- 1Department of Rehabilitation Medicine, The First Affiliated Hospital of Nanjing Medical University, Nanjing, China
- 2Division of Brain Rehabilitation, Department of Neurology, The First Affiliated Hospital of Nanjing Medical University, Nanjing, China
- 3Department of Neurology, The First Affiliated Hospital of Nanjing Medical University, Nanjing, China
- 4Intensive Care Unit, Wuxi No.2 People’s Hospital, Wuxi, China
- 5Department of Neurology, Xuzhou First People’s Hospital, Xuzhou, China
- 6Department of Neurology, The Affiliated Hospital of China University of Mining and Technology, Xuzhou, China
Background: Cognitive decline is the most dominant and patient-oriented symptom during the development of Alzheimer’s disease (AD) and mild cognitive impairment (MCI). This study was designed to test the feasibility of hybrid convolutional neural networks and long-short-term memory (CNN-LSTM) modeling driven early decision-tailoring with the predicted long-term cognitive conversion in AD and MCI.
Methods: Characteristics of patients with AD or MCI covering demographic features, clinical features, and time-dependent neuropsychological-related features were fused into the hybrid CNN-LSTM modeling to predict cognitive conversion based on a 4-point change in the AD assessment scale-cognition score. Treatment reassignment rates were estimated based on the actual and predicted cognitive conversion at 3 and 6 months according to the prespecified principle; that is if the ADAS-cog score of the patient declines less than 4 points or increases at either follow-up time point, the medical treatment recommended upon their diagnosis would be considered insufficient. Therefore, it is recommended to upgrade the medical treatment upon diagnosis. Actual and predicted treatment reassignment rates were compared in the general population and subpopulations categorized by age, gender, symptom severity, and the intervention subtypes.
Results: A total of 224 patients were included in the analysis. The hybrid CNN-LSTM model achieved the mean AUC of 0.735 (95% CI: 0.701–0.769) at 3 months and 0.853 (95% CI: 0.814–0.892) at 6 months in predicting cognitive conversion status. The AUC at 6 months was significantly impacted when data collected at 3 months were withdrawn. The predicted cognitive conversion suggested a revision of medical treatment in 46.43% (104/224) of patients at 3 months and 54.02% (121/224) at 6 months as compared with 62.05% (139/224) at 3 months (p = 0.001) and 62.50% (140/224) at 6 months (p = 0.069) according to their actual cognitive conversion. No significant differences were detected between treatment reassignment rates estimated based on actual and predicted cognitive conversion in all directions at 6 months.
Conclusion: Using the synergistic advances of deep learning modeling and featured longitudinal information, our hypothesis was preliminarily verified with the comparable predictive performance in cognitive conversion. Results provided the possibility of reassigned recommended treatment for those who may suffer from cognitive decline in the future. Considering the limited diversity of treatment strategies applied in this study, the real-world medical situation should be further simulated.
Background
According to the Alzheimer’s Disease International, the estimated prevalence of dementia is about 50 million people worldwide in 2018 and will be projected to triple in 2050 (Koszewicz et al., 2021). Alzheimer’s disease (AD) and mild cognitive impairment (MCI) are two major types of dementia, which have launched significant economic load and medical burden for the families and healthcare systems (Hill et al., 2017).
With the increasing efforts in predicting the occurrence and development of AD and MCI, age and gender have often been identified as the most important risk factors. Previous studies have shown that for elders aged 65 years or more, there were significantly more women who have developed AD compared with a high proportion of men who have developed MCI with an OR of 1.54 (95%CI: 1.2–2.0) (Petersen et al., 2010; Wu et al., 2017). Moreover, studies on biomarkers have formed an important basis for the early diagnosis of dementia (Hort et al., 2010). The β amyloid deposition, pathologic tau, and neurodegeneration (A-T-N) framework proposed by Jack et al. (2018) is intended to define the diagnosis of AD by the presence of amyloid-β and phosphorylated tau that measured either in plasma, cerebrospinal fluid (CSF), or neuroimaging. While in the search for prognostic biomarkers of dementia, the field still focused heavily on neuroimaging and CSF markers. For instance, several studies have reported that the progression of AD or MCI could be predicted by advanced neuroimaging techniques, including MRI findings, FDG-PET, or CSF examination (Jack et al., 1999; Killiany et al., 2000; Shaw et al., 2009; Zhang et al., 2012). In fact, professionals have advocated that at least one neuroimaging examination and several CSF/plasma examinations are needed for monitoring the progression of dementia (Langa and Levine, 2014). However, the major barrier leading to this destination is the poor compliance to these examinations and the difficulties in the collection of the corresponding information in real clinical practice. In addition, even these difficulties can be conquered, their specificity and accuracy in the prediction of prognosis of dementia have not been guaranteed in the current context (Carrillo et al., 2013; Hepp et al., 2016).
For the majority of patients diagnosed with AD and MCI, cognitive impairment is recognized as the most dominant and patient-oriented symptom. Progression of cognitive impairment is normally monitored through longitudinal neuropsychological assessments using cognitive scales such as the Alzheimer’s Disease Assessment Scale-Cognition (ADAS-Cog) or the Mini-Mental State Examination (MMSE). This longitudinal information can be scheduled and collected sequentially through face-to-face interviews in the hospital, community, or at home. According to Xue et al. (2018) neuropsychological assessments were proved to be more practical compared with neuroimaging and CSF/plasma examinations and more accurate in the reflection of cognitive function than baseline information alone. This inspired us to ask whether sequential neuropsychological-related information along with other clinical data can be used to predict cognitive trajectory. Upon this thought, the long-term cognitive decline can be foreseen with which medical treatment for AD or MCI could be redirected at the early stage. Considering the high heterogeneity in the individual progression of dementia and huge difficulties in the prediction of its trajectory, traditional statistical models may not be powerful enough to provide accurate predictive outcomes. In particular, the cognitive trajectory that was impacted by the sequential time features would probably not be captured by traditional regression algorithms (Verbeke et al., 2014). Upon this data signature, we were, therefore, promoted to construct a novel deep learning model comprised of convolutional neural networks (CNN) and long-short-term memory (LSTM) networks. This hybrid CNN-LSTM network is capable to handle longitudinal data with varying lengths of follow-ups, help capture temporal dynamics, and, therefore, make accurate predictions based on the sequential data (Hochreiter and Schmidhuber, 1997; Cho et al., 2014; Chung et al., 2014; Ioannou et al., 2020).
In this study, we used the subset data from three ongoing, longitudinal, cohort studies conducted by our research team. The aim of this study was (1) to explore the predictive potential of longitudinal neuropsychological information on cognitive conversion reflected by ADAS-Cog upon the diagnosis of AD and MCI and (2) to compare the reassignment rates estimated by the deep learning modeling according to the actual status. If the long-term cognitive status can be successfully predicted with our hybrid CNN-LSTM modeling, then recommendations on treatment redirection can be provided at the early stage of AD or MCI.
Materials and Methods
Patient Data
This study utilized three cohorts including 364 patients from the First Affiliated Hospital of Nanjing Medical University (ClinicalTrials.gov ID: NCT03090516 and Chinese Clinical Trial Registry ID: ChiCTR-INR-15007420) and 111 patients from communities in Nanjing, China. Patients from the above three cohorts were treated with either observation, exercise, monotherapy (donepezil or ginkgo biloba extract [GBE]), or donepezil and GBE combination. All of these patients met the following inclusion criteria: (1) diagnosed with AD or MCI according to the NINCDS/ADRDA guidelines; (2) MMSE score of 27 or less; (3) able to follow medical instruction or assessment requirement; (4) complete available baseline and longitudinal data, such as age, course of the disease, comorbidities, blood biochemical examination, clinical scales assessed during three visits (e.g., baseline, 3 months, and 6 months); and (5) signed informed consent (Cummings, 1993; Dubois et al., 2007). The flowchart of this study is shown in Figure 1.
Predictive Variables and Target Outcomes
As data from these cohorts were presented with a varying treatment plan, disease duration, visiting frequency, and inclusion criteria, all patients have no data beyond 6 months at this moment. We chose a 3-month spacing between time points based on the visit frequency of follow-up patients to ensure most patients had efficient data for model building (baseline, 3 months, and 6 months).
Data in the merged database were stored in EXCEL format. The predictors used in our deep learning model of cognitive conversion prediction that were originated from tables in the database but not limited to demographics, medical history, intervention subtype, neuropsychological outcomes, and laboratory and neuroelectrophysiological results are listed in Supplementary Table 1. Time-dependent variables such as ADAS-cog (Rosen et al., 1984), MMSE (Folstein et al., 1975), the instrumental activity of daily living (IADL) (Thomas et al., 1998), geriatric depression scale (GDS) (Defrancesco et al., 2018), quality of life-Alzheimer’s disease (QOL-AD) (Lawton, 1997), and neuropsychiatric inventory (NPI) (Cummings et al., 1994) for the evaluation of neurobehavioral status were recorded at 3 month intervals (Cummings, 1993; Logsdon et al., 2002; LaPlante, 2010; Benedetti et al., 2018; Jiang et al., 2020; Vik-Mo et al., 2020).
According to the guidelines published by the American College of Physicians and the American Academy of Family Physicians, a decline of 4 points or more in the ADAS-cog score is used to define a clinically important improvement (Qaseem et al., 2008). Based on this, we used ADAS-cog as the target outcome and adopted 4 points as the threshold so that patients with different cognitive conversion during follow-ups were further classified (Qaseem et al., 2008; Raina et al., 2008). Specifically, patients whose ADAS-cog score had a decline of 4 points or more were grouped as cognition improved (CI), and patients whose ADAS-cog score remained stable or increased were grouped as cognition not improved (CNI) (Sabbagh et al., 2020).
The Hybrid Convolutional Neural Networks and Long-Short-Term Memory Modeling
Data Preprocessing
We first excluded patients with missing feature variables covering more than 50% of the whole record number, as higher proportions of missing data limit the prediction ability of the proposed model. We then imputed missing data of remaining features with the mean or the mode of existing data in the same feature for continuous or categorical variables, respectively (Kang, 2013). Normalization was subsequently applied; therefore, all data are normalized to have zero mean and unit variance.
