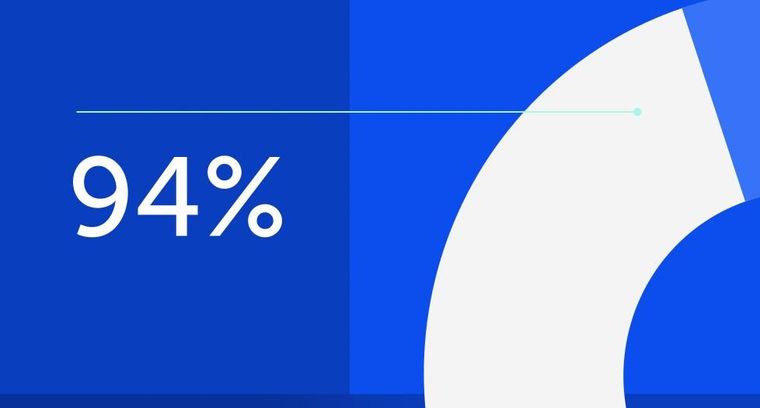
94% of researchers rate our articles as excellent or good
Learn more about the work of our research integrity team to safeguard the quality of each article we publish.
Find out more
ORIGINAL RESEARCH article
Front. Aging Neurosci., 10 February 2021
Sec. Neurocognitive Aging and Behavior
Volume 13 - 2021 | https://doi.org/10.3389/fnagi.2021.597455
This article is part of the Research TopicNeuroimaging Biomarkers and Cognition in Alzheimer’s disease SpectrumView all 32 articles
Background: Subjective cognitive decline (SCD) and amnestic mild cognitive impairment (aMCI) are regarded as part of the pre-clinical Alzheimer's disease (AD) spectrum. The insular subregional networks are thought to have diverse intrinsic connectivity patterns that are involved in cognitive and emotional processing. We set out to investigate convergent and divergent altered connectivity patterns of the insular subregions across the spectrum of pre-clinical AD and evaluated how well these patterns can differentiate the pre-clinical AD spectrum.
Method: Functional connectivity (FC) analyses in insular subnetworks were carried out among 38 patients with SCD, 56 patients with aMCI, and 55 normal controls (CNs). Logistic regression analyses were used to construct models for aMCI and CN, as well as SCD and CN classification. Finally, we conducted correlation analyses to measure the relationship between FCs of altered insular subnetworks and cognition.
Results: Patients with SCD presented with reduced FC in the bilateral cerebellum posterior lobe and increased FC in the medial frontal gyrus and the middle temporal gyrus. On the other hand, patients with aMCI largely presented with decreased FC in the bilateral inferior parietal lobule, the cerebellum posterior lobe, and the anterior cingulate cortex, as well as increased FC in the medial and inferior frontal gyrus, and the middle and superior temporal gyrus. Logistic regression analyses indicated that a model composed of FCs among altered insular subnetworks in patients with SCD was able to appropriately classify 83.9% of patients with SCD and CN, with an area under the receiver operating characteristic (ROC) curve (AUC) of 0.876, 81.6% sensitivity, and 81.8% specificity. A model consisting of altered insular subnetwork FCs in patients with aMCI was able to appropriately classify 86.5% of the patients with aMCI and CNs, with an AUC of 0.887, 80.4% sensitivity, and 83.6% specificity. Furthermore, some of the FCs among altered insular subnetworks were significantly correlated with episodic memory and executive function.
Conclusions: Patients with SCD and aMCI are likely to share similar convergent and divergent altered intrinsic FC patterns of insular subnetworks as the pre-clinical AD spectrum, and presented with abnormalities among subnetworks. Based on these abnormalities, individuals can be correctly differentiated in the pre-clinical AD spectrum. These results suggest that alterations in insular subnetworks can be utilized as a potential biomarker to aid in conducting a clinical diagnosis of the spectrum of pre-clinical AD.
Subjective cognitive decline (SCD) and amnestic mild cognitive impairment (aMCI), which are part of the clinical continuum of dementia progression and the spectrum of pre-clinical Alzheimer's disease (AD), are well-established risk factors for the development of AD (van der Flier et al., 2004; Stewart et al., 2008; Striepens et al., 2010; Scheef et al., 2012; Jessen et al., 2014b, 2020; Buckley et al., 2016; Xue et al., 2019). Many studies have suggested that individuals with SCD go on to develop aMCI, and then AD (Jessen et al., 2014b; Berger-Sieczkowski et al., 2019). Therefore, our in-depth understanding of neuroimaging-based continual pathology across the spectrum of pre-clinical AD can help assist in the development of a new method in pre-clinical AD diagnosis and treatment.
Neuroimaging can help reveal the pathological mechanisms behind AD progression. The insula and its network connectivity are anatomically connected to the limbic and frontal-parietal-temporal lobes and are functionally involved in a higher-order cognitive and emotional processing (Allen et al., 2008; Naqvi and Bechara, 2010), which has a significant role in AD progression (Fan et al., 2008; Guo et al., 2012). Numerous studies have reported the presence of insular gray matter atrophy in AD (Guo et al., 2012), as well as altered insular activities and networks in aMCI (Xie et al., 2012; Lin et al., 2017). In particular, studies have demonstrated that insula atrophy can help correctly distinguish patients with AD from normal controls (CNs; Fan et al., 2008; Guo et al., 2012). Therefore, it is reasonable to hypothesize that the insula is an extremely vulnerable area, as well as a critical hub, in delaying the progression of the pre-clinical spectrum of AD, including SCD and aMCI.
Recently, several studies have consistently suggested that the insula demonstrates heterogeneity and may be divided into the ventral anterior insula (vAI), the dorsal anterior insula (dAI), and the posterior insula (PI) (Deen et al., 2011; Peng et al., 2018; Lu et al., 2020). These insula subregional networks have different roles in information processing (Taylor et al., 2009) and therefore, have distinct intrinsic connectivity patterns (Deen et al., 2011; Peng et al., 2018; Lu et al., 2020). Studies have demonstrated that patients with aMCI and AD present with distinct disruption in the connectivity of insular subregions (Xie et al., 2012; Liu et al., 2018; Lu et al., 2020), which is associated with episodic memory deficits (Xie et al., 2012). Increasing evidence indicates that insular subregional networks can reveal neuroimaging-based continuum pathology across the spectrum of pre-clinical AD in a sensitive and specific manner. However, there is still a lack of knowledge with regards to altered network connectivity patterns of the insular subregions across the spectrum of pre-clinical AD (SCD and aMCI), as well as its relationship with cognition. In particular, it is unclear whether altered connectivity patterns of the insula subregions can help distinguish the spectrum of pre-clinical AD.
We conducted this study to identify altered intrinsic connectivity patterns of the insular subregions across the spectrum of pre-clinical AD. After identification of intrinsic connectivity pathology, we further evaluated how well this can be used to distinguish the spectrum of pre-clinical AD. Finally, we hypothesized that patients with SCD and aMCI present with different altered intrinsic connectivity patterns of the insular subregions. Additionally, we further hypothesized that integration of altered intrinsic functional connectivity (FC) can accurately distinguish individuals in the spectrum of pre-clinical AD.
The data used in this study were acquired from our in-home database, the Nanjing Brain Hospital-Alzheimer's Disease Spectrum Neuroimaging Project (NBH-ADsnp) (Nanjing, China), which is continuously updated. The relevant information obtained from the NBH-ADsnp is summarized in Supplementary Methods S.1. This study was granted approval by the responsible Human Participants Ethics Committee of the Affiliated Brain Hospital of Nanjing Medical University (Nos. 2018-KY010-01 and 2020-KY010-02), located in Nanjing, China. Signed informed consent was obtained from each participant.
