- 1Department of Neurology, Second Affiliated Hospital, School of Medicine, Zhejiang University, Hangzhou, China
- 2Department of Anesthesiology, Women’s Hospital, School of Medicine, Zhejiang University, Hangzhou, China
Objective: Aquaporin-4 (AQP4) facilitates a sleep-enhanced interstitial brain waste clearance system. This study was conducted to determine the clinical implication of AQP4 polymorphisms in Parkinson’s disease (PD).
Methods: Three-hundred and eighty-two patients with PD and 180 healthy controls with a mean follow-up time of 66.1 months from the Parkinson’s Progression Marker Initiative study were analyzed. We examined whether AQP4 SNPs were associated with an altered rate of motor or cognitive decline using linear mixed model and Cox regression. We then investigated whether AQP4 SNPs were associated with Aβ burden as measured by 18F Florbetapir standard uptake values. Furthermore, we examined if AQP4 SNPs moderated the association between REM sleep behavior disorder (RBD) and CSF biomarkers.
Results: In patients with PD, AQP4 rs162009 (AA/AG vs. GG) was associated with slower dementia conversion, better performance in letter-number sequencing and symbol digit modalities, lower Aβ deposition in the putamen, anterior cingulum, and frontotemporal areas. In the subgroup of high RBD screening questionnaire score, rs162009 AA/AG had a higher CSF Aβ42 level. rs162009 AA/AG also had better performance in semantic fluency in healthy controls. Besides, rs68006382 (GG/GA vs. AA) was associated with faster progression to mild cognitive impairment, worse performance in letter-number sequencing, semantic fluency, and symbol digit modalities in patients with PD.
Interpretation: Genetic variations of AQP4 and subsequent alterations of glymphatic efficacy might contribute to an altered rate of cognitive decline in PD. AQP4 rs162009 is likely a novel genetic prognostic marker of glymphatic function and cognitive decline in PD.
Introduction
Aquaporin-4 (AQP4) is a water channel that lies at the astrocytic endfeet around perivascular space in the brain (Nagelhus and Ottersen, 2013). AQP4 plays a central role in the glymphatic system, which facilitates exchange between cerebrospinal fluid (CSF) and interstitial fluid, and drains macromolecules from the brain to the periphery (Mestre et al., 2018). Strikingly, the AQP4-facilitated glymphatic system clears interstitial brain waste, including amyloid β (Aβ; Iliff et al., 2012), tau (Harrison et al., 2020), and α-synuclein (Zou et al., 2019) according to animal studies. The association between AQP4 and interstitial waste clearance was further validated by a postmortem study of aging human brains, which revealed a link between reduced perivascular localization of AQP4 and Aβ deposition (Zeppenfeld et al., 2017). In addition, Single Nucleotide Polymorphisms (SNPs) of the AQP4 gene were associated with brain Aβ uptake on PET and the rate of cognitive decline in the spectrum of Alzheimer’s disease (AD; Burfeind et al., 2017; Chandra et al., 2020).
Parkinson’s disease (PD) is pathologically characterized by intraneuronal α-synuclein accumulation (Jakes et al., 1994), although extracellular α-synuclein is present and might contribute to the seeding of the disease (Lee et al., 2014). In addition, AD pathologies, including extracellular amyloid and tau aggregates, are also found in PD (Robinson et al., 2018). Amyloid deposition as measured by PET was positive in 34% of patients with PD dementia and 5% of patients with PD-mild cognitive impairment (MCI; Petrou et al., 2015). Furthermore, even subthreshold amyloid might contribute to cognitive decline in PD. In patients with PD, lower baseline CSF Aβ42 is associated with a faster rate of cognitive decline (Compta et al., 2013; Alves et al., 2014; Bäckström et al., 2015), worse performance in executive function (Compta et al., 2009; Stav et al., 2015), and delayed memory recall (Hall et al., 2015). Therefore, it is likely that clearing efficacy of synuclein and AD co-pathology are associated with an altered rate of motor or cognitive progression in PD.
Glymphatic fluid transport is known to be sleep-enhanced (Xie et al., 2013) and regulated by circadian rhythms (Hablitz et al., 2020). Disrupted sleep is a potential mechanism accounting for glymphatic dysfunction in PD. REM sleep behavior disorder (RBD) is one of the most characteristic sleep disorders in PD (Xie et al., 2019). Concomitant RBD in patients with PD is associated with faster motor progression, and worse cognitive function (Pagano et al., 2018; Zhang et al., 2020). These patients exhibit elevated synuclein deposition across the brain according to a postmortem study (Postuma et al., 2015). Interestingly, they are also inclined to have lower CSF Aβ42 and higher total tau/Aβ42 levels (Pagano et al., 2018). The elevated synuclein and amyloid burden are potentially attributable to the reduced efficacy of the AQP4-facilitated glymphatic system as a result of sleep disturbance (Bohnen and Hu, 2019).
In this study, using longitudinal data from the Parkinson’s Progression Marker Initiative (PPMI), we tested the hypothesis that AQP4 SNPs were associated with altered CSF or PET biomarker values and rate of motor or cognitive decline in PD. We also studied if AQP4 SNPs modulate the association between RBD and CSF biomarkers.
Materials and Methods
Study Participants
Major inclusion criteria for the PD cohort in the PPMI were as follows: (1) drug naïve; (2) diagnosed with PD within the past 2 years; (3) Hoehn and Yahr stage 1 or 2 at baseline; (4) age 30 years or older; and (5) striatal dopaminergic dysfunction on SPECT.
To exclude the potential confounding effect of race and ethnicity, non-Hispanic Caucasian patients and healthy controls (HC) were included in our analysis. Causes of exclusion include: (1) no CSF biomarker study result; and (2) no whole-genome sequencing data. Overall, 382 patients and 180 HCs were included, their demographic information and clinical characteristics are presented in Table 1.
Standard Protocol Approvals, Registrations, and Patient Consents
The study was approved by the institutional review board at each PPMI site. All patients signed an informed consent form before their participation in the PPMI study.
Clinical Assessment
Cognitive function was evaluated on a yearly basis. Cognitive tests included the Montreal Cognitive Assessment for global cognition, the Hopkins Verbal Learning Test (HVLT) for memory, the Benton Judgment of Line Orientation Test (BJLOT) for visuospatial perception, the Semantic Fluency Test (SFT) for executive function, the Letter-Number Sequencing (LNS) and the Symbol Digit Modality Test (SDMT) for working memory and attention-processing speed. Cognitive categorization of normal, MCI, and dementia was performed by investigators at each site in accordance with MDS criteria (Emre et al., 2007; Litvan et al., 2012).
Motor symptoms were evaluated at baseline, 3, 6, 9, 12, 18, 24, 30, 36, 42, 48, 54, 60, 72, 84, 96, and 108 months. We analyzed Hoehn and Yahr stage that was rated during the off condition (levodopa/dopaminergic agonist withheld for at least 6 h prior to the visit).
