- 1Department of Neurology, Yonsei University College of Medicine, Seoul, South Korea
- 2Department of Neurology, Sanggye Paik Hospital, Inje University College of Medicine, Seoul, South Korea
- 3Department of Internal Medicine, Severance Hospital, Endocrine Research Institute, Yonsei University College of Medicine, Seoul, South Korea
- 4Biostatistics Collaboration Unit, Yonsei University College of Medicine, Seoul, South Korea
- 5Endocrine Research Institute, Severance Hospital, Seoul, South Korea
- 6Department of Health Promotion, Severance Health Check-Up, Severance Hospital, Yonsei University Health System, Seoul, South Korea
- 7Severance Biomedical Science Institute, Yonsei University College of Medicine, Seoul, South Korea
Parkinson’s disease (PD) and osteoporosis are degenerative diseases that have shared pathomechanisms. To investigate the associations of skull bone density with nigrostriatal dopaminergic degeneration and longitudinal motor prognosis in female patients with PD. We analyzed the data of 260 drug-naïve female PD patients aged ≥50 years old who were followed-up for ≥3 years after their first visit to the clinic with baseline dopamine transporter (DAT) imaging. We measured skull bone density as a surrogate marker for systemic bone loss by calculating the Hounsfield unit (HU) in computed tomography scans. A Cox proportional hazard model was built to compare the rates of levodopa-induced dyskinesia (LID) or wearing-off according to skull HU. Longitudinal changes in levodopa-equivalent dose (LED) during a 3-year follow-up were assessed using a linear mixed model. A lower skull HU was associated with lower baseline DAT availability in striatal subregions; however, this relationship was not significant after adjusting for age, disease duration, body mass index, and white matter hyperintensities. After adjusting for confounding factors, a lower skull HU was significantly associated with an increased risk of LID development (hazard ratio = 1.660 per 1 standard deviation decrease, p = 0.007) and wearing-off (hazard ratio = 1.613, p = 0.016) in younger (<67 years) but not in older patients. Furthermore, a lower skull HU was associated with a steeper increase in LED during follow-up in younger patients only (β = –21.99, p < 0.001). This study suggests that baseline skull bone density would be closely linked to motor prognosis in drug naïve women with PD.
Introduction
Parkinson’s disease (PD) is the second most common neurodegenerative disorder, characterized by the progressive loss of dopaminergic neurons in the substantia nigra of the midbrain (Braak et al., 2003). Aging is the most important risk factor for PD due to its close relation to PD pathogenesis and its contribution to disease progression (Collier et al., 2017; Lee et al., 2017).
Osteoporosis is a common systemic skeletal disease characterized by decreased bone mass and strength, leaving one susceptible to fragility fracture as a result of the aging process (Hernlund et al., 2013). One important feature of osteoporosis is the contribution of sex as a risk factor, in which the prevalence of osteoporosis is four times greater in women than in men (Alswat, 2017). Ample evidence has demonstrated an association between osteoporosis and PD. Osteoporosis is prevalent in PD patients, and accordingly, PD patients have a higher risk of osteoporosis and osteoporotic fractures (Torsney et al., 2014). A recent study has demonstrated that osteoporosis is a risk factor for the development of PD among women (Feng et al., 2021). This association may be ascribed to overlapping pathomechanisms, such as autophagy dysfunction, mitochondrial dysfunction and inflammation (Wang et al., 2016; Wang et al., 2020). Another common feature underpinning these two conditions is the effect of sex hormones. Specifically, menopause has been reported risk factor of both osteoporosis and PD (Ragonese et al., 2006; Ji and Yu, 2015), and postmenopausal estrogen use prevents the development of both diseases (Syed et al., 2008; Lee et al., 2019).
Until now, the effects of bone mass on nigrostriatal dopaminergic degeneration and longitudinal motor prognosis in PD patients have not yet been investigated. Conveniently, Hounsfield unit (HU) values of the skull bone can be obtained from computed tomography (CT) scans performed concurrently with dopamine transporter (DAT) imaging. Moreover, decreased skull bone HU has been proposed as a surrogate marker of systemic bone loss, which can be utilized as a tool for opportunistic osteoporosis screening (Na et al., 2018). In this study we hypothesized that low skull bone HU can predict poor long-term motor prognosis in female drug naïve PD patients. We performed an analysis of striatal DAT availability and clinical parameters of longitudinal disease progression, including longitudinal increases in dopaminergic medication doses and motor complication rates in female PD patients, all according to the severity of skull bone density loss.
Materials and methods
Participants
We retrospectively reviewed 660 de novo PD patients who were drug-naïve and visited the Movement Disorders outpatient clinic at the Severance Hospital from April 2009 to September 2015. We used the following inclusion criteria: (1) female sex, (2) age at diagnosis ≥50 years, and (3) follow-up duration ≥3 years. The cut-off value of age at diagnosis in this study was based on the median age of menopause in Korean women (Park et al., 2018), and PD was diagnosed according to the clinical diagnostic criteria of the United Kingdom Parkinson’s disease Society Brain Bank. Additionally, N-(3-[18F]fluoropropyl)-2β-carbon ethoxy-3β-(4-iodophenyl) nortropane positron emission tomography (18F-FP-CIT PET) scans revealed decreased DAT availability in the posterior putamen of all patients. Parkinsonian motor symptoms were assessed at initial visit under dopaminergic drug-naïve condition using the Unified Parkinson’s Disease Rating Scale Part III (UPDRS-III) (Park et al., 2020), and olfactory function was measured using the cross-cultural smell identification test (CCSIT). Depression was evaluated using the Beck Depression Inventory (BDI), and the Korean version of the Mini-Mental State Examination (MMSE) was used to assess general cognition among patients (Han et al., 2008). In the final cohort, we included 260 female de novo PD patients, excluding (1) 316 male patients, (2) 66 patients with follow-up duration <3 years, (3) 13 patients with age at diagnosis <50 years, and (4) 5 patients with imaging process error. All enrolled patients were followed-up for a mean period of 6.22 ± 1.99 years and did not present with additional atypical features, including poor response to dopaminergic medications, ataxia, prominent autonomic dysfunction, vertical gaze limitation, repeated unprovoked falls within 3 years of parkinsonian symptom onset, and cortical sensory loss during the follow-up period. All patients were also investigated for medical history and vascular risk factors, including hypertension, diabetes mellitus, dyslipidemia, cardiac disease, and ischemic stroke.
