- 1Department of Occupational and Environmental Health, School of Public Health, Guangxi Medical University, Guangxi, China
- 2Key Laboratory of Tumor Immunology and Microenvironmental Regulation, Guilin Medical University, Guilin, China
- 3Key Laboratory of Longevity and Aging-Related Diseases of Chinese Ministry of Education, Nanning, China
- 4Department of Environmental Health and Occupational Medicine, School of Public Health, Guilin Medical University, Guangxi, China
Background: The study aimed to investigate the relationship between transcription factor EB (TFEB) gene polymorphisms, including their haplotypes, and the cognitive functions of a selected population in Gongcheng County, Guangxi.
Methods: A case-control study approach was used. The case group comprised 339 individuals with cognitive impairment, as assessed by their Mini-Mental State Examination scores; the control population also comprised 339 individuals who were matched by sex and age (± 5 years) in a 1:1 ratio. TFEB gene polymorphisms were genotyped in 678 participants (190 men and 488 women, aged 30–91 years) by using the Sequenom MassARRAY platform.
Results: Multifactorial logistic regression analysis showed that in the dominant model, the risk of developing cognitive impairment was 1.547 times higher in cases with the TFEB rs14063A allele (AG + AA) than in those with the GG genotype (adjusted odds ratio [OR] = 1.547, Bonferroni correction confidence interval = 1.021–2.345). Meanwhile, the presence of the TFEB rs1062966T allele (CT + TT) was associated with a lower risk of cognitive impairment in comparison with the presence of the CC genotype (adjusted OR = 0.636, Bonferroni correction confidence interval = 0.405–0.998). In the co-dominant model, the risk of developing cognitive impairment was 1.553 times higher in carriers of the TFEB rs14063AG genotype than in carriers of the GG genotype (adjusted OR = 1.553, Bonferroni correction confidence interval = 1.007–2.397). After the Bonferroni correction and adjustment for confounding factors, the association of TFEB rs1062966 with cognitive function persisted in the analyses stratified by education level. Ethnically stratified analysis showed a significant association between TFEB rs1062966 and cognitive function in the Yao population. The multilocus linkage disequilibrium analysis indicated that the identified single nucleotide polymorphisms were not inherited independently. The haplotype analysis suggested that the rs14063A–rs1062966C–rs2278068C–rs1015149T haplotype of the TFEB gene increased the risk of cognitive impairment (P < 0.05) and that the rs14063G–rs1062966T–rs2278068C–rs1015149C haplotype was associated with a reduced risk of cognitive impairment (P < 0.05).
Conclusion: TFEB rs1062966 polymorphisms and their rs14063A–rs1062966C–rs2278068C–rs1015149T and rs14063G–rs1062966T–rs2278068C–rs1015149C haplotypes are genetic factors that may affect cognitive function among the rural Chinese population.
Introduction
Cognitive impairment, which is also regarded as a neurocognitive disorder, typically affects learning ability, memory, perceptual–motor function, language, attention, and problem solving (Robbins et al., 2019). Cognitive impairment mainly includes mild cognitive impairment and dementia. Cognitive impairment is a growing public health problem; for example, about 38.77 million people in China have been recorded as having mild cognitive impairment (Jia et al., 2020), and every year, about 8%–15% of them progress to dementia (Petersen, 2016). Previous studies have shown that cognitive function is mainly influenced by lifestyle, some genetic polymorphisms, and diseases (Lv et al., 2017; Zaki et al., 2019; Dokkedal et al., 2020; Zhang et al., 2020). Therefore, the research on genetic susceptibility can help to screen vulnerable groups and improve preventive measures.
Neurodegenerative diseases, such as Alzheimer’s disease (AD), Parkinson’s disease (PD), and Huntington’s disease (HD) are associated with dysregulation of autophagy (Cortes and La Spada, 2019). Insoluble amyloid–β (Aβ) peptide deposition is one of the key hallmarks of AD pathology (Querfurth and LaFerla, 2010; Gouras et al., 2015). In recent years, numerous studies have shown that enhanced Aβ peptide clearance helps prevent the progression of AD (Selkoe and Hardy, 2016; Sevigny et al., 2016). The induction of the autophagy–lysosome pathway is an important therapeutic strategy for the clearance of Aβ peptides (Wani et al., 2019; Luo et al., 2020). Autophagy is a pathway of cellular self-digestion and is particularly important in protein metabolism in the central nervous system (CNS). It is also a key pathway for intracellular protein clearance, and the abnormal regulation of autophagy levels and alterations in autophagic pathway-related proteins play an important role in the pathogenesis of various diseases related to neurological cognitive dysfunction (Xiao et al., 2015; Bao et al., 2016; Torra et al., 2018). Transcription factor EB (TFEB) is a master regulator for the transcription of genes involved in autophagy and lysosomal biogenesis (Settembre et al., 2011; Martini-Stoica et al., 2016) as it promotes the expression of genes required for autophagosome formation, lysosome biogenesis, and lysosome function. It is highly expressed in CNS. TFEB overexpression has been proved to ameliorate the progression of neurodegenerative diseases, including PD, HD, and AD, as well as other tauopathy diseases. A growing body of evidence suggests that protein aggregation and autophagy and/or lysosomal dysfunction are the main pathogenic mechanisms in such diseases (Menzies et al., 2015). In addition, TFEB induces the intracellular clearance of pathogenic factors in a variety of murine models of diseases, such as PD and AD (Napolitano and Ballabio, 2016). Studies have revealed that TFEB is implicated in the pathogenesis of many neurodegenerative diseases. However, the effects of TFEB gene polymorphisms on cognitive function in populations at home and abroad have not been reported.
Guangxi Gongcheng Yao Autonomous County is located in northeastern Guangxi and is characterized by a spatially aggregated population distribution, with minimal population movement and consistent lifestyle and environment. Hence, it is conducive to the study of the effects of genetic and environmental factors on cognitive function. In the present study, the cognitive function of the population in Guangxi Gongcheng County was assessed on the basis of the Mini-Mental State Examination (MMSE). In addition, the polymorphisms and haplotypes of the TFEB gene were analyzed by determining the TFEB gene polymorphisms, and the relationship between cognitive function and the TFEB gene among the Guangxi Gongcheng County population was preliminarily explored. The results of the study are expected to provide new ideas and strategies for the tertiary prevention of cognitive dysfunction in the population on the basis of the mastery of the association between cognitive function and the TFEB gene.
