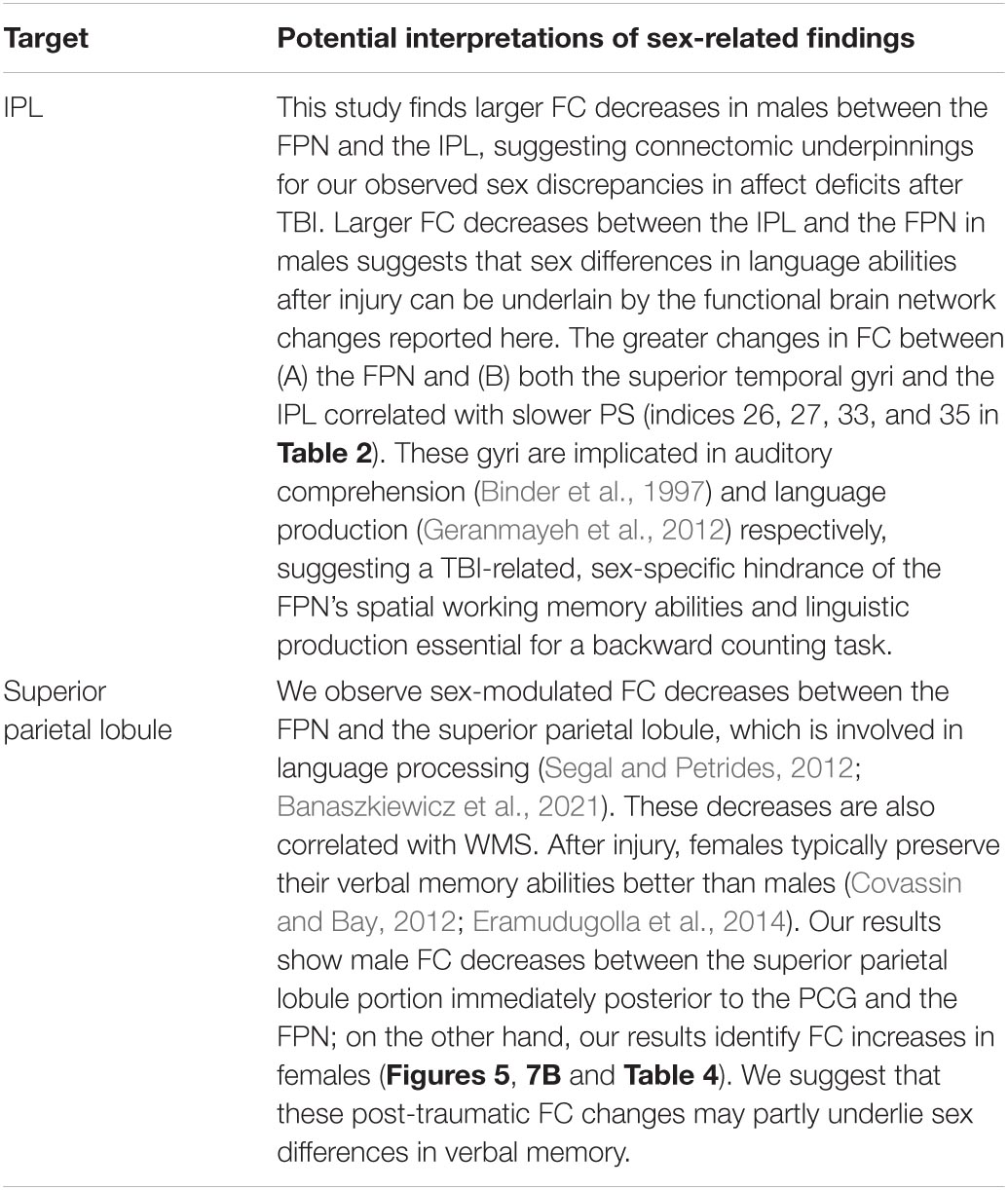
Table 10. Like Table 5, for the FPN.
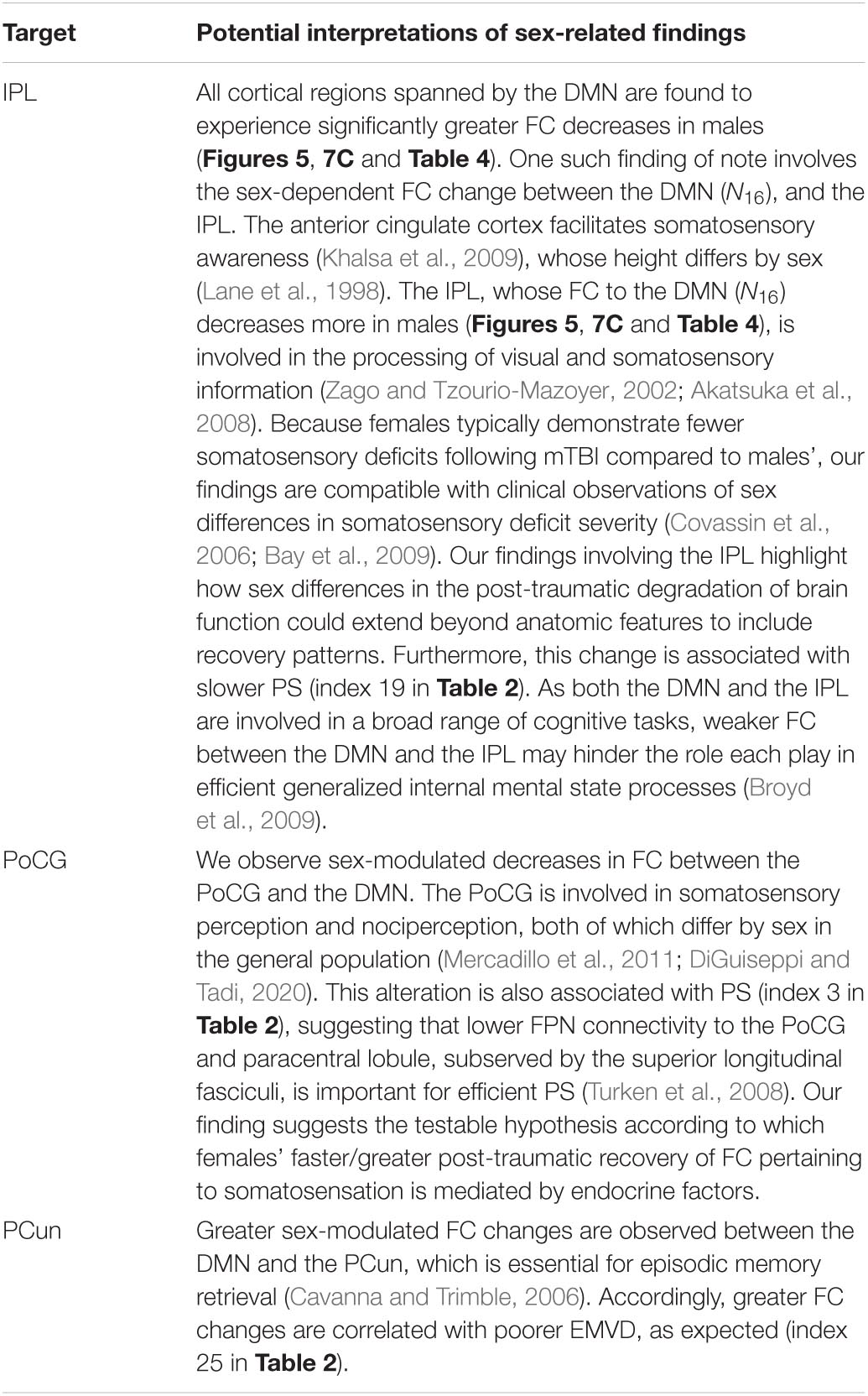
Table 11. Like Table 5, for the DMN.
The largest sex differences observed pertain to FC changes between portions of the VN and LN that overlap with the insula, entorhinal cortex, and with other temporal regions. Interestingly, these regions have been highlighted extensively by FC studies of AD and are associated with memory deficits (e.g., Killiany et al., 2000; Jack et al., 2002; Chen et al., 2016). TBI patients are at higher risk of AD, especially after repeated brain injuries (Faden and Loane, 2015). Irimia et al. (2020) found overlap in the DMNs of TBI and AD patients that include medial and lateral areas of the temporal lobe. These authors found extensive commonalities in DMN FC between AD and TBI patients, and between both patient groups and HCs. The extent of AD-like FC degradation within the DMNs of geriatric TBI patients were predicted based on their acute cognitive assessment scores, with specificity and sensitivity comparable to AD blood and imaging biomarkers. These findings may be related to the fact that the thickness of entorhinal cortex can predict cognitive decline with age and cognitive impairment severity in AD (Kordower et al., 2001; Velayudhan et al., 2013; Zhou et al., 2016). This is only one example of the complex relationship between mTBI-related changes in structural and functional connectomics, whose exploration is outside the scope of this study. Our results do support previous research that highlights similarities between mTBI and AD in terms of their shared FC degradation trajectories, and the reader is referred to our previous study on this topic for further details (Irimia et al., 2020).