Acquisition and quantitative analyses of 18F-FP-CIT PET scans
18F-FP-CIT PET scans were acquired using a GE PET-CT DSTe scanner (GE Discovery STE; GE Healthcare; Milwaukee, WI, USA), which obtains images with a three-dimensional resolution of 2.3-mm full width at half maximum. After fasting for ≥6 h, the patients were intravenously injected with 5 mCi (185 MBq) of 18F-FP-CIT. 90 min after the injection, PET images were acquired for 20 min in the three-dimensional mode at 120 kVp and 380 mA, and image processing was performed using the SPM8 software (Wellcome Department of Imaging Neuroscience, Institute of Neurology, UCL, London, UK) with Matlab 2013a for Windows (Math Works, Natick, MA, USA). Quantitative analyses in this procedure were based on volumes of interests (VOIs), which were defined based on a template in standard space. After imaging processing, all reconstructed PET images were spatially normalized into the Montreal Neurology Institute template space using a standard 18F-FP-CIT PET template which was generated from 18F-FP-CIT PET and T1-weighted magnetic resonance imaging (MRI) scans of 13 normal controls. Twelve VOIs of bilateral striatal subregions and one occipital VOI were drawn on a co-registered, spatially normalized, single T1-weighted MR and 18F-FP-CIT PET template image on the MRIcro version 1.37 software (Chris Rorden, Columbia, SC, USA) (Oh et al., 2012). The striatum was divided along the anterior-posterior commissure line on the transverse plane into dorsal and ventral portions. The ventral portion comprised two subregions: the ventral putamen and ventral striatum. Meanwhile, the dorsal portion was divided along the coronal anterior commissure plane into the following anterior and posterior subregions: the anterior caudate, posterior caudate, anterior putamen, and posterior putamen. These VOIs were adjusted using a minor translation in our in-house editing software, ANIQUE (Oh et al., 2014). DAT availability was calculated by the non-displaceable binding potential, which was defined as follows: (mean standardized uptake value of the striatal subregions VOI–mean standardized uptake value of the occipital VOI)/(mean standardized uptake of the occipital VOI) (Innis et al., 2007).
Skull bone Hounsfield unit measurement
Skull bone HU was measured using a single slice CT image at the predefined level where the lateral ventricles immediately disappear based on prior studies (Figure 1A; Na et al., 2018). To calculate skull bone HU from the entire skull area, a commercially available deep learning software, which can segment bone and soft tissues based on the 3D U-Net model was used (DeepCatch ver. 1.0.0.0, MEDICALIP Co. Ltd., Seoul, Korea) (Kim et al., 2021; Lee et al., 2021). To train the model for bone segmentation deployed in DeepCatch software, threshold of 450 HU, which was known to minimized differences of cortical bone estimate between higher resolution CT and histological methods in prior reports, (Poole et al., 2010; Treece et al., 2010) was applied to crudely segment outline of bone mask in each CT slice, followed by manual correction by expert radiologist with more than 10 years of experience. After applying DeepCatch 3D U-Net model to segment the skull bone mask, human inspection was performed with manual correction for the masks if required. Semi-automatic segmentation performance for the bone mask measured using the Dice similarity coefficient was calculated to be 98.6 ± 1.1% (a degree of overlap between U-Net-driven masks and reference masks, p < 0.001), and inter-rater reliability of skull bone HU measurement using the 3D U-Net model was noted to be good (intraclass correlation 0.99, p < 0.001) when assessed in 30 randomly sampled skull CT images. To determine whether skull bone density can reflect systemic bone loss in women, we evaluated the correlation between skull bone and lumbar spine HU in a separate health examination cohort, comprising community-dwelling individuals (Severance Health Check-Up cohort), which was approved by the Institutional Review Board of Severance Hospital (IRB No. 4-2021-0524). In that study, 77 women underwent an individual health examination package, including a 18F-fluorodeoxyglucose PET-CT scan of the whole body and brain performed at the same day in a commercial examination center (Severance Health Check-Up Center, Seoul, South Korea) between 2010 and 2020. After excluding those with ethnicities other than Korean and those <40 years of age, CT scans of 70 individuals were retrospectively analyzed (Siemens Biograph 40 TruePoint PET-CT, 120 kVp). Lumbar spine HU was then measured at the manually positioned ellipsoid region of interest in the mid-vertebral level of first lumbar spine (L1) using the MEDIP PRO software (Medical IP, Korea), a well validated method for CT-based opportunistic osteoporosis screening (Figure 1B; Jang et al., 2019). The skull bone and L1 HU showed a good positive correlation (Pearson correlation coefficient = 0.76, p < 0.001; Figure 1C).
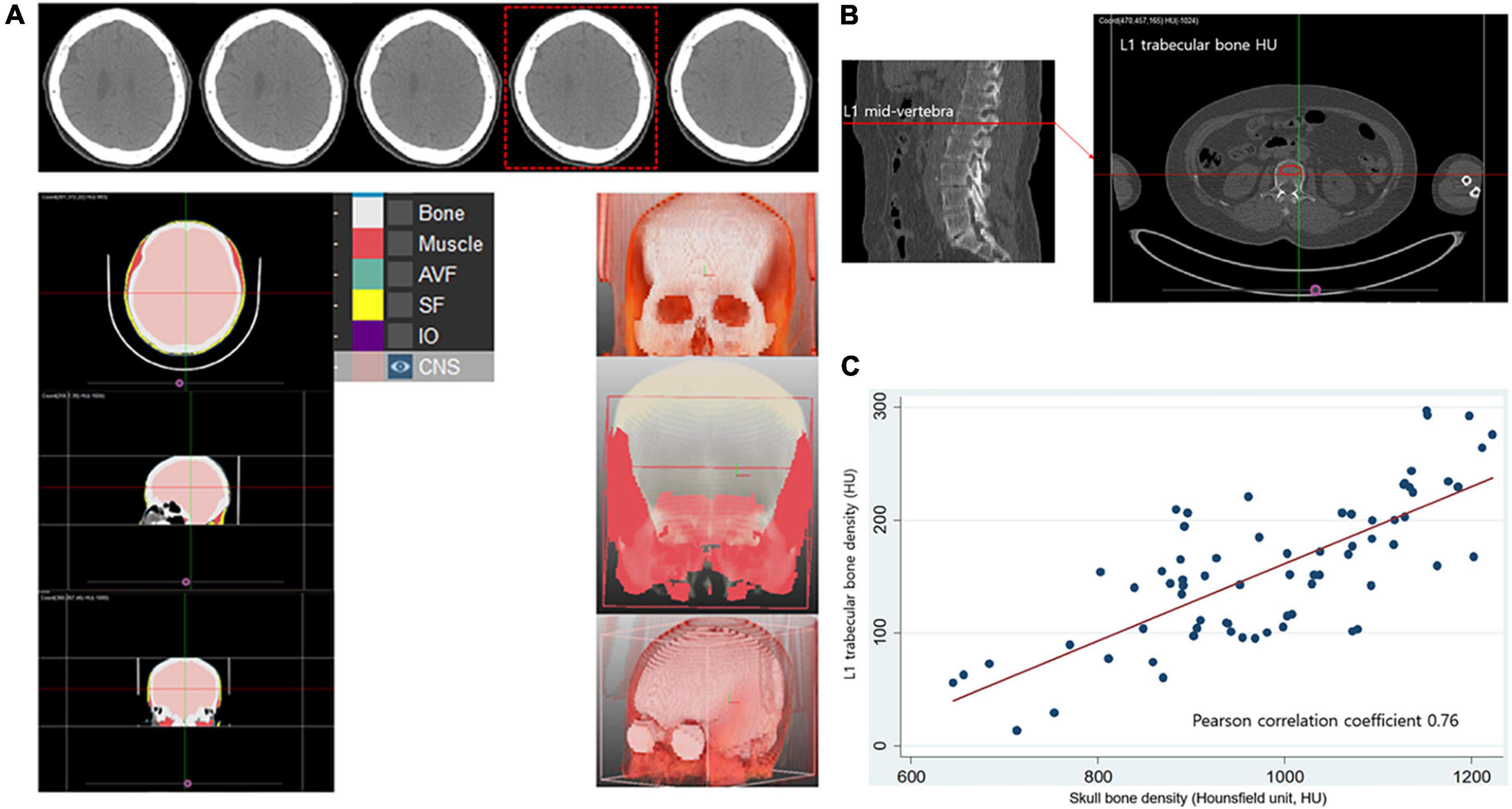
Figure 1. Measurement of Hounsfield unit in skull and lumbar bone and their correlation in healthy control. (A) Segmentation of skull bone and calculation of radiation attenuation value of skull bone area using automated deep learning software (DeepCatch, Medical IP, Korea). Skull bone HU values were measured using single slice CT image at the predefined level where the lateral ventricles immediately disappear. (B) Measurement of lumbar spine trabecular bone radiation attenuation. (C) Significant positive correlation (r = 0.76, p < 0.001) between skull bone density and lumbar spine trabecular bone density measured from computed tomography scans. HU, Hounsfield unit.
