- 1Department of Radiology, The First Affiliated Hospital of Wenzhou Medical University, Wenzhou, China
- 2Department of Radiology, The Second Affiliated Hospital, Zhejiang University School of Medicine, Hangzhou, China
- 3Institute for Medical Imaging Technology, School of Biomedical Engineering, Shanghai Jiao Tong University, Shanghai, China
Background: Before the apparent cognitive decline, subjects on the course of Alzheimer's disease (AD) can have significantly altered spontaneous brain activity, which could be potentially used for early diagnosis. As previous studies investigating local brain activity may suffer from the problem of cortical signal aliasing during volume-based analysis, we aimed to investigate the cortical functional alterations in the AD continuum using a surface-based approach.
Methods: Based on biomarker profile “A/T,” we included 11 healthy controls (HC, A–T–), 22 preclinical AD (CU, A+T+), 33 prodromal AD (MCI, A+T+), and 20 AD with dementia (d-AD, A+T+) from the Alzheimer's Disease Neuroimaging Initiative (ADNI) database. The amplitude of low-frequency fluctuation (ALFF) method was used to evaluate the changes of spontaneous brain activity, which was performed in the classic frequency band (0.01–0.08 Hz), slow-4 (0.027–0.073 Hz) band, and slow-5 (0.01–0.027 Hz) band.
Results: Under classic frequency band and slow-4 band, analysis of covariance (ANCOVA) showed that there were significant differences of standardized ALFF (zALFF) in the left posterior cingulate cortex (PCC) among the four groups. The post-hoc analyses showed that under the classic frequency band, the AD group had significantly decreased zALFF compared with the other three groups, and the cognitively unimpaired (CU) group had decreased zALFF compared with the healthy control (HC) group. Under the slow-4 band, more group differences were detected (HC > CU/MCI > d-AD). The accuracy of classifying CU, mild cognitive impairment (MCI), and AD from HC by left PCC activity under the slow-4 band were 0.774, 0.744, and 0.920, respectively. Moreover, the zALFF values of the left PCC had significant correlations with cerebrospinal fluid (CSF) biomarkers and neuropsychological tests.
Conclusions: Spontaneous brain activity in the left PCC may decrease in preclinical AD when cognitive functions were relatively normal. The combination of a surfaced-based approach and specific frequency band analysis may increase sensitivity for the identification of preclinical AD subjects.
Introduction
Alzheimer's disease (AD) is a major neurodegenerative disease in elderly adults that causes memory decline, executive function impairment, and dementia (Masters et al., 2015). Due to the irreversible damage of neurons, early detection and intervention may be particularly significant to reduce the damage of AD. According to the biological definition in 2018 by National Institute on Aging and Alzheimer's Association (NIA-AA), individuals with both positive biomarker profiles “A/T” (A+T+) can be diagnosed as biological AD (Jack et al., 2018). Meanwhile, according to cognitive status, they could be further classified into three groups: preclinical AD (cognitively unimpaired, CU), prodromal AD (mild cognitive impairment, MCI), and AD with dementia (dementia, d-AD) (Jack et al., 2018), representing different stages of the disease. These criteria provide an important basis for exploring early brain manifestations of the disease and developing early imaging markers.
Based on resting-state functional magnetic resonance imaging (rsfMRI), the amplitude of low-frequency fluctuations (ALFF) is a relatively reliable and reproducible method to measure local spontaneous brain activities (Zang et al., 2007; Margulies et al., 2010; Zuo et al., 2010). It has been widely used to analyze the functional differences among patients with AD, MCI, and healthy subjects. Several studies have found gradually disordered inherent activity in the brain and abnormalities of low-frequency oscillations in many brain regions mainly located in the posterior cingulate cortex (PCC), medial prefrontal cortex (MPFC), temporal regions, and superior frontal regions, suggesting that ALFF might be a potentially useful tool for detecting AD-related brain alterations (Han et al., 2011; Wang et al., 2011; Xi et al., 2012; Liang et al., 2014). Additionally, while brain activities under classic frequency bands (0.01–0.08 Hz or 0.01–0.1 Hz) were well-investigated, the inherent patterns of brain activity are sensitive to specific frequency bands (Buzsaki and Draguhn, 2004; Yang et al., 2018). It has been demonstrated that rs-fMRI signals of cortex and cistern may have different power distribution characteristics in different frequency ranges (Zou et al., 2008). Therefore, few studies divided the classic frequency band into several sub-bands and found that subjects within the AD continuum had frequency-dependent brain alterations, suggesting the unique contribution of AD pathologies (Liu et al., 2020; Yang et al., 2020).
Currently, most neuroimaging studies rely on volume-based analysis to reveal the effect of disease on regional brain activities, and spatial smoothing is a necessary step to reduce the influence of noise and individual differences in brain morphology. Nevertheless, due to large smoothing kernels (6–10 mm) are usually needed, this step can lead to the aliasing of signals from the adjacent cortices with distinct functions, resulting in decreased spatial accuracy and statistical power (Figure 1). As the cortex is a large surface, it can be unfolded. By expanding the brain to the surface space, mutual contamination between neighboring but functionally distinct cortices can be avoided (Brodoehl et al., 2020). Therefore, this method has better sensitivity and more accurate spatial positioning than the traditional volume-based method in locating blood oxygenation level-dependent (BOLD) signal sources within the cortex (Andrade et al., 2001; Jo et al., 2007). Previously, there were few studies based on surface analysis because of computational difficulties (Oosterhof et al., 2011; Tucholka et al., 2012). With the enhancement of computer performance and improvement of the method, surface-based functional analysis becomes relatively practical.
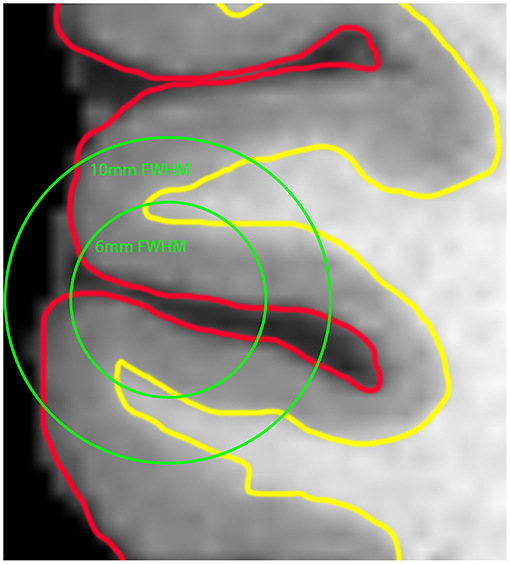
Figure 1. The red line represents the outermost gray matter (GM) of the brain, and the yellow line indicates the boundary between the GM and white matter (WM) of the brain. Using the full width at half maximum (FWHM) of 6 and 10 mm for voxel-based smoothing, it can be seen that the signals from GM located in different brain regions are more likely to be mixed with the increase of the smoothing kernel.
