- 1Department of Anesthesiology, The Third Central Clinical College of Tianjin Medical University, Tianjin, China
- 2Department of Anesthesiology, Nankai University Affinity the Third Central Hospital, Tianjin, China
- 3Tianjin Key Laboratory of Extracorporeal Life Support for Critical Diseases, Tianjin, China
- 4Artificial Cell Engineering Technology Research Center, Tianjin, China
- 5Tianjin Institute of Hepatobiliary Disease, Tianjin, China
Background and objective: Postoperative neurocognitive dysfunction (PND) occurs in up to 54% of older patients, giving rise to the heavy psychological and economic burdens to patients and society. To date, the development of PND biomarkers remains a challenge. Heterogeneous nuclear ribonucleoprotein A2/B1 (hnRNPA2/B1) is an RNA-binding protein whose prion-like structure is prone to mutation and hence leads to neurodegenerative diseases, but its expression changes in PND remains unclear. Here, we detect the preoperative hnRNPA2/B1 level in patients with PND, and to explore its value in the prediction and diagnosis of PND.
Methods: The study included 161 elderly patients undergoing lumbar decompression and fusion in Nankai University Affinity the Third Central Hospital from September 2021 to July 2022. Neuropsychological and psychometric evaluations were performed before surgery, 1 week and 3 months after surgery to diagnose the occurrence of PND, then the peripheral blood was collected from patients before induction of anesthesia. The concentration in plasma of hnRNPA2/B1 and amyloid-β 42 were determined by enzyme-linked immunosorbent assay. The median fluorescence intensity and mRNA levels of hnRNPA2/B1 in peripheral blood mononuclear cells was detected by indirect intracellular staining flow cytometry and quantitative real-time PCR, respectively.
Results: The preoperative hnRNPA2/B1 level in patients with PND was higher both in short-time and long-time follow-up. We found significantly higher concentrations of hnRNPA2/B1 in PND at 7 days after surgery (median, 72.26 pg/mL vs. 54.95 pg/mL, p = 0.022) compared with patients without PND, and so as 3 months after surgery (median, 102.93 pg/mL vs. 56.38 pg/mL, p = 0.012). The area under the curve (AUC) was predicted to be 0.686 at 7 days after surgery and 0.735 at 3 months. In addition, when combining several clinical information, the diagnostic efficiency of hnRNPA2/B1 for PND could further increase (AUC, 0.707 at 7 days, 0.808 at 3 months).
Conclusion: Based on the findings reported here, hnRNPA2/B1 may serve as a new and powerful predictive biomarker to identify elderly patients with PND.
Introduction
Postoperative neurocognitive dysfunction (PND) is a cognition-related complication, whose incidence fluctuates from 20 to 54% and increases with age (Needham et al., 2017; Carr et al., 2018; Evered and Silbert, 2018). This postoperative outcome is also independently associated with some adverse effects, including increased surgical morbidity and mortality, length of hospital stay, hospitalization and out-of-hospital care costs and functional disability (Dijkstra et al., 1999; Steinmetz et al., 2009; O’Gara et al., 2020). Considering these outcomes caused by PND, it is, therefore, vital to recognize individuals prone to PND as early as possible.
Exploring PND biomarkers would help clinicians stratify patients by risk and conduct individualized management, and such biomarkers could also provide clues to help elucidate the mechanisms behind PND. Currently, the majority of the detected PND biomarkers are related to nerve damage, neurotoxicity, astrocyte injury or neurotrophic effect. One potential PND marker is neuron-specific enolase (NSE), though considered as a reliable indicator of neuronal and brain damage, but it works not very well in the early stage of brain injury (Ramlawi et al., 2006). Its diagnostic sensitivity could be reduced for its non-specificity to brain tissue, then causing a high prevalence of false-positive results and even a reverse gradient change (Rappold et al., 2016; Danielson et al., 2018). Glial fibrillary acidic protein (GFAP), a cytoskeletal protein in astrocytes and highly specific to brain, is associated with the incidence and severity of PND (Rappold et al., 2016), but Ballweg et al. (2021) found that the receiver operating characteristic (ROC) curve of GFAP to diagnose PND was only 0.49. Therefore, there remains no standard biomarkers for diagnosis and prognosis of PND.
Considering the accelerated aging of the population, incremental number of operations and consequent increasing incidence of PND, we urgently need accurate biomarkers to identify high-risk PND populations. Heterogeneous nuclear ribonucleoprotein (hnRNP) A2/B1 is an important RNA-binding protein that participate in various processes of nucleic acid metabolism, and its expression changes and mutations are closely related to neurodegenerative diseases (Zhang et al., 2021). Our previous studies have found the up-regulation of hnRNPA2/B1 in hippocampal neurons would induce the cognitive impairment of rats (Wang et al., 2020). Despite hnRNPA2/B1 has been confirmed as a significant role in the development of neurodegenerative disorders, especially amyotrophic lateral sclerosis (ALS) and Alzheimer’s disease (AD), the relationship between hnRNPA2/B1 and PND remains unclear.
It’s reported that PND shares several common molecular pathways with dementia, including neuroinflammation (Le et al., 2014; Luo et al., 2019), oxidative stress (Netto et al., 2018), impaired synaptic function (Xiao et al., 2018; Gao et al., 2021), and the microbiota–gut-brain axis (Yang et al., 2018; Jiang et al., 2019). These pathologic changes may provide physiological basis to the conversion from PND to AD (Steinmetz et al., 2009; Evered et al., 2016), the latter resulting much heavier economic burden. Therefore, we explored the hnRNPA2/B1 level in elderly patients and confirmed the potential predictive value of it to PND so that more high-risk population of PND could be identified.
Materials and methods
Sample size
Based on the results of hnRNPA2/B1 predictive to ALS and the reported incidence of PND, the ratio between the PND and non-PND arms was predefined in the range of 1:3 to make age and gender reveal no differences between groups, and 140 patients were presumably required for the study (α = 0.05; 1−β = 0.9). Allowing an attrition rate of 10–20%, we would recruit 161 participants finally.
Participants
After obtaining the approval from the Ethics Committee of Nankai University Affinity the Third Central Hospital and the written informed consents from all patients, 161 consecutively admitted patients, with the age of more than 65 years, undergoing lumbar decompression and fusion within 4 h from September 2021 to July 2022 were enrolled in the study [SZX-IRB-SOP-016(F)-002-02]. The exclusion criteria were as follows: critical illness (preoperative ASA ≥ IV); history of mental illness or neurological disorders; intake of tranquilizers or antidepressants; suspected dementia or memory impairment with a score on the mini-mental state examination (MMSE) < 17 or Montreal Cognitive Assessment (MoCA) < 15; cancer; unwilling to comply with the procedures; or hearing loss.
Regarding the intraoperative anesthetic management, routine electrocardiogram, pulse oximetry, invasive blood pressure and bispectral index (BIS) were continuously monitored after the patients admitted to the operating room. All patients undergoing lumbar decompression and fusion surgery received general anesthesia with midazolam (0.05 mg/kg), sufentanil (0.3 μg/kg), etomidate (0.3 mg/kg), Cisatracurium (0.2 mg/kg), and was maintained with continuous infusion of propofol (1.2 μg/ml) and remifentanil (0.1–0.4 μg/kg/min) + 0.7 MAC sevoflurane during surgery to maintain the BIS value within 40–60. Patients under poor hemodynamic conditions should be treated timely. Additionally, all patients received postoperative analgesia via a patient-controlled analgesia device for the initial 48 h after surgery.
Cognition assessment
Neuropsychological tests were assessed pre-operatively (the day before the operation), 7 days and 3 months after surgery. The battery primarily focused on memory, learning, attention, executive functions, and cognitive flexibility, and included the following tests: MMSE, MoCA, Instrumental Activities of Daily Living (IADL) as well as Clinical Dementia Rating (CDR). Mild cognitive impairment (MCI) was identified in terms of education-specific cutoff points of total scores of MMSE and MoCA, respectively. According to MMSE norms, 17∼27 for illiterate individuals, 20∼27 for participants with elementary school education, and 24∼27 for those with middle school education and above (Mitchell, 2009). MoCA score in the range of 15∼24 indicates no dementia and below the threshold for diagnosis of AD (Memoria et al., 2013) and CDR is equal to 0.5. Patients with MCI would be enrolled in the study and then followed for 3 months.