Initially, 460 individuals, who were of Han Chinese origin and right-handed, were enrolled in the NBH-ADsnp database from the memory clinic of hospitals and local communities through the use of advertising and broadcasting. All patients with SCD (n = 116) and CNs (n = 190) were recruited from local communities, while aMCI subjects (n = 154) were enrolled from both memory clinics and local communities. According to our criteria, a total of 169 elderly individuals participated in this study. Among them, two CNs, two patients with SCD, and 16 patients with aMCI were excluded due to excessive head movement during MRI scan (defined as cumulative translation or rotation of >3.0 mm or 3.0° and individual time points with mean frame-wise displacement (FD) of >0.5 mm and scans with >50% volumes removed; Brady et al., 2019), and incomplete or missing MRI data. The remaining 55 CNs, 38 patients with SCD, and 56 patients with aMCI were enrolled in the final study. The inclusion and exclusion criteria were outlined in accordance with previously published studies (Jessen et al., 2014a, 2020; Chen et al., 2015a, 2016a, 2019a; Dubois et al., 2016; Xue et al., 2019). The diagnosis was performed independently by three neuropsychiatric physicians (Dr. Jiang Rao, Fuquan Zhang, and Xiangrong Zhang) who had 12–15 years of experience. Any disagreement between the three neuropsychiatric physicians was solved by reaching a consensus through group discussions.
The inclusion criteria of SCD subjects included the published SCD research criteria proposed by the Subjective Cognitive Decline Initiative (SCD-I; Jessen et al., 2014a). The detailed inclusion criteria were described in our previously published study (Xue et al., 2019), and is as follows: (a) self-reported persistent memory decline, confirmed by the caretaker or a family member; (b) a Subjective Cognitive Decline Questionnaire (SCD-Q) score > 5 (Hao et al., 2017; Yan et al., 2018; Cedres et al., 2019); (c) performance within a normal range on the Mini-Mental State Examination (MMSE) and the Montreal Cognitive Assessment (MoCA) (adjusted for age and education); (d) Clinical Dementia Rating (CDR) = 0; (e) subjects must be between the ages of 50 and 80; and (f) subjects must have Hamilton Depression Scale (HAMD) scores of < 7.
The inclusion criteria of aMCI subjects included the diagnostic criteria that were previously defined by Petersen et al. (1999) and the revised consensus standards reported by Winblad et al. (2004). The detailed inclusion criteria have been previously described in our published studies (Chen et al., 2016b, 2019a; Xue et al., 2019) and includes: (a) complaint of loss of memory, preferably corroborated by an informant or the subject for more than 3 months; (b) objective memory impairment (adjusted for age and educational level); (c) normal general cognitive function with MMSE score ≥ 24; (d) no or minimal impairment in daily life activities; (e) CDR = 0.5; (f) subjects must be between the ages of 50 and 80; (g) absence of dementia symptoms not sufficient to meet the criteria of the National Institute of Neurological and Communicative Disorders and Stroke or the AD and Related Disorders Association criteria for AD; and (h) subjects must have HAMD scores of < 7.
The inclusion criteria of CN were as follows: (a) no complaints of loss of memory; (b) normal cognitive performance (matched for age and education); (c) CDR = 0; (d) MMSE ≥ 26; (e) subjects must be between the ages of 50 and 80 (Chen et al., 2019b; Xue et al., 2019); and (f) subjects must have a HAMD score of < 7.
Detailed exclusion criteria for all subjects were described in our previously published studies (Chen et al., 2016b, 2019a; Xue et al., 2019), and included (a) past history of stroke (modified Hachinski Ischemic Scale score > 4), alcoholism, head injury, brain tumors, Parkinson's disease, epilepsy, encephalitis, major depression (excluded by HAMD), or other neurological or psychiatric diseases (excluded by clinical assessment and case history); (b) diagnosis of a major medical illness (i.e., cancer, anemia, thyroid dysfunction, syphilis, or HIV); (c) severe loss of vision or hearing; (d) inability to complete neuropsychological tests or a contraindication for MRI, and (e) T2-weighted MRI displaying major changes in white matter (WM), infarction, or additional lesions, as determined by the scan analysis done by two experienced radiologists. None of the subjects used any medications.
All participants underwent a standardized clinical evaluation protocol, including sequencing, demographic inventory, medical history, neurological and mental status examination, and MRI scan. The neuropsychologic tests were conducted by two different neurologists (Dr. Jiang Rao and Dr. Chen Xue) who had 3–5 years of experience. Specific demographics and neuropsychological characteristics of each group are provided in Table 1.
Table 1. Demographics, clinical measures, and head rotation parameters of aMCI, SCD, and CN subjects.
Neuropsychological assessments were similar to those used in our previous studies (Chen et al., 2015a, 2016a, 2019a,b; Xue et al., 2019). These assessments were utilized for evaluating the general cognitive function, episodic memory, speed of information processing, executive function, and visuospatial function. Details regarding each of the assessments are listed in Supplementary Methods.
The detailed parameters of MRI acquisition of the NBH-ADsnp are summarized in Supplementary Methods. Imaging analysts were blinded to the status of diagnosis.
We utilized MATLAB2015b and DPABI software (Yan et al., 2016) to preprocess all functional MRI (fMRI) data. The image processing procedure was conducted as previously described (Yan et al., 2013) and is summarized in Supplementary Methods. In brief, the first 10 time-points were removed, and the data were adjusted for time and motion effects with several nuisance variables [Friston 24-parameter model, with WM, cerebrospinal fluid (CSF), global signals and the linear trend removed; Ashburner and Friston, 2009]. Then, the functional images were spatially normalized, smoothed, and temporal band-pass filtered. The voxels within a group gray matter (GM) mask created by DARTEL were used for further analyses.
Structural MRI analysis was carried out during fMRI data preprocessing using the DPABI image processing software. First, we manually reoriented and shifted the structural images to define the anterior commissure as the origin. Next, we used the DARTEL technique for normalization and segmentation, which divided the structural images into GM, WM, and CSF (Almairac et al., 2018), and created a group-specific GM (functional connection analysis was restricted to voxels within the GM mask) and WM template. Then, the native and DARTEL versions were provided for GM, WM, and CSF tissues, and native versions were utilized to compute the global intracranial volumes (ITV).
Given that significant GM atrophies that are present within patients with SCD (Scheef et al., 2012) and aMCI (Trzepacz et al., 2013; Chen et al., 2015a,b, 2020), the anatomical differences between each group may affect the FCs of insular subregions. In order to investigate this issue, we computed ITV based on native GM, WM, and CSF in CN, SCD, and aMCI groups through the use of in-home MATLAB codes. We explored the differences among each group on the FC of insular subnetworks using ITV as an additional covariate in the general linear model (GLM) analysis.
We used three different approaches to control for head motion effect, both at an individual and group level. First, we excluded CNs and patients with SCD and aMCI that had excessive head motion (defined as cumulative translation or rotation >3.0 mm or 3.0°). Next, a Friston 24-parameter model was applied for regressing out the effects of head motion from the realigned data (Friston et al., 1996). Then, a “scrubbing” procedure was performed to scrub frames (volumes) that had an excessively high whole-brain root mean square (RMS) signal change over time in the preprocessed fMRI data for each participant (Sheline et al., 2010; Power et al., 2012; Van Dijk et al., 2012). Furthermore, any volume with an FD that was > 0.2 mm as nuisance covariates was regressed out, and scans with 50% of volumes were removed and discarded, as previously described in a study (Brady et al., 2019). Overall, two CNs, two patients with SCD, and 16 patients with aMCI were excluded on the account of excessive head movement. There were no significant changes with regards to between-group differences found in quality assurance parameters of head motion in qualified subjects (Table 1).
