- 1Department of Physiology, Faculty of Basic Medical Sciences, College of Health Sciences, Usmanu Danfodiyo University, Sokoto, Nigeria
- 2Centre for Advanced Medical Research and Training, Usmanu Danfodiyo University, Sokoto, Nigeria
- 3Department of Physiology, Faculty of Medicine, Universiti Kebangsaan Malaysia Medical Centre, Kuala Lumpur, Malaysia
- 4Department of Basic Medical Science, Kulliyyah of Medicine, International Islamic University Malaysia, Kuantan, Malaysia
- 5Department of Clinical Pharmacology, Faculty of Medicine, Menoufia University, Shebin El-Kom, Egypt
- 6Neurology Unit, Department of Medicine, Faculty of Medicine, Universiti Kebangsaan Malaysia Medical Centre, Kuala Lumpur, Malaysia
Alzheimer’s disease (AD) is an irreversible brain disorder associated with slow, progressive loss of brain functions mostly in older people. The disease processes start years before the symptoms are manifested at which point most therapies may not be as effective. In the hippocampus, the key proteins involved in the JAK2/STAT3 signaling pathway, such as p-JAK2-Tyr1007 and p-STAT3-Tyr705 were found to be elevated in various models of AD. In addition to neurons, glial cells such as astrocytes also play a crucial role in the progression of AD. Without having a significant effect on tau and amyloid pathologies, the JAK2/STAT3 pathway in reactive astrocytes exhibits a behavioral impact in the experimental models of AD. Cholinergic atrophy in AD has been traced to a trophic failure in the NGF metabolic pathway, which is essential for the survival and maintenance of basal forebrain cholinergic neurons (BFCN). In AD, there is an alteration in the conversion of the proNGF to mature NGF (mNGF), in addition to an increase in degradation of the biologically active mNGF. Thus, the application of exogenous mNGF in experimental studies was shown to improve the recovery of atrophic BFCN. Furthermore, it is now coming to light that the FGF7/FGFR2/PI3K/Akt signaling pathway mediated by microRNA-107 is also involved in AD pathogenesis. Vascular dysfunction has long been associated with cognitive decline and increased risk of AD. Vascular risk factors are associated with higher tau and cerebral beta-amyloid (Aβ) burden, while synergistically acting with Aβ to induce cognitive decline. The apolipoprotein E4 polymorphism is not just one of the vascular risk factors, but also the most prevalent genetic risk factor of AD. More recently, the research focus on AD shifted toward metabolisms of various neurotransmitters, major and minor nutrients, thus giving rise to metabolomics, the most important “omics” tool for the diagnosis and prognosis of neurodegenerative diseases based on an individual’s metabolome. This review will therefore proffer a better understanding of novel signaling pathways associated with neural and glial mechanisms involved in AD, elaborate potential links between vascular dysfunction and AD, and recent developments in “omics”-based biomarkers in AD.
Introduction
Alzheimer’s disease (AD) is a progressive brain disease that is attributed by the Alzheimer Association as the cause of 60–80% of dementia cases. Depending on the stage of the disease, it is characterized by factors that progress to hinder the performance of everyday activities, such as apathy, depression, impaired communication, disorientation, poor judgment, difficulty in swallowing and walking, and behavioral changes (Alzheimer’s Association, 2021). The duration takes to develop a continuum of these symptoms is determined by factors such as age, genetics, and sex (Vermunt et al., 2019). Current statistics show that over six million Americans aged 65 and above are living with AD, with a projection of about 13.8 million by 2060, and death cases have increased by 16% during the COVID-19 pandemic (Alzheimer’s Association, 2021). Aside from informal caregiving, the total cost of care payment for AD patients and related diseases was estimated at $355 billion in 2021.
The progressive cognitive decline in AD is associated with the accumulation of amyloid-beta (Aβ) and tau proteins (Selkoe and Hardy, 2016). Aβ is derived from the sequential cleavage of amyloid precursor protein (APP) by beta-secretase and gamma-secretase. The aggregation of Aβ thus forms oligomers that are toxic to the neurons (Haass and Selkoe, 2007). Tau, on the other hand, is derived from alternative splicing from the microtubule-associated protein tau (MAPT) gene to form soluble protein isoforms (Goedert et al., 1989). Several functional interactions have been revealed between Aβ and tau in the neural circuit damage and cognitive decline in AD (Tripathi and Kalita, 2019; Busche and Hyman, 2020). This is in a bid to encourage a broad approach in the design of a potential therapy.
Unfortunately, no treatment option is available to cure the disease to date (Husna Ibrahim et al., 2020). Recent approaches in the treatment of AD include exploring the potentials of some natural products with neuroprotective effects (Kamil et al., 2018, 2020; Prom-In et al., 2020; Kamal et al., 2021) and metabolites to modulate signaling pathways associated with neurovascular endothelium through multi-omic analyses (Corral-Jara et al., 2021). There have also been reports on cellular signaling-related sex-dependent effects under hyperglycemic and lipid stress (Nuthikattu et al., 2020, 2021). However, this review focuses on significant signaling pathways associated with the stages of AD, the potential links between vascular dysfunction and AD, as well as recent developments in “omics”-based approaches in AD. Without publication date restriction until May 2021, PubMed, ScienceDirect, and Google Scholar databases were searched for published articles containing the search terms related to “Alzheimer,” “dementia,” “signaling pathways,” “vascular dysfunction,” “cognitive impairment,” and “omics.” Relevant articles retrieved were thereafter included for synthesis.
Signaling Pathways Associated With Alzheimer’s Disease
From several studies, a group of researchers was able to develop a database containing a compilation of signaling pathways associated with AD, called AlzPathway1 (Mizuno et al., 2012). There are several components of the AlzPathway, which include the Aβ cleavage and degradation, apolipoprotein E (ApoE)-cholesterol pathway and NFT accumulation, acetylcholine production, Wnt signaling pathway, Ubiquitin mediated proteolysis, apoptosis, calcium signaling pathway, Notch signaling pathway, MAPK signaling pathway, abnormal ceramide accumulation, reactive oxidation process, neurotrophin signaling pathway, cell cycle, mTOR signaling pathway, lipid pathway, insulin pathway, and inflammation pathway (Mizuno et al., 2012). Here, we discuss newly emerging pathways as potential diagnostic and therapeutic targets.
In the 2021 Alzheimer Drug Development Pipeline, agents that are currently in clinical trials were divided into 3 categories; agents that are disease-modifying biologic, disease-modifying small molecule, and symptom-reducing small molecule (Cummings et al., 2021). Twenty-nine percentage of the disease-modifying therapies (DMTs) that were successfully shifted to the 3rd phase trials are targeting the amyloid. Meanwhile, out of 50 drugs that were repurposed for AD treatments, 10 of the agents proceed to Phase 3 clinical trials. AD drug development has been very challenging due to its heterogeneity in AD pathogenesis, with a 0.4% success rate as memantine is the only drug that has significant effects and was approved by FDA out of 244 drugs investigated in AD clinical trials from the year 2002 to 2012 (Fish et al., 2019).
As microglia activation also plays a role in the pathogenesis of AD, minocycline, an anti-inflammatory tetracycline drug was investigated in AD clinical trials due to its ability to penetrate the blood-brain barrier (BBB) while hindering the proinflammatory microglia. Besides, minocycline attenuates the fibrillization of Aβ, hence, halting the deposition of Aβ plagues and neuronal death in vitro (Romero-Miguel et al., 2021). However, 24 months of 400 mg minocycline treatment in a randomized clinical trial failed to delay the cognitive impairments in mild AD patients (Howard et al., 2020). This finding is parallel to the previous trials on non-steroidal anti-inflammatory drugs (NSAIDs) which failed to exert positive effects on delaying AD developments. Thus, it can be deduced that maybe although neuroinflammation is one of many pathways involved in the heterogenic pathogenesis of AD, targeting neuroinflammation alone may not be enough to exert significant neuroprotective effects in AD.
Vitamin E is an antioxidant consisting of α-, β-, γ-, and δ-tocophenols and tocotrienols, that can neutralize free radicals and ROS which eventually protecting the cellular membrane from the destructive oxidative stress (Lloret et al., 2019). α-tocopherol is a critical antioxidant in the brain due to the abundance α-tocopherol transfer protein (α-TTP) transporter for the regulation of vitamin E distribution in other tissues. Besides, vitamin E is also involved in the protein kinase C (PKC) pathway and exerts anti-inflammatory effects. The first clinical trial of vitamin E in 1997 showed a decline in progression of AD which remarkably shifted the attention toward the advancement of vitamin E studies in the development of AD treatments. However, several failed clinical trials later left the efficacy of vitamin E questionable. The study results may have been influenced by the discrepancies in the bioavailability of vitamin E such as the base nutritional status, intestinal differences, and rate of absorption for each cohort in the clinical trials, alongside the acclaimed heterogeneity of AD pathogenesis itself (Table 1).
The Neural Mechanisms Involved in Alzheimer’s Disease
Currently, the major theories related to the mechanisms involved in the pathogenesis of AD are the neuronal extracellular deposition of Aβ peptides (senile/amyloid plaques), and neuronal intracellular accumulation of hyperphosphorylated tau protein to form neurofibrillary tangles (NFTs). However, the major underlying factor for the cognitive and behavioral dysfunction observed in AD is synaptic dysfunction. For instance, human neuronal dysfunction is associated with increased Aβ and phosphorylated tau reduces synaptic strength due to its aggregation in the dendritic spine and consequent internalization of N-methyl-d-aspartic acid receptors (NMDARs) (Sperling et al., 2009; Palop and Mucke, 2010; Wesson et al., 2011). Other factors such as oxidative stress, which is increased in the brain in aging, have been shown to precede the formation of senile plaques and deposition of NFTs (Huang et al., 2016; Singh et al., 2016; Uddin and Kabir, 2019). Moreover, increased levels of inflammatory cytokines and associated genes have also been implicated in the development of AD (Hollingworth et al., 2011; López González et al., 2016). Some emerging neural mechanistic signaling pathways that have been associated with the pathogenesis of AD are discussed below.
The Nerve Growth Factor Metabolic Pathway
Nerve growth factor (NGF) is a member of the neurotrophin family, which plays a critical role in the functions of the central and peripheral nervous systems (PNSs). NGF was earlier recognized for its notable role in the development of the embryo, the differentiation and survival of the PNS, as well as the differentiation and maintenance of the PNS in adulthood (Levi-Montalcini and Angeletti, 1968; Levi-Montalcini, 1987). The role of NGF in the central nervous system (CNS) was later recognized from the expression of NGF receptors in the basal forebrain cholinergic neurons (BFCN) (Schwab et al., 1979; Seiler and Schwab, 1984).
NGF is mainly released as a precursor protein (proNGF) into the extracellular space, which is then cleaved by the action of a serine protease, plasmin, to mature NGF (mNGF) (Bruno and Cuello, 2006). Upon the release of proNGF by the postsynaptic neurons of the cortex and hippocampus, and its conversion to mNGF, the physiological roles of NGF are executed by binding to two specific cell-surface receptors, the tropomyosin-related kinase A (TrkA) receptor and p75 neurotrophin receptor (p75NTR). When bound to its receptor, predominantly TrkA, on pre-synaptic cholinergic neurons, NGF gets to the cell bodies of the innervating neurons, the BFCN, through a retrograde transport, to initiate, a signaling cascade for the release of acetylcholine. NGF maturation stimulated by plasmin is positively regulated by tissue plasminogen activator (tPA) and urokinase plasminogen activator (uPA), which convert plasminogen to plasmin, and are negatively regulated by plasminogen activator inhibitor 1 (PAI-1) and neuroserpin, inhibiting the activators. The mNGF degradation, on the other hand, is by activated matrix metalloproteinases (MMPs), for example, MMP-9 release upon neuronal stimulation, and regulated by the tissue inhibitor of metalloproteinases 1 (TIMP-1). Plasmin is as well responsible for the activation of proMMP-9 to MMP-9. In addition, it has also been shown that there is in-vitro and in-vivo degradation of mNGF by MMP-3, a protease produced in response to cholinergic stimulation in cortical cells. Although alteration in MMP-3 has been shown to be sex-specific, its level is associated with cognitive decline and AD pathology in humans in addition to its observed upregulation in the experimental model of AD (Pentz et al., 2021).
In AD, this coordinated array of activities is altered at different levels of the pathway. These include alteration in the conversion of proNGF to mNGF, downregulation of TrkA receptor, impaired retrograde signaling and transport, increased mNGF degradation, and inflammatory response due to reduced acetylcholine production and increased Aβ levels (Mitra et al., 2019). Therefore, the NGF metabolic pathways involve a coordinated activity of proteases responsible for NGF maturation (e.g., tPA, plasmin, and neuroserpin) as well as those involved in NGF degradation (e.g., MMP-3, MMP-9, and TIMP-1).
