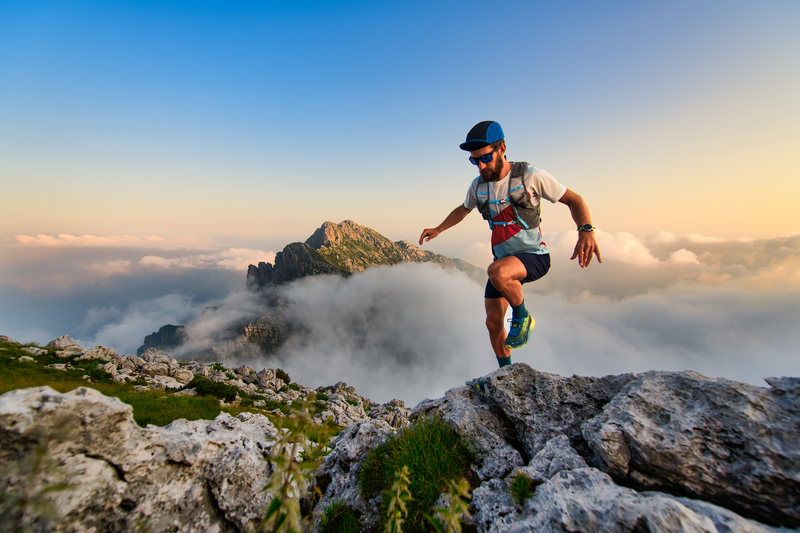
94% of researchers rate our articles as excellent or good
Learn more about the work of our research integrity team to safeguard the quality of each article we publish.
Find out more
ORIGINAL RESEARCH article
Front. Aging Neurosci. , 13 May 2021
Sec. Alzheimer's Disease and Related Dementias
Volume 13 - 2021 | https://doi.org/10.3389/fnagi.2021.619916
This article is part of the Research Topic Interplay of Genetic, Neural, Environmental and Behavioral Factors in the Context of Memory View all 4 articles
Background: Abnormal cholesterol metabolism is common in type 2 diabetes mellitus (T2DM) and causes dementia. Cholesterol 24S-hydroxylase (CYP46A1) converts cholesterol into 24S-hydroxycholesterol (24-OHC) and maintains cholesterol homeostasis in the brain.
Objective: This study aimed to investigate the roles of 24-OHC and the CYP46A1 (rs754203) polymorphism in patients with T2DM and mild cognitive impairment (MCI).
Methods: A total of 193 Chinese patients with T2DM were recruited into two groups according to the Montreal Cognitive Assessment (MoCA). Demographic and clinical data were collected, and neuropsychological tests were conducted. Enzyme-linked immunosorbent assay (ELISA) and Seqnome method were used to detect the concentration of plasma 24-OHC and the CYP46A1 rs754203 genotype, respectively.
Results: Compared with 118 healthy cognition participants, patients with MCI (n = 75) displayed a higher plasma level of 24-OHC and total cholesterol concentration (all p = 0.031), while no correlation was found between them. In the overall diabetes population, the plasma level of 24-OHC was negatively correlated with MoCA (r = −0.150, p = 0.039), and it was further proved to be an independent risk factor of diabetic MCI (OR = 1.848, p = 0.001). Additionally, patients with MCI and the CC genotype of CYP46A1 rs754203 showed the highest plasma level of 24-OHC even though the difference was not statistically significant, and they obtained low scores in both the verbal fluency test and Stroop color and word test A (p = 0.008 and p = 0.029, respectively).
Conclusion: In patients with T2DM, high plasma level of 24-OHC and the CC genotype carrier of CYP46A1 rs754203 may portend a high risk of developing early cognitive impairment, including attention and executive deficits.
Type 2 diabetes mellitus (T2DM) has become one of the global public health events with rapidly increasing incidence, and its chronic complications, including cognitive impairment, seriously endanger the patients. As a cognitive disorder syndrome between normal aging and dementia (Korolev et al., 2016; Kaneko et al., 2019), mild cognitive impairment (MCI) is the pre-clinical state of Alzheimer’s disease (AD). Given that adults with MCI tend to develop AD more rapidly than people of the same age who have no cognitive impairment (Petersen et al., 2001), approximately 10–15% of adults with MCI progress to AD per year (Petersen et al., 1999, 2001; Mitchell and Shiri-Feshki, 2009). Moreover, strong epidemiological studies have shown that diabetes is closely related to the occurrence of cognitive impairment (Gudala et al., 2013; Koekkoek et al., 2015; Zhang et al., 2017), and the incidence of MCI is higher in individuals with T2DM than in those without diabetes (Yuan and Wang, 2017). Thus, early diagnosis of MCI is crucial to prevent AD, especially in the high-risk diabetic population. To date, the mechanism of diabetes-associated MCI has not been fully elucidated. Many factors may be involved, such as high oxidative stress, mitochondrial dysfunction, dyslipidemia, insulin resistance, and impaired insulin signaling (Ahmad, 2013; Jayaraj et al., 2020).
Patients with diabetes are often accompanied with changes in cholesterol metabolism (Monnier et al., 1995). Previous research reported that insulin and diabetes can alter cholesterol metabolism in the brain (Suzuki et al., 2010). The brain is rich in cholesterol, accounting for 25% of the total body cholesterol (Björkhem and Meaney, 2004). Meanwhile, cholesterol metabolism was found to be related to the production and accumulation of aβ and the phosphorylation of tau protein (Yanagisawa, 2002), both of which play important roles in the occurrence of cognitive impairment. On the basis of the above-mentioned points, diabetes, cholesterol metabolism, and MCI are closely connected to one another. Cholesterol in the brain is produced in situ (Kacher et al., 2019). Cholesterol does not cross through the blood–brain barrier, and the brain’s cholesterol level is unaffected by peripheral cholesterol levels (Chobanian and Hollander, 1962; Jin et al., 2019). Therefore, special methods are needed to remove excess cholesterol from the brain so as to maintain the balance of cholesterol in the brain. Multiple enzymatic reactions can be considered to eliminate cholesterol in the brain, of which cholesterol 24-hydroxylation by CYP46A1 is quantitatively significant, accounting for the elimination of 75–85% of excess cholesterol from humans (Björkhem et al., 1998; Petrov and Pikuleva, 2019). Through cholesterol 24S-hydroxylase encoded by the CYP46A1 gene, cholesterol in the brain is converted into 24S-hydroxycholesterol (24-OHC), which is neurotoxic and can induce nerve cell death (Yamanaka et al., 2011). More than 90% of 24-OHC enters the blood through the blood–brain barrier (Kölsch et al., 2009). Cholesterol synthesis in the brain is balanced by the excretion of 24-OHC (Lund et al., 2003; Björkhem and Meaney, 2004). Meanwhile, more than 90% of 24-OHC in circulation is generated in the brain and can reflect the dynamic balance of cholesterol metabolism in the brain (Papassotiropoulos et al., 2000; Björkhem, 2006).
