- 1Department of Neurology, First Affiliated Hospital of China Medical University, Shenyang, China
- 2Key Laboratory of Cell Biology, Ministry of Public Health, Department of Cell Biology, China Medical University, Shenyang, China
- 3Key Laboratory of Medical Cell Biology, Ministry of Education, China Medical University, Shenyang, China
Parkinson’s disease (PD) is widely considered to be a disabling neurodegenerative disorder, which has been ranked second worldwide just after Alzheimer’s disease. Until present, a wide range of studies has focused on the role of circulating inflammatory cytokines in the development of PD. However, the causal relationship between circulating inflammatory cytokines and the risk and age at the onset of PD has not been elucidated. Hence, to evaluate the effects of circulating inflammatory cytokines on the risk or age at the onset of PD more accurately, we conducted this two-sample Mendelian randomization (MR) study involving summary statistics from genome-wide association studies (GWASs). Totally, we included a GWAS for inflammatory cytokines (8,293 participants), a meta-analysis of GWASs for PD risk (482,730 participants), and a GWAS dataset for age at the onset of PD (17,996 patients with PD). A total of 149 and 131 polymorphisms for exploring relationships between 19 inflammatory cytokines and the risk and age at the onset of PD were obtained as instrumental variants. Then, we used a total of five MR methods, including inverse-variance weighted (IVW), Wald ratio, MR Egger regression, weighted median, and MR-pleiotropy residual sum and outlier (MR-PRESSO) methods. Finally, we found a causal association between circulating levels of macrophage inflammatory protein-1 beta (MIP1b) and PD risk in the IVW method (OR: 1.06; 95% CI: 1.02–1.10; P = 0.001). Meanwhile, other MR estimates by weighted median and MR-PRESSO methods yielded similar effect estimates. Besides, we identified a suggestive association of interleukin-16 (IL-16) levels with PD risk (OR: 1.08; 95% CI: 1.00–1.17; P = 0.037). For age at PD onset, there was no evidence supporting its correlation with inflammatory cytokines. Our findings implied that MIP1b and IL-16 may be novel biomarkers and promising therapeutic targets for PD development.
Introduction
Parkinson’s disease (PD) is considered to be an aging-related neurodegenerative disease, characterized by a broad spectrum of clinical features, including tremor, bradykinesia, rigidity, postural instability, and dysautonomia (Jankovic, 2008). During the past decades, the number of individuals suffering from PD increased from 2.5 million in 1990 to 6.1 million in 2016 globally, and it brought great distress and economic burden to society and families (GBD 2016 Parkinson’s Disease Collaborators, 2018). In the United States alone, the overall annual cost related to PD was estimated to be US$51.9 billion in 2017 and was projected to continually increase and eventually surpass US$79 billion by 2037 (Yang et al., 2020). Thus, huge efforts have been made for exploring possible biomarkers and clarifying the pathogenesis for PD development (Schapira and Jenner, 2011; Lotankar et al., 2017).
Presently, an increasing number of evidence supported the vital role of inflammation in the pathogenesis of PD (Marogianni et al., 2020; Kline et al., 2021). It has been reported that systematic inflammation may destroy the permeability of the blood-brain barrier (BBB), activate microglia, and trigger neuroinflammation, which ultimately led to the degeneration of dopaminergic neurons and PD occurrence (Villaran et al., 2010; Joshi and Singh, 2018). Thus, as an indispensable part of the pathophysiological process of inflammation, inflammatory cytokines are suggested to be involved in PD development. Previous observational studies have demonstrated that circulating levels of several inflammatory cytokines were higher in individuals with PD than in healthy controls. For example, Pochmann et al. (2018) indicated that higher levels of monocyte chemotactic protein-1 (MCP-1) were detected in patients with PD than those in the control group. In a meta-analysis involving 2,654 individuals, elevated concentrations of interleukin-6 (IL-6) and IL-1β were observed in patients with PD but not in healthy controls (Qin et al., 2016). Moreover, several genetic polymorphisms near genes encoding MCP-1 and IL-1β were also indicated to be related to an increased risk of PD (Wahner et al., 2007; Wang et al., 2019). Additionally, it has been reported that higher expression of tumor necrosis factor-α (TNF-α) was associated with earlier onset of PD (Lindenau et al., 2017). However, due to the confounding factors, reverse causation, and limited sample size in these observational studies, the relationships between inflammatory cytokines and the risk and age at the onset of PD may be misled.
Mendelian randomization (MR) is considered to be a robust method, which could overcome the limitations of observational studies mentioned earlier by using genetic variants as instrumental variables and large-scale data from genome-wide association studies (GWASs). Hence, in this study, we introduced the MR method to assess the causal relationship between inflammatory cytokines and the risk and age at the onset of PD.
Materials and Methods
Study Design
In Figure 1, the overall design of this two-sample MR analysis is displayed. The study was based on publicly available data from GWASs on circulating inflammatory cytokines and PD, with detailed information listed in Supplementary Table 1. Since we used summary statistics from published studies, no additional ethical approval was required.
