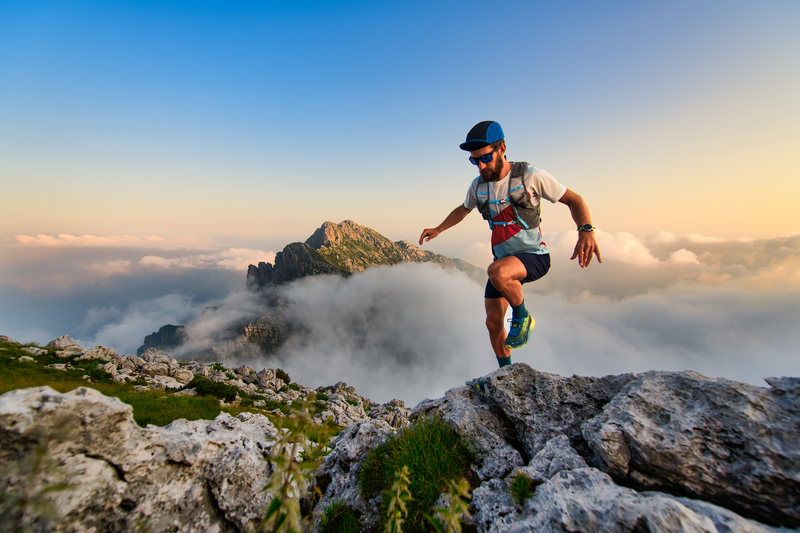
94% of researchers rate our articles as excellent or good
Learn more about the work of our research integrity team to safeguard the quality of each article we publish.
Find out more
ORIGINAL RESEARCH article
Front. Aging Neurosci. , 06 January 2023
Sec. Alzheimer's Disease and Related Dementias
Volume 14 - 2022 | https://doi.org/10.3389/fnagi.2022.1064667
This article is part of the Research Topic Advances in Connectome-Wide Association Studies (CWAS) Along the Neurodegeneration Trajectory View all 6 articles
Background: Alzheimer’s disease (AD) is one of most prevalent neurodegenerative diseases worldwide and characterized by cognitive decline and brain structure atrophy. While studies have reported substantial grey matter atrophy related to progression of AD, it remains unclear about brain regions with progressive grey matter atrophy, covariance connectivity, and the associations with cognitive decline in AD patients.
Objective: This study aims to investigate the grey matter atrophy, structural covariance connectivity abnormalities, and the correlations between grey matter atrophy and cognitive decline during AD progression.
Materials: We analyzed neuroimaging data of healthy controls (HC, n = 45) and AD patients (n = 40) at baseline (AD-T1) and one-year follow-up (AD-T2) obtained from the Alzheimer’s Disease Neuroimaging Initiative. We investigated AD-related progressive changes of grey matter volume, covariance connectivity, and the clinical relevance to further understand the pathological progression of AD.
Results: The results showed clear patterns of grey matter atrophy in inferior frontal gyrus, prefrontal cortex, lateral temporal gyrus, posterior cingulate cortex, insula, hippocampus, caudate, and thalamus in AD patients. There was significant atrophy in bilateral superior temporal gyrus (STG) and left caudate in AD patients over a one-year period, and the grey matter volume decrease in right STG and left caudate was correlated with cognitive decline. Additionally, we found reduced structural covariance connectivity between right STG and left caudate in AD patients. Using AD-related grey matter atrophy as features, there was high discrimination accuracy of AD patients from HC, and AD patients at different time points.
Alzheimer’s disease (AD) is one of most prevalent neurodegenerative diseases and characterized by brain atrophy in a variety of regions as revealed by non-invasive magnetic resonance imaging (MRI) technique. These regions included bilateral hippocampus, temporal lobes, superior and lateral temporal gyrus, parietal lobe, anterior and posterior cingulate cortices, thalamus, entorhinal cortex, and cerebellum (Baron et al., 2001; Busatto et al., 2003; Guo et al., 2010; Chapleau et al., 2016; Dicks et al., 2019; Kang et al., 2019; Van De Mortel et al., 2021). While early studies mainly focused on one clinical group of AD patients (Baron et al., 2001; Busatto et al., 2003; Guo et al., 2010), a growing number of recent explorations investigated brain atrophy in patients at different stages of cognitive decline (Thomann et al., 2008; Toniolo et al., 2018; Dicks et al., 2019; Van De Mortel et al., 2021). For example, a study including early and late mild cognitive impairment (EMCI, LMCI) and AD patients reported widespread and progressive grey matter atrophy, especially in bilateral temporal areas, hippocampus, and thalamus, in AD patients as compared to cognitively normal individuals (Van De Mortel et al., 2021). Moreover, a few longitudinal studies have shown progressive grey matter atrophy in AD patients over a one-year period (Anderson et al., 2012; Dicks et al., 2019). However, still little is known regarding the brain regions with progressive grey matter atrophy and the associations between the grey matter atrophy and rapid cognitive decline in AD patients.
Notably, a growing number of studies have also characterized the synchronous brain atrophy in ASD patients by employing a structural covariance network (SCN) approach (Hafkemeijer et al., 2016; Montembeault et al., 2016; Chang et al., 2018, 2021; Li et al., 2019). The SCN approach is essentially a correlation analysis for cross-sectional morphometric imaging data, which measures the synchronized grey matter atrophy undergoing common pathological processes between brain regions (Seeley et al., 2009; Alexander-Bloch et al., 2013; Evans, 2013). By investigating the underlying structural abnormalities from a network perspective, the SCN approach may provide additional valuable information to the pathology beyond the traditional voxel-based approach that focuses on local grey matter atrophy (Evans, 2013). It has been shown that there was reduced structural covariance connectivity between regions within the default mode network (DMN) in AD patients as compared to control adults (Spreng and Turner, 2013; Montembeault et al., 2016). Research has also investigated the SCN changes in different neuropathological stages of AD. It reported increased structural covariance connectivity at the early stage and decreased structural covariance connectivity at the late stage for both the DMN and the salience network, but continuously increased structural covariance connectivity for the executive control network along the AD neuropathological progression (Li et al., 2019). So far, however, no studies have investigated the progressive changes of SCN associated with the grey matter atrophy in AD patients.