RBD was evaluated with an RBD screening questionnaire (RBDSQ). The optimal cutoff value for probable RBD in PD is 6 (Stiasny-Kolster et al., 2007; Nomura et al., 2011). RBD symptoms are time-varying. To study if AQP4 modulates the association between RBD and CSF biomarker, we stratified patients into subgroups by their averaged RBDSQ score in the first 3 years, as CSF samples were collected from baseline to the 3rd year. Overall, there are four expected visits for RBDSQ evaluation during this time frame (baseline, 1st, 2nd, and 3rd-year). Patients who were absent for two or more of these scheduled visits were excluded due to insufficient available data to characterize RBD symptoms within this time frame (N = 40). Then we stratified patients into a high RBDSQ group if averaged 3-year RBDSQ lies in the top one-third (RBDSQ ≥ 5.5, N = 117), and a low RBDSQ group if averaged 3-year RBDSQ lies in the least one-third (RBDSQ ≤ 3, N = 113).
Genotyping and SNP Pruning
Whole-genome sequencing data were downloaded from the PPMI database. SNP pruning was undertaken using PLINK (Purcell et al., 2007). Genetic variants of AQP4 underwent quality control procedures. Specifically, SNPs that were not in Hardy-Weinberg equilibrium (p < 0.05), had a minor allele frequency of <5% were removed. Linkage disequilibrium-based SNP pruning was performed to reduce statistical redundancy and maintain coverage of the AQP4 gene. SNP pruning parameters were as follows: window size 10, increment 5, and variance inflation factor 2. Two SNP pairs left after pruning were in high linkage disequilibrium (rs1058427 and rs12968026: r2 = 0.96; rs335930 and rs455671: r2 = 0.86). Therefore, rs12968026 and rs455671 were removed from further statistical analysis, leaving 11 SNPs. The information of these SNPs was displayed in Table 2, Figure 1, and Supplementary Figure 1.
A dominant model was used for all AQP4 SNPs.
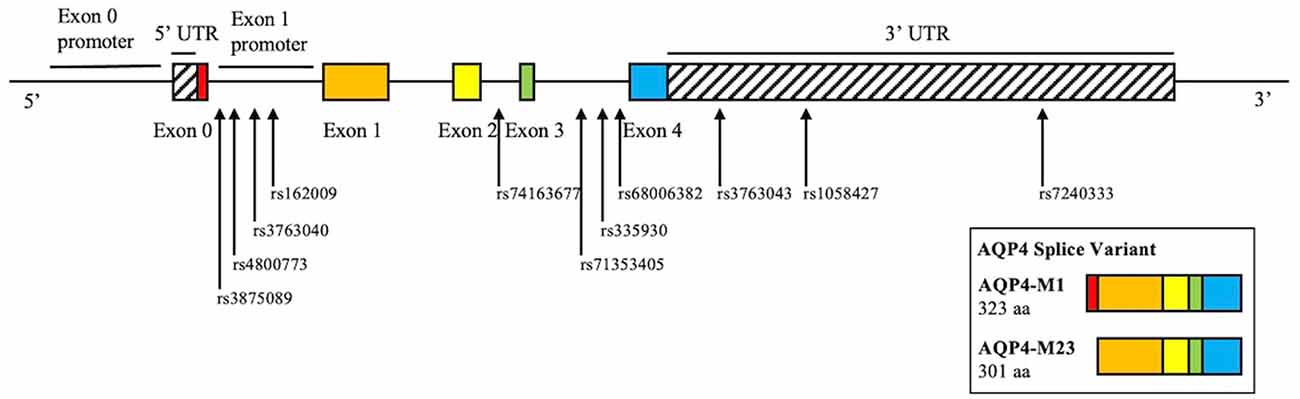
Figure 1. Schematic illustration of the investigated SNPs in the AQP4 gene. There are two isoforms of AQP4, M1, and M23. M1 consists of exon 0–4, M23 consists of exon 1–4. rs162009 locates in between exon 0 and 1, which presumably acts as the promoter of the M23 isoform.
Aβ Retention by PET
Overall, 36 patients who met the inclusion criteria of the current study underwent a Florbetaben (FBB) PET imaging for in vivo imaging of Aβ plaques. We analyzed standard uptake values (SUVs) downloaded from the PPMI dataset. SUV ratios (SUVRs) were calculated using the cerebellar cortex as reference.
Measurement of CSF Biomarkers
CSF was collected at baseline, 6, 12, 24, and 36 months. Levels of CSF Aβ42, total tau (t-tau), and phosphorylated tau (p-tau) at threonine 181 position were measured using Elecsys electrochemiluminescence immunoassays on the cobas e 601 analysis platform. CSF α-synuclein concentrations were analyzed using commercially available enzyme-linked immunosorbent assay kits. These values were downloaded from the PPMI database.
Statistical Analysis
Clinical and biomarker data were downloaded from the PPMI database in December 2020. Statistical analysis was performed using SPSS (IBM Corp, Armonk NY, USA). Graphs were plotted using GraphPad (San Diego, CA, USA).
For longitudinal cognitive test score analysis, mixed models with random slope and intercept and unstructured covariance were used. Fixed effects include age at baseline, sex, baseline MoCA score, years of education, Apolipoprotein-E (APOE) ε4 carriage, and AQP4 SNP of interest. The AQP4 SNP by time interaction effect was also included as a fixed effect in separate models. Time was treated as a continuous variable. The patient number was listed as a random effect.
Cox proportional hazards regressions were performed to study if AQP4 polymorphisms were associated with the rate of motor and cognitive deterioration. We defined events separately as: (1) progression to MCI for the first time; (2) progression to dementia for the first time; and (3) progression of Hoehn and Yahr stage for the first time. Age, sex, APOE ε4 carriage, years of education, and baseline MoCA score were covaried for conversion to MCI or dementia. Age, sex, baseline MDS-UPDRS-III, and levodopa equivalent dose were covaried for motor progression. Kaplan-Meier curves were plotted, and log-rank test results were presented.
APOE ε4 (Mata et al., 2014), glucocerebrosidase (GBA) variants (Alcalay et al., 2012), and Catechol-O-methyltransferase (COMT) Val158Met (rs4680; Egan et al., 2001; Williams-Gray et al., 2009) are previously established genetic markers of cognition in PD. COMT rs4680 data was extracted from whole-genome sequencing data. GBA variant summary from across different sequencing platforms was directly downloaded. Their distribution on AQP4 SNPs was tested using the Chi-Square test.
The association between AQP4 SNPs and SUVRs was examined using linear regression. Covariates included age, sex, APOE ε4 carriage, and disease duration at the time of PET scan.
CSF biomarkers (α-synuclein, Aβ, t-tau, p-tau) did not follow a normal distribution and were, therefore, log10 transformed in the statistical analysis. To determine whether CSF biomarkers differed with respect to AQP4 polymorphisms across time, linear mixed models with random intercept were used. Fixed effects included age at baseline, sex, time, and AQP4 SNP of interest. For CSF Aβ42, APOE ε4 carriage was also covaried. To determine whether AQP4 SNPs moderate the association between RBD and CSF biomarkers, the RBDSQ subgroup by AQP4 SNP interaction effect was tested.