According to the definition of PND that the neurocognitive results drop over one SD from baseline when following up (Newman et al., 2001), participants were evaluated whether have PND or not and then divided into PND1 or non-PND1 according to the follow-up results of 1 week after surgery, and PND2 or non-PND2 at 3 months after surgery.
Sample preparation
The blood samples were collected in EDTA-K2 anti-coagulation vacuum tubes before the induction of anesthesia and divided into three parts, applied to FCM, ELISA, and qRT-PCR. After centrifuging at 3,000 × g for 10 min, the plasma samples were stored at −80°C until analysis. Furthermore, the PBMCs were extracted using Lymphocyte Separation Medium (Human) (P8610, Solarbio, Beijing, China), washed with 1 × PBS (FZ1258, Solarbio, Beijing, China) 3 times totally and then flow cytometry and qRT-PCR are conducted.
Flow cytometry
After harvesting and suspending the PBMCs, add cell fixation and permeabilization (00-5523-00, Thermo Fisher Scientific Inc., Waltham, MA, USA), anti-hnRNPA2/B1 primary antibody (ab259894, Abcam, Toronto, ON, Canada) or Rabbit Monoclonal IgG (ab172730, Abcam, Toronto, ON, Canada) as isotype control and Goat F(ab’)2 Anti-Rabbit IgG (DyLight® 488, ab98507, Abcam, Toronto, ON, Canada) as the secondary antibody and incubate, respectively, at room temperature in the dark for about 30–60 min. Wash the cells 3 times by centrifugation at 400 × g for 5 min and resuspend after each incubation. Resuspend cells in 1% paraformaldehyde to prevent deterioration at last for extended storage as well as greater flexibility in planning time on the cytometer. When gating on cell populations by Flowjo data analysis software (TreeStar, USA), we would obtain the median fluorescence intensity of lymphocytes and monocytes to analyze the difference between groups.
Enzyme linked immunosorbent assay
Concentrations of hnRNPA2/B1 (SBJ-H2321, SenBeiJia, Jiangsu, China) and Aβ42 (E-EL-H543c, Elabscience, Houston, USA) were examined by ELISA kit. The kit and plasma samples were balanced to room temperature half an hour before use, and the operation is fully carried out following the manufacturer’s instructions. Notably, it should be detected within 15 min after adding the stop solution. The absorbance was read on a spectrophotometer (PerklnElmer, Waltham, MA, USA) at a wavelength of 450 nm. The concentrations of hnRNPA2/B1 and Aβ42 were calculated according to the standard curve and presented as pg/mg protein.
Quantitative real-time polymerase chain reaction
Total RNA was extracted from PBMC using the TRIzol reagent (15596026, Thermo Fisher Scientific Inc., Waltham, MA, USA) and its concentration was measured by spectrophotometer (Thermo Fisher Scientific, Waltham, MA, USA). The complementary DNA (cDNA) was obtained by the reverse transcription kit (RR036A, Takara, Japan) and then transcribed to mRNA using the SYBR Premix Ex Taq II (RR820A, Takara, Japan) on an ABI 7,500 instrument (Applied Biosystems, Foster City, CA, USA), with 3 duplicates set in each well. The mRNA levels were normalized to β-actin and the fold changes were calculated using the method of 2–ΔΔCt. The nucleotide sequences of the PCR primers (Sangon Biotech, Shanghai, China) are as follows:
β-actin mRNA: (forward 5′-CAC CAT TGG CAA TGA GCG GTT C -3′
reverse 5′-AGG TCT TTG CGG ATG TCC ACG T-3′)
hnRNPA2/B1 mRNA: (forward 5′- GCT TAA GCT TTG AAA CCA CAG A -3′
reverse 5′-CTT GAT CTT TTG CTT GCA GGA T-3′).
Statistical analysis
Descriptive results of continuous variables were presented as the means ± standard deviations (SD) if data follow a normal distribution and homogeneity of variance, otherwise median with interquartile range (IQR). Categorical data were expressed as a percentage or counts. Intergroup comparisons were compared by the independent sample t-test or Kruskal-Wallis H test. For comparisons of qualitative parameters, Chi-square or Fisher exact test was applied. Multivariate logistic regression analysis was used to determine the independent risk factors of PND based on the results of previous univariate analysis. Results were shown as odds ratio (OR) and 95% CI. Additionally, we built prediction models for the occurrence of PND and then evaluated its prediction effect by the ROC curve on the basis of the results of ELISA. All statistical analysis were performed using the SPSS 25.0 software (IBM Corp. Armonk, NY, USA), and P < 0.05 was considered to be statistically significant.
Results
Patients
Among the eligible 161 patients, 138 were included in the final data analyses (Figure 1). Postoperative short-term follow-up results showed that, no clinically statistical differences were observed with regard to the gender, BMI, ASA classification, hypertension, coronary artery heart disease, and duration of anesthesia, while patients with PND presented lower education level, longer duration of surgery and were subject to receive blood transfusion during surgery compared to those without PND. Postoperative results of long-term follow-up showed no difference in baseline information between two groups (Table 1, P < 0.05). Moreover, pre-/post-operative scores of neuropsychological tests also displayed difference between groups (Table 2, P < 0.05).
Preoperative heterogeneous nuclear ribonucleoprotein A2/B1 level increases in patients with postoperative neurocognitive dysfunction
Both the median fluorescence intensity and mRNA of preoperative hnRNPA2/B1 levels in the PND group were significantly higher than the other one. Besides, the median fluorescence intensity of hnRNPA2/B1 in monocytes was higher than that in lymphocytes (Figure 2). The preoperative hnRNPA2/B1 and Aβ42 levels in the PND group were significantly higher than that in the non-PND group, except for preoperative Aβ42 level at 7 days after surgery (Figure 3 and Table 3 P < 0.05). Moreover, we analyzed the sex differences of two biomarkers, hnRNPA2/B1 as well as Aβ42, and found no sex differences in both plasma biomarkers (Supplementary Table).