Recently, a causal SCN approach, i.e., causal relationships between morphometric features of different brain regions, has been proposed to better understand the underlying structural abnormalities related to AD progression, as it is likely that the atrophy in some brain regions has a causal influence on the atrophy of other regions (Zhang et al., 2017). In a recent study, causal SCN was evaluated in MCI and AD patients using the Granger causality analysis, and this study reported the atrophy in hippocampus, thalamus, and precuneus/posterior cingulate cortex had causal effects on the atrophy of other regions during AD progression (Qing et al., 2021). These findings demonstrate causal relationships among the brain atrophy in different regions related to the progression of AD. However, the causal SCN has not been examined longitudinally in AD patients. Thus, it is still unknown whether and how earlier brain atrophy patterns impact the progressive changes of grey matter atrophy in AD patients over time.
To fill in these gaps, in the present study, we first investigated the alternations in grey matter volume (GMV) in a cohort of AD patients at different time points as compared to healthy controls (HC). Here, to better detect AD-related GMV changes, we used a relatively new approach, i.e., grey matter based spatial statistics (Ball et al., 2013; Parvathaneni et al., 2017; Ouyang et al., 2019), which measures the GMV at the core of the cortical plate and thus alleviates partial volume effect and minimizes individual variability (Ouyang et al., 2019). Then, we examined the overlapped decrease and continuing decrease of GMV in AD patients as compared to HC, and progressive decrease of GMV in AD patients between different time points. We expected reduced GMV in AD patients at both time points as compared to HC and progressive decrease in GMV during AD progression. We tested whether the grey matter atrophy in AD patients would be associated with cognitive decline over a one-year period. To further understand the brain morphological abnormalities related to the AD pathological progression, we examined the SCN and also causal SCN between the regions showing grey matter atrophy, and hypothesized that there would be reduced SCN in AD patients. Finally, we explored whether these AD-related grey matter changes could serve as biomarkers to distinguish AD patients at different stages and HC using a linear support vector machine (SVM) approach.
The data included in this study were obtained from the Alzheimer’s Disease Neuroimaging Initiative (ADNI) database.1 The ADNI was launched in 2003 as a public-private partnership, led by Principal Investigator Michael W. Weiner, MD. The primary goal of ADNI has been to test whether serial MRI, positron emission tomography (PET), other biological markers, and clinical and neuropsychological assessment can be combined to measure the progression of MCI and early AD. For more details, see www.adni-info.org and previous publications (Weiner et al., 2010, 2017; Aisen et al., 2015).
Specifically, we included 40 AD subjects (22 males; mean age at baseline: 74.66 ± 7.23 years, range 56–88 years; mean age at one-year follow-up: 75.73 ± 7.25 years, range 57.0–89.3 years) and 45 HC (27 males; mean age 74.78 ± 4.95 years, range 66.6–84.8 years) in the present study. All the participants had demographic information (i.e., age, gender), clinical, and cognitive measures including Clinical Dementia Rating (CDR) (Morris, 1993) and Mini Mental State Examination (MMSE) (Folstein et al., 1975). All the AD patients at baseline (AD-T1, n = 40) and the majority of AD patients at one-year follow-up (AD-T2, n = 38) and HC (n = 43) completed the Montreal Cognitive Assessment (MoCA; Nasreddine et al., 2005). For the detailed demographics and clinical scores of all subjects, see Table 1.
Table 1. Demographic characteristics and clinical scores of AD patients at different time points (AD-T1, AD-T2) and healthy controls (HC).
High-resolution 3D structural images were acquired from 3 T SIEMENS scanners with a T1-weighted, magnetization prepared rapid gradient-echo (MPRAGE) sequence (TR/TI = 2300/900 ms, TE = 2.98 ms, FOV = 240 mm, 1 × 1 × 1.2 mm3, flip angle = 9°, Slice Thickness = 1.2 mm). Raw Digital Imaging and Communications in Medicine (DICOM) MRI scans were downloaded from the public ADNI site,2 reviewed for quality, and automatically corrected for spatial distortion caused by gradient nonlinearity and B1 field inhomogeneity.
Voxel-based morphometry (VBM) was performed using a conventional method with Statistical Parametric Mapping (SPM8, http://www.fil.ion.ucl.ac.uk), running on Matlab 2014b (MathWorks, Natick, MA, United States). First, MR images were visually inspected and then segmented into grey matter, white matter, and cerebrospinal fluid using SPM8’s standard unified segmentation module with the default tissue probability maps (Ashburner and Friston, 2005). Second, a study-specific grey matter template was derived from the entire image dataset using the diffeomorphic anatomical registration using lie algebra (DARTEL) (Ashburner, 2007). Third, after an initial affine registration of the DARTEL template to the grey matter tissue probability map, nonlinear warping of the segmented images was then performed to match the MNI space. Finally, for the intensity preservation of the grey matter concentration, normalized grey matter images were modulated with Jacobian determinants from the normalization procedure.
The GMV was measured on the cortical skeleton, that is, the center of the cortical plate, which is supposed to alleviate partial volume effects and enhance the measurement accuracy (Ouyang et al., 2019). To obtain the gray matter skeleton, the mean grey matter image was calculated across all the subjects including AD patients (both AD-T1 and AD-T2) and HC. Next, the cortical skeleton was created by applying the skeletonization function (i.e., “tbss_skeleton”) in TBSS of FSL3 to the averaged grey matter map. In this way, GMV was measured at the core cortical regions.
First, we examined the global changes in total GMV on the cortical skeleton between HC, AD-T1, and AD-T2 groups. The total GMV was extracted for each group separately, and then two-tailed two-sample t-tests (HC vs. AD-T1, HC vs. AD-T2) and two-tailed paired t-test (AD-T1 vs. AD-T2) were conducted to test the group differences.
