- 1Laboratory of Cognitive and Computational Neuroscience (Complutense University of Madrid - Universidad Politécnica de Madrid), Center for Biomedical Technology (CTB), Universidad Politécnica de Madrid (UPM), Madrid, Spain
- 2Department of Structure of Matter, Thermal Physics and Electronics, Complutense University of Madrid (UCM), Madrid, Spain
- 3Department of Experimental Psychology, Complutense University of Madrid (UCM), Madrid, Spain
- 4Foundation for Biomedical Research, University Hospital of Getafe, Getafe, Spain
- 5Centro de Investigación Biomédica en Red Fragilidad y Envejecimiento Saludable (CIBERFES), Spain
- 6Department of Medicine and Public Health, Rey Juan Carlos University, Madrid, Spain
- 7Department of Psychobiology and Methodology in Behavioral Sciences, Universidad Complutense de Madrid (UCM), Madrid, Spain
- 8Radiology Service, University Hospital of Getafe, Getafe, Spain
- 9Geriatric Service, University Hospital of Getafe, Getafe, Spain
- 10Centro de Investigación Biomédica en Red en Bioingeniería, Biomateriales y Nanomedicina (CIBER-BBN), Spain
Objective: The role of the central nervous system in the pathophysiology of frailty is controversial. We used magnetoencephalography (MEG) to search for abnormalities in the ongoing oscillatory neural activity of frail individuals without global cognitive impairment.
Methods: Fifty four older (≥70 years) and cognitively healthy (Mini-Mental State Examination ≥24) participants were classified as robust (0 criterion, n = 34) or frail (≥ 3 criteria, n = 20) following Fried's phenotype. Memory, language, attention, and executive function were assessed through well-validated neuropsychological tests. Every participant underwent a resting-state MEG and a T1-weighted magnetic resonance imaging scan. We performed MEG power spectral analyses to compare the electrophysiological profiles of frail and robust individuals. We used an ensemble learner to investigate the ability of MEG spectral power to discriminate frail from robust participants.
Results: We identified increased relative power in the frail group in the mu (p < 0.05) and sensorimotor (p < 0.05) frequencies across right sensorimotor, posterior parietal, and frontal regions. The ensemble learner discriminated frail from robust participants [area under the curve = 0.73 (95% CI = 0.49–0.98)]. Frail individuals performed significantly worse in the Trail Making Test, Digit Span Test (forward), Rey-Osterrieth Complex Figure, and Semantic Fluency Test.
Interpretation: Frail individuals without global cognitive impairment showed ongoing oscillatory alterations within brain regions associated with aspects of motor control, jointly to failures in executive function. Our results suggest that some physical manifestations of frailty might partly arise from failures in central structures relevant to sensorimotor and executive processing.
Introduction
Frailty is one of the major expressions of cumulative age-related decline that may precede disability in older adults, affecting 10–17% of the aged population (Collard et al., 2012). So far, frailty has been characterized through two main conceptual frameworks: as a predictive factor of adverse outcome in old people, and as the phenotypic expression of the combined effect of natural aging and chronic conditions. The complex pathophysiology of frailty has led to several controversies concerning the domains that integrate the syndrome, and the systems and organs involved in its development. In this regard, the participation of the central nervous system (CNS), and particularly brain structure and function, is one of the queries fueling debate. Several authors propose that the CNS is implicated in the pathophysiology of frailty, specifically causing the cognitive decline that some patients experience alongside the physical manifestations of the syndrome (Ávila-Funes et al., 2009). Accordingly, a particular subtype of frailty, cognitive frailty, was introduced in order to subsume those cases in which cognitive and physical disruptions coexist (Kelaiditi et al., 2013). However, even if frailty seems to be highly predictive of cognitive impairment and dementia (Rosado-Artalejo et al., 2017b), most frail patients do not exhibit significant cognitive impairment when assessed through general screening tools like the Mini-Mental State Examination (MMSE) (García-García et al., 2011). In view of this, other authors argue that CNS dysfunction is another manifestation of frailty's multisystem vulnerability, and as such, it should not be detached into specific subtypes. In practice, even if current clinical instruments for diagnosing frailty focus on testing physical items, they might also reveal expressions of brain dysfunctionality beyond cognition (Rodríguez-Mañas and Sinclair, 2014).
Despite the debate, the number of studies investigating the role of the CNS in frailty is still scarce (López-Sanz et al., 2018). Till now, only a handful of neuroimaging reports have identified brain structural (Newman et al., 2001; Buchman et al., 2014; Jung et al., 2014; Avila-Funes et al., 2017; Del Brutto et al., 2017; Kant et al., 2018; Maltais et al., 2020) and functional (Lammers et al., 2020; Suárez-Méndez et al., 2020) abnormalities associated with frailty as a unified entity. In general terms, these studies provided concurring evidence of white matter and ischemic damage in frail individuals. On the other hand, several studies connected CNS structural and functional disruptions to alterations in individual items commonly used to assess frailty, such as gait speed (Rosano et al., 2010; Yuan et al., 2015; Rosario et al., 2016) and handgrip strength (Seidler et al., 2015; Hirsiger et al., 2016). The absence of a more expanded literature is disconcerting considering the successful application of neuroimaging techniques to other fields of research [e.g., dementia (Jagust et al., 2006; Nakamura et al., 2018)] that could be mirrored in the study of frailty. In this work, we used magnetoencephalography (MEG) to investigate the existence of abnormalities in the ongoing oscillatory neural activity of frail individuals without global cognitive impairment. The aim of this work was to delve into the hypothesis that the CNS is involved in the pathophysiology of frailty in the absence of cognitive symptoms and that accordingly, changes in brain function should be observed in frail individuals without significant cognitive impairment that could suggest mild cognitive impairment or dementia. Additionally, we explored whether MEG power spectral properties could be used to train a classifier into discriminating frail from robust participants.
Materials and Methods
Participants
The sample of this study was recruited from an outpatient basis of the Geriatric Service of the Getafe University Hospital (Madrid, Spain). One hundred and seventy candidates qualified in the clinical records as frail or robust were originally contacted by phone and invited to participate. For each frail individual, two robust peers of the same age (± 1 year) and gender were included at the invitation stage. Candidates were classified as frail (if meeting ≥ 3 criteria) or robust (if meeting 0 criteria) according to Fried's phenotype criteria (Fried et al., 2001; García-García et al., 2011), namely involuntary weight loss (≥4.5 kg in the last year), self-reported exhaustion, sedentary behavior, poor handgrip strength, and reduced gait speed (Table 1). In order to maximize the findings of our study, and taking into account the sample size, those candidates classified as pre-frail (i.e., those meeting 1–2 criteria) were not considered for the study and excluded. Sixty older adults (robust and frail) were finally enrolled. All the participants were right-handed and native Spanish speakers.
During the initial screening session, an expert neuropsychologist (NP) evaluated the general cognitive status of all the pre-selected participants using the MMSE. For those participants achieving an MMSE score ≥ 24, the following domains were further assessed: (i) memory, (ii) language, (iii) attention, and (iv) executive function. Memory was evaluated with the Logical Memory Score (immediate and delayed recall) from the Wechsler Memory Scale-III (WMS-III, Spanish version), and the Rey-Osterrieth Complex Figure (ROCF). Language function was evaluated with the Boston Naming Test (BNT) and the Phonemic and Semantic Fluency Tests from the Controlled Oral Word Association Test (COWAT). Finally, attention and executive function were evaluated with the Trail Making Test parts A and B (TMTA and TMTB) and the Digit Span Test (forward and backward) of the WMS-III. The neuropsychological assessment was conducted on a single session, and completion times ranged from 43 to 57 min. Additionally, a functional evaluation including the Barthel Index for Activities of Daily Living (ADL) and the Short Physical Performance Battery (SPPB) was performed. The presence of comorbidities was also recorded for all participants.