Model Development and Training Details
A hybrid model comprised of cascaded classical CNN and state-of-art LSTM was constructed to forecast 3-month interval cognitive conversion status (CI vs. CNI at 3 months and 6 months) in individual patients (Figure 2). Briefly, CNN with stacked multiple full connection layers was used to aggregate and extract features of all non-sequential state information. We then applied the sigmoid activation function to generate the score of the non-sequential state (time-independent data) information. The detailed architecture of CNN was explained in Supplementary Figure 1. Afterward, the generated score was combined with all other time-dependent data, the corresponding time-sequential information (baseline or 3-month) as well as the cognitive conversion status (improved or not) to fuse into the LSTM network. This specialized LSTM model was trained to use the encoded latent representation from the previous time point as well as the multivariate relations of the current time point (Gers and Schmidhuber, 2001). Using this process, the underlying temporal characteristics in the given time-dependent ADAS-cog score were captured. Notably, the attention mechanism was applied to perform feature-weighted fusion across time steps to improve the prediction accuracy (Vaswani et al., 2017). This procedure was made to empower the model to find significant useful information related to the current output in the input data and eventually improve the quality of the output.
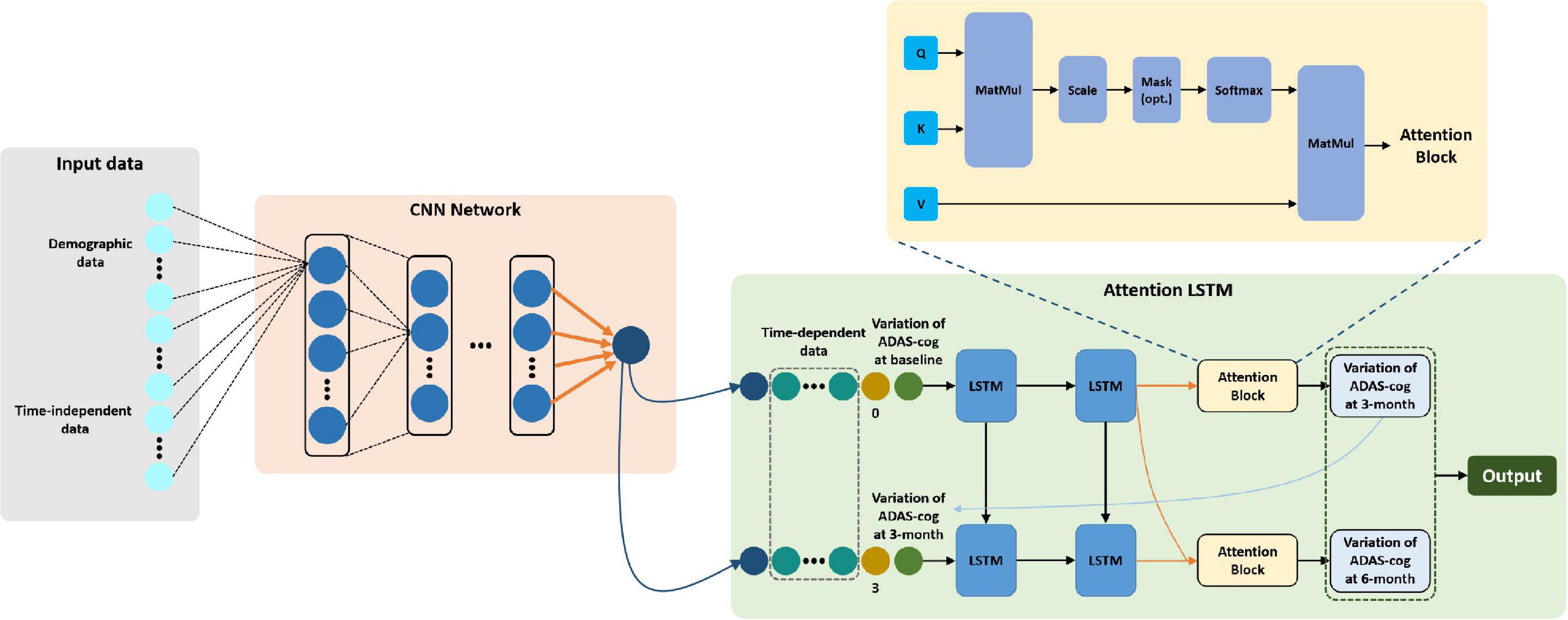
Figure 2. Workflow of CNN-LSTM modeling and architecture of proposed network composition. *The architecture of the hybrid CNN-LSTM model combines two modules. The CNN module (light pink) is composed of five fully connected layers and a rectified linear unit (ReLU) activation unit and then followed by a sigmoid activation function to generate a score representative of non-time dependent features. Next, the LSTM module (light green) was trained to extract both non- and time-dependent features to generate outputs for estimating cognitive conversion status in multiple time points. The attention block (light yellow) denoted an attention mechanism proposed by Vaswani et al. (2017) which was used to perform feature-weighted fusion across time steps to make an accurate prediction. *The yellow block used here refers to “scaled dot-product attention,” explicitly explained by Vaswani et al. (2017) Given a task-related query vector Q, it can calculate the attention value by calculating the attention distribution associated with the key (K) and assigning it to the value (V). This attention block is applied to find the “resonance” between hidden vectors from each time step, namely, find the most relevant embedding features for high-level representations recognition.
We implemented the above model building on the publicly available library sklearn and open framework PyTorch on Python 3.6 using a computer with one NVidia GTX 1080Ti GPU. The batch size was set to 3,600 when training the CNN-LSTM model, with the optimizer used as the Adam algorithm (Kingma and Ba, 2014). The learning rate was reduced from 0.01, and the number of iterations of training is 1,000 epochs. The output model was selected based on the epoch, resulting in the highest AUC in the validation cohorts. For training the specialized LSTM model, the teacher forcing strategy is used to improve the learning efficiency of the model.
Model Withdrawal Procedure
To explore the impact of the cognitive conversion status at each time step during the evaluation of the patient, we used the trained hybrid model to test and evaluate the robustness of a single time point on the ADAS-cog score; that is, by changing the model input through withdrawing the mid-point information (simulating situations of follow-up absence), the degree of impact of ADAS-cog at 3 months can be evaluated.
Model Performance Measurements and Statistical Analysis
Descriptive statistics (mean, standard deviation counts, and proportions) were provided to demonstrate the sample characteristics with respect to statistical quantitative and qualitative features.
For hybrid CNN-LSTM model evaluation, five-fold cross-validation was applied, and assembled ROC curves with AUCs were used to assess model performance at 3 and 6 months. Performance measurements including AUC, sensitivity, and specificity with 95% confidence interval (95% CI) were assessed using the optimal cut-off value (Youden index = sensitivity + specificity − 1).
We expected that the implementation of deep learning modeling could potentially guide the recommended treatment plan, especially on patients who may suffer from continuous cognitive decline during follow-ups. According to recommendations by multiple guidelines, it is assumed that if patient’s ADAS-cog score declines less than 4 points or increases at either follow-up time point (every 3 months), the medical treatment recommended upon their diagnosis would be considered insufficient for avoiding the cognitive decline in the future (Farlow and Cummings, 2007; Cummings et al., 2015; Grossberg et al., 2019). Therefore, it is recommended to redirect or upgrade the medical treatment. Upon the above rationales, it was hypothesized that if our deep learning modeling could successfully predict the long-term cognitive conversion, it could be used to guide the early decision-making redirection. Therefore, we first estimated the treatment reassignment rates based on the actual and predicted cognitive conversion (namely, the target outcome) at 3 and 6 months according to the above principle. The actual and predicted treatment reassignment rates were then compared with chi-square testing (adjusted if necessary) in the general population and according to age, gender, and symptom severity as well as intervention subtypes within all directions. STATA 16.0 (StataCorp LLC, TX, United States) was utilized to perform the above analysis, and the statistical significance was determined with p < 0.05.
Results
Demographics and Cognitive Conversion Distribution
Patient demographics and distribution according to cognitive conversion (e.g., improved or not improved) at 3 and 6 months are demonstrated in Table 1. A number of 224 patients were included, of whom 85 at 3 months and 84 at 6 months were identified as CI population, while 139 at 3 months and 140 at 6 months were identified as CNI. There were more men (137 accounted for 61.16%) than women (87 accounted for 38.84%) enrolled with an average age of 69.75 ± 8.52 years. Hypertension was the predominant comorbidity in 44 (19.64%) patients. Patients were referred for different treatments mainly based on the severity of cognitive impairment and the interests of the original cohorts in which they were enrolled, including 62 (27.68%) for observation, 22 (9.82%) for exercise, 55 (24.55%) for donepezil monotherapy, 38 (16.96%) of GBE monotherapy, and 47 (20.98%) for GBE and donepezil combination.
Predictive Performance of Hybrid Convolutional Neural Networks and Long-Short-Term Memory Modeling
We performed five-fold cross-validation to test the model stability and to obtain the predicted outcomes of all patients. Our hybrid CNN-LSTM modeling showed a considerable good predictive capacity in identifying cognitive conversion status at sequential time points. As shown in Figure 3, the hybrid model achieved a mean AUC of 0.735 (95% CI: 0.701–0.769) at 3 months and 0.853 (95% CI: 0.814–0.892) at 6 months. The predictive performance achieved by the proposed model was considered good at 3 and 6 months, with the detailed information provided in Figure 4 and Table 2.