As a member of the human cytochrome P450 family, CYP46A1 plays an important role in the pathogenesis of both diabetes and cognitive decline. The polymorphism of CYP46A1 rs754203 is closely related to the risk of T2DM onset (Rizvi et al., 2017), which also participates in the risk of AD incidence (Li et al., 2013). However, the relationship between the polymorphism of CYP46A1 rs754203 and AD remains controversial, with some studies confirming the link and others disproving it (Chalmers et al., 2004; Jin et al., 2013). Interestingly, patients with AD and the CYP46A1 TT genotype seem to have the highest level of plasma 24-OHC (Li et al., 2018), which suggests that the CYP46A1 rs754203 polymorphism may play a role in mediating the increased risk of AD by causing differences in blood 24-OHC concentration levels.
Although numerous studies have proved that CYP46A1 and its rs754203 polymorphisms play an etiologic role in T2DM and MCI, their involvement in diabetic MCI has not yet been elucidated. By comparing the plasma level of the 24-OHC concentration and the CYP46A1 rs754203 polymorphism in patients with T2DM with or without MCI, we aimed to clarify whether there is a significant difference in the plasma level of the 24-OHC concentration between the two groups and what role the CYP46A1 rs754203 polymorphism plays in the occurrence of MCI. The results of this work can help reveal how abnormal cholesterol metabolism participates in the occurrence of MCI in T2DM and identify the early warning signs of diabetic MCI.
The study was conducted among 193 patients with T2DM who were hospitalized in the Department of Endocrinology, Zhongda Hospital, Southeast University. All participants were of Chinese Han nationality and signed an informed consent form based on a program approved by the Research Ethics Committee of the Affiliated Zhongda Hospital of Southeast University. All methods were carried out in accordance with relevant guidelines and regulations.
We recruited a total of 193 patients diagnosed with T2DM based on the 1999 World Health Organization criteria for diabetes, the duration of which is greater than or equal to 3 years (Alberti and Zimmet, 1998). The patients were 40–75 years old and fully cooperated to finish the test in this study. According to the Montreal Cognitive Assessment (MoCA), a brief and sensitive screening test for MCI (Dautzenberg et al., 2020), we divided the participants into two groups: 75 were patients with diabetes and MCI (MoCA < 26) and 118 were patients with diabetes and normal cognitive function (MoCA ≥ 26). All of the patients with MCI satisfied the diagnostic criteria proposed by the MCI Working Group of the European Consortium on AD in 2006 (Portet et al., 2006) as follows: (1) cognitive complaints from patients or their families, (2) decline in cognitive function in the past year relative to previous abilities, as reported by the patient or an informant, with a clinical dementia rating score of 0.5, (3) cognitive disorders as evidenced by clinical evaluation (impairment in memory or in another cognitive domain), (4) the absence of major repercussions in daily life (however, a patient may report difficulties in performing complex day-to-day activities), and (5) the absence of dementia. The following exclusion criteria were considered in this study: acute complications of diabetes such as hypoglycemia coma, diabetic ketoacidosis, lactic acidosis, and hyperosmolar non-kenotic diabetic coma; cerebrovascular accident confirmed by cranial imaging scan in the past year; definitive diagnosis of neurodegenerative diseases, such as AD and Parkinson’s disease; suffering from depression within 2 months; history of drug abuse or dependence; use of psychotropic drugs within 3 months prior to screening (e.g., anti-Parkinson’s drugs, benzodiazepines, sedatives, and anti-epilepsy drugs); systemic diseases such as malignant tumors, anemia, and severe infections; thyroid diseases; and visual and auditory defects that prevent neuropsychological testing.
Demographic variables including age, sex, education, smoking, drinking history, height, weight, and contact information were obtained through standardized interviews. Detailed medical information such as the diabetes duration, use of insulin, and hypertension (including the duration of hypertension) was recorded. Data on weight and height were collected to calculate the body mass index: BMI = weight/height2 (kg/m2). Patients with systolic blood pressure ≥ 140 mmHg or diastolic blood pressure ≥ 90 mmHg are defined as hypertensive. Fasting blood samples were collected to measure fasting blood glucose (FBG), glycosylated hemoglobin (HbA1c,%), total cholesterol (TC), triglycerides (TG), low-density lipoprotein cholesterol (LDL-C), high-density lipoprotein cholesterol (HDL-C), apolipoprotein A1 (ApoA-1), and apolipoprotein B (ApoB). Blood glucose at 2 h after a meal (2h-PBG) was also measured. All the experimental data mentioned above were obtained from the laboratory of the Affiliated Zhongda Hospital of Southeast University.
The participants’ cognitive function including attention, immediate memory, delayed memory, semantic memory, executive function, psychomotor speed, and visual–spatial ability was assessed via some neuropsychological tests. MoCA was first carried out to determine whether the participant is defined as MCI; the participant who had less than 12 years of education was added an extra point to obtain the adjusted MoCA score (Nasreddine et al., 2005). Other neuropsychological tests composed of the mini-mental state exam (MMSE) (Folstein et al., 1975), digit span test (DST) (Leung et al., 2011), verbal fluency test (VFT) (Teng et al., 2013), clock drawing test (CDT) (Shulman, 2000), auditory verbal learning test (AVLT) (Can et al., 2016), logical memory test (LMT) (Chapman et al., 2016), Stroop color and word test (SCWT) (Scarpina and Tagini, 2017), and trail making tests A and B (TMT-A and TMT-B) (St-Hilaire et al., 2018) were also conducted. Each participant’s test lasted about 45 min. All the tests were conducted by an experienced neuropsychologist from the Zhongda Hospital of Southeast University. The neuropsychologist and all subjects were blinded to the study design.
Blood samples were obtained from the participants after overnight fasting and stored in tubes with EDTA. The plasma was then stored at −80°C until assay. The plasma 24-OHC concentration of all specimens was detected by Enzyme-linked immunosorbent assay (ELISA) kits on the same day (Nanjing Jin Yibai Biological Technology Co., Ltd.) according to the instructions of the manufacturer. The final dilution of the sample was fivefold. The detection range of the kit used was 1.2–42 ng/L. The intra- and inter-assay coefficients of variation were less than 9 and 11%, respectively.
CYP46A1 SNP (rs754203) was detected by the Sequenom method (CNKINGBIO, Beijing, China). First, DNA was extracted from blood samples by using a DNA purification kit (Puregene, Gentra System, Minneapolis, MN, United States) following the manufacturer’s instructions. Second, the target regions were amplified by PCR, and the PCR products were processed with iPLEX® Pro or iPLEX Gold reagents. Third, a manual pipettor was used to transfer the samples from microtiter plates to a SpectroCHIP®Array. Data that had been saved to the MassARRAY database were obtained from the SpectroCHIP® array by using the MassARRAY® Analyzer. Finally, with the help of Typer software, the data were automatically analyzed, and a genotyping report was acquired.