Materials and Methods
Study Populations
In this case–control study, the study population was drawn from the residents of Gongcheng County, Guangxi Zhuang Autonomous Region, who participated in a health survey in December 2018–December 2019. The following criteria were observed: (a) residents of the study area; (b) individuals who did not suffer from psychosis or other neurological disorders that may cause cognitive impairment, such as epilepsy, schizophrenia, alcoholism, and nervous system tumor; (c) individuals who did not suffer from severe visual or auditory impairment; (d) individuals who had not taken medication that could severely affect neurological function within the last 2 weeks. Then, 339 individuals with cognitive impairment according to the MMSE results were selected as the case group. The control population was matched by sex and age (± 5 years) at a 1:1 ratio. A total of 678 individuals aged 30–91 years were included. The research protocol was approved by the medical ethical committee of Guilin Medical University. All participants provided written informed consent.
Epidemiological Survey and Biochemical Measurements
Under the principle of informed consent, a standardized and uniform questionnaire was used. A face–to–face conversational questionnaire was administered to the study subjects by investigators who had received uniform and rigorous training. The main content of the questionnaire included information on demographics, past medical history, socioeconomic status, lifestyle, and cognitive functional status. Height (measured with a rangefinder with accuracy of up to 0.1 cm), weight (the participants were weighed with minimal clothing and with shoes off; accuracy was up to 0.1 kg), and waist circumference were measured as part of the physical examination. Body mass index (BMI) was calculated from the weight and height data, i.e., weight (kg)/height2 (m2). Fasting venous blood was taken on the day of the physical examination and transported to the Laboratory Department of Gongcheng Yao Autonomous County People’s Hospital through.
cold chain transportation method in the morning of the same day. Fasting blood glucose (FPG) was tested by a blood routine analyzer (Sysmex CS–1600, Shanghai, China). The concentrations of glycosylated hemoglobin, uric acid (UA), serum total cholesterol (TC), high–density lipoprotein cholesterol (HDL–C), low-density lipoprotein cholesterol (LDL–C), and serum triglycerides (TG) were determined using a blood biochemical detector (Hitachi 7600–020, Kyoto, Japan).
Cognitive Function Assessment
Cognitive function assessment was assessed by face-to-face interviews using the Chinese version of the MMSE. The MMSE scale is the most commonly used tool to screen for cognitive impairment in clinical, research, and community settings (Folstein et al., 1975; Ismail et al., 2010; Arevalo-Rodriguez et al., 2015) and is widely used in large–scale epidemiological studies of dementia with high validity and reliability (Cao et al., 2012; Bickel et al., 2018). MMSE assesses five dimensions, namely, orientation (10 points), attention and calculation (5 points), memory (6 points), language skills (8 points), and visual–spatial (1 point) for a total score of 30 points. High MMSE scores indicate good cognitive function. Several studies have proved that education exerts an impact on cognitive function (Crum et al., 1993; Li H. et al., 2016; Lovden et al., 2020). Given the strong influence of education on cognitive function, we used the MMSE score cut–off points for different literacy levels to determine whether the study participants suffered from cognitive decline. The cognitive impairment group was divided on the basis of a cut-off point of 16/17 (MMSE score < 17) for the participants with no formal education, 19/20 (MMSE score < 20) for those with 1–6 years of education, and 23/24 (MMSE score < 24) for those with > 6 years of education (Li H. et al., 2016); the rest belonged to the cognitively normal group.
Single Nucleotide Polymorphism Selection and Genotyping
A two--part locus screening strategy involving functional single nucleotide polymorphisms (SNPs) and validated SNPs, was used to screen for the important candidate gene TFEB. The strategy was as follows: (1) Functional regional SNP screening: The TFEB gene was searched in the NCBI-SNP1 website, and the TFEB gene promoter (upstream variant 2KB), 5′UTR, Exon (missense, synonymous), and functional SNP loci in the 3′UTR region (the relevant optimization parameter was minor allele frequency (MAF) in CHB > 0.05 according to HapMap or 1000Genomes database). The relevant literature was checked, and the results with disease susceptibility were annotated. The screened SNP loci were used for functional prediction using the NIH website2. The SNP loci screened by3 were used to perform linkage disequilibrium (LD) analysis on the SNP loci screened in the first step and annotate the fully linked loci with R (Jia et al., 2020) = 1. (2) Validated and hot SNP screening: SNP loci with susceptibility were screened via a Google Scholar search, and then the screened SNP loci were verified before functional prediction at http://snpinfo.niehs.nih.gov/. Finally, the SNP loci of the appealing candidate genes was optimized by discarding the loci with R (Jia et al., 2020) = 1 and keeping the loci with R (Jia et al., 2020) > 0.8 in the promoter region. After the appeal screening, rs1015149, rs1062966, rs14063, rs2273068, rs11754668, and rs73733015 loci of the TFEB gene for typing were screened.
Blood samples were drawn from the participants by a professional nurse. DNA was extracted from 1 mL of blood samples by using the Blood DNA Kit (Tiangen, Beijing, China). Genotyping of SNPs was conducted, and primer design and synthesis were performed by Bio Miao Biological Technology Co., Ltd. (Beijing, China) by using the Sequenom MassARRAY matrix–assisted laser desorption ionization time–of–flight mass spectrometry platform (Sequenom Inc., San Diego, CA, United States). The primer sequence information is shown in Supplementary Table 1. PCR (ABI) was used to amplify the DNA. A 384–well SpectroCHIP bioarray was used as the microarray.
Statistical Analysis
The data were statistically analyzed using SPSS22.0 statistical software. Normally distributed quantitative data were expressed in terms of mean ± SD, and the t-test of independence was used for comparison between groups. MMSE scores were not normally distributed and expressed as median and interquartile range, and comparison between groups was performed via the Wilcoxon or Kruskal–Wallis rank sum test. The qualitative data were presented in terms of percentage and were analyzed for the control and cognitive impairment groups by using a chi-square test or Fisher’s exact probability method. After adjusting for gender, age, education, and ethnicity as covariates, a multifactorial logistic regression model was used to calculate the odds ratio (OR) and 1–α confidence interval (CI) for assessing the association between the SNPs and cognitive function. A Bonferroni corrected p-value was applied to the multifactorial logistic regression p–values to account for the multiple testing of six different SNPs in the same samples (corrected α = 0.05/6 = 0.00833). LINK 1.90 software was used for the analysis of the Hardy--Weinberg equilibrium (HWE) and MAF statistics for the study population. Pair--wise LD and haplotype frequencies among the SNPs were analyzed using Haploview (Broad Institute of MIT and Harvard, United States, version 4.2). The analysis of the haplotypes comprising strongly linked SNP loci and risk of cognitive impairment was performed using SHEsis Main online software4. The criterion for significance was set at P < 0.05 for all tests.