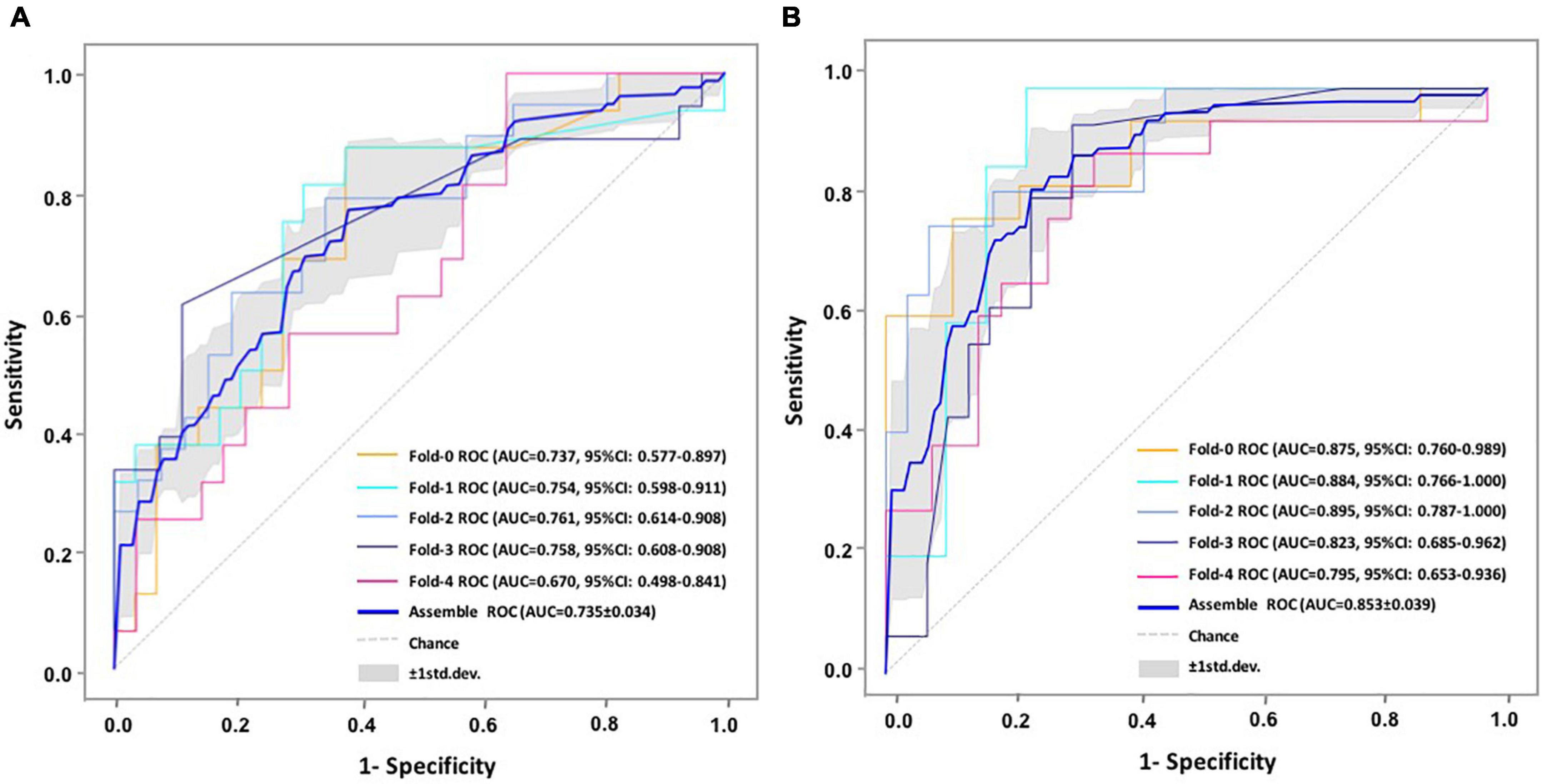
Figure 3. ROC comparisons of cognitive conversion at 3 and 6 months with CNN-LSTM modeling. (A) ROC comparisons of cognitive conversion at 3 months; (B) ROC comparisons of cognitive conversion at 6 months.
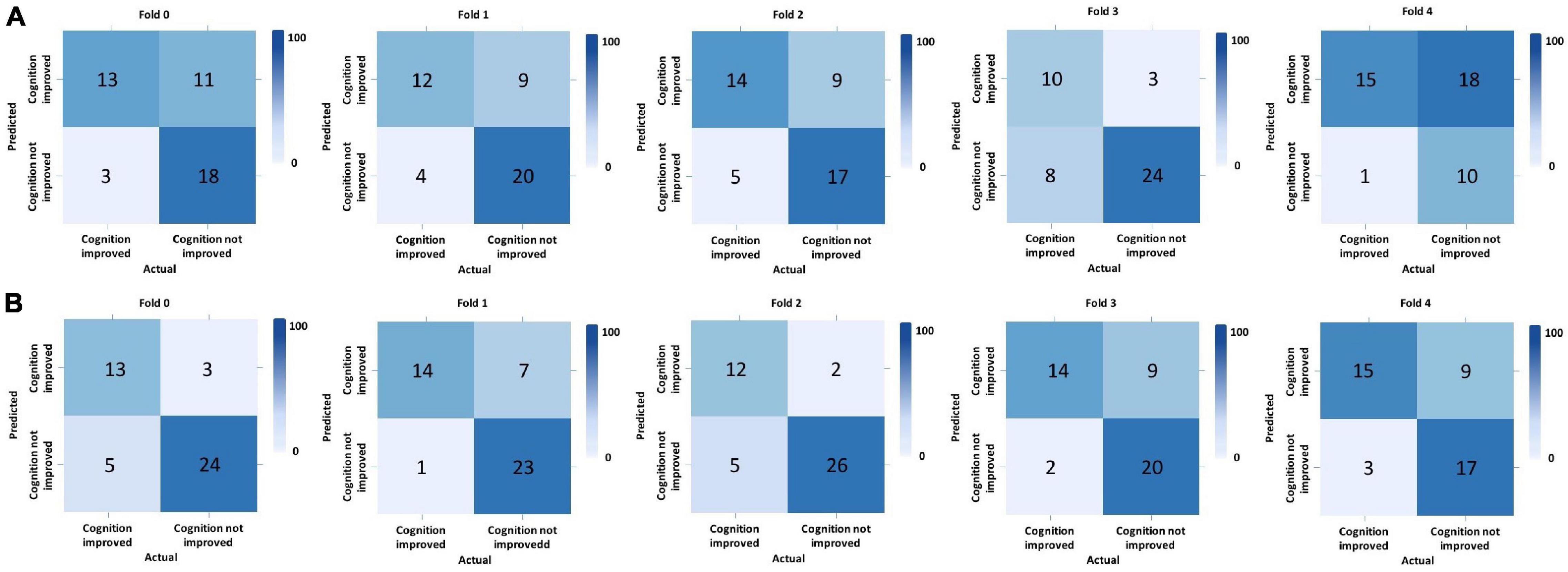
Figure 4. Predictive performance evaluation of CNN-LSTM modeling with confusion matrix at 3 and 6 months. *Computed classification confusion matrix using our hybrid CNN-LSTM modeling in five-fold cross-validation. (A) Confusion matrix at 3 months; (B) Confusion matrix at 6 months.
The proposed hybrid model generally provided accurately predicted outcomes in 70.09% of patients at 3 months and 81.70% at 6 months. To validate the generalizability of the proposed model, Figure 5 and Supplementary Table 2 provide the representative subgroup results according to age, gender, symptom severity, and intervention subtype between actual and predicted cognitive conversion at 3 and 6 months. Predictive performance was superior in male patients who were under observation at 3 months and exercise at 6 months.
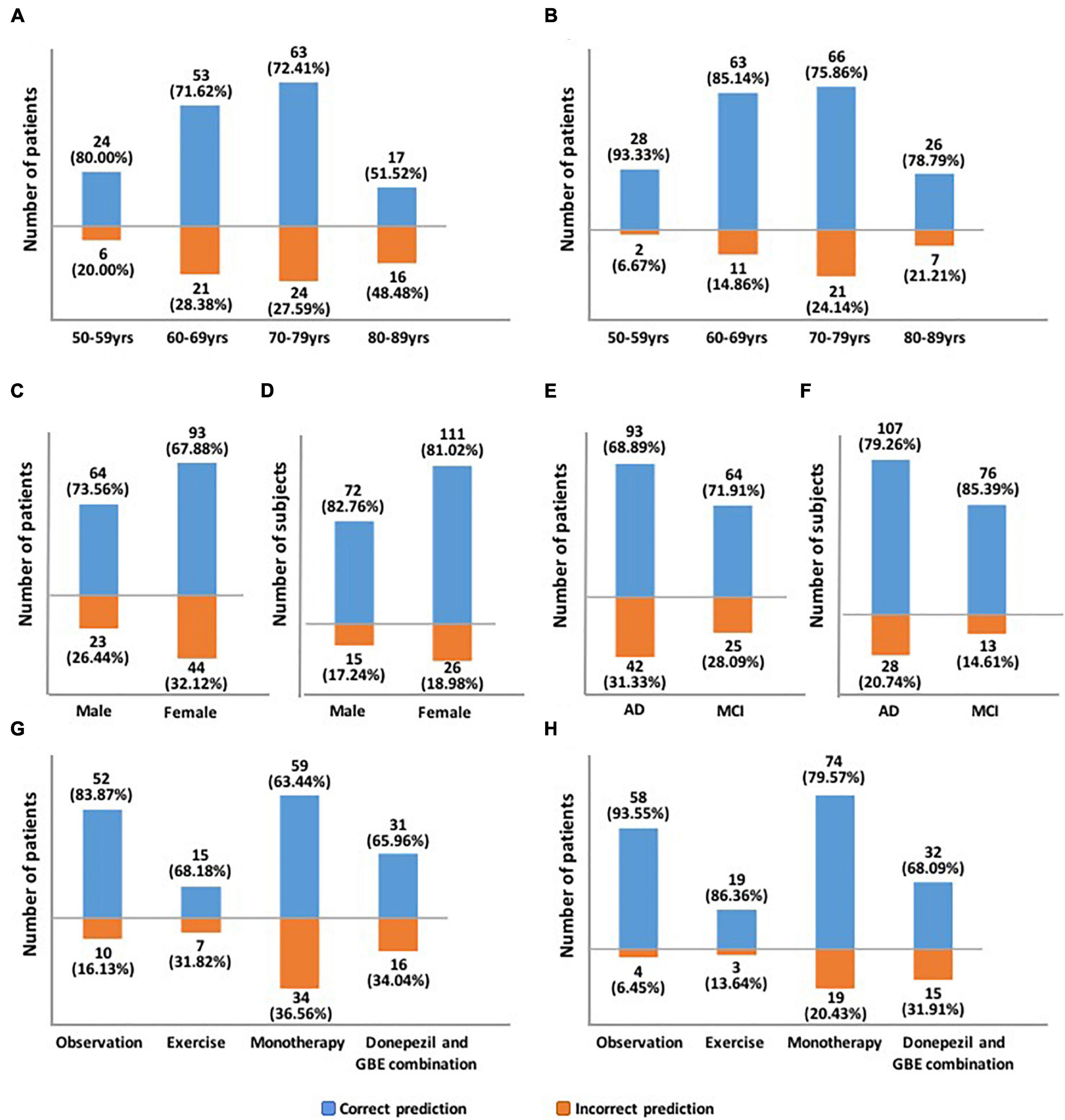
Figure 5. Demonstration of predictive accuracy stratified by age, gender, symptom severity, and intervention subtypes. (A) Predictive accuracy stratified by age at 3 months; (B) Predictive accuracy stratified by age at 6 months; (C) Predictive accuracy stratified by gender at 3 months; (D) Predictive accuracy stratified by gender at 6 months; (E) Predictive accuracy stratified by symptom severity at 3 months; (F) Predictive accuracy stratified by symptom severity at 6 months; (G) Predictive accuracy stratified by intervention subtypes at 3 months; (H) Predictive accuracy stratified by intervention subtypes at 6 months.