The study aimed to explore the differences of surface-based spontaneous brain activity patterns in different stages of patients with AD with common biomarkers. Based on the previous studies (Liang et al., 2014; Yang et al., 2018; Zeng et al., 2019), we hypothesized that: (1) patients with biologically defined AD might have abnormal brain activities within regions related to AD pathologies, such as MPFC and PCC. (2) These changes could represent different cognitive stages and are related to cognitive functions. (3) Compared to volume-based analysis, the surface-based approach could reveal more subtle brain alterations in the early stages of AD.
Materials and Methods
Subjects
We reviewed the Alzheimer's Disease Neuroimaging Initiative (ADNI) database and selected subjects with complete information, such as cerebrospinal fluid (CSF) biomarkers, 3D T1-weighted (T1w) images, rsfMRI images, and neuropsychological tests at the same time point. Based on the cutoff value of previous research on CSF biomarkers, the threshold of amyloid-β 1 to 42 peptide (Aβ1−42) is set at 192 ng/L, and the threshold of tau phosphorylated at the threonine 181 position (p-tau181p) is set at 23 ng/L (Shaw et al., 2009, 2011). After screening, the dataset contained 86 subjects, all from ADNI2.
According to the cutoff value and different cognitive stages, 11 CU individuals with the biomarker characteristic of “A–T–” were defined as healthy control (HC), 75 subjects with the biomarker characteristic of “A+T+” were further divided into three groups: 22 CU, 33 MCI, and 20 d-AD.
CSF Analyses
Cerebrospinal fluid data were downloaded from the ADNI database. Baseline CSF samples were collected in the morning after an overnight fast and then processed, Aβ1−42 and p-tau181p were measured subsequently as previously described (Shaw et al., 2009, 2011). In short, CSF was collected into collection tubes or syringes provided to each site, then, transferred into polypropylene transfer tubes within an hour after collection followed by frozen on dry ice, and transported overnight on dry ice to the ADNI Biomarker Core laboratory at the University of Pennsylvania Medical Center. These samples were thawed at room temperature (1 h) and gently mixed to prepare aliquots (0.5 ml). The aliquots were stored in bar code-labeled polypropylene vials at −80°C. Aβ1−42, t-tau, and p-tau181 were measured using the multiplex xMAP Luminex platform (Luminex Corp, Austin, TX, USA) with Innogenetics (INNO-BIA AlzBio3; Ghent, Belgium; for research use-only reagents) immunoassay kit-based reagents.
Imaging Acquisition
All participants underwent 3.0 T MR scans. Sequences were acquired as follows: (1) 3D T1w magnetization prepared rapid gradient echo (MPRAGE) sequence (acquisition plane = SAGITTAL; flip angle = 9.0 degree; Matrix X = 256.0 pixels; Matrix Y = 256.0 pixels; Matrix Z = 170.0; pixel spacing X = 1.0 mm; pixel spacing Y = 1.0 mm; slice thickness = 1.2 mm; TE = 3.1 ms; TI = 0.0 ms; TR = 6.8 ms); (2) rsfMRI echo-planar imaging sequence (flip angle = 80.0 degree; Matrix X = 64.0 pixels; Matrix Y = 64.0 pixels; pixel spacing X = 3.3 mm; pixel spacing Y = 3.3 mm; time points = 140; number of slices = 48; slice thickness = 3.3 mm; TE = 30.0 ms; TR = 3,000.0 ms).
Data Processing
Structural and functional MR images were processed using DPABISurf_V1.3 toolkit (http://rfmri.org/DPABISurf) and fMRIPrep 20.0.5 (https://fmriprep.org) (Esteban et al., 2019), which is based on Nipype 1.4.2 (https://github.com/nipy/nipype) (Gorgolewski et al., 2011).
Anatomical Data Preprocessing
The T1w image was corrected for intensity non-uniformity (INU) with N4BiasFieldCorrection (Tustison et al., 2010), distributed with ANTs 2.2.0 (Avants et al., 2008), and used as T1w-reference throughout the workflow. The T1w-reference was then skull-stripped with a Nipype implementation of the antsBrainExtraction.sh workflow (from ANTs), using OASIS30ANTs as the target template. Brain tissue segmentation of CSF, white matter (WM), and gray matter (GM) was performed on the brain-extracted T1w using fast (FSL 5.0.9) (Zhang et al., 2001). Brain surfaces were reconstructed using recon-all (FreeSurfer 6.0.1) (Dale et al., 1999), and the brain mask estimated previously was refined with a custom variation of the method to reconcile ANTs-derived and FreeSurfer-derived segmentation of the cortical gray matter of Mindboggle (Klein et al., 2017). Volume-based spatial normalization to one standard space (MNI152NLin2009cAsym) was performed through non-linear registration with antsRegistration (ANTs 2.2.0), using brain-extracted versions of both T1w reference and the T1w template. The following template was selected for spatial normalization: ICBM 152 Non-linear Asymmetrical template version 2009c (Fonov et al., 2009).
Functional Data Preprocessing
For the BOLD data of each subject, the following preprocessing was performed. The first 10 functional images volumes were discarded for the stabilization of the gradient magnetic field and the adaptation of the subjects need to take some time. A reference volume and its skull-stripped version were generated using a custom methodology of fMRIPrep. The BOLD reference was then co-registered to the T1w reference using bbregister (FreeSurfer) which implements boundary-based registration (Greve and Fischl, 2009). Head-motion parameters with respect to the BOLD reference (transformation matrices, and six corresponding rotation, and translation parameters) are estimated using mcflirt before any spatiotemporal filtering occurs (FSL 5.0.9) (Jenkinson et al., 2002). BOLD runs were slice-time corrected using 3dTshift from AFNI 20160207 (Cox and Hyde, 1997), and resampled onto the surface fsaverage5 for surface-based analysis (FreeSurfer reconstruction nomenclature) or onto the MNI152NLin2009cAsym space for volume-based analysis. Gridded (volumetric) resamplings were performed using antsApplyTransforms (ANTs), configured with Lanczos interpolation to minimize the smoothing effects of other kernels (Lanczos, 1964). Non-gridded (surface) resamplings were performed using mri_vol2surf (FreeSurfer). Automatic removal of motion artifacts using independent component analysis (ICA-AROMA) (Pruim et al., 2015) was performed on the preprocessed BOLD volumes. Several confounding time-series were calculated based on the preprocessed BOLD: framewise displacement (FD), DVARS (D referring to the temporal derivative of time courses, VARS referring to RMS variance over voxels), and three region-wise global signals (Power et al., 2012, 2014). Linear trends were then removed. The signals of the CSF, the WM, and the whole-brain were calculated using the CompCor (Behzadi et al., 2007) method and regressed out.