Results
Characteristics of Studied Population
The demographic parameters of the 678 study subjects are summarized in Table 1, where the median MMSE score was 21 and the interquartile range was 17–25. No statistically significant differences in mean age, sex ratio, BMI, ethnicity, smoking status, and alcohol consumption between the control and cognitively impaired groups (P > 0.05) were observed. In addition, a significant difference in the distribution of different education levels between the two groups (P < 0.05) was noted.
Genotype and Allele Frequencies
The SNP loci rs1015149, rs1062966, rs11754668, rs14063, rs2273068, and rs73733015 of the TFEB gene were successfully typed. Hence, all the above loci were located on chromosome 6. The MAFs of each locus were all greater than 0.05, which indicated non-low frequency variants. The genotype distribution of six SNPs in the cognitive impairment and control groups met the HWE (PHWE > 0.05 for all). Table 2 shows that the allele and genotype frequencies of the TFEB rs1062966 and rs14063 SNPs in this study differed between the control and cognitive impairment groups (P < 0.05). The frequency of TFEB rs73733015C allele was higher in the cognitive impairment group than in the control group, and the difference was statistically significant between the two groups (P < 0.05).
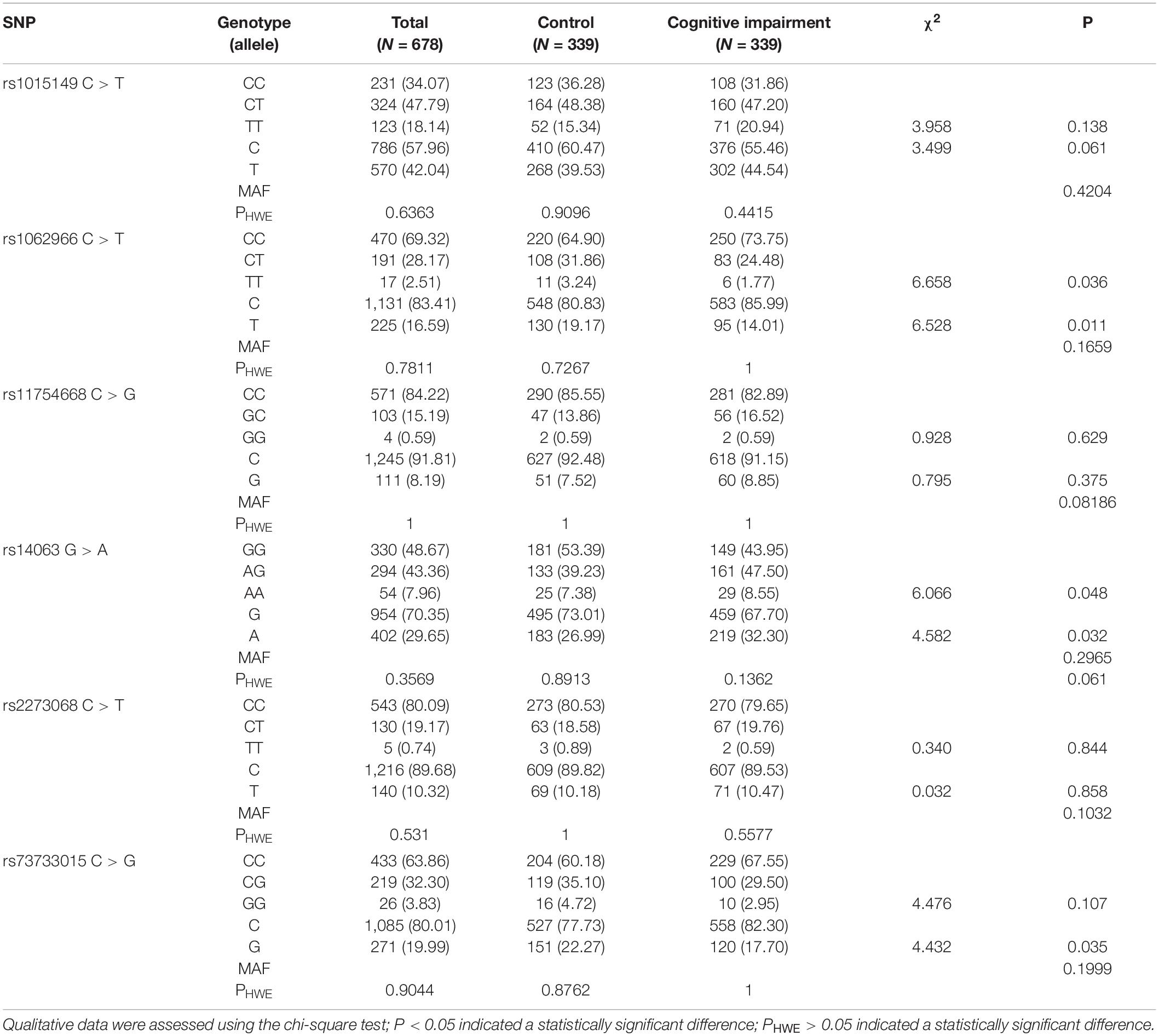
Table 2. Genotypic and allelic frequencies of TFEB SNPs in the control and cognitive impairment groups [n (%)].
Genotype and Allele Frequencies and Their Respective Associations With Cognitive Impairment
The associations of the six SNPs with cognitive impairment are shown in Table 3. Using multifactorial logistic regression, the associations of SNP locus genotypes and four SNP genetic models with cognitive impairment were analyzed. After Bonferroni correction and adjustment for age, gender, ethnicity, and education level as covariates, the association of TFEB rs1062966 and rs14063 SNPs with cognitive impairment was significant in the dominant model in the total population (P < 0.00833). Subjects carrying the TFEB rs1062966T allele (CT + TT) showed a lower risk of cognitive impairment than the subjects with the CC genotype (dominant model: adjusted OR = 0.636, Bonferroni correction confidence interval = 0.405–0.998, P = 0.008). The risk of developing cognitive impairment was 1.547 times higher in those carrying the TFEB rs14063A allele (AG + AA) than in those with the GG genotype (dominant model: adjusted OR = 1.547, Bonferroni correction confidence interval = 1.021–2.345, P = 0.006). In the co-dominant model, subjects carrying the TFEB rs14063AG genotype had an increased risk of cognitive impairment relative to those with the GG genotype, and the risk of cognitive impairment was 1.553 times higher in the AG carriers than in the GG carriers (co-dominant model: adjusted OR = 1.553, Bonferroni correction confidence interval = 1.007–2.397, P = 0.007). The association between genotype and cognitive functional status for the remaining SNP loci was not statistically significant.
