- 1Department of Geriatric Medicine, Institute of Clinical Medicine, Faculty of Medicine, University of Oslo, Oslo, Norway
- 2Department of Clinical Neurosciences (CNS), Center for Molecular Medicine CMM L8:01, Karolinska Institutet, Stockholm, Sweden
- 3Institute of Health and Society, Faculty of Medicine, University of Oslo, Oslo, Norway
- 4Department of Geriatric Medicine, The Memory Clinic, Oslo University Hospital, Oslo, Norway
- 5Norwegian National Advisory Unit on Ageing and Health, Vestfold Hospital Trust, Oslo, Norway
- 6Department of Neurobiology, Care Science and Society (NVS), Division of Clinical Geriatrics, Karolinska Institutet, Huddinge, Sweden
Accurate biomarkers of Alzheimer’s disease (AD) are essential for early diagnosis and intervention. Available biomarkers are not sufficient to permit the monitoring of AD progression over time, and additional biomarkers are required. Measures of aggregated amyloid-β (Aβ) could be useful biomarkers for AD. Here, we investigate whether levels of Thioflavin-T (ThT) positive amyloid aggregates, i.e., nanoplaques, in cerebrospinal fluid (CSF) could serve as useful biomarkers for AD. One-hundred and eighteen memory clinic patients were AT(N) classified, and CSF nanoplaque concentrations were compared between patients on the “Alzheimer’s continuum” (A+ patients) and patients with “Normal AD biomarkers” or “Non-AD pathologic change” (A− patients). CSF nanoplaque concentrations and sizes were quantified using the novel ThT-Fluorescence Correlation Spectroscopy (ThT-FCS) assay, and core biomarkers (Aβ42, total tau and phosphorylated tau) were determined by enzyme-linked immunosorbent assays. We investigated the association between nanoplaque concentrations and core biomarkers, and the diagnostic value of nanoplaque levels. Nanoplaque levels were increased in A+ patients compared to A− patients. Nanoplaque concentrations were negatively associated with Aβ42, but not related to total tau or phosphorylated tau measures. Quantification of nanoplaques did not improve the classification of patients on the Alzheimer’s continuum compared to the core biomarkers alone. Dynamic changes in nanoplaques concentration and size throughout AD stages should be explored in longitudinal studies.
Introduction
There is currently no cure for Alzheimer’s disease (AD), the leading cause of dementia worldwide. While detailed cellular and molecular mechanisms underlying AD remain elusive, the amyloid cascade hypothesis postulates that AD is caused by the accumulation of aggregated amyloid-β (Aβ) peptides (Hardy and Higgins, 1992; Selkoe and Hardy, 2016). It is hypothesized that these aggregates induce phosphorylation and aggregation of the tau protein into neurofibrillary tangles, before eventually forming amyloid plaques (Selkoe and Hardy, 2016). Indeed, Aβ pathology has been demonstrated to induce tau phosphorylation and aggregation in human neural cell culture models of AD (Choi et al., 2014; Kwak et al., 2020). It has been established that amyloid pathology develops several years before the first clinical symptoms of dementia arise (Jack et al., 2013). This preclinical AD stage is of great interest because it is believed to be the optimal period for pharmacological intervention (Blennow et al., 2015). Consequently, biomarkers indicative of the underlying pathological processes could be indispensable for identifying patients for clinical trials (Aluise et al., 2008), and are increasingly incorporated in AD diagnostic procedures (McKhann et al., 2011).
Several imaging and cerebrospinal fluid (CSF) biomarkers for AD have already been identified (Perrin et al., 2009; Jack et al., 2016). In CSF, three core biomarkers have been validated as markers of AD neuropathology (Blennow et al., 2015): low levels of Aβ42 are indicative of brain amyloid pathology, elevated levels of phosphorylated tau (P-tau) signify tau pathology, and elevated levels of total tau reflect neurodegeneration (T-tau). These markers, and their neuroimaging counterparts, each represent a biomarker group in the NIA-AA research framework (Jack et al., 2018). In this framework, markers are classified in an AT(N) system where “A” reflects markers of Aβ deposition, “T” reflects markers of pathologic tau, and “N” reflects markers of neurodegeneration. Each biomarker group can be defined as normal or abnormal (+/−), resulting in eight possible biomarker profiles.
While the core AD biomarkers accurately reflect AD neuropathology, they have several limitations. First, the core biomarkers do not inform on disease progression, because they are relatively stable in the clinical stages of AD (Dhiman et al., 2019). Second, the core biomarkers have limited use as primary endpoints in clinical trials, as they do not correlate well with cognitive function (Zhou et al., 2009; Williams et al., 2011; Radanovic et al., 2019), and changes in core biomarker levels do not correlate with cognitive change (Toledo et al., 2013). Accordingly, the AT(N) biomarker profiles have recently been found to have restricted use for the prediction of AD progression rates (Wattmo et al., 2020). Finally, the core biomarkers do not differentiate AD from other causes of dementia with sufficient accuracy (Blennow et al., 2012). There is therefore a need for supplemental biomarkers that permit monitoring of AD progression over time and reflect the response (if any) to therapeutic interventions.
Measures of aggregated Aβ are promising candidate biomarkers. Increased levels of monomeric Aβ do not cause neurodegeneration; the self-association of Aβ into aggregates is necessary (Funke et al., 2009). It is theorized that the lowering of CSF monomeric Aβ42 in preclinical AD is due to increased retention of Aβ in neurite plaques in the brain. In line with this, low CSF Aβ42 is found to correlate with increased brain uptake of amyloid ligands on amyloid-positron emission tomography (amyloid-PET; Forsberg et al., 2008; Müller et al., 2019). Consequently, measures of amyloid aggregates would be an early biomarker and reflect ongoing pathology (Holtta et al., 2013). As such, they could be useful markers of the disease process and potential treatment responses. The novel Thioflavin-T Fluorescence Correlation Spectroscopy (ThT-FCS) assay uses ThT to label and quantify structured amyloidogenic aggregates with high specificity. Recently, this method has been used to identify amyloid aggregates with single-molecule sensitivity; both synthetic aggregates in vitro (Tiiman et al., 2015) and aggregates in blood serum (Tiiman et al., 2019) and CSF (Aksnes et al., 2020).
In this article, we investigate the relationship between core CSF biomarkers and average nanoplaque concentrations and sizes determined using ThT-FCS, in memory clinic patients with different AT(N)-profiles.
Materials and Methods
Study Cohort
Patients from the Norwegian Registry of Persons Assessed for Cognitive Symptoms (NorCog), who had been referred for cognitive complaints to the Oslo University Hospital Memory Clinic, were included in the study. All patients were deemed to have sufficient cognitive capacity to provide informed consent at the time of inclusion, and all patients signed written informed consent. Patients were included between June 2014 and November 2018 and underwent CSF sampling as part of the diagnostic procedure. Clinical data were extracted from NorCog, or patient medical records if missing from the registry.
Clinical Assessment
The clinical assessment followed a standardized research protocol (Braekhus et al., 2011). Patients and their caregivers were interviewed about symptoms, medication, and medical history. The cognitive assessment included the Mini-Mental State Examination (MMSE; Folstein et al., 1975) and further cognitive tests. The extent of cognitive and functional impairment on the Clinical Dementia Rating scale (CDR; Hughes et al., 1982) was scored post hoc using all available information, by THE and ABK, experienced CDR raters. The physical assessment included blood and CSF sampling for all patients (N = 118), neuroimaging with magnetic resonance imaging (MRI, N = 114), and for a subset of patients, 18F-FDG PET (N = 71) and/or 18F-Flutemetamol-PET (N = 54). Atrophy on MRI was determined according to the cut-offs published by Ferreira and colleagues (Ferreira et al., 2015), 18F-Flutemetamol PET scans were visually classified as positive or negative in line with a validated reader program (Buckley et al., 2017), and 18F-FDG PET scans were evaluated according to the European Association of Nuclear Medicine Guidelines (Varrone et al., 2009).