Inclusion and Exclusion Criteria
Participants were required to be at least 70 years of age and to score ≥ 24 in the MMSE (indicative of normal cognition). Furthermore, participants were excluded from our study in case of (i) history of psychiatric disorders, neurological disorders, or drug consumption that could affect MEG activity (e.g., cholinesterase inhibitors), (ii) having a medical condition with increased risk of associated cognitive symptoms, (iii) severe head injury with loss of consciousness within the last 5 years, (iv) usual intake of alcohol (> 3 alcoholic beverages per day), (v) use of neuroleptics, major narcotics, sedative-hypnotics, or anticonvulsants, (vi) hearing or vision impairments that would preclude testing, and (vii) Axis I psychiatric diagnoses including depression. Participants with a history of stroke were excluded only if showing focal neurological symptoms in the neurological exam or a focal impairment in the magnetic resonance imaging (MRI) scan. Finally, individuals with pacemakers or metallic implants that could interfere with MEG or MRI were also excluded.
Out of the initial 170 candidates contacted, 56 refused to participate in the study: 40 were not interested after being informed of the proceedings, 5 refused due to fear of MEG or MRI, 4 lived away and could not attend the sessions, 6 were caretakers of dependent adults or children and could not attend the sessions, and 1 candidate had an upcoming surgery. Fourteen candidates did not contact back. One candidate had passed away. Among those favorable to enrolling, 5 candidates scored below the MMSE inclusion threshold, 10 candidates presented pacemakers (1), metallic implants (6), prosthesis (1), or stents (2). One candidate was excluded due to severe vision impairment, 1 candidate was excluded because he was pre-frail and had been misclassified, 2 candidates could not walk, 8 candidates were excluded due to their prescribed medication, and 9 due to diverse medical conditions. Two candidates were excluded because they had family members in the study, and 1 candidate was excluded due to not matching the inclusion criteria after a stroke. This information is summarized in the flow diagram depicted in Figure 1.
MEG Signal
Recordings
MEG data were acquired at the Laboratory of Cognitive and Computational Neuroscience (UCM-UPM) in the Center for Biomedical Technology (CTB) (Madrid, Spain) with a 306 channel (102 magnetometers, 204 planar gradiometers) Vectorview MEG system (Elekta AB, Stockholm, Sweden) situated in a magnetically shielded room (VacuumSchmelze GmbH, Hanau, Germany). The experimental procedure was carefully explained to all the participants upon their arrival at the Center. During the initial setup, we used a 3D Fastrak digitizer (Polhemus, Colchester, Vermont) to identify three fiducial points (nasion and bilateral preauricular points) and to outline the head shape of the participants by digitizing ~400 scalp points. We placed two Head Position Indicator (HPI) coils in the mastoids, and two in the forehead of the participants. The exact position of the HPI coils was acquired with the Fastrak digitizer to allow for head position estimation during the recording. Finally, we placed two electrooculogram (EOG) electrodes (above and below the left eye) to capture eye blinks and ocular movements, and two electrocardiogram (EKG) electrodes (forming a diagonal across the chest) to capture the electrocardiographic signal. After the initial setup, the participants were conducted to the MEG room where they were asked to sit comfortably and still throughout the recording. We recorded 5 min of electrophysiological activity while the participants rested awake with their eyes closed. MEG data were acquired with a sampling rate of 1,000 Hz and an online anti-alias band-pass filter between 0.1 and 330 Hz. The recordings were processed offline using a tempo-spatial filtering algorithm (tSSS) (Taulu and Hari, 2009) (Maxfilter software v 2.2, correlation limit = 0.9, correlation window = 10 s) to eliminate magnetic noise coming from outside of the head. The same algorithm was used to compensate for the movements of the head during the recording.
Pre-processing
We identified ocular, muscular, and jump artifacts within our data using the automatic artifact-detection algorithm of the Fieldtrip toolbox (Oostenveld et al., 2011) for Matlab (R2017b, Mathworks, Inc.). Afterward, a MEG expert visually inspected the data to verify the automatic detection. An algorithm based on independent components was used to remove the contributions of EOG and EKG signals from our data. Finally, the remaining data were segmented in epochs of 4 s of artifact-free activity: 77.5 [12.1] trials [mean (SD)] in the frail group, 81.0 [13.1] trials in the robust group, with no significant group-level effect regarding the number of trials (p = 0.33). Because of the high redundancy found in MEG data after spatial filtering, only magnetometers' data were used in our analyses (Garcés et al., 2017).
MRI Acquisition
Following the MEG recordings, a three-dimensional T1-weighted anatomical MRI was acquired for each participant at the Magnetosur clinic in Getafe (Madrid, Spain) with a 0.23 T open configuration (C-shaped) Philips Panorama MRI scanner (repetition time/echo time = 470/16 ms, flip angle 90°, slice thickness = 5 mm, 256 × 256 matrix, and field of view = 270 mm). The T1-weighted images were used for MEG source reconstruction. Additionally, the T1-weighted images were inspected by an experienced radiologist (ECG) to discard the presence of small vessel disease within our sample.
Source Reconstruction
The clean epochs were band-pass filtered between [2–30] Hz to remove both low-frequency noise and the power line artifact. Before filtering, epochs were padded with 2 s (2,000 samples) of real signal on both ends to avoid edge effects within the data. The source model consisted of 2,459 sources placed in a homogeneous grid of 1 cm in a Montreal Neurological Institute (MNI) template, converted to subject-space by an affine transformation. Each source was assigned to one of the 64 cortical areas defined in the Harvard-Oxford atlas. The lead field was calculated with a single-shell head model (Nolte, 2003) generated from the individual T1-weighted image using the Fieldtrip toolbox (Oostenveld et al., 2011). We employed a Linearly Constrained Minimum Variance (LCMV) beamformer (Van Veen et al., 1997) to obtain the source-space time-series using the lead field and building the beamformer filters with the epoch-averaged covariance matrix.
Spectral Analysis
We used the beamformer filters to reconstruct the epoched source-space time-series for each cortical source. Afterward, we calculated the MEG power spectra for every clean epoch using the Fast Fourier Transform (FFT) with a Hanning window for frequencies [2–30] Hz and frequency steps of 0.25 Hz. The resulting power spectra were averaged across epochs and normalized by the overall power in [2–30] Hz, thus obtaining the values of the relative power for each frequency step, source, and participant. We searched for group-level effects in the classical frequency bands: theta [4–8] Hz, alpha (mu) [8–13] Hz, and beta [12–30] Hz, and hypothesized further differences in human sensorimotor frequencies (SMR) [12–15] Hz.
Statistical Analysis
The frail and robust groups were matched for age and gender. Independent samples t-tests were used to perform group comparisons in the power-spectral analyses. The elevated number of statistical comparisons in the power spectral analyses made it necessary to apply two procedures for multiple comparisons correction. (1) We performed a Montecarlo permutation method based on the clustering of spatially contiguous significant sources (100,000 permutations, alpha = 0.05). The exact details of the cluster-based permutation test (CBPT) performed herein can be found in the original formulation by Maris and Oostenveld (2007). This method was performed using the Fieldtrip toolbox (Oostenveld et al., 2011) for Matlab (R2017b, Mathworks, Inc.). (2) To correct for the different frequency bands [theta, alpha (mu), beta, and SMR] we used the False Discovery Rate (FDR; Benjamini and Hochberg, 1995) (Q = 0.1). This method was performed using Matlab (R2017b, Mathworks, Inc.). For the statistical comparison of functional and neuropsychological scores, we used Fisher's exact test and Mann–Whitney U-test for continuous variables.