Given the effect of education level on cognitive function, the study population was further stratified into three groups for analysis in accordance with the number of years of formal education received. As shown in Supplementary Table 2, after Bonferroni correction and adjustment for gender, age and ethnicity, results showed a significant association between TFEB rs1062966 and cognitive function in the group with no formal education. In the co-dominant model, the presence of the TFEB rs1062966 CT genotype was more strongly associated with a reduced risk of cognitive impairment than the presence of the CC genotype (co-dominant model: adjusted OR = 0.510, Bonferroni correction confidence interval = 0.275–0.948, P = 0.004). In the dominant model, subjects carrying the TFEB rs1062966T allele (CT + TT) had a lower risk of cognitive impairment than those carrying the CC genotype (dominant model: adjusted OR = 0.510, Bonferroni correction confidence interval = 0.281–0.928, P = 0.003). In the overdominant model, the presence of the TFEB rs1062966CT genotype was more closely associated with a reduced risk of cognitive dysfunction than the presence of the CC + TT genotype (overdominant model: adjusted OR = 0.527, Bonferroni correction confidence interval = 0.285–0.974, P = 0.006). The remaining SNP loci were not statistically associated with cognitive function in any of the four genetic models (P > 0.0833).
Ethnic differences in genetic background might exist in different racial/ethnic groups. Therefore, the study population was further stratified by ethnicity for analysis. As shown in Supplementary Table 3, after Bonferroni correction and adjustment for sex, age, and education level, results showed a significant association between TFEB rs1062966 and cognitive function in the Yao minority group. In the co-dominant model, subjects carrying the CT genotype had a lower risk of cognitive impairment than those carrying the CC genotype (co-dominant model: adjusted OR = 0.563, Bonferroni correction confidence interval = 0.317–0.999, P = 0.008). In the dominant model, subjects carrying the TFEB rs1062966T allele (CT + TT) showed a lower risk of cognitive impairment than those carrying the CC genotype (dominant model: adjusted OR = 0.543, Bonferroni correction confidence interval = 0.311–0.946, P = 0.004). In other ethnic groups, SNP loci were not statistically different from cognitive function in any of the four genetic models (P > 0.0833).
Haplotypes and the Risk of Cognitive Impairment
Figure 1 shows the LD analysis of the SNPs of TFEB. Multilocus LD analyses indicated that the tested sites in the study population were not statistically independent and that the control and cognitive impairment groups showed strong LD (D’ = 0.89–0.99). As shown in Table 4, the dominant haplotypes were rs14063G–rs1062966C–rs2278068C–rs1015149C (>40% of the samples) and rs14063A–rs1062966C–rs2278068C–rs1015149T (>20% of the samples). The rs14063A–rs1062966C–rs2278068C–rs1015149T and rs14063G–rs1062966T–rs2278068C–rs1015149C haplotype frequencies were statistically different between the two groups (P < 0.05 for all). The rs14063A–rs1062966C–rs2278068C–rs1015149T haplotype was associated with an increased risk of cognitive impairment (OR = 1.370, 95% CI = 1.044–1.797, P = 0.022797), whereas the rs14063G–rs1062966T–rs2278068C–rs1015149C haplotype.
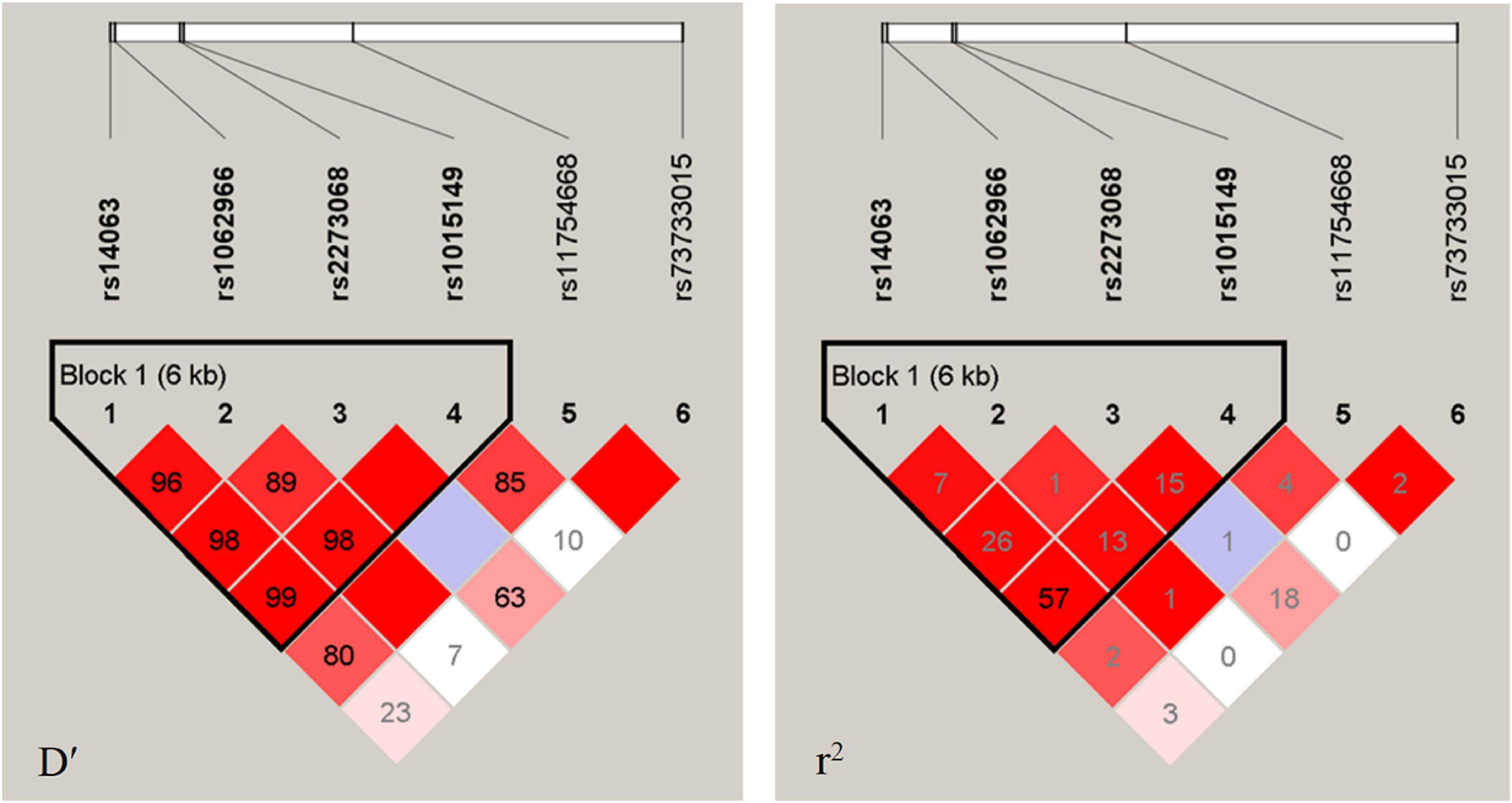
Figure 1. LD analysis of the TFEB SNPS in both populations. The LD degree was represented by pair-wise D′ and r2. The strength of LD is reflected by the color, and the correlation of LD increases as the color increases, with white being the weakest and crimson being the strongest.