Research diagnoses were determined retrospectively by THE and ABK, experienced memory clinic physicians. Cognitive impairment was staged as subjective cognitive decline (SCD), mild cognitive impairment (MCI) or dementia according to the SCD Initiative- (Jessen et al., 2014) and the National Institute of Aging and the Alzheimer’s Association (NIA-AA)-criteria (Albert et al., 2011; McKhann et al., 2011), respectively. Clinical diagnoses were grouped as Alzheimer’s clinical syndrome (corresponding to probable/possible AD according to the 2011 NIA-AA-criteria) or clinically non-AD (including SCD, vascular dementia (Sachdev et al., 2014), frontotemporal dementia (Rascovsky et al., 2011) and uncategorized cases). Patients with dementia with Lewy bodies, a non-AD amyloidogenic disorder, were excluded.
AT(N)-Classification
Patients were classified into eight AT(N) profiles based on all available biomarkers: for A, CSF Aβ42 levels and/or 18F-Flutemetamol classification; for T, CSF P-tau; for N, CSF T-tau, 18F-FDG and/or MRI results. In the case of discrepancies between the different biomarkers in one group, e.g., T-tau levels below the cut-off, but evidence of neuronal injury on MRI, the patient was classified as positive. The resulting eight AT(N) profiles were grouped into three biomarker categories: “Normal AD biomarkers” [A-T−(N−)], “Alzheimer’s continuum” [A + T−(N−), A + T + (N−), A + T + (N+), A + T−(N+)], or “Non-AD pathologic change” [A−T + (N−), A−T−(N+), A−T + (N+)]. Also, the profiles were divided into two groups (A +/A−) based on amyloid status only.
Analysis of CSF Core Biomarkers
Lumbar puncture with subsequent measurement of CSF core biomarkers (Aβ42, T-tau, and P-tau) was performed for all patients. Core biomarkers were analyzed with Innotest Kit enzyme-linked immunosorbent assays (Innogenetics, Ghent, Belgium) at the Department for Interdisciplinary Laboratory Medicine and Medical Biochemistry at Akershus University Hospital, Norway, which participates in the Alzheimer’s Association QC program for CSF biomarkers (Mattsson et al., 2011). Laboratory recommended cut-off values for a normal test were applied (Kalheim et al., 2018): Aβ42 above 700 pg/ml; P-tau below 80 pg/ml; and T-tau below 300 pg/ml for patients younger than 50 years, below 450 pg/ml for patients aged 50–69 years, and below 500 pg/ml for patients older than 70 years.
Analysis of CSF Nanoplaque Concentrations by ThT-FCS
CSF samples from all included patients were obtained from the NorCog biobank. Fluorescence intensity fluctuations were recorded using an individually modified ConfoCor3 system (Carl Zeiss, Jena, Germany; Vukojević et al., 2008). The procedure for ThT-FCS analysis is described in detail in Tiiman et al. (2019).
In brief, 1.6 μl of 2.5 mM ThT in deionized water was added to 200 μl CSF. Fluorescence intensity fluctuations were recorded in duplicates. The signal was collected for 3,000 s (30 series of 10 × 10 s measurements). Bursts in fluorescence intensity reflecting the passage of ThT-positive aggregates, i.e., nanoplaques, through the observation volume element were detected by automated fluorescence intensity fluctuation analysis. An increase in fluorescence intensity by a value that is more than five times larger than the standard deviation of the whole time series was denoted as a “single event.” The frequency of single events (fSEO), i.e., the total number of single events per hour, is a direct measure of the concentration of nanoplaques.
Analysis of CSF Nanoplaque Sizes
To analyze nanoplaque size, temporal autocorrelation curves (tACCs) were derived by temporal autocorrelation analysis of time series where single events were noted. The tACCs were normalized to the same amplitude, Gn(t) = 1, at time τ = 10 μs, and averaged across A− and A+ patients, and across “Normal AD biomarkers,” “Alzheimer’s continuum” and “Non-AD pathologic change” biomarker categories. Because only a small number of single events were observed for each individual, nanoplaque size could not be determined at the individual level. The averaged tACCs were evaluated using the Maximum Entropy Method for FCS (MEMFCS; Sengupta et al., 2003), a model-free fitting procedure developed to resolve FCS data based on a quasi-continuous distribution of highly heterogeneous diffusing components. MEMFCS was used to determine the distribution of diffusion times, which also reflects the size distribution.
Data Analysis
The researcher performing the ThT-FCS analysis was blinded to all patient information, including diagnosis and other biomarker data until data analysis was completed. Clinical diagnosis and AT(N)-classification was completed independent of the ThT-FCS analysis results.
Statistical Analysis
The fSEO variable was not normally distributed; the variable was log-transformed, and the transformed version was used for all analyses. Group differences were analyzed with ANOVA, t-tests, or Mann–Whitney U tests. Pearson product-moment correlations were performed between log(fSEO) and CSF Aβ42, T-tau, P-tau, MMSE, and CDR Sum of Boxes (CDR-SOB). The relationship between log(fSEO) and Aβ42 was further explored by multivariate linear regression. Logistic regression analysis was used to assess if logfSEO) could predict whether a patient belonged to the Alzheimer’s continuum. A univariate regression model with log(fSEO) as the only predictor was compared to two multivariate models, a clinical model including sex, age, APOEε4 status, and MMSE score as covariates and a core biomarker model including CSF Aβ42 and P-tau as covariates. Further, we assessed whether the inclusion of log(fSEO) in the multivariate models improved their explanatory power. Sensitivity and specificity for the classification of A+ and A− patients were compared for all biomarkers (Aβ42, P-tau, T-tau, and fSEO) by receiver operating characteristic (ROC) curves. Optimal cut-points were determined by Youden’s index. Statistical analyses were conducted in STATA 15.1 (StataCorp, College Station, TX, USA) and R 3.4.4 (R Foundation for Statistical Computing, Vienna, Austria).
Results
Demographic and clinical information for the 118 included patients is presented in Table 1. The amyloid groups were significantly different on all measures, except for years of education, P = 0.28. There is high consistency between clinical diagnosis and amyloid-positivity, 89% of patients categorized as A+ have clinical AD.
Nanoplaque Levels and Core Biomarker Levels
The relationships between CSF log(fSEO) levels and the core biomarkers are presented in Figure 1. There was a weak statistically significant negative correlation between CSF Aβ42 and log(fSEO) levels, Pearson correlation coefficient r = −0.20, P = 0.03. The relationship between CSF Aβ42 and log(fSEO) remained significant when adjusting for age, APOE ε4-status and MMSE score, β = −135.3, P = 0.004.