For more details of the pipeline, please refer the section corresponding to workflows in the documentation of fMRIPrep.
ALFF Analyses
The ALFFs analyses was based on the DPABISurf_V1.3 toolkit. The time series of each vertex on the surface or voxel in the 3d space was transformed into the frequency domain to obtain the power spectrum. The square root was then calculated at each frequency of the power spectrum and an average square root of 0.01–0.08 Hz at each vertex on the surface was obtained, considered as ALFF (Zang et al., 2007; Zou et al., 2008). The ALFF was then converted to a Z score by subtracting the global mean value and dividing it by the SD. Finally, we smoothed Z maps with a 10 mm full width at half maximum (FWHM) Gaussian kernel on the surface, or with a 6 mm FWHM Gaussian kernel on 3d volumes. Considering that dividing a classic frequency band into more precise sub-bands may better reflect specific brain activity changes, we further analyzed the frequency bands Slow-5 (0.01–0.027 Hz) and Slow-4 (0.027–0.073 Hz), which had been found related to GM neural oscillations and could detect changes in different cognitive stages (Zuo et al., 2010).
Statistical Analysis
For demographic and clinical data, ANOVA was used to compare the age, education, neuropsychological tests, and CSF biomarkers among four groups in SPSS 26.0. A chi-square test was employed for gender distribution difference assessment. The significance level was set at P < 0.05.
Analysis of covariance was used to find out brain activity changes among the four groups using the DPABISurf_V1.3 toolkit. Age, gender, education, and mean FD were taken as covariates. Permutation test with 5,000 permutations was used to find significant clusters, and multiple comparisons were corrected by the family-wise error rate (FWER) method with threshold-free cluster enhancement (TFCE) (Smith and Nichols, 2009). The statistical threshold was set to P < 0.025, as statistical analyses were performed in both hemispheres. For volume-based analysis, P < 0.05 was used as a threshold.
Clusters that showed significant group differences were saved as regions of interest (ROIs). We extracted standardized ALFF (zALFF) values from all subjects using these ROIs. Then pair-wise post-hoc comparisons were made in SPSS 26.0 and we marked out a group with a significant difference between the two groups. Bonferroni correction was used to correct the post-hoc comparisons (P < 0.05/6).
Furthermore, to investigate the classification performance of regional zALFF values, we made receiver operating characteristic (ROC) curves and calculated areas under the curve (AUCs). In summary, we separately measured the accuracy of discriminating HC from the other three groups (CU vs. HC, MCI vs. HC, and d-AD vs. HC). The accuracy, sensitivity, and specificity for each classifier were calculated.
Finally, correlation analyses were used to measure the relationships among zALFF values and neuropsychological scales as well as CSF biomarkers. Bonferroni correction was used to correct the correlation analyses (p < 0.05/9).
Results
Demographic and Clinical Characteristics
The demographic and clinical characteristics of the four groups are summarized in Table 1. Two CU, one MCI, and six d-AD were excluded for excessive head movement (head motion more than 3 mm or 3 degrees). In the current study, 11 HC, 20 CU, 32 MCI, and 14 d-AD subjects were finally enrolled. There was no significant difference in age, education, and mean FD among the four groups (P > 0.05). Gender, CSF biomarkers, and neuropsychological tests showed significant differences between at least two groups (Table 1). In general, the CSF pathological changes in MCI and d-AD groups were more obvious, and the neuropsychological scales of the d-AD group were significantly worse than the other three groups.
ALFF Analyses Under Three Frequency Bands
For surface-based analysis, ANCOVA analyses adjusting age, gender, education, and mean FD as covariates suggested that there were significant differences in spontaneous brain activity of left PCC among four groups under classic frequency band and slow-4 band. Figure 2 shows the brain regions where there are significant differences in brain spontaneous activity among the four groups under different frequency bands. Moreover, we added cluster size, MNI coordinates, peak value, and specific areas of the cerebral cortex [Human Connectome Project Multi-Modal Parcellation, HCP-MMP (Glasser et al., 2016)] to provide more detailed information (Table 2).
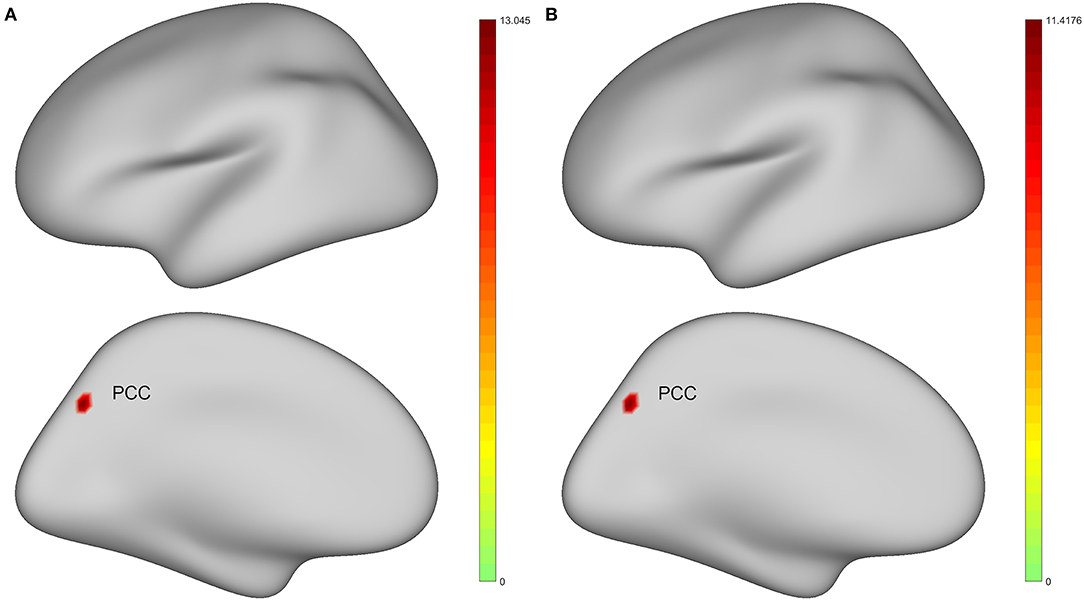
Figure 2. Brain regions with statistically significant differences in the standard amplitude of low-frequency fluctuations (zALFF) among healthy controls (HC), preclinical AD (CU), prodromal AD (MCI), and AD with dementia (d-AD). The results were obtained by analysis of covariance (ANCOVA) analysis after taking age, gender, education, and the mean framewise displacement (FD) as covariance [P < 0.025, permutation test number of 5,000, threshold-free cluster enhancement (TFCE), and family-wise error rate (FWER) correction]. (A) Under classic frequency band; (B) Under slow-4 band. Warmer color represents a more significant difference in statistical analysis. PCC, posterior cingulate cortex.