In HCs, we found no significant sex differences in FC changes between baseline and follow-up. Prior HC studies found relatively small changes in FC—and even no significant changes—across follow-up periods of 2–3 years (Odish et al., 2015; Ousdal et al., 2020). The absence of significant findings in our analysis is consistent with these prior studies and suggests strongly that our findings are related to mTBI effects rather than to typical aging across the timespan between imaging sessions. Presumably, any changes in HCs across 6 months, if any, are likely to be very small relative to those identified here in the mTBI group.
Interaction of Age and Sex
Although present, the interactions between the main effects of age and sex were limited to small clusters located primarily in the left hemisphere (IPL, supramarginal gyrus, and anterior cingulate cortex). Interpretation of the age × sex interaction within these regions is not straightforward due to their small spatial extent and effect size. Furthermore, the available functional neuroanatomy literature does not provide the spatial specificity required to formulate cautious interpretations of our findings at a level of spatial localization similar to that of the interaction clusters identified here.
Limitations
In this study, FC analysis results were mapped from the 17 RSN-scheme onto the seven canonical RSN-scheme. The advantages of this technique included (A) improved anatomo-functional localization, and (B) greater ability to summarize results across RSNs, since the 7-RSN scheme has fewer networks. Nevertheless, we did not use the same scheme for both the analysis and the summary, which may have caused relatively small inaccuracies when mapping results from one scheme onto the other. One additional limitation of this study is that, for logistical and methodological reasons, we did not study changes in FC between cortical regions and the subcortex. Future studies, however, should investigate these changes. Because fMRI can only resolve low-frequency BOLD signals, FCs involving high-frequency brain activity cannot be captured well using this technique. Alternative approaches like electro- and magnetoencephalography should be used to confirm and extend our studies further (Irimia et al., 2013a,b).
To find age-related effects, we split our cohort into two groups, each consisting of adults older or younger than 40, respectively. This threshold was convenient partly because it split our sample into approximately equal groups, thus resulting in a relatively balanced statistical design. However, because age is a continuous (rather than discrete) variable, the threshold in question is relatively arbitrary and other cutoffs may lead to different results (Irimia et al., 2015). One alternative to our approach could involve studying FC changes as a function of age at injury; nevertheless, due to the complexity of our findings, we deemed our current strategy to be preferable. Furthermore, although we did not interpret the statistical interaction between age and sex, this interaction was included in the GLM and its effect was regressed from our analysis before calculating effect sizes and FCs. Future studies should explore both the age-by-sex interaction and how FCs change as a function of age at injury.
Our sample focused on mTBI, which may limit the generalizability of our findings to moderate or severe TBI patients. Whereas explicit analysis of these severities is required, we speculate that the effects of older age and male sex on FC changes and cognitive outcomes would be exacerbated in a moderate-to-severe TBI group (Konstantinou et al., 2018). Bonnelle et al. (2011) found increased FC of the DMN in moderate-to-severe TBI patients, which correlated with deficits in sustained attention, indicating that higher TBI severity may lead to greater FC changes and poorer cognitive outcome. Finally, our study is observational rather than interventional; development and testing of interventions for mTBI-related impairments is beyond the scope of our work.
Conclusion
This study is the first to systematically investigate of FC changes in mTBI, with a focus on how RSN connectivity is modulated by age and sex. Using GLMs, we identified FCs between canonical RSNs that undergo significant changes between the acute and chronic stages of mTBI. Group comparisons reveal that older age at injury and male sex are risk factors for post-injury functional brain network degradation. In comparison to HCs, this study involves a longitudinal design that explores, in a systematic way, FC changes across all seven canonical RSNs of the human brain. We also detail potential neurophysiological substrates—as conceptualized in terms of RSN connectivity changes—that may underlie the cognitive deficits in memory, processing speed, verbal fluency, and abstract reasoning typically observed post-mTBI, especially in males. Importantly, FC changes involving the VAN and DAN may explain post-injury impairments of visual memory and attention, respectively. FPN changes may relate to the underpinnings of language processing deficits commonly observed in mTBI patients, and post-traumatic FC changes involving the DMN may be responsible for degradations in processing speed and memory. FC changes pertaining to the SMN can help to explain the functional connectomic bases of mTBI patients’ abnormal PS and the VN- and LN-related FC alterations aid in explaining the deficits of abstract reasoning and language processing abilities frequently documented after mTBI. These results constitute substantial progress in scientific understanding of the relationships between TBI, age, sex, and cognitive outcome as reflected by FC and its changes post-injury. Future research that examines the hypotheses proposed here will provide additional insight into the pathophysiological mechanisms underlying functional connectome changes after mTBI.
Alzheimer’s Disease Neuroimaging Initiative
Data collection and sharing for this project was funded by the Alzheimer’s Disease Neuroimaging Initiative (ADNI) (National Institutes of Health Grant U01 AG024904) and DOD ADNI (Department of Defense award number W81XWH-12-2-0012). ADNI was funded by the National Institute on Aging, the National Institute of Biomedical Imaging and Bioengineering, and through generous contributions from the following: AbbVie, Alzheimer’s Association; Alzheimer’s Drug Discovery Foundation; Araclon Biotech; BioClinica, Inc.; Biogen; Bristol-Myers Squibb Company; CereSpir, Inc.; Cogstate; Eisai Inc.; Elan Pharmaceuticals, Inc.; Eli Lilly and Company; EuroImmun; F. Hoffmann-La Roche Ltd and its affiliated company Genentech, Inc.; Fujirebio; GE Healthcare; IXICO Ltd.; Janssen Alzheimer Immunotherapy Research & Development, LLC.; Johnson & Johnson Pharmaceutical Research & Development LLC.; Lumosity; Lundbeck; Merck & Co., Inc.; Meso Scale Diagnostics, LLC.; NeuroRx Research; Neurotrack Technologies; Novartis Pharmaceuticals Corporation; Pfizer Inc.; Piramal Imaging; Servier; Takeda Pharmaceutical Company; and Transition Therapeutics. The Canadian Institutes of Health Research is providing funds to support ADNI clinical sites in Canada. Private sector contributions are facilitated by the Foundation for the National Institutes of Health (www.fnih.org). The grantee organization is the Northern California Institute for Research and Education, and the study is coordinated by the Alzheimer’s Therapeutic Research Institute at the University of Southern California. ADNI data are disseminated by the Laboratory for Neuro Imaging at the University of Southern California.
Data Availability Statement
MRI data acquired from HC and AD participants are publicly available from the ADNI database (http://adni.loni.usc.edu). For TBI participants, primary data generated during and/or analyzed during the current study are available subject to a data transfer agreement. At the request of some participants, their written permission is additionally required in some cases.
Ethics Statement
The studies involving human participants were reviewed and approved by Institutional Review Board at the University of Southern California. The patients/participants provided their written informed consent to participate in this study.
Author Contributions
AA: methodology, software, formal analysis, investigation, writing – original draft, writing – review and editing, and visualization. AM: methodology, software, formal analysis, investigation, data curation, writing – original draft, and writing – review and editing. PI: investigation, writing – original draft, and review and editing. MH: investigation and writing – review and editing. TF: investigation. AI: conceptualization, funding acquisition, project administration, supervision, writing – review and editing, and resources. All authors approved the final version of the manuscript for publication.
Funding
This work was supported by NIH grant R01 NS 100973 to AI, by DoD award W81-XWH-1810413 to AI, by a grant from The James J. and Sue Femino Foundation to AI, by a Hanson-Thorell Research Scholarship to AI, and by funding from the Undergraduate Research Associate Program (URAP) and the Center for Undergraduate Research in Viterbi Engineering (CURVE) at the University of Southern California, to AI.
Conflict of Interest
The authors declare that the research was conducted in the absence of any commercial or financial relationships that could be construed as a potential conflict of interest.
Publisher’s Note
All claims expressed in this article are solely those of the authors and do not necessarily represent those of their affiliated organizations, or those of the publisher, the editors and the reviewers. Any product that may be evaluated in this article, or claim that may be made by its manufacturer, is not guaranteed or endorsed by the publisher.
Acknowledgments
The authors are grateful to Nikhil N. Chaudhari for assistance with data archiving and retrieval, to Nahian F. Chowdhury for assistance with the visualization of results, as well as to Shania Wang, Judy Wang, and Jun Kim for assistance with data analysis.