Owing to the promising effect of NGF in ameliorating pathologic conditions associated with AD, a number of approaches have been made and ongoing to therapeutically deliver NGF to the diseased brain (Mitra et al., 2019). Studies in experimental model organisms have shown that the t-PA/plasmin system is an important factor that hinders the pathogenesis of AD, through its involvement in the clearance of Aβ microaggregates as well as inhibition of Aβ-induced neurodegeneration (Melchor et al., 2003; Oh et al., 2014). However, human cohort studies showed no significant alteration in the net enzymatic activities of plasmin in AD, although there was an observed increase in the mRNA level of both activators (tPA and uPA) and inhibitors (PAI-1 and α2-antiplasmin) of the plasminogen system, with increases more at the late Braak stage (Barker et al., 2012). Studies have reported increased levels of MMPs such as MMP-1, MMP-2, MMP-3, MMP-9, MMP-13, and MT1-MMP, in AD, as well as their neuroprotective effect and Aβ cleavage properties in the animal model of AD (Fragkouli et al., 2014; Kaminari et al., 2017). As beneficial as some of these proteins appear to be in AD, efforts to overexpress them have been reported to be detrimental (Wilcock et al., 2011; Terni and Ferrer, 2015), thus caution has to be taken in any chosen therapeutic approach.
The Janus Kinase/Signal Transducer and Activator of the Transcription Pathway
The Janus kinase/signal transducer and activator of the transcription (JAK/STAT) pathways ensure that an extracellular signal is translated into a transcriptional response through a direct mechanism.
The JAK/STAT signaling is a pathway responsible for the regulation of the cytokine responsive genes by transducing extracellular signals from ligands such as cytokines, hormones, and growth factors to the nucleus. This pathway is known to regulate processes such as cell growth and proliferation, differentiation, and apoptosis. The binding of these ligands to their receptors activates JAKs, which are of four family members: JAK1, JAK2, JAK3, and Tyk2 (tyrosine kinase 2). This will in turn phosphorylate the receptors as well as the JAKs. The STAT proteins, consisting of seven members: STAT1, STAT2, STAT3, STAT4, STAT5A, STAT5B, and STAT6, are therefore recruited to the sites formed by the phosphorylated JAKs. The STATs became phosphorylated and activated to form dimers. The dimerized STATs translocate to the nucleus to bind specific regulatory sequences and regulate gene expression (Kisseleva et al., 2002; Rawlings et al., 2004).
Evidence linking the involvement of oxidative stress to the pathogenesis of AD is buttressed by the role of reactive oxygen species in the activation of the JAK/STAT signaling pathway (Simon et al., 1998). Moreover, inhibition of the JAK/STAT pathway by various pharmacological agents has been shown to have a protective effect on AD, through the regulation of Nrf2 signaling (Sharma et al., 2020). In addition, the potential therapeutic role of receptor activation (e.g., TREM2) and folic acid supplementation has been associated with the modulation of the JAK/STAT signaling pathway (Li et al., 2016; Ruganzu et al., 2021). Micropeptides such as humanin and colivelin have also been shown to have neuroprotective effects in models of AD by activating the JAK2/STAT3 signaling pathway (Chiba et al., 2009). Moreover, a combinatorial study involving data from genetic, experimental, observational, and empirical analyses further emphasized the importance of the JAK-STAT signaling as a potential therapeutic target for AD (Nevado-Holgado et al., 2019).
Various experimental models have shown that phosphorylated STAT co-localizes with markers of astrocytes and microglia but not neurons. In addition, some genes such as Ntrk3, Cox17, and Grid2ip have been identified as the cell-specific target, because their predominant expression in astrocytes points to a specific role of STAT3 in AD pathology (Chintapaludi et al., 2020). One of the several functions of the astrocyte in the brain is the regulation of synaptic transmission, and protection and support for neurons, through the release of growth factors and cytokines (Murphy, 1993; Pascual et al., 2005). Astrocytes have been shown to play a crucial role in the degradation of amyloid plaques through the production of TGF-β to inhibit microglial activity, as well as the modulatory effect of ApoE, which is highly expressed in astrocytes (Vincent et al., 1997; Wegiel et al., 2000; Wyss-Coray et al., 2003; Koistinaho et al., 2004). Therefore, loss of astrocyte function has been found central to the pathogenesis of AD (Acosta et al., 2017). In AD, there is astrocyte involvement in oxidative stress, suppression of innate immunity, and increased expression of pro-inflammatory cytokines, suggesting the relevance of astrocyte-targeted anti-inflammatory therapy (Furman et al., 2012; González-Reyes et al., 2017; Kamal et al., 2020).
Increased expression of proteins such as glial fibrillary acidic protein (GFAP) was recognized as a “gold standard” biomarker of reactive astrocyte, a responsive state of astrocyte to a pathology (Eng et al., 2000). GFAP expression was shown to increase in the Braak stage (Simpson et al., 2010). Other potential protein biomarkers not entirely astrocyte-specific but have been shown to be altered in AD-related astrocyte dysfunction include excitatory amino acid transporters (EAATs) (of which EAAT2 has been shown to decrease with an increase in Braak stage) (Simpson et al., 2010), astrocyte-derived S100B, CD44, glutamine synthetase, and aldehyde dehydrogenase 1 family, member L1, (ALDH1L1) (Garwood et al., 2017).
The FGF7/FRFR2/PI3K/AKT Pathway
Fibroblast growth factors (FGFs) FGF family of growth factors is a large family of proteins with 22 identified ligands. They bind to high-affinity tyrosine kinase receptors, the FGFRs, to exert their cellular and physiological effects, which include proliferation, angiogenesis, invasion, and migration. This binding activates the intracellular tyrosine kinase domain of FGFR by phosphorylation of specific tyrosine residues. Once activated, the FGFR couple downstream intracellular signaling pathways, one of which is the PI3K/AKT signaling. In the PI3K/AKT pathway, the protein GRB2 is recruited, which then recruits another protein GAB1 to activate PI3K. Activated PI3K thereafter phosphorylates AKT (Beenken and Mohammadi, 2009; Ornitz and Itoh, 2015).
FGF/FGFR signaling is involved in the development of many organs in the body, including the brain, and its deregulation is associated with a variety of disease conditions (Xie et al., 2020). Among the family of FGFs, the mRNA levels of FGF7 have been shown to be elevated in patients with AD and cells treated with β-amyloid peptides (Aβ). In corroboration, overexpression of FGF7 in Aβ-treated cells was also shown to be associated with a reduction in cell viability and proliferative rate. Studies have indicated altered expression of miRNAs in the pathogenesis of AD and in experimental models of AD (Samadian et al., 2021). There is reduced expression of miR-107 in the cell model of AD and in CSF samples from AD patients (Chen et al., 2020). Interestingly, the 3′UTR of FGF7 was identified to have its binding site on the miR-107. Overexpression of miR-107 in AD model cells treated with Aβ reduced mRNA and protein levels of FGF7, and as well reduced inflammation and apoptosis, and restored cell viability (Chen et al., 2020). Western blotting analysis showed detection of FGFR2, PI3K, Akt, and Akt phosphorylation as a downstream pathway of FGF7. Therefore, data from this study implicates the involvement of the FGF7/FGFR2/PI3K/Akt signaling pathway in the pathogenesis of AD and hence a potential for therapeutic intervention (Figure 1).
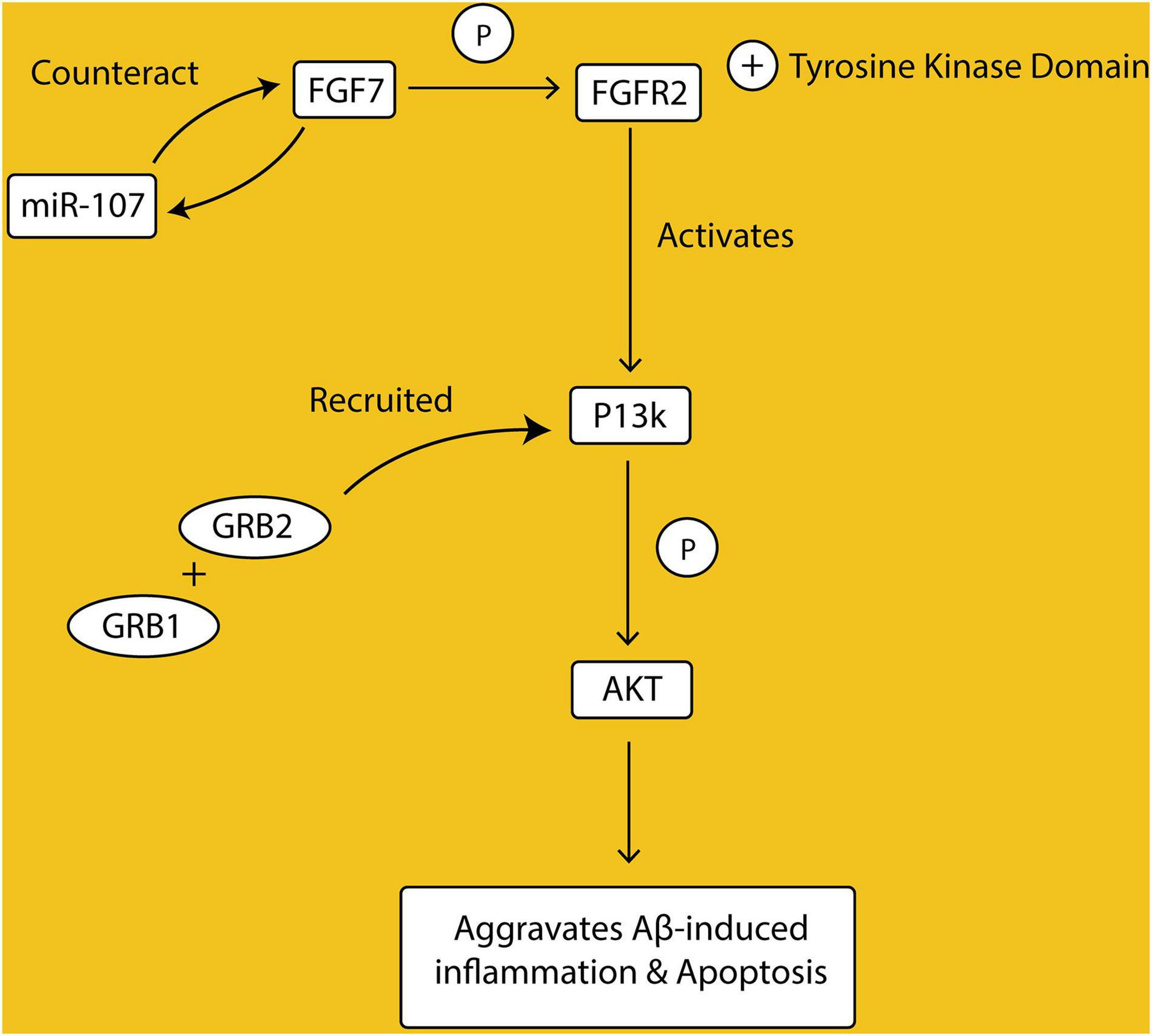
Figure 1. Fibroblast growth factors (FGFs) bind to their receptors (FGFRs), activating their tyrosine kinase domain through phosphorylation. FGF/FGFR complex initiates the downstream signaling, by recruiting Growth factor receptor-bound protein 2 (GRB2) and Growth factor receptor-bound protein 1 (GRB1), which then activate Phosphoinositide 3-kinases (P13K) and phosphorylate Protein kinase B (AKT). Activation of P13K/AKT pathway exacerbates amyloid beta-induced inflammation and apoptosis. FGF7 has a binding site on MicroRna17 (miR-107) and overexpression of miR-107 reduces FGF7 levels.
Some important proteins and their modulating factors associated with the above AD pathways are shown in Table 2.
Vascular Dysfunction and Cognitive Impairment
Recently, there has been an increasing interest in the relationship between vascular dysfunction and dementia. Clinicopathological and epidemiological studies have shown that various forms of systemic vascular disorders and cerebrovascular disease contribute to cognitive impairment and dementia (He et al., 2020). Several modifiable vascular risk factors are linked to AD type of dementia. These include hypertension, diabetes mellitus, metabolic syndrome, hypercholesterolemia, obesity, and smoking (Akinyemi et al., 2013). Vascular risk factors are associated with higher tau burden (Vemuri et al., 2017), higher cerebral beta-amyloid (Aβ) deposition (Langbaum et al., 2012; Gottesman et al., 2017), and act with Aβ synergistically to stimulate cognitive decline (Rabin et al., 2018).