In addition, we constructed the withdrawal model to further explore the importance of time-sequential data on the stability of our previously built CNN-LSTM modeling. As shown in Figure 3 and Supplementary Figure 2, without the incorporation of data collected at 3 months, the AUC at 6 months decreased from 0.853 (95% CI: 0.814–0.892) to 0.734 (95% CI: 0.678–0.790), indicating that the AUC was moderately impacted by the withdrawal of data collected at 3 months. Another evaluation matrix of CNN-LSTM based withdrawal modeling at 6 months is demonstrated in Supplementary Figure 3 and Table 3.
Recommended Treatment Reassignment Following Actual and Predicted Cognitive Conversion at 3 and 6 Months
Based on the prespecified principle, over half of the patients need reclassified treatment with a fraction of 62.05% (139/224) at 3 months and 62.50% (140/224) at 6 months as determined by their actual cognitive conversion. According to our hybrid CNN-LSTM algorithms, the predicted conversion could potentially guide the treatment reassignment in 46.43% (104/224) of patients at 3 months and 54.02% (121/224) at 6 months (Figure 6). No significant difference (p = 0.069) was noted in actual and predicted treatment reassignment at 6 months. We also estimated the treatment reassignment rate in subgroups categorized by age, gender, symptom severity, and intervention subtypes within all directions. Generally, no significant differences in treatment reassignment rates were detected between actual and predicted cognitive conversion in all directions at 6 months. Detailed statistics regarding subgroup analysis of treatment reassignment are provided in Table 4.
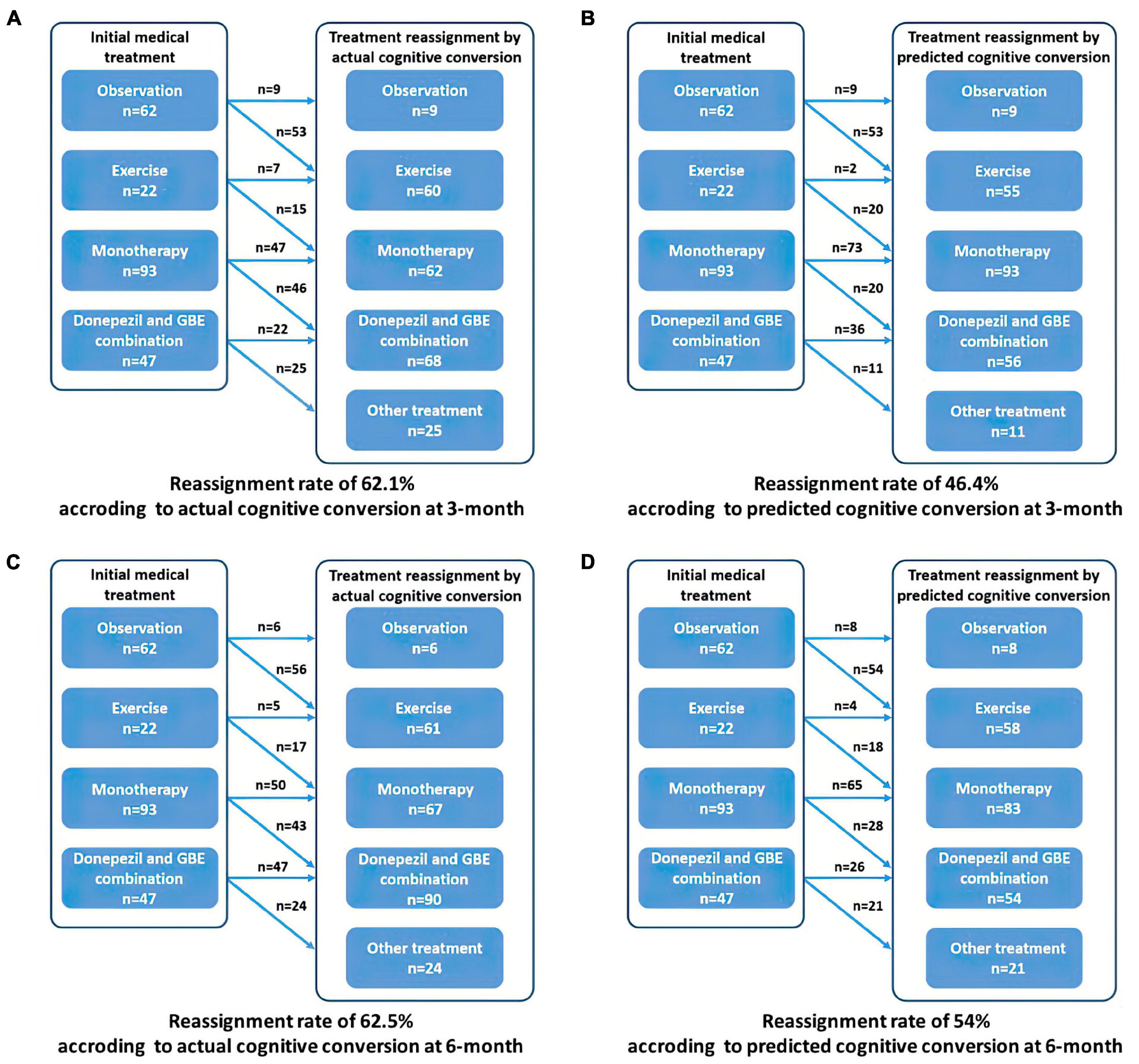
Figure 6. Recommended treatment reassignment following actual and predictive cognitive conversion at 3 and 6 months. (A) Treatment reassignment according to actual cognitive conversion at 3 months; (B) Treatment reassignment according to predicted cognitive conversion at 3 months; (C) Treatment reassignment according to actual cognitive conversion at 6 months; (D) Treatment reassignment according to predicted cognitive conversion at 6 months. *Another treatment indicates additional memantine, psychological interventions combined with pharmacological therapy or novel pharmacological approaches involving strategies to reduce amyloid and/or tau deposition.
Discussion
Progressive cognitive impairment is currently considered as the major symptom in patients with AD or MCI. Studies and reviews have suggested improved clinical outcomes (e.g., suspended or postponed cognitive decline) in patients who were managed upon early diagnosis (Livingston et al., 2017; Regan et al., 2017; Bachurin et al., 2018; Green et al., 2019; Mintun et al., 2021). Advanced diagnostic strategies (e.g., genetic or plasma screening) have been proposed, therefore early intervention could be delivered directly (Nakamura et al., 2018; Giau et al., 2019; Palmqvist et al., 2019; Karikari et al., 2020). However, there remain concerns regarding a fairly high proportion of the quick decline in cognitive function in a short period due to distinctions in terms of the progressive variation of dementia. In the view of medical professionals, this situation would be attributed to, to some extent, the lack of strategies available to guide treatment decision-making even the diagnosis of AD or MCI at the early stage.
The concept of artificial intelligence (AI) has recently permeated almost every sector of the healthcare system. Its recent expansion of plasma phosphor-tau (P-tau) and other biomarkers in the prediction regarding the risk of developing AD is an ideal example (Palmqvist et al., 2021). Nonetheless, there is an ongoing demand for the continual process of integrating and optimizing these synergistic advances in guiding medical decision-making in the real clinical context.
In this study, we first estimated the reassignment rates according to our prespecified principle (as mentioned earlier) using the datasets from three ongoing cohorts. Using AI techniques would allow us to compare the reassignment rates estimated by the deep learning modeling according to the actual status. Upon this perspective, our findings based on the predicted cognitive conversion at 3 and 6 months verified our hypothesis and provided preliminary recommendations on treatment reassignment at the early stage of AD or MCI.
Generally, predicted cognitive conversion according to our hybrid CNN-LSTM algorithms led to a recommendation of treatment reassignment in 46.43% (104/224) of patients at 3 months and 54.02% (121/224) at 6 months as compared with 62.05% (139/224) at 3 months (X2 = 11.017, p = 0.001) and 62.50% (140/224) at 6 months (X2 = 3.314, p = 0.069) according to their actual cognitive conversion (Figures 6–9 and Table 4). This indicated that a proportion of 74.7% of patients at 3 months and 86.4% at 6 months would be potentially benefited from deep learning modeling-guided treatment reassignment.