Supplementary Material
The Supplementary Material for this article can be found online at: https://www.frontiersin.org/articles/10.3389/fnagi.2022.852990/full#supplementary-material
Abbreviations
ADNI, Alzheimer’s disease neuroimaging initiative; BOLD, blood oxygenation level-dependent; BTACT, brief test of adult cognition by telephone; DAN, dorsal attention network; DMN, default mode network; EVMI, episodic verbal memory (immediate recall); EVMD, episodic verbal memory (delayed recall); FC, functional connectivity/correlation; fMRI, functional magnetic resonance imaging; FPN, frontoparietal network; FS-FAST, FreeSurfer Functional Analysis Stream; GLM, general linear model; HC, healthy control; IPL, inferior parietal lobule; IR, inductive reasoning; LN, limbic network; LOC, lateral occipital cortex; MFG, middle frontal gyrus; mTBI, mild traumatic brain injury; MTG, middle temporal gyrus; OA, older adult; PCG, precentral gyrus; PCun, precuneus; PoCG, postcentral gyrus; PS, processing speed; RS, resting state; RSN, resting state network; SFG, superior frontal gyrus; SMN, somatomotor network; TBI, traumatic brain injury; VAN, ventral attention network; VF, verbal fluency; VN, visual network; WM, white matter; WMS, working memory span; YA, younger adult.
Footnotes
- ^ adni.loni.usc.edu
- ^ https://surfer.nmr.mgh.harvard.edu
- ^ https://surfer.nmr.mgh.harvard.edu/fswiki/FsFast
References
Akatsuka, K., Noguchi, Y., Harada, T., Sadato, N., and Kakigi, R. (2008). Neural codes for somatosensory two-point discrimination in inferior parietal lobule: an fMRI study. Neuroimage 40, 852–858. doi: 10.1016/j.neuroimage.2007.12.013
Alexander, B. H., Barnes, H. M., Trimmer, E., Davidson, A. M., Ogola, B. O., Lindsey, S. H., et al. (2018). Stable density and dynamics of dendritic spines of cortical neurons across the estrous cycle while expressing differential levels of sensory-evoked plasticity. Front. Mol. Neurosci. 11:83. doi: 10.3389/fnmol.2018.00083
Alnawmasi, M. M., Chakraborty, A., Dalton, K., Quaid, P., Dunkley, B. T., and Thompson, B. (2019). The effect of mild traumatic brain injury on the visual processing of global form and motion. Brain Inj. 33, 1354–1363. doi: 10.1080/02699052.2019.1641842
Babbage, D. R., Zupan, B., Neumann, D., and Willer, B. (2018). Sex differences in response to emotion recognition training after traumatic brain injury. Brain Inj. 32, 1492–1499. doi: 10.1080/02699052.2018.1497811
Badhwar, A., Tam, A., Dansereau, C., Orban, P., Hoffstaedter, F., and Bellec, P. (2017). Resting-state network dysfunction in Alzheimer’s disease: a systematic review and meta-analysis. Alzheimer’s Dem. Diagn. Assess. Dis. Monit. 8, 73–85. doi: 10.1016/j.dadm.2017.03.007
Banaszkiewicz, A., Bola, L., Matuszewski, J., Szczepanik, M., Kossowski, B., Mostowski, P., et al. (2021). The role of the superior parietal lobule in lexical processing of sign language: insights from fMRI and TMS. Cortex 135, 240–254. doi: 10.1016/j.cortex.2020.10.025
Barbeau, E. B., Chai, X. J., Chen, J. K., Soles, J., Berken, J., Baum, S., et al. (2017). The role of the left inferior parietal lobule in second language learning: an intensive language training fMRI study. Neuropsychologia 98, 169–176. doi: 10.1016/j.neuropsychologia.2016.10.003
Bay, E., Sikorskii, A., and Saint-Arnault, D. (2009). Sex differences in depressive symptoms and their correlates after mild-to-moderate traumatic brain injury. J. Neurosci. Nurs. 41, 298–309. doi: 10.1097/jnn.0b013e3181b6be81
Binder, J. R., Frost, J. A., Hammeke, T. A., Cox, R. W., Rao, S. M., and Prieto, T. (1997). Human brain language areas identified by functional magnetic resonance imaging. J. Neurosci. 17, 353–362. doi: 10.1523/jneurosci.17-01-00353.1997
Bonnelle, V., Leech, R., Kinnunen, K. M., Ham, T. E., Beckmann, C. F., De Boissezon, X., et al. (2011). Default mode network connectivity predicts sustained attention deficits after traumatic brain injury. J. Neurosci. 31, 13442–13451. doi: 10.1523/JNEUROSCI.1163-11.2011
Borich, M., Babul, A.-N., Yuan, P. H., Boyd, L., and Virji-Babul, N. (2015). Alterations in resting-state brain networks in concussed adolescent athletes. J. Neurotr. 32, 265–271.
Bornhofen, C., and McDonald, S. (2008b). Emotion perception deficits following traumatic brain injury: a review of the evidence and rationale for intervention. J. Int. Neuropsychol. Soc. 14, 511–525. doi: 10.1017/S1355617708080703
Bornhofen, C., and McDonald, S. (2008a). Comparing strategies for treating emotion perception deficits in traumatic brain injury. J. Head Trauma Rehabil. 23, 103–115. doi: 10.1097/01.HTR.0000314529.22777.43
Bornhofen, C., and McDonald, S. (2008c). Treating deficits in emotion perception following traumatic brain injury. Neuropsychol. Rehabil. 18, 22–44. doi: 10.1080/09602010601061213
Braga, R. M., Fu, R. Z., Seemungal, B. M., Wise, R. J., and Leech, R. (2016). Eye movements during auditory attention predict individual differences in dorsal attention network activity. Front. Hum. Neurosci. 10:164. doi: 10.3389/fnhum.2016.00164
Broyd, S. J., Demanuele, C., Debener, S., Helps, S. K., James, C. J., and Sonuga-Barke, E. J. S. (2009). Default-mode brain dysfunction in mental disorders: a systematic review. Neurosci. Biobehav. Rev. 33, 279–296. doi: 10.1016/j.neubiorev.2008.09.002
Bunting, M. F., Cowan, N., and Colflesh, G. H. (2008). The deployment of attention in short-term memory tasks: trade-offs between immediate and delayed deployment. Memory Cogn. 36, 799–812. doi: 10.3758/mc.36.4.799
Butler, T., Imperato-Mcginley, J., Pan, H., Voyer, D., Cordero, J., Zhu, Y. S., et al. (2006). Sex differences in mental rotation: top-down versus bottom-up processing. Neuroimage 32, 445–456. doi: 10.1016/j.neuroimage.2006.03.030
Byom, L., Duff, M., Mutlu, B., and Turkstra, L. (2019). Facial emotion recognition of older adults with traumatic brain injury. Brain Inj. 33, 322–332. doi: 10.1080/02699052.2018.1553066
Caplan, H. W., Cox, C. S., and Bedi, S. S. (2017). Do microglia play a role in sex differences in TBI? J. Neurosci. Res. 95, 509–517. doi: 10.1002/jnr.23854
Catroppa, C., and Anderson, V. (1999). Recovery of educational skills following paediatric traumatic brain injury. Pediatr. Rehabil. 3, 167–175. doi: 10.1080/136384999289432
Cavanna, A. E., and Trimble, M. R. (2006). The precuneus: a review of its functional anatomy and behavioural correlates. Brain 129, 564–583. doi: 10.1093/brain/awl004
Chen, J., Shu, H., Wang, Z., Liu, D., Shi, Y., Xu, L., et al. (2016). Protective effect of APOE epsilon 2 on intrinsic functional connectivity of the entorhinal cortex is associated with better episodic memory in elderly individuals with risk factors for Alzheimer’s disease. Oncotarget 7, 58789–58801. doi: 10.18632/oncotarget.11289
Choi, G. S., Kim, O. L., Kim, S. H., Ahn, S. H., Cho, Y. W., Son, S. M., et al. (2012). Classification of cause of motor weakness in traumatic brain injury using diffusion tensor imaging. Arch. Neurol. 69, 363–367. doi: 10.1001/archneurol.2011.1930
Corbetta, M., and Shulman, G. L. (2002). Control of goal-directed and stimulus-driven attention in the brain. Nat. Rev. Neurosci. 3, 201–215. doi: 10.1038/nrn755
Covassin, T., and Bay, E. (2012). Are there gender differences in cognitive function, chronic stress, and neurobehavioral symptoms after mild-to-moderate traumatic brain injury? J. Neurosci. Nurs. 44, 124–133. doi: 10.1097/JNN.0b013e318252737d
Covassin, T., Swanik, C. B., Sachs, M., Kendrick, Z., Schatz, P., Zillmer, E., et al. (2006). Sex differences in baseline neuropsychological function and concussion symptoms of collegiate athletes. Br. J. Sports Med. 40, 923–927; discussion 927. doi: 10.1136/bjsm.2006.029496
Culham, J., He, S., Dukelow, S., and Verstraten, F. A. (2001). Visual motion and the human brain: what has neuroimaging told us? Acta Psychol. (Amst) 107, 69–94. doi: 10.1016/s0001-6918(01)00022-1
Culham, J. C., and Kanwisher, N. G. (2001). Neuroimaging of cognitive functions in human parietal cortex. Curr. Opin. Neurobiol. 11, 157–163. doi: 10.1016/s0959-4388(00)00191-4
Dale, A. M., Fischl, B., and Sereno, M. I. (1999). Cortical surface-based analysis: I. segmentation and surface reconstruction. Neuroimage 9, 179–194. doi: 10.1006/nimg.1998.0395
de la Plata, C. D. M., Hart, T., Hammond, F. M., Frol, A. B., Hudak, A., Harper, C. R., et al. (2008). Impact of age on long-term recovery from traumatic brain injury. Arch. Phys. Med. Rehabili. 89, 896–903. doi: 10.1016/j.apmr.2007.12.030
de Souza, N. L., Parker, R., Gonzalez, C. S., Ryan, J. D., and Esopenko, C. (2020). Effect of age at time of injury on long-term changes in intrinsic functional connectivity in traumatic brain injury. Brain Inj. 34, 1646–1654. doi: 10.1080/02699052.2020.1832257
DeAngelis, G. C., Ohzawa, I., and Freeman, R. D. (1995). Receptive-field dynamics in the central visual pathways. Trends Neurosci. 18, 451–458. doi: 10.1016/0166-2236(95)94496-r
Dehaene, S., and Dehaene-Lambertz, G. (2009). [Cognitive neuro-imaging : phylogenesis and ontogenesis]. Bull. Acad. Natl. Med. 193, 883–889.