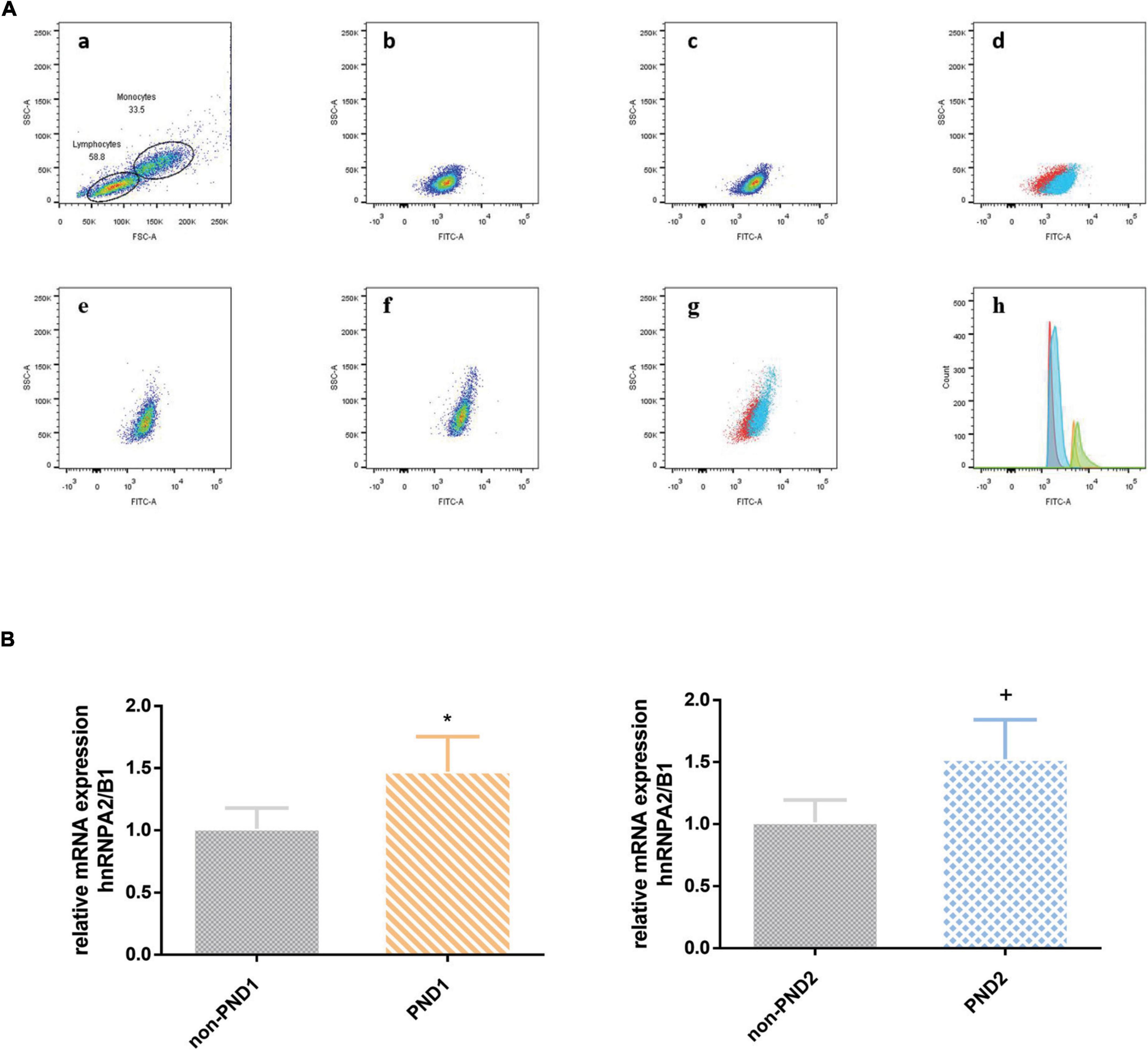
Figure 2. (A) The expression of hnRNPA2/B1 in PBMC was analyzed by FCM. The effect regions were created for FSC and SSC (a); median fluorescence intensity of hnRNPA2/B1 in lymphocytes between PND and non-PND (b,c), and the overlay of two groups (d; PND, blue; non-PND, red); median fluorescence intensity of hnRNPA2/B1 in monocytes between PND and non-PND (e,f), and the overlay of two groups (G; PND, blue; non-PND, red); histogram overlay of hnRNP A2/B1 expression in patients with PND compared to patients without PND in different cell population (h; lymphocytes of non-PND, red; lymphocytes of PND, blue; monocytes of non-PND, orange; monocytes of PND, green). (B) mRNA level analysis of hnRNPA2/B1 in PBMCs. Data are expressed as the mean ± SD. Note that mRNA expression of hnRNPA2/B1 in patients with PND significantly increased compared with those without PND. *p < 0.05 compared with group Non-PND1. +p < 0.05 compared with group Non-PND2.
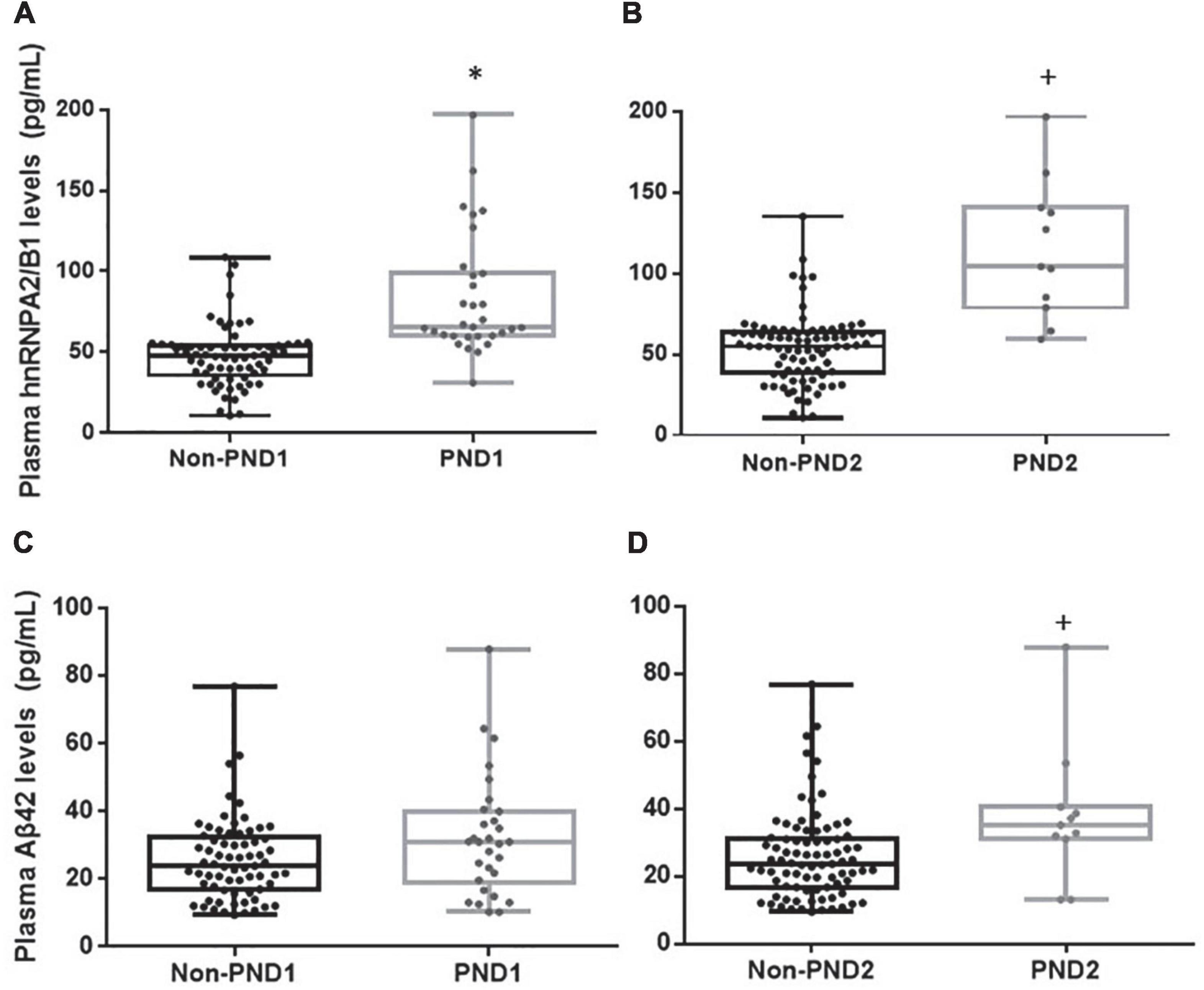
Figure 3. Plasma hnRNPA2/B1, Aβ42. Concentrations of hnRNPA2/B1 or Aβ42 in patients with or without PND at 7 days (A,C) and 3 months (B,D) after surgery. Boxes represent the median, the 25th and 75th percentiles, and bars indicate the range of data distribution. *p < 0.05 compared with group Non-PND1.+p < 0.05 compared with group Non-PND2.
Heterogeneous nuclear ribonucleoprotein A2/B1 is able to early predict the occurrence of postoperative neurocognitive dysfunction and has high diagnostic efficiency
As shown within Figure 4, univariate linear regression analysis showed that hnRNPA2/B1 had a stronger negative correlation with preoperative MoCA scores (R = −0.72, p < 0.001) than Aβ42 (R = −0.53, p < 0.001). The similar results could also be found in the correlation with preoperative MMSE scores (R = −0.50 in hnRNPA2/B1, R = −0.35 in Aβ42, p < 0.001).