In order to ensure reproducibility, test-retest reliability, and replicability using the fMRI metrics, we conducted a strict multiple comparison correction (Chen et al., 2018). That is, statistical maps were set with a threshold applying the permutation test with Threshold-Free Cluster Enhancement (TFCE; Smith and Nichols, 2009) and the family-wise error (FWE), as was implemented in DPABI (Yan et al., 2016).
For this study, we created six 6-mm radius spherical seeds. Our definition of insular subregions was in accordance with recent studies (Deen et al., 2011; Peng et al., 2018; Lu et al., 2020). Essentially, the insula was separated into six subregions, which included the left and right bilateral ventral anterior insula (L-vAI and R-vAI), the left and right bilateral dorsal anterior insula (L-dAI and R-dAI), and the left and right bilateral posterior insula (L-PI and R-PI) (Peng et al., 2018; Lu et al., 2020). Supplementary Table 1 details the Montreal Neurological Institute (MNI) seed coordinates.
For each participant, we extracted the average time courses for all voxels within each insula subregional seed and used that as the reference time course. Next, we conducted voxel-wise cross-correlation analysis between the averaged time courses of all voxels within the insular subregional seed, as well as each voxel in the remainder of the entire brain within the group-specific GM mask. Finally, we increased the normality of correlation coefficients, and a Fisher's z-transformation analysis was performed.
ANOVA and chi-square tests were conducted for a comparison of differences of the demographic data, clinical measures, and head rotation parameters among the SCD, aMCI, and CN subjects. Notably, in order to improve the statistical power of the study, we applied a re-sampling method of stationary bootstrap (10,000 bootstrap samplings) to obtain significance.
We also set out to improve statistical power by diminishing random variability, as was done in a previous study (Chen et al., 2016b). This was done by compositing neuropsychological assessments into four cognitive domains (episodic memory, information processing speed, executive function, and visuospatial function), and the raw scores were then transformed into four composite Z scores. The details of raw and composite scores for the neuropsychological tests are outlined in Supplementary Table 2.
Using GLM analysis, we evaluated the differences of the FCs of insula subregions among subjects with aMCI, SCD, and CN (1,000 permutations, TFCE-corrected; p < 0.001 and cluster size > 227 mm3). Then, we constructed a mask based on the different brain regions. Comparisons between any two groups (i.e., CN vs. SCD, CN vs. aMCI) were performed under this mask after adjusting for age, sex, education, ITV, and mean FD (1,000 permutations, TFCE-FWE-corrected p < 0.05, and cluster size > 227 mm3).
In order to further identify the FC patterns of insular subregions, closely related to patients with SCD and aMCI, we applied an approach based on binary logistic regression analysis using alterations in the identified ROIs as biomarkers to evaluate how well these can help distinguish patients with SCD or aMCI from CN subjects. The receiver operating characteristic (ROC) curve was applied to determine the classification power of this model, as well as to assess its accuracy, sensitivity, and specificity.
In order to empirically verify the behavioral significance of altered FC within insular subnetworks in patients with SCD and aMCI, a Pearson's correlation analysis was performed to calculate the relationship between abnormal FC patterns of insula subregions, cognition, and clinical measure (HAMD scores) after adjusting for age, sex, and education. Furthermore, we assessed the significance of these correlation analyses through the use of a leave-one-out cross-validation procedure in 10,000 Monte-Carlo simulation tests to measure how often these results would be expected by chance in a study of multiple comparisons and a re-sampling method of stationary bootstrap (10,000 bootstrap samplings) in order to obtain significance.
We found no significant differences with regard to age and gender across each of the three groups (p > 0.05; Table 1). In comparison with CNs, patients with aMCI had significantly decreased education level (p < 0.05), while patients with SCD were not significantly different. In comparison to CNs, patients with SCD only showed significantly increased SCD-Q and HAMD scores, while patients with aMCI exhibited significantly reduced MMSE, MoCA, and MDRS scores, higher SCD-Q and HAMD scores, and a significant decline in executive function, episodic memory, information processing speed, and visuospatial cognition (p < 0.05).
In the left vAI subnetwork, in comparison to CNs, patients with SCD displayed reduced FC in the left cerebellum posterior lobe and increased FC in the bilateral medial frontal gyrus (MFG), the right middle temporal gyrus (MTG), and the right inferior frontal gyrus (orbital part) (IFGorb) (PTFCE−FWE < 0.05, cluster size > 227 mm3) (Figure 1A, Table 2). Additionally, in the left dAI subnetwork, compared to CNs, patients with SCD showed decreased FC in the bilateral cerebellum posterior lobe and the left cerebellum anterior lobe, with increased FC in the left MTG (PTFCE−FWE < 0.05, cluster size > 227 mm3) (Figure 1B, Table 2). Furthermore, in the right PI subnetwork, patients with SCD exhibited increased FC in right MTG in comparison with CNs (PTFCE−FWE < 0.05, cluster size > 227 mm3) (Figure 1C, Table 2).
Figure 1. Insular subnetwork functional connectivity in patients with SCD compared to CNs. (A) The different brain regions of the FC of the left vAI subregion between CN and SCD. A bar chart indicating the quantitative comparison of functional connectivity between these regions. (B) FC of left dAI subregion between CN and SCD. A bar chart indicating the quantitative comparison of FC between these regions. (C) FC of the right PI subregion between CN and SCD. A bar chart indicating the quantitative comparison of FC between these regions. *PTFCE−FWE < 0.05. Note: only subnetworks that show significant differences in between-group comparisons are shown here. All results are displayed after adjusting for age, sex, education, ITV, and FD. A threshold of p < 0.05 was applied, and TFCE-FWE correction with cluster size >270 mm3. FC, functional connectivity; CN, normal control; SCD, subjective cognitive decline; L-vAI, left ventral anterior insula; L-dAI, left dorsal anterior insula; R-PI, right posterior insula; TFCE, threshold-free cluster enhancement; FEW, family-wise error; ITV, intracranial volume; FD, frame-wise displacement; CERpos.L, left cerebellum posterior lobe; CERpos.R, right cerebellum posterior lobe; MTG.R, right middle temporal gyrus; IFGorb.R, right inferior frontal gyrus, orbital part; MFG.R, right medial frontal gyrus; MFG.L, left medial frontal gyrus; MTG.L, left middle temporal gyrus.
Table 2. Comparisons of functional connectivity of insular subregions among aMCI, SCD, and CN subjects.
In the left vAI subnetwork, compared to CNs, patients with aMCI exhibited decreased FC in bilateral inferior parietal lobule (IPL) and increased FC in bilateral MFG, and right, middle, and superior temporal gyrus (STG; PTFCE−FWE < 0.05, cluster size > 227 mm3) (Figure 2A, Table 2). In addition, in the left dAI subnetwork, compared with CNs, patients with aMCI showed decreased FC in the bilateral cerebellum posterior lobe, the left cerebellum anterior lobe, and the bilateral anterior cingulate cortex (ACC) (PTFCE−FWE < 0.05, cluster size > 227 mm3) (Figure 2B, Table 2). Finally, in the right PI subnetwork, in comparison with CNs, patients with aMCI displayed increased FC in right IFGrob and IFGtri (triangular part) (PTFCE−FWE < 0.05, cluster size > 227 mm3) (Figure 2C, Table 2). All results were adjusted for age, sex, education, ITV, and FD.