Then, we examined the group differences in grey matter skeleton at the voxel-level between AD-T1 and HC and between AD-T2 and HC by conducting two-tailed two-sample t-tests. We further identified the overlapped decrease areas that showed significant differences in the comparison of both AD-T1 vs. HC and AD-T2 vs. HC, and continuing decrease areas that showed significant differences between the comparison of AD-T1 vs. HC and AD-T2 vs. HC. The resulting maps were corrected for multiple comparisons using the FDR method with p < 0.05.
We tested the progressive GMV changes in AD patients between two time points (i.e., AD-T2 vs. AD-T1) using two-tailed paired t-test. The significant clusters were corrected for multiple comparisons using the FDR method with p < 0.05.
Subsequently, we examined the clinical relevance of significant GMV changes between AD-T2 and AD-T1. Specifically, we extracted the mean GMV values within each region showing significant changes and calculated the change GMV values (AD-T2 - AD-T1) for each region which were then correlated with changes in the cognitive ability as measured by the MoCA (i.e., change scores of the MoCA between AD-T2 and AD-T1).
To examine whether and how regions showing significant grey matter atrophy were co-varied with each other, we calculated the structural covariance connectivity between these brain regions. First, overlapped decrease areas in AD patients at both time points vs. HC, continuing decrease areas from comparisons between AD-T2 vs. HC and AD-T1 vs. HC, and regions showing progressive decrease between AD-T2 and AD-T1, were selected as seed regions for covariance connectivity analysis. Next, mean GMV values within each seed region was calculated. Then, the correlations between mean GMV values of any two seed regions were computed using Pearson’s correlation coefficient. To identify abnormalities and progressive changes in the structural covariance connectivity in AD patients, we used a nonparametric permutation test to test the statistical significance of the between-group differences. We performed permutation tests with 10,000 permutations and recorded all of the differences between the two groups. Finally, we reported whether the between-group difference in the real structural covariance connectivity was out of 95% (two-tailed) of the supposed between-group differences.
In an exploratory analysis, we investigated the causal SCN using the Granger causal analysis. Specifically, we examined the causal effects of GMV within overlapped decrease areas in AD-T1 on GMV changes in AD-T2 (including continuing decrease areas and regions showing progressive changes). Since there was no clear chronological information for all the enrolled subjects, we performed the permutation test with 10,000 permutations to test the stability of the casual effects and recorded all of the causal correlations between GMV of the overlapped decrease areas in AD-T1 and GMV of continuing decrease areas and regions with progressive changes in AD-T2. The distribution and mean causal correlation values from 10,000 permutations were depicted and the significant level (set as p < 0.05) was determined by comparing all the permutated causal coefficients with zeros.
We applied a linear SVM approach to classify AD patients and HC using AD-based grey matter atrophy as features. Specifically, we calculated the mean GMV values within (a) overlapped decrease areas in AD patients at both time points vs. HC, (b) continuing decrease areas from comparisons between AD-T2 vs. HC and AD-T1 vs. HC, and (c) regions showing progressive decrease between AD-T2 and AD-T1. The mean GMV values of these regions were features for the classification analysis. And for the SVM analysis, we used the Matlab version of LIBSVM (Chang and Lin, 2011), a library for support vector machines, and the leave-one-out cross-validation (LOOCV) technique for SVM classifier validation. Given labeled training data, the SVM algorithm yielded an optimal hyperplane, which was applied to the classification of AD-T1 from HC, AD-T2 from HC, and AD-T1 from AD-T2, separately. The trained SVM’s classification performance was assessed using the following measures: accuracy, sensitivity, and specificity.
There were significant differences in total GMV between HC, AD-T1, and AD-T2, i.e., the greatest GMV in HC, following by AD-T1 and then AD-T2 (Figure 1). The results were corrected for multiple comparisons with the FDR method at p < 0.05.
Figure 1. Reduced total grey matter volume (GMV) in AD patients. There were significant differences in total GMV between HC and AD-T1, between HC and AD-T2, and between AD-T1 and AD-T2. *p < 0.05, ***p < 0.001.
As compared to HC, there were a wide range of regions showing significantly decreased GMV in AD patients at both time points, including inferior frontal gyrus, prefrontal cortex, lateral temporal gyrus, posterior cingulate cortex, insula, hippocampus, caudate, and thalamus (Figure 2A). These regions were identified as overlapped decrease areas, and the averaged GMV within these areas showed significant decrease between HC and AD-T1, between HC and AD-T2, and between AD-T1 and AD-T2 (Figure 2B). We further identified continuing decrease areas by comparing the differences between AD-T2 vs. HC and AD-T1 vs. HC, which included posterior cingulate cortex, ventral precentral gyrus, and lateral temporal gyrus; there was significant decrease in AD patients at both time points vs. HC and in AD-T2 vs. AD-T1 (Figure 2C).
Figure 2. Brain areas showing substantial atrophy in grey matter volume in AD patients. (A) There was reduced grey matter volume in inferior frontal gyrus, prefrontal cortex, lateral temporal gyrus, posterior cingulate cortex, insula, hippocampus, caudate, and thalamus in both AD-T1 and AD-T2 as compared to HC. (B) The areas that showed grey matter atrophy in both AD-T1 vs. HC and AD-T2 vs. HC were identified as overlapped decrease areas, and the mean grey matter volume values within these areas are significantly different between HC and AD-T1, between HC and AD-T2, and between AD-T1 and AD-T2. (C) The regions that showed differences between AD-T2 vs. HC and AD-T1 vs. HC were identified as continuing decrease areas, and the mean grey matter volume values within these regions are significantly different between HC and AD-T1, between HC and AD-T2, and between AD-T1 and AD-T2. All brain maps were corrected with the FDR method (p < 0.05, cluster size >30 voxels). Error bars represent the standard error of the mean. ***p < 0.001.
As shown in Figure 3, we observed significant grey matter atrophy in bilateral STG and left caudate in AD-T2 as compared to AD-T1 (FDR corrected p < 0.05, cluster size >30 voxels). Further, we found significantly positive correlations between change scores of the MoCA and change values of GMV in both right STG [r(36) = 0.44, p = 0.01] and left caudate [r(36) = 0.35, p = 0.03; Figure 4], but not in left STG [r(36) = 0.25, p = 0.12].