Discrimination Analysis
We performed the classification analysis after randomly splitting the study sample into a 2/3 discovery sample and a 1/3 validation sample to gain insight into the external validity. To test the ability of MEG spectral power to correctly classify frail and robust participants, we used an ensemble learner as implemented in R 3.5.1 (R Core Team, 2017) and the SuperLearner package (Polley et al., 2018). This ensemble combined three machine learning algorithms: Least Absolute Shrinkage and Selection Operator (LASSO) [as implemented in the glmnet package (Friedman et al., 2010)], a random forest classifier (as implemented in randomForest), and a support vector machine (SVM) classifier [as implemented in e1071 (Meyer et al., 2015)]. We used the default criteria for all classifiers. Because of the large number of variables (258, i.e., age, sex, and 4 frequency bands × 64 cortical areas) compared to the low number of participants, we included a screening step using LASSO for the random forest and the SVM classifiers, and the univariate Pearson correlation p < 0.1 for the LASSO classifier for variables to be included in the learner. In the ensemble learner, we maximized the Area Under the Curve (AUC) using leave-one-out cross-validation for model selection. We used the predicted probability of being frail from SuperLearner for Receiver Operating Characteristic (ROC) curve analysis and report the associated AUC as a measure for discrimination quality.
Ethical Issues
This study was approved by the Ethical Committee of the University Hospital of Getafe (Madrid, Spain). All participants provided signed informed consent prior to study enrollment.
Results
The sample of our study originally consisted of 60 participants, 6 of which were discarded for the analyses due to problems in the recorded signals. We did not observe significant group differences regarding age (p = 0.104), gender (p = 0.381), nor comorbidities. Frail participants obtained significantly poorer scores in the ADL [frail: 92.50 (80.00, 100.00) vs. robust: 100.00 (100.00, 100.00); p < 0.001] and the SPPB [frail: 7.00 (5.00, 8.00) vs. robust: 10.00 (9.00, 11.75); p < 0.001). As expected from the exclusion criteria, mean MMSE was over 24, with no significant differences between groups (p = 0.543). We found that frail participants attained significantly poorer results than robust counterparts in some neuropsychological tests examining executive function. Specifically, frail participants performed worse in TMTB (errors) [frail: 4.00 (3.00, 6.00) vs. robust: 2.00 (1.00, 4.75); p = 0.032) and the Digit Span Test (forward) [frail: 6.00 (4.75, 7.00) vs. robust: 7.00 (6.00, 8.00); p = 0.036]. Additionally, we observed significant group differences in the ROCF (immediate recall) [frail: 8.50 (4.62, 12.50) vs. robust: 12.50 (8.62, 15.50); p = 0.039], ROCF (delayed recall) [frail: 8.00 (2.75, 11.62) vs. robust: 11.00 (8.50, 14.00); p = 0.021], and the Semantic Fluency Test [frail: 10.50 (9.00, 14.25) vs. robust: 14.00 (11.00, 17.75); p = 0.045], indicating lower performance of the frail group. A summary of the demographic, clinical, functional, and neuropsychological information of the final sample can be found in Table 2.
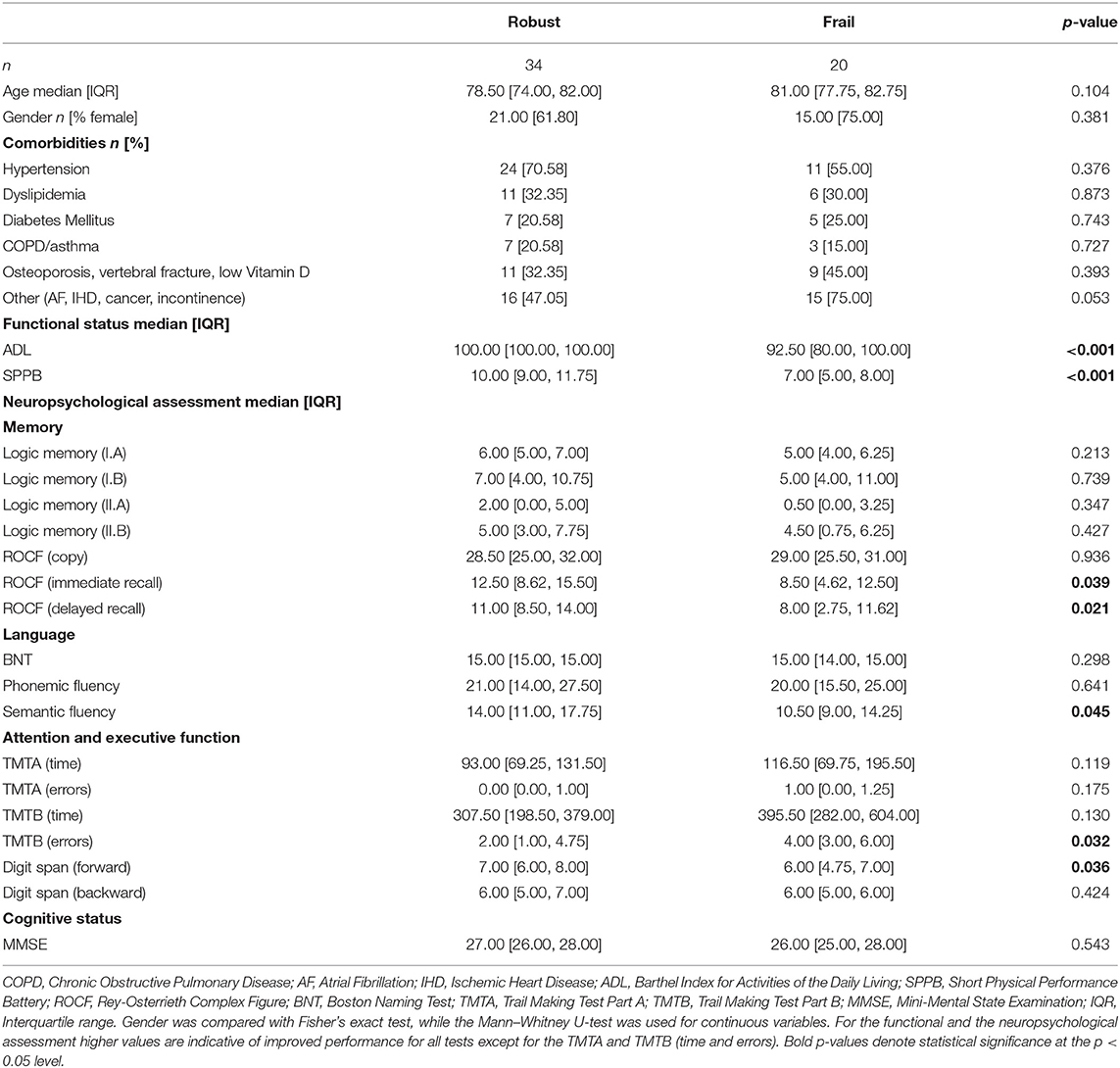
Table 2. Demographic, clinical, functional, and neuropsychological assessment data for each study group (robust and frail).
Among those who were classified as frail, 9 participants (45%) met 3 criteria, 10 (50%) met 4 criteria, and only 1 participant fulfilled the 5 criteria of Fried's phenotype. Concerning the prevalence of each criterion, fatigue (95%) and reduced handgrip strength (85%) were the most common, while involuntary weight loss (25%) was the least prevalent (Table 3).