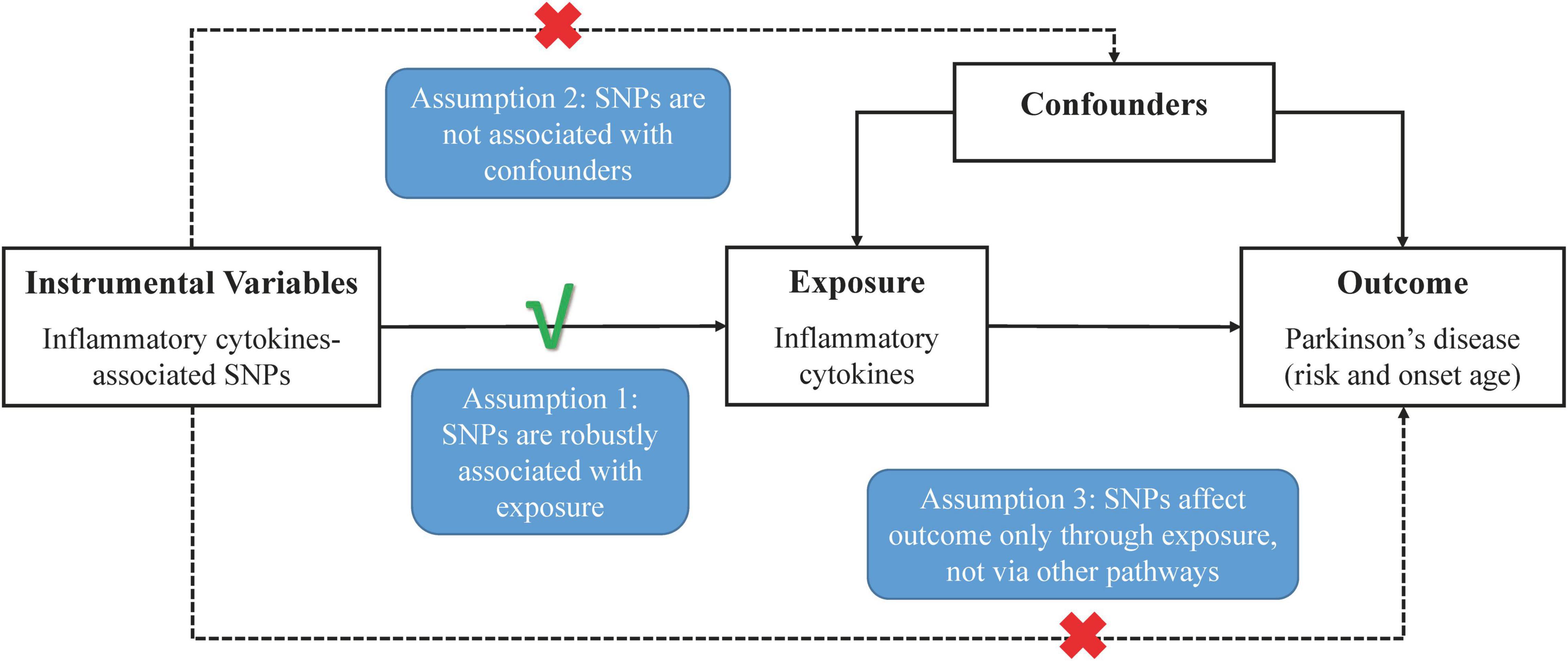
Figure 1. Schematic representation of two-sample Mendelian randomization analyses for circulating levels of inflammatory cytokines and risk of Parkinson’s disease.
Data Sources and Instrument Selection
Concerning PD risk, we included summary data from a GWAS meta-analysis involving IPDGC-NeuroX, UK Biobank, SGPD and IPDGC study with 33,674 cases and 449,056 controls (contributing studies outlined in Supplementary Table 1; Nalls et al., 2019). As for age at the onset of PD, estimates were extracted from a GWAS dataset including 17 cohorts with 17,996 cases with PD (Blauwendraat et al., 2019).
Regarding the instrument variables of inflammatory cytokines, we obtained genetic variants from one recently published GWAS on circulating inflammatory cytokines involving 8,293 European participants (Ahola-Olli et al., 2017). First, we obtained 6,368 single-nucleotide polymorphisms (SNPs) according to a genome-wide significance threshold of P < 5 × 10–8 and the false discovery rate (FDR) less than 5%. Then, for each cytokine, we pruned all selected SNPs in linkage disequilibrium (LD) (r2 < 0.1 in the European 1,000 genomes reference panel), retaining 199 genetic polymorphisms with the lowest P-value as an independent instrument. Next, to avoid pleiotropic effects, 38 SNPs associated with concentrations of more than one cytokine were excluded, leaving 161 polymorphisms focusing on 19 inflammatory cytokines for use. Finally, since 30 SNPs related to the circulating levels of cytokines were not available in the PD risk or age of PD onset datasets, we selected other SNPs in high LD for replacement in further MR analysis (r2 varying between 0.8 and 1). In the end, a total of 149 SNPs and 131 SNPs for detecting relationships between inflammatory cytokines and the risk and age at the onset of PD were included in this study separately. The detailed information of 19 cytokines and their related SNPs used as instrument variables is displayed in Supplementary Table 2.
Statistical Analyses
First, for those cytokines with only one related SNP, the Wald ratio method was used to compute the MR estimates for the association between one-SD elevated circulating cytokine levels and PD risk or age of PD onset, respectively. When over one variant was included, the inverse-variance weighted (IVW) method, as principal analysis, was used to evaluate the potential causal relationships between circulating levels of inflammatory cytokines and the risk and age at the onset of PD. For further assessing the heterogeneity of the causal estimates across different SNPs, Cochran’s Q-test was applied in the IVW method. Subsequently, we evaluated the possibility that the overall MR estimate was driven by a single SNP using leave-one-out analysis (Noyce et al., 2019). Specifically, we reran the MR analysis by using all SNPs and excluding only one outlier SNP at each time. Furthermore, three supplementary analyses, including MR Egger regression, weighted median, and MR-pleiotropy residual sum and outlier (MR-PRESSO) approaches, were performed for investigating the presence of pleiotropy and further evaluating the causal relationships (Bowden et al., 2015, 2016; Verbanck et al., 2018). In addition, we calculated the F-statistics to quantify the strength of the selected instruments, all of which were above the threshold of the F-statistics (F > 10) typically recommended for MR analyses. As 19 exposures were involved, we set the statistically significant P-value threshold to 2.63 × 10–3 (0.05/19) after the Bonferroni correction. The suggestive association was identified as a P-value between the conventional significance level (0.05) and the Bonferroni-corrected significance level. All above statistical analyses were conducted by using the R-statistical software (version 4.0.3) with related R packages, including MR, two-sample MR, as well as MR-PRESSO.
Results
Circulating Inflammatory Cytokines and Parkinson’s Disease Risk
As displayed in Table 1 and Supplementary Figure 1, by using a significant threshold of 2.63 × 10–3 (Bonferroni correction for the correlation test of 19 cytokines), we found that genetically predicted one-SD increment in circulating MIP1b levels was associated with 6% higher risk of PD based on 74 SNPs in the IVW method (OR: 1.06; 95% CI: 1.02–1.10; P = 0.001). Furthermore, we did not observe any significant heterogeneity as measured by Cochran’s Q-test (I2 = 0.5%, P = 0.465). Subsequent leave-one-out analysis showed that no single SNP dominated the IVW point estimate (Supplementary Figure 2). Similarly, a significant association was found between circulating levels of MIP1b and PD risk in the MR-PRESSO method (OR: 1.06; 95% CI: 1.02–1.10; P = 0.002). Consistent with these results, suggestive evidence of an adverse effect of circulating MIP1b levels on PD risk was also observed by the weighted median method (OR: 1.08; 95% CI: 1.02–1.14; P = 0.014). Although the significant statistical association was not detected by MR Egger regression, the estimate was directionally consistent with other principal and supplementary analyses (Table 1 and Figure 2). Additionally, there was no evidence for potential pleiotropy according to the intercept assessed by MR Egger regression; meanwhile, no outlier SNP was detected using the MR-PRESSO method.