Acquisition of fluid attenuated inversion recovery sequence images and grading of white matter hyperintensities
Of the 260 enrolled patients, 201 (77.3%) underwent brain MRI scans at the Severance Hospital using a 3.0 T scanner (Achieva; Philips Medical System, Best, Netherlands) at initial assessment, which included fluid attenuated inversion recovery (FLAIR) sequence images. The FLAIR sequence images were acquired with the following parameters: matrix, 512 × 512; slice number, 22; pixel spacing, 0.449 mm × 0.449 mm; slice thickness, 5 mm; gap, 2 mm; field of view, 230 mm; repetition time, 11,000 ms; echo time, 125 ms; inversion time, 2,800 ms; and flip angle, 90°. The remaining 59 (22.7%) patients underwent brain MRI scans with FLAIR sequence images at other hospitals before being referred to Severance Hospital. The visual rating scale of the white matter hyperintensities (WMHs) was assessed by two neurologists (SHJ and Y-gL) using the Scheltens scale, wherein the periventricular and lobar (frontal, parietal, temporal, and occipital) white matter hyperintensities, and the basal ganglia and infratentorial signal hyperintensities were rated separately in a semi-quantitative manner (Scheltens et al., 1993). The intra- and inter-rater reliability of total WMHs were high (intra class correlation coefficients = 0.984 and 0.966, respectively). A final consensus rating between the two raters was used for subsequent analyses.
Assessment of the development of levodopa-induced dyskinesia and wearing-off
Participants visited our outpatient clinic every 3–6 months, and two movement disorder experts (YHS and PHL) carefully assessed the presence of LID and wearing-off through a history obtained from the patients and caregivers or direct neurological examination at every visit (Jeong et al., 2021a). For consistency, the date on which the female PD patients or their caregivers reported LID or wearing-off occurrence or the date on which LID or wearing-off was first observed in the clinic was regarded as the date of LID or wearing-off occurrence in the medical records. To assess the effects of skull HU on the development of LID and wearing-off, the Cox regression model was used to estimate the hazard ratio (HR) and 95% confidence intervals (CIs), while adjusting for age at diagnosis, disease duration, baseline DAT availability in the posterior putamen, total WMHs, BMI, and levodopa equivalent dose (LED) at LID or wearing-off onset in patients with LID or wearing-off or LED at the last visit to the outpatient clinic in those without either.
Longitudinal assessment of the changes in levodopa-equivalent daily doses over time
The patients visited the outpatient clinic every 3–6 months and their dopaminergic medications were adjusted for effective symptom control by PHL and YHS according to their individual responses. At each visit, dopaminergic medication doses were assessed, and the LED was calculated using the following formula: levodopa × 1 + controlled-release levodopa × 0.75 + ropinirole × 20 + pramipexole × 100 + levodopa × 0.33 if they were on entacapone + selegiline × 10 + rasagiline × 100 (Tomlinson et al., 2010). Thereafter, a linear mixed model was used to compare the rate of longitudinal changes in LED for 3 years, and participants were added as random effects, whereas age at diagnosis, disease duration, and DAT availability in the posterior putamen, BMI, and total WMHs were added as fixed effect terms. The effect of the skull HU on longitudinal changes in LED was tested using a skull HU × time interaction term.
Statistical analyses
To investigate the effect of skull HU per a standard deviation (SD), we used individual z-transformed skull HU values in all analyses. A multivariate linear regression analysis was used to determine the independent effects of skull HU after adjusting for age at diagnosis, disease duration, BMI, and total WMHs, a linear mixed model was used to compare the rates of the longitudinal LED changes according to skull HU, and a log-rank test and Cox regression model were used to assess the effects of skull HU on the development of LID and wearing-off. Since age is a crucial factor for osteoporosis, we further investigated whether an age × skull HU interaction term was significant in multivariate regression, Cox regression, and linear mixed model analyses, wherein an interaction term with p < 0.2 was considered an interaction effect (Jeong et al., 2021b). If an interaction term was significant, we further performed subgroup analyses using the median split method to dichotomize PD patients into the young and old age groups. Statistical analyses were performed using the STATA 16.1 (StataCorp LLC, College Station, TX, USA) and R (v4.0)1 software, and results with a two-tailed p < 0.05 were considered statistically significant.
Results
Demographic and clinical characteristics
Demographic and clinical characteristics of the included patients are summarized in Table 1. Age at symptom onset and diagnosis was 64.58 ± 8.58 and 66.11 ± 8.49, respectively. The average disease duration was 17.14 ± 16.98 months, UPDRS-III score at the time of PD diagnosis was 22.72 ± 10.22, average years of education was 7.92 ± 4.16 years, and MMSE score at baseline was 26.34 ± 2.95. Additionally, the average CCSIT and BDI scores were 7.09 ± 2.22 and 13.66 ± 8.75, respectively, and the average skull bone HU was 709.88 ± 123.96.
Association between skull Hounsfield unit and striatal dopamine transporter availability at baseline
Dopamine transporter availability for each striatal sub-region is shown in Table 1. The lower skull HU was associated with lower baseline DAT availability in the anterior caudate (β = −0.176, SE = 0.041, p < 0.001), posterior caudate (β = −0.137, SE = 0.034, p < 0.001), anterior putamen (β = −0.114, SE = 0.039, p = 0.004), and ventral striatum (β = −0.111, SE = 0.035, p = 0.001). However, these associations were not statistically significant after adjustment for age at diagnosis, disease duration, BMI, and total WMHs. Furthermore, the age × skull HU interaction term in each striatal sub-region was not significant (Table 2).