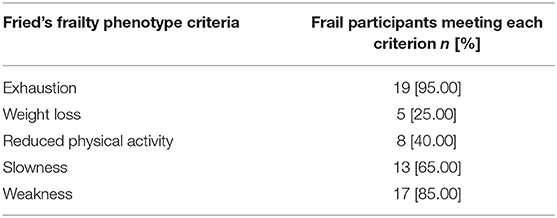
Table 3. Frail participants (number and percentage) meeting each of the criteria of Fried's frailty phenotype.
On another note, evidence of small vessel disease or major axonal decay was not observed in the MRI exams for any of the participants.
Differences in Relative Power
Significant group differences in relative power were found in the classical alpha (mu) and SMR frequency bands. We found no significant group differences in theta nor beta frequencies after multiple comparisons correction.
Alpha (mu) Frequency Band
We found significant group differences in alpha (mu) relative power after multiple comparisons correction (p = 0.02). Frail participants exhibited increased alpha (mu) relative power in comparison to their robust peers. The strongest differences were found in the right hemisphere (RH) affecting posterior parietal areas [e.g., supramarginal gyrus (SMG), angular gyrus (AG), and superior parietal lobe (SPL)], the primary somatosensory cortex, and the superior occipital cortex. Significant bilateral differences involved areas of the primary motor cortex (Figure 2A). These regions comprise those classically associated with traditional generators of mu activity (Pineda, 2005), thus henceforth, we will refer to our results within this range as mu oscillations.
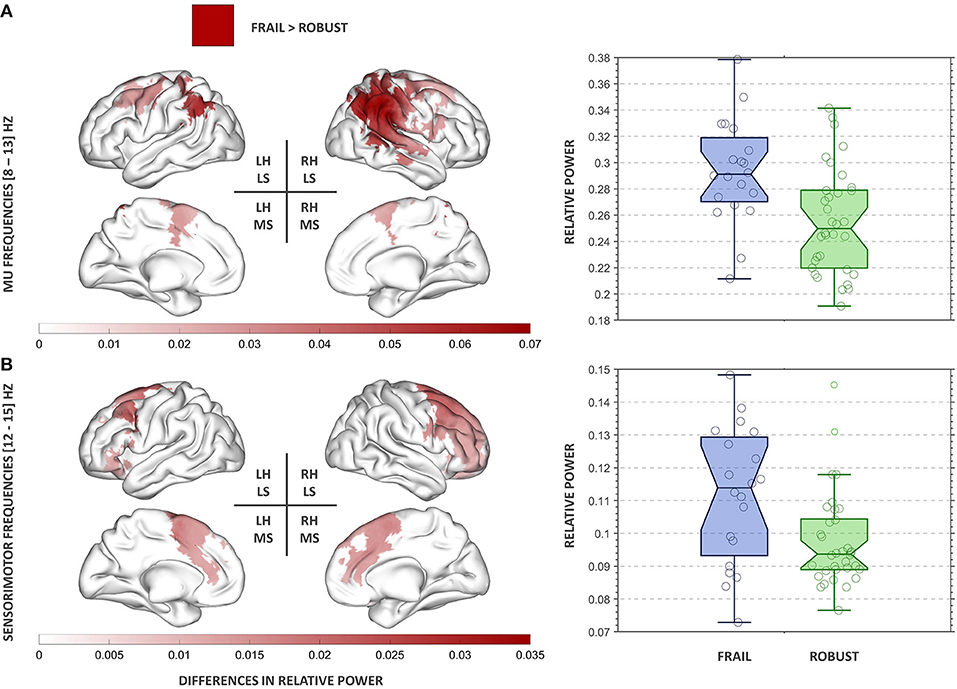
Figure 2. Results of the power spectral analysis in the mu (A) and SMR (B) frequency bands. On the left panel, clusters showing significant group differences in relative power are displayed for each frequency band. The red colormap indicates the strength of the increase in relative power observed in the frail group. On the right panel, the group relative power distributions for each cluster are presented. The boxplots inform of each participant's relative power value, the median, the 95% confidence interval of the median, and the boundaries of the quartiles. RH, right hemisphere; LH, left hemisphere; LS, lateral surface; MS, medial surface.
SMR Frequency Band
We found significant group differences in SMR relative power after multiple comparisons correction (p = 0.03). Frail participants exhibited increased SMR relative power in comparison to their robust peers. The strongest differences were found in frontal areas of the RH, including the premotor cortex, the supplementary motor area, and the paracingulate gyrus (Figure 2B).
Classification Analysis
To assure a valid classification and reduce the likelihood of overfitting using SuperLearner, we split the whole sample of 54 participants into a 2/3 discovery sample (n = 35, 11 frail, 24 robust) and 1/3 validation sample (n = 19, 9 frail, 10 robust). When we applied the algorithm obtained from this ensemble to the testing sample, we obtained an AUC = 0.73 (95% CIDelong = 0.49–0.98) which was borderline significant (p = 0.083). Using 5-fold cross-validation in the training sample the best machine learning classifier was the ensemble learner with an average AUC of 0.655.
Discussion
The comparison of the ongoing oscillatory neural activity of frail and robust individuals revealed significant differences in the mu and SMR frequency bands. Frail individuals exhibited increased relative power across sensorimotor, posterior parietal, and frontal areas predominantly localized in the RH. Additionally, frail individuals demonstrated poorer performance in the executive domain. Moreover, an ensemble learner showed promising results in the ability to correctly discriminate between frail and robust participants using MEG power spectral properties.
The analysis of group differences in relative power revealed highly RH lateralized increases in the oscillatory neural activity of frail participants. Frail participants exhibited increased mu relative power across right posterior parietal areas (e.g., SMG, AG, SPL) expanding to the sensorimotor cortex (precentral and postcentral gyri) and the superior occipital lobe. Group differences were most accentuated in the rSMG. This area of the somatosensory association cortex seems to be involved in several functions, from visual word recognition (Stoeckel et al., 2009) to verbal working memory (Deschamps et al., 2014). Importantly, rSMG seems to be involved in the processing of sensorimotor information. Different fMRI studies have revealed consistent SMG activations in response to proprioceptive-related stimuli (Naito, 2004a; Goble et al., 2012; Ben-Shabat et al., 2015), suggesting that the SMG might be a key region for the central processing of proprioception (Ben-Shabat et al., 2015). This proposing is further supported by lesion studies in post-stroke patients connecting damage to the SMG with persisting difficulties in the percept of limb position and movement (Findlater et al., 2018). These results are particularly meaningful considering the importance of accurate limb representations for the correct processing of motor commands and the carrying out of daily routines. Indeed, some of the physical issues observed in frailty (e.g., reduced gait speed, recurrent falls, decline in daily activities) could draw from deficiencies within internal representations that might challenge motor control in frail individuals.
The aforementioned suggestion is consistent with concurring evidence that connects several of the areas that showed abnormal oscillatory activity in the frail group, with a neural network subserving the representation of internal estimates of the body and its parts. Differences in mu relative power spread from the rSMG onto the rAG, rSPL, and sensorimotor cortex. The importance of the SPL in sensorimotor integration is largely documented, particularly concerning the maintenance of updated body estimates (Wolpert et al., 1998; Buneo and Andersen, 2006; Parkinson et al., 2010). The sensorimotor cortex has been associated with the processing of proprioception and kinesthesia (Naito, 2004a), and specifically with the somatic perception of limb movement (Naito et al., 2002; Naito, 2004b). Additionally, the rAG is thought to engage in further aspects of body representation (Spitoni et al., 2013), motor control (Farrer et al., 2008), and near-space perception (Bjoertomt, 2002). Following this, alterations in the oscillatory activity within this network might underlie deficiencies in proprioceptive-related processes in frail individuals. Different intervention programs have demonstrated positive results after incorporating (or focusing on) proprioceptive training in order to confront some of the physical manifestations of frailty (Sohn and Kim, 2015; Tarazona-Santabalbina et al., 2016; Pérez-Ros et al., 2020). The present study provides electrophysiological evidence to support these approaches. In general terms, the central processing of proprioception has been presented as an RH-dominated mechanism (Naito, 2004a; Goble et al., 2006; Goble and Brown, 2007), which might also explain why oscillatory abnormalities appear to be consistently RH lateralized in frail individuals.