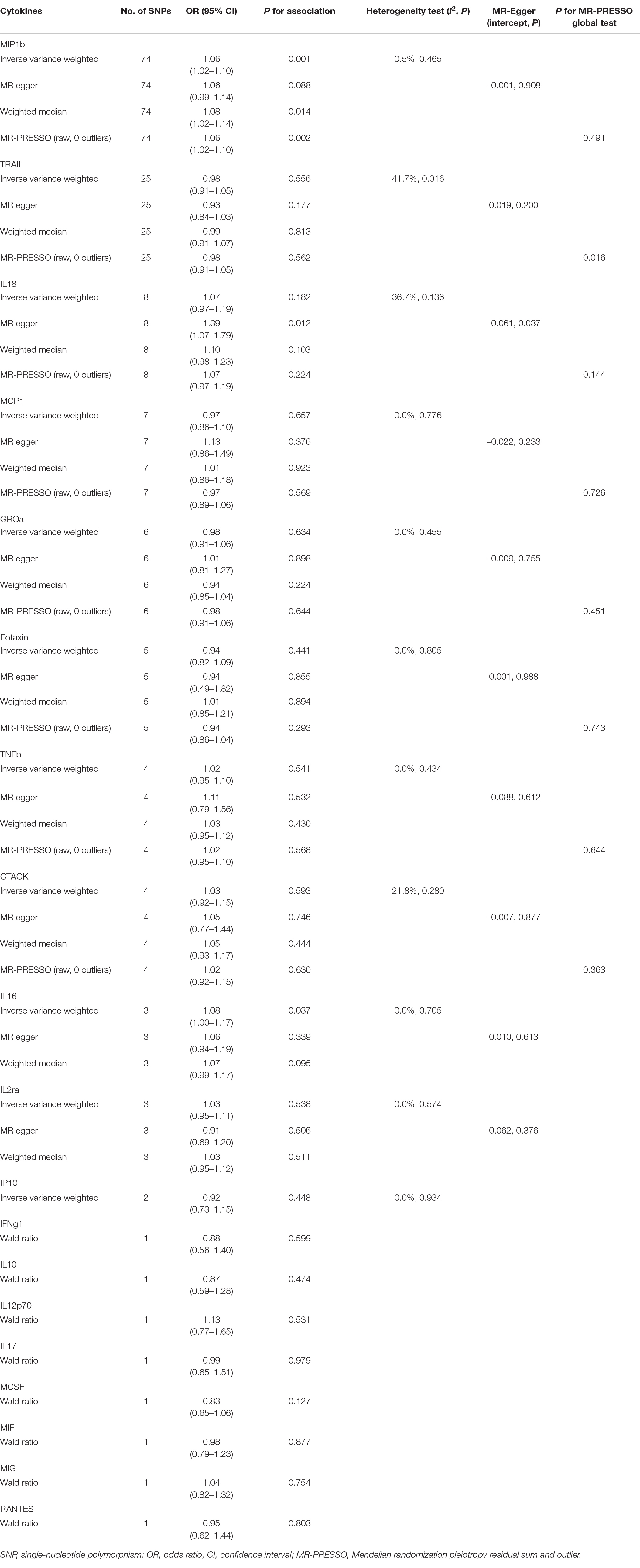
Table 1. MR analyses of genetically predicted levels of circulating inflammatory cytokines and risk of Parkinson’s disease.
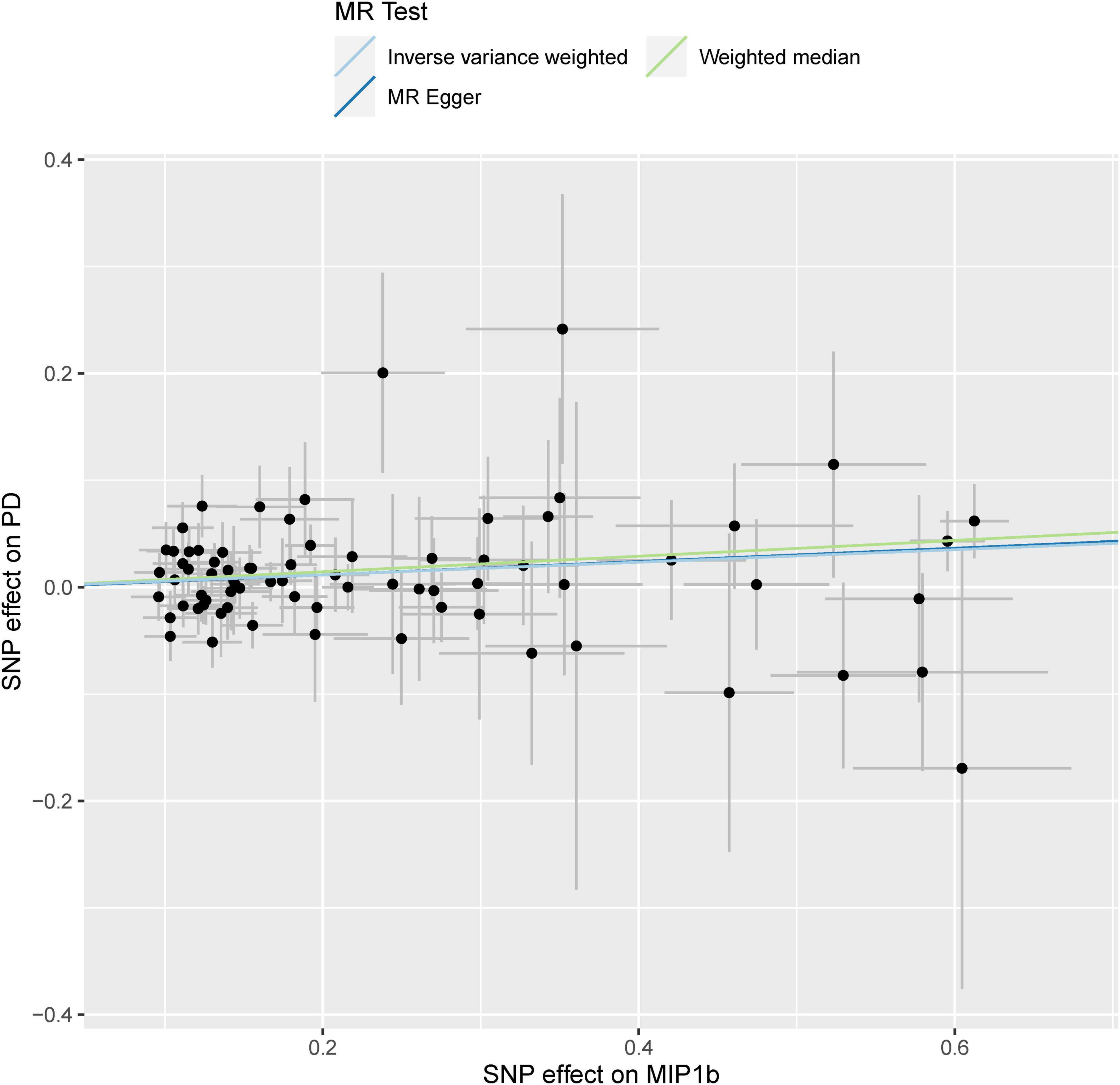
Figure 2. Scatterplot of genetic association with circulating levels of MIP1b against the genetic association with PD risk. Each black dot indicates an SNP, plotted by the estimate of SNP on the MIP1b levels and the estimate of SNP on PD risk with standard error bars. The slope of the line represents the causal relationship, and each method has a different line. PD, Parkinson’s disease; SNP, single-nucleotide polymorphism.