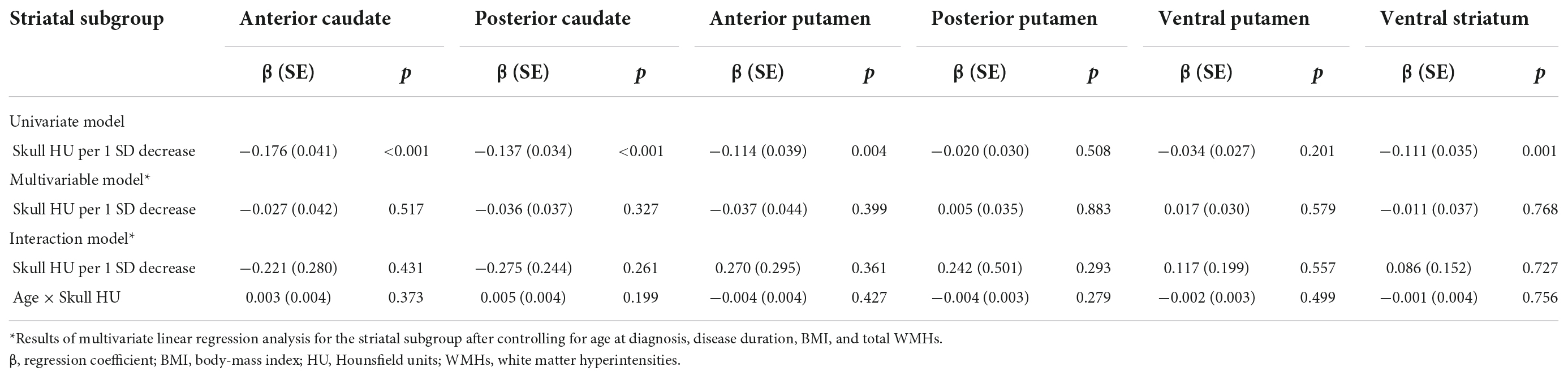
Table 2. Multiple linear regression analysis of dopamine transporter availability in each striatal subgroup.
Effect of skull Hounsfield unit on the development of levodopa-induced dyskinesia and wearing-off
During the follow-up period, LID and wearing-off developed in 83 (31.9%) and 75 (28.8%) of the 260 female PD patients, respectively. After adjusting for age at diagnosis, disease duration, BMI, total WMHs, and LED, lower skull HU tended to be associated with greater risks of developing LID (HR = 1.251 per 1 SD decrease of skull HU, 95% CI = 0.978–1.599, p = 0.074), whereas no significant association between baseline skull HU and the development of wearing-off was noted (HR = 1.142, 95% CI = 0.878–1.484, p = 0.321, Table 3). Since age × skull HU interaction terms were significant in the LID (p = 0.138) and wearing-off (p = 0.134) models, we performed subgroup analyses using the median split of age (median age = 67). Additionally, after adjusting for covariates, the age group × skull HU interaction terms were also found to be significant (LID, p = 0.164; wearing-off, p = 0.066). In the subgroup analyses, skull HU was a significant predictor for the development of LID (HR = 1.660, 95% CI = 1.150–2.398, p = 0.007) and wearing-off (HR = 1.613, 95% CI = 1.094–2.380, p = 0.016) in the young age group. However, there was no significant association between skull HU and the development of LID (HR = 1.019, 95% CI = 0.705–1.473, p = 0.919) or wearing-off (HR = 0.845, 95% CI = 0.565–1.268, p = 0.418) in the old age group (Table 4).
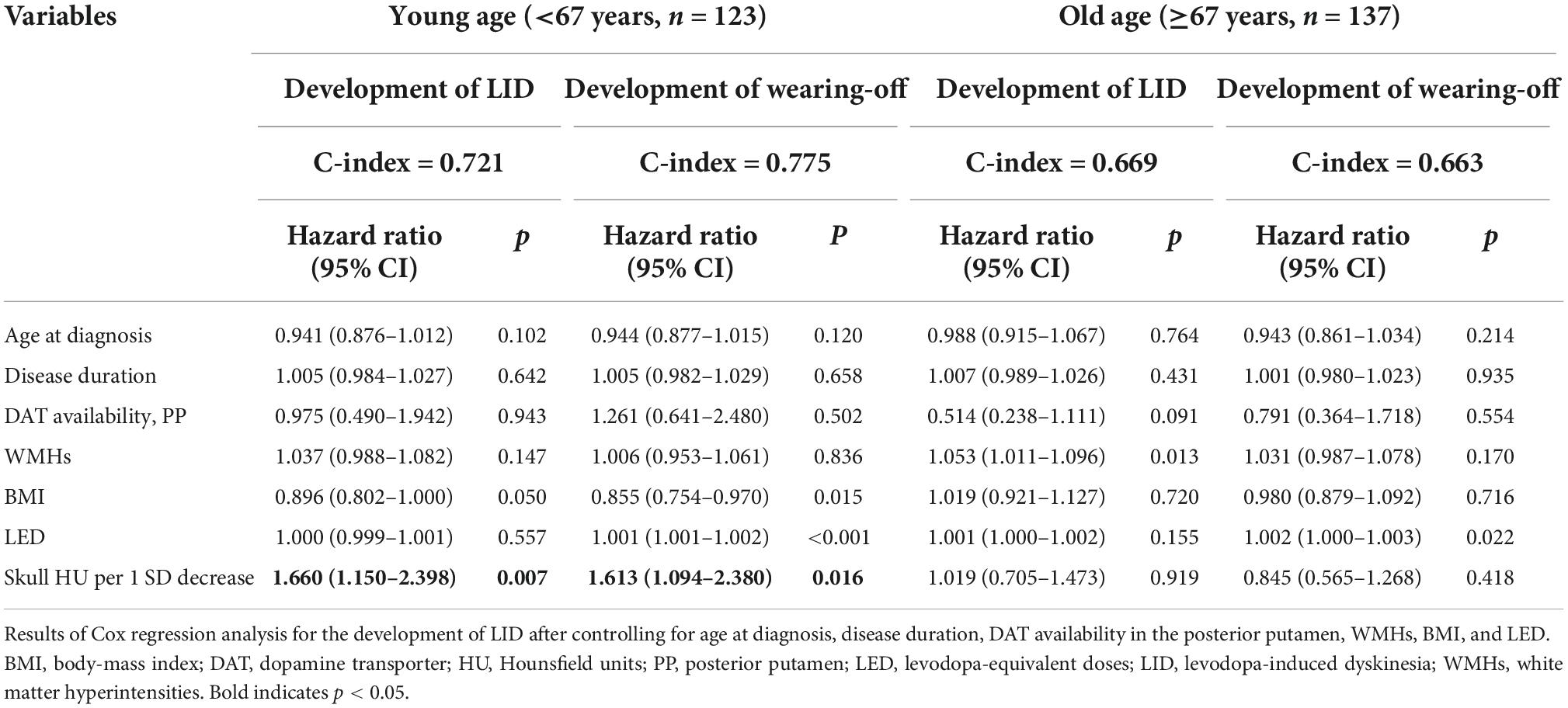
Table 4. Multivariate Cox hazard models for prediction of LID and wearing-off according to age subgroup.