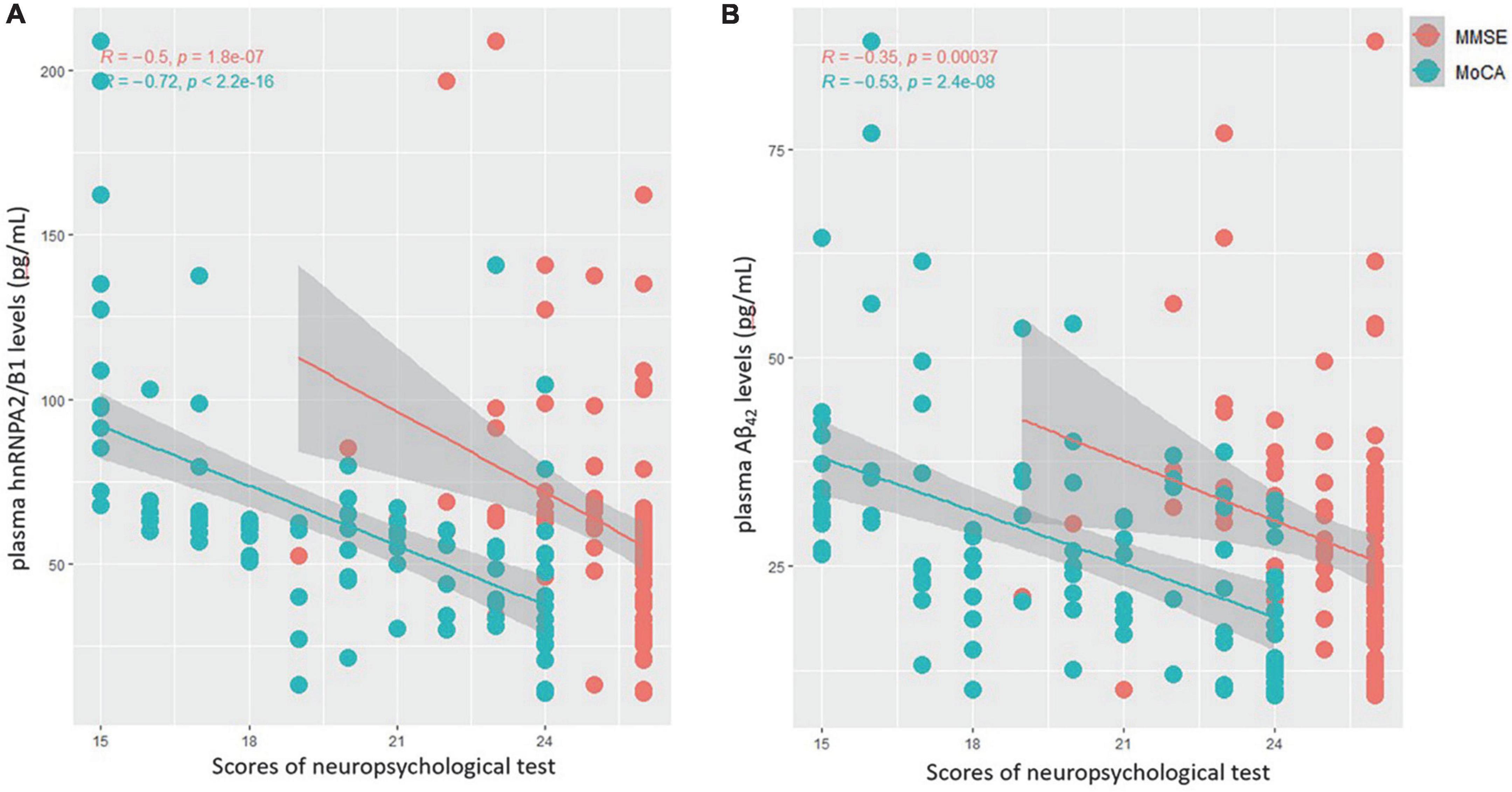
Figure 4. (A) Correlations between plasma hnRNPA2/B1 levels and MMSE(red) as well as MoCA(green) scores. (B) Correlations between plasma Aβ42 levels and MMSE(red) as well as MoCA(green) scores.
According to the ROC curve of PND1, the cut-off value (59.26 pg/mL, sen: 0.687, spe: 0.649) of preoperative hnRNPA2/B1 level was determined by Youden Index, and the AUC was 0.686 (95% CI 0.568∼0.804). The cut-off value (30.48 pg/mL, sen: 0.567, spe:0.714) of preoperative Aβ42 level was determined by Youden Index, and the AUC was 0.616 (95% CI 0.489∼0.744). In PND2, the cut-off value (75.53 pg/mL, sen:0.882, spe:0.636) of preoperative hnRNPA2/B1 level was determined by Youden Index, and the AUC was 0.735 (95% CI 0.525∼0.945). The cut-off value (31.10 pg/mL, sen:0.818, spe:0.747) of preoperative Aβ42 level was determined by Youden Index, and the AUC was 0.728 (95% CI 0.554∼0.903). In addition, combination hnRNPA2/B1 and Aβ42 could further increase the specificity of predictive value but the sensitivity of model did not improve. In the longer follow-up duration, the efficacy of these two biomarkers and their combination all increased a lot (Figure 5 and Table 4). To determine whether preoperative hnRNPA2/B1 level was an independent risk factor for PND, a multivariable logistic regression analysis was performed. The results showed that the preoperative hnRNPA2/B1 level was an independent risk factor of PND (Table 5). Finally, the model’s predictive effect was examined by the ROC curve (Figure 6).
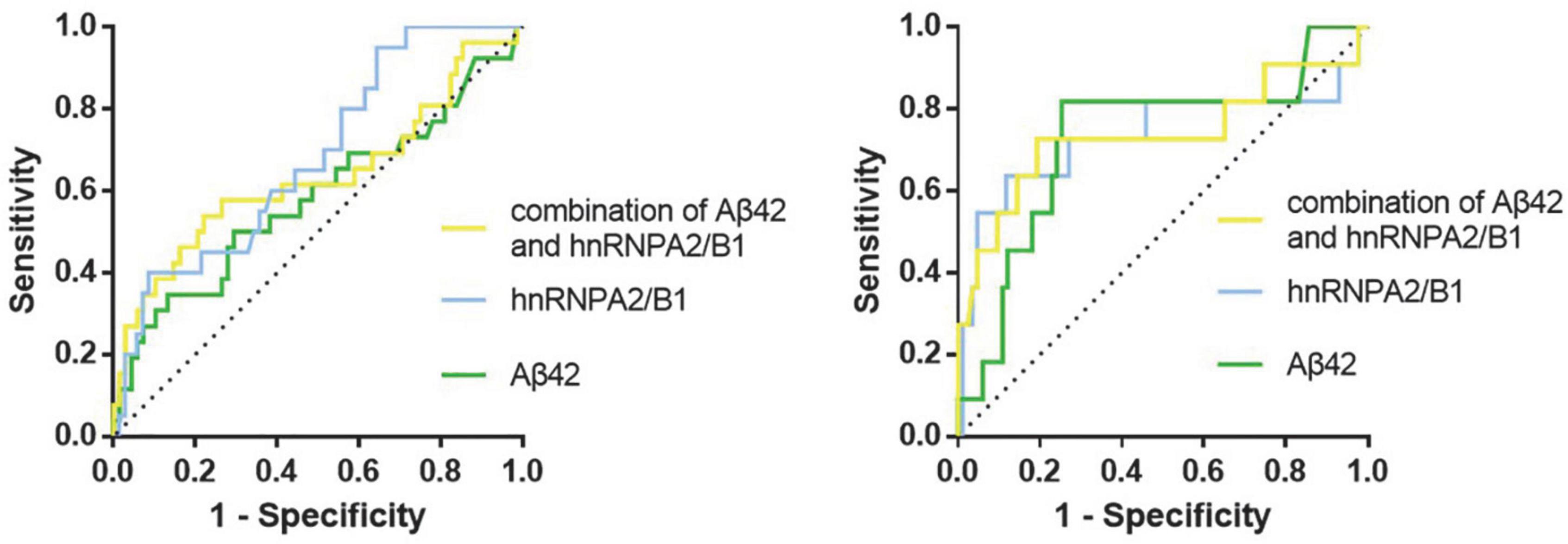
Figure 5. ROC curves for preoperative biomarkers. The figure showed the ROC curves for hnRNPA2/B1, Aβ42 and their combination at 7 days (Left) and 3 months (Right) after surgery, respectively.