To correct for multiple comparisons, the Benjamini-Hochberg procedure with a false discovery rate at 0.10 was used given the exploratory nature of this study. p < 0.05 was used as the threshold for statistical significance.
Result
AQP4 SNPs and Conversion to MCI and Dementia
We did not observe a significant association between AQP4 SNPs and motor progression as reflected by MDS-UPDRS-III score increment in mixed model and Hoehn and Yahr stage progression in Cox regression.
However, after adjusting for age, sex, APOE ε4 carriage, and baseline MoCA score, minor allele carrier status of rs68006382 was associated with accelerated conversion to MCI (HR = 1.973, 95% CI = 1.246–3.123, p = 0.004; Figure 2A), but not dementia (HR = 1.512, 95% CI = 0.753–3.034, p = 0.245). In addition, minor allele carrier status of rs162009 was associated with slower conversion to MCI (HR = 0.646, 95% CI = 0.409–1.019, p = 0.060), and dementia (HR = 0.473, 95% CI = 0.234–0.956, p = 0.037), although these effects were marginal (Figures 3A,B).
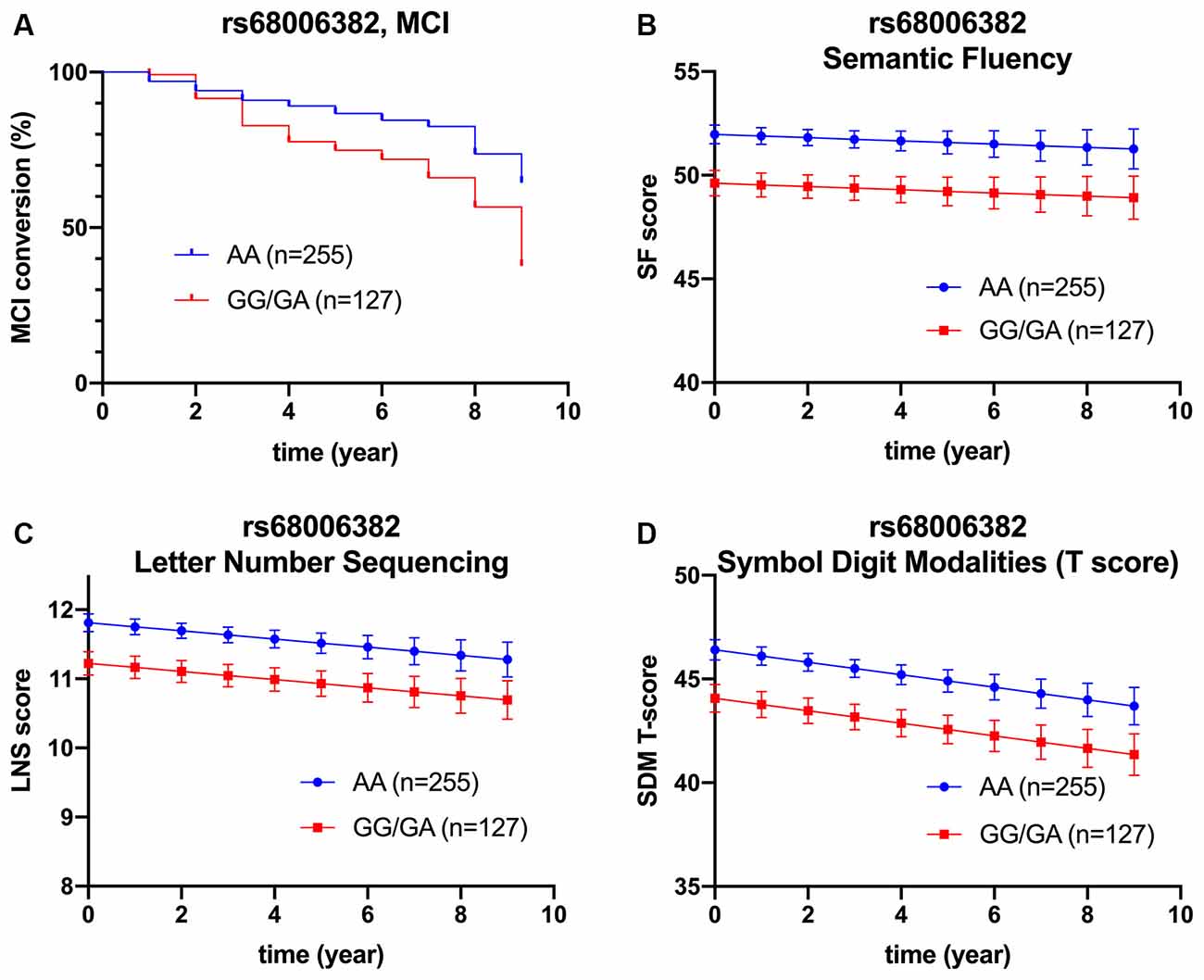
Figure 2. Association between rs68006382 and cognition. rs68006382 (GG/GA vs. AA) had faster conversion to MCI (HR = 1.973, 95% CI = 1.246–3.123, p = 0.004) (A). rs68006382 GG/GA genotype also had worse performance in semantic fluency test (β = −2.357, SE = 0.691, p = 0.001) (B), letter-number sequencing (β = −0.587, SE = 0.193, p = 0.003) (C), and symbol digit modalities test (β = −2.339, SE = 0.193, p = 0.002) (D). Covariates for cox regression and linear mixed model included age, sex, APOE ε4 carriage, years of education, and baseline MoCA score. Lines in Panels (B–D) represent the estimated marginal mean from the mixed model. MCI, mild cognitive impairment.