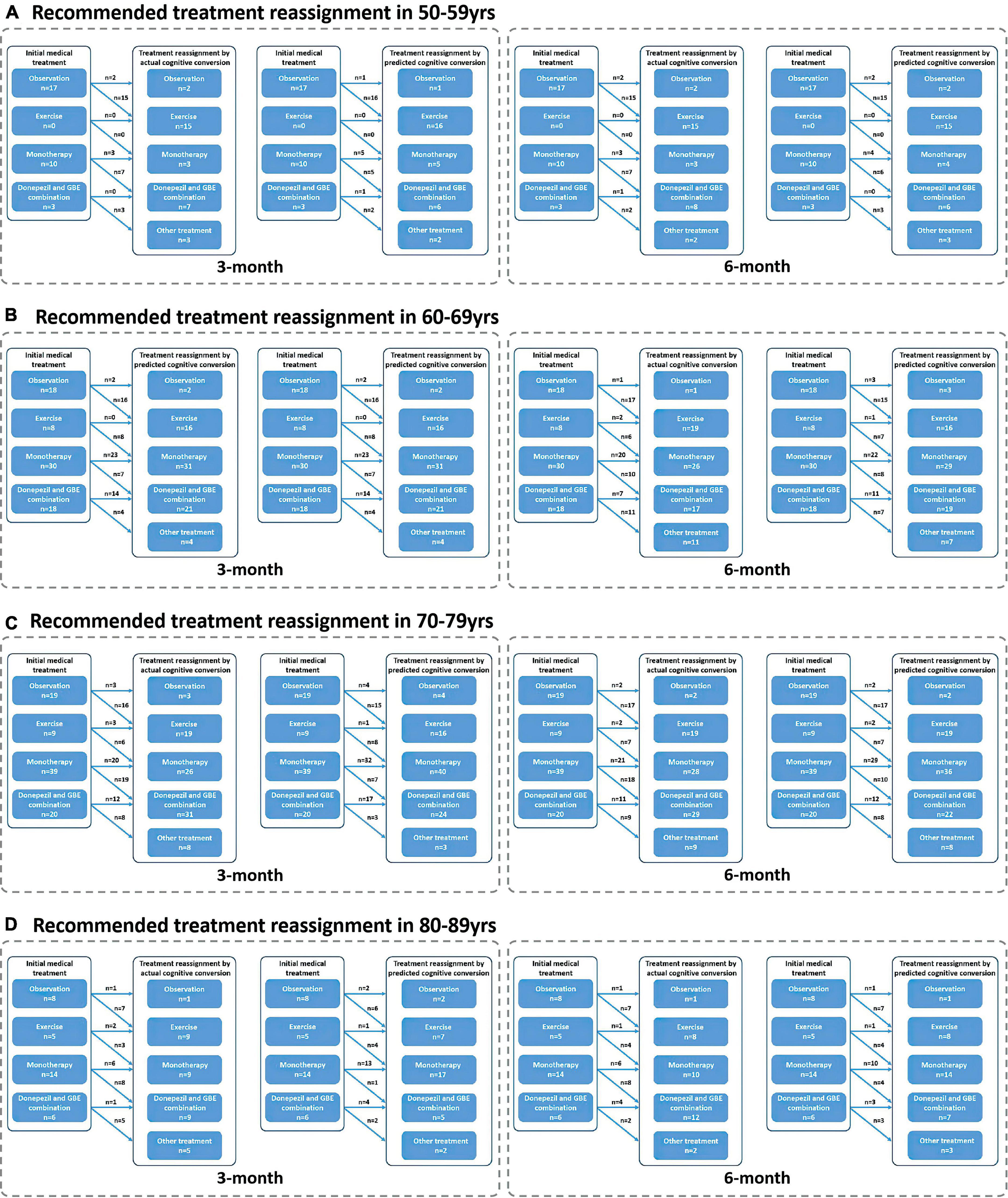
Figure 7. Recommended treatment reassignment following actual and predictive cognitive conversion according to age at 3 and 6 months. (A) Treatment reassignment according to actual and predicted cognitive conversion at 3 and 6 months in 50–59 years; (B) Treatment reassignment according to actual and predicted cognitive conversion at 3 and 6 months in 60–69 years; (C) Treatment reassignment according to actual and predicted cognitive conversion at 3 and 6 months in 70–79 years; (D) Treatment reassignment according to actual and predicted cognitive conversion at 3 and 6 months in 80–89 years. *Another treatment indicates additional memantine, psychological interventions combined with pharmacological therapy or novel pharmacological approaches involving strategies to reduce amyloid and/or tau deposition.
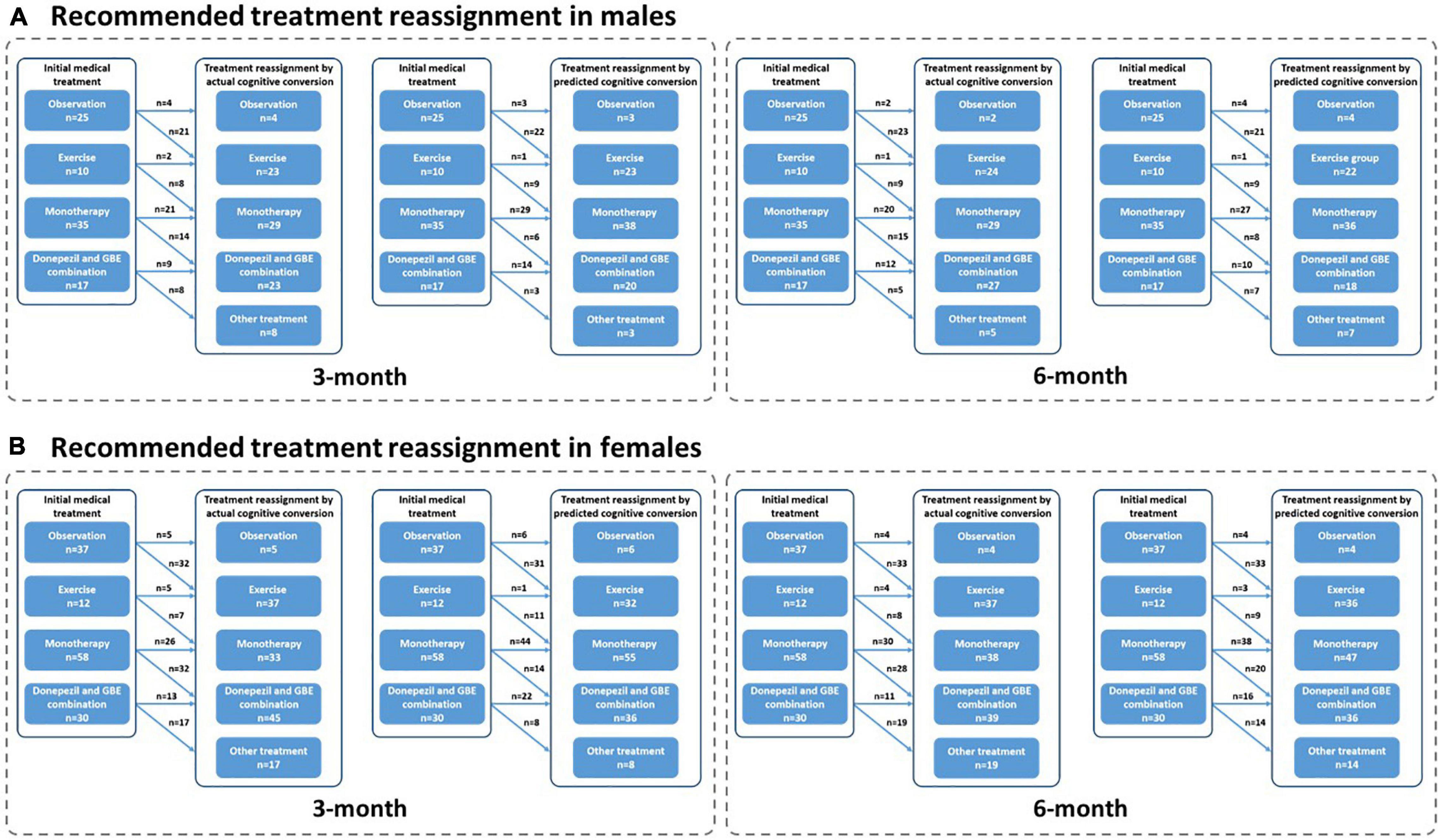
Figure 8. Recommended treatment reassignment following actual and predictive cognitive conversion according to gender at 3 and 6 months. (A) Treatment reassignment according to actual and predicted cognitive conversion at 3 and 6 months in men; (B) Treatment reassignment according to actual and predicted cognitive conversion at 3 and 6 months in women. *Another treatment indicates additional memantine, psychological interventions combined with pharmacological therapy or novel pharmacological approaches involving strategies to reduce amyloid and/or tau deposition.
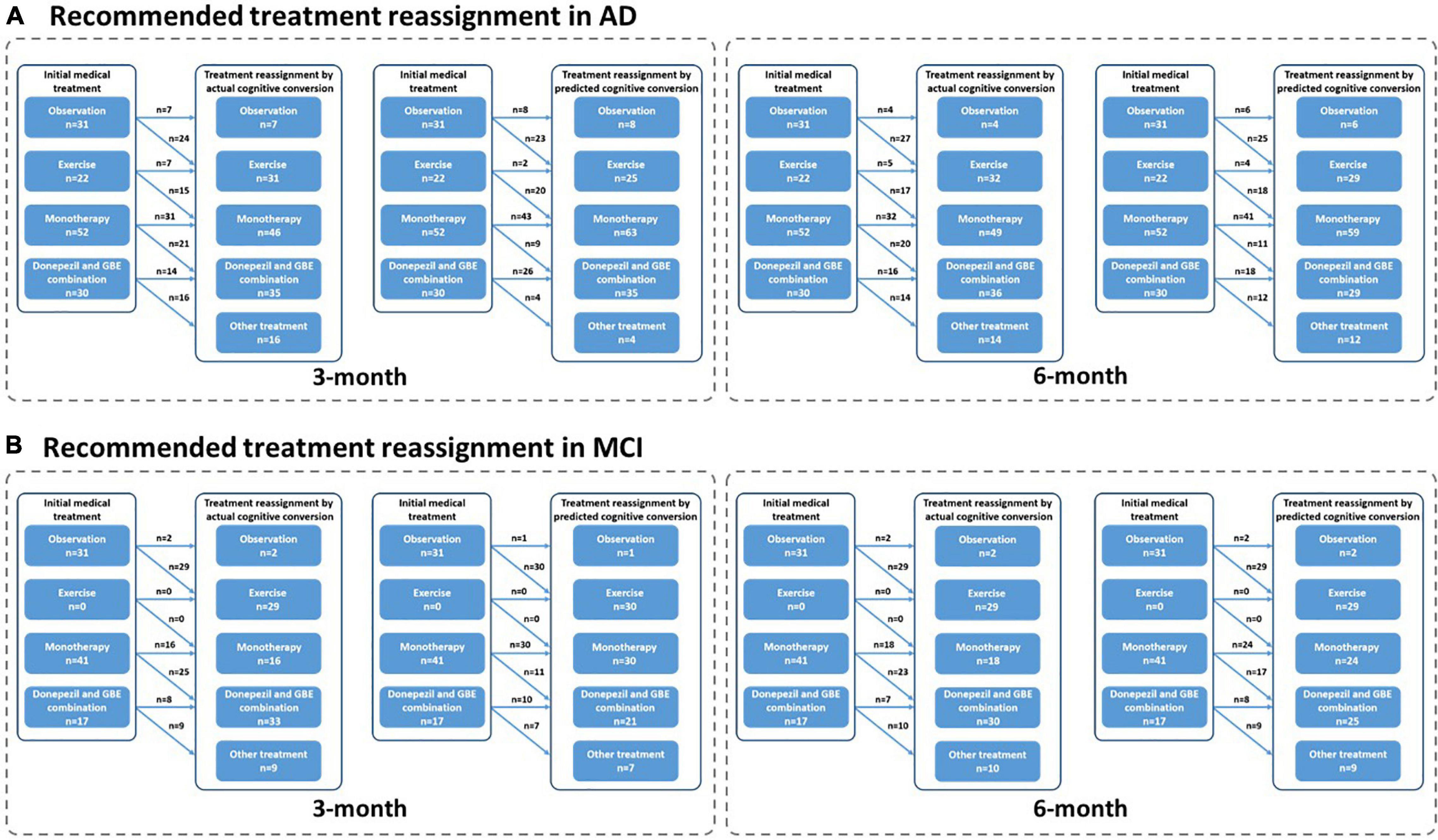
Figure 9. Recommended treatment reassignment following actual and predictive cognitive conversion according to symptom severity at 3 and 6 months. (A) Treatment reassignment according to actual and predicted cognitive conversion at 3 and 6 months in AD; (B) Treatment reassignment according to actual and predicted cognitive conversion at 3 and 6 months in MCI. *Another treatment indicates additional memantine, psychological interventions combined with pharmacological therapy or novel pharmacological approaches involving strategies to reduce amyloid and/or tau deposition.