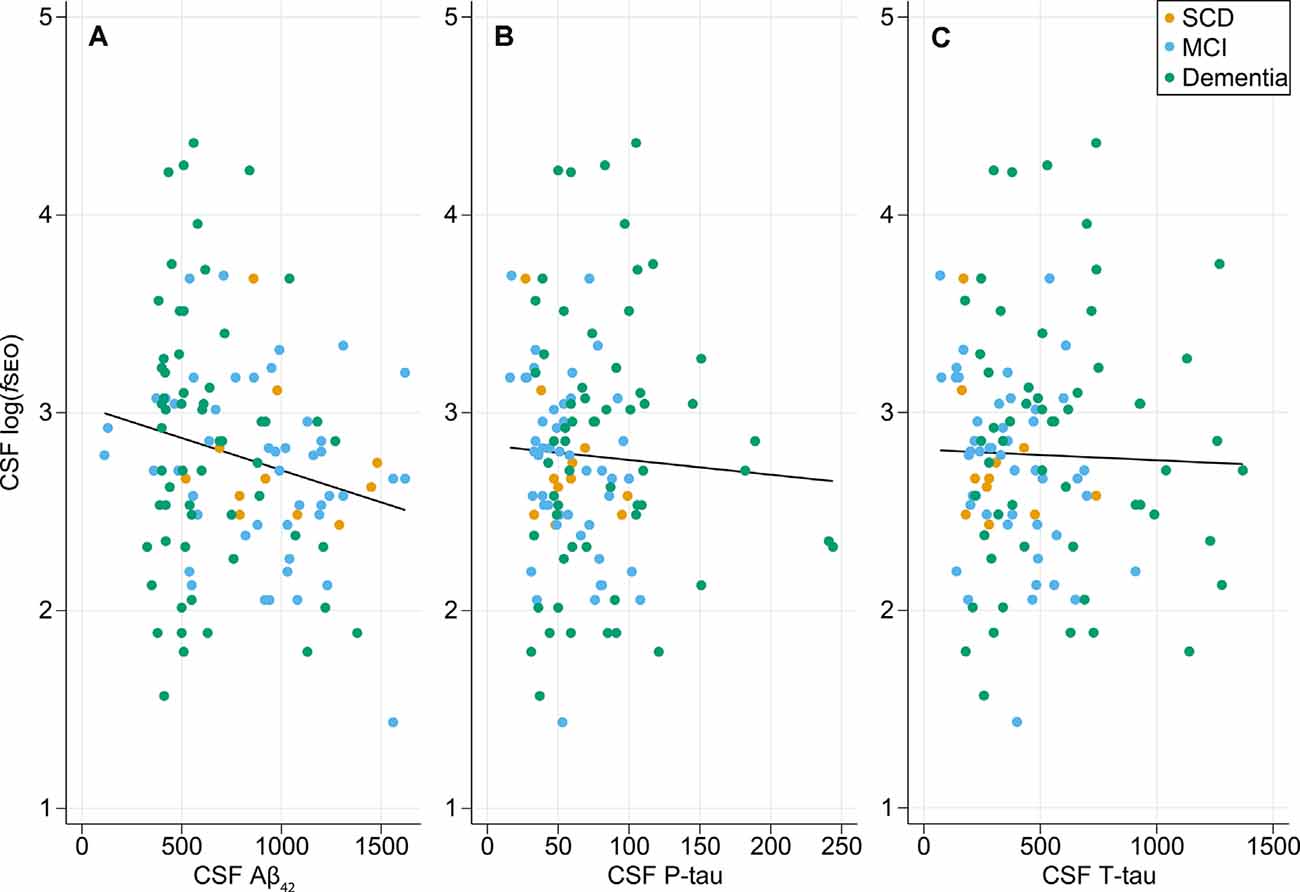
Figure 1. Relationship between cerebrospinal fluid (CSF) nanoplaque levels and core CSF biomarkers of AD. (A) Scatter plot of CSF logfSEO vs. CSF Aβ42, Pearson correlation coefficient, r = −0.20, P = 0.03. (B) Scatter plot of CSF logfSEO vs. CSF P-tau, Pearson r = −0.05, P = 0.58. (C) Scatter plot of CSF logfSEO vs. CSF T-tau, Pearson r = −0.03, P = 0.77.
There was no correlation between CSF log(fSEO) levels and T- or P-tau levels, P > 0.05. For the T-tau analysis, one extreme outlier with tau levels around 3,000 was excluded from the analysis. This, however, did not affect the conclusion.
Nanoplaque Levels and Disease Severity
There were no significant differences in log(fSEO) levels between the disease stages, P = 0.55, and Tukey’s post hoc test revealed no significant differences between neither the SCD and MCI groups, the SCD and dementia groups, nor the MCI and dementia groups, all P > 0.05. The distribution of log(fSEO) across disease stages is presented in Figure 2. CSF log(fSEO) levels were not associated with either MMSE-score or CDR-SOB, P > 0.05.
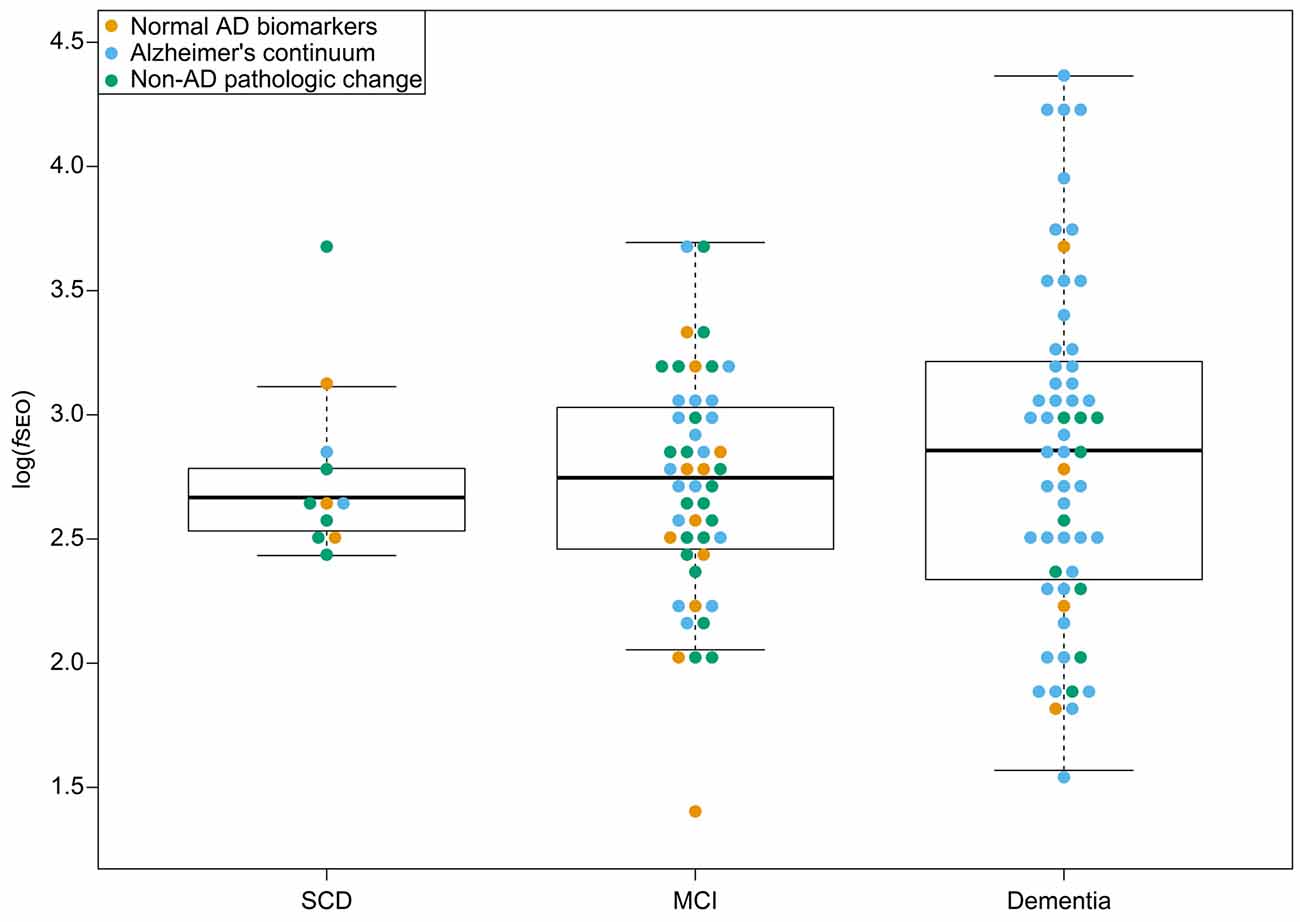
Figure 2. CSF nanoplaque levels according to disease stage. The box displays the first quartile (bottom line), the median (middle line), and the third quartile (top line). The upper whisker extends to the maximum data point (within 1.5 times the third quartile) and the lower whisker extends to the minimum data point (within 1.5 times the third quartile). Points beyond the whiskers represent outliers. Abbreviations: AD, Alzheimer’s disease; fSEO, frequency of single event occurrence; MCI, mild cognitive impairment; SCD, subjective cognitive decline.