Regarding interleukin-16 (IL-16), we identified a suggestive association between circulating IL-16 levels and PD risk in IVW analysis. Specifically, for one SD increment of IL-16 levels, the OR of PD risk was 1.08 (95% CI: 1.00–1.17; P = 0.037). Further analysis showed a lack of evidence of heterogeneity among SNPs in the suggestive association of IL-16 with PD risk (I2 = 0, P = 0.705). Meanwhile, additional supplementary analysis displayed a similar causal trend with the estimation using the IVW method. In addition, no potential pleiotropy was detected using the MR Egger method (Table 1).
Apart from MIP1b and IL-16, the other 17 cytokines (TRAIL, MCP1, GROa, eotaxin, TNFb, CTACK, IL2ra, IP10, IFNg1, IL10, IL12p70, IL18, IL17, MCSF, MIF, MIG, and RANTES) were not shown to be associated with PD risk in the main IVW analysis and three supplementary analyses (Table 1). For each cytokine, no marked heterogeneity was found between related SNPs, except for TRAIL (I2 = 41.7%, P = 0.016). Meanwhile, the P-values for the intercepts from Egger regression did not demonstrate any pleiotropy, while IL-18 was the exception (P = 0.037). Further, the MR-PRESSO test showed that no outlier was found for all cytokines, except for TRAIL (P = 0.016).
Circulating Inflammatory Cytokines and Age at the Onset of Parkinson’s Disease
Except for identifying the correlation between inflammatory cytokines and PD risk mentioned earlier, we also detected the causal relationships between the circulating levels of these cytokines and the age of PD onset. Unfortunately, there was no evidence to support the causal relationships between these 19 cytokines and age at the onset of PD (Table 2). Moreover, no statistical evidence of instrument heterogeneity was detected using Cochran’s Q-test except for IP10 (I2 = 79.1%, P = 0.029). When applying MR Egger regression, evidence of potential pleiotropy was only observed in GROa (P = 0.029), while other inflammatory cytokines did not show any pleiotropy. Besides, no SNP was detected as an outlier through the MR-PRESSO test (Table 2).
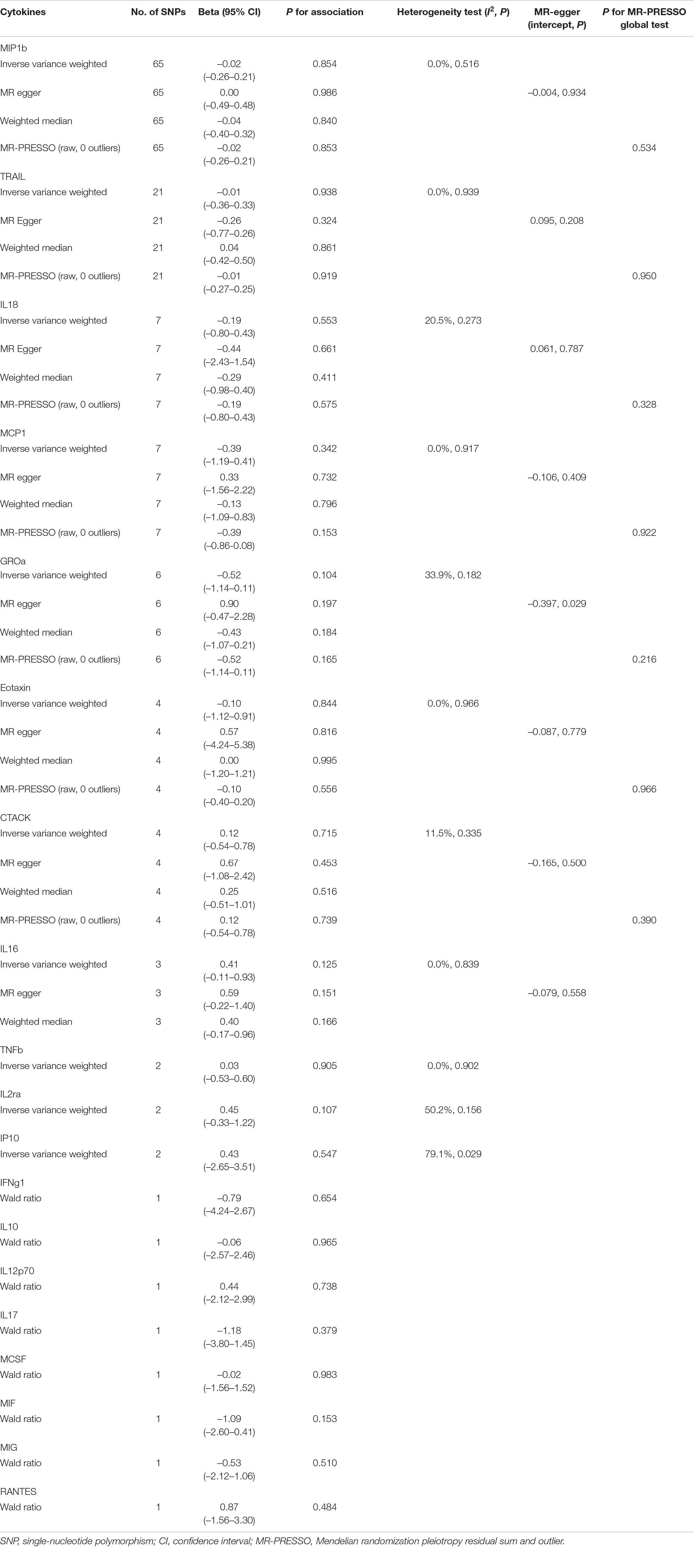
Table 2. MR analysis of genetically predicted levels of circulating inflammatory cytokines and age at onset of Parkinson’s disease.