Interestingly, patients referred for observation and treated with monotherapy (GBE/donepezil) were more likely to be reclassified according to their actual cognitive conversion. However, a discrepancy that occurred between the actual and predicted reassignment status, with 44.4% (20/43) of patients at 3 months and 65.1% (28/46) at 6 months in this subgroup to be benefited, would be mainly attributed to the declined predictive accuracy in patients treated with monotherapy (GBE/donepezil). Considering the advantages of the hybrid CNN-LSTM algorithms in handling temporal data and capturing long-term dependencies, it was not surprising that the predictive performance was superior at 6 months than 3 months (Lara-Benítez et al., 2021). This was mainly benefited from its automatic feature learning ability with multiple temporal data (e.g., data at baseline and 3 months), which further enriched the predictive performance at 6 months. However, the predictive algorithm is different at 3 months, especially without the application of CNN featured algorithms. This highlighted the importance of adding time-sequential data to compensate for the above limitation in improving reassignment accuracy, while the increased costs and workload due to additional follow-ups would be further considered and balanced. It is important to emphasize that our follow-up is limited to 6 months at present, and long-term follow-up would be valuable to assess whether predictive performance can be further improved when the cognitive decline slows down with less heterogeneity and variation (Cortes et al., 2007; Vellas et al., 2007; Rockwood et al., 2008; De Rui et al., 2014). In contrast, the debated effectiveness of monotherapy (e.g., GBE and donepezil) may also increase the predictive uncertainty at both 3 and 6 months (Schneider et al., 2005; Mazza et al., 2006; Yuan et al., 2017; Birks and Harvey, 2018). Nonetheless, our results provided opportunities when neurological clinicians recommended medical treatment upon the diagnosis of AD or MCI, our model redirected management in averagely 80% of cases according to the actual cognitive conversion at 3 and 6 months, offering the potential to avoid a cognitive decline in the future. Unfortunately, a proportion of up to 26.3% of patients was not followed by our deep learning modeling, despite the dramatic efforts to optimize predictive performance and improve reassignment accuracy. Perhaps, this reflected nuanced medical management regarding factors such as age, gender, symptom severity, individual sensitivity, and variations in response to medical treatment, compliance to medical treatment, or other factors, which were not included in this study. Results of our subgroup analysis in reassignment rates also supported the above assumption.
Importantly, our findings could be placed into the context of guidelines highlighting the evidence-based classification criteria according to symptomatology and physiopathology to enable real-world testing of the value of our proposed strategy in a fashion that may assist the decision-making upon the diagnosis of AD or MCI. Since the diversity of medical treatment was limited due to the utilization of datasets from three registered cohorts, it cannot completely represent the real-world situation. However, our study may serve as a template and has preliminarily verified by the application of deep learning modeling-guided decision-making in the treatment of dementia among AD or MCI. In fact, the currently proposed deep learning modeling successfully simulated the actual reassignment status, and several concerns are pending for investigation. For instance, the prognosis following the deep learning modeling-guided reassignment of medical treatment needs to be verified with well-designed studies before its application in real clinical circumstances. We used the increase in ADAS-cog score for more than 4 points as the cut-off to define cognitive decline. Similar to all neuropsychological assessments, the actual cognitive function may not be completely followed due to the sensitivity and specificity of ADAS-cog (Chu et al., 2000; Monllau et al., 2007; Rockwood et al., 2007; Balsis et al., 2012; Skinner et al., 2012; Verma et al., 2015). Although we reported the actual and predicted reassignment rates according to our prespecified principles, it was not totally evidence-based, indicating that some elements of referral bias cannot be excluded in this study. Our deep learning modeling was featured with handling time-sequential data especially for longitudinal cognitive scores extracted from multiple neuropsychological assessments, and the lack of data in terms of Aβ, tau, and other neurodegenerative biomarkers would consequently be assumed to impact the predictive performance of our deep learning modeling (Choi and Jin, 2018; Lee et al., 2019; Janelidze et al., 2020). In fact, plasma and CSF samples were not planned in the three registered cohort studies, highlighting that future studies must take these elements into account.
Conclusion
To sum up, we provided an example of hybrid CNN-LSTM modeling-driven early decision-tailoring in AD or MCI. Using deep learning modeling and featured longitudinal information, our hypothesis was preliminarily verified so that it may provide those suffering from cognitive decline in the future with chances to redirect treatment at the early stage. Considering the limited diversity of treatment strategies applied in this study, the real-world medical situation was not comprehensively simulated. Enlarged sample and diverse treatment datasets need to be further tested when considering the integration of this novel AI strategy into routine clinical practice of AD and MCI.
Data Availability Statement
The original contributions presented in the study are included in the article/Supplementary Material, further inquiries can be directed to the corresponding authors.
Ethics Statement
The studies involving human participants were reviewed and approved by the First Affiliated Hospital of Nanjing Medical University (Reference numbers: 2013-SRFA-089 and 2016-SR-134). The patients/participants provided their written informed consent to participate in this study.
Author Contributions
TW and YZ conceived the design of the study and prepared the original draft. TW and QH contributed to data curation, funding acquisition, supervision, and critical revision of the manuscript. YZ and YL were responsible for designing the statistical strategy. JW, YX, SY, WL, and HS were responsible for data acquisition. All authors read and contributed intellectually important content and approved the final manuscript.
Funding
This study was funded by the National Natural Science Foundation of China (Grant Nos. 81772454 and 81971237), the Jiangsu Municipal Science and Technology Bureau (Grant No. BE2017734), and the Nanjing Municipal Science and Technology Bureau (Grant No. 2019060002 [2021KF21]). The funding bodies had no role in the study design, data collection, analysis, and interpretation of data.
Conflict of Interest
The authors declare that the research was conducted in the absence of any commercial or financial relationships that could be construed as a potential conflict of interest.
Publisher’s Note
All claims expressed in this article are solely those of the authors and do not necessarily represent those of their affiliated organizations, or those of the publisher, the editors and the reviewers. Any product that may be evaluated in this article, or claim that may be made by its manufacturer, is not guaranteed or endorsed by the publisher.
Acknowledgments
The authors are grateful for the support of the medical and research staff of this study Xintong Zhang and Shenrui Wang, from the Department of Rehabilitation Medicine of the First Affiliated Hospital of Nanjing Medical University, are appreciated especially for their contribution to data processing and integration. Finally, the authors particularly appreciate the patients and families for their participation in this study.
Supplementary Material
The Supplementary Material for this article can be found online at: https://www.frontiersin.org/articles/10.3389/fnagi.2021.813923/full#supplementary-material
Abbreviations
AD, Alzheimer’s disease; MCI, mild cognitive impairment; CSF, cerebrospinal fluid; MRI, magnetic resonance imaging; FDG-PET, fluorodeoxyglucose positron emission tomography; ADAS-Cog, Alzheimer’s Disease Assessment Scale-Cognition; MMSE, Mini-Mental State Examination; GBE, ginkgo biloba extract; CNN, convolutional neural networks; LSTM, long-short-term memory; NINCDS-ADRDA, National Institute of Neurological and Communicative Disorders and Stroke and the Alzheimer’s Disease and Related Disorders Association; IADL, instrumental activity of daily living; GDS, geriatric depression scale; QOL-AD, quality of life-Alzheimer’s disease; NPI, neuropsychiatric inventory; CI, cognition improved; CNI, cognition not improved; HIS, Hachinski ischemic score; CDR, clinical dementia rating; DSST, digit symbol substitute test; TMT A, trail making test A; TMT B, trail making test B; ALT, alanine aminotransferase; TC, total cholesterol; TG, triglyceride; LDL, low density lipoprotein; ERP, event-related potentials; ROC, receiver operating curve; AUC, area under curve; CI, confidence interval; AUPRC, area under precision-recall curve; AI, artificial intelligence; P-tau, phosphor-tau; Aβ, beta-amyloid
References
Bachurin, S. O., Gavrilova, S. I., Samsonova, A., Barreto, G. E., and Aliev, G. (2018). Mild cognitive impairment due to Alzheimer disease: contemporary approaches to diagnostics and pharmacological intervention. Pharmacol. Res. 129, 216–226. doi: 10.1016/j.phrs.2017.11.021
Balsis, S., Unger, A. A., Benge, J. F., Geraci, L., and Doody, R. S. (2012). Gaining precision on the Alzheimer’s disease assessment scale-cognitive: a comparison of item response theory-based scores and total scores. Alzheimers Dement. 8, 288–294. doi: 10.1016/j.jalz.2011.05.2409
Benedetti, A., Wu, Y., Levis, B., Wilchesky, M., Boruff, J., Ioannidis, J. P. A., et al. (2018). Diagnostic accuracy of the geriatric depression scale-30, geriatric depression scale-15, geriatric depression Scale-5 and Geriatric Depression Scale-4 for detecting major depression: protocol for a systematic review and individual participant data meta-analysis. BMJ Open 8:e026598. doi: 10.1136/bmjopen-2018-026598
Birks, J. S., and Harvey, R. J. (2018). Donepezil for dementia due to Alzheimer’s disease. Cochrane Database Syst. Rev. 6:Cd001190. doi: 10.1002/14651858.CD001190.pub3
Carrillo, M. C., Blennow, K., Soares, H., Lewczuk, P., Mattsson, N., Oberoi, P., et al. (2013). Global standardization measurement of cerebral spinal fluid for Alzheimer’s disease: an update from the Alzheimer’s association global biomarkers consortium. Alzheimers Dement. 9, 137–140. doi: 10.1016/j.jalz.2012.11.003
Cho, K., Merrienboer, B. V., Bahdanau, D., and Bengio, Y. (2014). On the properties of neural machine translation: encoder-decoder approaches. arXiv [Preprint] arXiv:1409.1259,
Choi, H., and Jin, K. H. (2018). Predicting cognitive decline with deep learning of brain metabolism and amyloid imaging. Behav. Brain Res. 344, 103–109. doi: 10.1016/j.bbr.2018.02.017
Chu, L. W., Chiu, K. C., Hui, S. L., Yu, G. K., Tsui, W. J., and Lee, P. W. (2000). The reliability and validity of the Alzheimer’s disease assessment scale cognitive subscale (ADAS-Cog) among the elderly Chinese in Hong Kong. Ann. Acad. Med. Singap. 29, 474–485.