Nanoplaque Levels Across AT(N) Classifications
Nanoplaque levels across the eight AT(N)-profiles are presented in Figure 3A. Note that in this cohort there are no patients with the A + T + (N)- profile.
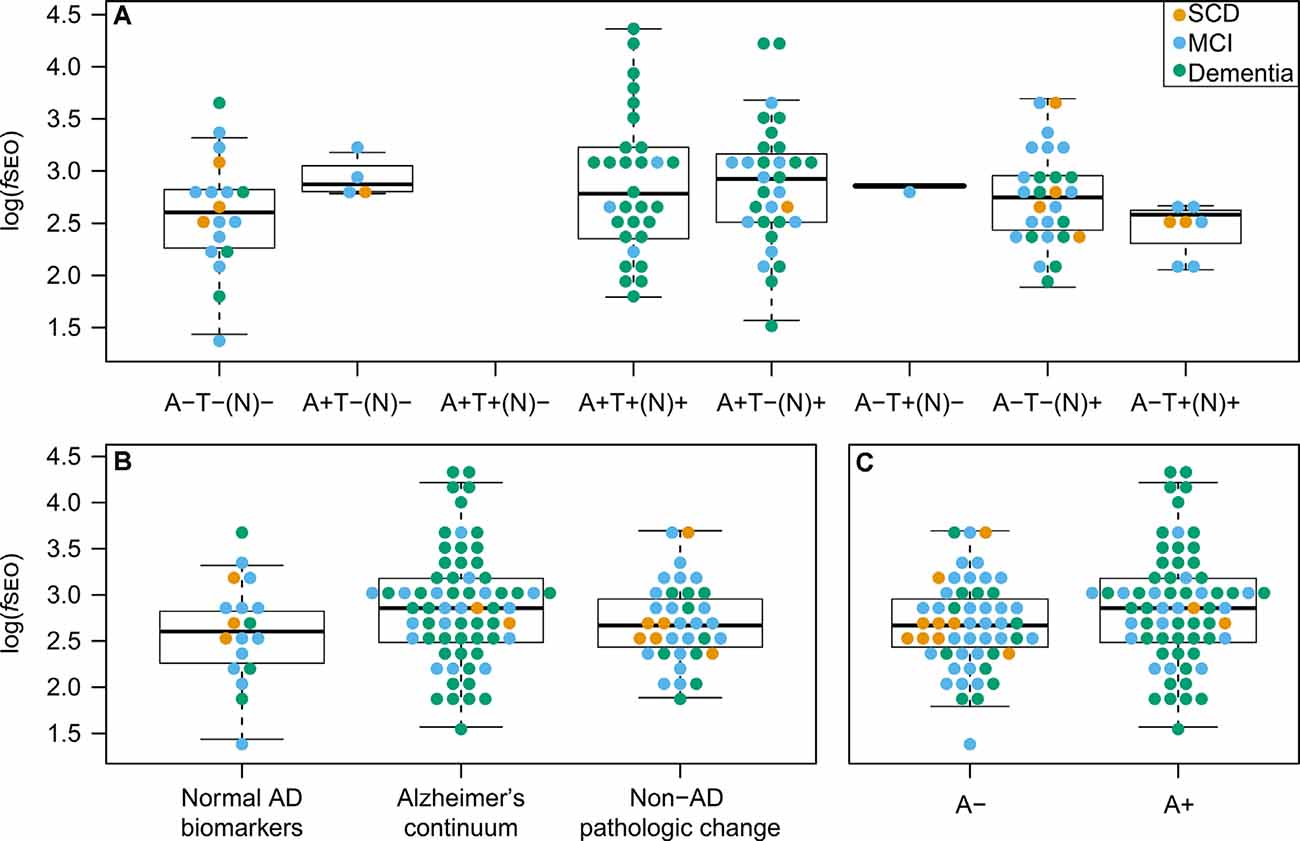
Figure 3. CSF nanoplaque levels according to AT(N) profile, AT(N) biomarker category, and amyloid status. (A) Nanoplaque levels across the eight AT(N) profiles. (B) Nanoplaque levels across the “Normal AD biomarkers”; “Alzheimer’s continuum” and “Non-AD pathologic change” biomarker categories. (C) Nanoplaque levels in A− and A+ groups, classified based on amyloid status only. The box displays the first quartile (bottom line), the median (middle line), and the third quartile (top line). The upper whisker extends to the maximum data point (within 1.5 times the third quartile) and the lower whisker extends to the minimum data point (within 1.5 times the third quartile). Points beyond the whiskers represent outliers. Abbreviations: AD, Alzheimer’s disease; fSEO, frequency of single event occurrence; MCI, mild cognitive impairment; SCD, subjective cognitive decline.
Nanoplaque levels were highest in patients on the Alzheimer’s continuum [log(fSEO) = 2.88], lower in patients with non-AD pathologic change [log(fSEO) = 2.69] and lowest in patients with normal AD biomarkers [log(fSEO) = 2.60], but this difference was not statistically significant, P = 0.10. The distribution across the three AT(N)-biomarker categories is presented Figure 3B.
When grouping patients by amyloid status alone, nanoplaque levels were significantly increased in A+ patients, log(fSEO) = 2.88 (95% confidence interval from 2.73 to 3.04) compared to A− patients, log(fSEO) = 2.66 (95% confidence interval from 2.53 to 2.79), P = 0.04. However, there was a large spread among the values, and a notable overlap between all groups, see Figure 3C.
Classification of Patients on Alzheimer’s Continuum
The results of the logistic regressions with amyloid status as the outcome variable are presented in Table 2. In the univariate model, increased levels of nanoplaques did not significantly increase the odds of being on the Alzheimer’s continuum, P > 0.05. In the core biomarker model, only Aβ42 significantly affected the odds of being on the Alzheimer’s continuum, odds-ratio 0.22 for a 100-unit increase (95% confidence interval from 0.10 to 0.45). All variables in the clinical model were significant predictors. The odds of being on the Alzheimer’s continuum were reduced for males, odds-ratio = 0.32, and with higher MMSE-scores, odds-ratio 0.84 per point. The odds were increased for APOE ε4-carriers, odds-ratio = 6.12 and with increased age, odds-ratio 1.80 for 5 years (95% confidence interval from 1.27 to 2.55).