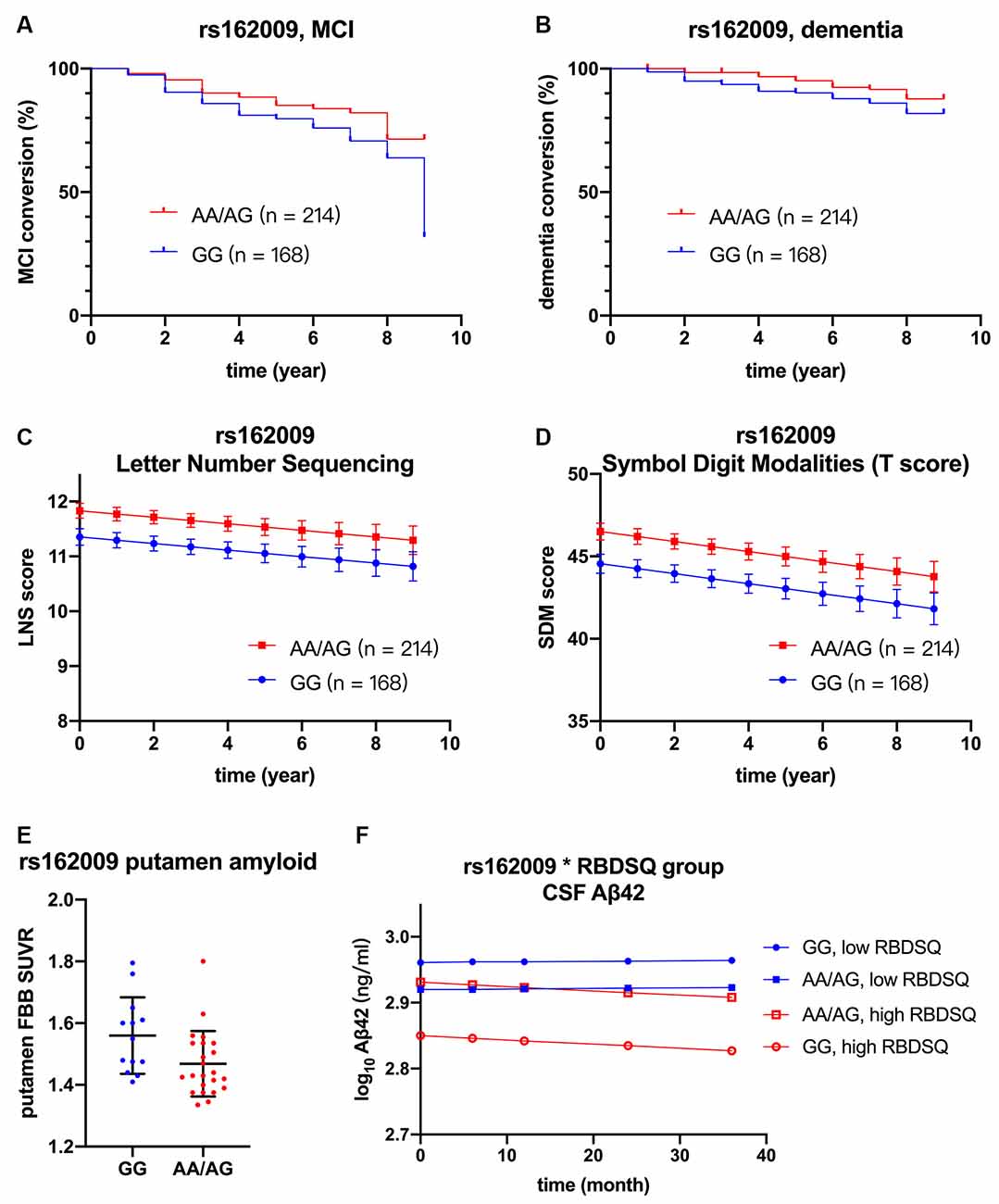
Figure 3. Association between rs162009, cognition, and biomarkers. rs162009 (AA/AG vs. GG) had slower conversion to MCI (HR = 0.646, 95% CI = 0.409–1.019, p = 0.060) (A) and dementia (HR = 0.473, 95% CI = 0.234–0.957, p = 0.037) (B), better performance in letter-number sequencing (β = 0.477, SE = 0.183, p = 0.009) (C), and symbol digit modalities test (β = 2.401, SE = 1.953, p = 0.006) (D). rs162009 AA/AG genotype also had lower putamen FBB SUVRs (β = −0.105, SE = 0.039, p = 0.012) (E). In the subgroup of patients with high RBDSQ score (averaged score during the first 3 year ≥ 5.5, N = 117), patients with rs162009 AA/AG genotype had higher CSF Aβ42 levels (β = 0.081, SE = 0.033, p = 0.017), indicating its protective effect on Aβ deposition (F). Covariates for cox regression and linear mixed model included age, sex, APOE ε4 carriage, years of education, and baseline MoCA score. Lines in Panels (C,D,F) represent the estimated marginal mean from the mixed model. FBB, 18F Florbetaben; MCI, mild cognitive impairment; RBDSQ, Rapid Eye Movement Sleep Behavior Disorder Screening Questionnaire; SUVRs, standard uptake value ratios.
AQP4 SNPs and Performance in Cognitive Subdomains
The association between AQP4 SNPs and cognitive subdomains is presented in Table 3.
Briefly, minor allele carrier status of rs68006382 was associated with worse performance in executive function, working memory, and attention (SFT: p = 0.001; LNS: p = 0.003; SDMT: p = 0.002; Figures 2B–D).
Minor allele carrier status of rs162009, on the other hand, was protective, with better performance in working memory and attention (LNS: p = 0.009; SDMT: p = 0.006; Figures 3C,D).
In addition, minor allele carrier status of rs3763043 and rs3763040 were associated with worse executive function (SFT: p = 0.003) and better visuospatial function (BJLOT: p = 0.007), respectively. Minor allele carrier of rs7240333 was associated with better memory (HVLT: p = 0.047), which was not significant after correction for multiple comparisons.
There was no significant SNP by time interaction effect in any of the tests. Notably, there was no significant main effect of time in HVLT (p = 0.457), LNS (p = 0.068), and SFT (p = 0.518), indicating the absence of decreasing scores in these subdomains over time. This was likely attributable to the high education level in the PPMI cohort, which amounts to an average of 15.56 years of education. Patients with a higher level of education are likely to have higher cognitive reserve and therefore slower cognitive decline in PD (Meng and D’Arcy, 2012; Hindle et al., 2014).
Association Between AQP4 and APOE ε4, COMT Val158Met, GBA Variants
Chi-Square test indicated that the distribution of COMT variants on rs3763043 minor allele carriers and non-carriers was statistically different (p = 0.027), indicating a potential confounding effect of COMT variants on the observed association between rs3763043 and SFT. The distribution of APOE ε4, COMT Val158Met, GBA variants on other AQP SNPs minor allele carrier vs. non-carrier was not significant (Supplementary Table 1).
AQP4 SNPs and 18F Florbetapir SUVR
Of the 36 patients who underwent 18F Florbetapir imaging, six (16.7%) were amyloid positive, if a cut-off of 1.43 for composite SUVR was used (Bullich et al., 2017; Fiorenzato et al., 2018). The average disease duration at the time of PET imaging was 4.73 ± 1.71 years. There was no statistically significant association between SNPs and composite SUVRs, although rs162009 was marginally associated lower composite SUVRs (β = −0.076, SE = 0.044, p = 0.094).
Given the observed association between minor allele carriers of rs162009 and more preserved cognitive performance, we explored its association with SUVRs in cortical and subcortical regions of interest (Table 4). There were decreased SUVRs in putamen (p = 0.012) and anterior cingulum (p = 0.048) even with a limited sample size (n = 36; Figure 3E). In addition, minor allele carriers of rs162009 were marginally associated with lower amyloid burden in the temporal (p = 0.070) and frontal (p = 0.072) cortex.