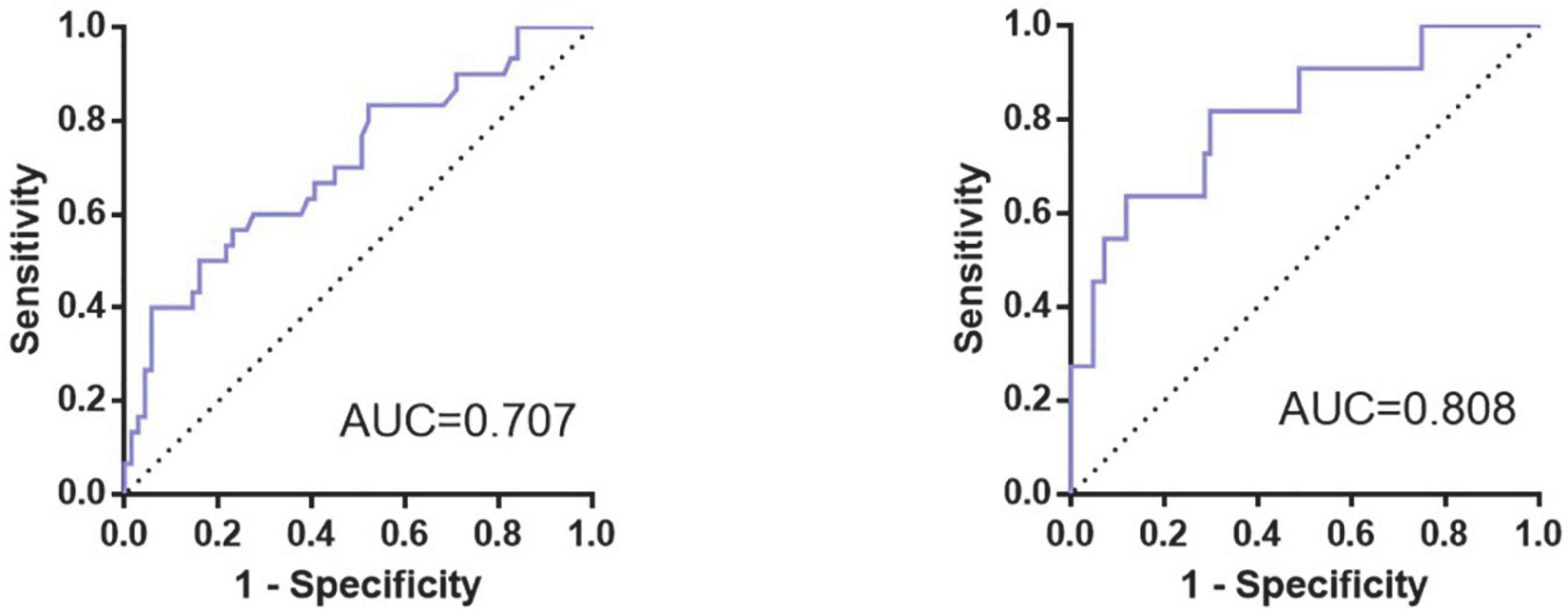
Figure 6. ROC curve for prediction model of occurrence of PND. The figure showed the ROC curves for the prediction model of PND at 7 days (Left) and 3 months (Right) after surgery, whose area under the curve (AUC) were 0.707 and 0.808, respectively.
Conclusion
Preoperative higher level of hnRNPA2/B1 is relevant to a higher risk of PND in patients after lumbar decompression and fusion. Therefore, it seems that hnRNPA2/B1 could be a clinically valuable biomarker to predict PND and patients with a higher preoperative hnRNPA2/B1 should be placed more emphasis. Considering that the number of the elderly who need surgical therapy is growing, more extensive clinical and basic studies are urgently needed to ensure our findings as well as further clarify the potential mechanisms.
Discussion
We identified PND in 31.3% of patients at 7 days after lumbar decompression and fusion and 10.9% at 3 months after surgery. Patients with higher preoperative hnRNPA2/B1 level tend to present cognitive decline for a more extended time after operation (median, 72.26 pg/mL in PND1, 102.93 pg/mL in PND2). High hnRNPA2/B1 was a significant predictor of PND and the cut-off value for hnRNPA2/B1 was 75.53 pg/mL (AUC, 0.735; 95% CI 0.525∼0.945), whose predictive value could improve further with the consideration of individual demographic data at baseline. Therefore, hnRNPA2/B1 is expected to become a new and powerful predictive marker to identify elderly patients with PND.
Current theories on the etiology of PND now include surgery-related factors such as surgery time, patient position and postoperative infection; anesthesia-related factors such as anesthesia method and intraoperative hypotension; and patient-related factors such as age and physical state. By analyzing several basic characteristics of enrolled patients, we found that there was significant difference in history of intraoperative blood transfusion, educational attainment, preoperative comorbidity, and the duration of surgery and anesthesia.
It’s generally believed that educational attainment can predict postoperative neurocognitive disorders to some degree, an effect that can be attributed to cognitive reserve as well as brain connectivity (Monk et al., 2008; Arenaza-Urquijo et al., 2013; Feinkohl et al., 2017b; Perry et al., 2017), which is in line with our findings. And we observed no clinically meaningful difference in age though there is no doubt that aging itself is the major independent risk factor for age-associated disorders. We think that’s because the age distribution of patients enrolled in our study mainly concentrated in the narrow age group of 65∼70 years old, some survey about the shifting architecture of cognition across the adult life span shows that compared to other age groups, there is no significant decline in long-term memory and working memory between 60 and 70 years old (Park and Reuter-Lorenz, 2009).
We also investigated the association of some preoperative comorbidities with the risk of PND, such as hypertension, diabetes, coronary artery heart disease. We found significant association for diabetes but not for hypertension or coronary artery heart disease with PND risk, which is consistent with the results of some studies (Feinkohl et al., 2017a,2018; Lachmann et al., 2018). Several lines of evidence suggest that its possible mechanism may be the changes in brain structure and function, such as impaired glucose metabolism, cerebral atrophy, cerebrovascular damage (Moran et al., 2019; van Sloten et al., 2020; Krell-Roesch et al., 2021). As for other preoperative complications, our findings differ from some research that a range of cardiovascular conditions, including hypertension and coronary artery heart disease, would attribute to cognitive dysfunction induced by cerebral small vessel lesions (Liu et al., 2018; Iadecola and Gottesman, 2019). We consider that is because majority of participants could take medicine punctually to monitor their blood pressure regularly, and antihypertensive agents are significantly associated with a lower risk of dementia or cognitive impairment (Hughes et al., 2020; de Menezes et al., 2021). Of course, there is another possibility that we should have assessed the severity of hypertension and duration of diagnosis which may be important to cognitive risk prediction.
In terms of surgical information, we analyzed the relationship of operative duration and history of intraoperative blood transfusion with PND. Lumbar decompression and fusion is a major operation with extensive trauma, long duration and large amount of intraoperative blood loss, resulting in various postoperative complications (Heemskerk et al., 2021). Blood transfusion is a lifesaving treatment, which can provide volume expansion and increase oxygen-carrying capacity. Nevertheless, transfusion of allogeneic blood products can also trigger enhanced acute inflammatory responses (Cata et al., 2013). We showed that history of intraoperative blood transfusion may contribute to short-term cognitive decline after surgery, while not to long-term cognitive performance. Several lines of findings show that some inflammatory factors would remarkably elevate due to blood transfusion, such as tumor necrosis factor (TNF)-α, interleukin (IL)-β, IL-6, and IL-8, and then lead to neurocognitive impairment (Urner et al., 2012; Ferraris et al., 2013). In addition, transfusion of old red blood cells (>14 days) frequently occurs in clinical practice, inducing neuroinflammation and the development of PND via cell-free hemoglobin (Zhu et al., 2014; Tan et al., 2015). Surgery-induced cognitive decline may be attributed to the microglial activation, and the latter would result the release of inflammatory factors and neuroendocrine hormone (Terrando et al., 2011; Subramaniyan and Terrando, 2019; Xiao et al., 2020). Therefore, longer duration of operation is associated with a stronger inflammatory response. Moreover, we found this perioperative stress doesn’t seem to cause long-term cognitive deficit.
Apart from paying attention to the baseline information and clinical data, we also explored the biomarkers of PND, aiming to provide guidance for clinical perioperative brain protection, and even promote the screening of patients before admission to hospital and facilitate the process of brain health.