Data were expressed as mean ± SD, median (interquartile range), or percentage. Student’s t-test and ANOVA were used for the analysis of normally distributed variables, with non-parametric Mann-Whitney U and Kruskal-Wallis tests used to analyze asymmetrically distributed variables. Chi-square (χ2) test was used to compare qualitative variables, explore the distribution of genotypes and allele frequencies, and determine deviations from the Hardy–Weinberg equilibrium. To examine the correlation between neuropsychological test scores and plasma 24-OHC concentrations, we used partial correlation. Logistic regression was conducted to identify independent variables that can significantly increase the risk of having MCI in patients with T2DM. SPSS 22.0 was conducted for statistical analysis (IBM Corporation). A p-value < 0.05 indicated statistical significance.
The baseline information of the patients with T2DM recruited in the research is shown in Table 1. Of the 193 patients enrolled in the study, 75 had MCI and 118 were cognitively normal. Patients with MCI were older, had a shorter education time, and suffered longer with hypertension than those with normal cognition. There was a difference in gender distribution between the two groups: more males were in the normal group, while more females were in the MCI group. Compared with the normal cognition group, the MCI group displayed higher levels of HbA1c and TC. A significant difference in the plasma 24-OHC concentration was found between the two groups (p = 0.031). Besides the characteristics mentioned above, other indicators such as history of smoking and drinking, BMI, percentage of patients with hypertension, SBP, DBP, diabetes duration, FBG, 2h-PBG, TG, LDL-c, HDL-c, use of insulin, ApoA1, and ApoB all showed no significant difference between the two groups (p > 0.05). In the neuropsychological test scores, except SCWT A number, significant differences were observed between the two groups, with high scores in the control group and low scores in the MCI group (p < 0.05).
Given that neuropsychological test scores and plasma 24-OHC concentrations were obviously different, partial correlations of plasma 24-OHC concentrations with neuropsychological tests in patients with T2DM were analyzed (Table 2). Among the patients with diabetes, the plasma 24-OHC concentration was negatively correlated with the MoCA score after adjusting the age, gender, and educational attainment (r = −0.150, p = 0.039), further confirming that the plasma 24-OHC concentration could reflect a cognitive status. No other correlations were discovered in terms of MMSE, DST, VFT, TMTA, TMTB, SCWT A time, SCWT A number, SCWT B time, SCWT B number, SCWT C time, SCWT C number, AVLT IR, AVLT DR, and plasma 24-OHC concentrations between total patients and MCI patients. We also explored the relationship between 24-OHC and TC and found no correlation between them (p > 0.05).
Table 2. Partial correlations of plasma 24-OHC concentrations with neuropsychological tests and plasma level of TC in patients with T2DM.
Table 3 shows the distributions of the CYP46A1 genotype and allele frequencies in the two groups. The distribution of the CYP46A1 genotype was consistent with the Hardy–Weinberg equilibrium in the MCI group and the control group. No difference was revealed in terms of distributions of the CYP46A1 genotype and allele frequencies between the MCI group and control group (p > 0.05).
Table 1 shows a significant difference in plasma 24-OHC concentration between the MCI group and the normal cognitive group. Therefore, we explored whether the plasma 24-OHC concentration among patients with different genotypes in each group varies. As shown in Table 4, regardless of the MCI group, normal group, or total participants, the plasma 24-OHC concentration did not show a significant difference among the three genotypic subgroups. Patients with MCI and the CC genotype showed the highest plasma level of 24-OHC, followed by the TC and TT genotype, even though it was not statistically significant.
To compare the cognitive differences among different genotypes, various neuropsychological test scores of the three genotypes (i.e., TT, TC, and CC) were compared, and the results are shown in Table 5. No significant differences were identified between genotypic subgroups in terms of neuropsychological test scores in the normal group (all p > 0.05). In the MCI group, VFT and SCWT A number were not the same in the three genotypic subgroups, and the difference was statistically significant (p = 0.019 and p = 0.033, respectively). Therefore, to further explore the specific differences between the two test scores in the three genotypic subgroups, LSD and all pairwise analyses were used (Table 6). The TT genotype had higher scores than the CC genotype, whether it was VFT or SCWT A number, suggesting that people with the CC genotype were more likely to have a cognitive impairment than those with the TT genotype (mean difference = 3.099 and 0.757; p = 0.008 and p = 0.029, respectively). Although the differences between TT and TC and between CC and TC were not statistically significant, people with more C genes had lower scores, suggesting that the C gene may be a risk factor of MCI.
To find the risk factors related to MCI in patients with T2DM, we first conducted simple logistic regression and incorporated the independent variables including age, gender, education level, BMI, hypertension duration, SBP, DBP, HbA1c, diabetes duration, history of smoking and drinking, FBG, 2h-PBG, TG, TC, LDL-c, HDL-c, use of insulin, genotype, and 24-OHC. The results showed that the independent variables associated with the occurrence of MCI were age, gender, education level, hypertension duration, HbA1c, TC, and 24-OHC (Table 7).
Table 7. Assessment results of the risk of having MCI in a simple logistic regression model in patients with T2DM.
Multivariable logistic regression was used, which showed that gender, education level, hypertension duration, HbA1c, and 24-OHC played a key role in the occurrence of MCI in patients with T2DM (Table 8).
Table 8. Assessment results of the risk of having MCI in a multivariable logistic regression model in patients with T2DM.
Our study demonstrated that plasma 24-OHC concentration played a negative role in the development of T2DM-associated MCI, as high levels were observed in patients with T2DM and MCI but low levels were noted in patients with T2DM and normal cognition. This result was further confirmed by our analysis, which revealed a negative correlation between the plasma 24-OHC concentration and MoCA after adjusting for age, gender, and educational attainment. No association between the genotype of CYP46A1 rs754203 and the plasma level of 24-OHC was found. Although the p-value was more than 0.05, patients with MCI and the CC genotype had the highest plasma level of 24-OHC among the tested patients. Among the patients with diabetes, those who carry a CC genotype may have a greater risk of developing MCI when compared to patients with a TT genotype. Logistic regression showed that females, low education level, long hypertension duration, and high HbA1c and plasma 24-OHC concentrations were associated with MCI in patients with T2DM. There was neither a significant difference in distributions of the CYP46A1 genotype between the MCI group and control group nor in plasma 24-OHC concentration among the three genotype subgroups.