Figure 2. Insular subnetwork functional connectivity in patients with aMCI compared to CNs. (A) FC of left vAI subregion between CN and aMCI patients. A bar chart indicating the quantitative comparison of FC between these regions. (B) Functional connectivity of the left dAI subregion between CN and aMCI patients. A bar chart indicating the quantitative comparison of FC between these regions. (C) FC of right PI subregion between CN and aMCI patients. A bar chart indicating the quantitative comparison of FC between these regions. *PTFCE−few < 0.05. Note: only the subnetworks that show between-group differences are shown here. All results are displayed after adjusting for age, sex, education, ITV, and FD. A threshold of p < 0.05 was applied, with a TFCE-FWE correction with cluster size > 270 mm3. FC, functional connectivity; CN, normal controls; aMCI, amnestic mild cognitive impairment; L-vAI, left ventral anterior insula; L-dAI, left dorsal anterior insula; R-PI, right posterior insula; TFCE, threshold-free cluster enhancement; FEW, family-wise error; ITV, intracranial volume; FD, frame-wise displacement; IPL.L, left inferior parietal lobule; IPL.R, right inferior parietal lobule; STG.R, right superior temporal gyrus; MTG.R, right middle temporal gyrus; MFG. L/R, left/right medial frontal gyrus; ACC.L/R, anterior cingulate cortex; CERpos.L, left cerebellum posterior lobe; CERpos/ant.L, left cerebellum posterior lobe/cerebellum anterior lobe; IFGorb.R, right inferior frontal gyrus, orbital part; IFGtri.R, right inferior frontal gyrus, triangular part.
However, it is important to note that other insular subnetworks did not show significant differences among CN, SCD, and aMCI subjects.
Binary logistic regression analyses revealed that the best-fitting model that combined altered insular subnetwork connectivity in patients with SCD was able to correctly classify 83.9% of SCD and CN subjects (χ2 = 66.239, p < 0.001; Nagelkerke R2 = 0.777). The ROC curve of each altered insular subnetwork connectivity is presented in Figure 3A. The best-fitting model for this analysis had an area under the ROC curve (AUC) of 0.876, with 81.6% sensitivity and 81.8% specificity.
Figure 3. Classification of individuals as SCD vs. CN and aMCI vs. CN using an MRI-based classifier. (A) ROC curve showcasing the classification power using an MRI-based “classifier” of SCD from CN. (B) ROC curve presetting the classification power using MRI-based “classifier” of aMCI from CN. Note: the values of AUC, sensitivity, and specificity are displayed in the lower right region of the figure. The combined line reflects the combination of indices of abnormal insular subnetwork functional connectivity. CN, normal control; SCD, subjective cognitive decline; aMCI, amnestic mild cognitive impairment; AUC, area under the ROC curve; ROC, receiver operating characteristic; vAI.L, left ventral anterior insula; dAI.L, left dorsal anterior insula; PI.R, right posterior insula; CERpos.L, left cerebellum posterior lobe; CERpos.R, right cerebellum posterior lobe; MTG.R, right middle temporal gyrus; IFGorb.R, right inferior frontal gyrus, orbital part; MFG.R, right medial frontal gyrus; MFG.L, left medial frontal gyrus; MTG.L, left middle temporal gyrus; IPL.L, left inferior parietal lobule; IPL.R, right inferior parietal lobule; STG.R, right superior temporal gyrus; ACC.L/R, anterior cingulate cortex; CERpos/ant.L, left cerebellum posterior lobe/cerebellum anterior lobe; IFGtri.R, right inferior frontal gyrus, triangular part.
Next, using binary logistic regression analyses, we found that the best-fitting model combining altered insular subnetwork connectivity in patients with aMCI was able to correctly classify 86.5% of aMCI and CN subjects (χ2 = 71.785, p < 0.001; Nagelkerke R2 = 0.635). The ROC curve of each altered insular subnetwork connectivity is displayed in Figure 3B. The best-fitting model had an AUC of 0.887, with 80.4% sensitivity and 83.6% specificity.
Functional connectivity between the left vAI and left cerebellum posterior lobe in patients with SCD was negatively correlated with episodic memory score (r = −0.357, p = 0.028) (Figure 4A). Furthermore, FC between the left vAI and left IPL within patients with aMCI was positively correlated with episodic memory score (r = 0.292; p = 0.034), while FC between left vAI and right STG (r = −0.348; p = 0.011), and FC between right PI and right IFGtri was negatively correlated with executive function scores (r = −0.388, p = 0.004) (Figures 4B–D). Furthermore, we did not find any correlation between depression (HAMD scores) and changes in FC in patients with SCD and aMCI (p > 0.05).
Figure 4. Relationship between abnormal insular subnetwork functional connectivity and cognition in patients with SCD and aMCI. (A) Relationship between the insular subnetwork FC and cognition in patients with SCD. (B–D) Relationship between the insular subnetwork FC and cognition in patients with aMCI. FC, functional connectivity; CN, normal control; SCD, subjective cognitive decline; aMCI, amnestic mild cognitive impairment; vAI.L, left ventral anterior insula; PI.R, right posterior insula; CERpos.L, left cerebellum posterior lobe; IPL.L, left inferior parietal lobule; STG.R, right superior temporal gyrus; IFGtri.R, right inferior frontal gyrus, triangular part; EM, episodic memory; EF, executive function.
To the best of our knowledge, this is the first study to evaluate altered FC patterns of insular subnetworks in the pre-clinical spectrum of AD, and investigate the relationship between altered FC and behavioral significance. Binary logistic regression analyses were further applied for the classification of pre-clinical AD stages. According to our study results, we discovered that altered FC, if found, were mainly in overlapping areas among insular subnetworks (vAI, dAI, and PI) (Nomi et al., 2018), part of which is correlated with the abnormal cognition. Nonetheless, similarities and differences do exist between the two pre-clinical AD stages, and this utilization of abnormalities is able to precisely distinguish the spectrum of pre-clinical AD. Taken together, our results could imply that altered FCs within insular subnetworks may act as a biomarker for prompt detection, intervention, and treatment for pre-clinical AD.
We detected reduced FC within the L-vAI and L-dAI of cerebellum posterior lobe in patients with SCD compared to CN subjects. Furthermore, early studies discovered that the cerebellum posterior lobe contributes to episodic memory coding (Fliessbach et al., 2007). Additionally, altered FC within this area is closely related to abnormal episodic memory in patients with aMCI (Bai et al., 2011). In accordance with earlier observations, we identified a negative relationship between altered FCs of vAI in the cerebellum posterior lobe and episodic memory. With a gradual deterioration of FC, the episodic memory scores of patients with SCD turn out to increase. One possible explanation for this phenomenon is that while the connection between the cerebellum and insula gradually collapses, the compensatory brain regions (i.e., IFG, MFG, and MTG) become abnormally activated. These excessive intrinsic FCs contribute to transiently improved clinical performance. Notably, a dysfunction in episodic memory is thought to be a typical syndrome of aMCI (Seo and Choo, 2016). Furthermore, apart from aMCI, altered FC in the cerebellum was found to be highly correlated with deteriorated episodic memory performance of patients with SCD. This result implies that SCD may share some similarities in neuronal deterioration with aMCI, and may actually represent as a prodromal aMCI stage.
In comparison to subjects with CN, patients with aMCI presented with reduced FCs of the L-vAI in IPL and in the cerebellum posterior lobe and ACC of L-dAI. IPL is known to be a region of heterogeneity that is associated with diverse brain networks, including DMN, salience network (SAN), and executive control network (ECN) (Wang et al., 2015). IPL has a role in multiple functions, such as the executive and salience effect (Uddin et al., 2011). Moreover, Kwok and Macaluso have demonstrated that IPL may be modulated under the episodic memory retrieval process, which indicates a tight relationship with episodic memory (Kwok and Macaluso, 2015). In addition, Xie et al. (2012) have reported that aberrant insular networks may play a key role in the processes of episodic memory. Consistently, we detected a positive correlation between FCs of L-vAI in IPL and episodic memory. With a reduction in FC, the severity of damage exceeds the degree of compensation, which leads to irreversible disrupted episodic memory performance. This implies decreased neuroactivity between IPL and L-vAI, which eventually causes worsened clinical performance. ACC is engaged in various processes, which includes attention, memory, and emotion (Fillinger et al., 2018). To date, numerous studies have demonstrated strong functional or structural connections between the dorsal ACC and dAI (Chang et al., 2013; Wiech et al., 2014; Ghaziri et al., 2017). Consistently, our result reveals a functional relationship between ACC and dAI, which indicates a potential deterioration mechanism of aMCI.
