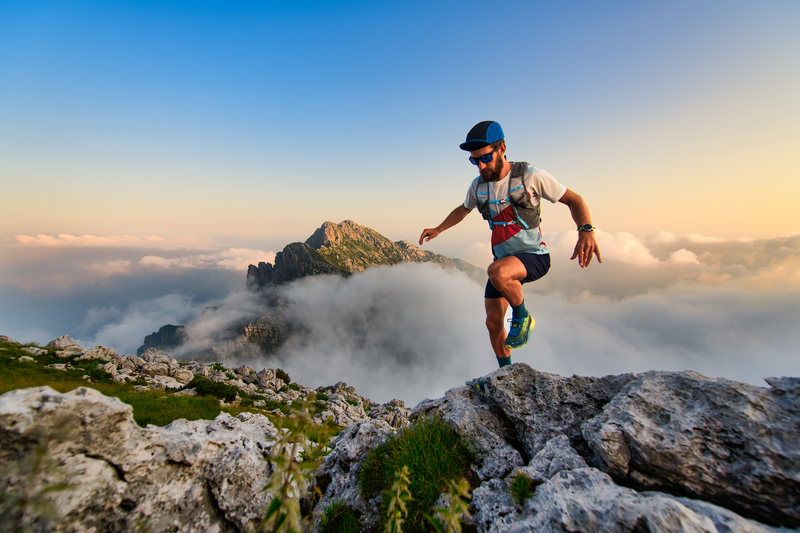
94% of researchers rate our articles as excellent or good
Learn more about the work of our research integrity team to safeguard the quality of each article we publish.
Find out more
ORIGINAL RESEARCH article
Front. Aging Neurosci. , 17 December 2021
Sec. Alzheimer's Disease and Related Dementias
Volume 13 - 2021 | https://doi.org/10.3389/fnagi.2021.721217
This article is part of the Research Topic Translational Advances in Alzheimer's, Parkinson's, and other Dementia: Molecular Mechanisms, Biomarkers, Diagnosis, and Therapies, Volume II View all 58 articles
The cognitive manifestations of Alzheimer’s disease (AD) are related to brain network degeneration, and genetic differences may mediate network degeneration. Several AD-susceptible loci have been reported to involve amyloid or tau cascades; however, their relationships with gray matter (GM) volume and cognitive outcomes have yet to be established. We hypothesized that single-nucleotide polymorphism genotype groups may interact with apolipoprotein E4 (ApoE4) status or independently exert an effect on cognitive outcomes. We also hypothesized that GM structural covariance networks (SCNs) may serve as an endophenotype of the genetic effect, which, in turn, may be related to neurobehavior test scores. Gray matter SCNs were constructed in 324 patients with AD using T1 magnetic resonance imaging with independent component analysis (ICA). We assessed the effects of 15 genetic loci (rs9349407, rs3865444, rs670139, rs744373, rs3851179, rs11136000, rs3764650, rs610932, rs6887649, rs7849530, rs4866650, rs3765728, rs34011, rs6656401, and rs597668) using additive, recessive, and dominant models on cognitive outcomes. Statistical analysis was performed to explore the independent role of each locus, interactions with ApoE4 status, and relationships to GM ICA network intensity score. For outcome measures, we used the Mini-Mental State Examination (MMSE), Cognitive Abilities Screening Instrument (CASI) total score, and short-term memory (STM) subscores, adjusted for the covariates of education, disease duration, and age. Clinically, the CD2AP G allele showed a protective role in MMSE, CASI total, and CASI-STM scores independently or via interactions with non-ApoE4 status, while the CR1 A genotype group was associated with lower STM subscores independent of ApoE4 status. Three loci showed synergic interactions with ApoE4: BIN 1, MS4A6A, and FTMT. Of the 15 meaningful ICA components, 5 SCNs (anterior and posterior hippocampus, right temporal, left thalamus, default mode network) showed relationships with general cognitive performance, in which only the ApoE4 and MS4A6A genotype groups were independently related to the hippocampus network. The genetic loci MS4A6A, BIN1, CLU, CR1, BIN1, PICALM, and FGF1 influenced the networks independently or in synergy. This study suggests that AD-susceptible loci may each exert clinical significance independently through interactions with ApoE4 status or through SCNs as an endophenotype and that this effect is associated with the cognitive outcomes.
The National Institutes on Aging-Alzheimer’s Association (NIA-AA) criteria (Jack et al., 2018) incorporate diagnostic biomarkers in the definition of Alzheimer’s disease (AD), and the key pathology is the presence of amyloid plaque and neurofibrillary tangles. From a genetic point of view, AD is a complex disease. For late-onset AD, the apolipoprotein E (ApoE) gene is the most significant example of a single-nucleotide polymorphism (SNP) that modifies susceptibility to AD (Singh et al., 2006). The clinical significance of ApoE4 on brain integrity has been shown in studies of people with AD (Martins et al., 2005), those with mild cognitive impairment (Risacher et al., 2013), and the non-demented elderly (Bretsky et al., 2003; Wisdom et al., 2011). The presence of the ApoE4 allele (Corder et al., 1993) remains the greatest risk factor for AD (Farrer et al., 1997), responsible for a 3- to 15-fold increase in risk. The synergic effect of the ApoE4 allele with other genetic loci has been reported in previous studies (Mahley, 1988), which showed that overall pathological cascades may influence neuropsychiatric outcomes (Hall et al., 2014). Although genetic effects have been extensively studied, only a few studies have shown reproducible results. The mechanisms by which ApoE influences the pathogenesis of AD have been proposed, including a role in the clearance of amyloid (Koistinaho et al., 2004; Shi et al., 2017; Ringland et al., 2020). However, whether this is via interactions between APoE4 and amyloid- or tau-related SNPs remains to be explored.
Recent large-scale genome-wide association studies (GWASs) have revealed several SNPs other than ApoE4 that are associated with susceptibility to AD. In a study by Lambert et al. (2013), a total of 19 GWAS loci were identified using a discovery sample of 17,008 patients with AD and 37,154 controls, followed by replication with 8,572 patients with AD and 11,312 controls. In 2019, a GWAS meta-analysis reported an additional 29 risk loci (Jansen et al., 2019). Collectively termed as AD-susceptible genes, they can only explain a small proportion of the heritability and influence of SNPs for risk prediction, and the results have been inconsistent among different ethnicities. For example, the rs11136000 polymorphism in the clusterin (CLU) gene has been significantly associated with AD (Zhu et al., 2018) in Caucasian and Asian populations, but not in people of African or Hispanic descent (Du et al., 2016). Genetic-gray matter (GM) interactions may be different among different ethnicities, and so the genetic effect on the cognitive outcomes may also be different. For AD, structural imaging is one of the most reliable tools to reveal correlations with cognitive measures, while links between reported genotype groups and neuroimaging biomarkers may help to understand how these SNPs modulate GM. The clinical significance of these risk loci and their interactions with brain networks may also help to understand the neurobiology of AD.