Previous studies reported elevated plasma 24-OHC concentration levels in patients with MCI and AD (Lütjohann et al., 2000; Zuliani et al., 2011), which was consistent with our results. This conclusion supports that the plasma level of the 24-OHC concentration is proportional to the degree of brain atrophy and the loss of active gray matter (Liu et al., 2016). However, other studies indicated that people with AD have low levels of plasma 24-OHC concentration (Bretillon et al., 2000; Solomon et al., 2009; Burlot et al., 2015), and no significant difference was found between the MCI group and normal group (Liu et al., 2016). The possible explanation for this contradiction may be that the patients of these studies were at different stages of the disease. One previous research reported that the highest plasma level of 24-OHC was observed in mild AD patients (Li et al., 2018). When brain tissue damage is less severe in the early stage of the disease (such as MCI and early AD), the 24-OHC in plasma obviously rises but declines at the advanced stage of the disease. This phenomenon may be due to the decreased cholesterol turnover in the brain in the late stages, after a large number of neurons have degenerated and died, resulting in a low production of 24-OHC (Papassotiropoulos et al., 2000; Testa et al., 2016).
Besides 24-OHC, 27-hydroxycholesterol (27-OHC) is also an important oxysterol in the human body. It is a selective estrogen receptor modulator, which may affect tissues containing estrogen receptors such as blood vessels, bones, and breasts (Umetani and Shaul, 2011). It is derived from the periphery and can reflect the oxidative metabolism of cholesterol in the periphery (Hughes et al., 2013). Given that it can enter the brain through the blood–brain barrier, 27-OHC has also been shown to be neurotoxic and influences the occurrence of neurological disease (Popp et al., 2012; Chen et al., 2019). However, its effects within the brain remain unclear, and it is not expected to contribute to the pool of cholesterol in the brain (Hughes et al., 2013). Other studies have reported that it is also related to many non-neurological diseases, such as breast cancer, prostate cancer, lung adenocarcinoma, and COVID-19 (Marwarha et al., 2017; Zhang et al., 2019; Marcello et al., 2020). Therefore, compared with 24-OHC, 27-OHC seems to lack a certain specificity for cognitive disorders. That is why we chose 24-OHC as a priority for exploration. However, the influence of 27-OHC in neurological diseases cannot be ignored, and further research on it in the future is necessary.
In previous studies, the relationship between the CYP46A1 rs754203 polymorphism and MCI or AD has been controversial. Some studies have reported that the TT genotype and T allele are risk factors for the incidence of AD (He et al., 2012), while other studies suggested the CC genotype and C allele instead (Golanska et al., 2005). Others reported no correlation between them (Desai et al., 2002). Our results showed no difference in the distribution of the three genotypes between the MCI and the control groups. Although we obtained negative results in genotype distribution, when exploring the relationship between the polymorphism of the CYP46A1 rs754203 gene and the scores of various cognitive tests, VFT and SCWT A number showed significant differences among the three genotype subgroups of the MCI group. VFT is commonly used to assess lexical access from orthographic and phonemic networks, which is useful to measure executive function (Henry et al., 2004). The SCWT is a neuropsychological test extensively used for both experimental and clinical purposes (Scarpina and Tagini, 2017). SCWT A TEST is a word task, which can measure selective attention, cognitive flexibility information, and cognitive inhibition (Rivera et al., 2017). Pair comparison revealed that the scores of patients with the CC genotype were significantly lower than those with the TT genotype. Interestingly, when pair comparisons of TT and TC and of CC and TC were conducted, patients with the C allele had lower scores on these two tests despite the p-value being greater than 0.5. Therefore, we speculate that the CC genotype of CYP46A1 rs754203 might be a risk factor for diabetic MCI. The reason why there was no difference in the distribution of the three genotypes in our study may be related to the small number of participants. Our patients were all from the same hospital in China, making the study relatively limited in regions. The distribution of gender and age between the two groups was uneven, which possibly affected the results of the study to some extent. Li et al. (2013) pointed out that the presence of APOE ε4 allele can strengthen the effect of the CC genotype on AD risk. However, this gene was not detected in our study due to technical problems, and this factor was not considered when analyzing the results, which may have also affected our results. This should be taken into account in our subsequent studies.
No significant difference in plasma 24-OHC was found among the three genotype subgroups in our research. Although the p-value was greater than 0.05, we observed that the carriers of the CC genotype in the MCI group had the highest plasma 24-OHC, followed by the TC genotype and TT genotype; these findings were completely opposite to a previous study. In a previous study, what confused the researchers was that they found that people with the CC genotype had a higher risk of developing AD, but those with the TT genotype seemed to show a higher plasma concentration of 24-OHC (Li et al., 2018). An accurate explanation was not explored, and they speculated that this result may mean that other mechanisms may exist between the CYP46A1 gene polymorphism and the pathology of diseases related to AD and MCI.
A close relationship exists among AD, MCI, and T2DM. Janson et al. (2004) suggested a strong link between the neurodegenerative processes that lead to the loss of cortical brain cells in AD and the loss of β-cells in T2DM. Many previous studies, including our study, have explored the relationship between CYP46A1 gene polymorphisms and AD or MCI and reached some inconsistent conclusions. Some studies focused on the relationship between the CYP46A1 gene polymorphism and T2DM. In Rizvi et al.’s (2017) study, CC, CT genotypes, and C allele were found to be positive risk factors for T2DM. On the basis of these interesting findings, we suspect that patients with the CC genotype are more likely to develop T2DM, so people with the CC genotype among those with T2DM are more severely ill and lose more islet cells; thus, they are more likely to develop MCI. Lipid oxidation may be one of the body’s responses to islet dysfunction. This may explain some of the possible mechanisms between the CYP46A1 gene polymorphism and the occurrence of cognitive impairment.
According to the data in Table 1, the patients with MCI were older, with more female cases than males, having a shorter time of education, with higher HbA1c and 24-OHC levels, and suffering from hypertension longer than those with normal cognition. These findings showed that these factors may be related to the onset of MCI. Subsequent logistic regression analysis, after controlling for confounding factors, further proved this relationship and found these factors to be independent risk factors of MCI in patients with T2DM. For these factors, related studies have been conducted in the past. For age, some found that the incidence of MCI increases with age (Roberts et al., 2012; Ward et al., 2012), while others have not found the overall age influence (Brodaty et al., 2013). Regarding the impact of gender, controversies persist. Some believe that there is no significant difference in incidence between genders (Overton et al., 2019), and some studies believe that women are at a higher risk than men (Ma et al., 2016). For hypertension, previous studies showed that long-term high blood pressure status can cause brain material atrophy or white matter damage, which is closely related to cognitive impairment (Nagai et al., 2008, 2010). In terms of education, numerous studies believe that a high level of education can reduce the risk of developing AD; in the MCI stage, education may have a protective effect on total brain volume (Sattler et al., 2012), thereby preventing the occurrence of cognitive impairment (Wada et al., 2018). The US National Institutes of Health highlighted diabetes mellitus, smoking, depression, mental inactivity, physical inactivity, poor diet, hypertension, obesity, and low educational attainment as risk factors of cognitive decline and AD (Daviglus et al., 2010; Barnes and Yaffe, 2011). Our findings are consistent with previously reported results; some of the factors were not covered in our research, so they can be further confirmed in future work.