Convergent altered intrinsic FC remains in MFG, STG, and MTG under L-vAI, and in IFG under R-PI. MFG and MTG both belong to DMN and are relevant in cognitive processes. The abnormally increased FCs of patients with aMCI between these two regions and L-vAI implies possible recruitment of activated DMN for an early functional complement. Additionally, STG is in charge of primary and secondary auditory and lingual processes (Luo et al., 2018). Furthermore, Mwansisya et al. (2017) have reported convergent neuronal abnormalities within activated STG, which is closely related to executive dysfunction in schizophrenia. Consistently, we discovered a negative relationship between executive function and altered FC of L-vAI in STG, which validates an intimate relationship between STG and executive dysfunction of patients with aMCI. These results indicate that aMCI (or AD) may share similarities with regard to psychopathology and compensatory mechanisms.
Subjective cognitive decline and aMCI share both similarities and differences in altered FC of insular subnetworks. To summarize, we found that there were compensatory brain regions in patients with SCD, including MFG, MTG, and IFG. In addition, we also discovered that the compensatory phenomenon exists in STG in patients with aMCI. Furthermore, altered FC in MTG was detected across all three insular subnetworks, suggesting that STG may represent the main compensatory region in patients with SCD. However, compensation in patients with aMCI is more prominent, which further reflects the adaptation of deepened atrophy. With regards to the distribution of affected regions, IFG and MFG belong to the frontal regions while MTG and STG belong to the temporal regions. With the ever-expanding influence of neurotoxicity, researchers have discovered the presence of excessive strengthened intrinsic neuroactivities among the frontal and temporal regions (Qi et al., 2010; Gour et al., 2011; Yang et al., 2018). Combined with our results, we infer that the frontal and temporal regions may serve as the major and the earliest regions that regulate compensatory activities in pre-clinical AD stages. These results suggest a tendency to recruit advanced brain regions to compensate for early functional loss.
In addition, our study showed no insular differences in FC in the SCD group compared to the aMCI group. This is a surprising finding given the clear-cut cognitive differences that are present between the SCD and aMCI groups, as well as the different stages that are attributed to these groups in the clinical and pathological progression of AD. Our results suggest that even though these groups are clinically different, their underlying insular pathology may not be. Previous studies have highlighted the similarities and the overlap of the cerebral amyloid burden between patients with SCD and MCI (Wolfsgruber et al., 2017; Jessen et al., 2018). The explanation may be that SCD, in the presence of AD pathology, may represent the stage at which there is the first subtle cognitive dysfunction in pre-clinical AD (Sperling et al., 2011). At this stage, SCD reflects an individual's experience of this subtle cognitive decline, which is still in a significant compensatory phase (Erk et al., 2011). Furthermore, our previous study discovered that the compensatory phenomenon of neural networks in SCD individuals is associated with clinical cognition measures (Xue et al., 2019). This further indicates that AD pathology occurs in a temporally ordered manner, along with disease progression (Jack et al., 2013; Chen et al., 2019a). Therefore, SCD is considered a highly attractive stage for future early interventions in pre-clinical AD, as the brain function remains largely preserved with intact compensatory processes.
Our results also indicated that SCD and aMCI subjects have significantly higher HAMD scores in comparison to controls, despite the fact none of the subjects were depressed (HAMD score < 7). This suggests that depression may be a confounding factor of insular connectivity changes in SCD and aMCI. Recently, Liew (2019) investigated the independent risk factors for neurocognitive disorders associated with depression in a large sample study. Their results suggested that SCD and depression were both independent risks of MCI/dementia, but the combination of depression and SCD was a higher risk compared to SCD alone. However, in order to avoid this confounding factor, all subjects had depression scores of <7. Furthermore, there were no correlations found between depression scores and insular connectivity. Therefore, it is reasonable to speculate that the connectivity changes seen are indicative of SCD and aMCI, and are not representative of a state of depression.
Even within the same group, there are differences among vAI, dAI, and PI. Based on our study results, we can infer that vAI is more sensitive with regard to the manifestation of compensation. Furthermore, vAI delivers more evident clinical manifestations of deterioration and compensation compared to dAI and PI and is associated with strong clinical significance. Liu et al. (2018) have reported that vAI, dAI, and PI are closely associated with SAN, ECN, and somatomotor network, respectively, indicating separate engagement in cognitive, affective, and interoceptive processes. The insula is highly associated with neuronal nodes that are related to episodic memory (Sugar and Moser, 2019) and possesses the function of executive control (Menon and Uddin, 2010). Our data have revealed that vAI is recruited in the functioning of episodic memory and executive function. Additionally, our results reveal a strong relationship between executive function and PI. Since there are distinct brain regions located within the hub of insular subnetworks, we infer that FC among these interactive subregions possesses multimodal functions. Under certain circumstances in which a specific function of a subregion is impaired, FC is increased within that region. However, further experimentation is required to verify specific mechanisms. Our preliminary results suggest that altered FC detection in pre-clinical AD patients may reversely play an essential role in revealing the internal relationships within insular subnetworks.
To date, this is the first time that aberrant FC of insular subnetworks has been applied to identify the stages of the spectrum of pre-clinical AD. Utilizing binary logistic regression, we were able to obtain two separate optimal models for each pre-clinical stage, SCD, and aMCI. The accuracy of classification for the SCD model was 83% (81% sensitivity and 81.8% specificity), while the accuracy of the aMCI model was 86.5% (80% sensitivity and 83.6% specificity). Through this comparison, we are able to conclude that the aMCI classification model has higher overall accuracy. While the SCD classification model has increased competence in the identification of real patients, the aMCI classification model possesses a greater capability of excluding healthy individuals. Based on these results, as well as a combination of abnormal FCs in several altered brain regions, which belong to the overlapping areas of vAI, dAI, and PI, we are able to precisely distinguish the spectrum of pre-clinical AD. Therefore, abnormal connectivity among these regions can be utilized as a tool for early clinical diagnosis, and these altered regions can also be used as therapeutic targets for early intervention and treatment.
There are several limitations to our study. Firstly, we have a small sample size, and only 55 CNs, 38 patients with SCD, and 56 patients with aMCI were included in this study. This may have led to a bias of the results. To enhance the statistical power of our results, we applied a non-parametric permutation test, which allows control of the false positive rate in cluster-level inference. Meanwhile, our NBH-ADsnp database is constantly being updated and recruits new volunteers, and therefore, we will further verify our conclusions shortly. Secondly, we studied the behavioral significance of FC abnormalities in its resting state, which has some limitations. The task-based fMRI may be utilized to verify these results and further evaluate the behavioral significance of FC alterations. Finally, we found no differences in FC between SCD and aMCI, which may limit the validity of insular FC measures in staging individuals that are on the spectrum of pre-clinical AD. This may suggest that insular FC features may simply serve as biological markers of disease, rather than markers of disease progression. The reason for these findings may be that aMCI subjects were recruited from the memory clinic and local communities. In the future, we hope to further expand the sample size in order to assess the characteristics of insular FC differences in patients with aMCI from the local community vs. patients enrolled from the memory clinic.
Subjective cognitive decline and aMCI, part of the spectrum of pre-clinical AD, share some convergent and divergent altered intrinsic connectivity of insular subnetworks, with differences in abnormalities existing among subnetworks. These abnormalities can be applied to accurately identify patients that are within the spectrum of pre-clinical AD, which suggests that aberrant functional connectivity within insular networks may serve as a strong potential biomarker in the diagnosis of pre-clinical AD stages.