DePompei, R., and Hotz, G. (2001). Pediatric speech and language disorders following TBI. J. Head. Trauma Rehabil. 16, vi–vii. doi: 10.1097/00001199-200110000-00001
DiGuiseppi, J., and Tadi, P. (2020). Neuroanatomy, Postcentral Gyrus. Treasure Island, FL: StatPearls Publishing.
Dimoska-Di Marco, A., Mcdonald, S., Kelly, M., Tate, R., and Johnstone, S. (2011). A meta-analysis of response inhibition and stroop interference control deficits in adults with traumatic brain injury (TBI). J. Clin. Exp. Neuropsychol. 33, 471–485. doi: 10.1080/13803395.2010.533158
Drager, B., Jansen, A., Bruchmann, S., Forster, A. F., Pleger, B., Zwitserlood, P., et al. (2004). How does the brain accommodate to increased task difficulty in word finding? A functional MRI study. Neuroimage 23, 1152–1160. doi: 10.1016/j.neuroimage.2004.07.005
du Boisgueheneuc, F., Levy, R., Volle, E., Seassau, M., Duffau, H., Kinkingnehun, S., et al. (2006). Functions of the left superior frontal gyrus in humans: a lesion study. Brain 129, 3315–3328. doi: 10.1093/brain/awl244
Dubner, R., and Zeki, S. M. (1971). Response properties and receptive fields of cells in an anatomically defined region of the superior temporal sulcus in the monkey. Brain Res. 35, 528–532. doi: 10.1016/0006-8993(71)90494-x
Engelen, T., De Graaf, T. A., Sack, A. T., and De Gelder, B. (2015). A causal role for inferior parietal lobule in emotion body perception. Cortex 73, 195–202. doi: 10.1016/j.cortex.2015.08.013
Eramudugolla, R., Bielak, A. A., Bunce, D., Easteal, S., Cherbuin, N., and Anstey, K. J. (2014). Long-term cognitive correlates of traumatic brain injury across adulthood and interactions with APOE genotype, sex, and age cohorts. J. Int. Neuropsychol. Soc. 20, 444–454.
Ewing-Cobbs, L., Barnes, M., Fletcher, J. M., Levin, H. S., Swank, P. R., and Song, J. (2004). Modeling of longitudinal academic achievement scores after pediatric traumatic brain injury. Dev. Neuropsychol. 25, 107–133. doi: 10.1080/87565641.2004.9651924
Ewing-Cobbs, L., Fletcher, J. M., Levin, H. S., Iovino, I., and Miner, M. E. (1998). Academic achievement and academic placement following traumatic brain injury in children and adolescents: a two-year longitudinal study. J. Clin. Exp. Neuropsychol. 20, 769–781. doi: 10.1076/jcen.20.6.769.1109
Faden, A. I., and Loane, D. J. (2015). Chronic neurodegeneration after traumatic brain injury: Alzheimer disease, chronic traumatic encephalopathy, or persistent neuroinflammation? Neurotherapeutics 12, 143–150. doi: 10.1007/s13311-014-0319-5
Farrant, K., and Uddin, L. Q. (2016). Atypical developmental of dorsal and ventral attention networks in autism. Dev. Sci. 19, 550–563. doi: 10.1111/desc.12359
Forlim, C. G., Klock, L., Bachle, J., Stoll, L., Giemsa, P., Fuchs, M., et al. (2020). Reduced resting-state connectivity in the precuneus is correlated with apathy in patients with schizophrenia. Sci. Rep. 10:2616. doi: 10.1038/s41598-020-59393-6
Frederikse, M. E., Lu, A., Aylward, E., Barta, P., and Pearlson, G. (1999). Sex differences in the inferior parietal lobule. Cereb Cortex 9, 896–901. doi: 10.1093/cercor/9.8.896
Furl, N., Garrido, L., Dolan, R. J., Driver, J., and Duchaine, B. (2011). Fusiform gyrus face selectivity relates to individual differences in facial recognition ability. J. Cogn. Neurosci. 23, 1723–1740. doi: 10.1162/jocn.2010.21545
Gagnon, I., Forget, R., Sullivan, S. J., and Friedman, D. (1998). Motor performance following a mild traumatic brain injury in children: an exploratory study. Brain Inj. 12, 843–853. doi: 10.1080/026990598122070
George, N., Dolan, R. J., Fink, G. R., Baylis, G. C., Russell, C., and Driver, J. (1999). Contrast polarity and face recognition in the human fusiform gyrus. Nat. Neurosci. 2, 574–580. doi: 10.1038/9230
Geranmayeh, F., Brownsett, S. L. E., Leech, R., Beckmann, C. F., Woodhead, Z., and Wise, R. J. S. (2012). The contribution of the inferior parietal cortex to spoken language production. Brain Lang. 121, 47–57. doi: 10.1016/j.bandl.2012.02.005
Gillis, M. M., and Hampstead, B. M. (2015). A two-part preliminary investigation of encoding-related activation changes after moderate to severe traumatic brain injury: hyperactivation, repetition suppression, and the role of the prefrontal cortex. Brain Imag. Behav. 9, 801–820. doi: 10.1007/s11682-014-9337-5
Gilmore, C. S., Camchong, J., Davenport, N. D., Nelson, N. W., Kardon, R. H., Lim, K. O., et al. (2016). Deficits in visual system functional connectivity after blast-related mild TBI are associated with injury severity and executive dysfunction. Brain Behav. 6:e00454. doi: 10.1002/brb3.454
Goldberg, G. (1985). Supplementary motor area structure and function: review and hypotheses. Behav. Brain Sci. 8, 567–588. doi: 10.1017/s0140525x00045167
Gölz, C., Kirchhoff, F. P., Westerhorstmann, J., Schmidt, M., Hirnet, T., Rune, G. M., et al. (2019). Sex hormones modulate pathogenic processes in experimental traumatic brain injury. J. Neurochem. 150, 173–187. doi: 10.1111/jnc.14678
Green, R. E., Turner, G. R., and Thompson, W. F. (2004). Deficits in facial emotion perception in adults with recent traumatic brain injury. Neuropsychologia 42, 133–141. doi: 10.1016/j.neuropsychologia.2003.07.005
Greenwood, P. M. (2007). Functional plasticity in cognitive aging: review and hypothesis. Neuropsychology 21, 657–673. doi: 10.1037/0894-4105.21.6.657
Grodd, W., Hulsmann, E., Lotze, M., Wildgruber, D., and Erb, M. (2001). Sensorimotor mapping of the human cerebellum: fMRI evidence of somatotopic organization. Hum. Brain Mapp. 13, 55–73. doi: 10.1002/hbm.1025
Guell, X., Arnold Anteraper, S., Gardner, A. J., Whitfield-Gabrieli, S., Kay-Lambkin, F., Iverson, G. L., et al. (2020). Functional connectivity changes in retired rugby league players: a data-driven functional magnetic resonance imaging study. J. Neurotr. 37, 1788–1796. doi: 10.1089/neu.2019.6782
Gupte, R., Brooks, W., Vukas, R., Pierce, J., and Harris, J. (2019). Sex differences in traumatic brain injury: what we know and what we should know. J. Neurotr. 36, 3063–3091. doi: 10.1089/neu.2018.6171
Halgren, E., Sherfey, J., Irimia, A., Dale, A. M., and Marinkovic, K. (2011). Sequential temporo-fronto-temporal activation during monitoring of the auditory environment for temporal patterns. Hum. Brain Mapp. 32, 1260–1276. doi: 10.1002/hbm.21106
Han, K., Chapman, S. B., and Krawczyk, D. C. (2016). Disrupted intrinsic connectivity among default, dorsal attention, and frontoparietal control networks in individuals with chronic traumatic brain injury. J. Neuropsychol. Soc. 22, 263–279. doi: 10.1017/S1355617715001393
Hawes, D. R., Vostroknutov, A., and Rustichini, A. (2012). Experience and abstract reasoning in learning backward induction. Front. Neurosci. 6:23. doi: 10.3389/fnins.2012.00023