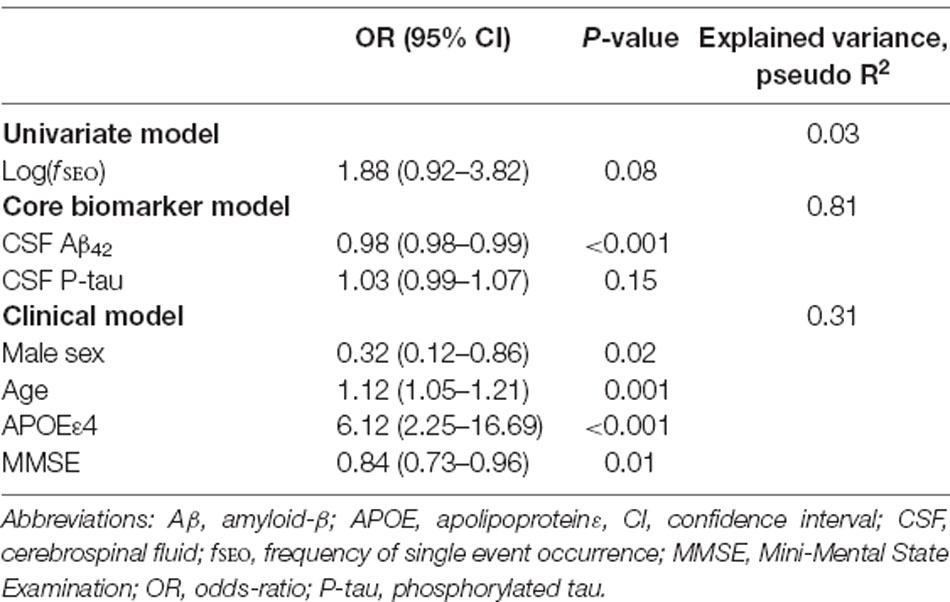
Table 2. Prediction of amyloid positivity (A+) by the univariate, core biomarker, and clinical models.
When included in the core biomarker model, log(fSEO) remained a non-significant predictor, odds-ratio = 2.48 (95% confidence interval from 0.34 to 18.17) and did not improve the model performance, likelihood-ratio test, χ2 = 0.94, P = 0.33. However, when adjusted for clinical variables, increased log(fSEO) levels significantly increased the risk of being on the Alzheimer’s continuum, odds-ratio = 4.36 (95% confidence interval from 1.44 to 13.18), P = 0.01. Inclusion of log(fSEO) increased the explained variance of the model, pseudo R2 = 0.37 and improved the model performance, likelihood-ratio test, χ2 = 8.04, P = 0.005.
The ROC-curves for the classification of patients on the Alzheimer’s continuum by CSF biomarkers Aβ42, T-tau, P-tau, and fSEO are presented in Figure 4. This figure also shows the area under the curve (AUC), cut-points, sensitivity, and specificity for each CSF marker. As expected, the CSF Aβ42 biomarker, based on which amyloid status was determined for most patients, had the highest sensitivity and specificity, and the largest AUC. The fSEO had the smallest AUC and lowest sensitivity.
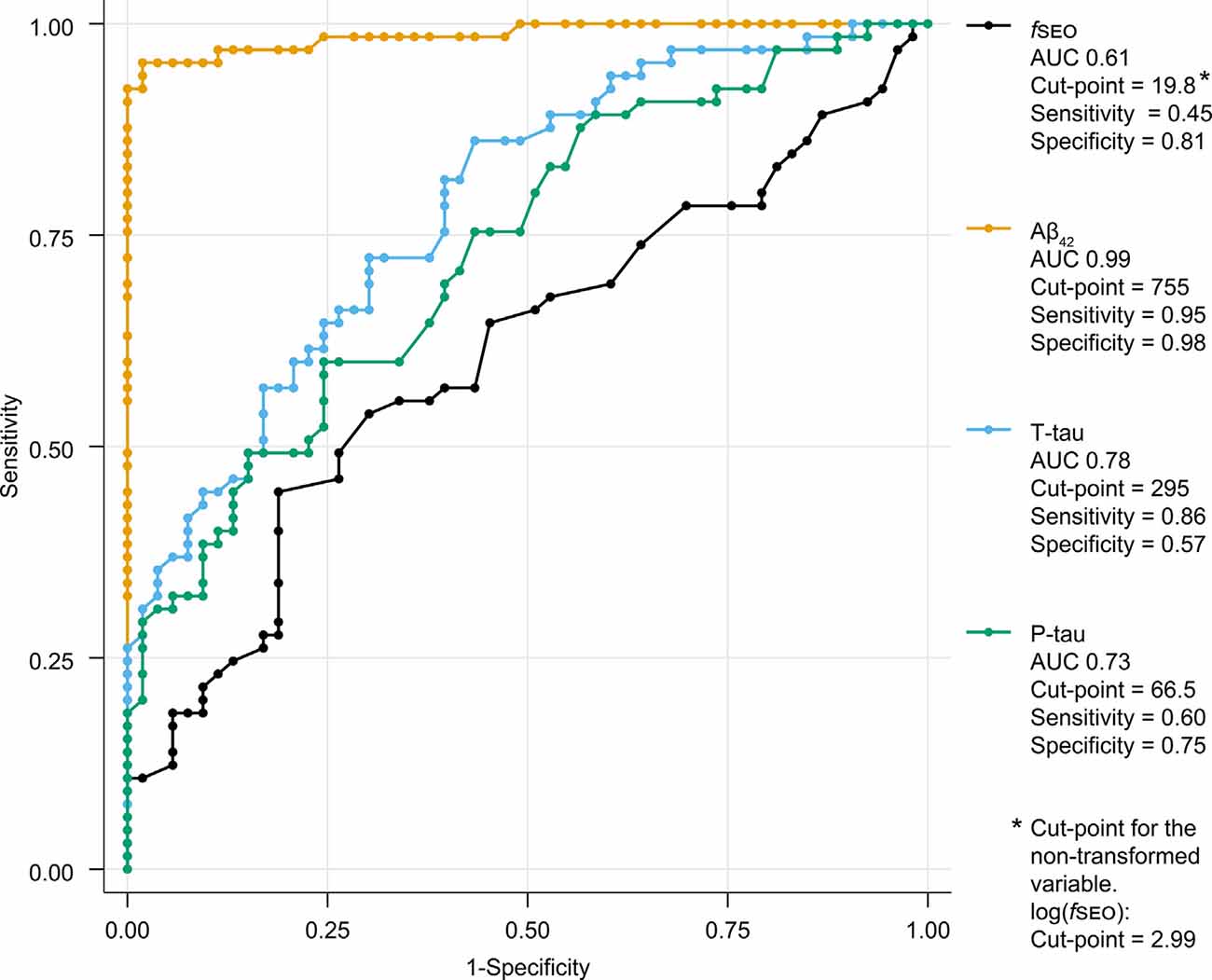
Figure 4. Receiver operating characteristic (ROC)-curves for classification of A+ and A− patients by CSF nanoplaque and core biomarker levels. The reported cut-point corresponds to the highest Youden’s index. Abbreviations: Aβ, amyloid-β; AUC, the area under the curve; fSEO, frequency of single event occurrence; P-tau, phosphorylated tau; T-tau, total tau.
Nanoplaque Size Distributions
The diffusion times for the amyloid groups and the three biomarker categories are presented in Figure 5. The A− group has two peaks, indicating the presence of larger and smaller amyloidogenic aggregates in a dynamic equilibrium. In the A+ group, the dominant size is between the two forms in the A− group. When separating the “Normal AD biomarkers” and “Non-AD pathologic change” categories that make up the A− group, it can be seen that the nanoplaque sizes are similar for patients on the Alzheimer’s continuum and patients with normal AD biomarkers, whilst the “Non-AD pathologic change” shows the pattern seen for the A-group, with both smaller and larger nanoplaques.