The raw data supporting the conclusions of this article will be made available by the authors, without undue reservation.
The studies involving human participants were reviewed and approved by the responsible Human Participants Ethics Committee of the Affiliated Brain Hospital of Nanjing Medical University. The patients/participants provided their written informed consent to participate in this study.
JC, XZ, SW, and HS: study design. SW, HS, GH, CX, WQ, JR, and FZ: data acquisition. SW and HS: data analyses. SW and HS: manuscript preparation. All authors contributed to the article and approved the submitted version.
This study was supported by the National Natural Science Foundation of China (Nos. 81701675; 2018YFC1314300; 81971255; and 81700666); the Key Project was supported by the Medical Science and technology development Foundation, Nanjing Department of Health (No. JQX18005); the Cooperative Research Project of Southeast University-Nanjing Medical University (No. 2018DN0031); the Key Research and Development Plan (Social Development) Project of Jiangsu Province (Nos. BE2018608 and BE2019610); the Innovation and Entrepreneurship Training Program for College Students in Jiangsu Province (Nos. 201810312061X and 201910312035Z); and the Key Scientific Research Projects of Colleges and Universities in Henan Province (No. 18A190003).
The authors declare that the research was conducted in the absence of any commercial or financial relationships that could be construed as a potential conflict of interest.
The Supplementary Material for this article can be found online at: https://www.frontiersin.org/articles/10.3389/fnagi.2021.597455/full#supplementary-material
Allen, J. S., Emmorey, K., Bruss, J., and Damasio, H. (2008). Morphology of the insula in relation to hearing status and sign language experience. J. Neurosci. 28, 11900–11905. doi: 10.1523/JNEUROSCI.3141-08.2008
Almairac, F., Duffau, H., and Herbet, G. (2018). Contralesional macrostructural plasticity of the insular cortex in patients with glioma: a VBM study. Neurology 91, e1902–e1908. doi: 10.1212/WNL.0000000000006517
Ashburner, J., and Friston, K. J. (2009). Computing average shaped tissue probability templates. Neuroimage 45, 333–341. doi: 10.1016/j.neuroimage.2008.12.008
Bai, F., Liao, W., Watson, D. R., Shi, Y., Yuan, Y., Cohen, A. D., et al. (2011). Mapping the altered patterns of cerebellar resting-state function in longitudinal amnestic mild cognitive impairment patients. J. Alzheimers. Dis. 23, 87–99. doi: 10.3233/JAD-2010-101533
Berger-Sieczkowski, E., Gruber, B., Stogmann, E., and Lehrner, J. (2019). Differences regarding the five-factor personality model in patients with subjective cognitive decline and mild cognitive impairment. Neuropsychiatrie 33, 35–45. doi: 10.1007/s40211-018-0292-z
Brady, R. O. Jr., Gonsalvez, I., Lee, I., Ongur, D., Seidman, L. J., Schmahmann, J. D., et al. (2019). Cerebellar-prefrontal network connectivity and negative symptoms in schizophrenia. Am. J. Psychiatry 176, 512–520. doi: 10.1176/appi.ajp.2018.18040429
Buckley, R. F., Maruff, P., Ames, D., Bourgeat, P., Martins, R. N., Masters, C. L., et al. (2016). Subjective memory decline predicts greater rates of clinical progression in preclinical Alzheimer's disease. Alzheimers. Dement 12, 796–804. doi: 10.1016/j.jalz.2015.12.013
Cedres, N., Machado, A., Molina, Y., Diaz-Galvan, P., Hernandez-Cabrera, J. A., Barroso, J., et al. (2019). Subjective cognitive decline below and above the age of 60: a multivariate study on neuroimaging, cognitive, clinical, and demographic measures. J. Alzheimers. Dis. 68, 295–309. doi: 10.3233/JAD-180720
Chang, L. J., Yarkoni, T., Khaw, M. W., and Sanfey, A. G. (2013). Decoding the role of the insula in human cognition: functional parcellation and large-scale reverse inference. Cerebral Cortex 23, 739–749. doi: 10.1093/cercor/bhs065
Chen, J., Chen, G., Shu, H., Chen, G., Ward, B. D., Wang, Z., et al. (2019a). Predicting progression from mild cognitive impairment to Alzheimer's disease on an individual subject basis by applying the CARE index across different independent cohorts. Aging 11, 2185–2201. doi: 10.18632/aging.101883
Chen, J., Duan, X., Shu, H., Wang, Z., Long, Z., Liu, D., et al. (2016a). Differential contributions of subregions of medial temporal lobe to memory system in amnestic mild cognitive impairment: insights from fMRI study. Sci. Rep. 6:26148. doi: 10.1038/srep26148
Chen, J., Shu, H., Wang, Z., Liu, D., Shi, Y., Zhang, X., et al. (2015a). The interaction of APOE genotype by age in amnestic mild cognitive impairment: a voxel-based morphometric study. J. Alzheimers. Dis. 43, 657–668. doi: 10.3233/JAD-141677
Chen, J., Shu, H., Wang, Z., Zhan, Y., Liu, D., Liao, W., et al. (2016b). Convergent and divergent intranetwork and internetwork connectivity patterns in patients with remitted late-life depression and amnestic mild cognitive impairment. Cortex 83, 194–211. doi: 10.1016/j.cortex.2016.08.001
Chen, J., Shu, H., Wang, Z., Zhan, Y., Liu, D., Liu, Y., et al. (2019b). Intrinsic connectivity identifies the sensory-motor network as a main cross-network between remitted late-life depression- and amnestic mild cognitive impairment-targeted networks. Brain Imaging Behav. 14, 1130–1142. doi: 10.1007/s11682-019-00098-4
Chen, J., Zhang, Z., and Li, S. (2015b). Can multi-modal neuroimaging evidence from hippocampus provide biomarkers for the progression of amnestic mild cognitive impairment? Neurosci. Bull 31, 128–140. doi: 10.1007/s12264-014-1490-8
Chen, S., Xu, W., Xue, C., Hu, G., Ma, W., Qi, W., et al. (2020). Voxelwise meta-analysis of gray matter abnormalities in mild cognitive impairment and subjective cognitive decline using activation likelihood estimation. J. Alzheimers Dis. 77, 1495–1512. doi: 10.3233/JAD-200659
Chen, X., Lu, B., and Yan, C. G. (2018). Reproducibility of R-fMRI metrics on the impact of different strategies for multiple comparison correction and sample sizes. Hum. Brain Mapp. 39, 300–318. doi: 10.1002/hbm.23843
Deen, B., Pitskel, N. B., and Pelphrey, K. A. (2011). Three systems of insular functional connectivity identified with cluster analysis. Cereb. Cortex 21, 1498–1506. doi: 10.1093/cercor/bhq186
Dubois, B., Hampel, H., Feldman, H. H., Scheltens, P., Aisen, P., Andrieu, S., et al. (2016). Preclinical Alzheimer's disease: definition, natural history, and diagnostic criteria. Alzheimers. Dement. 12, 292–323. doi: 10.1016/j.jalz.2016.02.002
Erk, S., Spottke, A., Meisen, A., Wagner, M., Walter, H., and Jessen, F. (2011). Evidence of neuronal compensation during episodic memory in subjective memory impairment. Arch. Gen. Psychiatry 68, 845–852. doi: 10.1001/archgenpsychiatry.2011.80