The Interaction Between AQP4 SNPs, Sleep Disturbance, and CSF Biomarkers
There was no significant association between AQP4 SNPs and CSF biomarkers in the whole cohort. Then we examined the association between RBD and CSF biomarkers. Our analysis concords with a previous study that RBD is associated with lower CSF Aβ42 using the same PPMI database (Pagano et al., 2018). There was no such association between RBD and α-synuclein or tau. Given the proposed role of AQP4 in solute drainage during sleep, we examined if AQP4 SNPs modulate this association. After controlling for APOE ε4 carriage, age, sex and time, minor allele of rs162009 was associated with higher CSF Aβ42 level in the high RBDSQ subgroup (β = 0.081, SE = 0.033, p = 0.017), but not in the low RBDSQ subgroup (subgroup by SNP interaction effect: β = −0.056, SE = 0.023, p = 0.014; Figure 3F).
AQP4 SNPs and Cognitive Performance in Healthy Controls
The association between AQP4 SNP and cognitive scales was demonstrated in Table 5. Notably, minor allele carrier status of rs162009 was associated with higher semantic fluency test performance (β = 2.209, SE = 0.913, p = 0.016), validating the association between rs162009 and cognitive performance observed in patients with PD.
In addition, multiple AQP4 SNPs (rs3763043, rs68006382, and rs74163677) were associated with HVLT performance. However, these associations were likely attributable to the fact that AQP4 plays a role in synaptic plasticity and therefore learning and memory (Hubbard et al., 2018; Woo et al., 2018).
During follow-up, 6/180 (3.33%) and 1/180 (0.56%) HCs developed MCI and dementia, respectively. Therefore, we did not perform Cox regressions in HCs.
Besides, there was no significant distribution difference of AQP4 SNPs in PD and HCs.
Discussion
Given the previously established association between AQP4 and cognitive performance in the spectrum of AD, we explored this association in a cohort of patients with PD. We found that rs162009 was associated with slower dementia conversion, better performance in LNS and SDMT, and lower amyloid burden in the putamen and anterior cingulum. This variant was also associated with higher CSF Aβ in the subgroup with a high RBDSQ score. Besides, rs68006382 was associated with faster MCI conversion and worse performance in LNS, SFT, and SDMT.
The implication of rs162009 AA/AG genotype was more compelling as this variant was associated with both better cognitive performance and lower amyloid deposition in multiple regions. In addition, its protective effect on cognition was also seen in HCs. One of the potential mechanisms to back up these clinical findings is that rs162009 likely increases the transcription of AQP4-M23 isoform and improves the glymphatic fluid circulation. There are two AQP4 splice variants, the M1 and M23. The M1 variant tends to be evenly distributed across the astrocytic membrane, while the M23 variant primarily locates at the astrocytic endfeet (Smith et al., 2014). rs162009 locates between exon 0 and exon 1 of the AQP4 gene (Figure 1), and putatively acts as a promoter for the AQP4-M23 splice variant, which potentially facilitates the the glymphatic fluid circulation (Iliff et al., 2012). However, this was primarily speculative, more mechanistic study is needed to explore the physiological effect of AQP4 SNPs.
The current findings add weight to previous observations that even subthreshold amyloid contributes to cognitive decline in non-demented PD. Interestingly, we observed a link between rs162009 and decreased putamen amyloid deposition. Increased striatal amyloid deposition was a prominent feature in PD-dementia autopsy (Kalaitzakis et al., 2008; Hepp et al., 2016). PD patients with combined amyloid pathology in cortex and striatum were associated with worse cognition than those with cortical amyloid pathology alone (Shah et al., 2016). In line with AD, it has been proposed that amyloid deposits in the neocortex first and striatum later (Shah et al., 2016). Therefore, the association between rs162009 and putamen SUVR might correspond to the slowed dementia conversion. The lower amyloid burden in the anterior cingulum (Petersen and Posner, 2012) and frontotemporal area (Randolph et al., 1993; Forn et al., 2009) in rs162009 minor allele carriers might correspond to better attention and working memory performance in these patients. However, AQP4 might modulate cognition in PD through other mechanisms such as synaptic plasticity (Hubbard et al., 2018; Woo et al., 2018), and neuroimmunological regulation (Ikeshima-Kataoka, 2016; Tamtaji et al., 2019).
The association between rs162009 and CSF Aβ42 in the subgroup of high RBDSQ score provides a novel insight into the interaction between RBD and AQP4-facilitated glymphatic clearance. Although glymphatic efficacy peaks during slow-wave sleep (Hablitz et al., 2019), changes in the EEG signature of non-REM sleep have been observed in patients with RBD (Sunwoo et al., 2020). Therefore, it is reasonable to assume that RBD reduces glymphatic capacity. In patients with high RBDSQ scores, disrupted sleep architecture likely reduced the clearing capacity of the glymphatic system, which is attributable to the AQP4 polymorphisms. The absence of significant association in the low RBDSQ subgroup likely reflected a situation where the glymphatic capacity exceeds the clearing need due to intact sleep. This finding provides indirect evidence that amyloid deposition as a result of disrupted glymphatic clearance might contribute to cognitive decline in RBD. This finding also highlights the prospects of targeting amyloid with immunotherapy in a subgroup of patients with PD (for instance, RBD and non-carriers of rs162009 minor allele).
We did not observe a significant association between AQP4 and motor progression or CSF α-synuclein. This was likely because α-synuclein was primarily intracellular, the need for interstitial clearance might be low when compared with extracellular Aβ. Although significant α-synuclein aggregation was reported in Aqp4 knockout mice (Xue et al., 2019), single nucleotide polymorphisms in humans likely exert a much smaller effect than genetic knockout.
The major limitations of the current study are as follows: (1) Significant associations observed in the current study are likely to be false positive. More work in other cohorts is needed to verify the effect of AQP4 SNPs on PD. (2) We observed a marginally significant effect of rs162009 on FBB SUVRs in a limited number of patients (n = 36). Future studies to validate the finding by expanding the patient sample size would be necessary. Besides, such a small number of patients who underwent FBB-PET would limit the statistical power to detect AQP4 variants that are potentially associated with FBB SUV, especially for those SNPs that have low minor allele frequency. (3) Caution must be taken when interpreting the interaction effect between rs162009 and RBDSQ subgroup. It is also likely that abnormal neuronal activation as a result of sleep disturbance causes elevated production and release of Aβ (Ovsepian and O’Leary, 2016; Lucey, 2020). As Aβ measured by CSF or PET represents the balance of production and clearance, future studies to measure glymphatic activity in vivo would be necessary. (4) Measuring RBD symptoms with RBDSQ is prone to subjectivity (Halsband et al., 2018). More studies are warranted to validate and explore the effect of various types of sleep disturbance with objective sleep assessment equipment such as polysomnography and actigraphy.
Conclusions
Genetic variations of AQP4 likely alter the glymphatic clearance of Aβ in the brain and subsequently the rate of cognitive decline in PD. AQP4 rs162009 is likely a novel prognostic marker of cognitive decline in PD. Our findings also provide indirect evidence that in PD, AQP4-facilitated clearance of interstitial amyloid might be disrupted in patients with probable REM sleep behavior disorder.