Recent research suggests that highly related regions show covariance in morphometric characteristics, the so-called structural covariance. Structural covariance networks (SCNs) have been associated with structural and functional connectivity, while genetic variations, developmental, degenerative, and disease staging have been shown to be important covariates of interest (Alexander-Bloch et al., 2013). SCNs are considered to be reproducible and heritable models that may represent disease-related or genetic-associated changes in topology (Huang et al., 2017; Chang et al., 2018a,b; Chang H. I. et al., 2019). We hypothesized that AD-susceptible genes may have greater covariance in GM and that the SCNs may serve as an endophenotype to reflect the influence of the risk gene. Using spatial independent component analysis (ICA), the structural covariance between topographically distant regions can be modeled without a priori knowledge (Biswal et al., 1995, 2010). In addition to fully automated spatial component maps of maximal statistical independence, a network integrity score (NIS) can be calculated for each ICA map. The NIS describes the strength of the individual expression in each network, and a higher NIS indicates a stronger individual expression of the identified network (Beckmann and Smith, 2004; Segall et al., 2012). Once an SCN pattern has been identified to distinguish one group from another, its expression can be prospectively quantified on an individual basis and correlated with the clinical or physiological measures of interest. GM SCNs modeled by ICA may help to clarify SNP-GM relationships, while the NIS can be used as a dependent variable to test the genetic interactions.
The NIA-AA criteria define AD as a disease status because higher diagnostic hierarchy is placed on the in vivo demonstration of neuropathological status rather than the presence of clinical symptoms. For a diagnosis of AD, the presence of amyloid and tau protein is essential (Jack et al., 2018). To understand the genetic effects of amyloid or tau on disease progression, we selected 15 amyloid- or tau-related SNPs belonging to the following genetic loci: CD2AP (rs9349407) (Furusawa et al., 2019), CD33 (rs3865444) (Bradshaw et al., 2013; Chen et al., 2021), MS4AE4 (rs670139) (Karch et al., 2012), BIN1 (rs744373) (Martiskainen et al., 2015), PICALM (rs3851179) (Zhao et al., 2015), CLU (rs11136000) (Chibnik et al., 2011), ABCA7 (rs3764650) (Bamji-Mirza et al., 2016; Zhao Q. F. et al., 2016; Ma et al., 2018), MS4A6A (rs610932) (Dos Santos et al., 2017), FTMT (rs6887649) (Yang et al., 2015), SPTLC1 (rs7849530) (Geekiyanage and Chan, 2011), Intergenic SNP (rs4866650) (Hohman et al., 2014), p73 (rs3765728) (Wetzel et al., 2008), FGF1 (rs34011) (Kimura et al., 1994), CR1 (rs6656401) (Chibnik et al., 2011; Biffi et al., 2012), and EXOC3L2 (rs597668) (Olgiati et al., 2011). In this study, we first tested whether each SNP exerted an effect on cognitive measures directly or via interactions with ApoE4. Then, we evaluated whether the genetic effects on cognitive measures were via an SCN as an endophenotype.
This study was conducted in accordance with the Declaration of Helsinki and was approved by the Institutional Review Board of Chang Gung Memorial Hospital. The study participants were treated at the Cognition and Aging Center, Department of General Neurology, Kaohsiung Chang Gung Memorial Hospital. The multidisciplinary team was composed of behavioral neurologists, psychiatrists, neuropsychologists, neuroradiologists, and experts in nuclear medicine. We enrolled patients with AD who were diagnosed according to the International Working Group-2 criteria (Dubois et al., 2014) and further confirmed using amyloid imaging (TW-ADNI1) if the consensus panel did not agree on the diagnosis. All of the patients were in a stable condition under acetylcholine esterase inhibitor treatment from the time of diagnosis. The exclusion criteria were a history of clinical stroke, a negative amyloid scan, a modified Hachinski ischemic score > 4, and depression. After checking the inclusion and exclusion criteria, a total of 324 subjects (152 males and 172 females) were included and underwent imaging and genetic tests.
After enrollment, the demographic data of each patient were recorded. We also recorded the time of the first symptom during interviews with family members so that an estimated duration of onset could be calculated. At baseline, a trained neuropsychologist administered the neurobehavioral tests using the Mini-Mental State Examination (MMSE) and Cognitive Abilities Screening Instrument (CASI) total score as a global assessment of cognitive function. The CASI contains nine subdomains. We used attention, verbal fluency, abstract thinking, and mental manipulation subdomain scores to assess executive function (Huang et al., 2013) and orientation, short- and long-term memory, language ability, and drawing as non-executive domains. As the salient feature of AD is short-term memory (STM) impairment, we used CASI-STM subscores as the major outcome for genetic correlations.
Single-nucleotide polymorphism genotyping was performed using MassARRAY technology with iPLEX Gold chemistry (Agena Bioscience, San Diego, CA, United States). The PCR primers and single base extension primers were designed using Assay Design Suite version 2.0 software (Redwood City, CA, United States). The genotyping analysis was performed using an iPLEX Gold Reagent Kit according to the instructions of the manufacturer. Briefly, 1 μl of DNA sample (10 ng/μl) was added to 5 μl of PCR reaction mixture containing 0.2 units of Taq polymerase, 2.5 pmol each of the PCR primers, and 25 mM each of the dNTPs. Thermocycling was started at 94°C for 2 min followed by 45 cycles of 94°C for 30 s, 56°C for 30 s, and 72°C for 1 min, and a final extension was done at 72°C for 1 min. Unincorporated dNTPs were dephosphorylated using 0.3 U of shrimp alkaline phosphatase. Purified amplicons were then subjected to primer extension using an iPLEX Gold Reagent Kit. Primer extension was performed using a cycling program of 94°C for 30 s, followed by 40 cycles of 94°C for 5 s, and 5 cycles of 52°C for 5 s, and 80°C for 5 s within 40 cycles, followed by a final extension at 72°C for 3 min. The extended reaction products were purified using cation exchange resin and then spotted onto a 384-format SpectroCHIP II array using a MassArray Nanodispenser RS1000 (San Diego, CA, United States). Mass determination was done on a MassARRAY Compact Analyzer. The resulting spectra were processed, and alleles were called using MassARRAY Typer 4.0 with model-based cluster analysis to analyze the genotypes of the SNPs. We tested 15 SNPs (rs9349407, rs3865444, rs670139, rs744373, rs3851179, rs11136000, rs3764650, rs610932, rs6887649, rs7849530, rs4866650, rs3765728, rs34011, rs6656401, and rs597668). The risk alleles and minor allele frequencies (MAFs) are listed in Supplementary Table 1. The ApoE genotype was determined using rs7412 and rs429358. ApoE4 carriers were defined as those with one or two E4 alleles.
Magnetic resonance images were acquired using a 3.0T magnetic resonance imaging (MRI) scanner (Excite, GE Medical Systems, Milwaukee, WI, United States). All MRI images were performed within 3 months of taking the cognitive tests. High-resolution structural images were acquired for spatial normalization using the following protocol: a T1-weighted, inversion-recovery-prepared, three-dimensional, gradient-recalled acquisition in a steady-state sequence with a repetition time/echo time/inversion time of 8,600 ms/minimal/450 ms, a 256 mm × 256 mm field of view, and a 1-mm slice sagittal thickness with a resolution of 0.5 mm × 0.5 mm × 1 mm.
Image preprocessing and statistical analysis were performed using SPM12 (Wellcome Trust Centre of Cognitive Neurology, University College London, United Kingdom2). The T1 images were reoriented, realigned, and normalized using the standard Montreal Neurological Institute space. The images were then segmented into GM and white matter. Related tissue segments were used to create a custom template using diffeomorphic anatomical registration with an exponentiated lie algebra approach, which is one of the highest ranking registration methods in patients with AD (Cuingnet et al., 2011). To correct for partial volume effects, the segmentation of tissue type was performed using partial volume estimation. During the modulation step, each voxel of the registered GM image was multiplied by the Jacobian of the warp field that defined the direction and amount of modulation. The modulated and warped GM images were then smoothed using a Gaussian kernel of 8 mm full width at half maximum.