Table 4. Prevalence of haplotype frequencies in the cognitive impairment and control groups [n (frequency)].
Was protective against cognitive function (OR = 0.690, 95% CI = 0.517–0.922, P = 0.011908).
Discussion
To our knowledge, our findings suggest for the first time that TFEB gene polymorphisms affect cognitive function. The main findings of the current research included the following aspects (1) TFEB rs1062966 SNP and rs14063G–rs1062966T–rs2278068C–rs1015149C haplotypes in the total population’s TFEB gene are associated with a reduced risk of cognitive impairment, whereas rs14063 SNP and rs14063A–rs1062966C–rs2278068C–rs1015149T haplotypes increase the risk of cognitive impairment. (2) The stratified analysis of education level shows that in the population with no formal education, the TFEB rs1062966 SNP is significantly associated with cognitive function. (3) Ethnically stratified analysis shows a significant association between TFEB rs1062966 SNP and cognitive function was observed in the Yao population.
TFEB is a basic helix–loop–helix leucine zipper transcription factor. To our knowledge, cognitive decline may be associated with an imbalance in the production and clearance of β-amyloid. TFEB is a major regulator of the lysosomal pathway, and lysosomal dysfunction is speculated to have a central role in enhanced Aβ generation (Lee et al., 2010; Neely et al., 2011; Coen et al., 2012; McBrayer and Nixon, 2013) or its impaired clearance (Majumdar et al., 2011; Lee et al., 2012). Therefore, one of the possible explanations for the effect of TFEB on cognitive function in the present study is that TFEB is a master inducer of lysosomal biogenesis (Settembre et al., 2011), especially in astrocytes. It stimulates lysosomal biogenesis and function, accelerates Aβ uptake and degradation, and significantly reduces Aβ levels (Xiao et al., 2014). TFEB promotes autophagosome–lysosomal fusion (Palmieri et al., 2011) to attenuate the effective trafficking of Aβ production. In addition, TFEB can dynamically regulate ALP by coordinating autophagy induction with enhanced lysosomal clearance (Polito et al., 2014). In response to AD, ALP has been shown to regulate amyloid precursor protein (APP) turnover and Aβ metabolism (Nixon and Yang, 2011; Harris and Rubinsztein, 2012), and enhanced TFEB function can stimulate ALP function and promote protein clearance and neuroprotection (Sardiello et al., 2009; Tsunemi et al., 2012).
Other studies found that exogenous TFEB expression stimulates lysosomal biogenesis in vitro, reduces APP full length and its shear fragments, attenuates Aβ production and release, and significantly shortens the half-life of APP in a lysosome-dependent manner (Xiao et al., 2015). The aberrant expression or degradation APP is associated with AD. Therefore, as a strategy to reduce Aβ production, accelerating the degradation of the whole APP in lysosomes is effective. The levels of the APP degradation product sAPPα are correlated with MMSE scores (Rosen et al., 2014; Yun et al., 2020) and sAPPα functions to protect synaptic plasticity and learning memory (Taylor et al., 2008). This finding is consistent with our finding that TFEB rs1062966SNP plays a protective role in cognitive function.
Furthermore, evidence suggests that the Tau protein hyperphosphorylation results in the formation of neurofibrillary tangles, which affect cognitive function (Mun et al., 2016), and TFEB can prevent cognitive impairment by selectively targeting the pathological tau species for clearance through the potent activation of multiple cellular degradative pathways (Polito et al., 2014; Wang et al., 2016; Chandra et al., 2018; Song et al., 2020; Xu et al., 2020). Moreover, a negative correlation has been observed between tau protein concentration and MMSE scores (Chen et al., 2019; Obrocki et al., 2020). Another study found that neuronal TFEB expression also reduces the half-life of Aβ (Xiao et al., 2015). TFEB transduction in neurons may stimulate Aβ uptake through macropinocytosis (Holmes et al., 2013). The findings of these studies suggested a beneficial role for TFEB in cognitive function. However, increased APP endocytosis by TFEB activation reportedly leads to enhanced β- and γ-cleavage, thereby accelerating Aβ production (Schneider et al., 2008; Xiao et al., 2012). This finding may explain why some individuals are at lower risk of cognitive impairment than others, whereas the predisposition of some persons to suffer cognitive deficits is due to autophagy impairment. In addition, the TFEB rs1062966 SNP is found to be associated with cognitive function in the Yao population in present study. Our results support previous research on racial/ethnic differences in cognition (Li M. et al., 2016; Tsai, 2018). This finding may be because different ethnic groups have different genetic backgrounds, or may be because the TFEB gene polymorphism is only part of a polygenic pattern that, together with environmental factors, acts in combination on cognitive function. Further investigation needs to be done in a large sample size. The association between TFEB gene polymorphisms and cognitive impairment observed in the present study indicates that polymorphisms at some SNP loci of the TFEB gene may affect TFEB gene expression and cognitive function. However, the exact mechanism of the association between TFEB gene polymorphisms and cognitive dysfunction is not clear. This issue may be explained at the molecular level by studying TFEB polymorphisms.
The following are the advantages of this study: (1) The study subjects are all from the same region with minimal differences in living environment and habits; these characteristics helped control the confounding factors. (2) On the basis of mastering the association between cognitive function and TFEB genes, new therapeutic targets for cognitive disorders may be provided. (3) Identifying the differences in TFEB mutation frequencies in the populations of the rural areas of Guangxi may provide new data for human genome research. The following are the potential limitations of this study: (1) One of the limitations of present study is its small sample size. Nevertheless, this study, being the first on TFEB gene polymorphism and cognitive function, may lay the foundation for future studies involving large samples. (2) Although the effect of the six SNPs of TFEB on cognitive function were detected, many potentially cognitive function-related SNPs were overlooked in the current study. (3) Although the association of TFEB SNPs with cognitive function in the present study was detected, many unmeasured environmental and genetic factors still need to be considered.