Discussion
Parkinson’s disease is a progressive and disabling neurodegenerative disease that mainly affects individuals in their later years of life and its course may vary from 6.9 to 14.3 years (Macleod et al., 2014; Marras et al., 2018). It has been reported that in 2016 alone, PD caused 211,296 deaths and 3.2 million disability-adjusted life-years globally (GBD 2016 Parkinson’s Disease Collaborators, 2018). Despite the long course of the disease and its huge impact on life expectance for elders, the intervention for PD prevention and therapy is still deficient, and the biological mechanism underlying PD etiology is not yet well understood. Thus, we took advantage of a two-sample MR analysis to investigate the causal role of circulating inflammatory cytokines in the risk and age at the onset of PD. In this study, higher MIP1b levels showed a causal relationship with the increased risk of PD, whereas another cytokine, IL-16, displayed a suggestive association.
Macrophage inflammatory protein-1 beta, also known as CCL4, is a chemotactic protein with 69 amino acids produced by various cells, including natural killer cells, T cells, B cells, and neutrophils (Menten et al., 2002; Mukaida et al., 2020). A previous study by Zhu et al. (2014) demonstrated that in the transgenic mice model of Alzheimer’s disease, MIP1b mRNA was 18 times higher than that of wild-type mice, indicating that MIP1b might be involved in the development of Alzheimer’s disease. In addition, MIP1b was also reported to be related to other neurodegenerative diseases, including multiple system atrophy and amyotrophic lateral sclerosis (Compta et al., 2019; Martinez et al., 2020). This evidence implied that MIP1b might participate in the initiation and progression of a neurodegenerative disorder. As for PD, in the past few decades, limited observational studies provided evidence of a possible association between MIP1b and PD risk. According to a previous case-control study involving 50 participants by Calvani et al. (2020), circulating levels of MIP1b were 2-fold higher in patients with PD than in controls, suggesting that it could be considered a possible biomarker for PD occurrence. Additionally, according to Brockmann’s study of 142 patients with PD, higher serum levels of MIP1b may be associated with more severe non-motor symptoms of PD, including cognitive impairment, sleep behavior disorder, and orthostatic dysfunction (Brockmann et al., 2017). Although these studies indicated an association between circulating levels of MIP1b and the occurrence and progression of PD, these results from observational studies were ambiguous and questionable. The reasons were as follows: due to the small sample size and potential confounding, the results of observational studies may be biased. Besides, the relationship between circulating levels of MIP1b and PD risk obtained from observational studies could not be determined as a causal association or even may even be a reverse causality. Therefore, we conducted this MR analysis and reached a reliable result that one-SD increment in the circulating levels of MIP1b may increase the risk of PD by 6%, indicating that lowering the levels of MIP1b may be a promising therapeutic strategy for PD.
From the perspective of biological mechanism, MIP1b may participate in the pathophysiology process of PD through the following pathways. On the one hand, MIP1b could induce the activation of macrophages by binding to its specific receptor, CCR5 (Cheung et al., 2009; Saika et al., 2012). Then, the activated macrophage might release high levels of pro-inflammatory cytokines, such as IL-1β, TNF-α, IL-6, and MIP-1α (Saika et al., 2012; Shapouri-Moghaddam et al., 2018). These cytokines could further disrupt the permeability of brain microvascular endothelial cells and the integrity of BBB (Al-Obaidi and Desa, 2018; Morris et al., 2018). On the other hand, MIP1b not only can immobilize glycosaminoglycans on the apical surface of microvascular endothelial cells but can also bind to the basal surfaces of endothelial cells as well as the subendothelial matrix. Both of the connections contributed to the establishment and maintenance of MIP1b gradients to help various circulating inflammatory cells pass through the BBB and enter the central nervous system (CNS) (Shukaliak and Dorovini-Zis, 2000). Subsequently, in response to these inflammatory mediators, microglia were activated and functionally polarized toward the pro-inflammatory M1 phenotype, which further induced the secretion of inflammatory cytokines, such as TNF-α, MCP-1, and IL-1β (Qin et al., 2007; Jo et al., 2017; Pajares et al., 2020). These chronically elevated inflammatory cytokines and activated inflammatory cells may, in turn, lead to chronic self-perpetuating neuroinflammation and eventually end up in the development of PD (Collins et al., 2012).
Regarding IL-16, it is a protein with 631 amino acids and is generally accepted to be a chemotactic cytokine for T cells (Cruikshank et al., 2000; Skundric et al., 2015). In our study, we found a suggestive association that one-SD increment in the circulating levels of IL-16 was associated with an 8% increase in PD risk. This could be explained by the following reasons: Similar to MIP1b, elevated IL-16 levels might also promote macrophages to release higher levels of pro-inflammatory cytokines and contribute to BBB breakdown (Persidsky et al., 2006; Huang et al., 2019). Besides, IL-16 could induce the activation of protein kinase C (PKC) and its translocation from cytosol to the membrane (Parada et al., 1996). Later, the PKC might phosphorylate the tight junction proteins of BBB leading to BBB damage (Li et al., 2018). Moreover, IL-16 could potentiate the inflammatory response by stimulating T cells to produce more pro-inflammatory cytokines (Skundric et al., 2015). Altogether, the production of pro-inflammatory cytokines, activation of immune cells, and continuous chronic inflammatory response could lead to PD occurrence.