Figure 3. Progressive atrophy in grey matter volume between AD-T2 and AD-T1. There was significant decrease in bilateral STG and left caudate in AD patients over a one-year period. Error bars represent the standard error of the mean. Error bars represent the standard error of the mean. L, left; R, right. ***p < 0.001.
Figure 4. Significant correlations between change scores in the MoCA and mean GMV changes in both right STG and left caudate. Changes indicate differences between AD-T2 and AD-T1. GMV, grey matter volume; STG, superior temporal gyrus. MoCA, Montreal Cognitive Assessment.
The structural covariance connectivity analysis showed significantly decreased strength of correlation between mean GMV values of the right STG and the left caudate in AD patients at both time points as compared to HC (Figure 5). The significant results were revealed by the permutation tests, which demonstrated the real structural covariance connectivity was out of 95% (two-tailed) of the supposed between-group differences.
Figure 5. Decreased structural covariance connectivity between right STG and left caudate in AD patients at both time points as compared to HC. The permutation tests demonstrated that the between-group difference in the real covariance connectivity was out of 95% (two-tailed) of the supposed between-group differences. The red lines indicate observed difference in the strength of correlations between STG volume and left caudate volume for HC vs. AD-T1 and HC vs. AD-T2. *p < 0.05.
The causal SCN analysis revealed that overlapped decrease areas in AD-T1 had strong causal effects on the continuing decrease areas, bilateral STG, and left caudate in AD-T2. The causal effects were defined as the mean causal correlation between GMV of the overlapped decrease areas and GMV of continuing decrease areas, bilateral STG, and left caudate in AD-T2 across 10,000 permutations, which were significant (p < 0.05) as tested by comparing all the permutated causal coefficients with zero (Figure 6).
Figure 6. Causal effects of overlapped decrease areas in AD-T1 on the continuing decrease areas, left and right STG, and left caudate in AD-T2. The red dash lines and the bar plots indicate the mean causal correlation between GMV of the overlapped decrease areas and GMV of continuing decrease areas, left and right STG, and left caudate in AD-T2 across 10,000 permutations.
For the classification analysis, 5 features including mean GMV values within overlapped decrease areas, continuing decrease areas, left and right STG, and left caudate were entered the prediction model. As displayed in Figure 7, the SVM classification analysis applying the LOOCV method achieved: (1) accuracy of 98.82%, sensitivity of 97.5%, and specificity of 100% for HC vs. AD-T1; (2) accuracy, sensitivity, and specificity of 100% for HC vs. AD-T2; (3) accuracy of 78.75%, sensitivity of 77.5%, and specificity of 80% for AD-T1 vs. AD-T2.
Figure 7. Classification results. There are high classification accuracy, sensitivity, and specificity for HC vs. AD-T1 (accuracy of 98.82%, sensitivity of 97.5%, and specificity of 100%), HC vs. AD-T2 (accuracy, sensitivity, and specificity of 100%), and AD-T1 vs. AD-T2 (accuracy of 78.75%, sensitivity of 77.5%, and specificity of 80%).
In the present study, we investigated grey matter atrophy in AD patients over a one-year period using a grey matter based spatial statistics approach (Parvathaneni et al., 2017), i.e., measures of grey matter volume on the cortical skeleton (Ball et al., 2013; Ouyang et al., 2019). We observed reduced total GMV and grey matter atrophy in a wide range of regions including inferior frontal gyrus, prefrontal cortex, lateral temporal gyrus, posterior cingulate cortex, insula, hippocampus, caudate, and thalamus in AD patients at both time points as compared to HC. We also found decreased GMV in bilateral STG and left caudate between AD-T2 and AD-T1, which was significantly correlated with concurrent cognitive decline (i.e., change scores in the MoCA). Further, we reported reduced structural covariance connectivity between right STG and left caudate in AD patients vs. HC, and significant causal effects of overlapped decrease areas in AD-T1 on continuing decrease areas, left and right STG, and left caudate in AD-T2. The classification analysis with AD-related grey matter atrophy as features demonstrated high accuracy, specificity, and sensitivity in classifying AD patients from HC, and AD-T1 from AD-T2. These findings provide new evidence for progressive brain structural abnormalities in AD patients over a one-year period, and associations between brain atrophy and cognitive decline in AD progression.
We observed that AD patients showed substantial grey matter atrophy in a wide range of brain regions, including inferior frontal gyrus, prefrontal cortex, lateral temporal gyrus, posterior cingulate cortex, insula, hippocampus, caudate, and thalamus. Most of these regions are consistent with those reported in previous studies (Karas et al., 2003; Zakzanis et al., 2003; Yang et al., 2012; Wang et al., 2015). However, in the current study, using the grey matter based spatial statistics, we also observed significant GMV differences in inferior frontal gyrus and insula. It may suggest that measurement of GMV at the core of the cortical plate is more sensitive to detecting group differences between AD patients and HC (Parvathaneni et al., 2017) as it alleviates partial volume effect and reduces individual variability (Ouyang et al., 2019).
One of the key findings is that we found significant decrease in the volume of bilateral STG and left caudate in AD patients over a one-year period. This finding of significant decrease in the volume of bilateral STG is consistent with that reported in a previous longitudinal study in AD patients showing fast atrophy in temporal lobes with time (Dicks et al., 2019). Reduced volume of template lobe and caudate has been found in a subtype of AD (i.e., Dominantly-Inherited Alzheimer’s Disease) as compared to noncarriers (Cash et al., 2013; Ryan et al., 2013). The significant reduction in the volume of caudate has also been observed in both MCI and AD patients as compared to normal adults (Barber et al., 2002; Almeida et al., 2003; Madsen et al., 2010; Jiji et al., 2013), while there was one study that reported no obvious change in the volume of caudate until the moderate stage of AD (Roh et al., 2011). Further, in agreement with previous studies (Looi et al., 2008; Liu et al., 2010; Jiji et al., 2013), we found significant correlations between change values of GMV in left caudate and cognitive decline in AD patients. For example, higher GMV values within caudate were correlated with higher cognitive function as indexed by MMSE scores across AD patients and control adults (Looi et al., 2008). Changes in the volume of caudate served as an indication of early AD (Jiji et al., 2013) and as predictors of the conversion from MCI to AD (Liu et al., 2010). Put together, these findings suggest STG and left caudate might be important neuroanatomical markers of pathological progression of AD.