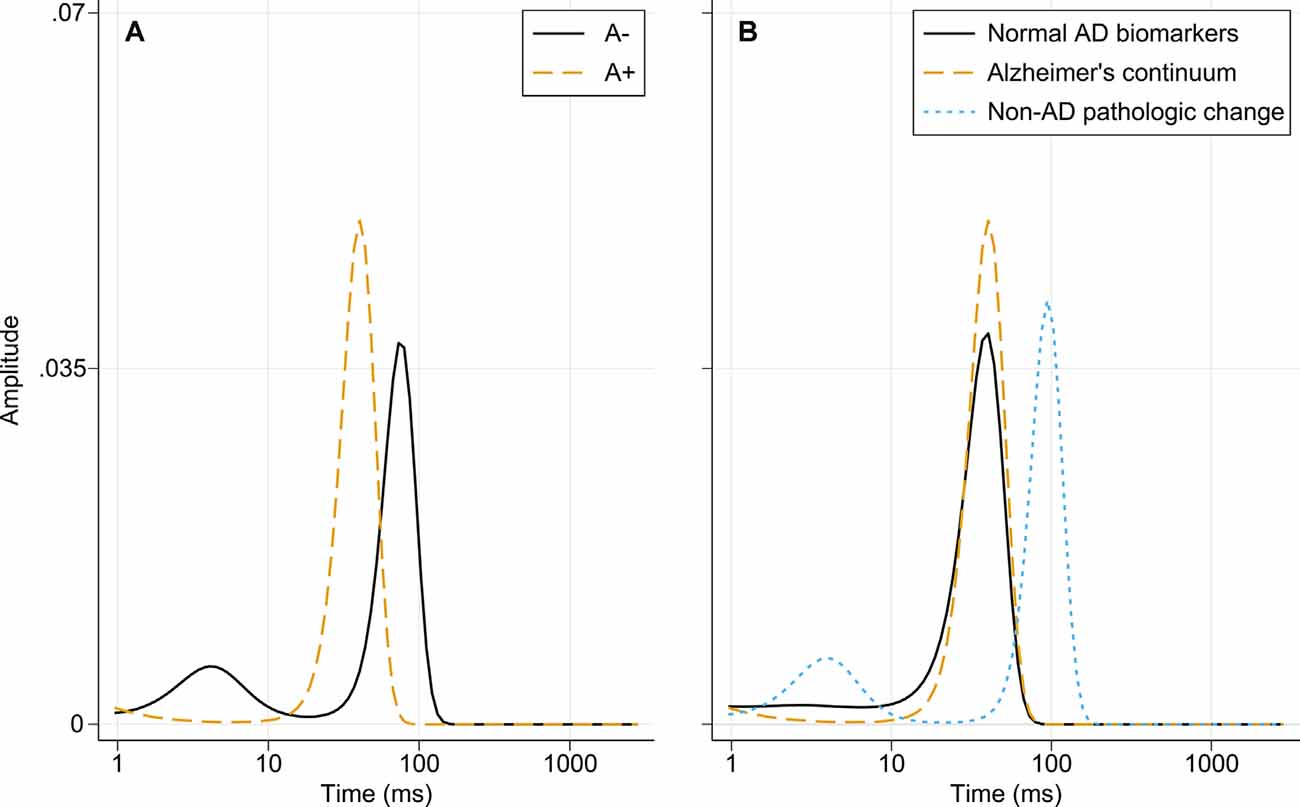
Figure 5. Distribution of diffusion times in different biomarker groups obtained using MEMFCS analysis of corresponding tACCs. (A) Diffusion times for the A− and A+ patient groups. (B) Diffusion times for the “Normal AD biomarkers,” “Alzheimer’s continuum” and “Non-AD pathologic change” biomarker categories. Abbreviations: AD, Alzheimer’s disease; MEMFCS, Maximum Entropy Method for Fluorescence Correlation Spectroscopy; tACC, temporal autocorrelation curves.
Discussion
It is hypothesized that advancing our understanding of amyloid aggregates in the AD pathogenic processes could be useful for intervention studies (Cremades and Dobson, 2018). In this study, we have investigated whether structured nanoplaques, ThT-binding amyloid aggregates enriched in secondary β-sheet structure, in CSF are related to the core biomarkers for AD. Using the recently developed ThT-FCS assay with single-molecule sensitivity, we have quantified the concentration and size of nanoplaques in the CSF. Increased levels of nanoplaques were associated with lowered CSF Aβ42, but not with measures of T-tau or P-tau.
The ThT-FCS assay selectively quantifies amyloidogenic aggregates with a β-sheet secondary structure (Tiiman et al., 2019). In contrast to monomeric Aβ, such aggregates are neurotoxic (Walsh et al., 1999; Ono and Tsuji, 2020); the appearance of nanoplaques could indicate pathological conversion from monomers to protofibrils. As ThT does not bind to monomers (LeVine, 1999), there is an excellent separation between the nanoplaque- and conventional Aβ42 measurements. While one previous study found no correlation between monomeric Aβ42 and amyloid oligomers in CSF (Holtta et al., 2013), we have shown a negative correlation between Aβ42 and nanoplaque levels. This supports the notion that the decrease in monomeric Aβ in AD CSF is partially, but not entirely, explained by its incorporation into oligomeric or fibrillar forms (Englund et al., 2009; Holtta et al., 2013). One caveat is that while ThT selectively binds aggregates with a β-sheet secondary structure, it cannot discriminate the primary structure (i.e., amino acid sequence) of the polypeptides in the nanoplaques. In addition to Aβ, several proteins, e.g., α-synuclein and prion protein, aggregate to a β-sheet structure (Soto and Pritzkow, 2018) and bind ThT (Xue et al., 2017; Cao and Yang, 2018). Hence, in theory, the detected nanoplaques could be composed of Aβ, other polypeptides, or a composite of the two (Luo et al., 2016). Moreover, Aβ aggregate folds that are not ThT-positive, i.e., that do not give rise to ThT fluorescence, may also exist.
Nanoplaque levels were increased in patients on Alzheimer’s continuum compared to patients without amyloid pathology. This is in line with previous research showing that amyloid oligomers and/or protofibrils are increased in the CSF of AD patients (Pitschke et al., 1998; Fukumoto et al., 2010; Santos et al., 2012; Holtta et al., 2013; Savage et al., 2014). However, there was substantial overlap between the biomarker groups and nanoplaque levels did not have high diagnostic accuracy for amyloid pathology. Unlike previous studies on oligomers in CSF (Fukumoto et al., 2010; Santos et al., 2012; Savage et al., 2014), nanoplaque levels were not associated with worse cognitive function in our cohort. It should be explored whether nanoplaque levels associate with changes in intra-individual cognitive performance over time. Recently, accurate plasma biomarkers such as p-tau217 have emerged, potentially revolutionizing AD diagnosis (Palmqvist et al., 2020). In the future, such markers may limit the use of CSF markers in clinical diagnosis. However, supplementary markers reflecting different aspects of AD pathology will likely continue to play an important role in furthering the understanding of AD etiology and mechanisms.