Vascular dysfunction is a significant early component of AD pathology (Sweeney et al., 2019). The brains of AD patients are usually marked by abnormal NFT and amyloid plaque deposition not just in and around the neurons, but also in the blood vessels and perivascular space as well (Merlini et al., 2016). This leads to morphological changes and dysfunction of the vascular wall components (Greenberg et al., 2004). The tau, amyloid, and vascular dysfunction collectively contributed toward a speedy cognitive decline (Merlini et al., 2016).
The dense network of macro- and microvasculature supplies the brain with a continuous but regulated cerebral blood flow (CBF). The neurovascular unit (NVU) describes a structural and functional relationship among the cerebral microvascular cells (endothelium, pericytes and adventitial cells), neurons, and glial cells (astrocytes, microglia and oligodendrocytes). The NVU ensures a coherent response to injury to any components of the unit that are vulnerable to amyloid (Iadecola, 2004). Cerebrovascular autoregulation and the BBB are essential to sustain the NVU’s integrity (Bell and Zlokovic, 2009).
Cerebrovascular autoregulation maintains the CBF despite the changes in mean arterial pressure, while the BBB restricts the entry of toxic substances into the brain through its impermeable membrane (Bell and Zlokovic, 2009). Endothelial dysfunction associated with vascular risk factors such as hypertension and diabetes mellitus may cause the breakdown of these defense mechanisms, leading to BBB leakage, loss of autoregulation, cerebral hypoperfusion, and hypoxia (Kivipelto et al., 2002; Solomon et al., 2009). Additionally, Aβ accumulation within the microvessels itself further aggravates the endothelial damage (Jeynes and Provias, 2006; Burgmans et al., 2013). Brain magnetic resonance imaging of patients with clinically diagnosed AD often showed white matter changes demonstrated as white matter hyperintensities (WMH). WMH corresponds to areas of reduced CBF and hypoxia (Yamaji et al., 1997; Takahashi et al., 2000).
Neuroimaging has emerged as a revolutionary method to capture the alteration in CBF and its link to cognitive impairment and AD. Among the neuroimaging techniques to measure CBF include but are not restricted to functional MRI, single-photon emission computed tomography, arterial spin-labeling MRI, and dynamic susceptibility contrast MRI (de la Torre, 2018). A prospective study using cerebral arterial spin-labeling-MRI (ASL) in cognitively intact elderly subjects showed that compared to subjects who did not deteriorate to MCI, subjects who developed MCI 18 months later had a global reduction in CBF as captured during baseline ASL (Xekardaki et al., 2015). This study shows that ASL is a useful neuroimaging method to predict the onset of MCI in cognitively intact individuals whose brains showed abnormal CBF. Nevertheless, decreased CBF has been persistently demonstrated in various brain regions before and during cognitive impairment and AD. The most affected brain regions are the hippocampus, posterior cingulate cortex, medial temporal lobe, and precuneus (Dai et al., 2009; Schuff et al., 2009; Alexopoulos et al., 2012; Park et al., 2012; Xekardaki et al., 2015). Since MCI is a high-risk state to progress into dementia, including AD, CBF assessment combined with neurocognitive tests can be used to find out the susceptibility of patients with MCI to convert to AD, thus allowing physicians to decide which patients need more vigorous intervention and close follow-up. The Alzheimer’s Disease Neuroimaging Initiative also recommended that neuroimaging for CBF should be appraised as the primary predictive biomarker for AD (Iturria-Medina et al., 2016).
Vascular disorders are strongly associated with disintegrated BBB and AD (Provias and Jeynes, 2014). The breach of the endothelial tight junctions that leads to intense vascular leakage at the BBB is a feature of the early stages of dementia and AD (Teri et al., 1997). The transport of Aβ across the BBB is typically regulated by two major transporter proteins, LDL-receptor-related protein-1 (LRP-1) and receptor for advanced glycation end products (RAGE). LRP-1 promotes the efflux of Aβ from the cerebral parenchyma to the vessels across the BBB (Deane et al., 2009). In contrast, RAGE facilitates Aβ influx from the circulation crossing the BBB into the cerebral parenchyma (Jeynes and Provias, 2008). An Aβ-RAGE interface enhances oxidative stress, neuroinflammation, and subsequent BBB disintegration, which further potentiates cerebral Aβ accumulation (Schmidt et al., 2009). Whereas P-glycoprotein (PGP) has been demonstrated to damper RAGE activity in brain capillary endothelial cells that compose the BBB (Candela et al., 2010). In short, an imbalance between LRP-1, RAGE, and PGP expression together with disintegrated BBB remarkably contributed to vascular dysfunction and AD.
Vasoactive regulatory proteins such as vascular endothelial growth factor (VEGF) and endothelial nitric oxide synthase (eNOS) also play a role in maintaining BBB integrity and therefore affect the cerebral Aβ transport (Farkas and Luiten, 2001; Jeynes and Provias, 2011). Patients with AD commonly have low serum VEGF levels (Mateo et al., 2007) and this is associated with progressive loss of cognitive function (Tang et al., 2013). Decreased expression of VEGF and eNOS within the cerebral microvasculature disrupts the normal angiogenesis and BBB integrity. This leads to less Aβ efflux across the BBB from the cerebral parenchyma into the circulation in the human brain with AD (Provias and Jeynes, 2014).
Genome-wide association studies have revealed a number of genetic associations with AD (Naj et al., 2017), of which the most important is the apolipoprotein E gene on chromosome 17 (APOE gene, apoE protein) (Apostolova et al., 2018). Other polymorphisms are associated with genes linked to lipid metabolism, inflammation, and intracellular trafficking, but none of them are as consistent as that of apoE (Bis et al., 2020). ApoE is a component of very-low-density lipoproteins that is involved in cholesterol transport among various cells in the body. ApoE4 allele frequency is higher in patients with vascular dementia, AD, and ischemic cerebrovascular disease (Ji et al., 1998). ApoE4 carriers with atherosclerosis commonly have co-morbid AD, cognitive impairment, and dementia (Slooter et al., 1999). AD patients who are ApoE4 carriers have a higher Aβ burden compared with non-carriers (Dorey et al., 2014). ApoE4 disrupts brain lipid metabolism, impedes CBF, and triggers cerebral amyloid angiopathy (Reinvang et al., 2013). The binding of Aβ to ApoE reduced LRP-1 functioning in mice (Bell et al., 2007). Furthermore, an in vitro BBB model comprising mouse brain capillary endothelial cells demonstrated that ApoE4 disrupts endothelial tight junction integrity and BBB permeability (Nishitsuji et al., 2011; Figure 2).
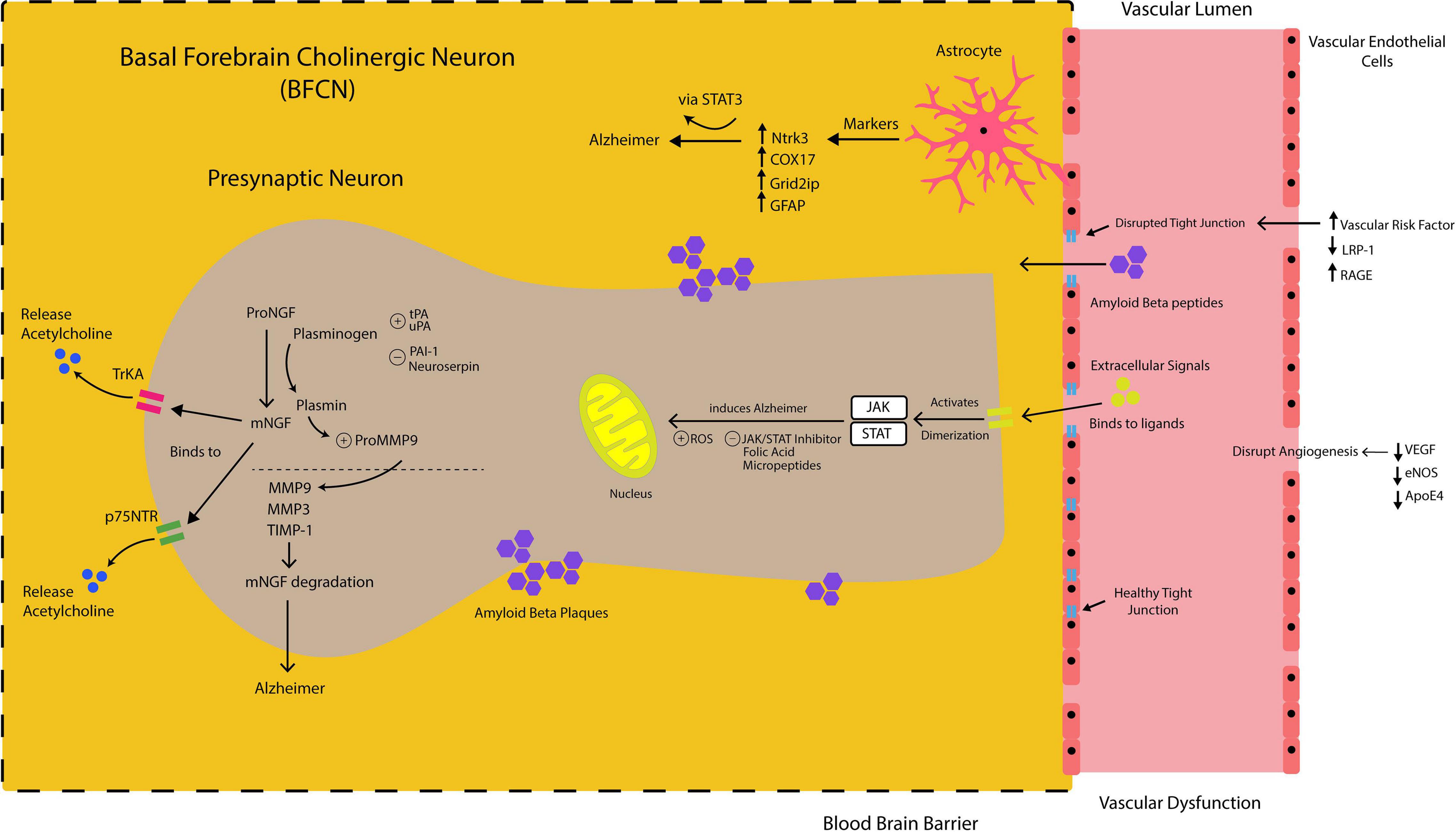
Figure 2. Nerve growth factor (NGF) metabolic pathway in basal forebrain cholinergic neuron (BFCN) is mainly regulated by proteases involved in NGF maturation [tissue plasminogen activator (tPA), urokinase plasminogen activator (uPA), Plasminogen Activator Inhibitor 1 (PAI-1) and Neuroserpin)] and those involved in NGF degradation [matrix metalloproteinases (MMP9, MMP3) and tissue inhibitor of metalloproteinases 1 (TIMP-1)]. Plasmin is also responsible for proMMP-9 activation to MMP9. Extracellular signals (i.e., cytokines, hormones, and growth factor) bind to their ligands and activate Janus Kinase/signal transducer and activator of transcription (JAK/STAT). This causes dimerization of the two and allows them to be translocated to the nucleus for transcriptional response. Reactive Oxygen Species (ROS) is involved in JAK/STAT-induced Alzheimer. JAK/STAT inhibitor, folic acid and micropeptides (i.e., humorin and colivelin) attenuate JAK/STAT-induced Alzheimer. Increased astrocytes biomarker Glial fibrillary acidic protein (GFAP) and Genes were predominantly expressed on Astrocytes- STAT3-induced Alzheimer’s disease (Neurotrophic Receptor Tyrosine Kinase 3 (ntrk3), Cytochrome C Oxidase Copper Chaperone (COX17), and Grid2 Interacting Protein (Grid2ip). Decreased vascular endothelial growth factor (VEGF), endothelial nitric oxide synthase (eNOS) and apolipoprotein e4 (ApoE4) disrupt normal angiogenesis. Increased vascular risk factor, receptor for advanced glycation end products (RAGE) and reduced LDL-receptor related protein-1 (LRP-1) disrupt healthy tight junction. Disruption of normal angiogenesis and healthy tight junction allow Amyloid-Beta peptides efflux from vessels to brain parenchyma and promoting Amyloid-Beta plaques formation.
Omics in Alzheimer’s Disease
Fresh molecular and high-throughput methods are shedding new insight into the pathways and networks at the heart of this complicated disease. This is required in order to go forward with the precision medicine paradigm toward the discovery of novel molecular markers, systematic risk categorization, and translational targeted medicines (Kosik, 2015; Castrillo and Oliver, 2016; Kovacs, 2016).