In addition to AD-related GMV decrease, we also observed significantly reduced structural covariance connectivity between right STG and left caudate in AD patients as compared to HC. The structural covariance connectivity has been suggested as an effective method to comprehensively investigate different networks related to AD (Montembeault et al., 2016; Li et al., 2019). A few studies have demonstrated abnormal structural covariance networks in AD patients as compared to HC (Hafkemeijer et al., 2016; Montembeault et al., 2016; Chang et al., 2018, 2021; Li et al., 2019). For example, a recent study explored the grey matter SCN along AD continuum and reported reduced SCN between DMN and salience network associated with AD neuropathological progression (Li et al., 2019). Here, we present significantly reduced structural covariance connectivity between right STG and left caudate in AD patients as compared to HC, but no differences between AD-T2 and AD-T1. However, there were significant causal effects of overlapped decrease areas in AD patients at baseline (AD-T1) on continuing decrease areas, bilateral STG, and left caudate in AD patients at one-year follow-up (AD-T2). These findings suggest grey matter structural covariance connectivity is valuable in providing supplementary information linked to progressive changes in AD patients. However, given the relatively small sample size in the current study, further research is needed to confirm these results.
Another interesting findings are the classification results based on AD-related grey matter atrophy. Applying a linear SVM approach, it reached high accuracy, specificity, and sensitivity in classifying HC and AD patients, and AD patients at different time points. Previous studies have suggested that the pattern of GMV decrease is potentially useful for the classification of AD from cognitive normal adults (CN) (Magnin et al., 2008; Guo et al., 2014; Kruthika et al., 2018). Indeed, including overlapped and continuing decrease areas and regions showing progressive decrease in AD patients as features of the classification analysis, there were high discrimination accuracy, sensitivity, and specificity in classifying AD and CN, and classifying AD-T1 and AD-T2, suggesting patterns of GMV decrease serve as sensitive features of identifying the pathological progression of AD.
There are two limitations worth noting when interpreting the results. First, to obtain a reasonable amount of longitudinal MRI data, we included MRI scans collected in multi-sites and different models (i.e., Verio, TrioTim, and Skyra) of SIEMENS 3.0 T scanners. And, to minimize the inhomogeneity of the imaging data, we only included data scanned with the same parameters, which limited the sample size in the present study. In future studies, MRI data with larger sample sizes are needed to confirm the findings reported here. Second, structural covariance connectivity did not allow for the assessment of connectivity strength at the individual level, so it is still unclear how the abnormal structural covariance connectivity relates to the cognitive decline in AD patients. Thus, it would be worth examining the relationship of structural covariance connectivity and cognitive function to further characterize the neuroanatomical changes underlying pathological progression in AD.
In conclusion, using a grey matter based spatial statistics, we observed that AD patients at both time points showed grey matter atrophy in inferior frontal gyrus, prefrontal cortex, lateral temporal gyrus, posterior cingulate cortex, insula, hippocampus, caudate, and thalamus as compared to HC. There was also significant GMV decrease in both bilateral STG and left caudate in AD patients over a one-year period, and GMV decrease in right STG and left caudate was positively correlated with the concurrent cognitive decline. Further, AD patients demonstrated reduced structural covariance connectivity between right STG and left caudate, and overlapped decrease areas in AD-T1 had causal effects on continuing decrease areas, bilateral STG, and left caudate in AD-T2. In addition, using patterns of AD-related GMV decrease as features, there were high discrimination accuracy, specificity, and sensitivity in classifying HC and AD patients, and classifying AD patients at different time points. Our study provides novel insights into the structural deterioration related to pathological progression of AD and highlights the role of STG and left caudate in cognitive decline in AD patients. These findings may serve as a clinical aid to the prognosis of AD progression.
Data used in the present study were obtained from the Alzheimer’s Disease Neuroimaging Initiative (ADNI) database (adni.loni.usc.edu). The ADNI was launched in 2003 as a public-private partnership led by Michael W. Weiner. The primary goal of the ADNI is to test whether magnetic resonance imaging (MRI), positron emission tomography (PET), other biological markers, and clinical and neuropsychological assessment can be combined to measure the progression of mild cognitive impairment (MCI) and early Alzheimer’s disease (AD). For more information, please visit www.adni-info.org. The tidy data used in the current study are available at https://github.com/Yaqiongxiao/Progressive.changes.GM.AD.
YX and JW contributed to the conception and design of this study. KH and YX contributed to the data acquisition from ADNI. JW contributed to the data analysis. YX drafted the manuscript. JW, LG, and SY reviewed the manuscript. All authors contributed to the article and approved the submitted version.
This work was supported by the Shenzhen Science and Technology Innovation Commission (JCYJ20200109144801736), the National Natural Science Foundation of China (32200808, 82001799), and the Natural Science Foundation of Yunnan Province (202001BC070001, 202102AA100053).