Similar studies were mostly based on people with no other particular diseases, whereas our study was conducted in patients with T2DM, which is a different point of innovation in our research. Our findings provide experimental evidence to some extent to reveal the pathogenesis of diabetes-related MCI, include some reference for future related research, and identify the possible indicators for early diagnosis and discovery of T2DM-associated MCI. Some possible risk factors for MCI were determined to help better prevent type 2 diabetic MCI. Despite these achievements, our research still had some shortcomings. First, many methods can be used for the determination of oxysterol, including gas chromatography–mass spectrometry, liquid chromatography–mass spectrometry, and liquid chromatography electro-spray ionization tandem mass spectrometry (Griffiths et al., 2013; Karuna et al., 2015). However, as a result of the limitations of our experimental technology, we could not determine the concentration of 24-OHC by these methods. We finally chose the ELISA method because its sensitivity and accuracy are slightly inferior to other methods. The literature revealed other studies that used the ELISA method to determine the plasma 24-OHC concentration (Roy et al., 2019). We used double-antibody sandwich ELISA, which has high sensitivity and high specificity, to maximize the reliability of the measurement results. Second, this work is a cross-sectional study, and the prevalence of MCI in the overall population was only 21.4% (Overton et al., 2019). The sample size of the participants and the number of patients with MCI and diabetes were both small, leading to poor matching of the two groups in terms of some baseline characteristics, such as age, education level, and hypertension duration. This possibly affected our results to a certain extent. Thus, when performing correlation analysis and logistic regression analysis, we adopted relevant statistical methods to control these confounding factors to minimize their possible impact. Third, our research was only carried out in patients with T2DM, and a group of non-diabetic people was lacking. Related research in these people must be carried out in the future to make the results more comprehensive. Fourth, the APOE ε4 allele is a key factor in the onset of AD and MCI; it exerts a remarkable influence on the relationship between the polymorphism of CYP46A1 and the onset of cognitive impairment (Li et al., 2013). However, our study did not detect this gene, so our research results lack accuracy and comprehensiveness. Lastly, our results showed no difference in the distribution of TT, CC, and TC between the two study groups. Only two cognitive-related test scores were different among the three subgroups, so we concluded that the CC genotype could lead to a higher incidence of MCI than the other genotypes. However, this result needs further scrutiny and should be interpreted with caution. Moreover, we only extracted the peripheral blood instead of the cerebrospinal fluid for testing due to operational inconvenience, so we can only speculate the situation of central lipid metabolism based on the results measured from the periphery.
In conclusion, early diagnosis of MCI is crucial to prevent further progression to AD. Our research have found that, in patients with T2DM, high plasma level of 24-OHC and the CC genotype carrier of CYP46A1 rs754203 may portend a high risk of developing early cognitive impairment, including attention and executive deficits. However, research based on a large sample size and wide population should be further conducted to explore the potential mechanisms.
All data generated or analyzed during this study are included in this article.
The studies involving human participants were reviewed and approved by the Research Ethics Committee of the Affiliated Zhongda Hospital of Southeast University. The patients/participants provided their written informed consent to participate in this study.
SW and YY contributed to the idea and revised the manuscript. JS carried out the design, conducted the study, and wrote the manuscript. WC and KA carried out the data collection. JJ and ST participated in the data analysis. HZ and WZ helped with data interpretation. All the authors read and approved the final manuscript.
This work was partially supported by the National Natural Science Foundation of China (Nos. 81570732, SW and 81870568, SW) and the Jiangsu Provincial Medical Youth Talent (QNRC2016819, YY).
The authors declare that the research was conducted in the absence of any commercial or financial relationships that could be construed as a potential conflict of interest.
We would like to express our heartfelt thanks to Guo, a neuropsychiatrist in the Department of Neurology, Zhongda Hospital, Southeast University, for her selfless help in conducting all the neuropsychological tests.
Ahmad, W. (2013). Overlapped metabolic and therapeutic links between Alzheimer and diabetes. Mol. Neurobiol. 47, 399–424. doi: 10.1007/s12035-012-8352-z
Alberti, K. G., and Zimmet, P. Z. (1998). Definition, diagnosis and classification of diabetes mellitus and its complications. Part 1: diagnosis and classification of diabetes mellitus provisional report of a WHO consultation. Diab. Med. 15, 539–553.
Barnes, D. E., and Yaffe, K. (2011). The projected effect of risk factor reduction on Alzheimer’s disease prevalence. Lancet. Neurol. 10, 819–828. doi: 10.1016/S1474-4422(11)70072-2
Björkhem, I. (2006). Crossing the barrier: oxysterols as cholesterol transporters and metabolic modulators in the brain. J. Int. Med. 260, 493–508. doi: 10.1111/j.1365-2796.2006.01725.x
Björkhem, I., Lütjohann, D., Diczfalusy, U., Ståhle, L., Ahlborg, G., and Wahren, J. (1998). Cholesterol homeostasis in human brain: turnover of 24S-hydroxycholesterol and evidence for a cerebral origin of most of this oxysterol in the circulation. J. Lipid Res. 39, 1594–1600.
Björkhem, I., and Meaney, S. (2004). Brain cholesterol: long secret life behind a barrier. Arterioscler Thromb Vasc. Biol. 24, 806–815. doi: 10.1161/01.ATV.0000120374.59826.1b
Bretillon, L., Sidén, A., Wahlund, L. O., Lütjohann, D., Minthon, L., Crisby, M., et al. (2000). Plasma levels of 24S-hydroxycholesterol in patients with neurological diseases. Neurosci. Lett. 293, 87–90. doi: 10.1016/s0304-3940(00)01466-x
Brodaty, H., Heffernan, M., Kochan, N. A., Draper, B., Trollor, J. N., Reppermund, S., et al. (2013). Mild cognitive impairment in a community sample: the sydney memory and ageing study. Alzheimers Dement 9, 310–317.e1. doi: 10.1016/j.jalz.2011.11.010
Burlot, M.-A., Braudeau, J., Michaelsen-Preusse, K., Potier, B., Ayciriex, S., Varin, J., et al. (2015). Cholesterol 24-hydroxylase defect is implicated in memory impairments associated with Alzheimer-like Tau pathology. Hum. Mol. Genet. 24, 5965–5976. doi: 10.1093/hmg/ddv268
Can, H., Doǧutepe, E., Torun Yazıhan, N., Korkman, H., and Erdoǧan Bakar, E. (2016). Construct validity of auditory verbal learning test. Turk Psikiyatri Derg 27, 195–203.