Conclusion
In conclusion, TFEB rs1062966 polymorphisms and their rs14063A–rs1062966C–rs2278068C–rs1015149T and rs14063G–rs1062966T–rs2278068C–rs1015149C haplotypes are genetic factors that may affect cognitive function among the rural Chinese population. The findings must be further investigated by prospective studies with large samples.
Data Availability Statement
The original contributions presented in the study are included in the article/Supplementary Material, further inquiries can be directed to the corresponding author/s.
Ethics Statement
The studies involving human participants were reviewed and approved by the Medical Ethical Committee of Guilin Medical University. The patients/participants provided their written informed consent to participate in this study.
Author Contributions
YW and SL analyzed the data and wrote the manuscript. JC analyzed the data and revised the manuscript. XT designed the study and reviewed the manuscript. JZ, MX, QL, CW, XM, SH, YL, TM, DT, TL, RG, and HL collected the relevant data. ZZ and JQ put forward the study topic and provided advice on the writing of the manuscript. All authors read and approved the final manuscript.
Funding
This study was supported by the National Natural Science Foundation of China (grant Nos. 81760577, 81960583, and 81560523), the Guangxi Science and Technology Development Project (grant Nos. AD17129003 and AD18050005), the Guangxi Natural Science Found for Innovation Research Team (grant No. 2019GXNSFGA245002), and the Guangxi Scholarship Fund of Guangxi Education Department of China.
Conflict of Interest
The authors declare that the research was conducted in the absence of any commercial or financial relationships that could be construed as a potential conflict of interest.
Publisher’s Note
All claims expressed in this article are solely those of the authors and do not necessarily represent those of their affiliated organizations, or those of the publisher, the editors and the reviewers. Any product that may be evaluated in this article, or claim that may be made by its manufacturer, is not guaranteed or endorsed by the publisher.
Acknowledgments
We are deeply appreciative of the participants in this study, and we thank all the staff for their support and assistance.
Supplementary Material
The Supplementary Material for this article can be found online at: https://www.frontiersin.org/articles/10.3389/fnagi.2021.757992/full#supplementary-material
Footnotes
- ^ http://www.ncbi.nlm.nih.gov/snp
- ^ http://snpinfo.niehs.nih.gov/
- ^ http://asia.ensembl.org/Homo_sapiens/Tools/LD?db=cor
- ^ http://analysis.bio-x.cn/myAnalysis.php
References
Arevalo-Rodriguez, I., Smailagic, N., Roqué, I., Figuls, M., Ciapponi, A., Sanchez-Perez, E., et al. (2015). Mini-Mental State Examination (MMSE) for the detection of Alzheimer’s disease and other dementias in people with mild cognitive impairment (MCI). Cochrane Database Syst. Rev. 2015:CD010783. doi: 10.1002/14651858
Bao, J., Zheng, L., Zhang, Q., Li, X., Zhang, X., Li, Z., et al. (2016). Deacetylation of TFEB promotes fibrillar Abeta degradation by upregulating lysosomal biogenesis in microglia. Protein Cell 7, 417–433. doi: 10.1007/s13238-016-0269-2
Bickel, H., Hendlmeier, I., Hessler, J. B., Junge, M. N., Leonhardt-Achilles, S., Weber, J., et al. (2018). The prevalence of dementia and cognitive impairment in hospitals. Dtsch Arztebl Int. 115, 733–740.
Cao, L., Hai, S., Lin, X. F., Shu, D. F., Wang, S., Yue, J. R., et al. (2012). Comparison of the saint louis university mental status examination, the mini-mental state examination, and the montreal cognitive assessment in detection of cognitive impairment in chinese elderly from the geriatric department. J. Am. Med. Dir. Assoc. 13, 626–629.
Chandra, S., Jana, M., and Pahan, K. (2018). Aspirin induces lysosomal biogenesis and attenuates amyloid plaque pathology in a mouse model of Alzheimer’s disease via PPARalpha. J. Neurosci. 38, 6682–6699. doi: 10.1523/JNEUROSCI.0054-18.2018
Chen, T. B., Lai, Y. H., Ke, T. L., Chen, J. P., Lee, Y. J., Lin, S. Y., et al. (2019). Changes in plasma amyloid and tau in a longitudinal study of normal aging, mild cognitive impairment, and Alzheimer’s disease. Dement. Geriatr. Cogn. Disord. 48, 180–195. doi: 10.1159/000505435
Coen, K., Flannagan, R. S., Baron, S., Carraro-Lacroix, L. R., Wang, D., Vermeire, W., et al. (2012). Lysosomal calcium homeostasis defects, not proton pump defects, cause endo-lysosomal dysfunction in PSEN-deficient cells. J. Cell Biol. 198, 23–35. doi: 10.1083/jcb.201201076
Cortes, C. J., and La Spada, A. R. (2019). TFEB dysregulation as a driver of autophagy dysfunction in neurodegenerative disease: molecular mechanisms, cellular processes, and emerging therapeutic opportunities. Neurobiol. Dis. 122, 83–93.
Crum, R. M., Anthony, J. C., Bassett, S. S., and Folstein, M. F. (1993). Population-based norms for the mini-mental state examination by age and educational level. JAMA 269, 2386–2391.
Dokkedal, U., Wod, M., Thinggaard, M., Hansen, T. G., Rasmussen, L. S., Christensen, K., et al. (2020). Apolipoprotein E epsilon4 and cognitive function after surgery in middle-aged and elderly Danish twins. Eur. J. Anaesthesiol. 37, 984–991. doi: 10.1097/EJA.0000000000001250
Folstein, M. F., Folstein, S. E., and McHugh, P. R. (1975). Mini-mental state”. a practical method for grading the cognitive state of patients for the clinician. J. Psychiatr. Res. 12, 189–198.
Gouras, G. K., Olsson, T. T., and Hansson, O. (2015). beta-Amyloid peptides and amyloid plaques in Alzheimer’s disease. Neurotherapeutics 12, 3–11. doi: 10.1007/s13311-014-0313-y
Harris, H., and Rubinsztein, D. C. (2012). Control of autophagy as a therapy for neurodegenerative disease. Nat. Rev. Neurol. 8, 108–117.
Holmes, B. B., DeVos, S. L., Kfoury, N., Li, M., Jacks, R., Yanamandra, K., et al. (2013). Heparan sulfate proteoglycans mediate internalization and propagation of specific proteopathic seeds. Proc. Natl. Acad. Sci. U S A 110, E3138–E3147. doi: 10.1073/pnas.1301440110
Ismail, Z., Rajji, T. K., and Shulman, K. I. (2010). Brief cognitive screening instruments: an update. Int. J. Geriatr. Psychiatry 25, 111–120. doi: 10.1002/gps.2306
Jia, L., Du, Y., Chu, L., Zhang, Z., Li, F., Lyu, D., et al. (2020). Prevalence, risk factors, and management of dementia and mild cognitive impairment in adults aged 60 years or older in China: a cross-sectional study. Lancet Public Health 5, e661–e671.