Data collection and sharing for this project was funded by the Alzheimer’s Disease Neuroimaging Initiative (ADNI) (National Institutes of Health Grant U01 AG024904) and DOD ADNI (Department of Defense award number W81XWH-12-2-0012). ADNI is funded by the National Institute on Aging, the National Institute of Biomedical Imaging and Bioengineering, and through generous contributions from the following: AbbVie, Alzheimer’s Association; Alzheimer’s Drug Discovery Foundation; Araclon Biotech; BioClinica, Inc.; Biogen; Bristol-Myers Squibb Company; CereSpir, Inc.; Cogstate; Eisai Inc.; Elan Pharmaceuticals, Inc.; Eli Lilly and Company; EuroImmun; F. Hoffmann-La Roche Ltd and its affiliated company Genentech, Inc.; Fujirebio; GE Healthcare; IXICO Ltd.; Janssen Alzheimer Immunotherapy Research & Development, LLC.; Johnson & Johnson Pharmaceutical Research & Development LLC.; Lumosity; Lundbeck; Merck & Co., Inc.; Meso Scale Diagnostics, LLC.; NeuroRx Research; Neurotrack Technologies; Novartis Pharmaceuticals Corporation; Pfizer Inc.; Piramal Imaging; Servier; Takeda Pharmaceutical Company; and Transition Therapeutics. The Canadian Institutes of Health Research is providing funds to support ADNI clinical sites in Canada. Private sector contributions are facilitated by the Foundation for the National Institutes of Health (www.fnih.org). The grantee organization is the Northern California Institute for Research and Education, and the study is coordinated by the Alzheimer’s Therapeutic Research Institute at the University of Southern California. ADNI data are disseminated by the Laboratory for Neuro Imaging at the University of Southern California.
The authors declare that the research was conducted in the absence of any commercial or financial relationships that could be construed as a potential conflict of interest.
All claims expressed in this article are solely those of the authors and do not necessarily represent those of their affiliated organizations, or those of the publisher, the editors and the reviewers. Any product that may be evaluated in this article, or claim that may be made by its manufacturer, is not guaranteed or endorsed by the publisher.
R and matlab code for implementing all the analyses can be found at https://github.com/Yaqiongxiao/Progressive.changes.GM.AD.
Aisen, P. S., Petersen, R. C., Donohue, M., and Weiner, M. W. (2015). Alzheimer’s Disease Neuroimaging Initiative 2 clinical Core: Progress and plans. Alzheimers Dement. 11, 734–739. doi: 10.1016/J.JALZ.2015.05.005
Alexander-Bloch, A., Giedd, J. N., and Bullmore, E. (2013). Imaging structural co-variance between human brain regions. Nat. Rev. Neurosci.. 2013 145, 322–336. doi: 10.1038/nrn3465
Almeida, O. P., Burton, E. J., McKeith, I., Gholkar, A., Burn, D., and O’Brien, J. T. (2003). MRI study of caudate nucleus volume in Parkinson’s disease with and without dementia with Lewy bodies and Alzheimer’s disease. Dement. Geriatr. Cogn. Disord. 16, 57–63. doi: 10.1159/000070676
Anderson, V. M., Schott, J. M., Bartlett, J. W., Leung, K. K., Miller, D. H., and Fox, N. C. (2012). Gray matter atrophy rate as a marker of disease progression in AD. Neurobiol. Aging 33, 1194–1202. doi: 10.1016/J.NEUROBIOLAGING.2010.11.001
Ashburner, J. (2007). A fast diffeomorphic image registration algorithm. NeuroImage 38, 95–113. doi: 10.1016/J.NEUROIMAGE.2007.07.007
Ashburner, J., and Friston, K. J. (2005). Unified segmentation. NeuroImage 26, 839–851. doi: 10.1016/J.NEUROIMAGE.2005.02.018
Ball, G., Srinivasan, L., Aljabar, P., Counsell, S. J., Durighel, G., Hajnal, J. V., et al. (2013). Development of cortical microstructure in the preterm human brain. Proc. Natl. Acad. Sci. U. S. A. 110, 9541–9546. doi: 10.1073/pnas.1301652110
Barber, R., McKeith, I., Ballard, C., and O’Brien, J. (2002). Volumetric MRI study of the caudate nucleus in patients with dementia with Lewy bodies, Alzheimer’s disease, and vascular dementia. J. Neurol. Neurosurg. Psychiatry 72, 406–407. doi: 10.1136/JNNP.72.3.406
Baron, J. C., Chételat, G., Desgranges, B., Perchey, G., Landeau, B., De La Sayette, V., et al. (2001). In vivo mapping of gray matter loss with voxel-based morphometry in mild Alzheimer’s disease. NeuroImage 14, 298–309. doi: 10.1006/NIMG.2001.0848
Busatto, G. F., Garrido, G. E. J., Almeida, O. P., Castro, C. C., Camargo, C. H. P., Cid, C. G., et al. (2003). A voxel-based morphometry study of temporal lobe gray matter reductions in Alzheimer’s disease. Neurobiol. Aging 24, 221–231. doi: 10.1016/S0197-4580(02)00084-2
Cash, D. M., Ridgway, G. R., Liang, Y., Ryan, N. S., Kinnunen, K. M., Yeatman, T., et al. (2013). The pattern of atrophy in familial Alzheimer disease. Neurology 81, 1425–1433. doi: 10.1212/WNL.0B013E3182A841C6
Chang, H. I., Chang, Y. T., Huang, C. W., Huang, K. L., Hsu, J. L., Hsu, S. W., et al. (2021). Structural covariance network as an endophenotype in Alzheimer’s disease-susceptible single-nucleotide polymorphisms and the correlations with cognitive outcomes. Front. Aging Neurosci. 13:721217. doi: 10.3389/fnagi.2021.721217
Chang, Y. T., Huang, C. W., Chang, W. N., Lee, J. J., and Chang, C. C. (2018). Altered functional network affects amyloid and structural covariance in Alzheimer’s disease. Biomed. Res. Int. 2018, 1–12. doi: 10.1155/2018/8565620