Table 2. Analysis of covariance (ANCOVA) results with age, gender, education, and the mean FD as covariates across healthy controls (HC), preclinical AD (CU), prodromal AD (MCI), and AD with dementia (d-AD).
The post-hoc analyses found that after Bonferroni correction, the zALFF values of the left PCC in the d-AD group were significantly decreased compared with the other three groups under the classic frequency band, and the CU group had decreased zALFF compared with the HC group (Table 3). In the slow-4 band, there were significant differences among the other two groups except for that non-significant difference between the CU group and MCI group.

Table 3. The standard amplitude of low-frequency fluctuations (zALFF) values extracted from the left posterior cingulate cortex (PCC) in each group under classic frequency band and Slow-4 band (controlled for age, gender, education, and the mean FD).
As for slow-5 band and volume-based analysis, there was no significant difference among the four groups.
Classification
The classification performance and ROC curves are depicted in Figure 3. The AUC values for the classification of CU vs. HC, MCI vs. HC, and d-AD vs. HC with classic frequency band features were 0.727, 0.722, and 0.961, respectively, and with slow-4 band features were 0.773, 0.733, and 0.955, respectively. Meanwhile, the accuracy for the classification of CU vs. HC, MCI vs. HC, and d-AD vs. HC with classic frequency band features were 0.710, 0.791, and 0.920, respectively, and with slow-4 band features were 0.774, 0.744, and 0.920, respectively.
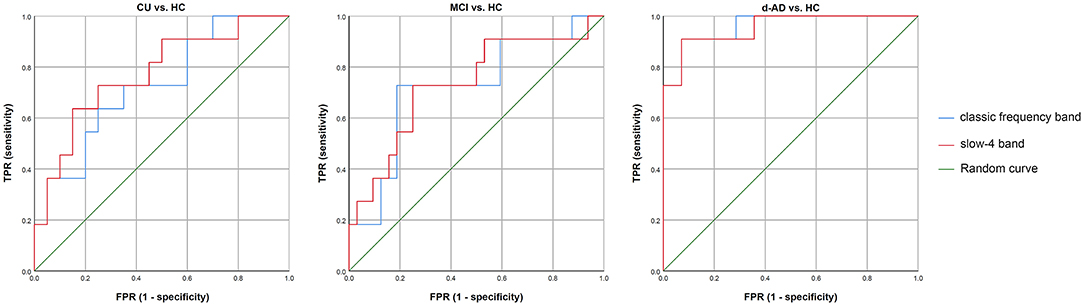
Figure 3. The classification performance and receiver operating characteristic (ROC) curves of CU vs. HC, MCI vs. HC, and d-AD vs. HC under classic frequency band and slow-4 band. HC, healthy control; CU, cognitively unimpaired; MCI, mild cognitive impairment; AD, Alzheimer's disease; d-AD, AD with dementia.
Relationships With CSF Biomarkers and Neuropsychological Tests
We correlated zALFF values of the left PCC with CSF biomarkers and neuropsychological scales (Table 4). Under both classic frequency band and slow-4 band, the zALFF values of the left PCC had significant positive correlations with Aβ1−42, Mini-mental State Examination (MMSE) total score, word immediate recall score, word delayed recall score, and Boston Naming test (BNT) score, and significant negative correlation with p-tau181p, total tau (t-tau), Trail Making Test A (TMT-A), and Trail Making Test B (TMT-B).
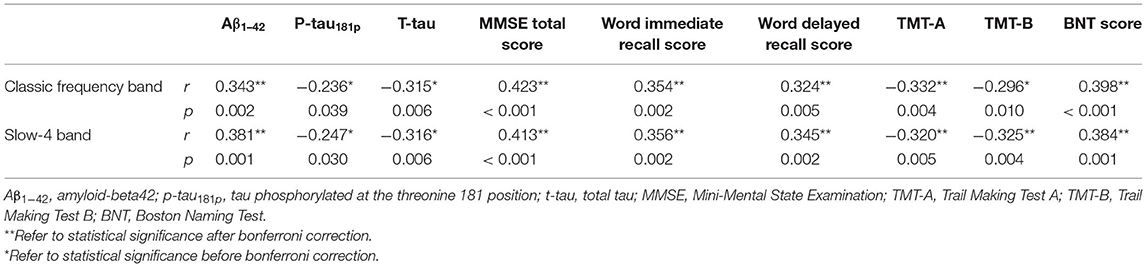
Table 4. Correlation between zALFF values of the left PCC with cerebrospinal fluid (CSF) biomarkers and neuropsychological scales.
Discussion
In this study, we used the surface-based approach and ALFF method to investigate spontaneous brain activity alterations in biological AD subjects. We found significantly different zALFF values in the left PCC region among the four groups, showing a decreasing gradient along with disease severity. Notably, CU subjects already had altered PCC activities, suggesting a very early effect of AD pathologies. Moreover, CSF biomarkers and neuropsychological tests showed significant correlations with zALFF values. Therefore, zALFF values of the left PCC may be a potential imaging marker for the early diagnosis of AD. Since there were no significant findings by the volume-based method, we suggest that a surface-based approach may increase the ability to detect such cortical function alteration.
We found significant differences in spontaneous brain activity of the left PCC region among the four groups. Further, post-hoc analyses showed that in the AD group the zALFF values of the left PCC decreased significantly compared with the other three groups, which is associated with CSF biomarkers and neuropsychological assessments, such as memory, language, and executive functions. PCC is the central part of the default mode network (DMN) associated with episodic memory retrieval. Moreover, it connects different subsystems of the DMN (Wagner et al., 2005; Buckner et al., 2008), which is a group of brain regions that support brain activity during resting state (Raichle et al., 2001) and plays a role in spontaneous cognition and functional balance with other brain systems (Raichle, 2015). As previous studies reported, cortical thinning, functional connectivity (FC) declining, glucose hypometabolism, and pathology aggregation in DMN areas have been observed in AD and MCI subjects or individuals at high risk of AD (Braak and Braak, 1991; Price and Morris, 1999; Dickerson et al., 2009; Gili et al., 2011; Brier et al., 2012; Hafkemeijer et al., 2012; Liguori et al., 2016; Lu et al., 2017). By using amyloid positron emission tomography (PET), early amyloid deposition in PCC and other regions in DMN have been detected in AD, MCI, and even at presymptomatic stages (Buckner et al., 2008; Johnson et al., 2014; Leech and Sharp, 2014). Meanwhile, most of the tau-dependent brain networks overlapped with ventral and dorsal DMN regions (Hoenig et al., 2018). The results corroborated previous findings of abnormal DMN in AD and its association with cognitive impairments.