Hillary, F., Slocomb, J., Hills, E., Fitzpatrick, N., Medaglia, J., Wang, J., et al. (2011). Changes in resting connectivity during recovery from severe traumatic brain injury. Int. J. Psychophysiol. 82, 115–123. doi: 10.1016/j.ijpsycho.2011.03.011
Hochberg, Y., and Benjamini, Y. (1990). More powerful procedures for multiple significance testing. Stat. Med. 9, 811–818. doi: 10.1002/sim.4780090710
Hong, Z., Ng, K. K., Sim, S. K. Y., Ngeow, M. Y., Zheng, H., Lo, J. C., et al. (2015). Differential age-dependent associations of gray matter volume and white matter integrity with processing speed in healthy older adults. NeuroImage 123, 42–50. doi: 10.1016/j.neuroimage.2015.08.034
Hsu, H. L., Chen, D. Y., Tseng, Y. C., Kuo, Y. S., Huang, Y. L., Chiu, W. T., et al. (2015). Sex differences in working memory after mild traumatic brain injury: a functional MR imaging study. Radiology 276, 828–835. doi: 10.1148/radiol.2015142549
Hsu, H. L., Yen-Ting Chen, D., Tseng, Y. C., Kuo, Y. S., Huang, Y. L., Chiu, W. T., et al. (2016). Sex differences in working memory after mild traumatic brain injury: a functional MR Imaging Study. Radiology 280:653. doi: 10.1148/radiol.2016164022
Hubber, P. J., Gilmore, C., and Cragg, L. (2014). The roles of the central executive and visuospatial storage in mental arithmetic: a comparison across strategies. Quart. J. Exp. Psychol. 67, 936–954. doi: 10.1080/17470218.2013.838590
Hunt, A. W., Mah, K., Reed, N., Engel, L., and Keightley, M. (2016). Oculomotor-based vision assessment in mild traumatic brain injury: a systematic review. J. Head. Trauma Rehabil. 31, 252–261. doi: 10.1097/HTR.0000000000000174
Iacoboni, M., Woods, R. P., Lenzi, G. L., and Mazziotta, J. C. (1997). Merging of oculomotor and somatomotor space coding in the human right precentral gyrus. Brain 120(Pt. 9), 1635–1645. doi: 10.1093/brain/120.9.1635
Irimia, A., and Bradshaw, L. A. (2005a). Artifact reduction in magnetogastrography using fast independent component analysis. Physiol. Meas 26, 1059–1073. doi: 10.1088/0967-3334/26/6/015
Irimia, A., and Bradshaw, L. A. (2005b). Ellipsoidal electrogastrographic forward modelling. Phys. Med. Biol. 50, 4429–4444. doi: 10.1088/0031-9155/50/18/012
Irimia, A., Goh, S. Y., Torgerson, C. M., Chambers, M. C., Kikinis, R., and Van Horn, J. D. (2013a). Forward and inverse electroencephalographic modeling in health and in acute traumatic brain injury. Clin. Neurophysiol. 124, 2129–2145. doi: 10.1016/j.clinph.2013.04.336
Irimia, A., Goh, S. Y., Torgerson, C. M., Stein, N. R., Chambers, M. C., Vespa, P. M., et al. (2013b). Electroencephalographic inverse localization of brain activity in acute traumatic brain injury as a guide to surgery, monitoring and treatment. Clin. Neurol. Neur. 115, 2159–2165. doi: 10.1016/j.clineuro.2013.08.003
Irimia, A., Maher, A. S., Chaudhari, N. N., Chowdhury, N. F., and Jacobs, E. B. (2020). Acute cognitive deficits after traumatic brain injury predict Alzheimer’s disease-like degradation of the human default mode network. GeroScience 42, 1411–1429. doi: 10.1007/s11357-020-00245-6
Irimia, A., Torgerson, C. M., Goh, S. Y., and Van Horn, J. D. (2015). Statistical estimation of physiological brain age as a descriptor of senescence rate during adulthood. Brain Imaging Behav. 9, 678–689. doi: 10.1007/s11682-014-9321-0
Irimia, A., and Van Horn, J. D. (2015). Functional neuroimaging of traumatic brain injury: advances and clinical utility. Neuropsychiatr Dis. Treat. 11, 2355–2365. doi: 10.2147/NDT.S79174
Iverson, K. M., Hendricks, A. M., Kimerling, R., Krengel, M., Meterko, M., Stolzmann, K. L., et al. (2011). Psychiatric diagnoses and neurobehavioral symptom severity among OEF/OIF VA patients with deployment-related traumatic brain injury: a gender comparison. Women’s Health Issues 21, S210–S217. doi: 10.1016/j.whi.2011.04.019
Iverson, K. M., Pogoda, T. K., Gradus, J. L., and Street, A. E. (2013). Deployment-related traumatic brain injury among operation enduring freedom/operation iraqi freedom veterans: associations with mental and physical health by gender. J. Women’s Health 22, 267–275. doi: 10.1089/jwh.2012.3755
Jack, C. R. Jr., Dickson, D. W., Parisi, J. E., Xu, Y. C., Cha, R. H., O’Brien, P. C., et al. (2002). Antemortem MRI findings correlate with hippocampal neuropathology in typical aging and dementia. Neurology 58, 750–757. doi: 10.1212/wnl.58.5.750
Jastorff, J., Clavagnier, S., Gergely, G., and Orban, G. A. (2011). Neural mechanisms of understanding rational actions: middle temporal gyrus activation by contextual violation. Cereb Cortex 21, 318–329. doi: 10.1093/cercor/bhq098
Jiang, L., Cao, X., Li, T., Tang, Y., Li, W., Wang, J., et al. (2016). Cortical thickness changes correlate with cognition changes after cognitive training: evidence from a chinese community study. Front. Aging Neurosci. 8:118–118. doi: 10.3389/fnagi.2016.00118
Jiao, Y., Lin, F., Wu, J., Li, H., Fu, W., Huo, R., et al. (2020). Plasticity in language cortex and white matter tracts after resection of dominant inferior parietal lobule arteriovenous malformations: a combined fMRI and DTI study. J. Neur. 2020, 1–8. doi: 10.3171/2019.12.JNS191987
Just, M. A., Carpenter, P. A., Keller, T. A., Eddy, W. F., and Thulborn, K. R. (1996). Brain activation modulated by sentence comprehension. Science 274, 114–116. doi: 10.1126/science.274.5284.114
Kaestner, E., Wu, X., Friedman, D., Dugan, P., Devinsky, O., Carlson, C., et al. (2021). The precentral gyrus contributions to the early time-course of grapheme-to-phoneme conversion. Neurobiol. Lang. 2, 1–28.
Kanwisher, N., Mcdermott, J., and Chun, M. M. (1997). The fusiform face area: a module in human extrastriate cortex specialized for face perception. J. Neurosci. 17, 4302–4311. doi: 10.1523/jneurosci.17-11-04302.1997
Karbasforoushan, H., Duffy, B., Blackford, J. U., and Woodward, N. D. (2015). Processing speed impairment in schizophrenia is mediated by white matter integrity. Psychol. Med. 45, 109–120. doi: 10.1017/S0033291714001111
Kasahara, M., Menon, D. K., Salmond, C. H., Outtrim, J. G., Tavares, J. V. T., Carpenter, T. A., et al. (2011). Traumatic brain injury alters the functional brain network mediating working memory. Brain Inj. 25, 1170–1187. doi: 10.3109/02699052.2011.608210
Kawabata, K., Watanabe, H., Hara, K., Bagarinao, E., Yoneyama, N., Ogura, A., et al. (2018). Distinct manifestation of cognitive deficits associate with different resting-state network disruptions in non-demented patients with Parkinson’s disease. J. Neurol. 265, 688–700. doi: 10.1007/s00415-018-8755-5
Khalsa, S. S., Rudrauf, D., Feinstein, J. S., and Tranel, D. (2009). The pathways of interoceptive awareness. Nat. Neurosci. 12, 1494–1496. doi: 10.1038/nn.2411
Killiany, R. J., Gomez-Isla, T., Moss, M., Kikinis, R., Sandor, T., Jolesz, F., et al. (2000). Use of structural magnetic resonance imaging to predict who will get Alzheimer’s disease. Ann. Neurol. 47, 430–439.