PND has been demonstrated to be associated with multiple factors, among which the theories of neuroinflammation and oxidative stress may be the mainstream. In recent years, there are mounting studies about the association between hnRNPA2/B1 and the exacerbation of cognitive performance (Mizukami et al., 2005; Shorter and Taylor, 2013; Lorente Pons et al., 2020). HnRNPA2/B1 could affect cognitive function through alternative splicing and hence lead to the over-expression of β-secretase 1, and which could increase the accumulation of amyloid precursor protein and soluble Aβ1–42 in cytoplasm and promote the phosphorylation of tau protein (Kolisnyk et al., 2017; Zhang et al., 2021). These pathological changes not only damage the neurons directly, but also indirectly trigger neuroinflammation by interacting synergistically with glial cells, ultimately making neurodegenerative lesion stabile and irreversible. What’s more, the prion-like domains (PrLD) of hnRNPA2/B1 are the critical components that drive liquid–liquid phase separation (LLPS) and subsequently contribute stress granule (SG) to the hydrogel phase transition (Lee et al., 2016), while the latter is the key mechanism of conversion from neurodegeneration to dementia in the elderly (Lu et al., 2020; Rossi et al., 2020). Consistently, we found that the elderly with PND usually had a higher preoperative hnRNPA2/B1 level and this biomarker could provide preferable specificity and sensitivity to predict PND. In summary, our finding that hnRNPA2/B1 combined with Aβ42 could further increase the predictive value is encouraging as it could accurately identify people at high risk of PND.
As for the logistic multivariable regression and the prediction model, we only took preoperative hnRNPA2/B1 and Aβ42 level, intraoperative blood transfusion event, educational level, diabetes, duration of surgery and age into consideration due to the size of the sample, the former six factors are significantly different in our single variable analysis. While age has been reported as comorbidities of PND in previous literature (Monk et al., 2008; Wu et al., 2016). With the result, we built a prediction model for PND, and its AUC of the ROC curve was 0.707 in short-term follow-up and 0.808 in a longer time. As a result, this model is meaningful to predict PND after major orthopedic surgery at the early stage, guiding proper care for the patients at high-risk of PND much earlier. Though there is a prediction model of postoperative cognitive outcomes for elderly orthopedic patients, this model was based on postoperative delirium only at 1 day after surgery (Wang et al., 2021). Therefore, our research is novel and profound with the consideration of long-term cognitive changes after surgery, while a larger scale of multicenter clinical researches need to be conducted in the future.
For the first time to explore the influence of hnRNPA2/B1 on the occurrence of PND after spinal surgery, our finding could give clinicians assistance to monitor and intervene the cognitive function in the elderly patients at the early stage. Sleep, pain, and cognition are three key intervenable targets in any multicomponent intervention designed to optimize perioperative brain (O’Gara et al., 2021). In consequence, we could reduce the occurrence and severity of PND by week-long cognitive training before surgery, relieving preoperative pain and tension, improving the sleeping conditions and so on (Ball et al., 2002; Sieber et al., 2011; Kamdar et al., 2013).
There are several limitations in our present study. Firstly, patients are usually discharged from hospital within 5 days, making it hard to collect blood samples in a longer follow-up period and investigate the dynamic changes of hnRNPA2/B1 in the development of disease as well as the severity of cognitive decline. Moreover, we only observed cognitive outcomes at 7 days and 3 months after surgery and did not perform longer follow-up. Therefore, longer-term impact of preoperative hnRNPA2/B1 level to AD conversion remains unknown. In the future, we will continue to follow up this population to clarify the relationship between hnRNPA2B1 and the outcome of PND and establish a complete perioperative warning system of MCI-PND-AD. A large-scale multicenter study will be carried out to further validate our findings as well. However, it should be noted that, we have indeed found some patients present a tendency to progress to dementia at the 3-month follow-up after surgery, whose neuropsychological performance is poorer than before and meet the criteria of dementia.
Briefly, our data expand the clinical value of hnRNPA2/B1 and suggest that patients occurring PND after lumbar decompression and fusion have higher hnRNPA2/B1 level as compared to controls. When taking baseline characteristics and biomarker signature of patients into account, we believe that increasing number of individuals at high risk of PND will be identified with high accuracy for they may benefit the most from timely interventions aimed at preventing pathological cognitive decline.
Data availability statement
The datasets presented in this study can be found in online repositories. The names of the repository/repositories and accession number(s) can be found in the article/Supplementary material.
Ethics statement
The studies involving human participants were reviewed and approved by the Medical Ethics Committee of Tianjin Third Central Hospital Nankai University Affinity the Third Central Hospital. The patients/participants provided their written informed consent to participate in this study.
Author contributions
HW: study design and manuscript revision. TX: data analysis and manuscript writing. CY: FCM. XW: follow-up of patients and post-operative data acquisition. LB: subjects’ recruitment. JM: qRT-PCR. MZ: ELISA. WH: peri- and intra-operative data acquisition. All authors contributed to the article and approved the submitted version.
Funding
This work was supported by grants from the National Natural Science Foundation of China (82071220), the Natural Science Foundation of Tianjin (20JCYBJC01290), the Science and Technology Foundation of Tianjin Health Commission (MS20013), and the Tianjin key Medical Discipline (Specialty) Construction Project (TJYXZDXK-072C).
Conflict of interest
The authors declare that the research was conducted in the absence of any commercial or financial relationships that could be construed as a potential conflict of interest.
Publisher’s note
All claims expressed in this article are solely those of the authors and do not necessarily represent those of their affiliated organizations, or those of the publisher, the editors and the reviewers. Any product that may be evaluated in this article, or claim that may be made by its manufacturer, is not guaranteed or endorsed by the publisher.
Supplementary material
The Supplementary Material for this article can be found online at: https://www.frontiersin.org/articles/10.3389/fnagi.2022.1034041/full#supplementary-material
References
Arenaza-Urquijo, E. M., Landeau, B., La Joie, R., Mevel, K., Mezenge, F., Perrotin, A., et al. (2013). Relationships between years of education and gray matter volume, metabolism and functional connectivity in healthy elders. Neuroimage 83, 450–457. doi: 10.1016/j.neuroimage.2013.06.053
Ball, K., Berch, D. B., Helmers, K. F., Jobe, J. B., Leveck, M. D., Marsiske, M., et al. (2002). Effects of cognitive training interventions with older adults: A randomized controlled trial. JAMA 288, 2271–2281. doi: 10.1001/jama.288.18.2271