Chang, C. C., and Lin, C. J. (2011). LIBSVM: A library for support vector machines. ACM Trans. Intell. Syst. Technol. 2, 1–27. doi: 10.1145/1961189.1961199
Chapleau, M., Aldebert, J., Montembeault, M., and Brambati, S. M. (2016). Atrophy in Alzheimer’s disease and semantic dementia: An ALE meta-analysis of voxel-based Morphometry studies. J. Alzheimers Dis. 54, 941–955. doi: 10.3233/JAD-160382
Dicks, E., Vermunt, L., van der Flier, W. M., Visser, P. J., Barkhof, F., Scheltens, P., et al. (2019). Modeling grey matter atrophy as a function of time, aging or cognitive decline show different anatomical patterns in Alzheimer’s disease. NeuroImage. Clin. 22:101786. doi: 10.1016/J.NICL.2019.101786
Evans, A. C. (2013). Networks of anatomical covariance. NeuroImage 80, 489–504. doi: 10.1016/J.NEUROIMAGE.2013.05.054
Folstein, M. F., Folstein, S. E., and McHugh, P. R. (1975). “Mini-mental state”. A practical method for grading the cognitive state of patients for the clinician. J. Psychiatr. Res. 12, 189–198. doi: 10.1016/0022-3956(75)90026-6
Guo, X., Wang, Z., Li, K., Li, Z., Qi, Z., Jin, Z., et al. (2010). Voxel-based assessment of gray and white matter volumes in Alzheimer’s disease. Neurosci. Lett. 468, 146–150. doi: 10.1016/J.NEULET.2009.10.086
Guo, Y., Zhang, Z., Zhou, B., Wang, P., Yao, H., Yuan, M., et al. (2014). Grey-matter volume as a potential feature for the classification of Alzheimer’s disease and mild cognitive impairment: an exploratory study. Neurosci. Bull.. 2014 303, 30, 477–489. doi: 10.1007/S12264-013-1432-X
Hafkemeijer, A., Möller, C., Dopper, E. G. P., Jiskoot, L. C., van den Berg-Huysmans, A. A., van Swieten, J. C., et al. (2016). Differences in structural covariance brain networks between behavioral variant frontotemporal dementia and Alzheimer’s disease. Hum. Brain Mapp. 37, 978–988. doi: 10.1002/hbm.23081
Jiji, S., Smitha, K. A., Gupta, A. K., Pillai, V. P. M., and Jayasree, R. S. (2013). Segmentation and volumetric analysis of the caudate nucleus in Alzheimer’s disease. Eur. J. Radiol. 82, 1525–1530. doi: 10.1016/J.EJRAD.2013.03.012
Kang, S. W., Jeon, S., Yoo, H. S., Chung, S. J., Lee, P. H., Sohn, Y. H., et al. (2019). Effects of Lewy body disease and Alzheimer disease on brain atrophy and cognitive dysfunction. Neurology 92, e2015–e2026. doi: 10.1212/WNL.0000000000007373
Karas, G. B., Burton, E. J., Rombouts, S. A. R. B., Van Schijndel, R. A., O’Brien, J. T., Scheltens, P., et al. (2003). A comprehensive study of gray matter loss in patients with Alzheimer’s disease using optimized voxel-based morphometry. NeuroImage 18, 895–907. doi: 10.1016/S1053-8119(03)00041-7
Kruthika, K. R., Rajeswari, P., and A., and Maheshappa, H. D., (2018). Classification of Alzheimer and MCI phenotypes on MRI data using SVM. Adv. Intell. Syst. Comput. 678, 263–275. doi: 10.1007/978-3-319-67934-1_23/COVER
Li, K., Luo, X., Zeng, Q., Huang, P., Shen, Z., Xu, X., et al. (2019). Gray matter structural covariance networks changes along the Alzheimer’s disease continuum. NeuroImage Clin. 23:101828. doi: 10.1016/j.nicl.2019.101828
Liu, Y., Paajanen, T., Zhang, Y., Westman, E., Wahlund, L. O., Simmons, A., et al. (2010). Analysis of regional MRI volumes and thicknesses as predictors of conversion from mild cognitive impairment to Alzheimer’s disease. Neurobiol. Aging 31, 1375–1385. doi: 10.1016/J.NEUROBIOLAGING.2010.01.022
Looi, J. C. L., Lindberg, O., Zandbelt, B. B., Östberg, P., Andersen, C., Botes, L., et al. (2008). Caudate nucleus volumes in frontotemporal lobar degeneration: differential atrophy in subtypes. AJNR Am. J. Neuroradiol. 29, 1537–1543. doi: 10.3174/AJNR.A1168
Madsen, S. K., Ho, A. J., Hua, X., Saharan, P. S., Toga, A. W., Jack, C. R., et al. (2010). 3D maps localize caudate nucleus atrophy in 400 Alzheimer’s disease, mild cognitive impairment, and healthy elderly subjects. Neurobiol. Aging 31, 1312–1325. doi: 10.1016/J.NEUROBIOLAGING.2010.05.002
Magnin, B., Mesrob, L., Kinkingnéhun, S., Pélégrini-Issac, M., Colliot, O., Sarazin, M., et al. (2008). Support vector machine-based classification of Alzheimer’s disease from whole-brain anatomical MRI. Neuroradiology. 2008 512, 73–83. doi: 10.1007/S00234-008-0463-X
Montembeault, M., Rouleau, I., Provost, J. S., and Brambati, S. M. (2016). Altered gray matter structural covariance networks in early stages of Alzheimer’s disease. Cereb. Cortex 26, 2650–2662. doi: 10.1093/cercor/bhv105
Morris, J. C. (1993). The clinical dementia rating (CDR): current version and scoring rules. Neurology 43, 2412–2414. doi: 10.1212/WNL.43.11.2412-A
Nasreddine, Z. S., Phillips, N. A., Bédirian, V., Charbonneau, S., Whitehead, V., Collin, I., et al. (2005). The Montreal cognitive assessment, MoCA: a brief screening tool for mild cognitive impairment. J. Am. Geriatr. Soc. 53, 695–699. doi: 10.1111/J.1532-5415.2005.53221.X
Ouyang, M., Jeon, T., Sotiras, A., Peng, Q., Mishra, V., Halovanic, C., et al. (2019). Differential cortical microstructural maturation in the preterm human brain with diffusion kurtosis and tensor imaging. Proc. Natl. Acad. Sci. U. S. A. 116, 4681–4688. doi: 10.1073/pnas.1812156116
Parvathaneni, P., Rogers, B. P., Huo, Y., Schilling, K. G., Hainline, A. E., Anderson, A. W., et al. (2017). Gray matter surface based spatial statistics (GS-BSS) in diffusion microstructure. Med. Image Comput. Comput. Assist. Interv. 10433, 638–646. doi: 10.1007/978-3-319-66182-7_73