The nanoplaques identified in CSF from patients on the Alzheimer’s continuum and patients with normal AD biomarkers were similar in size and, in these groups, the size distribution was dominated by one size only. In contrast, in CSF from patients with non-AD pathologic change, both larger and smaller nanoplaques, as compared to the other groups, were observed. The implications of these findings are uncertain. While it has been shown that ThT-reactive aggregates cause toxicity in the brain by invoking neuroinflammation (De et al., 2019a,b), it is not clear whether differently sized nanoplaques have different mechanisms of toxicity. Of note, the smallest aggregates labeled by ThT contain around 40 monomers, and thus the size distribution of smaller oligomeric aggregates is not known.
One strength of the current study is the thoroughly characterized memory clinic cohort, which includes both patients on the Alzheimer’s continuum and appropriate controls with different biomarker compositions. The cognitive function of all patients has been established by several cognitive tests. All patients had at least one biomarker available in all AT(N) categories, permitting their classification according to current research criteria. A further strength is the use of the novel ThT-FCS assay, as this assay is highly suitable for biomarker studies. FCS requires small sample volumes and can measure a wide range of molecular concentrations (from around 10 pM to 100 nM; Chatterjee et al., 2017). Also, ThT-FCS assay has the utmost sensitivity and can detect single aggregated particles without relying on signal-amplification, protein separation, or immune probes. Moreover, the ThT-FCS assay can uniquely measure the size of ThT-responsive structured aggregates. The fact that the diffusion time of small structured nanoplaques (τD1 = 3 ± 2 ms) is about 100 times slower than the diffusion of Rhodamine 6G (Rh6G) used for instrument calibration (τD,Rh6G = 29 ± 2 μs) and that the diffusion of the largest, not sedimented, nanoplaques (τD2 = 95 ± 20 ms) is about 3,275 times slower than the diffusion of Rh6G, suggests that the size of the ThT-responsive nanoplaques floating in the CSF are about 100 nm −2 μm. Of note, the size of the nanoplaques is estimated using the Stokes-Einstein equation, , where, D is the diffusion coefficient, k is the Boltzmann constant, T is the absolute temperature, η is the solvent viscosity and RH is the hydrodynamic radius of a hypothetical compact sphere in a viscous medium, the relationship , where τD is the translational diffusion time and is the radial radius of the FCS observation volume element, and the hydrodynamic radius for Rh6G, RH,Rh6G = 0.589 nm (Müller et al., 2008). Although this calculation applies to spherical molecules, it can be regarded here as a good enough first approximation. While the diagnostic utility of ThT-FCS does not appear to surpass that of the core biomarkers, this method could still be an important supplement to immune-based assays and contribute to a better understanding of protein aggregation and its role in AD pathology (Funke, 2011). Especially, this method could contribute to an improved understanding of conformation-dependent toxicity and the development of anti-aggregation interventions (Cremades and Dobson, 2018).
One limitation of the current study is that the intra-individual dynamics of nanoplaque levels and sizes over time cannot be inferred. Nanoplaque levels do not appear to increase linearly with disease severity or reduced levels of monomeric Aβ42, and the dynamics of the level and sizes of these aggregates throughout the disease process should be explored in longitudinal studies. The sample size was limited, and patients were not evenly distributed across groups; this, however, is reflective of the clinical population. Further, because the study includes a clinical population, relatively few patients with SCD were included. This is a limitation as it restricts the understanding of nanoplaque dynamics at this very early clinical stage; future studies should explore a potential role for nanoplaques at earlier disease stages.
Interestingly, ThT-reactive aggregates in CSF have recently been shown to exert toxicity by increasing neuroinflammation (De et al., 2019a). In another study, fibrillar, ThT-binding Aβ was found to increase blood-brain barrier permeability and associated inflammation in vitro (Parodi-Rullán et al., 2020). As such, further research should investigate the relationship between nanoplaque levels and markers of neuroinflammation. Specifically, it would be of interest to explore whether increased levels of nanoplaques predict an increase in markers of neuroinflammation. This possible link to neuroinflammation denotes potential applications of nanoplaque measurements, notably in the mechanistic understanding, diagnosis and monitoring of cerebral amyloid angiopathy-related inflammation (CAA-ri, DiFrancesco et al., 2015; Carmona-Iragui et al., 2016) and amyloid related imaging abnormalities (ARIA, Piazza and Winblad, 2016). CAA-ri is characterized by the spontaneous development of ARIA-like events, and has been put forth as a human spontaneous model of immunotherapy-induced ARIA (Piazza et al., 2013). Importantly, the concentration of CSF anti-Aβ autoantibodies is increased during the acute phases of CAA-ri (Piazza et al., 2013), and have been proposed as a promising biomarker for ARIA (Piazza and Winblad, 2016). However, the potential release of different, possibly neurotoxic, Aβ species by these autoantibodies in CAA-ri and ARIA has not been evaluated; this highlights a potential application for the ThT-FCS assay.
Conclusion
We found that CSF nanoplaque levels were negatively correlated with monomeric Aβ42, but not with T- or P-tau. While CSF nanoplaque levels were increased in patients on the Alzheimer’s continuum, this method did not identify patients with amyloid pathology with the same sensitivity and specificity as the core biomarkers and did not improve the classification of patients. This method may reflect other relevant pathology, and its relationship with inflammatory markers should be explored.
Data Availability Statement
The datasets presented in this article are not readily available because legal restrictions, imposed by the registry owners and the ethical committee, prevent us from publicly sharing the de-identified dataset due to sensitive patient information. The clinical data may be requested from the Norwegian Registry of Persons Assessed for Cognitive Symptoms at e-mail: post@aldringoghelse.no. The results of the ThT-FCS analysis are available upon reasonable request to the authors. All data availability is dependent on approval from the REC South East, contact at e-mail: post@helseforskning.etikkom.no. Requests to access the datasets should be directed to mari.aksnes@medisin.uio.no.
Ethics Statement
The studies involving human participants were reviewed and approved by Norwegian Regional Committees for Medical and Health Research Ethics, REC South East, 2017/223. The patients/participants provided their written informed consent to participate in this study.
Author Contributions
MA, AT, LT, NB, VV, and ABK designed the study. ABK and THE conducted the clinical assessment and diagnoses of the participants. AT did the ThT-FCS experimental and data analysis. MA did the statistical analyses and wrote the manuscript. MA, AT, NB, VV, and ABK interpreted the data. All authors read and approved the final manuscript for publication.
Funding
This work was supported by funding from the Olav Thon Foundation, The Norwegian Health Association (Nasjonalforeningen for Folkehelse), Swedish Foundation for Strategic Research (Stiftelsen för Strategisk Forskning; SBE13-0115), Swedish Research Council (Vetenskapsrådet; VR 2018-05337), Olle Engkvists Foundation (Stiftelsen Olle Engkvist Byggmästare; 199-0480), Magnus Bergvalls Foundation (Magnus Bergvalls Stiftelse; 2018-02642), and Region Stockholm (ALF projects 20180365 and 20190561).
Conflict of Interest
ABK and THE have worked on clinical trials for Roche (BN29553) and Boehringer-Ingelheim (1346.0023). AT, LT and VV have filed a patent application under the Patent Cooperation Treat (PCT): WO 2019/192969 A1 “Method for the Diagnosis of Amyloid-Associated Diseases.”
The remaining authors declare that the research was conducted in the absence of any commercial or financial relationships that could be construed as a potential conflict of interest.
Acknowledgments
We want to acknowledge the Norwegian Registry of Persons Assessed for Cognitive Symptoms (NorCog) for providing access to patient data and CSF samples. We thank the patients who have participated in NorCog.