It seems that the function of the left PCC is progressively impaired during the AD continuum and may serve as a stable imaging marker for monitoring the development of AD. Wang et al. (2011) reported that PCC showed the most significant ALFF difference among the AD, MCI, and healthy elderly groups. While ALFF values decreased in both AD and MCI groups, and the difference was only significant between patients with AD and healthy elderly (Wang et al., 2011). Similarly, Liang et al. (2014) found PCC had significant differences in ALFF values, and the trend of ALFF values was AD < late MCI < early MCI < NC. Most encouragingly, with a surface-based approach, in this study, we additionally found that ALFF values of left PCC were decreased in the CU group when the subjects had not shown any cognitive deficits. Further, using this index to classify CU and HC, the accuracy was 0.710. This may indicate that damage to the PCC began very early, possibly due to Aβ deposition (Johnson et al., 2014), and PCC ALFF values may have great potentials for early diagnosis. Notably, the volume-based analysis showed no significant difference between the four groups using the same statistical threshold. As previously proposed, the surface-based method would have better repeatability and sensibility than the volume-based approach. Also, it can provide additional guarantees for more spatially specific clusters (Oosterhof et al., 2011; Tucholka et al., 2012). Thus, we suggest that a surface-based approach would be beneficial for the detection of early cortical function alterations in AD.
It is worth noting that we only found changes in the left but no right PCC. In previous works of literature, results of laterality were indeed more common. The most reliable and sturdy metabolic changes for predicting conversion from aMCI to AD were hypometabolism in the left PCC/precuneus (Ma et al., 2018). In brain 18F-fluorodeoxyglucose (FDG) PET, a considerable number of patients with AD showed hemispheric asymmetries of hypometabolism (Murayama et al., 2016). Neuropathological studies have also shown that the left hemisphere was more likely to suffer from AD-related neurodegeneration than the right hemisphere (Janke et al., 2001). Also, in other fields, researchers have found that in primary progressive aphasia patients, atrophy, neuron loss, and disease-specific proteinopathy were more severe in the language-dominated hemispheres (Mesulam et al., 2014). Concerning most of the subjects who were right-handed, it is possible that increased intensity and frequency of usage of the left hemisphere resulted in faster AD-related neurodegeneration.
Among the three frequency bands, we found similar results in the classic frequency band and Slow-4 band but not the Slow-5 band. The previous studies on these three bands of AD have shown that the test-retest reliability of Slow-4 was greater and more widely distributed than that of Slow-5. In amnestic MCI, ALFF values in the left hippocampus and PCC were significantly reduced under the slow-4 band (Zhao et al., 2015). Compared with HC, ALFF values in the right putamen of MCI decreased in the slow-4 band (Ren et al., 2016). The Slow-4 band may be more sensitive to changes in AD pathologies. Furthermore, compared with the classic frequency band, zALFF values in the Slow-4 band additionally showed a statistical difference between the HC and MCI groups, suggesting that ALFF analysis within this frequency band could be more stable.
The current study is subject to limitations. First, the sample size was relatively small, and all the members of the control group were women. Although ADNI is the largest AD database and contains over a thousand samples, subjects with both rsfMRI scan and CSF biomarkers in the ADNI2 dataset are not common. Nevertheless, we found consistent changes in the left PCC, suggesting the robustness of the current method. Second, the research only focused on cross-sectional analysis, a longitudinal study is needed to fully demonstrate the progressive impairment of PCC function. Third, we only used one approach (ALFF) to find imaging markers. Future studies combining multi-parametric maps and multi-voxel pattern analysis methods may produce increased sensitivity for recognizing preclinical AD (Premi et al., 2016). Finally, we had not included PET biomarkers due to the scarcity of tau PET data. As CSF biomarkers could only reflect the pathological state but not pathological deposition in specific regions (Jack et al., 2018), further studies are needed for understanding the relationship between local brain activity changes and regional pathological accumulation.
In conclusion, we used a surface-based approach combined with specific frequency band analysis to explore the alterations of spontaneous brain activity in different stages of biologically defined patients with AD. We found consistently decreased ALFF in the left PCC of biological AD, even in preclinical AD when cognitive functions were relatively normal. It is the potential to be an early imaging marker for AD diagnosis and disease progression monitoring. Furthermore, with the continuous development of automatic and fast analytical methods, easier clinical access to this marker could be expected.
Data Availability Statement
The original contributions presented in the study are included in the article/supplementary material, further inquiries can be directed to the corresponding author/s.
Ethics Statement
The studies involving human participants were reviewed and approved by Alzheimer's Disease Neuroimaging Initiative. The patients/participants provided their written informed consent to participate in this study.
Author Contributions
PH, YY, and ZZ designed the study. ZZ and QZ collected clinical and MRI data. ZZ and LK analyzed the MRI data and wrote the protocol. QZ, XL, KL, XX, MZ, YY, and PH assisted with the organization of results and interpretation of findings. ZZ wrote the first draft of the manuscript. PH revised the manuscript. All authors contributed to the article and approved the submitted version.
Funding
This study was supported by the 13th Five-year Plan for National Key Research and Development Program of China (Grant No. 2016YFC1306600), the National Natural Science Foundation of China (Grant Nos. 81771820, 81571654, 81701647, 81901707, and 82001766), and the Natural Science Foundation of Zhejiang Province (Grant Nos. LSZ19H180001 and LQ20H180015), the Health Foundation for Creative Talents in Zhejiang Province, China (No: 2016), the Project Foundation for the College Young and Middle-aged Academic Leader of Zhejiang Province, China (No: 2017), and the Zhejiang province Postdoctoral Science Foundation. Data collection and sharing for this project were funded by the Alzheimer's Disease Neuroimaging Initiative (ADNI) (National Institutes of Health Grant U01 AG024904) and DOD ADNI (Department of Defense award number W81XWH-12-2-0012). ADNI was funded by the National Institute on Aging, the National Institute of Biomedical Imaging and Bioengineering, and through generous contributions from the following: AbbVie, Alzheimer's Association; Alzheimer's Drug Discovery Foundation; Araclon Biotech; BioClinica, Inc.; Biogen; Bristol-Myers Squibb Company; CereSpir, Inc.; Cogstate; Eisai Inc.; Elan Pharmaceuticals, Inc.; Eli Lilly and Company; EuroImmun; F. Hoffmann-La Roche Ltd and its affiliated company Genentech, Inc.; Fujirebio; GE Healthcare; IXICO Ltd.; Janssen Alzheimer Immunotherapy Research & Development, LLC.; Johnson & Johnson Pharmaceutical Research & Development LLC.; Lumosity; Lundbeck; Merck & Co., Inc.; Meso Scale Diagnostics, LLC.; NeuroRx Research; Neurotrack Technologies; Novartis Pharmaceuticals Corporation; Pfizer Inc.; Piramal Imaging; Servier; Takeda Pharmaceutical Company; and Transition Therapeutics. The Canadian Institutes of Health Research is providing funds to support ADNI clinical sites in Canada. Private sector contributions are facilitated by the Foundation for the National Institutes of Health (www.fnih.org). The grantee organization is the Northern California Institute for Research and Education, and the study is coordinated by the Alzheimer's Therapeutic Research Institute at the University of Southern California. ADNI data are disseminated by the Laboratory for Neuro Imaging at the University of Southern California.