Kinsella, G., Prior, M., Sawyer, M., Murtagh, D., Eisenmajer, R., Anderson, V., et al. (1995). Neuropsychological deficit and academic performance in children and adolescents following traumatic brain injury. J. Pediatr. Psychol. 20, 753–767. doi: 10.1093/jpepsy/20.6.753
Kliegel, M., Martin, M., and Jager, T. (2007). Development and validation of the cognitive telephone screening instrument (COGTEL) for the assessment of cognitive function across adulthood. J. Psychol. 141, 147–170. doi: 10.3200/JRLP.141.2.147-172
Knox, L., and Douglas, J. (2009). Long-term ability to interpret facial expression after traumatic brain injury and its relation to social integration. Brain Cogn. 69, 442–449. doi: 10.1016/j.bandc.2008.09.009
Kochunov, P., Coyle, T., Lancaster, J., Robin, D. A., Hardies, J., Kochunov, V., et al. (2010). Processing speed is correlated with cerebral health markers in the frontal lobes as quantified by neuroimaging. Neuroimage 49, 1190–1199. doi: 10.1016/j.neuroimage.2009.09.052
Koebli, J. R., Balasubramanian, V., and Zipp, G. P. (2020). An exploration of higher-level language comprehension deficits and factors influencing them following blast TBI in US veterans. Brain Inj. 34, 630–641. doi: 10.1080/02699052.2020.1725845
Konstantinou, N., Pettemeridou, E., Stamatakis, E. A., Seimenis, I., and Constantinidou, F. (2018). Altered resting functional connectivity is related to cognitive outcome in males with moderate-severe traumatic brain injury. Front. Neurol. 9:1163. doi: 10.3389/fneur.2018.01163
Kordower, J. H., Chu, Y., Stebbins, G. T., Dekosky, S. T., Cochran, E. J., Bennett, D., et al. (2001). Loss and atrophy of layer II entorhinal cortex neurons in elderly people with mild cognitive impairment. Ann. Neurol. 49, 202–213. doi: 10.1002/1531-8249(20010201)49:2<202::aid-ana40>3.0.co;2-3
Kramer, M. E., Chiu, C.-Y. P., Walz, N. C., Holland, S. K., Yuan, W., Karunanayaka, P., et al. (2008). Long-term neural processing of attention following early childhood traumatic brain injury: fMRI and neurobehavioral outcomes. J. Int. Neuropsychol. Soc. 14, 424–435. doi: 10.1017/S1355617708080545
Kraus, M. F., Little, D. M., Donnell, A. J., Reilly, J. L., Simonian, N., and Sweeney, J. A. (2007). Oculomotor function in chronic traumatic brain injury. Cogn. Behav. Neurol. 20, 170–178. doi: 10.1097/WNN.0b013e318142badb
Kuhtz-Buschbeck, J. P., Hoppe, B., Golge, M., Dreesmann, M., Damm-Stunitz, U., and Ritz, A. (2003). Sensorimotor recovery in children after traumatic brain injury: analyses of gait, gross motor, and fine motor skills. Dev. Med. Child. Neurol. 45, 821–828. doi: 10.1017/s001216220300152x
Kwon, H. G., and Jang, S. H. (2011). Optic radiation injury following traumatic epidural hematoma: diffusion tensor imaging study. NeuroRehabilitation 28, 383–387. doi: 10.3233/NRE-2011-0667
La, C., Young, B. M., Garcia-Ramos, C., Nair, V. A., and Prabhakaran, V. (2014). Characterizing recovery of the human brain following stroke: evidence from fMRI studies. Imaging Hum. Brain Health Dis. 2014, 485–506. doi: 10.1016/b978-0-12-418677-4.00020-8
Lachman, M. E., Agrigoroaei, S., Tun, P. A., and Weaver, S. L. (2014). Monitoring cognitive functioning: psychometric properties of the brief test of adult cognition by telephone. Assessment 21, 404–417. doi: 10.1177/1073191113508807
Lane, R. D., Reiman, E. M., Axelrod, B., Yun, L. S., Holmes, A., and Schwartz, G. E. (1998). Neural correlates of levels of emotional awareness. evidence of an interaction between emotion and attention in the anterior cingulate cortex. J. Cogn. Neurosci. 10, 525–535. doi: 10.1162/089892998562924
Larsson, J., and Heeger, D. J. (2006). Two retinotopic visual areas in human lateral occipital cortex. J. Neurosci. 26, 13128–13142. doi: 10.1523/JNEUROSCI.1657-06.2006
LeBlanc, J., Guise, E. D., Gosselin, N., and Feyz, M. (2006). Comparison of functional outcome following acute care in young, middle-aged and elderly patients with traumatic brain injury. Brain Inj. 20, 779–790. doi: 10.1080/02699050600831835
LeBlanc, J., Seresova, A., Laberge-Poirier, A., Tabet, S., Alturki, A. Y., Feyz, M., et al. (2021). Cognitive-communication performance following mild traumatic brain injury: Influence of sex, age, education, site of lesion and bilingualism. Int. J. Lang. Commun. Dis. 56, 130–144. doi: 10.1111/1460-6984.12589
Li, C. S., Huang, C., Constable, R. T., and Sinha, R. (2006). Gender differences in the neural correlates of response inhibition during a stop signal task. Neuroimage 32, 1918–1929. doi: 10.1016/j.neuroimage.2006.05.017
Linden, D. E. (2007). The working memory networks of the human brain. Neurosci. 13, 257–267. doi: 10.1177/1073858406298480
Liu, X., Chen, W., Hou, H., Chen, X., Zhang, J., Liu, J., et al. (2017). Decreased functional connectivity between the dorsal anterior cingulate cortex and lingual gyrus in Alzheimer’s disease patients with depression. Behav. Brain Res. 326, 132–138. doi: 10.1016/j.bbr.2017.01.037
Livny, A., Biegon, A., Kushnir, T., Harnof, S., Hoffmann, C., Fruchter, E., et al. (2017). Cognitive deficits post-traumatic brain injury and their association with injury severity and gray matter volumes. J. Neurotr. 34, 1466–1472. doi: 10.1089/neu.2016.4598
Mallas, E. J., De Simoni, S., Scott, G., Jolly, A. E., Hampshire, A., Li, L. M., et al. (2021). Abnormal dorsal attention network activation in memory impairment after traumatic brain injury. Brain 144, 114–127. doi: 10.1093/brain/awaa380
Matuskova, V., Ismail, Z., Nikolai, T., Markova, H., Cechova, K., Nedelska, Z., et al. (2021). Mild behavioral impairment is associated with atrophy of entorhinal cortex and hippocampus in a memory clinic cohort. Front. Aging Neurosci. 13:643271–643271. doi: 10.3389/fnagi.2021.643271
Maunsell, J. H., and Van Essen, D. C. (1983). Functional properties of neurons in middle temporal visual area of the macaque monkey. I. selectivity for stimulus direction, speed, and orientation. J. Neurophysiol. 49, 1127–1147. doi: 10.1152/jn.1983.49.5.1127
Mayer, A. R., Dorflinger, J. M., Rao, S. M., and Seidenberg, M. (2004). Neural networks underlying endogenous and exogenous visual–spatial orienting. NeuroImage 23, 534–541. doi: 10.1016/j.neuroimage.2004.06.027
Mercadillo, R. E., Diaz, J. L., Pasaye, E. H., and Barrios, F. A. (2011). Perception of suffering and compassion experience: brain gender disparities. Brain Cogn. 76, 5–14. doi: 10.1016/j.bandc.2011.03.019
Moen, K. T., Jorgensen, L., Olsen, A., Haberg, A., Skandsen, T., Vik, A., et al. (2014). High-level mobility in chronic traumatic brain injury and its relationship with clinical variables and magnetic resonance imaging findings in the acute phase. Arch. Phys. Med. Rehabil. 95, 1838–1845. doi: 10.1016/j.apmr.2014.04.014
Moore, D. W., Ashman, T. A., Cantor, J. B., Krinick, R. J., and Spielman, L. A. (2010). Does gender influence cognitive outcome after traumatic brain injury? Neuropsychol. Rehabil. 20, 340–354. doi: 10.1080/09602010903250928
Mosenthal, A. C., Lavery, R. F., Addis, M., Kaul, S., Ross, S., Marburger, R., et al. (2002). Isolated traumatic brain injury: age is an independent predictor of mortality and early outcome. J. Trauma 52, 907–911. doi: 10.1097/00005373-200205000-00015
Mushkudiani, N. A., Engel, D. C., Steyerberg, E. W., Butcher, I., Lu, J., Marmarou, A., et al. (2007). Prognostic value of demographic characteristics in traumatic brain injury: results from the IM.PACT study. J Neurotr. 24, 259–269. doi: 10.1089/neu.2006.0028
Nagy, K., Greenlee, M. W., and Kovacs, G. (2012). The lateral occipital cortex in the face perception network: an effective connectivity study. Front. Psychol. 3:141. doi: 10.3389/fpsyg.2012.00141
Nakata, H., Sakamoto, K., Ferretti, A., Gianni Perrucci, M., Del Gratta, C., Kakigi, R., et al. (2009). Negative BOLD effect on somato-motor inhibitory processing: an fMRI study. Neurosci. Lett. 462, 101–104. doi: 10.1016/j.neulet.2009.06.088
Niemeier, J. P., Marwitz, J. H., Lesher, K., Walker, W. C., and Bushnik, T. (2007). Gender differences in executive functions following traumatic brain injury. Neuropsychol. Rehabil. 17, 293–313. doi: 10.1080/09602010600814729
Nowicki, S. Jr., and Carton, J. (1993). The measurement of emotional intensity from facial expressions. J. Soc. Psychol. 133, 749–750. doi: 10.1080/00224545.1993.9713934
Odish, O. F., Van Den Berg-Huysmans, A. A., Van Den Bogaard, S. J., Dumas, E. M., Hart, E. P., Rombouts, S. A., et al. (2015). Longitudinal resting state fMRI analysis in healthy controls and premanifest Huntington’s disease gene carriers: a three-year follow-up study. Hum. Brain Mapp. 36, 110–119. doi: 10.1002/hbm.22616
Ousdal, O. T., Kaufmann, T., Kolskar, K., Vik, A., Wehling, E., Lundervold, A. J., et al. (2020). Longitudinal stability of the brain functional connectome is associated with episodic memory performance in aging. Hum. Brain Mapp. 41, 697–709. doi: 10.1002/hbm.24833
Palacios, E. M., Yuh, E. L., Chang, Y. S., Yue, J. K., Schnyer, D. M., Okonkwo, D. O., et al. (2017). Resting-state functional connectivity alterations associated with six-month outcomes in mild traumatic brain injury. J. Neurotr. 34, 1546–1557. doi: 10.1089/neu.2016.4752
Papoutsi, M., De Zwart, J. A., Jansma, J. M., Pickering, M. J., Bednar, J. A., and Horwitz, B. (2009). From phonemes to articulatory codes: an fMRI study of the role of Broca’s area in speech production. Cereb Cortex 19, 2156–2165.