The preprocessed spatially normalized modified GM images of the patients were concatenated to form a subject series and entered into the ICA process. Spatial ICA was carried out using the Multivariate Exploratory Linear Optimized Decomposition into Independent Components software package version 3.153. The resulting independent components were z-transformed and visualized using a threshold of z > 1.96 (p < 0.05). In general, the optimal number of components is related to the data size and the research questions. A lower number of components causes a loss of spatial information, while a higher number of components can result in less meaningful components. In this study, we set the number of independent components to 25. Among these 25 components, we determined 15 to be meaningful based on matching them with the published data and templates from a study investigating the social brain across childhood and adolescence (McCormick et al., 2018) and the BrainMap 70-component ICA template4.
We first tested whether each SNP exerted an effect on cognitive measures directly or via interactions (or synergistically) with ApoE4. Next, to evaluate whether the genetic effects on cognitive measures were via the SCN as an endophenotype, we explored the SCN-cognitive and SCN-SNP relationships. The SCN-cognitive relationships were explored using a linear regression model with the cognitive scores as the dependent variable and the NIS of each SCN as the independent variable, adjusted for age, education, and disease duration. Finally, we modeled how the 15 SNPs and ApoE4 status may affect the GM SCN (and SCN-SNP relationship) using a multivariate linear model.
Clinical data were expressed as mean ± standard deviation. The Student’s t-test was used to compare continuous variables, and the chi-square test was used to compare categorical variables. To examine the genotype effects on cognitive outcome measures, we used additive, dominant, and recessive models (Zhao F. et al., 2016). The dichotomization of the SNP genotype into different genetic models was based on the risk allele. Based on the classification, the additive model compared three groups: risk homozygotes, risk heterozygotes, and non-risk homozygotes. For the dominant model, the genotype group was dichotomized into risk (homozygote and heterozygotes) and non-risk groups. For the recessive group, the risk homozygotes were considered to be one group and compared with the risk heterozygotes and non-risk homozygotes. For each SNP, the risk allele is listed in Supplementary Table 1 according to a literature review of the Chinese-Han population, and binary group stratification was based on the Hardy-Weinberg equation for equilibrium. Using the MMSE, CASI total score, or STM subscore as dependent variables, we also explored the interactions between the susceptible SNPs and ApoE4 status (ApoE4 carriers and non-E4 carriers) using a linear regression model, with education, age, and duration of disease as covariates. All statistical analyses were conducted using SPSS software (SPSS version 22 for Windows®, SPSS Inc., Chicago, IL, United States). Statistical significance was set at p < 0.05.
For each component, the intensity was calculated and recorded as NIS, which was then Z-transformed in the regression model. Differences in the ICA NIS of each SNP were calculated using the Student’s t-test based on the predefined groups in Supplementary Table 1. To understand the clinical significance of each SCN, we used a linear regression model to explore the relationships between outcome measures (MMSE, CASI total score, and executive and non-executive domains) and NIS with education, age, and duration of disease as covariates. In addition, to understand the clinical significance of the identified SCN in the CASI subscores, we also calculated partial correlations between the extracted SCN NIS and the cognitive scores by setting the significance value at p < 0.05 and the covariates as age, educational level, and disease duration (years).
Finally, multivariate linear regression analysis was performed to assess the SCN-SNP relationships. Fifteen models were run (one for each SCN NIS), and each model consisted of the following variables: age, sex, the 15 SNPs, and APOE E4 status. For the SNPs that showed significance on NIS, we tested the interactions with ApoE4 status. The NIS served as the dependent variable, with the significant SNP, ApoE4, and interaction (SNP*ApoE4) as independent variables. All independent variables were entered in one block and the significance value was set at p < 0.05.
The demographic data and cognitive test scores are shown in Table 1. All of the enrolled patients had early-stage AD, as the mean duration of disease was 0.9 ± 1.12 years. Among the enrolled patients, 114 were E4 carriers (E4 homozygote n = 14). The risk alleles CD2AP, ABCA7, FTMT, SPTLC1, FGF1, CR1, and EXOC3L2 were found to be minor alleles (Supplementary Table 1). Of note, the MAFs of FTMT and CR1 were extremely low.
For cognitive outcomes (MMSE, CASI total scores, and STM subscores), gender had no effect when controlling for educational level. For those with an educational level ≤ 6 years (elementary school), 7–12 years (high school), and >12 years, none of the comparisons of test scores between the male and female patients were significant. There were age effects on MMSE (r = − 0.23, p < 0.0001), CASI total (r = − 0.259, p < 0.0001), and STM subscores (r = − 0.271, p < 0.0001). The educational effects on MMSE (r = 0.432, p < 0.0001), CASI total (r = 0.433, p < 0.0001), and STM subscores (r = 0.372, p < 0.0001) were also significant.
The results of the additive model, dominant model, and recessive model of each SNP on MMSE and CASI are shown in Tables 2, 3. The CD2AP G allele was associated with MMSE total score (Table 2) or CASI total score (Table 3) in the additive or dominant model, and the G allele was associated with higher scores. The CD2AP G allele (in the additive or dominant model) was also associated with a higher STM subscore (Table 4). The association between the CD2AP G allele and cognitive scores showed interactions with the non-ApoE4 genotype in MMSE (Table 2), CASI total score (Table 3), and STM subscore (Table 4). In the recessive model, the CR1 A allele was associated with a lower STM subscore (Table 4), while the risk of a detrimental effect was independent of ApoE4 status. Of note, the MAF of the A allele in CR1 was 0.029.
Table 2. General linear model for single nucleotide polymorphisms on mini-mental score examination*.
Table 3. General linear model for single nucleotide polymorphisms on cognitive ability screening instrument total scores*.
Table 4. General linear model for single nucleotide polymorphisms on cognitive ability screening instrument short-term memory score*.
Short-term memory is the salient feature in AD, and we found three additional SNPs that showed interactions with the ApoE4 gene: BIN 1, MS4A6A, and FTMT (Table 4 and Supplementary Figure 1). The E4 allele was related to lower scores in the BIN 1, FTMT, and MS4A6A alleles. The MAF of the G allele in FTMT was 0.082.
A total of 15 ICA components were constructed and considered to be meaningful networks (Figure 1). The MMSE and CASI total scores were considered to indicate general cognitive performance, and their relationships with significant NIS are shown in Figure 2. Among the significant networks, the anterior and posterior hippocampus, right temporal, left thalamus, and default mode network were the most important for general cognitive performance. We then used NIS as the dependent variable to explore its relationships with STM, executive domains, and non-executive domains, adjusted for age and educational level. The standardized beta coefficients (95% confidence intervals) and exact p-values are reported in Table 5. For STM, executive domains, and non-executive domains, the aforementioned five networks (Figure 2) showed statistical significance, while the dorsal attentional network also showed statistical significance. Finally, we examined the relationships between CASI subdomains and NIS (Supplementary Table 2). The results suggested that the NIS of the anterior and posterior hippocampus, right temporal, right thalamus, lateral cerebellum, and medial cerebellum (all p < 0.0001) were consistently related to the cognitive test scores adjusted for age and years of education.
Figure 1. Significant structural covariance network (A–O) showing group differences in signal intensities using independent component analysis.
Figure 2. The Z-transformed cognitive (A) Mini-Mental State Examination [MMSE] or (B) Cognitive Ability Screening Instrument [CASI]-structural covariance network relationships, adjusted for age, years of education, and disease duration (years). Dots represent each participant in this study, and the linear relationships and 95% confidence intervals of individuals are shown. r = partial correlation coefficient. The exact p-values are shown.