The implementation of a complete precision medicine strategy, as well as the creation of relevant biomarkers, are likely to result in significant advancements in recognizing, treating, and preventing AD.
AD and many other genetically heterogeneous illnesses (e.g., autism, other NDs, and some malignancies) are far too varied to be the sole cause or to be centered on genetic variants. Certain complicated disorders are distinguished by the following characteristics: (1) multifactorial in nature, integrating genetic, epigenomic, and interactomics factors; (2) primarily caused by “modified” networks that influence important modules and interactomes; and (3) deeply complicated, with a strong interplay between impacted and homeostatic defense systems. As a result, for many complex disorders, comprehensive system-level therapies are necessary (Castrillo and Oliver, 2016).
Advanced theoretical and statistical systems biology techniques are being applied to better understand the pathophysiology of multifactorial illnesses such as neurodegenerative illnesses. These methods include high-throughput omics techniques that investigate genome, transcriptome, proteome, and metabolome patterns and interactions, as well as integrative approaches (Castrillo and Oliver, 2016). Multi-omics trials are currently being utilized in integrated personal omics profiling (iPOP) longitudinal research to track panels of biomarkers and trends for diagnosis and customized therapy (Chen et al., 2012).
Among the most important systems-level techniques, including the use of omics technology to dissect AD (Tosto and Reitz, 2016), are 1. Transcriptomics: RNA-Seq to elucidate early patterns of dysregulation underlying ADe (Chen et al., 2016); experimental approaches applied to the analysis of RNA networks in AD (Pichler et al., 2017); RNA-Seq experiments demonstrating (Bai et al., 2014), 2. Proteomics investigations indicate that amyloid disrupts splicing pathways (Nuzzo et al., 2017), metalloproteomics and redox proteomics studies in AD (Hare et al., 2016), and plasma proteomics biomarkers in AD (Perneczky and Guo, 2016), 3. Metabolomics: AD-related changes in metabolic processes and networks (Toledo et al., 2017).
The human blood metabolome is made up of hundreds of tiny molecular species, most of which have a molecular weight of less than 1,500 Da (Daltons; 1.7 1027 kg) and are predominantly composed of monosaccharides, acylcarnitines, biogenic amines, amino acids, fatty acids, and complex lipids. The metabolome is regarded as a downstream linear mirror of the genome/epigenome, transcriptome, and proteome, sequentially and in close proximity to the clinical phenotype, using typical reductionistic methodologies. Although the aforementioned may be accurate from a systems biology standpoint, intricate interrelationships occur between the many “omic” levels (Yugi et al., 2016), which, if correctly integrated, are likely to give a greater understanding of a complicated disease state or human health.
Recent reports of metabolomic biomarkers for AD have been developed using specimens from cross-sectional studies analyzed with MS platforms, typically comparing metabolite abundances between control subjects and individuals with either prodromal or manifest AD (Klavins et al., 2015).
Recent approaches in the treatment of AD include exploring the potentials of some metabolites to modulate signaling pathways associated with neurovascular endothelium through multi-omic analyses (Corral-Jara et al., 2021). There have also been reports on cellular signaling-related sex-dependent effects under hyperglycemic and lipid stress (Nuthikattu et al., 2020, 2021). However, this review focuses on significant signaling pathways associated with the stages of AD, the potential links between vascular dysfunction and AD, as well as recent developments in “omics”-based approaches in AD. Without publication date restriction, PubMed, ScienceDirect, and Google Scholar databases were searched for published articles containing the search terms related to “Alzheimer,” “dementia,” “signaling pathways,” “vascular dysfunction,” “cognitive impairment,” and “omics.”
Summary and Future Directions
In this review, we discussed many signaling pathways and postulated mechanisms associated with AD. Mild cognitive impairment (MCI) can be an early stage of the disease continuum for dementia, including AD. In line with this, the aforementioned signaling markers are being increasingly studied as potential early diagnostic or prognostic markers for cognitive decline-related pathologies. As for the NGF pathway, a meta-analysis and systematic review of 170 studies reported a significant increase in the peripheral levels of NGF along with other proinflammatory markers in MCI compared with controls (Shen et al., 2019). More recently, markers associated with the NGF pathway, such as proNGF, plasminogen, neuroserpin, and MMP9 was shown to be elevated, whereas mNGF was downregulated in the brain tissue of MCI and AD patients (Pentz et al., 2020). In line with this, Senescence-Associated Secretory Phenotype (SASP) biomarkers, which is upstream of JAK/STAT signaling at the cellular level, has been reported to be elevated in the plasma of older adults with MCI, MCI + Major Depressive Disorder (MDD), and MDD (Diniz et al., 2021). To date no reports have directly implicated FGF7/FGFR2 as early markers of cognitive impairment at the clinical level, hence more studies should look into this.
It is important to distinguish between brain aging and AD dementia. With age, our brains experience natural structural, chemical and functional degenarations, as well as significant cognitive decline, which is characterized by brain shrinkage, decreased blood flow, synapse degeneration, and neurochemical alternations (Damoiseaux, 2017). Most of the human studies, on the other hand, seldom exclude prevalent health disorders associated with aging, such as hypertension, which may have an impact on how accurate the conclusions are. The study of age-related brain alterations in non-human animal models is therefore essential for distinguishing between normal aging and pathological brain abnormalities in humans. Studying the basic processes of aging in animals will consequently provide an essential foundation for the development of innovative therapies for age-related brain problems in people (Alexander et al., 2020).
Normal aging has been shown to be associated with significant changes in the brain network and the processes that it controls (Huang et al., 2015; Bonte et al., 2017). The human brain’s network has been reorganized as a result of aging-related changes in functional, metabolic, and structural connections, according to recent research. It has been discovered that functional connection reduces with age, both within and between numerous resting-state networks, including the default mode network, salience network, dorsal attention network, and sensorimotor network (Huang et al., 2015). The aged also have lower modularity and efficiency in their brain networks (Liu et al., 2014; Geerligs et al., 2015; Zhao et al., 2015), as well as a degeneration process in which the aging brain system transitions from a small-worldness network to a regular network in conjunction with normal aging. The findings of functional connectivity studies (Damoiseaux, 2017) show that age-related changes in structural network metrics are comparable to those seen in structural network metrics.
A good method for evaluating energy consumption in neurons is [18F] fluorodeoxyglucose positron emission tomography (18F-FDG PET), which is a reflection of neural transmission signals (Rocher et al., 2003). As a result, 18F-FDG PET is regarded as a functional neuroimaging tool for identifying changes in brain activity associated with advancing years of age. A large number of studies have been conducted using 18F-FDG PET to explore the changes in brain processes that occur as a result of normal aging throughout the past several decades. Overall, a considerable age-related reduction in glucose metabolism in the frontal lobe has been seen in most investigations (Pardo et al., 2007; Kalpouzos et al., 2009), which is consistent with other findings. Glucose uptake may be decreased as a result of tissue loss or shrinking (Bonte et al., 2017). As well as in metabolic brain networks, age-related alterations can be observed in association and paralimbic cortex areas, including increased clustering, decreased efficiency and robustness, as well as altered nodal centralities (Rocher et al., 2003; Liu et al., 2014). Whether the age-related changes in glucose metabolism are consistent between the rat brain and the human brain, particularly in terms of metabolic brain networks, has not been determined conclusively at this point. It is yet unknown if the metabolic network in the brains of old rats exhibits age-related alterations that are comparable to those observed in the brains of humans.
A recent study of single-cell transcriptome datasets from the human brain revealed a link between AD gene signals and microglia, as well as astrocytes and astrocyte-like cells. Studies of human microglia (MG) produced from embryonic stem cells in the mouse brain have shown the cells are transcriptionally like human primary microglia ex vivo and express genes associated with Alzheimer’s disease (AD). However, just one-third of the suspected Alzheimer’s disease risk genes have appropriate mouse orthologs (Mancuso et al., 2019), which is concerning. Furthermore, oligomeric amyloid-beta causes a different response in human microglia compared to mice microglia (MG). As research advances, it is becoming increasingly clear that signals from the CNS microenvironment are required to maintain microglial specification, and that the absence of these cues results in a dramatic disruption of the microglia phenotype, driving them toward an activated state. Additionally, certain well-known disease-associated genes (for example, triggering receptor expressed on myeloid cells 2 (TREM2)–membrane phospholipids–apolipoprotein E (APOE), CD33–sialic acid) play a role in the cross-talk between microglia and other brain cells.
Soreq et al. (2017) recently discovered significant differences in glial gene expression as well as neuronal and oligodendrocyte (OLG) cell counts in the brains of elderly people as compared to young people. A total of 1,231 postmortem brain samples from 134 people (ages 16–104), profiled by exon microarrays (10 brain areas), and 480 samples from the North American brain bank were analyzed (Soreq et al., 2017; Elmore et al., 2018). The 3′ microarray data from microglia-depleted animals (including comparisons between young and elderly mice) was also used to analyze the mice. Authors discovered considerable alterations in gene expression. Similarly, significant changes in AD may be observed in the future.
A detailed analysis of RNA-Seq data can be performed using machine learning and statistics, including classification methods [for example, unsupervised hierarchical classification (HCL) and bioinformatic algorithms], correlation analyses, and the detection of gene expression changes in cell-specific markers, pro-inflammatory genes, and splicing factors, as well as genes associated with the cell cycle. Linear regression may also be used to identify genes that have been changed. Functional Gene Ontology (GO) analysis, which is non-parametric, may detect changes in molecular functions (MF) and biological processes (BP) (Luo and Chen, 2016). The genes that were discovered can also be examined in the human cell Atlas (Regev et al., 2017). Aside from that, developments in genetics and sequencing have uncovered an abundance of disease-associated genetic modifications; nonetheless, precise models for molecular dissection are still needed to fully understand these changes.
The precision of molecular medicine is significantly improved by using a multi-omics approach. When it comes to precisely understanding the impact of mutations or therapies, single-cell studies are crucial. Transcriptional networks may be constructed from single-cell data, if necessary. The information obtained may aid in gaining a better understanding of the impact of altered genes on disease pathophysiology. Cell lines or model organisms can be used to determine the underlying cause of disease, but other technologies are required to complete the task. The introduction of single-cell RNA-Seq had a significant influence on the field. It is possible to obtain accurate cell state comparisons by comparing healthy samples to sick samples in the same experiment. Major faults may be identified via cell harmony analysis, and genetic rescue of mutations is also conceivable after investigations of cell state models and more clinical Alzheimer’s disease samples. Additionally, differentiating gene expression across distinct cell states may be investigated.
When analyzing data, clustering is a way of grouping closely related observational findings into groupings called “clusters” that are based on the results of numerous variables for each subject. Because it serves as a critical intermediatory tool in experimental investigations, it is incredibly significant in scientific research. However, it is particularly valuable in gene analysis because it contributes to the knowledge of specific cell processes. This easy and helpful adaptation to a variety of different scientific fields has not only enabled its widespread usage but has also facilitated the creation of a variety of distinct clustering methodologies. Because of this, these sophisticated approaches may be utilized to struttingly analyze data in order to give trustworthy gene analysis (Park et al., 2009; Habib et al., 2020). Other research has discovered that previously illness-associated astrocytes are changed in AD and aging using single-cell RNA-Seq, demonstrating that astrocytes are linked to hereditary and age-related disease factors (Habib et al., 2020).
Conclusion
The pathogenesis of AD is multifactorial. Continuous discovery of novel signaling pathways in AD reflects the complex nature of the disease. Such complexity should be addressed in patients’ management for better treatment outcomes, and tools such as omics are better fit to be incorporated into treatment plans. Vascular dysfunction has a deleterious impact on the brain that builds up to AD pathogenesis. Since measures to tackle the vascular risk factors are available, the future prevalence of AD can be minimized by employing strategies that preserve cardiovascular health.
Author Contributions
JK contributed to the conceptual framework and design of the manuscript. MA, KS, AU, WM, HK, NHI, and JK drafted the manuscript. HK contributed to the preparation of figures and figure legends. MA, KS, AU, WM, CK, and JK critically revised the manuscript. All authors contributed to the article and approved the submitted version.
Funding
This study was supported by the GUP-2018-055.
Conflict of Interest
The authors declare that the research was conducted in the absence of any commercial or financial relationships that could be construed as a potential conflict of interest.
Publisher’s Note
All claims expressed in this article are solely those of the authors and do not necessarily represent those of their affiliated organizations, or those of the publisher, the editors and the reviewers. Any product that may be evaluated in this article, or claim that may be made by its manufacturer, is not guaranteed or endorsed by the publisher.