Longitudinal assessment of the changes in levodopa-equivalent doses among the groups
All enrolled PD patients were treated with dopaminergic medications for at least 3 years. In the linear mixed model, the skull HU × time interaction term was statistically significant (β = 14.66, SE = 3.42, p < 0.001) after adjusting for age at diagnosis, disease duration, DAT availability in the posterior putamen, BMI, total WMHs, skull HU, time, and skull HU × time, indicating that patients with low skull HU value had a rapidly increased LED (Figure 2A). Moreover, since age × skull HU × time interaction terms were significant in this model (p = 0.008, Table 5), we performed subgroup analyses using the median split of age. In the subgroup analyses, skull HU was a significant predictor for rapid LED increase over time in the younger subgroup (β = 21.99, SE = 4.41, p < 0.001, Figure 2B), whereas no significant association between skull HU and longitudinal LED increase was observed in the old age group (β = 0.70, SE = 5.96, p = 0.907, Figure 2C).
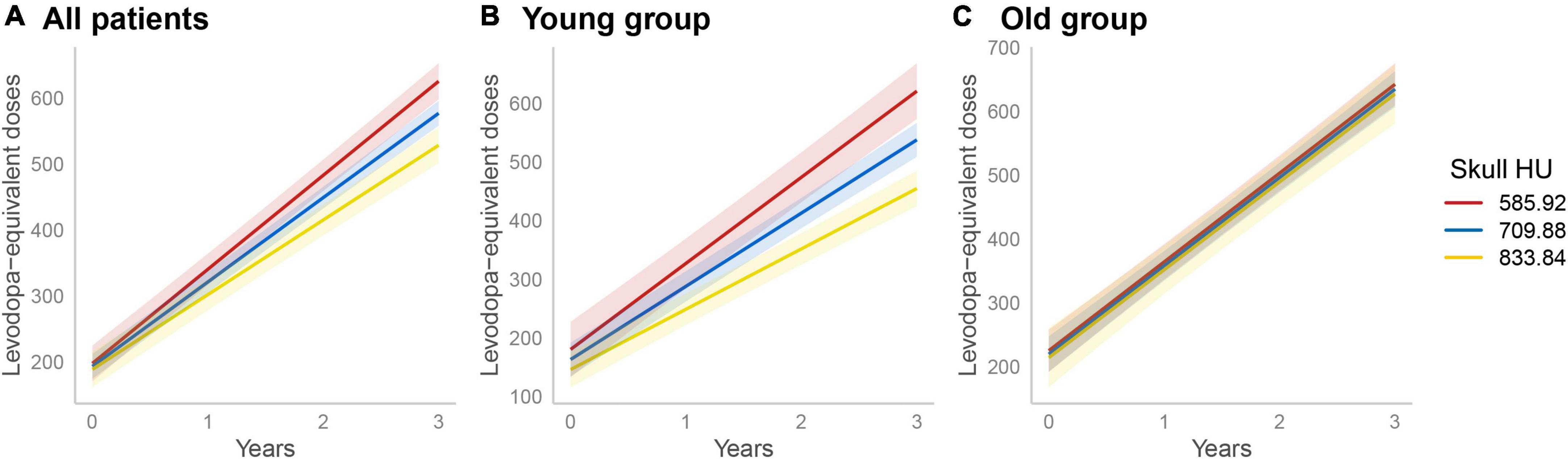
Figure 2. Longitudinal increases in levodopa-equivalent doses. A linear mixed model analysis showed significant difference in longitudinal LED increase according to skull HU values (skull HU per 1 SD decrease × Time, p < 0.001) (A). In the subgroup analysis, longitudinal LED increase significantly differed according to skull HU in the young age group (B), but not in the old age group (C). The light red, blue, or yellow band represents the 95% confidence interval in each point of skull HU. LED, levodopa-equivalent doses; HU, Hounsfield unit; SD, standard deviation.
Discussion
Baseline skull HU was used as a surrogate marker for osteoporosis in this study. Although T-score measured at the lumbar spine or proximal femur using dual-energy x-ray absorptiometry (DXA) is the gold standard for osteoporosis diagnosis, a previous study suggested that HU value measured in CT scans could be a reliable alternative method for bone mineral density and osteoporosis assessment (Pompe et al., 2016). This is especially true, in HU values obtained from the trabecular region of interest in the lumbar vertebrae, showing good reliability and sensitivity in osteoporosis detection, as defined based on the DXA T-score (Jang et al., 2019). Furthermore, several studies have shown that skull HU was significantly associated with T-score, allowing its usage as an opportunistic screening tool for osteoporosis (Na et al., 2018). Similarly, in the present study, we demonstrated that skull HU had a strong positive correlation with L1 HU, consistent with previous findings. Based on this, we investigated the associations of low skull bone density with nigrostriatal dopaminergic degeneration and the clinical course of drug-naïve female PD patients. The major findings were as follows. First, there was no association between skull HU and baseline DAT availability. Second, baseline skull HU was a significant predictor of the development of LID and wearing-off in relatively younger PD patients; however, this association was not observed in older PD patients. Lastly, baseline skull HU was closely associated with longitudinal LED in younger PD patients. Together, these findings suggest that baseline skull HU is closely associated with motor prognosis in drug naïve female PD patients in an age-dependent manner.
To the best of our knowledge, this is the first study to explore whether early-stage bone density was associated with baseline nigrostriatal dopaminergic neuronal density in female PD patients. Although the univariate linear regression analyses showed that lower skull HU values were associated with lower baseline DAT availability in the caudate, anterior putamen, and ventral striatum, these associations disappeared on multivariate linear regression analysis after adjusting for covariates. This was an unexpected result, considering the common pathomechanisms between osteoporotic bone density and dopaminergic neural loss. Previous studies have shown contradictory results regarding the association between osteoporosis and parkinsonian motor deficits that are closely coupled with dopaminergic neuronal density. Two studies showed the association between bone density and motor deficits in PD patients (Bezza et al., 2008; Kamanli et al., 2008), however, these studies had no adjustment for confounding factors. Moreover, Lorefält et al. (2007) failed to demonstrate significant relationship between bone mineral density and UPDRS-III score. Taken together, it appears that bone density is not a critical determinant of dopaminergic neural loss at the time of PD diagnosis. In postmenopausal osteoporotic patients, the detrimental effect of estrogen deficiency (Leranth et al., 2000) on nigrostriatal dopaminergic neurons could be counteracted with higher levels of osteocalcin (Kalaiselvi et al., 2013; Guo et al., 2018; Shan et al., 2019), wherein this offset effect may become gradually faint over time, since the deleterious effect of a diminished estrogen level exceeds the influence of osteocalcin (Kim et al., 2015; Jung et al., 2016). Given the complex relationship between estrogen, osteocalcin, bone mass, and nigrostriatal degeneration in PD, there is a need for its elucidation in future studies.