Data Availability Statement
Publicly available datasets were analyzed in this study. This data can be found here: https://www.ppmi-info.org/access-data-specimens/download-data.
Ethics Statement
Ethical approval was not provided for this study on human participants because this study obtained and analyzed data from the PPMI dataset. Ethical approval was obtained at each individual PPMI participating site. The patients/participants provided their written informed consent to participate in the PPMI study.
Author Contributions
YF, JP, and BZ contributed to the conception and design of the study. YF, SD, and CJ performed the statistical analysis. YF and SD wrote the first draft of the manuscript. All authors contributed to the article and approved the submitted version.
Funding
This work was supported by the National Natural Science Foundation of China’s Major Regional International Cooperation Project (No. 81520108010), the National Natural Science Foundation of China (No. 81771216), the Key Research and Development Program of Zhejiang Province (No. 2020C03020), and the Natural Science Foundation of Zhejiang Province (No. LY18H090003).
Conflict of Interest
The authors declare that the research was conducted in the absence of any commercial or financial relationships that could be construed as a potential conflict of interest.
Publisher’s Note
All claims expressed in this article are solely those of the authors and do not necessarily represent those of their affiliated organizations, or those of the publisher, the editors and the reviewers. Any product that may be evaluated in this article, or claim that may be made by its manufacturer, is not guaranteed or endorsed by the publisher.
Acknowledgments
For up-to-date information on the study, visit www.ppmi-info.org. PPMI—a public-private partnership—is funded by the Michael J. Fox Foundation for Parkinson’s Research funding partners Abbvie, Allergan, Amathus therapeutics, Avid Radiopharmaceuticals, Biogen, BioLegend, Bristol-Myers Squibb, Celgene, Denali, GE Healthcare, Genentech, GlaxoSmithKline, Handl Therapeutics, Insitro, Janssen Neuroscience, Lilly, Lundbeck, Merck, Meso Scale Discovery, Pfizer, Piramal, Prevail, Roche, Sanofi Genzyme, Servier, Takeda, Teva, UCB, Verily, Voyager Therapeutics, Golub Capital. The full list of funding partners is found at www.ppmi-info.org/about-ppmi/who-we-are/study-sponsors.
Supplementary Materials
The Supplementary Material for this article can be found online at: https://www.frontiersin.org/articles/10.3389/fnagi.2021.740491/full#supplementary-material.
References
Alcalay, R. N., Caccappolo, E., Mejia-Santana, H., Tang, M.-X., Rosado, L., Orbe Reilly, M., et al. (2012). Cognitive performance of GBA mutation carriers with early-onset PD. Neurology 78, 1434–1440. doi: 10.1212/WNL.0b013e318253d54b
Alves, G., Lange, J., Blennow, K., Zetterberg, H., Andreasson, U., Forland, M. G., et al. (2014). CSF Aβ42 predicts early-onset dementia in Parkinson disease. Neurology 82, 1784–1790. doi: 10.1212/WNL.0000000000000425
Appelboom, G., Bruce, S., Duren, A., Piazza, M., Monahan, A., Christophe, B., et al. (2015). Aquaporin-4 gene variant independently associated with oedema after intracerebral haemorrhage. Neurol. Res. 37, 657–661. doi: 10.1179/1743132815Y.0000000047
Bäckström, D. C., Eriksson Domellöf, M., Linder, J., Olsson, B., Öhrfelt, A., Trupp, M., et al. (2015). Cerebrospinal fluid patterns and the risk of future dementia in early, incident Parkinson disease. JAMA Neurol. 72, 1175–1182. doi: 10.1001/jamaneurol.2015.1449
Bohnen, N. I., and Hu, M. T. M. (2019). Sleep disturbance as potential risk and progression factor for Parkinson’s disease. J. Parkinsons Dis. 9, 603–614. doi: 10.3233/JPD-191627
Bullich, S., Seibyl, J., Catafau, A. M., Jovalekic, A., Koglin, N., Barthel, H., et al. (2017). Optimized classification of 18F-Florbetaben PET scans as positive and negative using an SUVR quantitative approach and comparison to visual assessment. Neuroimage Clin. 15, 325–332. doi: 10.1016/j.nicl.2017.04.025
Burfeind, K. G., Murchison, C. F., Westaway, S. K., Simon, M. J., Erten-Lyons, D., Kaye, J. A., et al. (2017). The effects of noncoding aquaporin-4 single-nucleotide polymorphisms on cognition and functional progression of Alzheimer’s disease. Alzheimers Dement. (NY) 3, 348–359. doi: 10.1016/j.trci.2017.05.001
Chandra, A., Farrell, C., Wilson, H., Dervenoulas, G., De Natale, E. R., and Politis, M. (2020). Aquaporin-4 polymorphisms predict amyloid burden and clinical outcome in the Alzheimer’s disease spectrum. Neurobiol. Aging 97, 1–9. doi: 10.1016/j.neurobiolaging.2020.06.007
Compta, Y., Martí, M. J., Ibarretxe-Bilbao, N., Junqué, C., Valldeoriola, F., Muñoz, E., et al. (2009). Cerebrospinal tau, phospho-tau and beta-amyloid and neuropsychological functions in Parkinson’s disease: CSF and neuropsychological markers in PD. Mov. Disord. 24, 2203–2210. doi: 10.1002/mds.22594
Compta, Y., Pereira, J. B., Ríos, J., Ibarretxe-Bilbao, N., Junqué, C., Bargalló, N., et al. (2013). Combined dementia-risk biomarkers in Parkinson’s disease: a prospective longitudinal study. Parkinsonism Relat. Disord. 19, 717–724. doi: 10.1016/j.parkreldis.2013.03.009
Dardiotis, E., Siokas, V., Marogianni, C., Aloizou, A.-M., Sokratous, M., Paterakis, K., et al. (2019). AQP4 tag SNPs in patients with intracerebral hemorrhage in greek and polish population. Neurosci. Lett. 696, 156–161. doi: 10.1016/j.neulet.2018.12.025
Egan, M. F., Goldberg, T. E., Kolachana, B. S., Callicott, J. H., Mazzanti, C. M., Straub, R. E., et al. (2001). Effect of COMT Val108/158 Met genotype on frontal lobe function and risk for schizophrenia. Proc. Natl. Acad. Sci. U S A 98, 6917–6922. doi: 10.1073/pnas.111134598