There were several advantages involved in our study. This was the first MR analysis for clarifying the causality of inflammatory cytokines levels with the risk and age at the onset of PD. Another merit was the two-sample MR method that provided robust causal associations by minimizing confounding factors and avoiding reverse causality. Besides, we used data from publicly available GWASs, which included a large number of patients with PD and controls, thus affording a great power to explore the causal relationship. Finally, except for the IVW method, we also used three supplementary analyses, including MR Egger regression, weighted median, and MR-PRESSO approaches. Directional consistency of the results across multiple MR approaches reinforced the reliability of associations. Nevertheless, the results of this MR analysis should be noted in the context of the following limitations. First, since potential pleiotropy, outlier, and heterogeneity can be detected, the relationship between inflammatory cytokines, including TRAIL, IP10, IL-18, and GROa, and the risk or age at the onset of PD should be interpreted with caution. Second, we used summary data from GWASs of mostly European adults, which limited the generality of the observed causal associations to other populations with different genetic backgrounds. Third, cytokines are not something constitutively expressed in the body. During inflammation, specific inflammatory cytokines are induced to a high level but for only a short period. All of those dynamic changes cannot be addressed by MR analysis. Fourth, we focused on the causal effect of peripheral cytokines levels on the risk or age at the onset of PD and supported that systematic peripheral inflammation and chronic cytokine disturbance may contribute to the development of PD. However, a recent study by Wijeyekoon et al. (2020) suggested that some cytokines in the CSF did not correlate well with blood. Thus, our analysis cannot reveal the potential causal relationship between the levels of inflammatory cytokines in CSF and the risk or age at the onset of PD. Last but not the least, not all inflammatory cytokines were analyzed in our MR analysis due to the exclusion criteria and the limited number of cytokines analyzed in the previous GWAS.
Conclusion
In conclusion, this MR analysis showed a causal relationship between circulating levels of MIP1b and PD risk and a suggestive association between IL-16 and PD. Additionally, there was no evidence in support of causality between inflammatory cytokines and age at the onset of PD. Our findings brought new insights into the pathogenesis of PD and indicated that MIP1b and IL-16 could be regarded as novel biomarkers and potential therapeutic targets for PD development.
Data Availability Statement
The original contributions presented in the study are included in the article/Supplementary Material, further inquiries can be directed to the corresponding author/s.
Author Contributions
XL designed the research. YZ and XZ had full access to all the data in the study and took responsibility for the integrity of the data and the accuracy of the data analysis. YZ and NG wrote the manuscript and performed the data analysis. All authors contributed to the statistical analysis, critically reviewed the manuscript during the writing process, and approved the final version to be published.
Funding
This work was supported by the National Natural Science Foundation of China (grant nos. 81400950, 81801053, and 81872044) and the Natural Science Foundation of Liaoning Province (grant no. 2019-MS-365).
Conflict of Interest
The authors declare that the research was conducted in the absence of any commercial or financial relationships that could be construed as a potential conflict of interest.
Publisher’s Note
All claims expressed in this article are solely those of the authors and do not necessarily represent those of their affiliated organizations, or those of the publisher, the editors and the reviewers. Any product that may be evaluated in this article, or claim that may be made by its manufacturer, is not guaranteed or endorsed by the publisher.
Supplementary Material
The Supplementary Material for this article can be found online at: https://www.frontiersin.org/articles/10.3389/fnagi.2022.811059/full#supplementary-material
Supplementary Figure 1 | Forest plot of the causal effect estimates of MIP1b-associated SNPs on PD risk. Each black dot indicates the causal estimate of MIP1b on PD using an SNP by the Wald ratio method with 95% confidence interval bars. The MR estimate using all SNPs with the IVW method is also shown (red dot with 95% confidence interval bars). PD, Parkinson’s disease; SNP, single-nucleotide polymorphism; MR, Mendelian randomization; IVW, inverse-variance weighted.
Supplementary Figure 2 | Leave-one-out analysis of MIP1b-associated SNPs on PD risk. It was conducted to determine whether the causal effect of circulating MIP1b levels on PD risk was disproportionately influenced by a single SNP. The black dot and bars indicate the MR estimate and 95% confidence interval when the specific SNP is removed. The overall MR analysis, including all SNPs, is also presented for comparison (red dot with 95% confidence interval bars). SNP, single-nucleotide polymorphism; PD, Parkinson’s disease; MR, Mendelian randomization.
References
Ahola-Olli, A. V., Wurtz, P., Havulinna, A. S., Aalto, K., Pitkanen, N., Lehtimaki, T., et al. (2017). Genome-wide association study identifies 27 loci influencing concentrations of circulating cytokines and growth factors. Am. J. Hum. Genet. 100, 40–50. doi: 10.1016/j.ajhg.2016.11.007
Al-Obaidi, M. M. J., and Desa, M. N. M. (2018). Mechanisms of blood brain barrier disruption by different types of bacteria, and bacterial-host interactions facilitate the bacterial pathogen invading the brain. Cell Mol. Neurobiol. 38, 1349–1368. doi: 10.1007/s10571-018-0609-2
Blauwendraat, C., Heilbron, K., Vallerga, C. L., Bandres-Ciga, S., von Coelln, R., Pihlstrom, L., et al. (2019). Parkinson’s disease age at onset genome-wide association study: defining heritability, genetic loci, and alpha-synuclein mechanisms. Mov. Disord. 34, 866–875. doi: 10.1002/mds.27659
Bowden, J., Davey Smith, G., and Burgess, S. (2015). Mendelian randomization with invalid instruments: effect estimation and bias detection through egger regression. Int. J. Epidemiol. 44, 512–525. doi: 10.1093/ije/dyv080
Bowden, J., Davey Smith, G., Haycock, P. C., and Burgess, S. (2016). Consistent estimation in mendelian randomization with some invalid instruments using a weighted median estimator. Genet. Epidemiol. 40, 304–314. doi: 10.1002/gepi.21965
Brockmann, K., Schulte, C., Schneiderhan-Marra, N., Apel, A., Pont-Sunyer, C., Vilas, D., et al. (2017). Inflammatory profile discriminates clinical subtypes in LRRK2-associated Parkinson’s disease. Eur. J. Neurol. 24, 427–e426. doi: 10.1111/ene.13223
Calvani, R., Picca, A., Landi, G., Marini, F., Biancolillo, A., Coelho-Junior, H. J., et al. (2020). A novel multi-marker discovery approach identifies new serum biomarkers for Parkinson’s disease in older people: an EXosomes in PArkiNson Disease (EXPAND) ancillary study. Geroscience 42, 1323–1334. doi: 10.1007/s11357-020-00192-2
Cheung, R., Malik, M., Ravyn, V., Tomkowicz, B., Ptasznik, A., and Collman, R. G. (2009). An arrestin-dependent multi-kinase signaling complex mediates MIP-1beta/CCL4 signaling and chemotaxis of primary human macrophages. J. Leukoc. Biol. 86, 833–845. doi: 10.1189/jlb.0908551
Collins, L. M., Toulouse, A., Connor, T. J., and Nolan, Y. M. (2012). Contributions of central and systemic inflammation to the pathophysiology of Parkinson’s disease. Neuropharmacology 62, 2154–2168. doi: 10.1016/j.neuropharm.2012.01.028
Compta, Y., Dias, S. P., Giraldo, D. M., Perez-Soriano, A., Munoz, E., Saura, J., et al. (2019). Cerebrospinal fluid cytokines in multiple system atrophy: a cross-sectional Catalan MSA registry study. Parkinsonism. Relat. Disord. 65, 3–12. doi: 10.1016/j.parkreldis.2019.05.040
Cruikshank, W. W., Kornfeld, H., and Center, D. M. (2000). Interleukin-16. J. Leukoc. Biol. 67, 757–766.