Qing, Z., Chen, F., Lu, J., Lv, P., Li, W., Liang, X., et al. (2021). Causal structural covariance network revealing atrophy progression in Alzheimer’s disease continuum. Hum. Brain Mapp. 42, 3950–3962. doi: 10.1002/hbm.25531
Roh, J. H., Qiu, A., Seo, S. W., Soon, H. W., Kim, J. H., Kim, G. H., et al. (2011). Volume reduction in subcortical regions according to severity of Alzheimer’s disease. J. Neurol. 258, 1013–1020. doi: 10.1007/S00415-010-5872-1
Ryan, N. S., Keihaninejad, S., Shakespeare, T. J., Lehmann, M., Crutch, S. J., Malone, I. B., et al. (2013). Magnetic resonance imaging evidence for presymptomatic change in thalamus and caudate in familial Alzheimer’s disease. Brain 136, 1399–1414. doi: 10.1093/BRAIN/AWT065
Seeley, W. W., Crawford, R. K., Zhou, J., Miller, B. L., and Greicius, M. D. (2009). Neurodegenerative diseases target large-scale human brain networks. Neuron 62, 42–52. doi: 10.1016/J.NEURON.2009.03.024
Spreng, R. N., and Turner, G. R. (2013). Structural covariance of the default network in healthy and pathological aging. J. Neurosci. 33, 15226–15234. doi: 10.1523/JNEUROSCI.2261-13.2013
Thomann, P. A., Schläfer, C., Seidl, U., Santos, V. D., Essig, M., and Schröder, J. (2008). The cerebellum in mild cognitive impairment and Alzheimer’s disease – A structural MRI study. J. Psychiatr. Res. 42, 1198–1202. doi: 10.1016/J.JPSYCHIRES.2007.12.002
Toniolo, S., Serra, L., Olivito, G., Marra, C., Bozzali, M., and Cercignani, M. (2018). Patterns of cerebellar gray matter atrophy across Alzheimer’s disease progression. Front. Cell. Neurosci. 12:430. doi: 10.3389/FNCEL.2018.00430/BIBTEX
Van De Mortel, L. A., Thomas, R. M., and Van Wingen, G. A. (2021). Grey matter loss at different stages of cognitive decline: A role for the thalamus in developing Alzheimer’s disease. J. Alzheimers Dis. 83, 705–720. doi: 10.3233/JAD-210173
Wang, W. Y., Yu, J. T., Liu, Y., Yin, R. H., Wang, H. F., Wang, J., et al. (2015). Voxel-based meta-analysis of grey matter changes in Alzheimer’s disease. Transl. Neurodegener. 4, 1–9. doi: 10.1186/S40035-015-0027-Z/FIGURES/2
Weiner, M. W., Aisen, P. S., Jack, C. R., Jagust, W. J., Trojanowski, J. Q., Shaw, L., et al. (2010). The Alzheimer’s Disease Neuroimaging Initiative: Progress report and future plans. Alzheimers Dement. 6, 202–211.e7. doi: 10.1016/J.JALZ.2010.03.007
Weiner, M. W., Veitch, D. P., Aisen, P. S., Beckett, L. A., Cairns, N. J., Green, R. C., et al. (2017). The Alzheimer’s Disease Neuroimaging Initiative 3: continued innovation for clinical trial improvement. Alzheimers Dement. 13, 561–571. doi: 10.1016/J.JALZ.2016.10.006
Yang, J., Pan, P., Song, W., Huang, R., Li, J., Chen, K., et al. (2012). Voxelwise meta-analysis of gray matter anomalies in Alzheimer’s disease and mild cognitive impairment using anatomic likelihood estimation. J. Neurol. Sci. 316, 21–29. doi: 10.1016/J.JNS.2012.02.010
Zakzanis, K. K., Graham, S. J., and Campbell, Z. (2003). A meta-analysis of structural and functional brain imaging in dementia of the Alzheimer’s type: A neuroimaging profile. Neuropsychol. Rev. 13, 1–18. doi: 10.1023/A:1022318921994
Keywords: Alzheimer’s disease, grey matter atrophy, progressive changes, superior temporal regions, left caudate, cognitive decline
Citation: Xiao Y, Wang J, Huang K, Gao L and Yao S (2023) Progressive structural and covariance connectivity abnormalities in patients with Alzheimer’s disease. Front. Aging Neurosci. 14:1064667. doi: 10.3389/fnagi.2022.1064667
Received: 08 October 2022; Accepted: 13 December 2022;
Published: 06 January 2023.
Edited by:
Fermín Segovia, University of Granada, SpainReviewed by:
Diego Castillo-Barnes, University of Granada, SpainCopyright © 2023 Xiao, Wang, Huang, Gao, and Yao. This is an open-access article distributed under the terms of the Creative Commons Attribution License (CC BY). The use, distribution or reproduction in other forums is permitted, provided the original author(s) and the copyright owner(s) are credited and that the original publication in this journal is cited, in accordance with accepted academic practice. No use, distribution or reproduction is permitted which does not comply with these terms.
*Correspondence: Yaqiong Xiao, ✉ eWFxLnhpYW9AZ21haWwuY29t
†Data used in preparation of this article were obtained from the Alzheimer’s Disease Neuroimaging Initiative (ADNI) database (adni.loni.usc.edu). As such, the investigators within the ADNI contributed to the design and implementation of ADNI and/or provided data but did not participate in analysis or writing of this report. A complete listing of ADNI investigators can be found at: http://adni.loni.usc.edu/wp-content/uploads/how_to_apply/ADNI_Acknowledgement_List.pdf
Disclaimer: All claims expressed in this article are solely those of the authors and do not necessarily represent those of their affiliated organizations, or those of the publisher, the editors and the reviewers. Any product that may be evaluated in this article or claim that may be made by its manufacturer is not guaranteed or endorsed by the publisher.
Research integrity at Frontiers
Learn more about the work of our research integrity team to safeguard the quality of each article we publish.