Ballweg, T., White, M., Parker, M., Casey, C., Bo, A., Farahbakhsh, Z., et al. (2021). Association between plasma tau and postoperative delirium incidence and severity: A prospective observational study. Br. J. Anaesth. 126, 458–466. doi: 10.1016/j.bja.2020.08.061
Carr, Z. J., Cios, T. J., Potter, K. F., and Swick, J. T. (2018). Does dexmedetomidine ameliorate postoperative cognitive dysfunction? A brief review of the recent literature. Curr. Neurol. Neurosci. Rep. 18:64. doi: 10.1007/s11910-018-0873-z
Cata, J. P., Wang, H., Gottumukkala, V., Reuben, J., and Sessler, D. I. (2013). Inflammatory response, immunosuppression, and cancer recurrence after perioperative blood transfusions. Br. J. Anaesth. 110, 690–701. doi: 10.1093/bja/aet068
Danielson, M., Reinsfelt, B., Westerlind, A., Zetterberg, H., Blennow, K., and Ricksten, S. E. (2018). Effects of methylprednisolone on blood-brain barrier and cerebral inflammation in cardiac surgery-a randomized trial. J. Neuroinflammation 15:283. doi: 10.1186/s12974-018-1318-y
de Menezes, S. T., Giatti, L., Brant, L. C. C., Griep, R. H., Schmidt, M. I., Duncan, B. B., et al. (2021). Hypertension, prehypertension, and hypertension control: Association with decline in cognitive performance in the ELSA-Brasil cohort. Hypertension 77, 672–681. doi: 10.1161/HYPERTENSIONAHA.120.16080
Dijkstra, J. B., Houx, P. J., and Jolles, J. (1999). Cognition after major surgery in the elderly: Test performance and complaints. Br. J. Anaesth. 82, 867–874. doi: 10.1093/bja/82.6.867
Evered, L., Silbert, B., Scott, D. A., Ames, D., Maruff, P., and Blennow, K. (2016). Cerebrospinal fluid biomarker for Alzheimer disease predicts postoperative cognitive dysfunction. Anesthesiology 124, 353–361. doi: 10.1097/ALN.0000000000000953
Evered, L. A., and Silbert, B. S. (2018). Postoperative cognitive dysfunction and noncardiac surgery. Anesth. Analg. 127, 496–505. doi: 10.1213/ANE.0000000000003514
Feinkohl, I., Winterer, G., and Pischon, T. (2017a). Diabetes is associated with risk of postoperative cognitive dysfunction: A meta-analysis. Diabetes Metab. Res. Rev. 33:e2884. doi: 10.1002/dmrr.2884
Feinkohl, I., Winterer, G., Spies, C. D., and Pischon, T. (2017b). Cognitive reserve and the risk of postoperative cognitive dysfunction. Dtsch. Arztebl. Int. 114, 110–117. doi: 10.3238/arztebl.2017.0110
Feinkohl, I., Winterer, G., and Pischon, T. (2018). Associations of dyslipidaemia and lipid-lowering treatment with risk of postoperative cognitive dysfunction: A systematic review and meta-analysis. J. Epidemiol. Commun. Health 72, 499–506. doi: 10.1136/jech-2017-210338
Ferraris, V. A., Ballert, E. Q., and Mahan, A. (2013). The relationship between intraoperative blood transfusion and postoperative systemic inflammatory response syndrome. Am. J. Surg. 205, 457–465. doi: 10.1016/j.amjsurg.2012.07.042
Gao, S., Zhang, S., Zhou, H., Tao, X., Ni, Y., Pei, D., et al. (2021). Role of mTOR-regulated autophagy in synaptic plasticity related proteins downregulation and the reference memory deficits induced by anesthesia/surgery in aged mice. Front. Aging Neurosci. 13:628541. doi: 10.3389/fnagi.2021.628541
Heemskerk, J. L., Oluwadara Akinduro, O., Clifton, W., Quinones-Hinojosa, A., and Abode-Iyamah, K. O. (2021). Long-term clinical outcome of minimally invasive versus open single-level transforaminal lumbar interbody fusion for degenerative lumbar diseases: A meta-analysis. Spine J. 21, 2049–2065. doi: 10.1016/j.spinee.2021.07.006
Hughes, D., Judge, C., Murphy, R., Loughlin, E., Costello, M., Whiteley, W., et al. (2020). Association of blood pressure lowering with incident dementia or cognitive impairment: A systematic review and meta-analysis. JAMA 323, 1934–1944. doi: 10.1001/jama.2020.4249
Iadecola, C., and Gottesman, R. F. (2019). Neurovascular and cognitive dysfunction in hypertension. Circ. Res. 124, 1025–1044. doi: 10.1161/CIRCRESAHA.118.313260
Jiang, X. L., Gu, X. Y., Zhou, X. X., Chen, X. M., Zhang, X., Yang, Y. T., et al. (2019). Intestinal dysbacteriosis mediates the reference memory deficit induced by anaesthesia/surgery in aged mice. Brain Behav. Immun. 80, 605–615. doi: 10.1016/j.bbi.2019.05.006
Kamdar, B. B., King, L. M., Collop, N. A., Sakamuri, S., Colantuoni, E., Neufeld, K. J., et al. (2013). The effect of a quality improvement intervention on perceived sleep quality and cognition in a medical ICU. Crit. Care Med. 41, 800–809. doi: 10.1097/CCM.0b013e3182746442
Kolisnyk, B., Al-Onaizi, M., Soreq, L., Barbash, S., Bekenstein, U., Haberman, N., et al. (2017). Cholinergic surveillance over hippocampal RNA metabolism and Alzheimer’s-like pathology. Cereb. Cortex 27, 3553–3567. doi: 10.1093/cercor/bhw177
Krell-Roesch, J., Syrjanen, J. A., Vassilaki, M., Lowe, V. J., Vemuri, P., Mielke, M. M., et al. (2021). Brain regional glucose metabolism, neuropsychiatric symptoms, and the risk of incident mild cognitive impairment: The mayo clinic study of aging. Am. J. Geriatr. Psychiatry 29, 179–191. doi: 10.1016/j.jagp.2020.06.006
Lachmann, G., Feinkohl, I., Borchers, F., Ottens, T. H., Nathoe, H. M., Sauer, A. M., et al. (2018). Diabetes, but not hypertension and obesity, is associated with postoperative cognitive dysfunction. Dement. Geriatr. Cogn. Disord. 46, 193–206. doi: 10.1159/000492962
Le, Y., Liu, S., Peng, M., Tan, C., Liao, Q., Duan, K., et al. (2014). Aging differentially affects the loss of neuronal dendritic spine, neuroinflammation and memory impairment at rats after surgery. PLoS One 9:e106837. doi: 10.1371/journal.pone.0106837
Lee, K. H., Zhang, P., Kim, H. J., Mitrea, D. M., Sarkar, M., Freibaum, B. D., et al. (2016). C9orf72 dipeptide repeats impair the assembly, dynamics, and function of membrane-less organelles. Cell 167, 774–788.e17. doi: 10.1016/j.cell.2016.10.002
Liu, Y., Dong, Y. H., Lyu, P. Y., Chen, W. H., and Li, R. (2018). Hypertension-induced cerebral small vessel disease leading to cognitive impairment. Chin. Med. J. 131, 615–619. doi: 10.4103/0366-6999.226069
Lorente Pons, A., Higginbottom, A., Cooper-Knock, J., Alrafiah, A., Alofi, E., Kirby, J., et al. (2020). Oligodendrocyte pathology exceeds axonal pathology in white matter in human amyotrophic lateral sclerosis. J. Pathol. 251, 262–271. doi: 10.1002/path.5455
Lu, J., Cao, Q., Hughes, M. P., Sawaya, M. R., Boyer, D. R., Cascio, D., et al. (2020). CryoEM structure of the low-complexity domain of hnRNPA2 and its conversion to pathogenic amyloid. Nat. Commun. 11:4090. doi: 10.1038/s41467-020-17905-y
Luo, A., Yan, J., Tang, X., Zhao, Y., Zhou, B., and Li, S. (2019). Postoperative cognitive dysfunction in the aged: The collision of neuroinflammaging with perioperative neuroinflammation. Inflammopharmacology 27, 27–37. doi: 10.1007/s10787-018-00559-0
Memoria, C. M., Yassuda, M. S., Nakano, E. Y., and Forlenza, O. V. (2013). Brief screening for mild cognitive impairment: Validation of the Brazilian version of the Montreal cognitive assessment. Int. J. Geriatr. Psychiatry 28, 34–40. doi: 10.1002/gps.3787
Mitchell, A. J. (2009). A meta-analysis of the accuracy of the mini-mental state examination in the detection of dementia and mild cognitive impairment. J. Psychiatr. Res. 43, 411–431. doi: 10.1016/j.jpsychires.2008.04.014
Mizukami, K., Ishikawa, M., Iwakiri, M., Ikonomovic, M. D., Dekosky, S. T., Kamma, H., et al. (2005). Immunohistochemical study of the hnRNP A2 and B1 in the hippocampal formations of brains with Alzheimer’s disease. Neurosci. Lett. 386, 111–115. doi: 10.1016/j.neulet.2005.05.070
Monk, T. G., Weldon, B. C., Garvan, C. W., Dede, D. E., Van Der Aa, M. T., Heilman, K. M., et al. (2008). Predictors of cognitive dysfunction after major noncardiac surgery. Anesthesiology 108, 18–30. doi: 10.1097/01.anes.0000296071.19434.1e