Acknowledgments
The authors would like to thank the Faculty of Medicine, National University of Malaysia.
Footnotes
References
Acosta, C., Anderson, H. D., and Anderson, C. M. (2017). Astrocyte dysfunction in Alzheimer disease. J. Neurosci. Res. 95, 2430–2447. doi: 10.1002/jnr.24075
Akhter, H., Katre, A., Li, L., Liu, X., and Liu, R.-M. (2011). Therapeutic potential and anti-amyloidosis mechanisms of tert-butylhydroquinone for Alzheimer’s disease. J. Alzheimers Dis. 26, 767–778. doi: 10.3233/JAD-2011-110512
Akhter, H., Huang, W.-T., van Groen, T., Kuo, H.-C., Miyata, T., and Liu, R.-M. (2018). A small molecule inhibitor of plasminogen activator inhibitor-1 reduces brain amyloid-β load and improves memory in an animal model of Alzheimer’s disease. J. Alzheimers Dis. 64, 447–457. doi: 10.3233/JAD-180241
Alexander, G. E., Lin, L., Yoshimaru, E. S., Bharadwaj, P. K., Bergfield, K. L., Hoang, L. T., et al. (2020). Age-related regional network covariance of magnetic resonance imaging gray matter in the rat. Front Aging Neurosci. 12:267. doi: 10.3389/fnagi.2020.00267
Alexopoulos, P., Sorg, C., Förschler, A., Grimmer, T., Skokou, M., Wohlschläger, A., et al. (2012). Perfusion abnormalities in mild cognitive impairment and mild dementia in Alzheimer’s disease measured by pulsed arterial spin labeling MRI. Eur. Arch. Psychiatry Clin. Neurosci. 262, 69–77. doi: 10.1007/s00406-011-0226-2
Alzheimer’s Association (2021). 2021 Alzheimer’s disease facts and figures. Alzheimers Dement. 17, 327–406. doi: 10.1002/alz.12328
Apostolova, L. G., Risacher, S. L., Duran, T., Stage, E. C., Goukasian, N., West, J. D., et al. (2018). Associations of the top 20 Alzheimer disease risk variants with brain amyloidosis. JAMA Neurol. 75, 328–341. doi: 10.1001/jamaneurol.2017.4198
Bai, B., Chen, P. C., Hales, C. M., Wu, Z., Pagala, V., High, A. A., et al. (2014). Integrated approaches for analyzing U1-70K cleavage in Alzheimer’s disease. J. Proteome Res. 13, 4526–4534. doi: 10.1021/pr5003593
Barker, R., Kehoe, P. G., and Love, S. (2012). Activators and inhibitors of the plasminogen system in Alzheimer’s disease. J. Cell. Mol. Med. 16, 865–876. doi: 10.1111/j.1582-4934.2011.01394.x
Beenken, A., and Mohammadi, M. (2009). The FGF family: biology, pathophysiology and therapy. Nat. Rev. Drug Discov. 8, 235–253. doi: 10.1038/nrd2792
Bell, R. D., Sagare, A. P., Friedman, A. E., Bedi, G. S., Holtzman, D. M., Deane, R., et al. (2007). Transport pathways for clearance of human Alzheimer’s amyloid β-peptide and apolipoproteins E and J in the mouse central nervous system. J. Cereb. Blood Flow Metab. 27, 909–918. doi: 10.1038/sj.jcbfm.9600419
Bell, R. D., and Zlokovic, B. V. (2009). Neurovascular mechanisms and blood–brain barrier disorder in Alzheimer’s disease. Acta Neuropathol. 118, 103–113. doi: 10.1007/s00401-009-0522-3
Bis, J. C., Jian, X., Kunkle, B. W., Chen, Y., Hamilton-Nelson, K. L., Bush, W. S., et al. (2020). Whole exome sequencing study identifies novel rare and common Alzheimer’s-Associated variants involved in immune response and transcriptional regulation. Mol. Psychiatry 25, 1859–1875. doi: 10.1038/s41380-018-0112-7
Bonte, S., Vandemaele, P., Verleden, S., Audenaert, K., Deblaere, K., Goethals, I., et al. (2017). Healthy brain ageing assessed with 18F-FDG PET and age-dependent recovery factors after partial volume effect correction. Eur. J. Nucl. Med. Mol. Imaging 44, 838–849. doi: 10.1007/s00259-016-3569-0
Bruno, M. A., and Cuello, A. C. (2006). Activity-dependent release of precursor nerve growth factor, conversion to mature nerve growth factor, and its degradation by a protease cascade. Proc. Natl. Acad. Sci. U.S.A. 103, 6735–6740. doi: 10.1073/pnas.0510645103
Burgmans, S., van de Haar, H. J., Verhey, F. R., and Backes, W. H. (2013). Amyloid-β interacts with blood-brain barrier function in dementia: a systematic review. J. Alzheimers Dis. 35, 859–873. doi: 10.3233/JAD-122155
Busche, M. A., and Hyman, B. T. (2020). Synergy between amyloid-β and tau in Alzheimer’s disease. Nat. Neurosci. 23, 1183–1193. doi: 10.1038/s41593-020-0687-6
Candela, P., Gosselet, F., Saint-Pol, J., Sevin, E., Boucau, M.-C., Boulanger, E., et al. (2010). Apical-to-basolateral transport of amyloid-β peptides through blood-brain barrier cells is mediated by the receptor for advanced glycation end-products and is restricted by P-glycoprotein. J. Alzheimers Dis. 22, 849–859. doi: 10.3233/JAD-2010-100462
Castrillo, J. I., and Oliver, S. G. (2016). Alzheimer’s as a systems-level disease involving the interplay of multiple cellular networks. Methods Mol. Biol. 1303, 3–48. doi: 10.1007/978-1-4939-2627-5_1
Chen, B. J., Mills, J. D., Janitz, C., and Janitz, M. (2016). RNA-sequencing to elucidate early patterns of dysregulation underlying the onset of Alzheimer’s disease. Methods Mol. Biol. 1303, 327–347. doi: 10.1007/978-1-4939-2627-5_20
Chen, R., Mias, G. I., Li-Pook-Than, J., Jiang, L., Lam, H. Y., Chen, R., et al. (2012). Personal omics profiling reveals dynamic molecular and medical phenotypes. Cell 148, 1293–1307. doi: 10.1016/j.cell.2012.02.009
Chen, W., Wu, L., Hu, Y., Jiang, L., Liang, N., Chen, J., et al. (2020). MicroRNA-107 ameliorates damage in a cell model of Alzheimer’s disease by mediating the FGF7/FGFR2/PI3K/Akt pathway. J. Mol. Neurosci. 70, 1589–1597. doi: 10.1007/s12031-020-01600-0
Chiba, T., Yamada, M., and Aiso, S. (2009). Targeting the JAK2/STAT3 axis in Alzheimer’s disease. Expert Opin. Ther. Targets 13, 1155–1167. doi: 10.1517/14728220903213426
Chintapaludi, S. R., Uyar, A., Jackson, H. M., Acklin, C. J., Wang, X., Sasner, M., et al. (2020). Staging Alzheimer’s disease in the brain and retina of B6.APP/PS1 mice by transcriptional profiling. J. Alzheimers Dis. 73, 1421–1434. doi: 10.3233/JAD-190793
Corral-Jara, K. F., Nuthikattu, S., Rutledge, J., Villablanca, A., Morand, C., Schroeter, H., et al. (2021). Integrated multi-omic analyses of the genomic modifications by gut microbiome-derived metabolites of epicatechin, 5-(4′-Hydroxyphenyl)-γ-valerolactone, in TNFalpha-stimulated primary human brain microvascular endothelial cells. Front. Neurosci. 15:622640. doi: 10.3389/fnins.2021.622640
Cummings, J., Morstorf, T., and Lee, G. (2016). Alzheimer’s drug-development pipeline: 2016. Alzheimers Dement. 2, 222–232. doi: 10.1016/j.trci.2016.07.001
Cummings, J., Lee, G., Mortsdorf, T., Ritter, A., and Zhong, K. (2017). Alzheimer’s disease drug development pipeline: 2017. Alzheimers Dement. 3, 367–384. doi: 10.1016/j.trci.2017.05.002
Cummings, J., Lee, G., Ritter, A., and Zhong, K. (2018). Alzheimer’s disease drug development pipeline: 2018. Alzheimers Dement. 4, 195–214. doi: 10.1016/j.trci.2018.03.009
Cummings, J., Lee, G., Ritter, A., Sabbagh, M., and Zhong, K. (2019). Alzheimer’s disease drug development pipeline: 2019. Alzheimers Dement. 5, 272–293. doi: 10.1016/j.trci.2019.05.008
Cummings, J., Lee, G., Ritter, A., Sabbagh, M., and Zhong, K. (2020). Alzheimer’s disease drug development pipeline: 2020. Alzheimers Dement. 6:e12050. doi: 10.1002/trc2.12050
Cummings, J., Lee, G., Zhong, K., Fonseca, J., and Taghva, K. (2021). Alzheimer’s disease drug development pipeline: 2021. Alzheimers Dement. 7:e12179. doi: 10.1002/trc2.12179
Dai, W., Lopez, O. L., Carmichael, O. T., Becker, J. T., Kuller, L. H., and Gach, H. M. (2009). Mild cognitive impairment and alzheimer disease: patterns of altered cerebral blood flow at MR imaging. Radiology 250, 856–866. doi: 10.1148/radiol.2503080751
Damoiseaux, J. S. (2017). Effects of aging on functional and structural brain connectivity. Neuroimage 160, 32–40. doi: 10.1016/j.neuroimage.2017.01.077
Deane, R., Bell, R., Sagare, A., and Zlokovic, B. (2009). Clearance of amyloid-β peptide across the blood-brain barrier: implication for therapies in Alzheimer’s disease. CNS Neurol. Disord. Drug Targets 8, 16–30. doi: 10.2174/187152709787601867
de la Torre, J. (2018). The vascular hypothesis of Alzheimer’s disease: a key to preclinical prediction of dementia using neuroimaging. J. Alzheimers Dis. 63, 35–52. doi: 10.3233/JAD-180004
Diniz, B. S., Vieira, E. M., Mendes-Silva, A. P., Bowie, C. R., Butters, M. A., Fischer, C. E., et al. (2021). Mild cognitive impairment and major depressive disorder are associated with molecular senescence abnormalities in older adults. Alzheimers Dement. 7:e12129. doi: 10.1002/trc2.12129
Dorey, E., Chang, N., Liu, Q. Y., Yang, Z., and Zhang, W. (2014). Apolipoprotein E, amyloid-beta, and neuroinflammation in Alzheimer’s disease. Neurosci. Bull. 30, 317–330. doi: 10.1007/s12264-013-1422-z
ElAli, A., Bordeleau, M., Thériault, P., Filali, M., Lampron, A., and Rivest, S. (2016). Tissue-plasminogen activator attenuates Alzheimer’s disease-related pathology development in APPswe/PS1 mice. Neuropsychopharmacology 41, 1297–1307. doi: 10.1038/npp.2015.279
Elmore, M. R., Hohsfield, L. A., Kramár, E. A., Soreq, L., Lee, R. J., Pham, S. T., et al. (2018). Replacement of microglia in the aged brain reverses cognitive, synaptic, and neuronal deficits in mice. Aging Cell 17:e12832. doi: 10.1111/acel.12832
Eng, L. F., Ghirnikar, R. S., and Lee, Y. L. (2000). Glial fibrillary acidic protein: GFAP-thirty-one years (1969-2000). Neurochem. Res. 25, 1439–1451. doi: 10.1023/a:1007677003387
Farkas, E., and Luiten, P. G. (2001). Cerebral microvascular pathology in aging and Alzheimer’s disease. Prog. Neurobiol. 64, 575–611. doi: 10.1016/S0301-0082(00)00068-X
Fish, P. V., Steadman, D., Bayle, E. D., and Whiting, P. (2019). New approaches for the treatment of Alzheimer’s disease. Bioorg. Med. Chem. Lett. 29, 125–133. doi: 10.1016/j.bmcl.2018.11.034