The differences in relative power between frail and robust participants were restrained to the mu and SMR frequency bands. Mu oscillations arise across sensorimotor regions in the absence of movement or movement-related imagery. During movement, mu oscillations are suppressed by the desynchronizing activity of sensorimotor neurons leading to a power reduction in the mu range (Pineda, 2005). Mu suppression is thought to reflect sensorimotor processing within fronto-parietal areas and has been widely studied in the context of the human mirror neuron system (Hobson and Bishop, 2016). The extent up to which movement-related activity suppresses mu synchronization seems to be proportional to the peak power values attained at rest (Van Leeuwen et al., 1978). In consequence, the aberrant mu hypersynchrony observed in frail individuals at rest might negatively impact mu suppression during movement planning and execution. An analogous proposing could apply to SMR oscillations that also occur under circumstances of sustained immobility (Sterman, 1996). SMR activity is maximally detected across brain regions neighboring the motor strip, albeit our results showed a cluster of increased SMR relative power in the frail group spreading from right posterior frontal areas, including the premotor cortex and the supplementary motor area, to the anterior prefrontal cortex. Ample evidence connects these regions with mechanisms of gait (Jor'dan et al., 2017), particularly under demanding conditions [also reliant on neurovascular coupling (Jor'dan et al., 2020)] [e.g., high speed (Suzuki et al., 2004), walking while talking (Holtzer et al., 2011), obstacle negotiation (Mirelman et al., 2017), and precision stepping (Koenraadt et al., 2014)]. Additional functions of the prefrontal cortex include balance control (Mihara et al., 2008) and executive function (Seniów, 2012; Barbas and García-Cabezas, 2016). Prefrontal recruitment seems to increase with age, both during normal and complex walking conditions (Mirelman et al., 2017). In the latter case, higher prefrontal activation has been associated with increased gait variability in older adults, possibly suggesting a compensatory effect in the face of complex motor demands (Mirelman et al., 2017). These deficiencies might be intensified in frail individuals showing abnormal oscillatory neural activity across these regions and generalized walking difficulties. According to recent research, non-invasive interventions in the anterior prefrontal cortex might be used as a means of improving gait mechanisms in such functionally-limited populations (Manor et al., 2018).
Even though frail participants showed a slightly decreased mean performance in several cognitive tests, we did not observe significant differences in their cognitive status. None of the frail participants in our study could classify as suffering cognitive impairment attending to their neuropsychological assessment, even if they demonstrated a significantly reduced performance in some specific neuropsychological tests when compared to their robust peers. Frail participants attained lower scores in measures of executive function such as the TMTB and the Digit Span Test (forward), and also in the recall trials of the ROCF and the Semantic Fluency Test, the latter also connected with processes of executive control (Shao et al., 2014). The reduced executive performance observed in the frail group is admittedly in agreement with previous reports associating poor executive function with an increased risk of functional decline, disability, and frailty (Bell-McGinty et al., 2002; Rosado-Artalejo et al., 2017a). Specifically, performance in the TMT has been acknowledged as an independent predictor of mobility impairment, decline in lower limb function, and mortality in cohorts of older adults (Vazzana et al., 2010). Frail participants also scored worse in the recall (immediate and delayed) trials of the ROCF but not in the copying task, suggesting that lower performance in the ROCF was in this case not mediated by executive function but by additional visuomotor memory deficiencies in the frail group.
This work serves as an explorative approach to increase our understanding of the role of the CNS in the development of frailty from an electrophysiological perspective. As such, it suffers from including only a limited number of participants sourced from a single study setting. This shortcoming makes our cohort a non-representative sample of the general population of older age, however, it also guarantees that frailty was assessed homogeneously in the study. The reduced sample size limited the possibility of adjusting the analyses for every desirable variable (e.g., socio-economic conditions, educational level), nevertheless, the potential bias introduced by these sources should be reduced as participants were recruited from a single hospital that serves a specific neighborhood characterized by very similar socio-economic and educational profiles. Frail and robust participants were comparable in terms of comorbidities and, as expected, functional performance was worse in frail participants. In addition, it is important to mention that our results concerned frail individuals that were classified within Fried's phenotype framework. Consequently, our findings cannot be automatically extrapolated to frail samples classified within a different conceptual framework (i.e., accumulation of deficits or biopsychosocial models) whose definition of frailty incorporates items related to neurological or cognitive deficits explicitly excluded in our study.
As a whole, our findings reveal the presence of abnormalities in the ongoing oscillatory neural activity of frail individuals within well-defined areas of the brain mediating proprioceptive and motor processes. Alterations within these central mechanisms, jointly to further peripheral factors commonly found in frail individuals (e.g., sarcopenia), might contribute to several of the physical manifestations generally recognized as the physical components of frailty (i.e., loss of gait speed, handgrip strength, physical activity, and balance). The sample of our study only included participants that were free of global cognitive impairment. Bearing this, our results suggest that frail individuals that could have been framed under the definition of physical frailty show evidence of brain dysfunction, also in the absence of substantial anatomical injury. Accordingly, the use of frailty as a recognizable clinical entity with different pathophysiological substrates, including alterations in brain function, is in our opinion, most appropriate than its subdivision into specific subtypes. In addition, all the participants were free of ischemic cerebral lesions, another potential, although controversial, pathophysiological factor involving the CNS into generating frailty without cognitive symptoms (Kant et al., 2019). All things considered, our results seem to support the view that the CNS contributes to the unfolding of the so-called physical phenotype of frailty in patients without global cognitive impairment by a mechanism different from ischemic, microvascular lesions.
Data Availability Statement
The datasets used and/or analyzed during the current study are available from the corresponding author on reasonable request.
Ethics Statement
The studies involving human participants were reviewed and approved by University Hospital of Getafe, Getafe, Spain. The patients/participants provided their written informed consent to participate in this study. Written informed consent was obtained from the individual(s) for the publication of any potentially identifiable images or data included in this article.
Author Contributions
IS-M carried out the MEG studies, the analysis of the data, and participated in the writing of the first draft and the final version of the manuscript. SW was involved in the general design of the study, coordinated its implementation, and made substantial contributions to the analyses and writing of the manuscript. DL-S participated in the analyses of the data and the writing of the manuscript. NP, RB, and MV were responsible for the recruitment of the participants, their functional and clinical phenotyping, the design of the psychological assessment, and the clinical groundwork and logistics. EC was involved in interpreting the MRI images. FM, FP, and LR-M were involved in the conception, design, supervision, and analysis of the data. They also participated in the writing of the first draft and the final version of the manuscript. All authors contributed to the article and approved the submitted version.
Funding
This work was supported by the Spanish Ministry of Economy, Industry, and Competitiveness, co-financed by the European Regional Development Funds (RD120001/0043), the Centro de Investigación Biomédica en Red en Fragilidad y Envejecimiento Saludable-CIBERFES (CB16/10/00464), and the project Neurocentro (B2017/BMD-3760) funded by the Community of Madrid. SW was funded by FIS16/02125 from Instituto de Salud Carlos III and 2018-T1/BMD-11226 Talent Attraction Program from the Community of Madrid, Spain - co-financed by European Development Funds (FEDER) Una manera de hacer Europa.