Table 5. Linear regression model between network intensity score analysis and cognitive test scores (Z transformed).
Using the binary classifications as described in Supplementary Table 1, seven SNPs were found to have an independent effect on NIS (Figure 3). To avoid possible false positives based on the 16 × 15 SCN-SNP matrix and a p-value of 0.05, we further explored the SCN-SNP relationships (Table 6) using a multivariate linear model. The NIS served as the dependent variable, and the independent variables included ApoE4 status, rs9349407, rs3865444, rs670139, rs744373, rs3851179, rs11136000, rs3764650, rs610932, rs6887649, rs7849530, rs4866650, rs3765728, rs34011, rs6656401, and rs597668, adjusted for age and sex. Among the tested 16 genetic loci, the significance of the 7 SNPs (ApoE4, MS4A6A, BIN1, CLU, CR1, PICALM, and FGF1) showed independent or collinear relationships with the SCNs. The predictive roles of CLU and CR1 on supplementary motor cortex SCNs were independent, and no interactions with ApoE4 were found.
Figure 3. Significant group differences between the risk and non-risk allele (A–G) in network intensity score (p < 0.05). X axis: SNP group, Y axis: Z transformed network intensity values.
Table 6. Risk allele per structural covariance network of 15 tested single nucleotide polymorphisms (SNP) and ApoE4.
In this study, we tested the effects of amyloid- or tau-related genotypes on cognitive measurements and evaluated whether the genetic effects on cognitive measures were via SCNs as an endophenotype. We found that three different mechanisms modulated the relationships among AD-susceptible loci, GM network, and cognitive measures. First, an independent role of the genetic effect on cognitive test scores was found, as the CD2AP and CR1 genotypes were directly related to CASI and MMSE scores. Second, the effects of amyloid- or tau-related genotypes on cognitive measures interacted or had a synergic effect with ApoE4 status. Finally, we identified several SCNs that may serve as endophenotypes of AD-susceptible loci in predicting cognitive outcomes. The SCNs showing clinical significance with cognitive scores included the hippocampal, temporal, thalamus, default mode network, and dorsal attention network. In multivariate regression, seven genotype groups were found to either exert an independent effect or synergistic effect on these SCNs. As the SCNs were associated with different cognitive domains, the identification of genotype-SCN relationships may help to understand the neurobiology of SNPs on the cognitive features in AD.
The role of rs9349407 as a risk SNP in Han Chinese populations has been reported (Xiao et al., 2015); however, the findings have been inconsistent (Jiao et al., 2015). One meta-analysis suggested that rs9349407 C is a risk allele for susceptibility to AD in East Asian, American, Canadian, and European populations (Chen et al., 2015). In our additive and dominant models, patients with AD with the rs9349407 G allele had higher CASI total and STM subscores, especially the non-E4 carriers. The minor allele C of rs9349407 has been associated with neuritic plaque burden, which may explain why non-E4 carriers with the G allele may have higher cognitive test scores (Shulman et al., 2013).
In this study, we only enrolled patients with clinical AD, and we tested whether risk SNP alleles or protective SNP alleles affected cognitive test scores or SCNs. Although not all of our patients with AD received amyloid scans, our exclusion criteria reduced the possibility of non-AD pathologies. Rs9349407 is a polymorphism in the CD2AP gene that translates the scaffolding molecule for signal transduction. Loss of function of CD2AP has been linked to enhanced Aβ production, tau-induced neurotoxicity, abnormal neurite structure modulation, and reduced blood-brain barrier integrity, which has been implicated in AD pathogenesis (Dubey et al., 2018; Ramos de Matos et al., 2018).
CR1 is an AD susceptibility locus that also influences AD-related traits on neuritic plaque deposition and episodic memory decline. As STM is the salient feature in AD, it was used in this study as a dependent variable in the SNP genetic model analysis. We identified the independent role of the rs6656401 A allele on lower STM subscores, consistent with a previous meta-analysis of greater risk with the minor allele A in AD (Shen et al., 2015). A coding variant in the long homologous repeat D region of the CR1 gene, rs4844609 (Ser1610Thr), has been associated with episodic memory decline and been shown to account for the known effect of SNP rs6656401 (Keenan et al., 2012). CR1 risk allele A was a minor allele with a low MAF in this study, and the relationship with lower STM may be due to the small number of patients with the A allele. However, three studies have confirmed the association between AD susceptibility and the rs6656401 A allele in Chinese patients (Zhang et al., 2010; Chen et al., 2012; Jin et al., 2012), although another study showed no association (Li et al., 2011). Rs6656401 is in the CR1 gene, and complement system activation in the clearance of amyloid has been proposed to be a possible mechanism of the risk associated with rs6656401 (Zhang et al., 2010). Although the independent role of rs6656401 minor allele on lower STM was confirmed in this study, it is worth noting that the A allele was a minor allele and the MAF was only 0.029. Therefore, the findings related to CR1 in this study should be interpreted with caution.
To understand the effect, we also evaluated SCN intensity and explored whether the relationships between the rs6656401 A allele and cognitive test results may have been modulated by SCNs. As shown in our regression model, the dorsal attention SCN intensity and supplementary motor cortex SCN were significantly different between the risk and protective alleles, and the network intensity was also related to the executive and non-executive domains. Therefore, we suggest that the dorsal attention network is the endophenotype of the link between rs6656401 and cognitive outcomes.
Based on the relationships between SCN and cognitive test scores, we identified several significant networks that predicted general or isolated cognitive function in the patients (Table 5, Figure 2, and Supplementary Table 2). For these significant networks, we identified independent or synergic effects in seven genotype groups that predicted the network integrity. As the clinical significance of SCNs was established in regression analysis with different cognitive domains, SCNs may have served as the endophenotype between the risk SNP group and cognitive test scores.
By definition, SCNs are based on the similarity of the same microstructural variations and thus may be influenced by factors influencing underlying structures such as the expression of common genetic traits during development. The independent role of ApoE4 on the anterior hippocampus and precentral and supplementary motor cortex SCN was established in this study. As the hippocampal SCN intensity scores were also correlated with STM subscores in our patients with AD, this finding supports the role of ApoE4 in linking salient cognitive and biosignature features in AD.
An important finding in this study is the relationship between ApoE4 and the anterior hippocampal area. Traditional cytoarchitectonic distribution of the hippocampus (Frederickson et al., 1983) is a convoluted GM structure encompassing three architectonically distinct regions: the fascia dentata, the CA region (which can be subdivided into CA1–CA4 fields), and the subicular complex. In this study, two hippocampus SCNs in ICA (anterior vs. posterior hippocampus) showed ApoE4 genotype group differences. The SCN of the hippocampal axis associated with ApoE4 showing the anterior-posterior axis is consistent with task-related activities or connectivity patterns (Colombo et al., 1998; Przeździk et al., 2019). A similar anterior-posterior organization has recently been reported, suggesting that the gene expression is linked to the distinct molecular gradient in the hippocampus (Vogel et al., 2020). Among the 15 preselected SNPs and ApoE4, ApoE4 had an independent role on the anterior hippocampal axis, in contrast to MS4A6A on the posterior axis. The association with cognitive test scores in hippocampal SCN intensity scores and the interactions between the hippocampus and cortical/subcortical structures demonstrate its close integration within large-scale degenerative systems.