We found that baseline skull HU was not a significant predictor of the development of LID and wearing-off, but it was a significant predictor of longitudinal LED increase. Since skull HU was significantly correlated with age, and the relationship between aging and osteoporosis has been well established, we proceeded to perform an interaction analysis in the longitudinal model and found that there was a significant interaction effect between skull HU and age in the statistical model. Interestingly, subgroup analyses using the median split of age showed that only the patients with lower skull HU values had a greater risk of developing LID and wearing-off, and required more longitudinal LED over time in the relatively younger age group. Considering that the development of LID or wearing off is closely coupled with pre-and post-synaptic dopaminergic factors (Bezard et al., 2001; Cenci and Lundblad, 2006) and LED changes are indirectly associated with disease severity (Ayala et al., 2017), these findings provided indirect evidence of an age-dependent detrimental effect of osteoporosis on the disease progression of PD. Although the exact mechanism is uncertain, the common pathomechanisms underlying osteoporosis and PD may contribute to disease progression. Autophagy dysfunction, mitochondrial dysfunction, and inflammation play an important role in PD pathogenesis and have also been linked with bone health (Wang et al., 2016; Wang et al., 2020). In terms of age- dependent manner, we can find a clue in the distinct pathophysiology between postmenopausal osteoporosis and senile osteoporosis. Postmenopausal osteoporosis occurs between 50 and 65 years of age and is primarily due to estrogen deficiency, whereas senile osteoporosis develops after 70 years of age and is mainly due to the deficiency of mineral and vitamin and increased bone turnover (Hawkins et al., 2021). Considering that estrogen has been established as a protective hormone in PD (Ragonese et al., 2006; Lee et al., 2019), the low bone density in younger PD patients of this study may have been attributed to estrogen deficiency, which consecutively leads to unfavorable motor prognosis. Meanwhile, osteoporosis in the old age group may most likely be senile osteoporosis, which is not relevant to estrogen hormone and may have no effect on PD prognosis.
In this study, we enrolled a relatively large number of consecutive female patients with de novo PD, which may have minimized patient-sampling biases. A relatively long follow-up duration could provide more appropriate information about the long-term motor prognosis in PD patients. However, this study has some limitations. First, we investigated our hypothesis on the basis of a strong correlation between skull HU and L1 HU in healthy controls. Thus, further studies should investigate the association of osteoporosis with prognosis of PD using T-scores measured via DXA, which was previously established as the gold standard for osteoporosis diagnosis. Analysis on trajectory of bone density (either by DXA or CT-derived skull bone density) according to levodopa treatment can also be a future research topic of interest. Also, because bone densities in other body parts were not available in female PD patients in the present study, future studies are needed to show that the relationship between skull HU and L1 HU maintains in PD patients. Second, we assessed clinical progression based on the longitudinal changes in LED, which may have not accurately reflected the motor status of PD patients. Moreover, as this was a retrospective study, LID and wearing-off were determined based on medical records, which precluded definitive conclusions. Third, considering that menopause is one of the greatest risk factors for osteoporosis (Ji and Yu, 2015), the lack of information regarding menopause status could have acted as a confounding factor, although we attempted to minimize this effect by enrolling female PD patients with age ≥50, which is above the median age of natural menopause (Park et al., 2018). Fourth, this study did not consider physical activity, which is also an important preventive factor in both osteoporosis and motor prognosis in PD patients (Castrogiovanni et al., 2016; Schenkman et al., 2018). Finally, although the effect of drugs used for osteoporosis on PD patients remains unclear, information on osteoporosis treatment was unobtainable in this study, which may act as an unmeasured confounder. Nevertheless, our study at least provided a novel proof-of-concept that systemic bone loss measured using skull bone HU in this study may be associated with motor prognosis in female patients with PD, which need to be validated in larger prospective studies with consecutive DXA testing.
In conclusion, the present study demonstrated that baseline skull HU, which is easily obtainable in DAT scan, could be a predictor of longitudinal motor prognosis in young female PD patients. Further studies investigating whether treatment for osteoporosis can alter the clinical course of PD are warranted.
Data availability statement
The de-identified data that support the findings of this study are available from the corresponding author upon request. The data are not publicly available due to privacy or ethical restriction.
Ethics statement
The studies involving human participants were reviewed and approved by Yonsei University Severance Hospital Institutional Review Board (IRB No. 4-2014-0637). Written informed consent for participation was not required for this study in accordance with the national legislation and the institutional requirements.
Author contributions
SHJ and NH: conceptualization, data curation, formal analysis, investigation, methodology, resources, visualization, and writing—original draft. HSL: formal analysis and methodology. SH, Y-gL, and YL: data curation and investigation. YR and YHS: investigation and writing—review and editing. PHL: conceptualization, investigation, methodology, resources, writing—original draft, and writing—review and editing. All authors contributed to the article and approved the submitted version.
Funding
This work was supported by Basic Science Research Program through the National Research Foundation of Korea (NRF) funded by the Ministry of Science, ICT and Future Planning (NRF-2019R1A2C2085462).
Conflict of interest
The authors declare that the research was conducted in the absence of any commercial or financial relationships that could be construed as a potential conflict of interest.
Publisher’s note
All claims expressed in this article are solely those of the authors and do not necessarily represent those of their affiliated organizations, or those of the publisher, the editors and the reviewers. Any product that may be evaluated in this article, or claim that may be made by its manufacturer, is not guaranteed or endorsed by the publisher.
Footnotes
References
Alswat, K. A. (2017). Gender disparities in osteoporosis. J. Clin. Med. Res. 9, 382–387. doi: 10.14740/jocmr2970w
Ayala, A., Triviño-Juárez, J. M., Forjaz, M. J., Rodríguez-Blázquez, C., Rojo-Abuin, J.-M., and Martínez-Martín, P. (2017). Parkinson’s disease severity at 3 years can be predicted from non-motor symptoms at baseline. Front. Neurol. 8:551. doi: 10.3389/fneur.2017.00551
Bezard, E., Brotchie, J. M., and Gross, C. E. (2001). Pathophysiology of levodopa-induced dyskinesia: Potential for new therapies. Nat. Rev. Neurosci. 2, 577–588. doi: 10.1038/35086062
Bezza, A., Ouzzif, Z., Naji, H., Achemlal, L., Mounach, A., Nouijai, M., et al. (2008). Prevalence and risk factors of osteoporosis in patients with Parkinson’s disease. Rheumatol. Int. 28, 1205–1209. doi: 10.1007/s00296-008-0632-6
Braak, H., Del Tredici, K., Rüb, U., De Vos, R. A., Jansen Steur, E. N., and Braak, E. (2003). Staging of brain pathology related to sporadic Parkinson’s disease. Neurobiol. Aging 24, 197–211. doi: 10.1016/S0197-4580(02)00065-9
Castrogiovanni, P., Trovato, F. M., Szychlinska, M. A., Nsir, H., Imbesi, R., and Musumeci, G. (2016). The importance of physical activity in osteoporosis. From the molecular pathways to the clinical evidence. Histol. Histopathol. 31, 1183–1194.