Fragkouli, A., Tsilibary, E. C., and Tzinia, A. K. (2014). Neuroprotective role of MMP-9 overexpression in the brain of Alzheimer’s 5xFAD mice. Neurobiol. Dis. 70, 179–189. doi: 10.1016/j.nbd.2014.06.021
Furman, J. L., Sama, D. M., Gant, J. C., Beckett, T. L., Murphy, M. P., Bachstetter, A. D., et al. (2012). Targeting astrocytes ameliorates neurologic changes in a mouse model of Alzheimer’s disease. J. Neurosci. Res. 32, 16129–16140. doi: 10.1523/JNEUROSCI.2323-12.2012
Garwood, C. J., Ratcliffe, L. E., Simpson, J. E., Heath, P. R., Ince, P. G., and Wharton, S. B. (2017). Review: astrocytes in Alzheimer’s disease and other age-associated dementias: a supporting player with a central role. Neuropathol. Appl. Neurobiol. 43, 281–298. doi: 10.1111/nan.12338
Geerligs, L., Renken, R. J., Saliasi, E., Maurits, N. M., and Lorist, M. M. (2015). A brain-wide study of age-related changes in functional connectivity. Cereb. Cortex 25, 1987–1999. doi: 10.1093/cercor/bhu012
Goedert, M., Spillantini, M. G., Jakes, R., Rutherford, D., and Crowther, R. A. (1989). Multiple isoforms of human microtubule-associated protein tau: sequences and localization in neurofibrillary tangles of Alzheimer’s disease. Neuron 3, 519–526. doi: 10.1016/0896-6273(89)90210-9
González-Reyes, R. E., Nava-Mesa, M. O., Vargas-Sánchez, K., Ariza-Salamanca, D., and Mora-Muñoz, L. (2017). Involvement of astrocytes in Alzheimer’s disease from a neuroinflammatory and oxidative stress perspective. Front. Mol. Neurosci. 10:427. doi: 10.3389/fnmol.2017.00427
Gottesman, R. F., Schneider, A. L., Zhou, Y., Coresh, J., Green, E., Gupta, N., et al. (2017). Association between midlife vascular risk factors and estimated brain amyloid deposition. JAMA 317, 1443–1450. doi: 10.1001/jama.2017.3090
Greenberg, S. M., Gurol, M. E., Rosand, J., and Smith, E. E. (2004). Amyloid angiopathy–related vascular cognitive impairment. Stroke 35, 2616–2619. doi: 10.1161/01.STR.0000143224.36527.44
Habib, N., McCabe, C., Medina, S., Varshavsky, M., Kitsberg, D., Dvir-Szternfeld, R., et al. (2020). Disease-associated astrocytes in Alzheimer’s disease and aging. Nat. Neurosci. 23, 701–706. doi: 10.1038/s41593-020-0624-8
Haass, C., and Selkoe, D. J. (2007). Soluble protein oligomers in neurodegeneration: lessons from the Alzheimer’s amyloid beta-peptide. Nat. Rev. Mol. Cell Biol. 8, 101–112. doi: 10.1038/nrm2101
Hanzel, C. E., Iulita, M. F., Eyjolfsdottir, H., Hjorth, E., Schultzberg, M., Eriksdotter, M., et al. (2014). Analysis of matrix metallo-proteases and the plasminogen system in mild cognitive impairment and Alzheimer’s disease cerebrospinal fluid. J. Alzheimers Dis. 40, 667–678. doi: 10.3233/JAD-132282
Hare, D. J., Rembach, A., and Roberts, B. R. (2016). The emerging role of metalloproteomics in Alzheimer’s disease research. Methods Mol. Biol. 1303, 379–389. doi: 10.1007/978-1-4939-2627-5_22
He, J.-T., Zhao, X., Xu, L., and Mao, C.-Y. (2020). Vascular risk factors and Alzheimer’s disease: blood-brain barrier disruption, metabolic syndromes, and molecular links. J. Alzheimers Dis. 73, 39–58. doi: 10.3233/JAD-190764
Hollingworth, P., Harold, D., Jones, L., Owen, M. J., and Williams, J. (2011). Alzheimer’s disease genetics: current knowledge and future challenges. Int. J. Geriatr. Psychiatry 26, 793–802. doi: 10.1002/gps.2628
Howard, R., Zubko, O., Bradley, R., Harper, E., Pank, L., O’brien, J., et al. (2020). Minocycline at 2 different dosages vs placebo for patients with mild Alzheimer disease: a randomized clinical trial. JAMA Neurol. 77, 164–174. doi: 10.1001/jamaneurol.2019.3762
Huang, C. C., Hsieh, W. J., Lee, P. L., Peng, L. N., Liu, L. K., Lee, W. J., et al. (2015). Age-related changes in resting-state networks of a large sample size of healthy elderly. CNS Neurosci. Ther. 21, 817–825. doi: 10.1111/cns.12396
Huang, W.-J., Zhang, X., and Chen, W.-W. (2016). Role of oxidative stress in Alzheimer’s disease. Biomed. Rep. 4, 519–522. doi: 10.3892/br.2016.630
Husna Ibrahim, N., Yahaya, M. F., Mohamed, W., Teoh, S. L., Hui, C. K., and Kumar, J. (2020). Pharmacotherapy of Alzheimer’s disease: seeking clarity in a time of uncertainty. Front. Pharmacol. 11:261. doi: 10.3389/fphar.2020.00261
Iturria-Medina, Y., Sotero, R. C., Toussaint, P. J., Mateos-Pérez, J. M., and Evans, A. C. (2016). Early role of vascular dysregulation on late-onset Alzheimer’s disease based on multifactorial data-driven analysis. Nat. Commun. 7, 1–14. doi: 10.1038/ncomms11934
Iadecola, C. (2004). Neurovascular regulation in the normal brain and in Alzheimer’s disease. Nat. Rev. Neurosci. 5, 347–360. doi: 10.1038/nrn1387
Jeynes, B., and Provias, J. (2006). The possible role of capillary cerebral amyloid angiopathy in Alzheimer lesion development: a regional comparison. Acta Neuropathol. 112, 417–427. doi: 10.1007/s00401-006-0099-z
Jeynes, B., and Provias, J. (2008). Evidence for altered LRP/RAGE expression in Alzheimer lesion pathogenesis. Curr. Alzheimer Res. 5, 432–437. doi: 10.2174/156720508785908937
Jeynes, B., and Provias, J. (2011). The case for blood–brain barrier dysfunction in the pathogenesis of Alzheimer’s disease. J. Neurosci. Res. 89, 22–28. doi: 10.1002/jnr.22527
Ji, Y., Urakami, K., Adachi, Y., Maeda, M., Isoe, K., and Nakashima, K. (1998). Apolipoprotein E polymorphism in patients with Alzheimer’s disease, vascular dementia and ischemic cerebrovascular disease. Dement. Geriatr. Cogn. Disord. 9, 243–245. doi: 10.1159/000017068
Kalpouzos, G., Chételat, G., Baron, J. C., Landeau, B., Mevel, K., Godeau, C., et al. (2009). Voxel-based mapping of brain gray matter volume and glucose metabolism profiles in normal aging. Neurobiol. Aging 30, 112–124. doi: 10.1016/j.neurobiolaging.2007.05.019
Kamal, H., Kamil, K., Nelson, T., Noor, A. L., Amiruddin, M. K., Singh, P., et al. (2021). Effects of traditional and convenient edible bird’s nest on spatial learning of male Sprague-Dawley rats. Sains Malays. 50, 3537–3545. doi: 10.17576/jsm-2021-5012-06
Kamal, H., Tan, G. C., Ibrahim, S. F., Shaikh, M. F., Mohamed, I. N., Mohamed, R. M. P., et al. (2020). Alcohol use disorder, neurodegeneration, Alzheimer’s and Parkinson’s disease: interplay between oxidative stress, neuroimmune response and excitotoxicity. Front. Cell. Neurosci. 14:282. doi: 10.3389/fncel.2020.00282
Kamil, K., Kumar, J., Yazid, M. D., and Idrus, R. B. H. (2018). Olive and its phenolic compound as the promising neuroprotective agent. Sains Malays. 47, 2811–2820. doi: 10.17576/jsm-2018-4711-24
Kamil, K., Yazid, M. D., Idrus, R. B. H., and Kumar, J. (2020). Hydroxytyrosol promotes proliferation of human Schwann cells: an in vitro study. Int. J. Environ. Res. Public Health 17:4404. doi: 10.3390/ijerph17124404
Kaminari, A., Giannakas, N., Tzinia, A., and Tsilibary, E. C. (2017). Overexpression of matrix metalloproteinase-9 (MMP-9) rescues insulin-mediated impairment in the 5XFAD model of Alzheimer’s disease. Sci. Rep. 7:683. doi: 10.1038/s41598-017-00794-5
Kisseleva, T., Bhattacharya, S., Braunstein, J., and Schindler, C. W. (2002). Signaling through the JAK/STAT pathway, recent advances and future challenges. Gene 285, 1–24. doi: 10.1016/s0378-1119(02)00398-0
Kivipelto, M., Helkala, E.-L., Laakso, M. P., Hänninen, T., Hallikainen, M., Alhainen, K., et al. (2002). Apolipoprotein E ε4 allele, elevated midlife total cholesterol level, and high midlife systolic blood pressure are independent risk factors for late-life Alzheimer disease. Ann. Intern. Med. 137, 149–155. doi: 10.7326/0003-4819-137-3-200208060-00006
Klavins, K., Koal, T., Dallmann, G., Marksteiner, J., Kemmler, G., and Humpel, C. (2015). The ratio of phosphatidylcholines to lysophosphatidylcholines in plasma differentiates healthy controls from patients with Alzheimer’s disease and mild cognitive impairment. Alzheimers Dement. (DADM) 1, 295–302. doi: 10.1016/j.dadm.2015.05.003
Koistinaho, M., Lin, S., Wu, X., Esterman, M., Koger, D., Hanson, J., et al. (2004). Apolipoprotein E promotes astrocyte colocalization and degradation of deposited amyloid-beta peptides. Nat. Med. 10, 719–726. doi: 10.1038/nm1058
Kosik, K. S. (2015). Personalized medicine for effective Alzheimer disease treatment. JAMA Neurol. 72, 497–498. doi: 10.1001/jamaneurol.2014.3445
Kovacs, G. G. (2016). Molecular pathological classification of neurodegenerative diseases: turning towards precision medicine. Int. J. Mol. Sci. 17:189. doi: 10.3390/ijms17020189
Langbaum, J. B., Chen, K., Launer, L. J., Fleisher, A. S., Lee, W., Liu, X., et al. (2012). Blood pressure is associated with higher brain amyloid burden and lower glucose metabolism in healthy late middle-age persons. Neurobiol. Aging 33, 827.e11–9. doi: 10.1016/j.neurobiolaging.2011.06.020
Levi-Montalcini, R. (1987). The nerve growth factor: thirty-five years later. EMBO J. 6, 1145–1154. doi: 10.1002/j.1460-2075.1987.tb02347.x
Levi-Montalcini, R., and Angeletti, P. U. (1968). Nerve growth factor. Physiol. Rev. 48, 534–569. doi: 10.1152/physrev.1968.48.3.534
Li, W., Liu, H., Yu, M., Zhang, X., Zhang, Y., Liu, H., et al. (2016). Folic acid alters methylation profile of JAK-STAT and long-term depression signaling pathways in Alzheimer’s disease models. Mol. Neurobiol. 53, 6548–6556. doi: 10.1007/s12035-015-9556-9
Liu, Z., Ke, L., Liu, H., Huang, W., and Hu, Z. (2014). Changes in topological organization of functional PET brain network with normal aging. PLoS One 9:e88690. doi: 10.1371/journal.pone.0088690
Lloret, A., Esteve, D., Monllor, P., Cervera-Ferri, A., and Lloret, A. (2019). The effectiveness of vitamin E treatment in Alzheimer’s disease. Int. J. Mol. Sci. 20:879. doi: 10.3390/ijms20040879