Chung, J., Gulcehre, C., Cho, K. H., and Bengio, Y. (2014). Empirical evaluation of gated recurrent neural networks on sequence modeling. arXiv [Preprint] arXiv:1412.3555,
Cortes, F., Portet, F., Touchon, J., and Vellas, B. (2007). Six and 18-month changes in mild to moderate Alzheimer’s patients treated with acetylcholinesterase inhibitors: what can we learn for clinical outcomes of therapeutic trials? J. Nutr. Health Aging 11, 330–337.
Cummings, J. L. (1993). Mini-mental state examination. norms, normals, and numbers. JAMA 269, 2420–2421.
Cummings, J. L., Isaacson, R. S., Schmitt, F. A., and Velting, D. M. (2015). A practical algorithm for managing Alzheimer’s disease: what, when, and why? Ann. Clin. Transl. Neurol. 2, 307–323. doi: 10.1002/acn3.166
Cummings, J. L., Mega, M., Gray, K., Rosenberg-Thompson, S., Carusi, D. A., and Gornbein, J. (1994). the neuropsychiatric inventory: comprehensive assessment of psychopathology in dementia. Neurology 44, 2308–2314. doi: 10.1212/wnl.44.12.2308
De Rui, M., Coin, A., Granziera, S., Girardi, A., Catanzaro, S., Manzato, E., et al. (2014). Short- and long-term efficacy of cholinesterase inhibitors in older adults with alzheimer’s disease and mixed dementia: results of a 21-month observational study. Panminerva Med. 16.
Defrancesco, M., Pechlaner, R., Kiechl, S., Willeit, J., Deisenhammer, E. A., Hinterhuber, H., et al. (2018). What characterizes depression in old age? Results from the bruneck study. Pharmacopsychiatry 51, 153–160. doi: 10.1055/s-0043-119417
Dubois, B., Feldman, H. H., Jacova, C., Dekosky, S. T., Barberger-Gateau, P., Cummings, J., et al. (2007). Research criteria for the diagnosis of Alzheimer’s disease: revising the NINCDS-ADRDA criteria. Lancet Neurol. 6, 734–746. doi: 10.1016/s1474-4422(07)70178-3
Farlow, M. R., and Cummings, J. L. (2007). Effective pharmacologic management of Alzheimer’s disease. Am. J. Med. 120, 388–397. doi: 10.1016/j.amjmed.2006.08.036
Folstein, M. F., Folstein, S. E., and McHugh, P. R. (1975). Mini-mental state. A practical method for grading the cognitive state of patients for the clinician. J. Psychiatr. Res. 12, 189–198. doi: 10.1016/0022-3956(75)90026-6
Gers, F. A., and Schmidhuber, E. (2001). LSTM recurrent networks learn simple context-free and context-sensitive languages. IEEE Trans. Neural. Netw. 12, 1333–1340. doi: 10.1109/72.963769
Giau, V. V., Bagyinszky, E., Yang, Y. S., Youn, Y. C., An, S. S. A., and Kim, S. Y. (2019). Genetic analyses of early-onset Alzheimer’s disease using next generation sequencing. Sci. Rep. 9:8368. doi: 10.1038/s41598-019-44848-2
Green, C., Handels, R., Gustavsson, A., Wimo, A., Winblad, B., Sköldunger, A., et al. (2019). Assessing cost-effectiveness of early intervention in Alzheimer’s disease: an open-source modeling framework. Alzheimers Dement. 15, 1309–1321. doi: 10.1016/j.jalz.2019.05.004
Grossberg, G. T., Tong, G., Burke, A. D., and Tariot, P. N. (2019). Present algorithms and future treatments for Alzheimer’s disease. J. Alzheimers Dis. 67, 1157–1171. doi: 10.3233/jad-180903
Hepp, D. H., Vergoossen, D. L., Huisman, E., Lemstra, A. W., Berendse, H. W., Rozemuller, A. J., et al. (2016). Distribution and load of amyloid-β pathology in parkinson disease and dementia with lewy bodies. J. Neuropathol. Exp. Neurol. 75, 936–945. doi: 10.1093/jnen/nlw070
Hill, N. T., Mowszowski, L., Naismith, S. L., Chadwick, V. L., Valenzuela, M., and Lampit, A. (2017). Computerized cognitive training in older adults with mild cognitive impairment or dementia: a systematic review and meta-analysis. Am. J. Psychiatry 174, 329–340. doi: 10.1176/appi.ajp.2016.16030360
Hochreiter, S., and Schmidhuber, J. (1997). Long short-term memory. Neural Computation 9, 1735–1780.
Hort, J., O’Brien, J. T., Gainotti, G., Pirttila, T., Popescu, B. O., Rektorova, I., et al. (2010). EFNS guidelines for the diagnosis and management of Alzheimer’s disease. Eur. J. Neurol. 17, 1236–1248. doi: 10.1111/j.1468-1331.2010.03040.x
Ioannou, G. N., Tang, W., Beste, L. A., Tincopa, M. A., Su, G. L., Van, T., et al. (2020). Assessment of a deep learning model to predict hepatocellular carcinoma in patients with hepatitis C cirrhosis. JAMA Netw. Open 3:e2015626. doi: 10.1001/jamanetworkopen.2020.15626
Jack, C. R. Jr., Bennett, D. A., Blennow, K., Carrillo, M. C., Dunn, B., Haeberlein, S. B., et al. (2018). NIA-AA research framework: toward a biological definition of Alzheimer’s disease. Alzheimers Dement. 14, 535–562. doi: 10.1016/j.jalz.2018.02.018
Jack, C. R. Jr., Petersen, R. C., Xu, Y. C., O’Brien, P. C., Smith, G. E., Ivnik, R. J., et al. (1999). Prediction of AD with MRI-based hippocampal volume in mild cognitive impairment. Neurology 52, 1397–1403. doi: 10.1212/wnl.52.7.1397
Janelidze, S., Mattsson, N., Palmqvist, S., Smith, R., Beach, T. G., Serrano, G. E., et al. (2020). Plasma P-tau181 in Alzheimer’s disease: relationship to other biomarkers, differential diagnosis, neuropathology and longitudinal progression to Alzheimer’s dementia. Nat. Med. 26, 379–386. doi: 10.1038/s41591-020-0755-1
Jiang, Y., Yang, H., Zhao, J., Wu, Y., Zhou, X., and Cheng, Z. (2020). Reliability and concurrent validity of Alzheimer’s disease assessment scale - cognitive subscale, Chinese version (ADAS-Cog-C) among Chinese community-dwelling older people population. Clin. Neuropsychol. 34(sup1) 43–53. doi: 10.1080/13854046.2020.1750704
Kang, H. (2013). The prevention and handling of the missing data. Korean J. Anesthesiol. 64, 402–406. doi: 10.4097/kjae.2013.64.5.402
Karikari, T. K., Pascoal, T. A., Ashton, N. J., Janelidze, S., Benedet, A. L., Rodriguez, J. L., et al. (2020). Blood phosphorylated tau 181 as a biomarker for Alzheimer’s disease: a diagnostic performance and prediction modelling study using data from four prospective cohorts. Lancet Neurol. 19, 422–433. doi: 10.1016/s1474-4422(20)30071-5
Killiany, R. J., Gomez-Isla, T., Moss, M., Kikinis, R., Sandor, T., Jolesz, F., et al. (2000). Use of structural magnetic resonance imaging to predict who will get Alzheimer’s disease. Ann. Neurol. 47, 430–439.
Kingma, D. P., and Ba, J. J. A. P. A. (2014). Adam: a method for stochastic optimization. arXiv [Preprint] arXiv:1412.6980,
Koszewicz, M., Jaroch, J., Brzecka, A., Ejma, M., Budrewicz, S., Mikhaleva, L. M., et al. (2021). Dysbiosis is one of the risk factor for stroke and cognitive impairment and potential target for treatment. Pharmacol. Res. 164:105277. doi: 10.1016/j.phrs.2020.105277
Langa, K. M., and Levine, D. A. (2014). The diagnosis and management of mild cognitive impairment: a clinical review. JAMA 312, 2551–2561. doi: 10.1001/jama.2014.13806
LaPlante, M. P. (2010). The classic measure of disability in activities of daily living is biased by age but an expanded IADL/ADL measure is not. J. Gerontol. B Psychol. Sci. Soc. Sci. 65, 720–732. doi: 10.1093/geronb/gbp129
Lara-Benítez, P., Carranza-García, M., and Riquelme, J. C. (2021). An experimental review on deep learning architectures for time series forecasting. Int. J. Neural. Syst. 31:2130001. doi: 10.1142/s0129065721300011
Lawton, M. P. (1997). Assessing quality of life in Alzheimer disease research. Alzheimer Dis. Assoc. Disord. 11(Suppl. 6) 91–99.