Chalmers, K. A., Culpan, D., Kehoe, P. G., Wilcock, G. K., Hughes, A., and Love, S. (2004). APOE promoter, ACE1 and CYP46 polymorphisms and beta-amyloid in Alzheimer’s disease. Neuroreport 15, 95–98. doi: 10.1097/00001756-200401190-00019
Chapman, K. R., Bing-Canar, H., Alosco, M. L., Steinberg, E. G., Martin, B., Chaisson, C., et al. (2016). Mini mental state examination and logical memory scores for entry into Alzheimer’s disease trials. Alzheimer’s Res. Therapy 8:9. doi: 10.1186/s13195-016-0176-z
Chen, S., Zhou, C., Yu, H., Tao, L., An, Y., Zhang, X., et al. (2019). 27-Hydroxycholesterol contributes to lysosomal membrane permeabilization-mediated pyroptosis in co-cultured SH-SY5Y cells and C6 cells. Front. Mol. Neurosci. 12:14. doi: 10.3389/fnmol.2019.00014
Chobanian, A. V., and Hollander, W. (1962). Body cholesterol metabolism in man. I. the equilibration of serum and tissue cholesterol. J. Clin. Invest. 41, 1732–1737. doi: 10.1172/JCI104631
Dautzenberg, G., Lijmer, J., and Beekman, A. (2020). Diagnostic accuracy of the Montreal Cognitive Assessment (MoCA) for cognitive screening in old age psychiatry: determining cutoff scores in clinical practice. avoiding spectrum bias caused by healthy controls. Int. J. Geriatric Psychiatry 35, 261–269. doi: 10.1002/gps.5227
Daviglus, M. L., Bell, C. C., Berrettini, W., Bowen, P. E., Connolly, E. S. Jr., Cox, N. J., et al. (2010). National institutes of health state-of-the-science conference statement: preventing alzheimer disease and cognitive decline. Annals Int. Med. 153, 176–181. doi: 10.7326/0003-4819-153-3-201008030-00260
Desai, P., DeKosky, S. T., and Kamboh, M. I. (2002). Genetic variation in the cholesterol 24-hydroxylase (CYP46) gene and the risk of Alzheimer’s disease. Neurosci. Lett. 328, 9–12. doi: 10.1016/s0304-3940(02)00443-3
Folstein, M. F., Folstein, S. E., and McHugh, P. R. (1975). Mini-mental state”. a practical method for grading the cognitive state of patients for the clinician. J. Psychiatric Res. 12, 189–198. doi: 10.1016/0022-3956(75)90026-6
Golanska, E., Hulas-Bigoszewska, K., Wojcik, I., Rieske, P., Styczynska, M., Peplonska, B., et al. (2005). CYP46: a risk factor for Alzheimer’s disease or a coincidence? Neurosci. Lett. 383, 105–108. doi: 10.1016/j.neulet.2005.03.049
Griffiths, W. J., Crick, P. J., and Wang, Y. (2013). Methods for oxysterol analysis: past, present and future. Biochem. Pharmacol. 86, 3–14. doi: 10.1016/j.bcp.2013.01.027
Gudala, K., Bansal, D., Schifano, F., and Bhansali, A. (2013). Diabetes mellitus and risk of dementia: a meta-analysis of prospective observational studies. J. Diab. Invest. 4, 640–650. doi: 10.1111/jdi.12087
He, X.-M., Zhang, Z.-X., Zhang, J.-W., Zhou, Y.-T., Wu, C.-B., Tang, M.-N., et al. (2012). An intronic CYP46A1 polymorphism is associated with Alzheimer disease in a Chinese Han population. J. Mol. Neurosci. MN. 47, 514–518. doi: 10.1007/s12031-012-9778-5
Henry, J. D., Crawford, J. R., and Phillips, L. H. (2004). Verbal fluency performance in dementia of the Alzheimer’s type: a meta-analysis. Neuropsychologia 42, 1212–1222. doi: 10.1016/j.neuropsychologia.2004.02.001
Hughes, T. M., Rosano, C., Evans, R. W., and Kuller, L. H. (2013). Brain cholesterol metabolism, oxysterols, and dementia. J. Alzheimers Dis. 33, 891–911. doi: 10.3233/jad-2012-121585
Janson, J., Laedtke, T., Parisi, J. E., O’Brien, P., Petersen, R. C., and Butler, P. C. (2004). Increased risk of type 2 diabetes in Alzheimer disease. Diabetes 53, 474–481. doi: 10.2337/diabetes.53.2.474
Jayaraj, R. L., Azimullah, S., and Beiram, R. (2020). Diabetes as a risk factor for Alzheimer’s disease in the middle east and its shared pathological mediators. Saudi J. Biol. Sci. 27, 736–750. doi: 10.1016/j.sjbs.2019.12.028
Jin, C., Zhang, F., Zhu, J., Yuan, J., Xia, M., Xu, Q., et al. (2013). Association of CYP46 gene polymorphism with sporadic Alzheimer’s disease in Chinese Han populations: a meta-analysis. Int. J. Neurosci. 123, 226–232. doi: 10.3109/00207454.2012.751533
Jin, U., Park, S. J., and Park, S. M. (2019). Cholesterol metabolism in the brain and its association with Parkinson’s Disease. Exp. Neurobiol. 28, 554–567. doi: 10.5607/en.2019.28.5.554
Kacher, R., Lamazière, A., Heck, N., Kappes, V., Mounier, C., Despres, G., et al. (2019). CYP46A1 gene therapy deciphers the role of brain cholesterol metabolism in Huntington’s disease. Brain 142, 2432–2450. doi: 10.1093/brain/awz174
Kaneko, H., Kimura, N., Nojima, S., Abe, K., Aso, Y., and Matsubara, E. (2019). Diagnosis of mild cognitive impairment using multiple neuroimaging modalities in addition to the Mini-Mental State Examination. Geriat. Gerontol. Int. 19, 1193–1197. doi: 10.1111/ggi.13789
Karuna, R., Christen, I., Sailer, A. W., Bitsch, F., and Zhang, J. (2015). Detection of dihydroxycholesterols in human plasma using HPLC-ESI-MS/MS. Steroids 99, 131–138. doi: 10.1016/j.steroids.2015.02.002
Koekkoek, P. S., Kappelle, L. J., van den Berg, E., Rutten, G. E., and Biessels, G. J. (2015). Cognitive function in patients with diabetes mellitus: guidance for daily care. Lancet Neurol. 14, 329–340. doi: 10.1016/s1474-4422(14)70249-2
Kölsch, H., Lütjohann, D., Jessen, F., Popp, J., Hentschel, F., Kelemen, P., et al. (2009). CYP46A1 variants influence Alzheimer’s disease risk and brain cholesterol metabolism. Eur. Psychiatry 24, 183–190. doi: 10.1016/j.eurpsy.2008.12.005
Korolev, I. O., Symonds, L. L., Bozoki, A. C., Alzheimer’s Disease, and Neuroimaging, I. (2016). Predicting progression from mild cognitive impairment to Alzheimer’s dementia using clinical, MRI, and plasma biomarkers via probabilistic pattern classification. PLoS One 11:e0138866. doi: 10.1371/journal.pone.0138866
Leung, J. L. M., Lee, G. T. H., Lam, Y. H., Chan, R. C. C., and Wu, J. Y. M. (2011). The use of the digit span test in screening for cognitive impairment in acute medical inpatients. Int. Psychogeriat. 23, 1569–1574. doi: 10.1017/S1041610211000792
Li, L., Yin, Z., Liu, J., Li, G., Wang, Y., Yan, J., et al. (2013). CYP46A1 T/C polymorphism associated with the APOE ε4 allele increases the risk of Alzheimer’s disease. J. Neurol. 260, 1701–1708. doi: 10.1007/s00415-012-6690-4
Li, L., Zeng, F., Liu, Y.-H., Li, H.-Y., Dong, S.-Y., Peng, Z.-Y., et al. (2018). CYP46A1 and the APOEε4 allele polymorphisms correlate with the risk of Alzheimer’s Disease. Mol. Neurobiol. 55, 8179–8187. doi: 10.1007/s12035-018-0952-9
Liu, Q., An, Y., Yu, H., Lu, Y., Feng, L., Wang, C., et al. (2016). Relationship between oxysterols and mild cognitive impairment in the elderly: a case-control study. Lipids Health Dis. 15:177. doi: 10.1186/s12944-016-0344-y
Lund, E. G., Xie, C., Kotti, T., Turley, S. D., Dietschy, J. M., and Russell, D. W. (2003). Knockout of the cholesterol 24-hydroxylase gene in mice reveals a brain-specific mechanism of cholesterol turnover. J. Biol. Chem. 278, 22980–22988. doi: 10.1074/jbc.M303415200
Lütjohann, D., Papassotiropoulos, A., Björkhem, I., Locatelli, S., Bagli, M., Oehring, R. D., et al. (2000). Plasma 24S-hydroxycholesterol (cerebrosterol) is increased in Alzheimer and vascular demented patients. J. Lipid Res. 41, 195–198.