Long, Q.-H., Wu, Y.-G., He, L.-L., Ding, L., Tan, A.-H., Shi, H.-Y., et al. (2021). Suan-Zao-Ren Decoction ameliorates synaptic plasticity through inhibition of the Aβ deposition and JAK2/STAT3 signaling pathway in AD model of APP/PS1 transgenic mice. Chin. Med. 16:14. doi: 10.1186/s13020-021-00425-2
López González, I., Garcia-Esparcia, P., Llorens, F., and Ferrer, I. (2016). Genetic and transcriptomic profiles of inflammation in neurodegenerative diseases: alzheimer, parkinson, creutzfeldt-jakob and tauopathies. Int. J. Mol. Sci. 17:206. doi: 10.3390/ijms17020206
Luo, Q., and Chen, Y. (2016). Long noncoding RNAs and Alzheimer’s disease. Clin. Interv. Aging 11:867. doi: 10.2147/CIA.S107037
Mancuso, R., Van Den Daele, J., Fattorelli, N., Wolfs, L., Balusu, S., Burton, O., et al. (2019). Stem-cell-derived human microglia transplanted in mouse brain to study human disease. Nat. Neurosci. 22, 2111–2116. doi: 10.1038/s41593-019-0525-x
Mateo, I., Llorca, J., Infante, J., Rodríguez-Rodríguez, E., Fernández-Viadero, C., Pena, N., et al. (2007). Low serum VEGF levels are associated with Alzheimer’s disease. Acta Neurol. Scand. 116, 56–58. doi: 10.1111/j.1600-0404.2006.00775.x
Melchor, J. P., Pawlak, R., and Strickland, S. (2003). The tissue plasminogen activator-plasminogen proteolytic cascade accelerates amyloid-beta (Abeta) degradation and inhibits Abeta-induced neurodegeneration. J. Neurosci. 23, 8867–8871. doi: 10.1523/JNEUROSCI.23-26-08867.2003
Merlini, M., Wanner, D., and Nitsch, R. M. (2016). Tau pathology-dependent remodelling of cerebral arteries precedes Alzheimer’s disease-related microvascular cerebral amyloid angiopathy. Acta Neuropathol. 131, 737–752. doi: 10.1007/s00401-016-1560-2
Mitra, S., Behbahani, H., and Eriksdotter, M. (2019). Innovative therapy for Alzheimer’s disease-with focus on biodelivery of NGF. Front. Neurosci. 13:38. doi: 10.3389/fnins.2019.00038
Mizuno, S., Iijima, R., Ogishima, S., Kikuchi, M., Matsuoka, Y., Ghosh, S., et al. (2012). AlzPathway: a comprehensive map of signaling pathways of Alzheimer’s disease. BMC Syst. Biol. 6:52. doi: 10.1186/1752-0509-6-52
Murphy, S. (1993). Astrocytes: Pharmacology and Function. Cambridge, MA: Academic Press, doi: 10.1046/j.1471-4159.1994.63031186.x
Naj, A. C., Schellenberg, G. D., and Alzheimer’s Disease Genetics Consortium. (2017). Genomic variants, genes, and pathways of Alzheimer’s disease: an overview. Am. J. Med. Genet. B Neuropsychiatr. Genet. 174, 5–26. doi: 10.1002/ajmg.b.32499
Nevado-Holgado, A. J., Ribe, E., Thei, L., Furlong, L., Mayer, M.-A., Quan, J., et al. (2019). Genetic and real-world clinical data, combined with empirical validation, nominate jak-stat signaling as a target for Alzheimer’s disease therapeutic development. Cells 8:425. doi: 10.3390/cells8050425
Nishitsuji, K., Hosono, T., Nakamura, T., Bu, G., and Michikawa, M. (2011). Apolipoprotein E regulates the integrity of tight junctions in an isoform-dependent manner in an in vitro blood-brain barrier model. J. Biol. Chem. 286, 17536–17542. doi: 10.1074/jbc.M111.225532
Nuthikattu, S., Milenkovic, D., Rutledge, J. C., and Villablanca, A. C. (2020). Sex-dependent molecular mechanisms of lipotoxic injury in brain microvasculature: implications for dementia. Int. J. Mol. Sci. 21:8146. doi: 10.3390/ijms21218146
Nuthikattu, S., Milenkovic, D., Norman, J. E., Rutledge, J., and Villablanca, A. (2021). Inhibition of soluble epoxide hydrolase is protective against the multiomic effects of a high glycemic diet on brain microvascular inflammation and cognitive dysfunction. Nutrients 13:3913. doi: 10.3390/nu13113913
Nuzzo, D., Inguglia, L., Walters, J., Picone, P., and Di Carlo, M. (2017). A shotgun proteomics approach reveals a new toxic role for Alzheimer’s disease Aβ peptide: spliceosome impairment. J Proteome Res. 16, 1526–1541. doi: 10.1021/acs.jproteome.6b00925
Akinyemi, R. O., Mukaetova-Ladinska, E. B., Attems, J., Ihara, M., and Kalaria, R. N. (2013). Vascular risk factors and neurodegeneration in ageing related dementias: Alzheimer’s disease and vascular dementia. Curr. Alzheimer Res. 10, 642–653.
Oh, S. B., Byun, C. J., Yun, J.-H., Jo, D.-G., Carmeliet, P., Koh, J.-Y., et al. (2014). Tissue plasminogen activator arrests Alzheimer’s disease pathogenesis. Neurobiol. Aging 35, 511–519. doi: 10.1016/j.neurobiolaging.2013.09.020
Ornitz, D. M., and Itoh, N. (2015). The Fibroblast Growth Factor signaling pathway. Wiley Interdiscip. Rev. Dev. Biol. 4, 215–266. doi: 10.1002/wdev.176
Palop, J. J., and Mucke, L. (2010). Amyloid-beta-induced neuronal dysfunction in Alzheimer’s disease: from synapses toward neural networks. Nat. Neurosci. 13, 812–818. doi: 10.1038/nn.2583
Pardo, J. V., Lee, J. T., Sheikh, S. A., Surerus-Johnson, C., Shah, H., Munch, K. R., et al. (2007). Where the brain grows old: decline in anterior cingulate and medial prefrontal function with normal aging. Neuroimage 35, 1231–1237. doi: 10.1016/j.neuroimage.2006.12.044
Park, E., Cho, M., and Ki, C. S. (2009). Correct use of repeated measures analysis of variance. Kor. J. Lab. Med. 29, 1–9. doi: 10.3343/kjlm.2009.29.1.1
Park, K. W., Yoon, H. J., Kang, D.-Y., Kim, B. C., Kim, S., and Kim, J. W. (2012). Regional cerebral blood flow differences in patients with mild cognitive impairment between those who did and did not develop Alzheimer’s disease. Psychiatry Res. 203, 201–206. doi: 10.1016/j.pscychresns.2011.12.007
Park, S. Y., Kim, H. Y., Park, H. J., Shin, H. K., Hong, K. W., and Kim, C. D. (2016). Concurrent treatment with taxifolin and cilostazol on the lowering of β-amyloid accumulation and neurotoxicity via the suppression of P-JAK2/P-STAT3/NF-κB/BACE1 signaling pathways. PLoS One 11:e0168286. doi: 10.1371/journal.pone.0168286
Pascual, O., Casper, K. B., Kubera, C., Zhang, J., Revilla-Sanchez, R., and Sul, J.-Y. (2005). Astrocytic purinergic signaling coordinates synaptic networks. Science 310, 113–116. doi: 10.1126/science.1116916
Pentz, R., Iulita, M. F., Ducatenzeiler, A., Bennett, D. A., and Cuello, A. C. (2020). The human brain NGF metabolic pathway is impaired in the pre-clinical and clinical continuum of Alzheimers disease. Mol. Psychiatry 26, 6023–6037. doi: 10.1038/s41380-020-0797-2
Pentz, R., Iulita, M. F., Mikutra-cencora, M., Ducatenzeiler, A., Bennett, D. A., and Cuello, A. C. (2021). Neurobiology of Disease A new role for matrix metalloproteinase-3 in the NGF metabolic pathway: proteolysis of mature NGF and sex-specific differences in the continuum of Alzheimer’s pathology. Neurobiol. Dis. 148:105150. doi: 10.1016/j.nbd.2020.105150
Perneczky, R., and Guo, L. H. (2016). Plasma proteomics biomarkers in Alzheimer’s disease: latest advances and challenges. Methods Mol. Biol. 1303, 521–529. doi: 10.1007/978-1-4939-2627-5_32
Pichler, S., Gu, W., Hartl, D., Gasparoni, G., Leidinger, P., Keller, A., et al. (2017). The miRNome of Alzheimer’s disease: consistent downregulation of the miR-132/212 cluster. Neurobiol. Aging 50, 167.e1–167.e10. doi: 10.1016/j.neurobiolaging.2016.09.019
Prom-In, S., Kaewsrichan, J., Wangpradit, N., Hui, C. K., Yahaya, M. F., Kamisah, Y., et al. (2020). Abelmoschus esculentus (L.) moench’s peel powder improves high-fat-diet-induced cognitive impairment in C57BL/6J mice. Int. J. Environ. Res. Public Health 17:5513. doi: 10.3390/ijerph17155513
Provias, J., and Jeynes, B. (2014). The role of the blood-brain barrier in the pathogenesis of senile plaques in Alzheimer’s disease. Int. J. Alzheimers Dis. 2014:191863.
Rabin, J. S., Schultz, A. P., Hedden, T., Viswanathan, A., Marshall, G. A., Kilpatrick, E., et al. (2018). Interactive associations of vascular risk and β-amyloid burden with cognitive decline in clinically normal elderly individuals: findings from the Harvard Aging Brain Study. JAMA Neurol. 75, 1124–1131. doi: 10.1001/jamaneurol.2018.1123
Rawlings, J. S., Rosler, K. M., and Harrison, D. A. (2004). The JAK/STAT signaling pathway. J. Cell Sci. 117, 1281–1283. doi: 10.1242/jcs.00963
Regev, A., Teichmann, S. A., Lander, E. S., Amit, I., Benoist, C., Birney, E., et al. (2017). Science forum: the human cell atlas. eLife 6:e27041. doi: 10.7554/eLife.27041
Reinvang, I., Espeseth, T., and Westlye, L. T. (2013). APOE-related biomarker profiles in non-pathological aging and early phases of Alzheimer’s disease. Neurosci. Biobehav. Rev. 37, 1322–1335. doi: 10.1016/j.neubiorev.2013.05.006
Rocher, A. B., Chapon, F., Blaizot, X., Baron, J. C., and Chavoix, C. (2003). Resting-state brain glucose utilization as measured by PET is directly related to regional synaptophysin levels: a study in baboons. Neuroimage 20, 1894–1898. doi: 10.1016/j.neuroimage.2003.07.002
Romero-Miguel, D., Lamanna-Rama, N., Casquero-Veiga, M., Gómez-Rangel, V., Desco, M., and Soto-Montenegro, M. L. (2021). Minocycline in neurodegenerative and psychiatric diseases: an update. Eur. J. Neurol. 28, 1056–1081. doi: 10.1111/ene.14642
Ruganzu, J. B., Zheng, Q., Wu, X., He, Y., Peng, X., Jin, H., et al. (2021). TREM2 overexpression rescues cognitive deficits in APP/PS1 transgenic mice by reducing neuroinflammation via the JAK/STAT/SOCS signaling pathway. Exp. Neurol. 336:113506. doi: 10.1016/j.expneurol.2020.113506
Samadian, M., Gholipour, M., Hajiesmaeili, M., Taheri, M., and Ghafouri-Fard, S. (2021). The Eminent Role of microRNAs in the pathogenesis of Alzheimer’s disease. Front. Aging Neurosci. 13:641080. doi: 10.3389/fnagi.2021.641080
Schmidt, A. M., Sahagan, B., Nelson, R. B., Selmer, J., Rothlein, R., and Bell, J. M. (2009). The role of RAGE in amyloid-beta peptide-mediated pathology in Alzheimer’s disease. Curr. Opin. Invest. Drugs (London, England: 2000) 10, 672–680.