A previous study reported interactions between MS4A and CLU (Lambert et al., 2013) or CD33 on conferring the risk of AD. Meanwhile, common variants of MS4A6A (rs610932), MS4A4E (rs670139), CD33 (rs3865444), CD2AP (rs9349407), CLU, and PICALM have been associated with memory decline (Hollingworth et al., 2011; Naj et al., 2011; Karch et al., 2012). These reports emphasize the risk and role of MS4A6A in disease progression, while our results emphasize the role of MS4A6A (rs610932) and posterior hippocampus in the clinical features of AD. Similar to a report with a smaller AD cohort (Chang Y. T. et al., 2019), the dissociation of the hippocampus (anterior or posterior) emphasizes the interplay of genetic loci on anatomy.
The most commonly affected brain region in AD studies is the default mode network, and the deposition of amyloid or tau protein is localized in this network (Hansson et al., 2017; Jones et al., 2017). The default mode network is regarded to be an early neuroimaging biosignature (Chang et al., 2015), and a recent report suggested that the default mode network may be comprised of multiple, spatially dissociated but interactive components (Andrews-Hanna et al., 2010), of which two subsystems are of particular interest. Our SCN results of associations with BIN1 and CLU are consistent with cores in the posterior cingulate cortex and anterior medial prefrontal cortex, which is known as the “dorsal medial prefrontal cortex subsystem” (or midline core subsystem). The genetic locus rs744373 near BIN1 (OR = 1.13; 95% CI, 1.06–1.21 per copy of the minor allele; p = 1.59 × 10–11) has been related to AD, with the possible mechanism involving tau-related cascade (Franzmeier et al., 2019). BIN1 also modulated the inferior cerebellum and left thalamus and could predict cognitive scores in this study.
This study has several limitations. First, we enrolled subjects with early-stage AD, and we did not include a control group or subjects with late-stage AD. As the SNPs were preselected from GWAS results showing significance in AD susceptibility, the inclusion of a control group may have helped to elucidate whether these SNPs exerted similar GM modulation patterns in healthy elderly subjects as in those with AD. However, as these SNPs each exert different functional activities on the pathogenetic mechanisms in AD, the use of a pure AD population may help to maximize the effect of each SNP on regional GM networks. As a structural covariance matrix is defined by estimating the interregional correlations of cortical volumes between all possible pairs of regions defined by anatomy, SCN construction relies on both the spatial patterns of morphometric and signal similarities. Given the differences in brain morphometry in controls, it would be difficult to match all influential factors. In this study, we did not include a late-stage AD group based on two considerations. First, we wanted to examine interactions between SCNs and SNPs in a more uniform population, so only subjects with early-stage AD were included. Second, the enrollment of subjects with late-stage AD may have included SCNs related to disease progression; however, this was not the major purpose of this study. The effects of susceptible SNPs and disease progression are important scientific questions. However, we focused on the initial hypothesis and tested the endophenotypic role of SCNs in cognitive outcomes in a uniform population with early-stage AD. A larger sample size including subjects with different stages of AD may help to extend the understanding of susceptible SNPs and brain degeneration patterns.
Another limitation is the estimation of the number of components for ICA analysis. Most studies have used 12–30 components in structural networks or resting-state networks. In this study, we constructed SCN networks and filtered the clinical significance of a network using correlation analysis with cognitive measures and by matching with the ICA template (see footnote 4). From a methodological aspect, the groupwise structural covariance analysis relied on the morphological properties of each voxel with the rest of the brain across a group of participants. The registration of the structural data of a single participant to the template involves linear and non-linear deformation that can result in inaccuracies in subregions. In this report, we emphasized well-characterized networks to explain the SNP effect, where the network significance was established by correlations with cognitive measures. Finally, the use of 15 AD-susceptible SNPs to validate the underlying pathological mechanisms may have oversimplified the genetic interactions. However, these SNPs were chosen based on their significance in large-scale GWASs in which they were all involved in amyloid or tau cascades, so the hypothesis was to test their effect on cognitive scores. We only tested interactions between each SNP and ApoE4 status, as ApoE4 remains the strongest predictor. The interpretations of interactions between SNPs and SCNs were based on a literature review of possible alterations in functional pathways and may not fully explain the in vivo situation. Therefore, the findings should be interpreted with caution.
In AD, our findings demonstrated amyloid or tau-related SNP effects that may influence SCNs independently or synergistically with ApoE4. The use of SCNs as an endophenotype allowed us to assume the independent and synergistic role of putative SNPs to predict cognitive measures. The complex interplay among these SNPs in our study suggests that the hierarchical order of SNPs modulates GM networks.
The raw data supporting the conclusions of this article will be made available by the authors, without undue reservation.
The studies involving human participants were conducted in accordance with the Declaration of Helsinki and were reviewed and approved by the Institutional Review Board of Chang Gung Memorial Hospital. Written informed consent to participate in this study was provided by the participants.
C-WH, K-LH, J-LH, S-WH, S-JT, W-NC, C-CL, S-HH, and C-CC contributed to the acquisition and analysis of data. H-IC, Y-TC, and C-CC drafted a significant portion of the manuscript and figures. All authors read and approved the final manuscript.
This work was supported by grants CMRPG8J0522, CMRPG8J0842, and CMRPG8K1531 from Chang Gung Memorial Hospital and 109-2314-B-182A-082 from the Ministry of Science and Technology (MOST), Taiwan, to C-CC.
The authors declare that the research was conducted in the absence of any commercial or financial relationships that could be construed as a potential conflict of interest.
The reviewer K-JT declared a shared affiliation, with no collaboration, with one of the authors, Y-TC, to the handling editor at the time of the review.
All claims expressed in this article are solely those of the authors and do not necessarily represent those of their affiliated organizations, or those of the publisher, the editors and the reviewers. Any product that may be evaluated in this article, or claim that may be made by its manufacturer, is not guaranteed or endorsed by the publisher.
We thank the Genomics Center for Clinical and Biotechnological Applications of the National Core Facility for Biopharmaceuticals, Taiwan (MOST 109-2740-B-010-002), for genotyping. We thank the participants and their families for their generosity.
The Supplementary Material for this article can be found online at: https://www.frontiersin.org/articles/10.3389/fnagi.2021.721217/full#supplementary-material
Supplementary Figure 1 | Effect of interactions with the ApoE4 gene on STM scores was found in CD2AP, BIN 1, MS4A6A, and FTMT.
Alexander-Bloch, A., Giedd, J. N., and Bullmore, E. (2013). Imaging structural co-variance between human brain regions. Nat. Rev. Neurosci. 14, 322–336. doi: 10.1038/nrn3465
Andrews-Hanna, J. R., Reidler, J. S., Sepulcre, J., Poulin, R., and Buckner, R. L. (2010). Functional-anatomic fractionation of the brain’s default network. Neuron 65, 550–562. doi: 10.1016/j.neuron.2010.02.005
Bamji-Mirza, M., Li, Y., Najem, D., Liu, Q. Y., Walker, D., Lue, L. F., et al. (2016). Genetic Variations in ABCA7 Can Increase Secreted Levels of Amyloid-beta40 and Amyloid-beta42 Peptides and ABCA7 Transcription in Cell Culture Models. J. Alzheimers Dis. 53, 875–892.
Beckmann, C. F., and Smith, S. M. (2004). Probabilistic independent component analysis for functional magnetic resonance imaging. IEEE Trans. Med. Imaging 23, 137–152. doi: 10.1109/tmi.2003.822821
Biffi, A., Shulman, J. M., Jagiella, J. M., Cortellini, L., Ayres, A. M., Schwab, K., et al. (2012). Genetic variation at CR1 increases risk of cerebral amyloid angiopathy. Neurology 78, 334–341. doi: 10.1212/WNL.0b013e3182452b40
Biswal, B., Yetkin, F. Z., Haughton, V. M., and Hyde, J. S. (1995). Functional connectivity in the motor cortex of resting human brain using echo-planar MRI. Magn. Reson. Med. 34, 537–541.