Conflict of Interest
The authors declare that the research was conducted in the absence of any commercial or financial relationships that could be construed as a potential conflict of interest.
Publisher's Note
All claims expressed in this article are solely those of the authors and do not necessarily represent those of their affiliated organizations, or those of the publisher, the editors and the reviewers. Any product that may be evaluated in this article, or claim that may be made by its manufacturer, is not guaranteed or endorsed by the publisher.
Acknowledgments
We would like to thank all the subjects for participating in this research.
References
Andrade, A., Kherif, F., Mangin, J. F., Worsley, K. J., Paradis, A. L., Simon, O., et al. (2001). Detection of fMRI activation using cortical surface mapping. Hum. Brain Mapp. 12, 79–93. doi: 10.1002/1097-0193(200102)12:2<79::AID-HBM1005>3.0.CO;2-I
Avants, B. B., Epstein, C. L., Grossman, M., and Gee, J. C. (2008). Symmetric diffeomorphic image registration with cross-correlation: evaluating automated labeling of elderly and neurodegenerative brain. Med. Image Anal. 12, 26–41. doi: 10.1016/j.media.2007.06.004
Behzadi, Y., Restom, K., Liau, J., and Liu, T. T. (2007). A component based noise correction method (CompCor) for BOLD and perfusion based fMRI. Neuroimage 37, 90–101. doi: 10.1016/j.neuroimage.2007.04.042
Braak, H., and Braak, E. (1991). Neuropathological stageing of Alzheimer-related changes. Acta Neuropathol. 82, 239–259. doi: 10.1007/BF00308809
Brier, M. R., Thomas, J. B., Snyder, A. Z., Benzinger, T. L., Zhang, D., Raichle, M. E., et al. (2012). Loss of intranetwork and internetwork resting state functional connections with Alzheimer's disease progression. J. Neurosci. 32, 8890–8899. doi: 10.1523/JNEUROSCI.5698-11.2012
Brodoehl, S., Gaser, C., Dahnke, R., Witte, O. W., and Klingner, C. M. (2020). Surface-based analysis increases the specificity of cortical activation patterns and connectivity results. Sci. Rep. 10:5737. doi: 10.1038/s41598-020-62832-z
Buckner, R. L., Andrews-Hanna, J. R., and Schacter, D. L. (2008). The brain's default network: anatomy, function, and relevance to disease. Ann. N. Y. Acad. Sci. U. S. A. 1124, 1–38. doi: 10.1196/annals.1440.011
Buzsaki, G., and Draguhn, A. (2004). Neuronal oscillations in cortical networks. Science 304, 1926–1929. doi: 10.1126/science.1099745
Cox, R. W., and Hyde, J. S. (1997). Software tools for analysis and visualization of fMRI data. NMR Biomed. 10, 171–78 doi: 10.1002/(SICI)1099-1492(199706/08)10:4/5<171::AID-NBM453>3.0.CO;2-L
Dale, A. M., Fischl, B., and Sereno, M. I. (1999). Cortical surface-based analysis. I. Segmentation and surface reconstruction. Neuroimage 9, 179–194. doi: 10.1006/nimg.1998.0395
Dickerson, B. C., Bakkour, A., Salat, D. H., Feczko, E., Pacheco, J., Greve, D. N., et al. (2009). The cortical signature of Alzheimer's disease: regionally specific cortical thinning relates to symptom severity in very mild to mild AD dementia and is detectable in asymptomatic amyloid-positive individuals. Cereb. Cortex 19, 497–510. doi: 10.1093/cercor/bhn113
Esteban, O., Markiewicz, C. J., Blair, R. W., Moodie, C. A., Isik, A. I., Erramuzpe, A., et al. (2019). fMRIPrep: a robust preprocessing pipeline for functional MRI. Nat. Methods 16, 111–116. doi: 10.1038/s41592-018-0235-4
Fonov, V. S., Evans, A. C., Mckinstry, R. C., Almli, C. R., and Collins, D. L. (2009). Unbiased nonlinear average age-appropriate brain templates from birth to adulthood. NeuroImage 47, S102–S102. doi: 10.1016/S1053-8119(09)70884-5
Gili, T., Cercignani, M., Serra, L., Perri, R., Giove, F., Maraviglia, B., et al. (2011). Regional brain atrophy and functional disconnection across Alzheimer's disease evolution. J. Neurol. Neurosurg. Psychiatry 82, 58–66. doi: 10.1136/jnnp.2009.199935
Glasser, M. F., Coalson, T. S., Robinson, E. C., Hacker, C. D., Harwell, J., Yacoub, E., et al. (2016). A multi-modal parcellation of human cerebral cortex. Nature 536, 171–178. doi: 10.1038/nature18933
Gorgolewski, K., Burns, C. D., Madison, C., Clark, D., Halchenko, Y. O., Waskom, M. L., et al. (2011). Nipype: a flexible, lightweight and extensible neuroimaging data processing framework in python. Front. Neuroinform. 5:13. doi: 10.3389/fninf.2011.00013
Greve, D. N., and Fischl, B. (2009). Accurate and robust brain image alignment using boundary-based registration. Neuroimage 48, 63–72. doi: 10.1016/j.neuroimage.2009.06.060
Hafkemeijer, A., van der Grond, J., and Rombouts, S. A. (2012). Imaging the default mode network in aging and dementia. Biochim. Biophys. Acta 1822, 431–441. doi: 10.1016/j.bbadis.2011.07.008
Han, Y., Wang, J., Zhao, Z., Min, B., Lu, J., Li, K., et al. (2011). Frequency-dependent changes in the amplitude of low-frequency fluctuations in amnestic mild cognitive impairment: a resting-state fMRI study. Neuroimage 55, 287–295. doi: 10.1016/j.neuroimage.2010.11.059
Hoenig, M. C., Bischof, G. N., Seemiller, J., Hammes, J., Kukolja, J., Onur, O. A., et al. (2018). Networks of tau distribution in Alzheimer's disease. Brain 141, 568–581. doi: 10.1093/brain/awx353
Jack, C. R. Jr., Bennett, D. A., Blennow, K., Carrillo, M. C., Dunn, B., Haeberlein, S. B., et al. (2018). NIA-AA research framework: toward a biological definition of Alzheimer's disease. Alzheimers Dement. 14, 535–562. doi: 10.1016/j.jalz.2018.02.018
Janke, A. L., de Zubicaray, G., Rose, S. E., Griffin, M., Chalk, J. B., and Galloway, G. J. (2001). 4D deformation modeling of cortical disease progression in Alzheimer's dementia. Magn. Reson. Med. 46, 661–666. doi: 10.1002/mrm.1243