Conflict of Interest
The authors declare that the research was conducted in the absence of any commercial or financial relationships that could be construed as a potential conflict of interest.
References
Ávila-Funes, J. A., Amieva, H., Barberger-Gateau, P., Le Goff, M., Raoux, N., Ritchie, K., et al. (2009). Cognitive impairment improves the predictive validity of the phenotype of frailty for adverse health outcomes: the three-city study. J. Am. Geriatr. Soc. 57, 453–461. doi: 10.1111/j.1532-5415.2008.02136.x
Avila-Funes, J. A., Pelletier, A., Meillon, C., Catheline, G., Periot, O., Treviño-Frenk, I., et al. (2017). Vascular cerebral damage in frail older adults: the AMImage study. J. Gerontol. A Biol. Sci. Med. Sci. 72, 971–977. doi: 10.1093/gerona/glw347
Barbas, H., and García-Cabezas, M. Á. (2016). How the prefrontal executive got its stripes. Curr. Opin. Neurobiol. 40, 125–134. doi: 10.1016/j.conb.2016.07.003
Bell-McGinty, S., Podell, K., Franzen, M., Baird, A. D., and Williams, M. J. (2002). Standard measures of executive function in predicting instrumental activities of daily living in older adults. Int. J. Geriatr. Psychiatry 17, 828–834. doi: 10.1002/gps.646
Benjamini, Y., and Hochberg, Y. (1995). Controlling the false discovery rate: a practical and powerful approach to multiple testing. J. R. Stat. Soc. 57, 289–300. doi: 10.1111/j.2517-6161.1995.tb02031.x
Ben-Shabat, E., Matyas, T. A., Pell, G. S., Brodtmann, A., and Carey, L. M. (2015). The right supramarginal gyrus is important for proprioception in healthy and stroke-affected participants: a functional MRI study. Front. Neurol. 6:248. doi: 10.3389/fneur.2015.00248
Bjoertomt, O. (2002). Spatial neglect in near and far space investigated by repetitive transcranial magnetic stimulation. Brain 125, 2012–2022. doi: 10.1093/brain/awf211
Buchman, A. S., Yu, L., Wilson, R. S., Boyle, P. A., Schneider, J. A., and Bennett, D. A. (2014). Brain pathology contributes to simultaneous change in physical frailty and cognition in old age. J. Gerontol. A Biol. Sci. Med. Sci. 69, 1536–1544. doi: 10.1093/gerona/glu117
Buneo, C. A., and Andersen, R. A. (2006). The posterior parietal cortex: sensorimotor interface for the planning and online control of visually guided movements. Neuropsychologia 44, 2594–2606. doi: 10.1016/j.neuropsychologia.2005.10.011
Collard, R. M., Boter, H., Schoevers, R. A., and Oude Voshaar, R. C. (2012). Prevalence of frailty in community-dwelling older persons: a systematic review. J. Am. Geriatr. Soc. 60, 1487–1492. doi: 10.1111/j.1532-5415.2012.04054.x
Del Brutto, O. H., Mera, R. M., Cagino, K., Fanning, K. D., Milla-Martinez, M. F., Nieves, J. L., et al. (2017). Neuroimaging signatures of frailty: a population-based study in community-dwelling older adults (the atahualpa project). Geriatr. Gerontol. Int. 17, 270–276. doi: 10.1111/ggi.12708
Deschamps, I., Baum, S. R., and Gracco, V. L. (2014). On the role of the supramarginal gyrus in phonological processing and verbal working memory: evidence from rTMS studies. Neuropsychologia 53, 39–46. doi: 10.1016/j.neuropsychologia.2013.10.015
Farrer, C., Frey, S. H., Van Horn, J. D., Tunik, E., Turk, D., Inati, S., et al. (2008). The angular gyrus computes action awareness representations. Cereb. Cortex 18, 254–261. doi: 10.1093/cercor/bhm050
Findlater, S. E., Hawe, R. L., Semrau, J. A., Kenzie, J. M., Yu, A. Y., Scott, S. H., et al. (2018). Lesion locations associated with persistent proprioceptive impairment in the upper limbs after stroke. NeuroImage Clin. 20, 955–971. doi: 10.1016/j.nicl.2018.10.003
Fried, L. P., Tangen, C. M., Walston, J. D., Newman, A. B., Hirsch, C., Gottdiener, J., et al. (2001). Frailty in older adults: evidence for a phenotype. J. Gerontol. A Biol. Sci. Med. Sci. 56, 146–156. doi: 10.1093/gerona/56.3.M146
Friedman, J., Hastie, T., and Tibshirani, R. (2010). regularization paths for generalized linear models via coordinate descent. J. Stat. Softw. 33, 1–22. doi: 10.18637/jss.v033.i01
Garcés, P., López-Sanz, D., Maest,ú, F., and Pereda, E. (2017). Choice of magnetometers and gradiometers after signal space separation. Sensors 17, 1–13. doi: 10.3390/s17122926
García-García, F. J., Gutiérrez-Ávila, G., Alfaro-Acha, A., Amor Andres, M. S., De La Torre Lanza, M. D. L. A., Escribano Aparicio, M. V., et al. (2011). The prevalence of frailty syndrome in an older population from Spain. The toledo study for healthy aging. J. Nutr. Heal. Aging 15, 852–856. doi: 10.1007/s12603-011-0075-8
Goble, D. J., and Brown, S. H. (2007). Task-dependent asymmetries in the utilization of proprioceptive feedback for goal-directed movement. Exp. Brain Res. 180, 693–704. doi: 10.1007/s00221-007-0890-7
Goble, D. J., Coxon, J. P., Van Impe, A., Geurts, M., Van Hecke, W., Sunaert, S., et al. (2012). The neural basis of central proprioceptive processing in older versus younger adults: an important sensory role for right putamen. Hum. Brain Mapp. 33, 895–908. doi: 10.1002/hbm.21257
Goble, D. J., Lewis, C. A., and Brown, S. H. (2006). Upper limb asymmetries in the utilization of proprioceptive feedback. Exp. Brain Res. 168, 307–311. doi: 10.1007/s00221-005-0280-y
Hirsiger, S., Koppelmans, V., Mérillat, S., Liem, F., Erdeniz, B., Seidler, R. D., et al. (2016). Structural and functional connectivity in healthy aging: associations for cognition and motor behavior. Hum. Brain Mapp. 37, 855–867. doi: 10.1002/hbm.23067
Hobson, H. M., and Bishop, D. V. M. (2016). Mu suppression - a good measure of the human mirror neuron system? Cortex 82, 290–310. doi: 10.1016/j.cortex.2016.03.019
Holtzer, R., Mahoney, J. R., Izzetoglu, M., Izzetoglu, K., Onaral, B., and Verghese, J. (2011). fNIRS study of walking and walking while talking in young and old individuals. J. Gerontol. A Biol. Sci. Med. Sci. 66, 879–887. doi: 10.1093/gerona/glr068
Jagust, W., Gitcho, A., Sun, F., Kuczynski, B., Mungas, D., and Haan, M. (2006). Brain imaging evidence of preclinical Alzheimer's disease in normal aging. Ann. Neurol. 54, 673–681. doi: 10.1002/ana.20799
Jor'dan, A. J., Manor, B., Iloputaife, I., Habtemariam, D. A., Bean, J. F., Sorond, F. A., et al. (2020). Diminished locomotor control is associated with reduced neurovascular coupling in older adults. J. Gerontol. A Biol. Sci. Med. Sci. 75, 1516–1522. doi: 10.1093/gerona/glz006