Emre, M., Aarsland, D., Brown, R., Burn, D. J., Duyckaerts, C., Mizuno, Y., et al. (2007). Clinical diagnostic criteria for dementia associated with Parkinson’s disease. Mov. Disord. 22, 1689–1707. doi: 10.1002/mds.21507
Fiorenzato, E., Biundo, R., Cecchin, D., Frigo, A. C., Kim, J., Weis, L., et al. (2018). Brain amyloid contribution to cognitive dysfunction in early-stage Parkinson’s disease: the PPMI dataset. J. Alzheimers Dis. 66, 229–237. doi: 10.3233/JAD-180390
Forn, C., Belloch, V., Bustamante, J. C., Garbin, G., Parcet-Ibars, M. à., Sanjuan, A., et al. (2009). A symbol digit modalities test version suitable for functional MRI studies. Neurosci. Lett. 456, 11–14. doi: 10.1016/j.neulet.2009.03.081
Hablitz, L. M., Plá, V., Giannetto, M., Vinitsky, H. S., Stæger, F. F., Metcalfe, T., et al. (2020). Circadian control of brain glymphatic and lymphatic fluid flow. Nat. Commun. 11:4411. doi: 10.1038/s41467-020-18115-2
Hablitz, L. M., Vinitsky, H. S., Sun, Q., Stæger, F. F., Sigurdsson, B., Mortensen, K. N., et al. (2019). Increased glymphatic influx is correlated with high EEG delta power and low heart rate in mice under anesthesia. Sci. Adv. 5:eaav5447. doi: 10.1126/sciadv.aav5447
Hall, S., Surova, Y., Öhrfelt, A., Zetterberg, H., Lindqvist, D., and Hansson, O. (2015). CSF biomarkers and clinical progression of Parkinson disease. Neurology 84, 57–63. doi: 10.1212/WNL.0000000000001098
Halsband, C., Zapf, A., Sixel-Döring, F., Trenkwalder, C., and Mollenhauer, B. (2018). The REM sleep behavior disorder screening questionnaire is not valid in de novo Parkinson’s disease. Mov. Disord. Clin. Pract. 5, 171–176. doi: 10.1002/mdc3.12591
Harrison, I. F., Ismail, O., Machhada, A., Colgan, N., Ohene, Y., Nahavandi, P., et al. (2020). Impaired glymphatic function and clearance of tau in an Alzheimer’s disease model. Brain 143, 2576–2593. doi: 10.1093/brain/awaa179
Hepp, D. H., Vergoossen, D. L. E., Huisman, E., Lemstra, A. W., Bank, N. B., Berendse, H. W., et al. (2016). Distribution and load of amyloid-β pathology in Parkinson disease and dementia with lewy bodies. J. Neuropathol. Exp. Neurol. 75, 936–945. doi: 10.1093/jnen/nlw070
Hindle, J. V., Martyr, A., and Clare, L. (2014). Cognitive reserve in Parkinson’s disease: a systematic review and meta-analysis. Parkinsonism Relat. Disord. 20, 1–7. doi: 10.1016/j.parkreldis.2013.08.010
Hubbard, J. A., Szu, J. I., and Binder, D. K. (2018). The role of aquaporin-4 in synaptic plasticity, memory and disease. Brain Res. Bull. 136, 118–129. doi: 10.1016/j.brainresbull.2017.02.011
Ikeshima-Kataoka, H. (2016). Neuroimmunological implications of AQP4 in astrocytes. Int. J. Mol. Sci. 17:1306. doi: 10.3390/ijms17081306
Iliff, J. J., Wang, M., Liao, Y., Plogg, B. A., Peng, W., Gundersen, G. A., et al. (2012). A paravascular pathway facilitates CSF flow through the brain parenchyma and the clearance of interstitial solutes, including amyloid. Sci. Transl. Med. 4:147ra111. doi: 10.1126/scitranslmed.3003748
Jakes, R., Spillantini, M. G., and Goedert, M. (1994). Identification of two distinct synucleins from human brain. FEBS Lett. 345, 27–32. doi: 10.1016/0014-5793(94)00395-5
Kalaitzakis, M. E., Graeber, M. B., Gentleman, S. M., and Pearce, R. K. B. (2008). Striatal β-amyloid deposition in Parkinson disease with dementia. J. Neuropathol. Exp. Neurol. 67, 155–161. doi: 10.1097/NEN.0b013e31816362aa
Lee, H.-J., Bae, E.-J., and Lee, S.-J. (2014). Extracellular α-synuclein—a novel and crucial factor in Lewy body diseases. Nat. Rev. Neurol. 10, 92–98. doi: 10.1038/nrneurol.2013.275
Litvan, I., Goldman, J. G., Tröster, A. I., Schmand, B. A., Weintraub, D., Petersen, R. C., et al. (2012). Diagnostic criteria for mild cognitive impairment in Parkinson’s disease: movement disorder society task force guidelines: PD-MCI diagnostic criteria. Mov. Disord. 27, 349–356. doi: 10.1002/mds.24893
Lucey, B. P. (2020). It’s complicated: the relationship between sleep and Alzheimer’s disease in humans. Neurobiol. Dis. 144:105031. doi: 10.1016/j.nbd.2020.105031
Mata, I. F., Leverenz, J. B., Weintraub, D., Trojanowski, J. Q., Hurtig, H. I., Van Deerlin, V. M., et al. (2014). APOE, MAPT and SNCA genes and cognitive performance in Parkinson disease. JAMA Neurol. 71:1405. doi: 10.1001/jamaneurol.2014.1455
Meng, X., and D’Arcy, C. (2012). Education and dementia in the context of the cognitive reserve hypothesis: a systematic review with meta-analyses and qualitative analyses. PLoS One 7:e38268. doi: 10.1371/journal.pone.0038268
Mestre, H., Hablitz, L. M., Xavier, A. L., Feng, W., Zou, W., Pu, T., et al. (2018). Aquaporin-4-dependent glymphatic solute transport in the rodent brain. eLife 7:e40070. doi: 10.7554/eLife.40070
Nagelhus, E. A., and Ottersen, O. P. (2013). Physiological roles of aquaporin-4 in brain. Physiol. Rev. 93, 1543–1562. doi: 10.1152/physrev.00011.2013
Nomura, T., Inoue, Y., Kagimura, T., Uemura, Y., and Nakashima, K. (2011). Utility of the REM sleep behavior disorder screening questionnaire (RBDSQ) in Parkinson’s disease patients. Sleep Med. 12, 711–713. doi: 10.1016/j.sleep.2011.01.015
Ovsepian, S. V., and O’Leary, V. B. (2016). Neuronal activity and amyloid plaque pathology: an update. J. Alzheimers Dis. 49, 13–19. doi: 10.3233/JAD-150544
Pagano, G., De Micco, R., Yousaf, T., Wilson, H., Chandra, A., and Politis, M. (2018). REM behavior disorder predicts motor progression and cognitive decline in Parkinson disease. Neurology 91, e894–e905. doi: 10.1212/WNL.0000000000006134
Petersen, S. E., and Posner, M. I. (2012). The attention system of the human brain: 20 years after. Annu. Rev. Neurosci. 35, 73–89. doi: 10.1146/annurev-neuro-062111-150525
Petrou, M., Dwamena, B. A., Foerster, B. R., MacEachern, M. P., Bohnen, N. I., Müller, M. L., et al. (2015). Amyloid deposition in Parkinson’s disease and cognitive impairment: a systematic review: systematic review: amyloid in Parkinsonian dementia. Mov. Disord. 30, 928–935. doi: 10.1002/mds.26191