Ma, F., Wu, T., Zhao, J., Ji, L., Song, A., Zhang, M., et al. (2016). Prevalence of mild cognitive impairment and its subtypes among chinese older adults: role of vascular risk factors. Dement. Geriatr. Cogn. Disord. 41, 261–272. doi: 10.1159/000446507
Marcello, A., Civra, A., Milan Bonotto, R., Nascimento Alves, L., Rajasekharan, S., Giacobone, C., et al. (2020). The cholesterol metabolite 27-hydroxycholesterol inhibits SARS-CoV-2 and is markedly decreased in COVID-19 patients. Redox Biol. 36:101682. doi: 10.1016/j.redox.2020.101682
Marwarha, G., Raza, S., Hammer, K., and Ghribi, O. (2017). 27-hydroxycholesterol: a novel player in molecular carcinogenesis of breast and prostate cancer. Chem. Phys. Lipids 207, 108–126. doi: 10.1016/j.chemphyslip.2017.05.012
Mitchell, A. J., and Shiri-Feshki, M. (2009). Rate of progression of mild cognitive impairment to dementia–meta-analysis of 41 robust inception cohort studies. Acta Psychiatrica Scand. 119, 252–265. doi: 10.1111/j.1600-0447.2008.01326.x
Monnier, L., Colette, C., Percheron, C., and Descomps, B. (1995). [Insulin, diabetes and cholesterol metabolism]. C R Seances Soc. Biol. Fil. 189, 919–931.
Nagai, M., Hoshide, S., Ishikawa, J., Shimada, K., and Kario, K. (2008). Ambulatory blood pressure as an independent determinant of brain atrophy and cognitive function in elderly hypertension. J. Hypertens. 26, 1636–1641. doi: 10.1097/HJH.0b013e3283018333
Nagai, M., Hoshide, S., and Kario, K. (2010). Hypertension and dementia. Am. J. Hypertens. 23, 116–124. doi: 10.1038/ajh.2009.212
Nasreddine, Z. S., Phillips, N. A., Bédirian, V., Charbonneau, S., Whitehead, V., Collin, I., et al. (2005). The montreal cognitive assessment, MoCA: a brief screening tool for mild cognitive impairment. J. Am. Geriat. Soc. 53, 695–699. doi: 10.1111/j.1532-5415.2005.53221.x
Overton, M., Pihlsgård, M., and Elmståhl, S. (2019). Prevalence and incidence of mild cognitive impairment across subtypes, age, and sex. Dement. Geriatr. Cogn. Disord. 47, 219–232. doi: 10.1159/000499763
Papassotiropoulos, A., Lütjohann, D., Bagli, M., Locatelli, S., Jessen, F., Rao, M. L., et al. (2000). Plasma 24S-hydroxycholesterol: a peripheral indicator of neuronal degeneration and potential state marker for Alzheimer’s disease. Neuroreport 11, 1959–1962. doi: 10.1097/00001756-200006260-00030
Petersen, R. C., Doody, R., Kurz, A., Mohs, R. C., Morris, J. C., Rabins, P. V., et al. (2001). Current concepts in mild cognitive impairment. Arch. Neurol. 58, 1985–1992. doi: 10.1001/archneur.58.12.1985
Petersen, R. C., Smith, G. E., Waring, S. C., Ivnik, R. J., Tangalos, E. G., and Kokmen, E. (1999). Mild cognitive impairment: clinical characterization and outcome. Arch. Neurol. 56, 303–308. doi: 10.1001/archneur.56.3.303
Petrov, A. M., and Pikuleva, I. A. (2019). Cholesterol 24-Hydroxylation by CYP46A1: benefits of modulation for Brain diseases. Neurotherapeutics 16, 635–648. doi: 10.1007/s13311-019-00731-6
Popp, J., Lewczuk, P., Kölsch, H., Meichsner, S., Maier, W., Kornhuber, J., et al. (2012). Cholesterol metabolism is associated with soluble amyloid precursor protein production in Alzheimer’s disease. J. Neurochem. 123, 310–316. doi: 10.1111/j.1471-4159.2012.07893.x
Portet, F., Ousset, P. J., Visser, P. J., Frisoni, G. B., Nobili, F., Scheltens, P., et al. (2006). Mild cognitive impairment (MCI) in medical practice: a critical review of the concept and new diagnostic procedure. report of the MCI working group of the european consortium on Alzheimer’s disease. J. Neurol. Neurosurg. Psychiatry 77, 714–718. doi: 10.1136/jnnp.2005.085332
Rivera, D., Morlett-Paredes, A., Peñalver Guia, A. I., Irías Escher, M. J., Soto-Añari, M., Aguayo Arelis, A., et al. (2017). Stroop color-word interference test: normative data for Spanish-speaking pediatric population. NeuroRehabilitation 41, 605–616. doi: 10.3233/nre-172246
Rizvi, S., Raza, S. T., Mehdi, S. R., Siddiqi, Z., Eba, A., and Mahdi, F. (2017). The relationship between multidrug resistance protein 1(rs1045642) and Cholesterol 24-hydroxylase (rs754203) genes polymorphism with type 2 diabetes mellitus. Br. J. Biomed. Sci. 74, 30–35. doi: 10.1080/09674845.2016.1264212
Roberts, R. O., Geda, Y. E., Knopman, D. S., Cha, R. H., Pankratz, V. S., Boeve, B. F., et al. (2012). The incidence of MCI differs by subtype and is higher in men: the mayo clinic study of aging. Neurology 78, 342–351. doi: 10.1212/WNL.0b013e3182452862
Roy, D., Chakrabarti, S. S., Banerjee, A., Sharma, P., Biswas, A., and Chakrabarti, S. (2019). Serum 24-hydroxycholesterol in probable Alzheimer’s dementia: reexploring the significance of a tentative Alzheimer’s disease biomarker. Aging Med. (Milton) 2, 74–81. doi: 10.1002/agm2.12068
Sattler, C., Toro, P., Schönknecht, P., and Schröder, J. (2012). Cognitive activity, education and socioeconomic status as preventive factors for mild cognitive impairment and Alzheimer’s disease. Psychiatry Res. 196, 90–95. doi: 10.1016/j.psychres.2011.11.012
Scarpina, F., and Tagini, S. (2017). The stroop color and word test. Front. Psychol. 8:557–557. doi: 10.3389/fpsyg.2017.00557
Shulman, K. I. (2000). Clock-drawing: is it the ideal cognitive screening test? Int. J. Geriatr. Psychiatry 15, 548–561.