Fan, Y., Resnick, S. M., Wu, X., and Davatzikos, C. (2008). Structural and functional biomarkers of prodromal Alzheimer's disease: a high-dimensional pattern classification study. Neuroimage 41, 277–285. doi: 10.1016/j.neuroimage.2008.02.043
Fillinger, C., Yalcin, I., Barrot, M., and Veinante, P. (2018). Efferents of anterior cingulate areas 24a and 24b and midcingulate areas 24a' and 24b' in the mouse. Brain Struct. Funct. 223, 1747–1778. doi: 10.1007/s00429-017-1585-x
Fliessbach, K., Trautner, P., Quesada, C. M., Elger, C. E., and Weber, B. (2007). Cerebellar contributions to episodic memory encoding as revealed by fMRI. Neuroimage 35, 1330–1337. doi: 10.1016/j.neuroimage.2007.02.004
Friston, K. J., Williams, S., Howard, R., Frackowiak, R. S., and Turner, R. (1996). Movement-related effects in fMRI time-series. Magn. Reson. Med. 35, 346–355. doi: 10.1002/mrm.1910350312
Ghaziri, J., Tucholka, A., Girard, G., Houde, J.-C., Boucher, O., Gilbert, G., et al. (2017). The corticocortical structural connectivity of the human insula. Cerebral Cortex 27, 1216–1228. doi: 10.1093/cercor/bhv308
Gour, N., Ranjeva, J.-P., Ceccaldi, M., Confort-Gouny, S., Barbeau, E., Soulier, E., et al. (2011). Basal functional connectivity within the anterior temporal network is associated with performance on declarative memory tasks. Neuroimage 58, 687–697. doi: 10.1016/j.neuroimage.2011.05.090
Guo, X., Han, Y., Chen, K., Wang, Y., and Yao, L. (2012). Mapping joint grey and white matter reductions in Alzheimer's disease using joint independent component analysis. Neurosci. Lett. 531, 136–141. doi: 10.1016/j.neulet.2012.10.038
Hao, L., Wang, X., Zhang, L., Xing, Y., Guo, Q., Hu, X., et al. (2017). Prevalence, risk factors, and complaints screening tool exploration of subjective cognitive decline in a large cohort of the Chinese population. J. Alzheimers. Dis. 60, 371–388. doi: 10.3233/JAD-170347
Jack, C. R. Jr., Knopman, D. S., Jagust, W. J., Petersen, R. C., Weiner, M. W., Aisen, P. S., et al. (2013). Tracking pathophysiological processes in Alzheimer's disease: an updated hypothetical model of dynamic biomarkers. Lancet Neurol. 12, 207–216. doi: 10.1016/S1474-4422(12)70291-0
Jessen, F., Amariglio, R. E., Buckley, R. F., van der Flier, W. M., Han, Y., Molinuevo, J. L., et al. (2020). The characterisation of subjective cognitive decline. Lancet Neurol. 19, 271–278. doi: 10.1016/S1474-4422(19)30368-0
Jessen, F., Amariglio, R. E., van Boxtel, M., Breteler, M., Ceccaldi, M., Chetelat, G., et al. (2014a). A conceptual framework for research on subjective cognitive decline in preclinical Alzheimer's disease. Alzheimers. Dement 10, 844–852. doi: 10.1016/j.jalz.2014.01.001
Jessen, F., Spottke, A., Boecker, H., Brosseron, F., Buerger, K., Catak, C., et al. (2018). Design and first baseline data of the DZNE multicenter observational study on predementia Alzheimer's disease (DELCODE). Alzheimers. Res. Ther. 10:15. doi: 10.1186/s13195-017-0314-2
Jessen, F., Wolfsgruber, S., Wiese, B., Bickel, H., Mosch, E., Kaduszkiewicz, H., et al. (2014b). AD dementia risk in late MCI, in early MCI, and in subjective memory impairment. Alzheimers. Dement 10, 76–83. doi: 10.1016/j.jalz.2012.09.017
Kwok, S. C., and Macaluso, E. (2015). Exogenous features versus prior experiences modulate different subregions of the right IPL during episodic memory retrieval. Sci. Rep. 5:11248. doi: 10.1038/srep11248
Liew, T. M. (2019). Depression, subjective cognitive decline, and the risk of neurocognitive disorders. Alzheimers. Res. Ther. 11:70. doi: 10.1186/s13195-019-0527-7
Lin, F., Ren, P., Lo, R. Y., Chapman, B. P., Jacobs, A., Baran, T. M., et al. (2017). Insula and inferior frontal gyrus' activities protect memory performance against alzheimer's disease pathology in old age. J. Alzheimers Dis. 55, 669–678. doi: 10.3233/JAD-160715
Liu, X., Chen, X., Zheng, W., Xia, M., Han, Y., Song, H., et al. (2018). Altered functional connectivity of insular subregions in Alzheimer's disease. Front. Aging Neurosci. 10:107. doi: 10.3389/fnagi.2018.00107
Lu, L., Li, F., Chen, H., Wang, P., Zhang, H., Chen, Y. C., et al. (2020). Functional connectivity dysfunction of insular subdivisions in cognitive impairment after acute mild traumatic brain injury. Brain Imaging Behav. 14, 941–948. doi: 10.1007/s11682-020-00288-5
Luo, X., Jiaerken, Y., Huang, P., Xu, X. J., Qiu, T., Jia, Y., et al. (2018). Alteration of regional homogeneity and white matter hyperintensities in amnestic mild cognitive impairment subtypes are related to cognition and CSF biomarkers. Brain Imaging Behav. 12, 188–200. doi: 10.1007/s11682-017-9680-4
Menon, V., and Uddin, L. Q. (2010). Saliency, switching, attention and control: a network model of insula function. Brain Struct. Funct. 214, 655–667. doi: 10.1007/s00429-010-0262-0
Mwansisya, T. E., Hu, A., Li, Y., Chen, X., Wu, G., Huang, X., et al. (2017). Task and resting-state fMRI studies in first-episode schizophrenia: a systematic review. Schizophr. Res. 189, 9–18. doi: 10.1016/j.schres.2017.02.026
Naqvi, N. H., and Bechara, A. (2010). The insula and drug addiction: an interoceptive view of pleasure, urges, and decision-making. Brain Struct. Funct. 214, 435–450. doi: 10.1007/s00429-010-0268-7
Nomi, J. S., Schettini, E., Broce, I., Dick, A. S., and Uddin, L. Q. (2018). Structural connections of functionally defined human insular subdivisions. Cerebral Cortex 28, 3445–3456. doi: 10.1093/cercor/bhx211
Peng, X., Lin, P., Wu, X., Gong, R., Yang, R., and Wang, J. (2018). Insular subdivisions functional connectivity dysfunction within major depressive disorder. J. Affect. Disord. 227, 280–288. doi: 10.1016/j.jad.2017.11.018
Petersen, R. C., Smith, G. E., Waring, S. C., Ivnik, R. J., Tangalos, E. G., and Kokmen, E. (1999). Mild cognitive impairment: clinical characterization and outcome. Arch. Neurol. 56, 303–308. doi: 10.1001/archneur.56.3.303
Power, J. D., Barnes, K. A., Snyder, A. Z., Schlaggar, B. L., and Petersen, S. E. (2012). Spurious but systematic correlations in functional connectivity MRI networks arise from subject motion. Neuroimage 59, 2142–2154. doi: 10.1016/j.neuroimage.2011.10.018
Qi, Z., Wu, X., Wang, Z., Zhang, N., Dong, H., Yao, L., et al. (2010). Impairment and compensation coexist in amnestic MCI default mode network. Neuroimage 50, 48–55. doi: 10.1016/j.neuroimage.2009.12.025
Scheef, L., Spottke, A., Daerr, M., Joe, A., Striepens, N., Kolsch, H., et al. (2012). Glucose metabolism, gray matter structure, and memory decline in subjective memory impairment. Neurology 79, 1332–1339. doi: 10.1212/WNL.0b013e31826c1a8d
Seo, E. H., and Choo, I. L. H. (2016). Amyloid-independent functional neural correlates of episodic memory in amnestic mild cognitive impairment. Eur. J. Nucl. Med. Mol. Imaging 43, 1088–1095. doi: 10.1007/s00259-015-3261-9