Pei, Y. L., and O’Brien, K. H. (2021). Reading abilities post traumatic brain injury in adolescents and adults: a systematic review and meta-analysis. Am. J. Speech Lang. Pathol. 30, 789–816. doi: 10.1044/2020_AJSLP-20-00213
Penfield, W., and Boldrey, E. (1937). Somatic motor and sensory representation in the cerebral cortex of man as studied by electrical stimulation. Brain 60, 389–443.
Petersen, R. C., Aisen, P. S., Beckett, L. A., Donohue, M. C., Gamst, A. C., Harvey, D. J., et al. (2010). Alzheimer’s disease neuroimaging initiative (ADNI): clinical characterization. Neurology 74, 201–209. doi: 10.1212/WNL.0b013e3181cb3e25
Prigatano, G. P., and Pribram, K. H. (1982). Perception and memory of facial affect following brain injury. Percept Mot Skills 54, 859–869. doi: 10.2466/pms.1982.54.3.859
Rajalingham, R., Kar, K., Sanghavi, S., Dehaene, S., and Dicarlo, J. J. (2020). The inferior temporal cortex is a potential cortical precursor of orthographic processing in untrained monkeys. Nat. Commun. 11:3886. doi: 10.1038/s41467-020-17714-3
Ratcliff, J. J., Greenspan, A. I., Goldstein, F. C., Stringer, A. Y., Bushnik, T., Hammond, F. M., et al. (2007). Gender and traumatic brain injury: do the sexes fare differently? Brain Inj. 21, 1023–1030. doi: 10.1080/02699050701633072
Richard, N. M., O’connor, C., Dey, A., Robertson, I. H., and Levine, B. (2018). External modulation of the sustained attention network in traumatic brain injury. Neuropsychology 32, 541–553. doi: 10.1037/neu0000442
Rigon, A., Duff, M. C., Mcauley, E., Kramer, A. F., and Voss, M. W. (2016a). Is traumatic brain injury associated with reduced inter-hemispheric functional connectivity? A study of large-scale resting state networks following traumatic brain injury. J. Neurotr. 33, 977–989. doi: 10.1089/neu.2014.3847
Rigon, A., Turkstra, L., Mutlu, B., and Duff, M. (2016b). The female advantage: sex as a possible protective factor against emotion recognition impairment following traumatic brain injury. Cogn. Affect. Behav. Neurosci. 16, 866–875. doi: 10.3758/s13415-016-0437-0
Robles, D. J., Dharani, A., Rostowsky, K. A., Chaudhari, N. N., Ngo, V., Zhang, F., et al. (2021). Older Age, Male Sex, and Cerebral Microbleeds Predict White Matter Loss After Traumatic Brain Injury [Epub ahead of print].
Rosenberg-Lee, M., Barth, M., and Menon, V. (2011). What difference does a year of schooling make? Maturation of brain response and connectivity between 2nd and 3rd grades during arithmetic problem solving. Neuroimage 57, 796–808. doi: 10.1016/j.neuroimage.2011.05.013
Rotshtein, P., Malach, R., Hadar, U., Graif, M., and Hendler, T. (2001). Feeling or features: different sensitivity to emotion in high-order visual cortex and amygdala. Neuron 32, 747–757. doi: 10.1016/s0896-6273(01)00513-x
Saban, K. L., Smith, B. M., Collins, E. G., and Pape, T. L. (2011). Sex differences in perceived life satisfaction and functional status one year after severe traumatic brain injury. J. Womens Health (Larchmt) 20, 179–186. doi: 10.1089/jwh.2010.2334
Sanchez-Carrion, R., Fernandez-Espejo, D., Junque, C., Falcon, C., Bargallo, N., Roig, T., et al. (2008). A longitudinal fMRI study of working memory in severe TBI patients with diffuse axonal injury. Neuroimage 43, 421–429. doi: 10.1016/j.neuroimage.2008.08.003
Santhanam, P., Teslovich, T., Wilson, S. H., Yeh, P.-H., Oakes, T. R., and Weaver, L. K. (2019). Decreases in white matter integrity of ventro-limbic pathway linked to post-traumatic stress disorder in mild traumatic brain injury. J. Neurotr. 36, 1093–1098. doi: 10.1089/neu.2017.5541
Schremm, A., Noven, M., Horne, M., Soderstrom, P., Van Westen, D., and Roll, M. (2018). Cortical thickness of planum temporale and pars opercularis in native language tone processing. Brain Lang. 176, 42–47. doi: 10.1016/j.bandl.2017.12.001
Scolari, M., Seidl-Rathkopf, K. N., and Kastner, S. (2015). Functions of the human frontoparietal attention network: evidence from neuroimaging. Curr. Opin. Behav. Sci. 1, 32–39. doi: 10.1016/j.cobeha.2014.08.003
Segal, E., and Petrides, M. (2012). The anterior superior parietal lobule and its interactions with language and motor areas during writing. Eur. J. Neurosci. 35, 309–322. doi: 10.1111/j.1460-9568.2011.07937.x
Senathi-Raja, D., Ponsford, J., and Schonberger, M. (2010). Impact of age on long-term cognitive function after traumatic brain injury. Neuropsychology 24, 336–344. doi: 10.1037/a0018239
Sharp, D. J., Beckmann, C. F., Greenwood, R., Kinnunen, K. M., Bonnelle, V., De Boissezon, X., et al. (2011). Default mode network functional and structural connectivity after traumatic brain injury. Brain 134, 2233–2247. doi: 10.1093/brain/awr175
Shumskaya, E., Andriessen, T. M., Norris, D. G., and Vos, P. E. (2012). Abnormal whole-brain functional networks in homogeneous acute mild traumatic brain injury. Neurology 79, 175–182. doi: 10.1212/WNL.0b013e31825f04fb
Siegel, M., Donner, T. H., Oostenveld, R., Fries, P., and Engel, A. K. (2008). Neuronal synchronization along the dorsal visual pathway reflects the focus of spatial attention. Neuron 60, 709–719. doi: 10.1016/j.neuron.2008.09.010
Singh, R., Mason, S., Lecky, F., and Dawson, J. (2018). Prevalence of depression after TBI in a prospective cohort: the SHEFBIT study. Brain Inj. 32, 84–90. doi: 10.1080/02699052.2017.1376756
Slobounov, S. M., Gay, M., Zhang, K., Johnson, B., Pennell, D., Sebastianelli, W., et al. (2011). Alteration of brain functional network at rest and in response to YMCA physical stress test in concussed athletes: RsFMRI study. Neuroimage 55, 1716–1727. doi: 10.1016/j.neuroimage.2011.01.024
Southwell, D. G., Riva, M., Jordan, K., Caverzasi, E., Li, J., Perry, D. W., et al. (2017). Language outcomes after resection of dominant inferior parietal lobule gliomas. J. Neur. 127, 781–789. doi: 10.3171/2016.8.JNS16443
Steel, J., Ferguson, A., Spencer, E., and Togher, L. (2017). Language and cognitive communication disorder during post-traumatic amnesia: profiles of recovery after TBI from three cases. Brain Inj. 31, 1889–1902. doi: 10.1080/02699052.2017.1373200
Stein, D. G. (2008). Progesterone exerts neuroprotective effects after brain injury. Brain Res. Rev. 57, 386–397. doi: 10.1016/j.brainresrev.2007.06.012
Stevens, M. C., Lovejoy, D., Kim, J., Oakes, H., Kureshi, I., and Witt, S. T. (2012). Multiple resting state network functional connectivity abnormalities in mild traumatic brain injury. Brain Imag. Behav. 6, 293–318. doi: 10.1007/s11682-012-9157-4
Takayama, Y., Kinomoto, K., and Nakamura, K. (2004). Selective impairment of the auditory-verbal short-term memory due to a lesion of the superior temporal gyrus. Eur. Neurol. 51, 115–117. doi: 10.1159/000076792