Moran, C., Beare, R., Wang, W., Callisaya, M., Srikanth, V., and Alzheimer’s Disease Neuroimaging Initiative [ADNI]. (2019). Type 2 diabetes mellitus, brain atrophy, and cognitive decline. Neurology 92, e823–e830. doi: 10.1212/WNL.0000000000006955
Needham, M. J., Webb, C. E., and Bryden, D. C. (2017). Postoperative cognitive dysfunction and dementia: What we need to know and do. Br. J. Anaesth. 119, i115–i125. doi: 10.1093/bja/aex354
Netto, M. B., De Oliveira Junior, A. N., Goldim, M., Mathias, K., Fileti, M. E., Da Rosa, N., et al. (2018). Oxidative stress and mitochondrial dysfunction contributes to postoperative cognitive dysfunction in elderly rats. Brain Behav. Immun. 73, 661–669. doi: 10.1016/j.bbi.2018.07.016
Newman, M. F., Kirchner, J. L., Phillips-Bute, B., Gaver, V., Grocott, H., Jones, R. H., et al. (2001). Longitudinal assessment of neurocognitive function after coronary-artery bypass surgery. N. Engl. J. Med. 344, 395–402. doi: 10.1056/NEJM200102083440601
O’Gara, B. P., Gao, L., Marcantonio, E. R., and Subramaniam, B. (2021). Sleep, pain, and cognition: Modifiable targets for optimal perioperative brain health. Anesthesiology 135, 1132–1152. doi: 10.1097/ALN.0000000000004046
O’Gara, B. P., Mueller, A., Gasangwa, D. V. I., Patxot, M., Shaefi, S., Khabbaz, K., et al. (2020). Prevention of early postoperative decline: A randomized, controlled feasibility trial of perioperative cognitive training. Anesth. Analg. 130, 586–595. doi: 10.1213/ANE.0000000000004469
Park, D. C., and Reuter-Lorenz, P. (2009). The adaptive brain: Aging and neurocognitive scaffolding. Annu. Rev. Psychol. 60, 173–196. doi: 10.1146/annurev.psych.59.103006.093656
Perry, A., Wen, W., Kochan, N. A., Thalamuthu, A., Sachdev, P. S., and Breakspear, M. (2017). The independent influences of age and education on functional brain networks and cognition in healthy older adults. Hum. Brain Mapp. 38, 5094–5114. doi: 10.1002/hbm.23717
Ramlawi, B., Rudolph, J. L., Mieno, S., Khabbaz, K., Sodha, N. R., Boodhwani, M., et al. (2006). Serologic markers of brain injury and cognitive function after cardiopulmonary bypass. Ann. Surg. 244, 593–601. doi: 10.1097/01.sla.0000239087.00826.b4
Rappold, T., Laflam, A., Hori, D., Brown, C., Brandt, J., Mintz, C. D., et al. (2016). Evidence of an association between brain cellular injury and cognitive decline after non-cardiac surgery. Br. J. Anaesth. 116, 83–89. doi: 10.1093/bja/aev415
Rossi, S., Rompietti, V., Antonucci, Y., Giovannini, D., Scopa, C., Scaricamazza, S., et al. (2020). UsnRNP trafficking is regulated by stress granules and compromised by mutant ALS proteins. Neurobiol. Dis. 138:104792. doi: 10.1016/j.nbd.2020.104792
Shorter, J., and Taylor, J. P. (2013). Disease mutations in the prion-like domains of hnRNPA1 and hnRNPA2/B1 introduce potent steric zippers that drive excess RNP granule assembly. Rare Dis. 1:e25200. doi: 10.4161/rdis.25200
Sieber, F. E., Mears, S., Lee, H., and Gottschalk, A. (2011). Postoperative opioid consumption and its relationship to cognitive function in older adults with hip fracture. J. Am. Geriatr. Soc. 59, 2256–2262. doi: 10.1111/j.1532-5415.2011.03729.x
Steinmetz, J., Christensen, K. B., Lund, T., Lohse, N., Rasmussen, L. S., and Group, I. (2009). Long-term consequences of postoperative cognitive dysfunction. Anesthesiology 110, 548–555. doi: 10.1097/ALN.0b013e318195b569
Subramaniyan, S., and Terrando, N. (2019). Neuroinflammation and perioperative neurocognitive disorders. Anesth. Analg. 128, 781–788. doi: 10.1213/ANE.0000000000004053
Tan, H., Bi, J., Wang, Y., Zhang, J., and Zuo, Z. (2015). Transfusion of old RBCs induces neuroinflammation and cognitive impairment. Crit. Care Med. 43, e276–e286. doi: 10.1097/CCM.0000000000001023
Terrando, N., Eriksson, L. I., Ryu, J. K., Yang, T., Monaco, C., Feldmann, M., et al. (2011). Resolving postoperative neuroinflammation and cognitive decline. Ann. Neurol. 70, 986–995. doi: 10.1002/ana.22664
Urner, M., Herrmann, I. K., Buddeberg, F., Schuppli, C., Roth Z’graggen, B., Hasler, M., et al. (2012). Effects of blood products on inflammatory response in endothelial cells in vitro. PLoS One 7:e33403. doi: 10.1371/journal.pone.0033403
van Sloten, T. T., Sedaghat, S., Carnethon, M. R., Launer, L. J., and Stehouwer, C. D. A. (2020). Cerebral microvascular complications of type 2 diabetes: Stroke, cognitive dysfunction, and depression. Lancet Diabetes Endocrinol. 8, 325–336. doi: 10.1016/S2213-8587(19)30405-X
Wang, G., Zhang, L., Qi, Y., Chen, G., Zhou, J., Zhu, H., et al. (2021). Development and validation of a postoperative delirium prediction model for elderly orthopedic patients in the intensive care unit. J. Healthc. Eng. 2021:9959077. doi: 10.1155/2021/9959077
Wang, J., Yang, C., Wang, H., Li, D., Li, T., Sun, Y., et al. (2020). A new rat model of chronic cerebral hypoperfusion resulting in early-stage vascular cognitive impairment. Front. Aging Neurosci. 12:86. doi: 10.3389/fnagi.2020.00086
Wu, C., Wang, R., Li, X., and Chen, J. (2016). Preoperative serum MicroRNA-155 expression independently predicts postoperative cognitive dysfunction after laparoscopic surgery for colon cancer. Med. Sci. Monit. 22, 4503–4508. doi: 10.12659/MSM.898397
Xiao, J. Y., Xiong, B. R., Zhang, W., Zhou, W. C., Yang, H., Gao, F., et al. (2018). PGE2-EP3 signaling exacerbates hippocampus-dependent cognitive impairment after laparotomy by reducing expression levels of hippocampal synaptic plasticity-related proteins in aged mice. CNS Neurosci. Ther. 24, 917–929. doi: 10.1111/cns.12832
Xiao, Q. X., Liu, Q., Deng, R., Gao, Z. W., and Zhang, Y. (2020). Postoperative cognitive dysfunction in elderly patients undergoing hip arthroplasty. Psychogeriatrics 20, 501–509. doi: 10.1111/psyg.12516
Yang, X. D., Wang, L. K., Wu, H. Y., and Jiao, L. (2018). Effects of prebiotic galacto-oligosaccharide on postoperative cognitive dysfunction and neuroinflammation through targeting of the gut-brain axis. BMC Anesthesiol. 18:177. doi: 10.1186/s12871-018-0642-1
Zhang, Q., Zhang, J., Ye, J., Li, X., Liu, H., Ma, X., et al. (2021). Nuclear speckle specific hnRNP D-like prevents age- and AD-related cognitive decline by modulating RNA splicing. Mol. Neurodegener. 16:66. doi: 10.1186/s13024-021-00485-w
Keywords: postoperative neurocognitive dysfunction, biomarker, lumbar decompression and fusion, HnRNPA2/B1, Aβ42
Citation: Xia T, Yang C, Wang X, Bai L, Ma J, Zhao M, Hua W and Wang H (2022) Heterogeneous nuclear ribonucleoprotein A2/B1 as a novel biomarker in elderly patients for the prediction of postoperative neurocognitive dysfunction: A prospective nested case-control study. Front. Aging Neurosci. 14:1034041. doi: 10.3389/fnagi.2022.1034041
Received: 01 September 2022; Accepted: 03 October 2022;
Published: 21 October 2022.
Edited by:
Jiaqiang Zhang, Zhengzhou University, ChinaReviewed by:
Hao Wang, Shanghai Jiao Tong University, ChinaHeather M. Wilkins, University of Kansas Medical Center Research Institute, United States
Copyright © 2022 Xia, Yang, Wang, Bai, Ma, Zhao, Hua and Wang. This is an open-access article distributed under the terms of the Creative Commons Attribution License (CC BY). The use, distribution or reproduction in other forums is permitted, provided the original author(s) and the copyright owner(s) are credited and that the original publication in this journal is cited, in accordance with accepted academic practice. No use, distribution or reproduction is permitted which does not comply with these terms.
*Correspondence: Haiyun Wang, why819@126.com