Lee, C. Y. D., Tse, W., Smith, J. D., and Landreth, G. E. (2012). Apolipoprotein E promotes beta-amyloid trafficking and degradation by modulating microglial cholesterol levels. J. Biol. Chem. 287, 2032–2044. doi: 10.1074/jbc.M111.295451
Lee, J. H., Yu, W. H., Kumar, A., Lee, S., Mohan, P. S., Peterhoff, C. M., et al. (2010). Lysosomal proteolysis and autophagy require presenilin 1 and are disrupted by Alzheimer-related PS1 mutations. Cell 141, 1146–1158.
Li, H., Jia, J., and Yang, Z. (2016). Mini-Mental state examination in elderly Chinese: a population-based normative study. J. Alzheimers. Dis. 53, 487–496. doi: 10.3233/JAD-160119
Li, M., Chang, H., and Xiao, X. (2016). BDNF Val66Met polymorphism and bipolar disorder in European populations: a risk association in case-control, family-based and GWAS studies. Neurosci. Biobehav. Rev. 68, 218–233.
Lovden, M., Fratiglioni, L., Glymour, M. M., Lindenberger, U., and Tucker-Drob, E. M. (2020). Education and cognitive functioning across the life span. Psychol. Sci. Public Interest. 21, 6–41. doi: 10.1177/1529100620920576
Luo, R., Su, L. Y., Li, G., Yang, J., Liu, Q., Yang, L. X., et al. (2020). Activation of PPARA-mediated autophagy reduces Alzheimer disease-like pathology and cognitive decline in a murine model. Autophagy 16, 52–69.
Lv, Y. B., Zhu, P. F., Yin, Z. X., Kraus, V. B., Threapleton, D., Chei, C. L., et al. (2017). A u-shaped association between blood pressure and cognitive impairment in chinese elderly. J. Am. Med. Dir. Assoc. 18, 193.e7–193.e13.
Majumdar, A., Capetillo-Zarate, E., Cruz, D., Gouras, G. K., and Maxfield, F. R. (2011). Degradation of Alzheimer’s amyloid fibrils by microglia requires delivery of ClC-7 to lysosomes. Mol. Biol. Cell 22, 1664–1676. doi: 10.1091/mbc.E10-09-0745
Martini-Stoica, H., Xu, Y., Ballabio, A., and Zheng, H. (2016). The autophagy-lysosomal pathway in neurodegeneration: a TFEB perspective. Trends Neurosci. 39, 221–234.
McBrayer, M., and Nixon, R. A. (2013). Lysosome and calcium dysregulation in Alzheimer’s disease: partners in crime. Biochem. Soc. Trans. 41, 1495–1502. doi: 10.1042/BST20130201
Menzies, F. M., Fleming, A., and Rubinsztein, D. C. (2015). Compromised autophagy and neurodegenerative diseases. Nat. Rev. Neurosci. 16, 345–357. doi: 10.1038/nrn3961
Mun, M. J., Kim, J. H., Choi, J. Y., and Jang, W. C. (2016). Genetic polymorphisms of interleukin genes and the risk of Alzheimer’s disease: an update meta-analysis. Meta Gene 8, 1–10.
Napolitano, G., and Ballabio, A. (2016). TFEB at a glance. J. Cell Sci. 129, 2475–2481. doi: 10.1242/jcs.146365
Neely, K. M., Green, K. N., and LaFerla, F. M. (2011). Presenilin is necessary for efficient proteolysis through the autophagy-lysosome system in a gamma-secretase-independent manner. J. Neurosci. 31, 2781–2791. doi: 10.1523/Jneurosci.5156-10.2010
Nixon, R. A., and Yang, D. S. (2011). Autophagy failure in Alzheimer’s disease–locating the primary defect. Neurobiol. Dis. 43, 38–45.
Obrocki, P., Khatun, A., Ness, D., Senkevich, K., Hanrieder, J., Capraro, F., et al. (2020). Perspectives in fluid biomarkers in neurodegeneration from the 2019 biomarkers in neurodegenerative diseases course-a joint PhD student course at University College London and University of Gothenburg. Alzheimers Res. Ther. 12:20. doi: 10.1186/s13195-020-00586-6
Palmieri, M., Impey, S., Kang, H., di Ronza, A., Pelz, C., Sardiello, M., et al. (2011). Characterization of the CLEAR network reveals an integrated control of cellular clearance pathways. Hum. Mol. Genet. 20, 3852–3866. doi: 10.1093/hmg/ddr306
Petersen, R. C. (2016). Mild cognitive impairment. Continuum (Minneap Minn) 22, 404–418. doi: 10.1212/CON.0000000000000313
Polito, V. A., Li, H., Martini-Stoica, H., Wang, B., Yang, L., Xu, Y., et al. (2014). Selective clearance of aberrant tau proteins and rescue of neurotoxicity by transcription factor EB. EMBO Mol. Med. 6, 1142–1160. doi: 10.15252/emmm.201303671
Querfurth, H. W., and LaFerla, F. M. (2010). Alzheimer’s disease. N. Engl. J. Med. 362, 329–344. doi: 10.1056/NEJMra0909142
Robbins, R. N., Scott, T., Joska, J. A., and Gouse, H. (2019). Impact of urbanization on cognitive disorders. Curr. Opin. Psychiatry 32, 210–217. doi: 10.1097/YCO.0000000000000490
Rosen, C., Rosen, H., Andreasson, U., Bremell, D., Bremler, R., Hagberg, L., et al. (2014). Cerebrospinal fluid biomarkers in cardiac arrest survivors. Resuscitation 85, 227–232. doi: 10.1016/j.resuscitation.2013
Sardiello, M., Palmieri, M., di Ronza, A., Medina, D. L., Valenza, M., Gennarino, V. A., et al. (2009). A gene network regulating lysosomal biogenesis and function. Science 325, 473–477. doi: 10.1126/science.1174447
Schneider, A., Rajendran, L., Honsho, M., Gralle, M., Donnert, G., Wouters, F., et al. (2008). Flotillin-dependent clustering of the amyloid precursor protein regulates its endocytosis and amyloidogenic processing in neurons. J. Neurosci. 28, 2874–2882. doi: 10.1523/JNEUROSCI.5345-07.2008
Selkoe, D. J., and Hardy, J. (2016). The amyloid hypothesis of Alzheimer’s disease at 25 years. EMBO Mol. Med. 8, 595–608. doi: 10.15252/emmm.201606210
Settembre, C., Di Malta, C., Polito, V. A., Garcia Arencibia, M., Vetrini, F., Erdin, S., et al. (2011). TFEB links autophagy to lysosomal biogenesis. Science 332, 1429–1433. doi: 10.1126/science.1204592
Sevigny, J., Chiao, P., Bussiere, T., Weinreb, P. H., Williams, L., Maier, M., et al. (2016). The antibody aducanumab reduces Abeta plaques in Alzheimer’s disease. Nature 537, 50–56. doi: 10.1038/nature19323
Song, J. X., Malampati, S., Zeng, Y., Durairajan, S. S. K., Yang, C. B., Tong, B. C., et al. (2020). A small molecule transcription factor EB activator ameliorates beta-amyloid precursor protein and Tau pathology in Alzheimer’s disease models. Aging Cell 19:e13069. doi: 10.1111/acel.13069
Taylor, C. J., Ireland, D. R., Ballagh, I., Bourne, K., Marechal, N. M., Turner, P. R., et al. (2008). Endogenous secreted amyloid precursor protein-alpha regulates hippocampal NMDA receptor function, long-term potentiation and spatial memory. Neurobiol. Dis. 31, 250–260.