Takehara-Nishiuchi, K. (2014). Entorhinal cortex and consolidated memory. Neurosci. Res. 84, 27–33. doi: 10.1016/j.neures.2014.02.012
Tallon-Baudry, C., Bertrand, O., Henaff, M. A., Isnard, J., and Fischer, C. (2005). Attention modulates gamma-band oscillations differently in the human lateral occipital cortex and fusiform gyrus. Cereb Cortex 15, 654–662. doi: 10.1093/cercor/bhh167
Tun, P. A., and Lachman, M. E. (2006). Telephone assessment of cognitive function in adulthood: the brief test of adult cognition by telephone. Age Ageing 35, 629–632. doi: 10.1093/ageing/afl095
Turken, U., Whitfield-Gabrieli, S., Bammer, R., Baldo, J. V., Dronkers, N. F., and Gabrieli, J. D. E. (2008). Cognitive processing speed and the structure of white matter pathways: convergent evidence from normal variation and lesion studies. NeuroImage 42, 1032–1044. doi: 10.1016/j.neuroimage.2008.03.057
Turkstra, L. S., Dixon, T. M., and Baker, K. K. (2004). Theory of mind and social beliefs in adolescents with traumatic brain injury. NeuroRehabilitation 19, 245–256. doi: 10.3233/nre-2004-19307
Turkstra, L. S., Mutlu, B., Ryan, C. W., Despins Stafslien, E. H., Richmond, E. K., Hosokawa, E., et al. (2020). Sex and gender differences in emotion recognition and theory of mind after TBI: a narrative review and directions for future research. Front. Neurol. 11:59. doi: 10.3389/fneur.2020.00059
Umarova, R. M., Saur, D., Schnell, S., Kaller, C. P., Vry, M.-S., Glauche, V., et al. (2010). Structural connectivity for visuospatial attention: significance of ventral pathways. Cerebral cortex 20, 121–129. doi: 10.1093/cercor/bhp086
Umbach, G., Rugg, M., Worrell, G., Sperling, M., Gross, R., Jobst, B., et al. (2019). Intracranial EEG reveals bihemispheric parietal and extra parietal brain networks supporting mental arithmetic. bioRxiv. * bioRxiv, 542142,
Unsworth, N., Miller, A. L., and Robison, M. K. (2019). Individual differences in encoding strategies and free recall dynamics. Quart. J. Exp. Psychol. 72, 2495–2508. doi: 10.1177/1747021819847441
Utevsky, A. V., Smith, D. V., and Huettel, S. A. (2014). Precuneus is a functional core of the default-mode network. J. Neurosci. 34, 932–940. doi: 10.1523/jneurosci.4227-13.2014
Vakhtin, A. A., Calhoun, V. D., Jung, R. E., Prestopnik, J. L., Taylor, P. A., and Ford, C. C. (2013). Changes in intrinsic functional brain networks following blast-induced mild traumatic brain injury. Brain Inj. 27, 1304–1310. doi: 10.3109/02699052.2013.823561
Valentine, T., Powell, J., Davidoff, J., Letson, S., and Greenwood, R. (2006). Prevalence and correlates of face recognition impairments after acquired brain injury. Neuropsychol. Rehabil. 16, 272–297. doi: 10.1080/09602010500176443
Velayudhan, L., Proitsi, P., Westman, E., Muehlboeck, J. S., Mecocci, P., Vellas, B., et al. (2013). Entorhinal cortex thickness predicts cognitive decline in Alzheimer’s disease. J. Alzheimers Dis. 33, 755–766. doi: 10.3233/JAD-2012-121408
Vergara, V. M., Mayer, A. R., Damaraju, E., Kiehl, K. A., and Calhoun, V. (2017). Detection of mild traumatic brain injury by machine learning classification using resting state functional network connectivity and fractional anisotropy. J. Neurotr. 34, 1045–1053. doi: 10.1089/neu.2016.4526
Vossel, S., Geng, J. J., and Fink, G. R. (2014). Dorsal and ventral attention systems: distinct neural circuits but collaborative roles. Neuroscientist 20, 150–159. doi: 10.1177/1073858413494269
Wang, L., Li, Y., Metzak, P., He, Y., and Woodward, T. S. (2010). Age-related changes in topological patterns of large-scale brain functional networks during memory encoding and recognition. Neuroimage 50, 862–872. doi: 10.1016/j.neuroimage.2010.01.044
Wang, S., Hu, L., Cao, J., Huang, W., Sun, C., Zheng, D., et al. (2018). Sex differences in abnormal intrinsic functional connectivity after acute mild traumatic brain injury. Front. Neural. Circ. 12:107. doi: 10.3389/fncir.2018.00107
Weiner, K. S., and Zilles, K. (2016). The anatomical and functional specialization of the fusiform gyrus. Neuropsychologia 83, 48–62. doi: 10.1016/j.neuropsychologia.2015.06.033
Yeo, B. T., Krienen, F. M., Sepulcre, J., Sabuncu, M. R., Lashkari, D., Hollinshead, M., et al. (2011). The organization of the human cerebral cortex estimated by intrinsic functional connectivity. J. Neurophysiol. 106, 1125–1165. doi: 10.1152/jn.00338.2011
Zago, L., and Tzourio-Mazoyer, N. (2002). Distinguishing visuospatial working memory and complex mental calculation areas within the parietal lobes. Neurosci. Lett. 331, 45–49. doi: 10.1016/s0304-3940(02)00833-9
Zhou, M., Zhang, F., Zhao, L., Qian, J., and Dong, C. (2016). Entorhinal cortex: a good biomarker of mild cognitive impairment and mild Alzheimer’s disease. Rev. Neurosci. 27, 185–195. doi: 10.1515/revneuro-2015-0019
Zhu, Y., Li, Z., Bai, L., Tao, Y., Sun, C., Li, M., et al. (2014). Loss of microstructural integrity in the limbic-subcortical networks for acute symptomatic traumatic brain injury. Biomed Res. Int. 2014:548392. doi: 10.1155/2014/548392
Keywords: functional neuroimaging, human connectome, neurophysiology, default-mode network, attention, limbic system
Citation: Amgalan A, Maher AS, Imms P, Ha MY, Fanelle TA and Irimia A (2022) Functional Connectome Dynamics After Mild Traumatic Brain Injury According to Age and Sex. Front. Aging Neurosci. 14:852990. doi: 10.3389/fnagi.2022.852990
Received: 11 January 2022; Accepted: 05 April 2022;
Published: 18 May 2022.
Edited by:
Ian M. McDonough, University of Alabama, United StatesReviewed by:
Corina Bondi, University of Pittsburgh, United StatesJames Meabon, University of Washington, United States
Erica Kornblith, University of California, San Francisco, United States
Copyright © 2022 Amgalan, Maher, Imms, Ha, Fanelle and Irimia. This is an open-access article distributed under the terms of the Creative Commons Attribution License (CC BY). The use, distribution or reproduction in other forums is permitted, provided the original author(s) and the copyright owner(s) are credited and that the original publication in this journal is cited, in accordance with accepted academic practice. No use, distribution or reproduction is permitted which does not comply with these terms.
*Correspondence: Andrei Irimia, aXJpbWlhQHVzYy5lZHU=
†Data used in this study were obtained from the Alzheimer’s Disease Neuroimaging Initiative (ADNI) database (adni.loni.usc.edu). The investigators of the ADNI contributed to the design and implementation of ADNI and/or provided data but did not participate in the analysis or writing of this report. A complete listing of ADNI investigators can be found at http://adni.loni.usc.edu/wp-content/uploads/how_to_apply/ADNI_Acknowledgement_List.pdf