Jenkinson, M., Bannister, P., Brady, M., and Smith, S. (2002). Improved optimization for the robust and accurate linear registration and motion correction of brain images. NeuroImage 17, 825–841. doi: 10.1006/nimg.2002.1132
Jo, H. J., Lee, J. M., Kim, J. H., Shin, Y. W., Kim, I. Y., Kwon, J. S., et al. (2007). Spatial accuracy of fMRI activation influenced by volume- and surface-based spatial smoothing techniques. Neuroimage 34, 550–564. doi: 10.1016/j.neuroimage.2006.09.047
Johnson, S. C., Christian, B. T., Okonkwo, O. C., Oh, J. M., Harding, S., Xu, G., et al. (2014). Amyloid burden and neural function in people at risk for Alzheimer's Disease. Neurobiol. Aging 35, 576–584. doi: 10.1016/j.neurobiolaging.2013.09.028
Klein, A., Ghosh, S. S., Bao, F. S., Giard, J., Hame, Y., Stavsky, E., et al. (2017). Mindboggling morphometry of human brains. PLoS Comput. Biol. 13:e1005350. doi: 10.1371/journal.pcbi.1005350
Lanczos, C. (1964). Evaluation of noisy data. J. Soc. Indust. Appl. Math. Ser. B Num. Anal. 1, 76–85. doi: 10.1137/0701007
Leech, R., and Sharp, D. J. (2014). The role of the posterior cingulate cortex in cognition and disease. Brain 137 (Pt 1), 12–32. doi: 10.1093/brain/awt162
Liang, P., Xiang, J., Liang, H., Qi, Z., Li, K., and Alzheimer's Disease NeuroImaging Initiative. (2014). Altered amplitude of low-frequency fluctuations in early and late mild cognitive impairment and Alzheimer's disease. Curr. Alzheimer Res. 11, 389–398. doi: 10.2174/1567205011666140331225335
Liguori, C., Chiaravalloti, A., Sancesario, G., Stefani, A., Sancesario, G. M., Mercuri, N. B., et al. (2016). Cerebrospinal fluid lactate levels and brain [18F]FDG PET hypometabolism within the default mode network in Alzheimer's disease. Eur. J. Nucl. Med. Mol. Imaging 43, 2040–2049. doi: 10.1007/s00259-016-3417-2
Liu, P., Jia, X. Z., Chen, Y., Yu, Y., Zhang, K., Lin, Y. J., et al. (2020). Gut microbiota interacts with intrinsic brain activity of patients with amnestic mild cognitive impairment. CNS Neurosci. Ther. 27, 163–73 doi: 10.1111/cns.13451
Lu, H., Ma, S. L., Wong, S. W., Tam, C. W., Cheng, S. T., Chan, S. S., et al. (2017). Aberrant interhemispheric functional connectivity within default mode network and its relationships with neurocognitive features in cognitively normal APOE epsilon 4 elderly carriers. Int. Psychogeriatr. 29, 805–814. doi: 10.1017/S1041610216002477
Ma, H. R., Sheng, L. Q., Pan, P. L., Wang, G. D., Luo, R., Shi, H. C., et al. (2018). Cerebral glucose metabolic prediction from amnestic mild cognitive impairment to Alzheimer's dementia: a meta-analysis. Transl. Neurodegener. 7:9. doi: 10.1186/s40035-018-0114-z
Margulies, D. S., Bottger, J., Long, X., Lv, Y., Kelly, C., Schafer, A., et al. (2010). Resting developments: a review of fMRI post-processing methodologies for spontaneous brain activity. MAGMA 23, 289–307. doi: 10.1007/s10334-010-0228-5
Masters, C. L., Bateman, R., Blennow, K., Rowe, C. C., Sperling, R. A., and Cummings, J. L. (2015). Alzheimer's disease. Nat. Rev. Dis. Prim. 1:15056. doi: 10.1038/nrdp.2015.56
Mesulam, M. M., Weintraub, S., Rogalski, E. J., Wieneke, C., Geula, C., and Bigio, E. H. (2014). Asymmetry and heterogeneity of Alzheimer's and frontotemporal pathology in primary progressive aphasia. Brain 137 (Pt 4), 1176–1192. doi: 10.1093/brain/awu024
Murayama, N., Ota, K., Kasanuki, K., Kondo, D., Fujishiro, H., Fukase, Y., et al. (2016). Cognitive dysfunction in patients with very mild Alzheimer's disease and amnestic mild cognitive impairment showing hemispheric asymmetries of hypometabolism on (1)(8)F-FDG PET. Int. J. Geriatr. Psychiatry 31, 41–48. doi: 10.1002/gps.4287
Oosterhof, N. N., Wiestler, T., Downing, P. E., and Diedrichsen, J. (2011). A comparison of volume-based and surface-based multi-voxel pattern analysis. Neuroimage 56, 593–600. doi: 10.1016/j.neuroimage.2010.04.270
Power, J. D., Barnes, K. A., Snyder, A. Z., Schlaggar, B. L., and Petersen, S. E. (2012). Spurious but systematic correlations in functional connectivity MRI networks arise from subject motion. Neuroimage 59, 2142–2154. doi: 10.1016/j.neuroimage.2011.10.018
Power, J. D., Mitra, A., Laumann, T. O., Snyder, A. Z., Schlaggar, B. L., and Petersen, S. E. (2014). Methods to detect, characterize, and remove motion artifact in resting state fMRI. Neuroimage 84, 320–341. doi: 10.1016/j.neuroimage.2013.08.048
Premi, E., Cauda, F., Costa, T., Diano, M., Gazzina, S., Gualeni, V., et al. (2016). Looking for neuroimaging markers in frontotemporal lobar degeneration clinical trials: a multi-voxel pattern analysis study in granulin disease. J. Alzheimers Dis. 51, 249–262. doi: 10.3233/JAD-150340
Price, J. L., and Morris, J. C. (1999). Tangles and plaques in nondemented aging and “preclinical” Alzheimer's disease. Ann. Neurol. 45, 358–368. doi: 10.1002/1531-8249(199903)45:3<358::AID-ANA12>3.0.CO;2-X
Pruim, R. H.R., Mennes, M., van Rooij, D., Llera, A., Buitelaar, J. K., and Beckmann, C. F. (2015). ICA-AROMA: A robust ICA-based strategy for removing motion artifacts from fMRI data. Neuroimage 112, 267–277. doi: 10.1016/j.neuroimage.2015.02.064
Raichle, M. E. (2015). The brain's default mode network. Annu. Rev. Neurosci. 38, 433–447. doi: 10.1146/annurev-neuro-071013-014030
Raichle, M. E., MacLeod, A. M., Snyder, A. Z., Powers, W. J., Gusnard, D. A., and Shulman, G. L. (2001). A default mode of brain function. Proc. Natl. Acad. Sci. U. S. A. 98, 676–682. doi: 10.1073/pnas.98.2.676
Ren, P., Lo, R. Y., Chapman, B. P., Mapstone, M., Porsteinsson, A., Lin, F., et al. (2016). Longitudinal alteration of intrinsic brain activity in the striatum in mild cognitive impairment. J. Alzheimers Dis. 54, 69–78. doi: 10.3233/JAD-160368