Biswal, B. B., Mennes, M., Zuo, X. N., Gohel, S., Kelly, C., Smith, S. M., et al. (2010). Toward discovery science of human brain function. Proc. Natl. Acad. Sci. U. S. A. 107, 4734–4739.
Bradshaw, E. M., Chibnik, L. B., Keenan, B. T., Ottoboni, L., Raj, T., Tang, A., et al. (2013). CD33 Alzheimer’s disease locus: altered monocyte function and amyloid biology. Nat. Neurosci. 16, 848–850. doi: 10.1038/nn.3435
Bretsky, P., Guralnik, J. M., Launer, L., Albert, M., and Seeman, T. E. (2003). The role of APOE-epsilon4 in longitudinal cognitive decline: MacArthur Studies of Successful Aging. Neurology 60, 1077–1081. doi: 10.1212/01.wnl.0000055875.26908.24
Chang, C. C., Chang, Y. T., Huang, C. W., Tsai, S. J., Hsu, S. W., Huang, S. H., et al. (2018a). Associations of Bcl-2 rs956572 genotype groups in the structural covariance network in early-stage Alzheimer’s disease. Alzheimers Res. Ther. 10:17. doi: 10.1186/s13195-018-0344-4
Chang, C. C., Tsai, S. J., Chen, N. C., Huang, C. W., Hsu, S. W., Chang, Y. T., et al. (2018b). Catechol-O-Methyltransferase Val158Met Polymorphism on Striatum Structural Covariance Networks in Alzheimer’s Disease. Mol. Neurobiol. 55, 4637–4649. doi: 10.1007/s12035-017-0668-2
Chang, H. I., Chang, Y. T., Tsai, S. J., Huang, C. W., Hsu, S. W., Liu, M. E., et al. (2019). MAOA-VNTR Genotype Effects on Ventral Striatum-Hippocampus Network in Alzheimer’s Disease: analysis Using Structural Covariance Network and Correlation with Neurobehavior Performance. Mol. Neurobiol. 56, 4518–4529. doi: 10.1007/s12035-018-1394-0
Chang, Y. T., Huang, C. W., Chang, Y. H., Chen, N. C., Lin, K. J., Yan, T. C., et al. (2015). Amyloid burden in the hippocampus and default mode network: relationships with gray matter volume and cognitive performance in mild stage Alzheimer disease. Medicine 94:e763. doi: 10.1097/MD.0000000000000763
Chang, Y. T., Mori, E., Suzuki, M., Ikeda, M., Huang, C. W., Lee, J. J., et al. (2019). APOE-MS4A genetic interactions are associated with executive dysfunction and network abnormality in clinically mild Alzheimer’s disease. Neuroimage Clin. 21:101621. doi: 10.1016/j.nicl.2018.101621
Chen, H., Wu, G., Jiang, Y., Feng, R., Liao, M., Zhang, L., et al. (2015). Analyzing 54,936 Samples Supports the Association Between CD2AP rs9349407 Polymorphism and Alzheimer’s Disease Susceptibility. Mol. Neurobiol. 52, 1–7. doi: 10.1007/s12035-014-8834-2
Chen, L. H., Kao, P. Y., Fan, Y. H., Ho, D. T., Chan, C. S., Yik, P. Y., et al. (2012). Polymorphisms of CR1, CLU and PICALM confer susceptibility of Alzheimer’s disease in a southern Chinese population. Neurobiol. Aging 33, 210.e1–210.e7. doi: 10.1016/j.neurobiolaging.2011.09.016
Chen, M. J., Ramesha, S., Weinstock, L. D., Gao, T., Ping, L., Xiao, H., et al. (2021). Extracellular signal-regulated kinase regulates microglial immune responses in Alzheimer’s disease. J. Neurosci. Res. 99, 1704–1721.
Chibnik, L. B., Shulman, J. M., Leurgans, S. E., Schneider, J. A., Wilson, R. S., Tran, D., et al. (2011). CR1 is associated with amyloid plaque burden and age-related cognitive decline. Ann. Neurol. 69, 560–569. doi: 10.1002/ana.22277
Colombo, M., Fernandez, T., Nakamura, K., and Gross, C. G. (1998). Functional differentiation along the anterior-posterior axis of the hippocampus in monkeys. J. Neurophysiol. 80, 1002–1005.
Corder, E. H., Saunders, A. M., Strittmatter, W. J., Schmechel, D. E., Gaskell, P. C., Small, G. W., et al. (1993). Gene dose of apolipoprotein E type 4 allele and the risk of Alzheimer’s disease in late onset families. Science 261, 921–923. doi: 10.1126/science.8346443
Cuingnet, R., Gerardin, E., Tessieras, J., Auzias, G., Lehericy, S., Habert, M. O., et al. (2011). Automatic classification of patients with Alzheimer’s disease from structural MRI: a comparison of ten methods using the ADNI database. Neuroimage 56, 766–781. doi: 10.1016/j.neuroimage.2010.06.013
Dos Santos, L. R., Pimassoni, L. H. S., Sena, G. G. S., Camporez, D., Belcavello, L., Trancozo, M., et al. (2017). Validating GWAS Variants from Microglial Genes Implicated in Alzheimer’s Disease. J. Mol. Neurosci. 62, 215–221.
Du, W., Tan, J., Xu, W., Chen, J., and Wang, L. (2016). Association between clusterin gene polymorphism rs11136000 and late-onset Alzheimer’s disease susceptibility: a review and meta-analysis of case-control studies. Exp. Ther. Med. 12, 2915–2927. doi: 10.3892/etm.2016.3734
Dubey, H., Gulati, K., and Ray, A. (2018). Recent studies on cellular and molecular mechanisms in Alzheimer’s disease: focus on epigenetic factors and histone deacetylase. Rev. Neurosci. 29, 241–260. doi: 10.1515/revneuro-2017-0049
Dubois, B., Feldman, H. H., Jacova, C., Hampel, H., Molinuevo, J. L., Blennow, K., et al. (2014). Advancing research diagnostic criteria for Alzheimer’s disease: the IWG-2 criteria. Lancet Neurol. 13, 614–629.