GBD 2016 Parkinson’s Disease Collaborators (2018). Global, regional, and national burden of Parkinson’s disease, 1990-2016: a systematic analysis for the Global Burden of Disease Study 2016. Lancet Neurol. 17, 939–953. doi: 10.1016/S1474-4422(18)30295-3
Huang, Y., Du, K. L., Guo, P. Y., Zhao, R. M., Wang, B., Zhao, X. L., et al. (2019). IL-16 regulates macrophage polarization as a target gene of mir-145-3p. Mol. Immunol. 107, 1–9. doi: 10.1016/j.molimm.2018.12.027
Jankovic, J. (2008). Parkinson’s disease: clinical features and diagnosis. J. Neurol. Neurosurg. Psychiatry 79, 368–376.
Jo, M., Kim, J. H., Song, G. J., Seo, M., Hwang, E. M., and Suk, K. (2017). Astrocytic Orosomucoid-2 modulates microglial activation and neuroinflammation. J. Neurosci. 37, 2878–2894. doi: 10.1523/JNEUROSCI.2534-16.2017
Joshi, N., and Singh, S. (2018). Updates on immunity and inflammation in Parkinson disease pathology. J. Neurosci. Res. 96, 379–390. doi: 10.1002/jnr.24185
Kline, E. M., Houser, M. C., Herrick, M. K., Seibler, P., Klein, C., West, A., et al. (2021). Genetic and environmental factors in Parkinson’s disease converge on immune function and inflammation. Mov. Disord. 36, 25–36. doi: 10.1002/mds.28411
Li, H., Zhu, L., Feng, J., Hu, X., Li, C., and Zhang, B. (2018). Hydrogen sulfide decreases blood-brain barrier damage via regulating protein Kinase C and tight junction after cardiac arrest in rats. Cell Physiol. Biochem. 47, 994–1006. doi: 10.1159/000490166
Lindenau, J. D., Altmann, V., Schumacher-Schuh, A. F., Rieder, C. R., and Hutz, M. H. (2017). Tumor necrosis factor alpha polymorphisms are associated with Parkinson’s disease age at onset. Neurosci. Lett. 658, 133–136. doi: 10.1016/j.neulet.2017.08.049
Lotankar, S., Prabhavalkar, K. S., and Bhatt, L. K. (2017). Biomarkers for Parkinson’s disease: recent advancement. Neurosci. Bull. 33, 585–597. doi: 10.1007/s12264-017-0183-5
Macleod, A. D., Taylor, K. S., and Counsell, C. E. (2014). Mortality in Parkinson’s disease: a systematic review and meta-analysis. Mov. Disord. 29, 1615–1622.
Marogianni, C., Sokratous, M., Dardiotis, E., Hadjigeorgiou, G. M., Bogdanos, D., and Xiromerisiou, G. (2020). Neurodegeneration and inflammation-an interesting interplay in Parkinson’s disease. Int. J. Mol. Sci. 21:8421. doi: 10.3390/ijms21228421
Marras, C., Beck, J. C., Bower, J. H., Roberts, E., Ritz, B., Ross, G. W., et al. (2018). Prevalence of Parkinson’s disease across North America. NPJ Parkinsons Dis. 4:21. doi: 10.1038/s41531-018-0058-0
Martinez, H. R., Escamilla-Ocanas, C. E., Camara-Lemarroy, C. R., Gonzalez-Garza, M. T., Moreno-Cuevas, J., and Garcia Sarreon, M. A. (2020). Increased cerebrospinal fluid levels of cytokines monocyte chemoattractant protein-1 (MCP-1) and macrophage inflammatory protein-1beta (MIP-1beta) in patients with amyotrophic lateral sclerosis. Neurologia 35, 165–169. doi: 10.1016/j.nrl.2017.07.020
Menten, P., Wuyts, A., and Van Damme, J. (2002). Macrophage inflammatory protein-1. Cytokine Growth Factor Rev. 13, 455–481.