Solomon, A., Leoni, V., Kivipelto, M., Besga, A., Oksengård, A. R., Julin, P., et al. (2009). Plasma levels of 24S-hydroxycholesterol reflect brain volumes in patients without objective cognitive impairment but not in those with Alzheimer’s disease. Neurosci. Lett. 462, 89–93. doi: 10.1016/j.neulet.2009.06.073
St-Hilaire, A., Parent, C., Potvin, O., Bherer, L., Gagnon, J.-F., Joubert, S., et al. (2018). Trail making tests A and B: regression-based normative data for Quebec french-speaking mid and older aged adults. Clin. Neuropsychol. 32, 77–90. doi: 10.1080/13854046.2018.1470675
Suzuki, R., Lee, K., Jing, E., Biddinger, S. B., McDonald, J. G., Montine, T. J., et al. (2010). Diabetes and insulin in regulation of brain cholesterol metabolism. Cell Metab. 12, 567–579. doi: 10.1016/j.cmet.2010.11.006
Teng, E., Leone-Friedman, J., Lee, G. J., Woo, S., Apostolova, L. G., Harrell, S., et al. (2013). Similar verbal fluency patterns in amnestic mild cognitive impairment and Alzheimer’s disease. Arch. Clin. Neuropsychol. 28, 400–410. doi: 10.1093/arclin/act039
Testa, G., Staurenghi, E., Zerbinati, C., Gargiulo, S., Iuliano, L., Giaccone, G., et al. (2016). Changes in brain oxysterols at different stages of Alzheimer’s disease: their involvement in neuroinflammation. Redox Biol. 10, 24–33. doi: 10.1016/j.redox.2016.09.001
Umetani, M., and Shaul, P. W. (2011). 27-Hydroxycholesterol: the first identified endogenous SERM. Trends Endocrinol. Metab. 22, 130–135. doi: 10.1016/j.tem.2011.01.003
Wada, M., Noda, Y., Shinagawa, S., Chung, J. K., Sawada, K., Ogyu, K., et al. (2018). Effect of education on Alzheimer’s disease-related neuroimaging biomarkers in healthy controls, and participants with mild cognitive impairment and alzheimer’s disease: a cross-sectional study. J. Alzheimers Dis. 63, 861–869. doi: 10.3233/jad-171168
Ward, A., Arrighi, H. M., Michels, S., and Cedarbaum, J. M. (2012). Mild cognitive impairment: disparity of incidence and prevalence estimates. Alzheimers Dement. 8, 14–21. doi: 10.1016/j.jalz.2011.01.002
Yamanaka, K., Saito, Y., Yamamori, T., Urano, Y., and Noguchi, N. (2011). 24(S)-hydroxycholesterol induces neuronal cell death through necroptosis, a form of programmed necrosis. J. Biol. Chem. 286, 24666–24673. doi: 10.1074/jbc.M111.236273
Yanagisawa, K. (2002). Cholesterol and pathological processes in Alzheimer’s disease. J. Neurosci. Res. 70, 361–366. doi: 10.1002/jnr.10348
Yuan, X.-Y., and Wang, X.-G. (2017). Mild cognitive impairment in type 2 diabetes mellitus and related risk factors: a review. Rev. Neurosci. 28, 715–723. doi: 10.1515/revneuro-2017-0016
Zhang, J., Chen, C., Hua, S., Liao, H., Wang, M., Xiong, Y., et al. (2017). An updated meta-analysis of cohort studies: diabetes and risk of Alzheimer’s disease. Diab. Res. Clin. Pract. 124, 41–47. doi: 10.1016/j.diabres.2016.10.024
Zhang, L., Liu, M., Liu, J., Li, X., Yang, M., Su, B., et al. (2019). 27-Hydroxycholesterol enhanced osteoclastogenesis in lung adenocarcinoma microenvironment. J. Cell Physiol. 234, 12692–12700. doi: 10.1002/jcp.27883
Keywords: type 2 diabetes mellitus, executive function, attention function, mild cognitive impairment, 24S-hydroxycholesterol
Citation: Shi J, Jia J, Tian S, Zhang H, An K, Zhu W, Cao W, Yuan Y and Wang S (2021) Increased Plasma Level of 24S-Hydroxycholesterol and Polymorphism of CYP46A1 SNP (rs754203) Are Associated With Mild Cognitive Impairment in Patients With Type 2 Diabetes. Front. Aging Neurosci. 13:619916. doi: 10.3389/fnagi.2021.619916
Received: 21 October 2020; Accepted: 29 March 2021;
Published: 13 May 2021.
Edited by:
Jessica L. Teeling, University of Southampton, United KingdomReviewed by:
Jade De Oliveira, Federal University of Rio Grande do Sul, BrazilCopyright © 2021 Shi, Jia, Tian, Zhang, An, Zhu, Cao, Yuan and Wang. This is an open-access article distributed under the terms of the Creative Commons Attribution License (CC BY). The use, distribution or reproduction in other forums is permitted, provided the original author(s) and the copyright owner(s) are credited and that the original publication in this journal is cited, in accordance with accepted academic practice. No use, distribution or reproduction is permitted which does not comply with these terms.
*Correspondence: Shaohua Wang, Z3lqd3NoQDEyNi5jb20=; Yang Yuan, eWFuZ195dWFuMDAwQHNpbmEuY29t
Disclaimer: All claims expressed in this article are solely those of the authors and do not necessarily represent those of their affiliated organizations, or those of the publisher, the editors and the reviewers. Any product that may be evaluated in this article or claim that may be made by its manufacturer is not guaranteed or endorsed by the publisher.
Research integrity at Frontiers
Learn more about the work of our research integrity team to safeguard the quality of each article we publish.