Postuma, R. B., Gagnon, J.-F., Bertrand, J.-A., Génier Marchand, D., and Montplaisir, J. Y. (2015). Parkinson risk in idiopathic REM sleep behavior disorder. Neurology 84, 1104–1113. doi: 10.1212/WNL.0000000000001364
Purcell, S., Neale, B., Todd-Brown, K., Thomas, L., Ferreira, M. A. R., Bender, D., et al. (2007). PLINK: a tool set for whole-genome association and population-based linkage analyses. Am. J. Hum. Genet. 81, 559–575. doi: 10.1086/519795
Randolph, C., Braun, A. R., Goldberg, T. E., and Chase, T. N. (1993). Semantic fluency in Alzheimer’s, Parkinson’s and Huntington’s disease: dissociation of storage and retrieval failures. Neuropsychology 7, 82–88. doi: 10.1037/0894-4105.7.1.82
Robinson, J. L., Lee, E. B., Xie, S. X., Rennert, L., Suh, E., Bredenberg, C., et al. (2018). Neurodegenerative disease concomitant proteinopathies are prevalent, age-related and APOE4-associated. Brain 141, 2181–2193. doi: 10.1093/brain/awy146
Shah, N., Frey, K. A., Müller, L. T. M., Petrou, M., Kotagal, V., Koeppe, R. A., et al. (2016). Striatal and cortical β-amyloidopathy and cognition in Parkinson’s disease: striatal β-amyloid and cognition in PD. Mov. Disord. 31, 111–117. doi: 10.1002/mds.26369
Smith, A. J., Jin, B.-J., Ratelade, J., and Verkman, A. S. (2014). Aggregation state determines the localization and function of M1- and M23-aquaporin-4 in astrocytes. J. Cell Biol. 204, 559–573. doi: 10.1083/jcb.201308118
Stav, A. L., Aarsland, D., Johansen, K. K., Hessen, E., Auning, E., and Fladby, T. (2015). Amyloid-β and α-synuclein cerebrospinal fluid biomarkers and cognition in early Parkinson’s disease. Parkinsonism Relat. Disord. 21, 758–764. doi: 10.1016/j.parkreldis.2015.04.027
Stiasny-Kolster, K., Mayer, G., Schäfer, S., Möller, J. C., Heinzel-Gutenbrunner, M., and Oertel, W. H. (2007). The REM sleep behavior disorder screening questionnaire—A new diagnostic instrument. Mov. Disord. 22, 2386–2393. doi: 10.1002/mds.21740
Sunwoo, J.-S., Cha, K. S., Byun, J.-I., Jun, J.-S., Kim, T.-J., Shin, J.-W., et al. (2020). Nonrapid eye movement sleep electroencephalographic oscillations in idiopathic rapid eye movement sleep behavior disorder: a study of sleep spindles and slow oscillations. Sleep 44:zsaa160. doi: 10.1093/sleep/zsaa160
Tamtaji, O. R., Behnam, M., Pourattar, M. A., Jafarpour, H., and Asemi, Z. (2019). Aquaporin 4: a key player in Parkinson’s disease. J. Cell. Physiol. 234, 21471–21478. doi: 10.1002/jcp.28871
Williams-Gray, C. H., Evans, J. R., Goris, A., Foltynie, T., Ban, M., Robbins, T. W., et al. (2009). The distinct cognitive syndromes of Parkinson’s disease: 5 year follow-up of the CamPaIGN cohort. Brain 132, 2958–2969. doi: 10.1093/brain/awp245
Woo, J., Kim, J. E., Im, J. J., Lee, J., Jeong, H. S., Park, S., et al. (2018). Astrocytic water channel aquaporin-4 modulates brain plasticity in both mice and humans: a potential gliogenetic mechanism underlying language-associated learning. Mol. Psychiatry 23, 1021–1030. doi: 10.1038/mp.2017.113
Wu, Y.-F., Sytwu, H.-K., and Lung, F.-W. (2020). Polymorphisms in the human aquaporin 4 gene are associated with schizophrenia in the southern chinese han population: a case-control study. Front. Psychiatry 11:596. doi: 10.3389/fpsyt.2020.00596
Xie, F., Gao, X., Yang, W., Chang, Z., Yang, X., Wei, X., et al. (2019). Advances in the research of risk factors and prodromal biomarkers of Parkinson’s disease. ACS Chem. Neurosci. 10, 973–990. doi: 10.1021/acschemneuro.8b00520
Xie, L., Kang, H., Xu, Q., Chen, M. J., Liao, Y., Thiyagarajan, M., et al. (2013). Sleep drives metabolite clearance from the adult brain. Science 342, 373–377. doi: 10.1126/science.1241224
Xue, X., Zhang, W., Zhu, J., Chen, X., Zhou, S., Xu, Z., et al. (2019). Aquaporin-4 deficiency reduces TGF-β1 in mouse midbrains and exacerbates pathology in experimental Parkinson’s disease. J. Cell. Mol. Med. 23, 2568–2582. doi: 10.1111/jcmm.14147
Zeppenfeld, D. M., Simon, M., Haswell, J. D., D’Abreo, D., Murchison, C., Quinn, J. F., et al. (2017). Association of perivascular localization of aquaporin-4 with cognition and Alzheimer disease in aging brains. JAMA Neurol. 74, 91–99. doi: 10.1001/jamaneurol.2016.4370
Zhang, F., Niu, L., Liu, X., Liu, Y., Li, S., Yu, H., et al. (2020). Rapid eye movement sleep behavior disorder and neurodegenerative diseases: an update. Aging Dis. 11, 315–326. doi: 10.14336/AD.2019.0324
Keywords: Parkinson’s disease, cognitive dysfunction, aquaporin-4, amyloid plaque, REM sleep behavior disorder
Citation: Fang Y, Dai S, Jin C, Si X, Gu L, Song Z, Gao T, Chen Y, Yan Y, Yin X, Pu J and Zhang B (2022) Aquaporin-4 Polymorphisms Are Associated With Cognitive Performance in Parkinson’s Disease. Front. Aging Neurosci. 13:740491. doi: 10.3389/fnagi.2021.740491
Received: 13 July 2021; Accepted: 04 October 2021;
Published: 09 March 2022.
Edited by:
Dennis Qing Wang, Southern Medical University, ChinaReviewed by:
Julien Rossignol, Central Michigan University, United StatesSharad Purohit, Augusta University, United States
Rongfang Que, Southern Medical University, China
Copyright © 2022 Fang, Dai, Jin, Si, Gu, Song, Gao, Chen, Yan, Yin, Pu and Zhang. This is an open-access article distributed under the terms of the Creative Commons Attribution License (CC BY). The use, distribution or reproduction in other forums is permitted, provided the original author(s) and the copyright owner(s) are credited and that the original publication in this journal is cited, in accordance with accepted academic practice. No use, distribution or reproduction is permitted which does not comply with these terms.
*Correspondence: Baorong Zhang, brzhang@zju.edu.cn; Jiali Pu, jialipu@zju.edu.cn
† These authors have contributed equally to this work and share first authorship