Torra, A., Parent, A., Cuadros, T., Rodríguez-Galván, B., Ruiz-Bronchal, E., Ballabio, A., et al. (2018). Overexpression of TFEB drives a pleiotropic neurotrophic effect and prevents Parkinson’s disease-related neurodegeneration. MolecularTherapy 26, 1552–1567.
Tsai, S. J. (2018). Critical issues in BDNF Val66Met genetic studies of neuropsychiatric disorders. Front. Mol. Neurosci. 11:156. doi: 10.3389/fnmol.2018.00156
Tsunemi, T., Ashe, T. D., Morrison, B. E., Soriano, K. R., Au, J., Roque, R. A., et al. (2012). PGC-1alpha rescues Huntington’s disease proteotoxicity by preventing oxidative stress and promoting TFEB function. Sci. Transl. Med. 4:142ra97. doi: 10.1126/scitranslmed.3003799
Wang, H., Wang, R., Carrera, I., Xu, S., and Lakshmana, M. K. (2016). TFEB overexpression in the P301S model of tauopathy mitigates increased PHF1 levels and lipofuscin puncta and rescues memory deficits. eNeuro 3:ENEURO.0042-16.2016.
Wani, A., Gupta, M., Ahmad, M., Shah, A. M., Ahsan, A. U., Qazi, P. H., et al. (2019). Alborixin clears amyloid-beta by inducing autophagy through PTEN-mediated inhibition of the AKT pathway. Autophagy 15, 1810–1828.
Xiao, Q., Gil, S. C., Yan, P., Wang, Y., Han, S., Gonzales, E., et al. (2012). Role of phosphatidylinositol clathrin assembly lymphoid-myeloid leukemia (PICALM) in intracellular amyloid precursor protein (APP) processing and amyloid plaque pathogenesis. J. Biol. Chem. 287, 21279–21289. doi: 10.1074/jbc.M111.338376
Xiao, Q., Yan, P., Ma, X., Liu, H., Perez, R., Zhu, A., et al. (2014). Enhancing astrocytic lysosome biogenesis facilitates Abeta clearance and attenuates amyloid plaque pathogenesis. J. Neurosci. 34, 9607–9620. doi: 10.1523/JNEUROSCI.3788-13.2014
Xiao, Q., Yan, P., Ma, X., Liu, H., Perez, R., Zhu, A., et al. (2015). Neuronal-Targeted TFEB accelerates lysosomal degradation of APP, reducing abeta generation and amyloid plaque pathogenesis. J. Neurosci. 35, 12137–12151. doi: 10.1523/JNEUROSCI.0705-15.2015
Xu, Y., Du, S., Marsh, J. A., Horie, K., Sato, C., Ballabio, A., et al. (2020). TFEB regulates lysosomal exocytosis of tau and its loss of function exacerbates tau pathology and spreading. Mol. Psychiatry doi: 10.1038/s41380-020-0738-0Online ahead of print.
Yun, S. M., Cho, S. J., Jo, C., Park, M. H., Han, C., and Koh, Y. H. (2020). Elevation of plasma soluble amyloid precursor protein beta in Alzheimer’s disease. Arch. Gerontol. Geriatr. 87:103995.
Zaki, N. F. W., Saleh, E., Elwasify, M., Mahmoud, E., Zaki, J., Spence, D. W., et al. (2019). The association of BDNF gene polymorphism with cognitive impairment in insomnia patients. Prog. Neuro-Psychopharmacol. Biol. Psychiatry 88, 253–264.
Zhang, P. D., Lv, Y. B., Li, Z. H., Yin, Z. X., Li, F. R., Wang, J. N., et al. (2020). Age, period, and cohort effects on activities of daily living, physical performance, and cognitive functioning impairment among the oldest-old in China. J. Gerontol. A Biol. Sci. Med. Sci. 75, 1214–1221. doi: 10.1093/gerona/glz196
Keywords: cognitive function, TFEB, single nucleotide polymorphism, haplotype, autophagy
Citation: Wei Y, Liu S, Cai J, Tang X, Zhang J, Xu M, Liu Q, Wei C, Mo X, Huang S, Lin Y, Mai T, Tan D, Luo T, Gou R, Lu H, Qin J and Zhang Z (2021) Associations of TFEB Gene Polymorphisms With Cognitive Function in Rural Chinese Population. Front. Aging Neurosci. 13:757992. doi: 10.3389/fnagi.2021.757992
Received: 13 August 2021; Accepted: 19 November 2021;
Published: 14 December 2021.
Edited by:
Hongguang Xia, Zhejiang University, ChinaReviewed by:
Jose Felix Moruno-Manchon, University of Texas Health Science Center at Houston, United StatesBo Yan, Jining Medical University, China
Copyright © 2021 Wei, Liu, Cai, Tang, Zhang, Xu, Liu, Wei, Mo, Huang, Lin, Mai, Tan, Luo, Gou, Lu, Qin and Zhang. This is an open-access article distributed under the terms of the Creative Commons Attribution License (CC BY). The use, distribution or reproduction in other forums is permitted, provided the original author(s) and the copyright owner(s) are credited and that the original publication in this journal is cited, in accordance with accepted academic practice. No use, distribution or reproduction is permitted which does not comply with these terms.
*Correspondence: Jian Qin, qinjian@gxmu.edu.cn; Zhiyong Zhang, rpazz@163.com
†These authors have contributed equally to this work