Jor'dan, A. J., Poole, V. N., Iloputaife, I., Milberg, W., Manor, B., Esterman, M., et al. (2017). Executive network activation is linked to walking speed in older adults: functional MRI and TCD ultrasound evidence from the MOBILIZE boston study. J. Gerontol. A Biol. Sci. Med. Sci. 72, 1669–1675. doi: 10.1093/gerona/glx063
Jung, H. W., Kim, S. W., Yoon, S. J., Choi, J. Y., Kim, K., and Il, Kim, C. H. (2014). Associations between frailty, retinal microvascular changes, and cerebral white matter abnormalities in Korean older adults. J. Am. Geriatr. Soc. 62, 2209–2210. doi: 10.1111/jgs.13114
Kant, I. M. J., de Bresser, J., van Montfort, S. J. T., Aarts, E., Verlaan, J. J., Zacharias, N., et al. (2018). The association between brain volume, cortical brain infarcts, and physical frailty. Neurobiol. Aging 70, 247–253. doi: 10.1016/j.neurobiolaging.2018.06.032
Kant, I. M. J., Mutsaerts, H. J. M. M., van Montfort, S. J. T., Jaarsma-Coes, M. G., Witkamp, T. D., Winterer, G., et al. (2019). The association between frailty and MRI features of cerebral small vessel disease. Sci. Rep. 9:11343. doi: 10.1038/s41598-019-47731-2
Kelaiditi, E., Cesari, M., Canevelli, M., Abellan Van Kan, G., Ousset, P. J., Gillette-Guyonnet, S., et al. (2013). Cognitive frailty: rational and definition from an (I.A.N.A./I.A.G.G.) international consensus group. J. Nutr. Heal. Aging 17, 726–734. doi: 10.1007/s12603-013-0367-2
Koenraadt, K. L. M., Roelofsen, E. G. J., Duysens, J., and Keijsers, N. L. W. (2014). Cortical control of normal gait and precision stepping: an fNIRS study. Neuroimage (85 Pt. 1), 415–422. doi: 10.1016/j.neuroimage.2013.04.070
Lammers, F., Zacharias, N., Borchers, F., Mörgeli, R., Spies, C. D., and Winterer, G. (2020). Functional connectivity of the supplementary motor network is associated with Fried's modified frailty score in the elderly. J. Gerontol. A Biol. Sci. Med. Sci. 75, 2239–2248. doi: 10.1093/gerona/glz297
López-Sanz, D., Suárez-Méndez, I., Bernab,é, R., Pasquín, N., Rodríguez-Mañas, L., Maest,ú, F., et al. (2018). Scoping review of neuroimaging studies investigating frailty and frailty components. Front. Med. 5:284. doi: 10.3389/fmed.2018.00284
Maltais, M., de Souto Barreto, P., Perus, L., Mangin, J. F., Grigis, A., Chupin, M., et al. (2020). Prospective associations between diffusion tensor imaging parameters and frailty in older adults. J. Am. Geriatr. Soc. 68, 1050–1055. doi: 10.1111/jgs.16343
Manor, B., Zhou, J., Harrison, R., Lo, O. Y., Travison, T. G., Hausdorff, J. M., et al. (2018). Transcranial direct current stimulation may improve cognitive-motor function in functionally limited older adults. Neurorehabil. Neural Repair 32:788–798. doi: 10.1177/1545968318792616
Maris, E., and Oostenveld, R. (2007). Nonparametric statistical testing of EEG- and MEG-data. J. Neurosci. Methods 164, 177–190. doi: 10.1016/j.jneumeth.2007.03.024
Meyer, D., Dimitriadou, E., Hornik, K., Weingessel, A., and Leisch, F. (2015). e1071: Misc Functions of the Department of Statistics (e1071), TU Wien, 2014. R package version, 1.6-3.
Mihara, M., Miyai, I., Hatakenaka, M., Kubota, K., and Sakoda, S. (2008). Role of the prefrontal cortex in human balance control. Neuroimage 43, 329–336. doi: 10.1016/j.neuroimage.2008.07.029
Mirelman, A., Maidan, I., Bernad-Elazari, H., Shustack, S., Giladi, N., and Hausdorff, J. M. (2017). Effects of aging on prefrontal brain activation during challenging walking conditions. Brain Cogn. 115, 41–46. doi: 10.1016/j.bandc.2017.04.002
Naito, E. (2004a). Dominance of the right hemisphere and role of area 2 in human kinesthesia. J. Neurophysiol. 93, 1020–1034. doi: 10.1152/jn.00637.2004
Naito, E. (2004b). Sensing limb movements in the motor cortex: how humans sense limb movement. Neuroscientist 10, 73–82. doi: 10.1177/1073858403259628
Naito, E., Roland, P. E., and Ehrsson, H. H. (2002). I feel my hand moving: a new role of the primary motor cortex in somatic perception of limb movement. Neuron 36, 979–988. doi: 10.1016/S0896-6273(02)00980-7
Nakamura, A., Cuesta, P., Fernández, A., Arahata, Y., Iwata, K., Kuratsubo, I., et al. (2018). Electromagnetic signatures of the preclinical and prodromal stages of Alzheimer's disease. Brain 141, 1470–1485. doi: 10.1093/brain/awy044
Newman, A. B., Gottdiener, J. S., McBurnie, M. A., Hirsch, C. H., Kop, W. J., Tracy, R., et al. (2001). Associations of subclinical cardiovascular disease with frailty. J. Gerontol. A Biol. Sci. Med. Sci. 56, M158–M166. doi: 10.1093/gerona/56.3.M158
Nolte, G. (2003). The magnetic lead field theorem in the quasi-static approximation and its use for magnetoenchephalography forward calculation in realistic volume conductors. Phys. Med. Biol. 48, 3637–3652. doi: 10.1088/0031-9155/48/22/002
Oostenveld, R., Fries, P., Maris, E., and Schoffelen, J. M. (2011). FieldTrip: open source software for advanced analysis of MEG, EEG, and invasive electrophysiological data. Comput. Intell. Neurosci. 2011:156869. doi: 10.1155/2011/156869
Orme, J., Reis, J., and Herz, E. (1986). Factorial and discriminant validity of the center for epidemiological studies depression (CES-D) scale. J. Clin. Psychol. 42, 28–33. doi: 10.1002/1097-4679(198601)42:1<28::AID-JCLP2270420104>3.0.CO;2-T
Parkinson, A., Condon, L., and Jackson, S. R. (2010). Parietal cortex coding of limb posture: in search of the body-schema. Neuropsychologia 48, 3228–3234. doi: 10.1016/j.neuropsychologia.2010.06.039
Pérez-Ros, P., Vila-Candel, R., and Martínez-Arnau, F. M. (2020). A home-based exercise program focused on proprioception to reduce falls in frail and pre-frail community-dwelling older adults. Geriatr. Nurs. 41, 436–444. doi: 10.1016/j.gerinurse.2020.01.017
Pineda, J. A. (2005). The functional significance of mu rhythms: translating “seeing” and “hearing” into “doing.” Brain Res. Rev. 50, 57–68. doi: 10.1016/j.brainresrev.2005.04.005
Polley, E., Ledell, E., Kennedy, C., and Lendle, S. (2018). Package “SuperLearner”. doi: 10.1093/english/efy032
R Core Team (2017). R: A Language and Environment for Statistical Computing. Vienna: R Foundation Statistical Computing. Available online at: http://www.R-project.org/
Rodríguez-Mañas, L., and Sinclair, A. J. (2014). Frailty: the quest for new domains, clinical definitions and subtypes. Is this justified on new evidence emerging? J. Nutr. Health Aging 18, 92–94. doi: 10.1007/s12603-013-0433-9