Morris, G., Fernandes, B. S., Puri, B. K., Walker, A. J., Carvalho, A. F., and Berk, M. (2018). Leaky brain in neurological and psychiatric disorders: drivers and consequences. Aust. N. Z. J. Psychiatry 52, 924–948. doi: 10.1177/0004867418796955
Mukaida, N., Sasaki, S. I., and Baba, T. (2020). CCL4 Signaling in the tumor microenvironment. Adv. Exp. Med. Biol. 1231, 23–32. doi: 10.1007/978-3-030-36667-4_3
Nalls, M. A., Blauwendraat, C., Vallerga, C. L., Heilbron, K., Bandres-Ciga, S., Chang, D., et al. (2019). Identification of novel risk loci, causal insights, and heritable risk for Parkinson’s disease: a meta-analysis of genome-wide association studies. Lancet Neurol. 18, 1091–1102. doi: 10.1016/S1474-4422(19)30320-5
Noyce, A. J., Bandres-Ciga, S., Kim, J., Heilbron, K., Kia, D., Hemani, G., et al. (2019). The Parkinson’s disease mendelian randomization research portal. Mov. Disord. 34, 1864–1872. doi: 10.1002/mds.27873
Pajares, M., Rojo, A. I., Manda, G., Bosca, L., and Cuadrado, A. (2020). Inflammation in Parkinson’s disease: mechanisms and therapeutic implications. Cells 9:1687. doi: 10.3390/cells9071687
Parada, N. A., Cruikshank, W. W., Danis, H. L., Ryan, T. C., and Center, D. M. (1996). IL-16- and other CD4 ligand-induced migration is dependent upon protein kinase C. Cell Immunol. 168, 100–106. doi: 10.1006/cimm.1996.0054
Persidsky, Y., Ramirez, S. H., Haorah, J., and Kanmogne, G. D. (2006). Blood-brain barrier: structural components and function under physiologic and pathologic conditions. J. Neuroimmune Pharmacol. 1, 223–236. doi: 10.1007/s11481-006-9025-3
Pochmann, D., Peccin, P. K., da Silva, I. R. V., Dorneles, G. P., Peres, A., Nique, S., et al. (2018). Cytokine modulation in response to acute and chronic aquatic therapy intervention in Parkinson disease individuals: a pilot study. Neurosci. Lett. 674, 30–35. doi: 10.1016/j.neulet.2018.03.021
Qin, L., Wu, X., Block, M. L., Liu, Y., Breese, G. R., Hong, J. S., et al. (2007). Systemic LPS causes chronic neuroinflammation and progressive neurodegeneration. Glia 55, 453–462. doi: 10.1002/glia.20467
Qin, X. Y., Zhang, S. P., Cao, C., Loh, Y. P., and Cheng, Y. (2016). Aberrations in peripheral inflammatory cytokine levels in parkinson disease: a systematic review and meta-analysis. JAMA Neurol. 73, 1316–1324. doi: 10.1001/jamaneurol.2016.2742
Saika, F., Kiguchi, N., Kobayashi, Y., Fukazawa, Y., and Kishioka, S. (2012). CC-chemokine ligand 4/macrophage inflammatory protein-1beta participates in the induction of neuropathic pain after peripheral nerve injury. Eur. J. Pain 16, 1271–1280. doi: 10.1002/j.1532-2149.2012.00146.x
Schapira, A. H., and Jenner, P. (2011). Etiology and pathogenesis of Parkinson’s disease. Mov. Disord. 26, 1049–1055.
Shapouri-Moghaddam, A., Mohammadian, S., Vazini, H., Taghadosi, M., Esmaeili, S. A., Mardani, F., et al. (2018). Macrophage plasticity, polarization, and function in health and disease. J. Cell. Physiol. 233, 6425–6440. doi: 10.1002/jcp.26429
Shukaliak, J. A., and Dorovini-Zis, K. (2000). Expression of the beta-chemokines RANTES and MIP-1 beta by human brain microvessel endothelial cells in primary culture. J. Neuropathol. Exp. Neurol. 59, 339–352. doi: 10.1093/jnen/59.5.339
Skundric, D. S., Cruikshank, W. W., Montgomery, P. C., Lisak, R. P., and Tse, H. Y. (2015). Emerging role of IL-16 in cytokine-mediated regulation of multiple sclerosis. Cytokine 75, 234–248. doi: 10.1016/j.cyto.2015.01.005
Verbanck, M., Chen, C. Y., Neale, B., and Do, R. (2018). Publisher Correction: Detection of widespread horizontal pleiotropy in causal relationships inferred from Mendelian randomization between complex traits and diseases. Nat. Genet. 50:1196. doi: 10.1038/s41588-018-0164-2
Villaran, R. F., Espinosa-Oliva, A. M., Sarmiento, M., De Pablos, R. M., Arguelles, S., Delgado-Cortes, M. J., et al. (2010). Ulcerative colitis exacerbates lipopolysaccharide-induced damage to the nigral dopaminergic system: potential risk factor in Parkinson‘s disease. J. Neurochem. 114, 1687–1700. doi: 10.1111/j.1471-4159.2010.06879.x
Wahner, A. D., Sinsheimer, J. S., Bronstein, J. M., and Ritz, B. (2007). Inflammatory cytokine gene polymorphisms and increased risk of Parkinson disease. Arch. Neurol. 64, 836–840. doi: 10.1001/archneur.64.6.836
Wang, Y., Zhou, M., Wang, Y., Jiang, D., and Deng, X. (2019). Association of polymorphisms in the MCP-1 and CCR2 genes with the risk of Parkinson’s disease. J. Neural. Transm. 126, 1465–1470. doi: 10.1007/s00702-019-02072-2
Wijeyekoon, R. S., Moore, S. F., Farrell, K., Breen, D. P., Barker, R. A., and Williams-Gray, C. H. (2020). Cerebrospinal fluid cytokines and neurodegeneration-associated proteins in Parkinson’s disease. Mov. Disord. 35, 1062–1066. doi: 10.1002/mds.28015
Yang, W., Hamilton, J. L., Kopil, C., Beck, J. C., Tanner, C. M., Albin, R. L., et al. (2020). Current and projected future economic burden of Parkinson’s disease in the U.S. NPJ Parkinsons Dis. 6:15. doi: 10.1038/s41531-020-0117-1
Keywords: cytokines, inflammation, Mendelian randomization, macrophage inflammatory protein-1 beta, interleukin-16, Parkinson’s disease
Citation: Zhao Y, Zhang X, Guo N, Tian D, Zhang C, Mu C, Han C, Zhu R, Zhang J and Liu X (2022) Genetically Predicted Levels of Circulating Inflammatory Cytokines and the Risk and Age at Onset of Parkinson’s Disease: A Two-Sample Mendelian Randomization Study. Front. Aging Neurosci. 14:811059. doi: 10.3389/fnagi.2022.811059
Received: 08 November 2021; Accepted: 31 January 2022;
Published: 01 March 2022.
Edited by:
Robert Petersen, Central Michigan University, United StatesReviewed by:
Pingyi Xu, First Affiliated Hospital of Guangzhou Medical University, ChinaZhaoqi Yan, Gladstone Institutes, United States
Copyright © 2022 Zhao, Zhang, Guo, Tian, Zhang, Mu, Han, Zhu, Zhang and Liu. This is an open-access article distributed under the terms of the Creative Commons Attribution License (CC BY). The use, distribution or reproduction in other forums is permitted, provided the original author(s) and the copyright owner(s) are credited and that the original publication in this journal is cited, in accordance with accepted academic practice. No use, distribution or reproduction is permitted which does not comply with these terms.
*Correspondence: Xu Liu, dmFsZW50aW5lMTEyMEAxMjYuY29t
†These authors have contributed equally to this work and share first authorship