Schuff, N., Matsumoto, S., Kmiecik, J., Studholme, C., Du, A., Ezekiel, F., et al. (2009). Cerebral blood flow in ischemic vascular dementia and Alzheimer’s disease, measured by arterial spin-labeling magnetic resonance imaging. Alzheimers Dement. 5, 454–462. doi: 10.1016/j.jalz.2009.04.1233
Schwab, M. E., Otten, U., Agid, Y., and Thoenen, H. (1979). Nerve growth factor (NGF) in the rat CNS: absence of specific retrograde axonal transport and tyrosine hydroxylase induction in locus coeruleus and substantia nigra. Brain Res. 168, 473–483. doi: 10.1016/0006-8993(79)90303-2
Seiler, M., and Schwab, M. E. (1984). Specific retrograde transport of nerve growth factor (NGF) from neocortex to nucleus basalis in the rat. Brain Res. 300, 33–39. doi: 10.1016/0006-8993(84)91338-6
Selkoe, D. J., and Hardy, J. (2016). The amyloid hypothesis of Alzheimer’s disease at 25 years. EMBO Mol. Med. 8, 595–608. doi: 10.15252/emmm.201606210
Sharma, V., Kaur, A., and Singh, T. G. (2020). Counteracting role of nuclear factor erythroid 2-related factor 2 pathway in Alzheimer’s disease. Biomed. Pharmacother. 129:110373. doi: 10.1016/j.biopha.2020.110373
Shen, X. N., Niu, L. D., Wang, Y. J., Cao, X. P., Liu, Q., Tan, L., et al. (2019). Inflammatory markers in Alzheimer’s disease and mild cognitive impairment: a meta-analysis and systematic review of 170 studies. J. Neurol. Neurosurg. Psychiatry 90, 590–598. doi: 10.1136/jnnp-2018-319148
Simon, A. R., Rai, U., Fanburg, B. L., and Cochran, B. H. (1998). Activation of the JAK-STAT pathway by reactive oxygen species. Am. J. Physiol. 275, C1640–C1652. doi: 10.1152/ajpcell.1998.275.6.C1640
Simpson, J. E., Ince, P. G., Lace, G., Forster, G., Shaw, P. J., Matthews, F., et al. (2010). Astrocyte phenotype in relation to Alzheimer-type pathology in the ageing brain. Neurobiol. Aging 31, 578–590. doi: 10.1016/j.neurobiolaging.2008.05.015
Singh, S. K., Castellani, R., and Perry, G. (2016). “Oxidative stress and Alzheimer’s disease,” in Inflammation, Aging, and Oxidative Stress, eds S. Bondy and A. Campbell (Cham: Springer), 189–198. doi: 10.1007/978-3-319-33486-8_10
Slooter, A., Cruts, M., Ott, A., Bots, M., Witteman, J., Hofman, A., et al. (1999). The effect of APOE on dementia is not through atherosclerosis: the Rotterdam Study. Neurology 53, 1593–1593. doi: 10.1212/WNL.53.7.1593
Solomon, A., Kivipelto, M., Wolozin, B., Zhou, J., and Whitmer, R. A. (2009). Midlife serum cholesterol and increased risk of Alzheimer’s and vascular dementia three decades later. Dement. Geriatr. Cogn. Disord. 28, 75–80. doi: 10.1159/000231980
Soreq, L., Rose, J., Soreq, E., Hardy, J., Trabzuni, D., Cookson, M. R., et al. (2017). Major shifts in glial regional identity are a transcriptional hallmark of human brain aging. Cell Rep. 18, 557–570. doi: 10.1016/j.celrep.2016.12.011
Sperling, R. A., Laviolette, P. S., O’Keefe, K., O’Brien, J., Rentz, D. M., Pihlajamaki, M., et al. (2009). Amyloid deposition is associated with impaired default network function in older persons without dementia. Neuron 63, 178–188. doi: 10.1016/j.neuron.2009.07.003
Sweeney, M. D., Montagne, A., Sagare, A. P., Nation, D. A., Schneider, L. S., Chui, H. C., et al. (2019). Vascular dysfunction—the disregarded partner of Alzheimer’s disease. Alzheimers Dement. 15, 158–167. doi: 10.1016/j.jalz.2018.07.222
Takahashi, W., Takagi, S., Ide, M., Shohtsu, A., and Shinohara, Y. (2000). Reduced cerebral glucose metabolism in subjects with incidental hyperintensities on magnetic resonance imaging. J. Neurol. Sci. 176, 21–27. doi: 10.1016/S0022-510X(00)00286-0
Tang, H., Mao, X., Xie, L., Greenberg, D. A., and Jin, K. (2013). Expression level of vascular endothelial growth factor in hippocampus is associated with cognitive impairment in patients with Alzheimer’s disease. Neurobiol. Aging 34, 1412–1415. doi: 10.1016/j.neurobiolaging.2012.10.029
Teri, L., McCurry, S. M., and Logsdon, R. G. (1997). Memory, thinking, and aging. What we know about what we know. West. J. Med. 167:269.
Terni, B., and Ferrer, I. (2015). Abnormal expression and distribution of MMP2 at initial stages of Alzheimer’s disease-related pathology. J. Alzheimers Dis. 46, 461–469. doi: 10.3233/JAD-142460
Toledo, J. B., Arnold, M., Kastenmüller, G., Chang, R., Baillie, R. A., Han, X., et al. (2017). Metabolic network failures in Alzheimer’s disease-A biochemical road map. Alzheimers Dement. 13, 965–984. doi: 10.1016/j.jalz.2017.01.020
Tosto, G., and Reitz, C. (2016). Use of “omics” technologies to dissect neurologic disease. Handb. Clin. Neurol. 138, 91–106. doi: 10.1016/B978-0-12-802973-2.00006-9
Tripathi, T., and Kalita, P. (2019). Synergistic effect of amyloid-β and tau disrupts neural circuits. ACS Chem. Neurosci. 10, 1129–1130. doi: 10.1021/acschemneuro.9b00037
Uddin, M. S., and Kabir, M. T. (2019). “Oxidative stress in Alzheimer’s disease: molecular hallmarks of underlying vulnerability,” in Biological, Diagnostic and Therapeutic Advances in Alzheimer’s Disease, eds G. Ashraf and A. Alexiou (Berlin: Springer), 91–115. doi: 10.1007/978-981-13-9636-6_5
Vemuri, P., Lesnick, T. G., Przybelski, S. A., Knopman, D. S., Lowe, V. J., Graff-Radford, J., et al. (2017). Age, vascular health, and Alzheimer disease biomarkers in an elderly sample. Ann. Neurol. 82, 706–718. doi: 10.1002/ana.25071
Vermunt, L., Sikkes, S. A. M., van den Hout, A., Handels, R., Bos, I., van der Flier, W. M., et al. (2019). Duration of preclinical, prodromal, and dementia stages of Alzheimer’s disease in relation to age, sex, and APOE genotype. Alzheimers Dement. 15, 888–898. doi: 10.1016/j.jalz.2019.04.001
Vincent, V. A., Tilders, F. J., and Van Dam, A. M. (1997). Inhibition of endotoxin-induced nitric oxide synthase production in microglial cells by the presence of astroglial cells: a role for transforming growth factor beta. Glia 19, 190–198.
Wegiel, J., Wang, K. C., Tarnawski, M., and Lach, B. (2000). Microglia cells are the driving force in fibrillar plaque formation, whereas astrocytes are a leading factor in plague degradation. Acta Neuropathol. 100, 356–364. doi: 10.1007/s004010000199
Wesson, D. W., Nixon, R. A., Levy, E., and Wilson, D. A. (2011). Mechanisms of neural and behavioral dysfunction in Alzheimer’s disease. Mol. Neurobiol. 43, 163–179. doi: 10.1007/s12035-011-8177-1
Wilcock, D. M., Morgan, D., Gordon, M. N., Taylor, T. L., Ridnour, L. A., Wink, D. A., et al. (2011). Activation of matrix metalloproteinases following anti-Aβ immunotherapy; implications for microhemorrhage occurrence. J. Neuroinflammation 8:115. doi: 10.1186/1742-2094-8-115
Wyss-Coray, T., Loike, J. D., Brionne, T. C., Lu, E., Anankov, R., Yan, F., et al. (2003). Adult mouse astrocytes degrade amyloid-beta in vitro and in situ. Nat. Med. 9, 453–457. doi: 10.1038/nm838
Xekardaki, A., Rodriguez, C., Montandon, M.-L., Toma, S., Tombeur, E., Herrmann, F. R., et al. (2015). Arterial spin labeling may contribute to the prediction of cognitive deterioration in healthy elderly individuals. Radiology 274, 490–499. doi: 10.1148/radiol.14140680
Xie, Y., Su, N., Yang, J., Tan, Q., Huang, S., Jin, M., et al. (2020). FGF/FGFR signaling in health and disease. Signal. Transduct. Target. Ther. 5:181. doi: 10.1038/s41392-020-00222-7
Yamaji, S., Ishii, K., Sasaki, M., and Imamura, T. (1997). Changes in cerebral blood flow and oxygen metabolism related to magnetic resonance imaging white matter hyperintensities in Alzheimer’s disease. J. Nucl. Med. 38:1471.
Yugi, K., Kubota, H., Hatano, A., and Kuroda, S. (2016). Trans-omics: how to reconstruct biochemical networks across multiple ‘omic’layers. Trends Biotechnol. 34, 276–290. doi: 10.1016/j.tibtech.2015.12.013
Zhang, Z.-H., Yu, L.-J., Hui, X.-C., Wu, Z.-Z., Yin, K.-L., Yang, H., et al. (2014). Hydroxy-safflor yellow A attenuates Aβ1–42-induced inflammation by modulating the JAK2/STAT3/NF-κB pathway. Brain Res. 1563, 72–80. doi: 10.1016/j.brainres.2014.03.036
Zhao, T., Cao, M., Niu, H., Zuo, X. N., Evans, A., He, Y., et al. (2015). Age-related changes in the topological organization of the white matter structural connectome across the human lifespan. Hum. Brain Mapp. 36, 3777–3792. doi: 10.1002/hbm.22877
Glosarry
AD, Alzheimer’s disease; COVID-19, corona virus disease-2019; HIV, human immunodeficiency virus; APP, amyloid precursor protein; Aβ, amyloid beta; MAPT, microtubule-associated protein tau; ApoE, apolipoprotein E; NFT, neurofibrillary tangles; Wnt, Wingless-related integration site; MAPK, mitogen-activated protein kinase;NFTs, neurofibrillary tangles; NMDARs, N-methyl-d-aspartic acid receptors; NGF, Nerve growth factor; PNS, peripheral nervous system; CNS, central nervous system; BFCN, basal forebrain cholinergic neurons; proNGF, precursor nerve growth factor; mNGF, mature nerve growth factor; TrkA, tropomyosin-related kinase A; p75NTR, p75 neurotrophin receptor; tPA, tissue plasminogen activator; uPA, urokinase plasminogen activator; PAI-1, plasminogen activator inhibitor 1; MMPs, matrix metalloproteinases; MMP-9, matrix metalloproteinases; TIMP-1, tissue inhibitor of metalloproteinases 1; proMMP-9, precursor matrix metalloproteinases-9; MMP-3, matrix metalloproteinases-3; MMP-1, matrix metalloproteinases-1; MMP-2, matrix metalloproteinases-2; MMP-13, matrix metalloproteinases-13; MT1-MMP, Membrane type 1 matrix metalloproteinase; JAK/STAT, Janus kinase/signal transducer and activator of the transcription; JAK1, Janus kinase 1; JAK2, Janus kinase 2; JAK3, Janus kinase 3; Tyk2, Tyrosine kinase 2; STAT1, signal transducer and activator of the transcription 1; STAT2, signal transducer and activator of the transcription 2; STAT3, signal transducer and activator of the transcription 3; STAT4, signal transducer and activator of the transcription 4; STAT5A, signal transducer and activator of the transcription5A; STAT5B, signal transducer and activator of the transcription 5B; and STAT6, signal transducer and activator of the transcription 6; Nrf2, nuclear erythroid 2-related factor 2; TREM2, triggering receptor expressed on myeloid cells 2; Ntrk3 neurotrophic tyrosine kinase receptor type 3; Cox17, Cytochrome C Oxidase Copper Chaperone; Grid2ip, Grid2 Interacting Protein; TGF-β, transforming growth factor-beta; GFP, glial fibrillary acidic protein; EAATs, excitatory amino acid transporters; ALDH1L1, aldehyde dehydrogenase 1 family member L1; FGFs, Fibroblast growth factors; FGFRs, Fibroblast growth factor receptors; AKT, Protein kinase B; P13K, phosphatidylinositol 3-kinases; GRB2, Growth factor receptor-bound protein 2; GAB1; GRB2 Associated Binding Protein 1; CSF, cerebrospinal fluid; FGF7, Fibroblast Growth Factor 7; mir-107, MicroRNA-107.
Keywords: Alzheimer, diagnose, therapeutic, JAK, NGF, omic, dementia, vascular
Citation: Abubakar MB, Sanusi KO, Ugusman A, Mohamed W, Kamal H, Ibrahim NH, Khoo CS and Kumar J (2022) Alzheimer’s Disease: An Update and Insights Into Pathophysiology. Front. Aging Neurosci. 14:742408. doi: 10.3389/fnagi.2022.742408
Received: 16 July 2021; Accepted: 25 February 2022;
Published: 30 March 2022.
Edited by:
Maria Grazia Giovannini, University of Florence, ItalyReviewed by:
Vijay Karkal Hegde, Texas Tech University, United StatesTimothy Michael Ellmore, City College of New York (CUNY), United States
Idris Long, University of Science Malaysia, Malaysia
Igor Nikolayevich Iezhitsa, International Medical University, Malaysia
Copyright © 2022 Abubakar, Sanusi, Ugusman, Mohamed, Kamal, Ibrahim, Khoo and Kumar. This is an open-access article distributed under the terms of the Creative Commons Attribution License (CC BY). The use, distribution or reproduction in other forums is permitted, provided the original author(s) and the copyright owner(s) are credited and that the original publication in this journal is cited, in accordance with accepted academic practice. No use, distribution or reproduction is permitted which does not comply with these terms.
*Correspondence: Jaya Kumar, jayakumar@ukm.edu.my