Cenci, M. A., and Lundblad, M. (2006). Post- versus presynaptic plasticity in L-DOPA-induced dyskinesia. J. Neurochem. 99, 381–392. doi: 10.1111/j.1471-4159.2006.04124.x
Collier, T. J., Kanaan, N. M., and Kordower, J. H. (2017). Aging and Parkinson’s disease: Different sides of the same coin? Mov. Disord. 32, 983–990. doi: 10.1002/mds.27037
Feng, S. H., Huang, Y. P., Yeh, K. C., and Pan, S. L. (2021). Osteoporosis and the risk of Parkinson’s disease: A nationwide, propensity score-matched, longitudinal follow-up study. J. Clin. Endocrinol. Metab. 106, e763–e771. doi: 10.1210/clinem/dgaa864
Guo, X.-Z., Shan, C., Hou, Y.-F., Zhu, G., Tao, B., Sun, L.-H., et al. (2018). Osteocalcin ameliorates motor dysfunction in a 6-hydroxydopamine-induced Parkinson’s disease rat model through AKT/GSK3β signaling. Front. Mol. Neurosci. 11:343. doi: 10.3389/fnmol.2018.00343
Han, C., Jo, S. A., Jo, I., Kim, E., Park, M. H., and Kang, Y. (2008). An adaptation of the Korean mini-mental state examination (K-MMSE) in elderly Koreans: Demographic influence and population-based norms (the AGE study). Arch. Gerontol. Geriatr. 47, 302–310. doi: 10.1016/j.archger.2007.08.012
Hawkins, F., Garla, V., Allo, G., Males, D., Mola, L., and Corpas, E. (2021). “Chapter 5 - Senile and postmenopausal osteoporosis: Pathophysiology, diagnosis, and treatment,” in Endocrinology of aging, ed. E. Corpas (Amsterdam: Elsevier), 131–169. doi: 10.1016/B978-0-12-819667-0.00005-6
Hernlund, E., Svedbom, A., Ivergård, M., Compston, J., Cooper, C., Stenmark, J., et al. (2013). Osteoporosis in the European union: Medical management, epidemiology and economic burden. A report prepared in collaboration with the International Osteoporosis Foundation (IOF) and the European Federation of Pharmaceutical Industry Associations (EFPIA). Arch. Osteoporos. 8:136. doi: 10.1007/s11657-013-0136-1
Innis, R. B., Cunningham, V. J., Delforge, J., Fujita, M., Gjedde, A., Gunn, R. N., et al. (2007). Consensus nomenclature for in vivo imaging of reversibly binding radioligands. J. Cereb. Blood Flow Metab. 27, 1533–1539. doi: 10.1038/sj.jcbfm.9600493
Jang, S., Graffy, P. M., Ziemlewicz, T. J., Lee, S. J., Summers, R. M., and Pickhardt, P. J. (2019). Opportunistic osteoporosis screening at routine abdominal and thoracic CT: Normative L1 trabecular attenuation values in more than 20 000 adults. Radiology 291, 360–367. doi: 10.1148/radiol.2019181648
Jeong, S. H., Chung, S. J., Yoo, H. S., Hong, N., Jung, J. H., Baik, K., et al. (2021a). Beneficial effects of dipeptidyl peptidase-4 inhibitors in diabetic Parkinson’s disease. Brain 144, 1127–1137. doi: 10.1093/brain/awab015
Jeong, S. H., Lee, H. S., Jung, J. H., Baik, K., Lee, Y. H., Yoo, H. S., et al. (2021b). White matter hyperintensities, dopamine loss, and motor deficits in de novo Parkinson’s disease. Mov. Disord. 36, 1411–1419. doi: 10.1002/mds.28510
Ji, M.-X., and Yu, Q. (2015). Primary osteoporosis in postmenopausal women. Chronic Dis. Transl. Med. 1, 9–13. doi: 10.1016/j.cdtm.2015.02.006
Jung, K. Y., Kim, K. M., Ku, E. J., Kim, Y. J., Lee, D. H., Choi, S. H., et al. (2016). Age- and sex-specific association of circulating osteocalcin with dynamic measures of glucose homeostasis. Osteoporos. Int. 27, 1021–1029. doi: 10.1007/s00198-015-3315-7
Kalaiselvi, V. S., Prabhu, K., Ramesh, M., and Venkatesan, V. (2013). The association of serum osteocalcin with the bone mineral density in post menopausal women. J. Clin. Diagn. Res. 7, 814–816.