Shaw, L. M., Vanderstichele, H., Knapik-Czajka, M., Clark, C. M., Aisen, P. S., Petersen, R. C., et al. (2009). Cerebrospinal fluid biomarker signature in Alzheimer's disease neuroimaging initiative subjects. Ann. Neurol. 65, 403–413. doi: 10.1002/ana.21610
Shaw, L. M., Vanderstichele, H., Knapik-Czajka, M., Figurski, M., Coart, E., Blennow, K., et al. (2011). Qualification of the analytical and clinical performance of CSF biomarker analyses in ADNI. Acta. Neuropathol. 121, 597–609. doi: 10.1007/s00401-011-0808-0
Smith, S. M., and Nichols, T. E. (2009). Threshold-free cluster enhancement: addressing problems of smoothing, threshold dependence and localisation in cluster inference. Neuroimage 44, 83–98. doi: 10.1016/j.neuroimage.2008.03.061
Tucholka, A., Fritsch, V., Poline, J. B., and Thirion, B. (2012). An empirical comparison of surface-based and volume-based group studies in neuroimaging. Neuroimage 63, 1443–1453. doi: 10.1016/j.neuroimage.2012.06.019
Tustison, N. J., Avants, B. B., Cook, P. A., Zheng, Y., Egan, A., Yushkevich, P. A., et al. (2010). N4ITK: improved N3 bias correction. IEEE Trans. Med. Imaging 29, 1310–1320. doi: 10.1109/TMI.2010.2046908
Wagner, A. D., Shannon, B. J., Kahn, I., and Buckner, R. L. (2005). Parietal lobe contributions to episodic memory retrieval. Trends Cogn. Sci. 9, 445–453. doi: 10.1016/j.tics.2005.07.001
Wang, Z., Yan, C., Zhao, C., Qi, Z., Zhou, W., Lu, J., et al. (2011). Spatial patterns of intrinsic brain activity in mild cognitive impairment and Alzheimer's disease: a resting-state functional MRI study. Hum. Brain Mapp. 32, 1720–1740. doi: 10.1002/hbm.21140
Xi, Q., Zhao, X. H., Wang, P. J., Guo, Q. H., Yan, C. G., and He, Y. (2012). Functional MRI study of mild Alzheimer's disease using amplitude of low frequency fluctuation analysis. Chin. Med. J. 125, 858–862.
Yang, L., Yan, Y., Li, Y., Hu, X., Lu, J., Chan, P., et al. (2020). Frequency-dependent changes in fractional amplitude of low-frequency oscillations in Alzheimer's disease: a resting-state fMRI study. Brain Imaging Behav. 14, 2187–2201. doi: 10.1007/s11682-019-00169-6
Yang, L., Yan, Y., Wang, Y., Hu, X., Lu, J., Chan, P., et al. (2018). Gradual disturbances of the amplitude of low-frequency fluctuations (ALFF) and fractional ALFF in Alzheimer spectrum. Front. Neurosci. 12:975. doi: 10.3389/fnins.2018.00975
Zang, Y. F., He, Y., Zhu, C. Z., Cao, Q. J., Sui, M. Q., Liang, M., et al. (2007). Altered baseline brain activity in children with ADHD revealed by resting-state functional MRI. Brain Dev. 29, 83–91. doi: 10.1016/j.braindev.2006.07.002
Zeng, Q., Luo, X., Li, K., Wang, S., Zhang, R., Hong, H., et al. (2019). Distinct spontaneous brain activity patterns in different biologically-defined alzheimer's disease cognitive stage: a preliminary study. Front. Aging Neurosci. 11:350. doi: 10.3389/fnagi.2019.00350
Zhang, Y., Brady, M., and Smith, S. (2001). Segmentation of brain MR images through a hidden Markov random field model and the expectation-maximization algorithm. IEEE Trans. Med. Imaging 20, 45–57. doi: 10.1109/42.906424
Zhao, Z. L., Fan, F. M., Lu, J., Li, H. J., Jia, L. F., Han, Y., et al. (2015). Changes of gray matter volume and amplitude of low-frequency oscillations in amnestic MCI: an integrative multi-modal MRI study. Acta Radiol. 56, 614–621. doi: 10.1177/0284185114533329
Zou, Q. H., Zhu, C. Z., Yang, Y., Zuo, X. N., Long, X. Y., Cao, Q. J., et al. (2008). An improved approach to detection of amplitude of low-frequency fluctuation (ALFF) for resting-state fMRI: fractional ALFF. J. Neurosci. Methods 172, 137–141. doi: 10.1016/j.jneumeth.2008.04.012
Keywords: Alzheimer's disease, A/T/N system, resting-state functional magnetic resonance imaging, amplitude of low frequency fluctuation, spontaneous brain activity, surface-based analysis
Citation: Zhu Z, Zeng Q, Kong L, Luo X, Li K, Xu X, Zhang M, Huang P and Yang Y (2021) Altered Spontaneous Brain Activity in Subjects With Different Cognitive States of Biologically Defined Alzheimer's Disease: A Surface-Based Functional Brain Imaging Study. Front. Aging Neurosci. 13:683783. doi: 10.3389/fnagi.2021.683783
Received: 22 March 2021; Accepted: 30 July 2021;
Published: 30 August 2021.
Edited by:
Alessandro Martorana, University of Rome Tor Vergata, ItalyReviewed by:
Enrico Premi, University of Brescia, ItalyClaudia Jacova, Pacific University, United States
Copyright © 2021 Zhu, Zeng, Kong, Luo, Li, Xu, Zhang, Huang and Yang. This is an open-access article distributed under the terms of the Creative Commons Attribution License (CC BY). The use, distribution or reproduction in other forums is permitted, provided the original author(s) and the copyright owner(s) are credited and that the original publication in this journal is cited, in accordance with accepted academic practice. No use, distribution or reproduction is permitted which does not comply with these terms.
*Correspondence: Yunjun Yang, yyjunjim@163.com; Peiyu Huang, huangpy@zju.edu.cn
†These authors have contributed equally to this work and share the first authorship
‡Data used in the preparation of this article were obtained from the Alzheimer's Disease Neuroimaging Initiative (ADNI) database (http://adni.loni.usc.edu). As such, the investigators within the ADNI contributed to the design and implementation of ADNI and/or provided data but did not participate in the analysis or writing of this report. A complete listing of ADNI investigators can be found at: http://adni.loni.usc.edu/wp-content/uploads/how_to_apply/ADNI_Acknowledgement_List.pdf