Rosado-Artalejo, C., Carnicero, J. A., Losa-Reyna, J., Castillo, C., Cobos-Antoranz, B., Alfaro-Acha, A., et al. (2017a). Global performance of executive function is predictor of risk of frailty and disability in older adults. J. Nutr. Health Aging 21, 980–987. doi: 10.1007/s12603-017-0895-2
Rosado-Artalejo, C., Carnicero, J. A., Losa-Reyna, J., Guadalupe-Grau, A., Castillo-Gallego, C., Gutiérrez-Ávila, G., et al. (2017b). Cognitive performance across 3 frailty phenotypes: toledo study for healthy aging. J. Am. Med. Dir. Assoc. 18, 785–790. doi: 10.1016/j.jamda.2017.04.008
Rosano, C., Sigurdsson, S., Siggeirsdottir, K., Phillips, C. L., Garcia, M., Jonsson, P. V., et al. (2010). Magnetization transfer imaging, white matter hyperintensities, brain atrophy and slower gait in older men and women. Neurobiol. Aging 31, 1197–1204. doi: 10.1016/j.neurobiolaging.2008.08.004
Rosario, B. L., Rosso, A. L., Aizenstein, H. J., Harris, T., Newman, A. B., Satterfield, S., et al. (2016). Cerebral white matter and slow gait: contribution of hyperintensities and normal-appearing parenchyma. J. Gerontol. A Biol. Sci. Med. Sci. 71, 968–973. doi: 10.1093/gerona/glv224
Seidler, R., Erdeniz, B., Koppelmans, V., Hirsiger, S., Mérillat, S., and Jäncke, L. (2015). Associations between age, motor function, and resting state sensorimotor network connectivity in healthy older adults. Neuroimage 108, 47–59. doi: 10.1016/j.neuroimage.2014.12.023
Seniów, J. (2012). Executive dysfunctions and frontal syndromes. Front. Neurol. Neurosci. 30, 50–53. doi: 10.1159/000333407
Shao, Z., Janse, E., Visser, K., and Meyer, A. S. (2014). What do verbal fluency tasks measure? Predictors of verbal fluency performance in older adults. Front. Psychol. 5:772. doi: 10.3389/fpsyg.2014.00772
Sohn, J., and Kim, S. (2015). Falls study: Proprioception, postural stability, and slips. Biomed. Mater. Eng. 26, S693–S703. doi: 10.3233/BME-151361
Spitoni, G. F., Pireddu, G., Cimmino, R. L., Galati, G., Priori, A., Lavidor, M., et al. (2013). Right but not left angular gyrus modulates the metric component of the mental body representation: A tDCS study. Exp. Brain Res. 228, 63–72. doi: 10.1007/s00221-013-3538-9
Sterman, M. B. (1996). Physiological origins and functional correlates of EEG rhythmic activities: implications for self-regulation. Biofeedback Self Regul. 21, 3–33. doi: 10.1007/BF02214147
Stoeckel, C., Gough, P. M., Watkins, K. E., and Devlin, J. T. (2009). Supramarginal gyrus involvement in visual word recognition. Cortex 45, 1091–1096. doi: 10.1016/j.cortex.2008.12.004
Suárez-Méndez, I., Doval, S., Walter, S., Pasquín, N., Bernab,é, R., Gallo, E. C., et al. (2020). Functional connectivity disruption in frail older adults without global cognitive deficits. Front. Med. 7:322. doi: 10.3389/fmed.2020.00322
Suzuki, M., Miyai, I., Ono, T., Oda, I., Konishi, I., Kochiyama, T., et al. (2004). Prefrontal and premotor cortices are involved in adapting walking and running speed on the treadmill: an optical imaging study. Neuroimage 23, 1020–1026. doi: 10.1016/j.neuroimage.2004.07.002
Tarazona-Santabalbina, F. J., Gómez-Cabrera, M. C., Pérez-Ros, P., Martínez-Arnau, F. M., Cabo, H., Tsaparas, K., et al. (2016). A multicomponent exercise intervention that reverses frailty and improves cognition, emotion, and social networking in the community-dwelling frail elderly: a randomized clinical trial. J. Am. Med. Dir. Assoc. 17, 426–433. doi: 10.1016/j.jamda.2016.01.019
Taulu, S., and Hari, R. (2009). Removal of magnetoencephalographic artifacts with temporal signal-space separation: demonstration with single-trial auditory-evoked responses. Hum. Brain Mapp. 30, 1524–1534. doi: 10.1002/hbm.20627
Taylor, H. L., Jacobs, D. R., Schucker, B., Knudsen, J., Leon, A. S., and Debacker, G. (1978). A questionnaire for the assessment of leisure time physical activities. J. Chronic Dis. 31, 741–755. doi: 10.1016/0021-9681(78)90058-9
Van Leeuwen, W. S., Wieneke, G., Spoelstra, P., and Versteeg, H. (1978). Lack of bilateral coherence of mu rhythm. Electroencephalogr. Clin. Neurophysiol. 44, 140–146. doi: 10.1016/0013-4694(78)90260-2
Van Veen, B. D., Van Drongelen, W., Yuchtman, M., and Suzuki, A. (1997). Localization of brain electrical activity via linearly constrained minimum variance spatial filtering. IEEE Trans. Biomed. Eng. 44, 867–880. doi: 10.1109/10.623056
Vazzana, R., Bandinelli, S., Lauretani, F., Volpato, S., Lauretani, F., Di Iorio, A., et al. (2010). Trail making test predicts physical impairment and mortality in older persons. J. Am. Geriatr. Soc. 58, 719–723. doi: 10.1111/j.1532-5415.2010.02780.x
Wolpert, D. M., Goodbody, S. J., and Husain, M. (1998). Maintaining internal representations: the role of the human superior parietal lobe. Nat. Neurosci. 1, 529–533. doi: 10.1038/2245
Keywords: frailty, neuroimaging, magnetoencephalography, electrophysiology, aging
Citation: Suárez-Méndez I, Walter S, López-Sanz D, Pasquín N, Bernabé R, Castillo Gallo E, Valdés M, del Pozo F, Maestú F and Rodríguez-Mañas L (2021) Ongoing Oscillatory Electrophysiological Alterations in Frail Older Adults: A MEG Study. Front. Aging Neurosci. 13:609043. doi: 10.3389/fnagi.2021.609043
Received: 22 September 2020; Accepted: 28 January 2021;
Published: 18 February 2021.
Edited by:
Yang Jiang, University of Kentucky, United StatesReviewed by:
Henning U. Voss, Cornell University, United StatesMario Ulises Pérez-Zepeda, Dalhousie University, Canada
Copyright © 2021 Suárez-Méndez, Walter, López-Sanz, Pasquín, Bernabé, Castillo Gallo, Valdés, del Pozo, Maestú and Rodríguez-Mañas. This is an open-access article distributed under the terms of the Creative Commons Attribution License (CC BY). The use, distribution or reproduction in other forums is permitted, provided the original author(s) and the copyright owner(s) are credited and that the original publication in this journal is cited, in accordance with accepted academic practice. No use, distribution or reproduction is permitted which does not comply with these terms.
*Correspondence: Leocadio Rodríguez-Mañas, bGVvY2FkaW8ucm9kcmlndWV6QHNhbHVkLm1hZHJpZC5vcmc=
†These authors have contributed equally to this work and share first authorship
‡Deceased