Lee, G., Nho, K., Kang, B., Sohn, K. A., and Kim, D. (2019). Predicting Alzheimer’s disease progression using multi-modal deep learning approach. Sci. Rep. 9:1952. doi: 10.1038/s41598-018-37769-z
Livingston, G., Sommerlad, A., Orgeta, V., Costafreda, S. G., Huntley, J., Ames, D., et al. (2017). Dementia prevention, intervention, and care. Lancet 390, 2673–2734. doi: 10.1016/s0140-6736(17)31363-6
Logsdon, R. G., Gibbons, L. E., McCurry, S. M., and Teri, L. (2002). Assessing quality of life in older adults with cognitive impairment. Psychosom. Med. 64, 510–519. doi: 10.1097/00006842-200205000-00016
Mazza, M., Capuano, A., Bria, P., and Mazza, S. (2006). Ginkgo biloba and donepezil: a comparison in the treatment of Alzheimer’s dementia in a randomized placebo-controlled double-blind study. Eur. J. Neurol. 13, 981–985. doi: 10.1111/j.1468-1331.2006.01409.x
Mintun, M. A., Lo, A. C., Duggan Evans, C., Wessels, A. M., Ardayfio, P. A., Andersen, S. W., et al. (2021). Donanemab in early Alzheimer’s disease. N. Engl. J. Med. 384, 1691–1704. doi: 10.1056/NEJMoa2100708
Monllau, A., Pena-Casanova, J., Blesa, R., Aguilar, M., Bohm, P., Sol, J. M., et al. (2007). [Diagnostic value and functional correlations of the ADAS-Cog scale in Alzheimer’s disease: data on NORMACODEM project]. Neurologia 22, 493–501.
Nakamura, A., Kaneko, N., Villemagne, V. L., Kato, T., Doecke, J., Doré, V., et al. (2018). High performance plasma amyloid-β biomarkers for Alzheimer’s disease. Nature 554, 249–254. doi: 10.1038/nature25456
Palmqvist, S., Janelidze, S., Stomrud, E., Zetterberg, H., Karl, J., Zink, K., et al. (2019). Performance of fully automated plasma assays as screening tests for alzheimer disease-related β-amyloid status. JAMA Neurol. 76, 1060–1069. doi: 10.1001/jamaneurol.2019.1632
Palmqvist, S., Tideman, P., Cullen, N., Zetterberg, H., Blennow, K., Dage, J. L., et al. (2021). Prediction of future Alzheimer’s disease dementia using plasma phospho-tau combined with other accessible measures. Nat. Med. 27, 1034–1042. doi: 10.1038/s41591-021-01348-z
Petersen, R. C., Roberts, R. O., Knopman, D. S., Geda, Y. E., Cha, R. H., Pankratz, V. S., et al. (2010). Prevalence of mild cognitive impairment is higher in men, The Mayo Clinic Study of Aging. Neurology 75, 889–897. doi: 10.1212/WNL.0b013e3181f11d85
Qaseem, A., Snow, V., Cross, J. T. Jr., Forciea, M. A., Hopkins, R. Jr., Shekelle, P., et al. (2008). Current pharmacologic treatment of dementia: a clinical practice guideline from the American College of physicians and the American Academy of family physicians. Ann. Intern. Med. 148, 370–378. doi: 10.7326/0003-4819-148-5-200803040-00008
Raina, P., Santaguida, P., Ismaila, A., Patterson, C., Cowan, D., Levine, M., et al. (2008). Effectiveness of cholinesterase inhibitors and memantine for treating dementia: evidence review for a clinical practice guideline. Ann. Intern. Med. 148, 379–397. doi: 10.7326/0003-4819-148-5-200803040-00009
Regan, B., Wells, Y., Farrow, M., O’Halloran, P., and Workman, B. (2017). MAXCOG-maximizing cognition: a randomized controlled trial of the efficacy of goal-oriented cognitive rehabilitation for people with mild cognitive impairment and early alzheimer disease. Am. J. Geriatr. Psychiatry 25, 258–269. doi: 10.1016/j.jagp.2016.11.008
Rockwood, K., Dai, D., and Mitnitski, A. (2008). Patterns of decline and evidence of subgroups in patients with Alzheimer’s disease taking galantamine for up to 48 months. Int. J. Geriatr. Psychiatry 23, 207–214. doi: 10.1002/gps.1864
Rockwood, K., Fay, S., Gorman, M., Carver, D., and Graham, J. E. (2007). The clinical meaningfulness of ADAS-Cog changes in Alzheimer’s disease patients treated with donepezil in an open-label trial. BMC Neurol. 7:26. doi: 10.1186/1471-2377-7-26
Rosen, W. G., Mohs, R. C., and Davis, K. L. (1984). A new rating scale for Alzheimer’s disease. Am. J. Psychiatry 141, 1356–1364. doi: 10.1176/ajp.141.11.1356
Sabbagh, M., Sadowsky, C., Tousi, B., Agronin, M. E., Alva, G., Armon, C., et al. (2020). Effects of a combined transcranial magnetic stimulation (TMS) and cognitive training intervention in patients with Alzheimer’s disease. Alzheimers Dement. 16, 641–650. doi: 10.1016/j.jalz.2019.08.197
Schneider, L. S., DeKosky, S. T., Farlow, M. R., Tariot, P. N., Hoerr, R., and Kieser, M. (2005). A randomized, double-blind, placebo-controlled trial of two doses of Ginkgo biloba extract in dementia of the Alzheimer’s type. Curr. Alzheimer Res. 2, 541–551. doi: 10.2174/156720505774932287
Shaw, L. M., Vanderstichele, H., Knapik-Czajka, M., Clark, C. M., Aisen, P. S., Petersen, R. C., et al. (2009). Cerebrospinal fluid biomarker signature in Alzheimer’s disease neuroimaging initiative subjects. Ann. Neurol. 65, 403–413. doi: 10.1002/ana.21610
Skinner, J., Carvalho, J. O., Potter, G. G., Thames, A., Zelinski, E., Crane, P. K., et al. (2012). The Alzheimer’s disease assessment scale-cognitive-plus (ADAS-Cog-Plus): an expansion of the ADAS-Cog to improve responsiveness in MCI. Brain Imaging Behav. 6, 489–501. doi: 10.1007/s11682-012-9166-3
Thomas, V. S., Rockwood, K., and McDowell, I. (1998). Multidimensionality in instrumental and basic activities of daily living. J. Clin. Epidemiol. 51, 315–321. doi: 10.1016/s0895-4356(97)00292-8
Vaswani, A., Shazeer, N., Parmar, N., Uszkoreit, J., Jones, L., Gomez, A. N., et al. (2017). Attention is all you need. arXiv [Preprint] arXiv:1706.03762,
Vellas, B., Andrieu, S., Cantet, C., Dartigues, J. F., and Gauthier, S. (2007). Long-term changes in ADAS-cog: what is clinically relevant for disease modifying trials in Alzheimer? J. Nutr. Health Aging 11, 338–341.
Verbeke, G., Fieuws, S., Molenberghs, G., and Davidian, M. (2014). The analysis of multivariate longitudinal data: a review. Stat. Methods Med. Res. 23, 42–59. doi: 10.1177/0962280212445834
Verma, N., Beretvas, S. N., Pascual, B., Masdeu, J. C., and Markey, M. K. (2015). New scoring methodology improves the sensitivity of the Alzheimer’s disease assessment scale-cognitive subscale (ADAS-Cog) in clinical trials. Alzheimers Res. Ther. 7:64. doi: 10.1186/s13195-015-0151-0
Vik-Mo, A. O., Giil, L. M., Borda, M. G., Ballard, C., and Aarsland, D. (2020). The individual course of neuropsychiatric symptoms in people with Alzheimer’s and Lewy body dementia: 12-year longitudinal cohort study. Br. J. Psychiatry 216, 43–48. doi: 10.1192/bjp.2019.195
Wu, Y. T., Beiser, A. S., Breteler, M. M. B., Fratiglioni, L., Helmer, C., Hendrie, H. C., et al. (2017). The changing prevalence and incidence of dementia over time - current evidence. Nat. Rev. Neurol. 13, 327–339. doi: 10.1038/nrneurol.2017.63
Xue, H., Zhai, J., He, R., Zhou, L., Liang, R., and Yu, H. (2018). Moderating role of positive aspects of caregiving in the relationship between depression in persons with Alzheimer’s disease and caregiver burden. Psychiatry Res. 261, 400–405. doi: 10.1016/j.psychres.2017.12.088
Yuan, Q., Wang, C. W., Shi, J., and Lin, Z. X. (2017). Effects of Ginkgo biloba on dementia: an overview of systematic reviews. J. Ethnopharmacol. 195, 1–9. doi: 10.1016/j.jep.2016.12.005
Keywords: Alzheimer’s disease, mild cognitive impairment, decision-tailoring, deep learning, Alzheimer’s disease assessment scale, cognitive conversion, medical treatment reassignment
Citation: Zheng Y, Liu Y, Wu J, Xie Y, Yang S, Li W, Sun H, He Q and Wu T (2022) Predicted Cognitive Conversion in Guiding Early Decision-Tailoring on Patients With Cognitive Impairment. Front. Aging Neurosci. 13:813923. doi: 10.3389/fnagi.2021.813923
Received: 12 November 2021; Accepted: 06 December 2021;
Published: 02 February 2022.
Edited by:
Fushun Wang, Nanjing University of Chinese Medicine, ChinaCopyright © 2022 Zheng, Liu, Wu, Xie, Yang, Li, Sun, He and Wu. This is an open-access article distributed under the terms of the Creative Commons Attribution License (CC BY). The use, distribution or reproduction in other forums is permitted, provided the original author(s) and the copyright owner(s) are credited and that the original publication in this journal is cited, in accordance with accepted academic practice. No use, distribution or reproduction is permitted which does not comply with these terms.
*Correspondence: Ting Wu, d3V0aW5nODAwMDBAMTI2LmNvbQ==; Qing He, aGVxaW5nODAwMDBAc2luYS5jb20=
†These authors have contributed equally to this work