Kamanli, A., Ardicoglu, O., Ozgocmen, S., and Yoldas, T. K. (2008). Bone mineral density in patients with Parkinson’s disease. Aging Clin. Exp. Res. 20, 277–279. doi: 10.1007/BF03324774
Kim, J. E., Park, S. J., Kim, Y. C., Min, S. I., Ha, J., Kim, Y. S., et al. (2021). Deep learning-based quantification of visceral fat volumes predicts posttransplant diabetes mellitus in kidney transplant recipients. Front. Med. 8:632097. doi: 10.3389/fmed.2021.632097
Kim, M. Y., Im, S.-W., and Park, H. M. (2015). The demographic changes of menopausal and geripausal women in Korea. J. Bone Metab. 22, 23–28. doi: 10.11005/jbm.2015.22.1.23
Lee, J. E., Choi, J.-K., Lim, H. S., Kim, J. H., Cho, J. H., Kim, G. S., et al. (2017). The prevalence and incidence of Parkinson’s disease in South Korea: A 10-year nationwide population–based study. J. Korean Neurol. Assoc. 35, 191–198. doi: 10.17340/jkna.2017.4.1
Lee, Y. H., Cha, J., Chung, S. J., Yoo, H. S., Sohn, Y. H., Ye, B. S., et al. (2019). Beneficial effect of estrogen on nigrostriatal dopaminergic neurons in drug-naïve postmenopausal Parkinson’s disease. Sci. Rep. 9:10531. doi: 10.1038/s41598-019-47026-6
Lee, Y. S., Hong, N., Witanto, J. N., Choi, Y. R., Park, J., Decazes, P., et al. (2021). Deep neural network for automatic volumetric segmentation of whole-body CT images for body composition assessment. Clin. Nutr. 40, 5038–5046. doi: 10.1016/j.clnu.2021.06.025
Leranth, C., Roth, R. H., Elsworth, J. D., Naftolin, F., Horvath, T. L., and Redmond, D. E. (2000). Estrogen is essential for maintaining nigrostriatal dopamine neurons in primates: Implications for Parkinson’s disease and memory. J. Neurosci. 20, 8604–8609. doi: 10.1523/JNEUROSCI.20-23-08604.2000
Lorefält, B., Toss, G., and Granérus, A. K. (2007). Bone mass in elderly patients with Parkinson’s disease. Acta Neurol. Scand. 116, 248–254. doi: 10.1111/j.1600-0404.2007.00875.x
Na, M. K., Won, Y. D., Kim, C. H., Kim, J. M., Cheong, J. H., Ryu, J. I., et al. (2018). Opportunistic osteoporosis screening via the measurement of frontal skull Hounsfield units derived from brain computed tomography images. PLoS One 13:e0197336. doi: 10.1371/journal.pone.0197336
Oh, J. S., Oh, M., Chung, S. J., and Kim, J. S. (2014). Cerebellum-specific 18F-FDG PET analysis for the detection of subregional glucose metabolism changes in spinocerebellar ataxia. Neuroreport 25, 1198–1202. doi: 10.1097/WNR.0000000000000247
Oh, M., Kim, J. S., Kim, J. Y., Shin, K. H., Park, S. H., Kim, H. O., et al. (2012). Subregional patterns of preferential striatal dopamine transporter loss differ in Parkinson disease, progressive supranuclear palsy, and multiple-system atrophy. J. Nucl Med. 53, 399–406. doi: 10.2967/jnumed.111.095224
Park, C. Y., Lim, J. Y., and Park, H. Y. (2018). Age at natural menopause in Koreans: Secular trends and influences thereon. Menopause 25, 423–429. doi: 10.1097/GME.0000000000001019
Park, J., Koh, S.-B., Kwon, K.-Y., Kim, S. J., Kim, J. W., Kim, J.-S., et al. (2020). Validation study of the official korean version of the movement disorder society-unified Parkinson’s disease rating scale. J. Clin. Neurol. 16, 633–645. doi: 10.3988/jcn.2020.16.4.633
Pompe, E., De Jong, P. A., De Jong, W. U., Takx, R. A., Eikendal, A. L., Willemink, M. J., et al. (2016). Inter-observer and inter-examination variability of manual vertebral bone attenuation measurements on computed tomography. Eur. Radiol. 26, 3046–3053. doi: 10.1007/s00330-015-4145-x
Poole, K. E., Mayhew, P. M., Rose, C. M., Brown, J. K., Bearcroft, P. J., Loveridge, N., et al. (2010). Changing structure of the femoral neck across the adult female lifespan. J. Bone Miner. Res. 25, 482–491. doi: 10.1359/jbmr.090734
Ragonese, P., D’amelio, M., Callari, G., Salemi, G., Morgante, L., and Savettieri, G. (2006). Age at menopause predicts age at onset of Parkinson’s disease. Mov. Disord. 21, 2211–2214. doi: 10.1002/mds.21127
Scheltens, P., Barkhof, F., Leys, D., Pruvo, J. P., Nauta, J. J., Vermersch, P., et al. (1993). A semiquantative rating scale for the assessment of signal hyperintensities on magnetic resonance imaging. J. Neurol. Sci. 114, 7–12. doi: 10.1016/0022-510X(93)90041-V
Schenkman, M., Moore, C. G., Kohrt, W. M., Hall, D. A., Delitto, A., Comella, C. L., et al. (2018). Effect of high-intensity treadmill exercise on motor symptoms in patients with de novo Parkinson disease: A phase 2 randomized clinical trial. JAMA Neurol. 75, 219–226. doi: 10.1001/jamaneurol.2017.3517
Shan, C., Ghosh, A., Guo, X.-Z., Wang, S.-M., Hou, Y.-F., Li, S.-T., et al. (2019). Roles for osteocalcin in brain signalling: Implications in cognition- and motor-related disorders. Mol. Brain 12:23. doi: 10.1186/s13041-019-0444-5
Syed, F. A., Oursler, M. J., Hefferanm, T., Peterson, J. M., Riggs, B. L., and Khosla, S. (2008). Effects of estrogen therapy on bone marrow adipocytes in postmenopausal osteoporotic women. Osteoporos. Int. 19, 1323–1330. doi: 10.1007/s00198-008-0574-6
Tomlinson, C. L., Stowe, R., Patel, S., Rick, C., Gray, R., and Clarke, C. E. (2010). Systematic review of levodopa dose equivalency reporting in Parkinson’s disease. Mov. Disord. 25, 2649–2653. doi: 10.1002/mds.23429
Torsney, K. M., Noyce, A. J., Doherty, K. M., Bestwick, J. P., Dobson, R., and Lees, A. J. (2014). Bone health in Parkinson’s disease: A systematic review and meta-analysis. J. Neurol. Neurosurg. Psychiatry 85, 1159–1166. doi: 10.1136/jnnp-2013-307307
Treece, G. M., Gee, A. H., Mayhew, P. M., and Poole, K. E. (2010). High resolution cortical bone thickness measurement from clinical CT data. Med. Image Anal. 14, 276–290. doi: 10.1016/j.media.2010.01.003
Wang, B., Abraham, N., Gao, G., and Yang, Q. (2016). Dysregulation of autophagy and mitochondrial function in Parkinson’s disease. Transl. Neurodegener. 5:19. doi: 10.1186/s40035-016-0065-1
Keywords: Parkinson’s disease, osteopenia/osteoporosis, dopamine transporter (DAT) imaging, prognosis, skull bone density
Citation: Jeong SH, Hong N, Lee HS, Han S, Lee Y-g, Lee Y, Rhee Y, Sohn YH and Lee PH (2022) Low skull bone density is associated with poor motor prognosis in women with Parkinson’s disease. Front. Aging Neurosci. 14:1053786. doi: 10.3389/fnagi.2022.1053786
Received: 26 September 2022; Accepted: 27 October 2022;
Published: 15 November 2022.
Edited by:
Brian C. Clark, Ohio University, United StatesReviewed by:
Wen-Cheng Xiong, Case Western Reserve University, United StatesRandee Hunter, The Ohio State University, United States
Copyright © 2022 Jeong, Hong, Lee, Han, Lee, Lee, Rhee, Sohn and Lee. This is an open-access article distributed under the terms of the Creative Commons Attribution License (CC BY). The use, distribution or reproduction in other forums is permitted, provided the original author(s) and the copyright owner(s) are credited and that the original publication in this journal is cited, in accordance with accepted academic practice. No use, distribution or reproduction is permitted which does not comply with these terms.
*Correspondence: Phil Hyu Lee, phlee@yuhs.ac
†These authors have contributed equally to this work and share first authorship