Farrer, L. A., Cupples, L. A., Haines, J. L., Hyman, B., Kukull, W. A., Mayeux, R., et al. (1997). Effects of age, sex, and ethnicity on the association between apolipoprotein E genotype and Alzheimer disease. A meta-analysis. APOE and Alzheimer Disease Meta Analysis Consortium. JAMA 278, 1349–1356. doi: 10.1001/jama.278.16.1349
Franzmeier, N., Rubinski, A., Neitzel, J., Ewers, M. and Alzheimer’s Disease Neuroimaging Initiative [ADNI] (2019). The BIN1 rs744373 SNP is associated with increased tau-PET levels and impaired memory. Nat. Commun. 10:1766. doi: 10.1038/s41467-019-09564-5
Frederickson, C. J., Klitenick, M. A., Manton, W. I., and Kirkpatrick, J. B. (1983). Cytoarchitectonic distribution of zinc in the hippocampus of man and the rat. Brain Res. 273, 335–339. doi: 10.1016/0006-8993(83)90858-2
Furusawa, K., Takasugi, T., Chiu, Y. W., Hori, Y., Tomita, T., Fukuda, M., et al. (2019). CD2-associated protein (CD2AP) overexpression accelerates amyloid precursor protein (APP) transfer from early endosomes to the lysosomal degradation pathway. J. Biol. Chem. 294, 10886–10899. doi: 10.1074/jbc.RA118.005385
Geekiyanage, H., and Chan, C. (2011). MicroRNA-137/181c regulates serine palmitoyltransferase and in turn amyloid beta, novel targets in sporadic Alzheimer’s disease. J. Neurosci. 31, 14820–14830. doi: 10.1523/JNEUROSCI.3883-11.2011
Hall, J. R., Wiechmann, A. R., Johnson, L. A., Edwards, M., Barber, R. C., Cunningham, R., et al. (2014). The Impact of APOE Status on Relationship of Biomarkers of Vascular Risk and Systemic Inflammation to Neuropsychiatric Symptoms in Alzheimer’s Disease. J. Alzheimers Dis. 40, 887–896. doi: 10.3233/JAD-131724
Hansson, O., Grothe, M. J., Strandberg, T. O., Ohlsson, T., Hagerstrom, D., Jogi, J., et al. (2017). Tau Pathology Distribution in Alzheimer’s disease Corresponds Differentially to Cognition-Relevant Functional Brain Networks. Front. Neurosci. 11:167. doi: 10.3389/fnins.2017.00167
Hohman, T. J., Koran, M. E., Thornton-Wells, T. A. and Alzheimer’s Neuroimaging Initiative (2014). Genetic variation modifies risk for neurodegeneration based on biomarker status. Front. Aging Neurosci. 6:183. doi: 10.3389/fnagi.2014.00183
Hollingworth, P., Harold, D., Sims, R., Gerrish, A., Lambert, J. C., Carrasquillo, M. M., et al. (2011). Common variants at ABCA7, MS4A6A/MS4A4E, EPHA1, CD33 and CD2AP are associated with Alzheimer’s disease. Nat. Genet. 43, 429–435. doi: 10.1038/ng.803
Huang, C. W., Chang, W. N., Huang, S. H., Lui, C. C., Chen, N. C., Chang, Y. T., et al. (2013). Impact of homocysteine on cortical perfusion and cognitive decline in mild Alzheimer’s dementia. Eur. J. Neurol. 20, 1191–1197. doi: 10.1111/ene.12159
Huang, C. W., Hsu, S. W., Tsai, S. J., Chen, N. C., Liu, M. E., Lee, C. C., et al. (2017). Genetic effect of interleukin-1 beta (C-511T) polymorphism on the structural covariance network and white matter integrity in Alzheimer’s disease. J. Neuroinflammation 14:12. doi: 10.1186/s12974-017-0791-z
Jack, C. R. Jr., Bennett, D. A., Blennow, K., Carrillo, M. C., Dunn, B., Haeberlein, S. B., et al. (2018). NIA-AA Research Framework: toward a biological definition of Alzheimer’s disease. Alzheimers Dement. 14, 535–562. doi: 10.1016/j.jalz.2018.02.018
Jansen, I. E., Savage, J. E., Watanabe, K., Bryois, J., Williams, D. M., Steinberg, S., et al. (2019). Genome-wide meta-analysis identifies new loci and functional pathways influencing Alzheimer’s disease risk. Nat. Genet. 51, 404–413.
Jiao, B., Liu, X., Zhou, L., Wang, M. H., Zhou, Y., Xiao, T., et al. (2015). Polygenic Analysis of Late-Onset Alzheimer’s Disease from Mainland China. PLoS One 10:e0144898. doi: 10.1371/journal.pone.0144898
Jin, C., Li, W., Yuan, J., Xu, W., and Cheng, Z. (2012). Association of the CR1 polymorphism with late-onset Alzheimer’s disease in Chinese Han populations: a meta-analysis. Neurosci. Lett. 527, 46–49. doi: 10.1016/j.neulet.2012.08.032
Jones, D. T., Graff-Radford, J., Lowe, V. J., Wiste, H. J., Gunter, J. L., Senjem, M. L., et al. (2017). Tau, amyloid, and cascading network failure across the Alzheimer’s disease spectrum. Cortex 97, 143–159. doi: 10.1016/j.cortex.2017.09.018
Karch, C. M., Jeng, A. T., Nowotny, P., Cady, J., Cruchaga, C., and Goate, A. M. (2012). Expression of novel Alzheimer’s disease risk genes in control and Alzheimer’s disease brains. PLoS One 7:e50976. doi: 10.1371/journal.pone.0050976
Keenan, B. T., Shulman, J. M., Chibnik, L. B., Raj, T., Tran, D., and Sabuncu, M. R. (2012). A coding variant in CR1 interacts with APOE-epsilon4 to influence cognitive decline. Hum. Mol. Genet. 21, 2377–2388. doi: 10.1093/hmg/dds054
Kimura, H., Tooyama, I., and McGeer, P. L. (1994). Acidic FGF expression in the surroundings of senile plaques. Tohoku J. Exp. Med. 174, 279–293. doi: 10.1620/tjem.174.279
Koistinaho, M., Lin, S., Wu, X., Esterman, M., Koger, D., Hanson, J., et al. (2004). Apolipoprotein E promotes astrocyte colocalization and degradation of deposited amyloid-beta peptides. Nat. Med. 10, 719–726. doi: 10.1038/nm1058
Lambert, J. C., Ibrahim-Verbaas, C. A., Harold, D., Naj, A. C., Sims, R., Bellenguez, C., et al. (2013). Meta-analysis of 74,046 individuals identifies 11 new susceptibility loci for Alzheimer’s disease. Nat. Genet. 45, 1452–1458. doi: 10.1038/ng.2802
Li, H. L., Shi, S. S., Guo, Q. H., Ni, W., Dong, Y., Liu, Y., et al. (2011). PICALM and CR1 variants are not associated with sporadic Alzheimer’s disease in Chinese patients. J. Alzheimers Dis. 25, 111–117.
Ma, F. C., Zong, Y., Wang, H. F., Li, J. Q., Cao, X. P., and Tan, L. (2018). ABCA7 genotype altered Abeta levels in cerebrospinal fluid in Alzheimer’s disease without dementia. Ann. Transl. Med. 6:437. doi: 10.21037/atm.2018.07.04
Mahley, R. W. (1988). Apolipoprotein E: cholesterol transport protein with expanding role in cell biology. Science 240, 622–630. doi: 10.1126/science.3283935
Martins, C. A., Oulhaj, A., de Jager, C. A., and Williams, J. H. (2005). APOE alleles predict the rate of cognitive decline in Alzheimer disease: a nonlinear model. Neurology 65, 1888–1893. doi: 10.1212/01.wnl.0000188871.74093.12
Martiskainen, H., Viswanathan, J., Nykanen, N. P., Kurki, M., Helisalmi, S., Natunen, T., et al. (2015). Transcriptomics and mechanistic elucidation of Alzheimer’s disease risk genes in the brain and in vitro models. Neurobiol. Aging 36, 1221.e15–28. doi: 10.1016/j.neurobiolaging.2014.09.003
McCormick, E. M., van Hoorn, J., Cohen, J. R., and Telzer, E. H. (2018). Functional connectivity in the social brain across childhood and adolescence. Soc. Cogn. Affect. Neurosci. 13, 819–830.
Naj, A. C., Jun, G., Beecham, G. W., Wang, L. S., Vardarajan, B. N., Buros, J., et al. (2011). Common variants at MS4A4/MS4A6E, CD2AP, CD33 and EPHA1 are associated with late-onset Alzheimer’s disease. Nat. Genet. 43, 436–441.