Sheline, Y. I., Raichle, M. E., Snyder, A. Z., Morris, J. C., Head, D., Wang, S., et al. (2010). Amyloid plaques disrupt resting state default mode network connectivity in cognitively normal elderly. Biol. Psychiatry 67, 584–587. doi: 10.1016/j.biopsych.2009.08.024
Smith, S. M., and Nichols, T. E. (2009). Threshold-free cluster enhancement: addressing problems of smoothing, threshold dependence and localisation in cluster inference. Neuroimage 44, 83–98. doi: 10.1016/j.neuroimage.2008.03.061
Sperling, R. A., Aisen, P. S., Beckett, L. A., Bennett, D. A., Craft, S., Fagan, A. M., et al. (2011). Toward defining the preclinical stages of Alzheimer's disease: recommendations from the National Institute on Aging-Alzheimer's Association workgroups on diagnostic guidelines for Alzheimer's disease. Alzheimers. Dement 7, 280–292. doi: 10.1016/j.jalz.2011.03.003
Stewart, R., Dufouil, C., Godin, O., Ritchie, K., Maillard, P., Delcroix, N., et al. (2008). Neuroimaging correlates of subjective memory deficits in a community population. Neurology 70, 1601–1607. doi: 10.1212/01.wnl.0000310982.99438.54
Striepens, N., Scheef, L., Wind, A., Popp, J., Spottke, A., Cooper-Mahkorn, D., et al. (2010). Volume loss of the medial temporal lobe structures in subjective memory impairment. Dement. Geriatr. Cogn. Disord. 29, 75–81. doi: 10.1159/000264630
Sugar, J., and Moser, M.-B. (2019). Episodic memory: neuronal codes for what, where, and when. Hippocampus 29, 1190–1205. doi: 10.1002/hipo.23132
Taylor, K. S., Seminowicz, D. A., and Davis, K. D. (2009). Two systems of resting state connectivity between the insula and cingulate cortex. Hum. Brain Mapp. 30, 2731–2745. doi: 10.1002/hbm.20705
Trzepacz, P. T., Yu, P., Bhamidipati, P. K., Willis, B., Forrester, T., Tabas, L., et al. (2013). Frontolimbic atrophy is associated with agitation and aggression in mild cognitive impairment and Alzheimer's disease. Alzheimers. Dement 9, S95–S104 e101. doi: 10.1016/j.jalz.2012.10.005
Uddin, L. Q., Supekar, K. S., Ryali, S., and Menon, V. (2011). Dynamic reconfiguration of structural and functional connectivity across core neurocognitive brain networks with development. J. Neurosci. 31, 18578–18589. doi: 10.1523/JNEUROSCI.4465-11.2011
van der Flier, W. M., van Buchem, M. A., Weverling-Rijnsburger, A. W., Mutsaers, E. R., Bollen, E. L., Admiraal-Behloul, F., et al. (2004). Memory complaints in patients with normal cognition are associated with smaller hippocampal volumes. J. Neurol. 251, 671–675. doi: 10.1007/s00415-004-0390-7
Van Dijk, K. R., Sabuncu, M. R., and Buckner, R. L. (2012). The influence of head motion on intrinsic functional connectivity MRI. Neuroimage 59, 431–438. doi: 10.1016/j.neuroimage.2011.07.044
Wang, Z., Xia, M., Dai, Z., Liang, X., Song, H., He, Y., et al. (2015). Differentially disrupted functional connectivity of the subregions of the inferior parietal lobule in Alzheimer's disease. Brain Struct. Funct. 220, 745–762. doi: 10.1007/s00429-013-0681-9
Wiech, K., Jbabdi, S., Lin, C. S., Andersson, J., and Tracey, I. (2014). Differential structural and resting state connectivity between insular subdivisions and other pain-related brain regions. Pain 155, 2047–2055. doi: 10.1016/j.pain.2014.07.009
Winblad, B., Palmer, K., Kivipelto, M., Jelic, V., Fratiglioni, L., Wahlund, L. O., et al. (2004). Mild cognitive impairment–beyond controversies, towards a consensus: report of the International Working Group on Mild Cognitive Impairment. J. Intern. Med. 256, 240–246. doi: 10.1111/j.1365-2796.2004.01380.x
Wolfsgruber, S., Polcher, A., Koppara, A., Kleineidam, L., Frolich, L., Peters, O., et al. (2017). Cerebrospinal fluid biomarkers and clinical progression in patients with subjective cognitive decline and mild cognitive impairment. J. Alzheimers. Dis. 58, 939–950. doi: 10.3233/JAD-161252
Xie, C., Bai, F., Yu, H., Shi, Y., Yuan, Y., Chen, G., et al. (2012). Abnormal insula functional network is associated with episodic memory decline in amnestic mild cognitive impairment. Neuroimage 63, 320–327. doi: 10.1016/j.neuroimage.2012.06.062
Xue, C., Yuan, B., Yue, Y., Xu, J., Wang, S., Wu, M., et al. (2019). Distinct disruptive patterns of default mode subnetwork connectivity across the spectrum of preclinical Alzheimer's disease. Front. Aging Neurosci. 11:307. doi: 10.3389/fnagi.2019.00307
Yan, C. G., Craddock, R. C., Zuo, X. N., Zang, Y. F., and Milham, M. P. (2013). Standardizing the intrinsic brain: towards robust measurement of inter-individual variation in 1000 functional connectomes. Neuroimage 80, 246–262. doi: 10.1016/j.neuroimage.2013.04.081
Yan, C. G., Wang, X. D., Zuo, X. N., and Zang, Y. F. (2016). DPABI: data processing & analysis for (resting-state) brain imaging. Neuroinformatics 14, 339–351. doi: 10.1007/s12021-016-9299-4
Yan, T., Wang, W., Yang, L., Chen, K., Chen, R., and Han, Y. (2018). Rich club disturbances of the human connectome from subjective cognitive decline to Alzheimer's disease. Theranostics 8, 3237–3255. doi: 10.7150/thno.23772
Keywords: amnestic mild cognitive impairment, subjective cognitive decline, fMRI, insular subnetwork, functional connectivity, episodic memory, executive function
Citation: Wang S, Sun H, Hu G, Xue C, Qi W, Rao J, Zhang F, Zhang X and Chen J (2021) Altered Insular Subregional Connectivity Associated With Cognitions for Distinguishing the Spectrum of Pre-clinical Alzheimer's Disease. Front. Aging Neurosci. 13:597455. doi: 10.3389/fnagi.2021.597455
Received: 21 August 2020; Accepted: 15 January 2021;
Published: 10 February 2021.
Edited by:
Oury Monchi, University of Calgary, CanadaReviewed by:
Takahito Yoshizaki, Keio University, JapanCopyright © 2021 Wang, Sun, Hu, Xue, Qi, Rao, Zhang, Zhang and Chen. This is an open-access article distributed under the terms of the Creative Commons Attribution License (CC BY). The use, distribution or reproduction in other forums is permitted, provided the original author(s) and the copyright owner(s) are credited and that the original publication in this journal is cited, in accordance with accepted academic practice. No use, distribution or reproduction is permitted which does not comply with these terms.
*Correspondence: Jiu Chen, ZXJpY2NzdEBhbGl5dW4uY29t; Xiangrong Zhang, ZHJ4cnpAaG90bWFpbC5jb20=
Disclaimer: All claims expressed in this article are solely those of the authors and do not necessarily represent those of their affiliated organizations, or those of the publisher, the editors and the reviewers. Any product that may be evaluated in this article or claim that may be made by its manufacturer is not guaranteed or endorsed by the publisher.
Research integrity at Frontiers
Learn more about the work of our research integrity team to safeguard the quality of each article we publish.