Olgiati, P., Politis, A. M., Papadimitriou, G. N., De Ronchi, D., and Serretti, A. (2011). Genetics of late-onset Alzheimer’s disease: update from the alzgene database and analysis of shared pathways. Int. J. Alzheimers Dis. 2011:832379. doi: 10.4061/2011/832379
Przeździk, I., Faber, M., Fernandez, G., Beckmann, C. F., and Haak, K. V. (2019). The functional organisation of the hippocampus along its long axis is gradual and predicts recollection. Cortex 119, 324–335. doi: 10.1016/j.cortex.2019.04.015
Ramos de Matos, M., Ferreira, C., Herukka, S. K., Soininen, H., Janeiro, A., Santana, I., et al. (2018). Quantitative Genetics Validates Previous Genetic Variants and Identifies Novel Genetic Players Influencing Alzheimer’s Disease Cerebrospinal Fluid Biomarkers. J. Alzheimers Dis. 66, 639–652. doi: 10.3233/JAD-180512
Ringland, C., Schweig, J. E., Paris, D., Shackleton, B., Lynch, C. E., Eisenbaum, M., et al. (2020). Apolipoprotein E isoforms differentially regulate matrix metallopeptidase 9 function in Alzheimer’s disease. Neurobiol. Aging 95, 56–68. doi: 10.1016/j.neurobiolaging.2020.06.018
Risacher, S. L., Kim, S., Shen, L., Nho, K., Foroud, T., Green, R. C., et al. (2013). The role of apolipoprotein E (APOE) genotype in early mild cognitive impairment (E-MCI). Front. Aging Neurosci. 5:11. doi: 10.3389/fnagi.2013.00011
Segall, J. M., Allen, E. A., Jung, R. E., Erhardt, E. B., Arja, S. K., Kiehl, K., et al. (2012). Correspondence between structure and function in the human brain at rest. Front. Neuroinform. 6:10. doi: 10.3389/fninf.2012.00010
Shen, N., Chen, B., Jiang, Y., Feng, R., Liao, M., Zhang, L., et al. (2015). An Updated Analysis with 85,939 Samples Confirms the Association Between CR1 rs6656401 Polymorphism and Alzheimer’s Disease. Mol. Neurobiol. 51, 1017–1023. doi: 10.1007/s12035-014-8761-2
Shi, Y., Yamada, K., Liddelow, S. A., Smith, S. T., Zhao, L., Luo, W., et al. (2017). ApoE4 markedly exacerbates tau-mediated neurodegeneration in a mouse model of tauopathy. Nature 549, 523–527. doi: 10.1038/nature24016
Shulman, J. M., Chen, K., Keenan, B. T., Chibnik, L. B., Fleisher, A., Thiyyagura, P., et al. (2013)., Genetic susceptibility for Alzheimer disease neuritic plaque pathology. JAMA Neurol. 70, 1150–1157.
Singh, P. P., Singh, M., and Mastana, S. S. (2006). APOE distribution in world populations with new data from India and the UK. Ann. Hum. Biol. 33, 279–308. doi: 10.1080/03014460600594513
Vogel, J. W., La Joie, R., Grothe, M. J., Diaz-Papkovich, A., Doyle, A., and Vachon-Presseau, E. (2020). A molecular gradient along the longitudinal axis of the human hippocampus informs large-scale behavioral systems. Nat. Commun. 11:960. doi: 10.1038/s41467-020-14518-3
Wetzel, M. K., Naska, S., Laliberte, C. L., Rymar, V. V., Fujitani, M., Biernaskie, J. A., et al. (2008). p73 regulates neurodegeneration and phospho-tau accumulation during aging and Alzheimer’s disease. Neuron 59, 708–721. doi: 10.1016/j.neuron.2008.07.021
Wisdom, N. M., Callahan, J. L., and Hawkins, K. A. (2011). The effects of apolipoprotein E on non-impaired cognitive functioning: a meta-analysis. Neurobiol. Aging 32, 63–74. doi: 10.1016/j.neurobiolaging.2009.02.003
Xiao, Q., Liu, Z. J., Tao, S., Sun, Y. M., Jiang, D., Li, H. L., et al. (2015). Risk prediction for sporadic Alzheimer’s disease using genetic risk score in the Han Chinese population. Oncotarget 6, 36955–36964. doi: 10.18632/oncotarget.6271
Yang, H., Guan, H., Yang, M., Liu, Z., Takeuchi, S., Yanagisawa, D., et al. (2015). Upregulation of mitochondrial ferritin by proinflammatory cytokines: implications for a role in Alzheimer’s disease. J. Alzheimers Dis. 45, 797–811. doi: 10.3233/JAD-142595
Zhang, Q., Yu, J. T., Zhu, Q. X., Zhang, W., Wu, Z. C., Miao, D., et al. (2010). Complement receptor 1 polymorphisms and risk of late-onset Alzheimer’s disease. Brain Res. 1348, 216–221. doi: 10.1016/j.brainres.2010.06.018
Zhao, Q. F., Wan, Y., Wang, H. F., Sun, F. R., Hao, X. K., Tan, M. S., et al. (2016). ABCA7 Genotypes Confer Alzheimer’s Disease Risk by Modulating Amyloid-beta Pathology. J. Alzheimers Dis. 52, 693–703. doi: 10.3233/JAD-151005
Zhao, Z., Sagare, A. P., Ma, Q., Halliday, M. R., Kong, P., Kisler, K., et al. (2015). Central role for PICALM in amyloid-beta blood-brain barrier transcytosis and clearance. Nat. Neurosci. 18, 978–987. doi: 10.1038/nn.4025
Keywords: Alzheimer’s disease, single nucleotide polymorphism, independent component analysis, apolipoprotein E4, structural covariance network, network integrity score
Citation: Chang H-I, Chang Y-T, Huang C-W, Huang K-L, Hsu J-L, Hsu S-W, Tsai S-J, Chang W-N, Lee C-C, Huang S-H and Chang C-C (2021) Structural Covariance Network as an Endophenotype in Alzheimer’s Disease-Susceptible Single-Nucleotide Polymorphisms and the Correlations With Cognitive Outcomes. Front. Aging Neurosci. 13:721217. doi: 10.3389/fnagi.2021.721217
Received: 06 June 2021; Accepted: 04 November 2021;
Published: 17 December 2021.
Edited by:
Jiehui Jiang, Shanghai University, ChinaReviewed by:
Kuen-Jer Tsai, National Cheng Kung University, TaiwanCopyright © 2021 Chang, Chang, Huang, Huang, Hsu, Hsu, Tsai, Chang, Lee, Huang and Chang. This is an open-access article distributed under the terms of the Creative Commons Attribution License (CC BY). The use, distribution or reproduction in other forums is permitted, provided the original author(s) and the copyright owner(s) are credited and that the original publication in this journal is cited, in accordance with accepted academic practice. No use, distribution or reproduction is permitted which does not comply with these terms.
*Correspondence: Chiung-Chih Chang, bmV1cjA5OUBhZG0uY2dtaC5vcmcudHc=
†These authors have contributed equally to this work
Disclaimer: All claims expressed in this article are solely those of the authors and do not necessarily represent those of their affiliated organizations, or those of the publisher, the editors and the reviewers. Any product that may be evaluated in this article or claim that may be made by its manufacturer is not guaranteed or endorsed by the publisher.
Research integrity at Frontiers
Learn more about the work of our research integrity team to safeguard the quality of each article we publish.