References
Aksnes, M., Müller, E. G., Tiiman, A., Edwin, T. H., Terenius, L., Revheim, M. E., et al. (2020). Amyloidogenic nanoplaques in cerebrospinal fluid: relationship to amyloid brain uptake and clinical Alzheimer’s disease in a memory clinic cohort. J. Alzheimers Dis. 77, 831–842. doi: 10.3233/JAD-200237
Albert, M. S., DeKosky, S. T., Dickson, D., Dubois, B., Feldman, H. H., Fox, N. C., et al. (2011). The diagnosis of mild cognitive impairment due to Alzheimer’s disease: recommendations from the National Institute on Aging-Alzheimer’s Association workgroups on diagnostic guidelines for Alzheimer’s disease. Alzheimers Dement. 7, 270–279. doi: 10.1016/j.jalz.2011.03.008
Aluise, C. D., Sowell, R. A., and Butterfield, D. A. (2008). Peptides and proteins in plasma and cerebrospinal fluid as biomarkers for the prediction, diagnosis and monitoring of therapeutic efficacy of Alzheimer’s disease. Biochim. Biophys. Acta 1782, 549–558. doi: 10.1016/j.bbadis.2008.07.008
Blennow, K., Dubois, B., Fagan, A. M., Lewczuk, P., de Leon, M. J., and Hampel, H. (2015). Clinical utility of cerebrospinal fluid biomarkers in the diagnosis of early Alzheimer’s disease. Alzheimers Dement. 11, 58–69. doi: 10.1016/j.jalz.2014.02.004
Blennow, K., Zetterberg, H., and Fagan, A. M. (2012). Fluid biomarkers in Alzheimer disease. Cold Spring Harb. Perspect. Med. 2:a006221. doi: 10.1101/cshperspect.a006221
Braekhus, A., Ulstein, I., Wyller, T. B., and Engedal, K. (2011). The memory clinic–outpatient assessment when dementia is suspected. Tidsskr. Nor. Laegeforen. 131, 2254–2257. doi: 10.4045/tidsskr.11.0786
Buckley, C. J., Sherwin, P. F., Smith, A. P. L., Wolber, J., Weick, S. M., and Brooks, D. J. (2017). Validation of an electronic image reader training programme for interpretation of [18F]flutemetamol β-amyloid PET brain images. Nucl. Med. Commun. 38, 234–241. doi: 10.1097/MNM.0000000000000633
Cao, K. J., and Yang, J. (2018). Translational opportunities for amyloid-targeting fluorophores. Chem. Commun. 54, 9107–9118. doi: 10.1039/c8cc03619e
Carmona-Iragui, M., Fernández-Arcos, A., Alcolea, D., Piazza, F., Morenas-Rodriguez, E., Antón-Aguirre, S., et al. (2016). Cerebrospinal fluid anti-amyloid-β autoantibodies and amyloid PET in cerebral amyloid angiopathy-related inflammation. J. Alzheimers Dis. 50, 1–7. doi: 10.3233/JAD-150614
Chatterjee, M., Noding, B., Willemse, E. A. J., Koel-Simmelink, M. J. A., van der Flier, W. M., Schild, D., et al. (2017). Detection of contactin-2 in cerebrospinal fluid (CSF) of patients with Alzheimer’s disease using Fluorescence Correlation Spectroscopy (FCS). Clin. Biochem. 50, 1061–1066. doi: 10.1016/j.clinbiochem.2017.08.017
Choi, S. H., Kim, Y. H., Hebisch, M., Sliwinski, C., Lee, S., D’Avanzo, C., et al. (2014). A three-dimensional human neural cell culture model of Alzheimer’s disease. Nature 515, 274–278. doi: 10.1038/nature13800
Cremades, N., and Dobson, C. M. (2018). The contribution of biophysical and structural studies of protein self-assembly to the design of therapeutic strategies for amyloid diseases. Neurobiol. Dis. 109, 178–190. doi: 10.1016/j.nbd.2017.07.009
De, S., Whiten, D. R., Ruggeri, F. S., Hughes, C., Rodrigues, M., Sideris, D. I., et al. (2019a). Soluble aggregates present in cerebrospinal fluid change in size and mechanism of toxicity during Alzheimer’s disease progression. Acta Neuropathol. Commun. 7:120. doi: 10.1186/s40478-019-0777-4
De, S., Wirthensohn, D. C., Flagmeier, P., Hughes, C., Aprile, F. A., Ruggeri, F. S., et al. (2019b). Different soluble aggregates of Aβ42 can give rise to cellular toxicity through different mechanisms. Nat. Commun. 10:1541. doi: 10.1038/s41467-019-09477-3
Dhiman, K., Blennow, K., Zetterberg, H., Martins, R. N., and Gupta, V. B. (2019). Cerebrospinal fluid biomarkers for understanding multiple aspects of Alzheimer’s disease pathogenesis. Cell. Mol. Life Sci. 76, 1833–1863. doi: 10.1007/s00018-019-03040-5
DiFrancesco, J. C., Touat, M., Caulo, M., Gallucci, M., Garcin, B., Levy, R., et al. (2015). Recurrence of cerebral amyloid angiopathy-related inflammation: a report of two cases from the iCAβ international network. J. Alzheimers Dis. 46, 1071–1077. doi: 10.3233/JAD-150070
Englund, H., Degerman Gunnarsson, M., Brundin, R. M., Hedlund, M., Kilander, L., Lannfelt, L., et al. (2009). Oligomerization partially explains the lowering of Aβ42 in Alzheimer’s disease cerebrospinal fluid. Neurodegener. Dis. 6, 139–147. doi: 10.1159/000225376
Ferreira, D., Cavallin, L., Larsson, E. M., Muehlboeck, J. S., Mecocci, P., Vellas, B., et al. (2015). Practical cut-offs for visual rating scales of medial temporal, frontal and posterior atrophy in Alzheimer’s disease and mild cognitive impairment. J. Intern. Med. 278, 277–290. doi: 10.1111/joim.12358
Folstein, M. F., Folstein, S. E., and McHugh, P. R. (1975). “Mini-mental state”. A practical method for grading the cognitive state of patients for the clinician. J. Psychiatr. Res. 12, 189–198. doi: 10.1016/0022-3956(75)90026-6
Forsberg, A., Engler, H., Almkvist, O., Blomquist, G., Hagman, G., Wall, A., et al. (2008). PET imaging of amyloid deposition in patients with mild cognitive impairment. Neurobiol Aging 29, 1456–1465. doi: 10.1016/j.neurobiolaging.2007.03.029
Fukumoto, H., Tokuda, T., Kasai, T., Ishigami, N., Hidaka, H., Kondo, M., et al. (2010). High-molecular-weight β-amyloid oligomers are elevated in cerebrospinal fluid of Alzheimer patients. FASEB J. 24, 2716–2726. doi: 10.1096/fj.09-150359
Funke, S. A. (2011). Detection of soluble amyloid-β oligomers and insoluble high-molecular-weight particles in CSF: development of methods with potential for diagnosis and therapy monitoring of Alzheimer’s disease. Int. J. Alzheimers Dis. 2011:151645. doi: 10.4061/2011/151645
Funke, S. A., Birkmann, E., and Willbold, D. (2009). Detection of Amyloid-beta aggregates in body fluids: a suitable method for early diagnosis of Alzheimer’s disease? Curr. Alzheimer Res. 6, 285–289. doi: 10.2174/156720509788486536
Hardy, J. A., and Higgins, G. A. (1992). Alzheimer’s disease: the amyloid cascade hypothesis. Science 256, 184–185. doi: 10.1126/science.1566067
Holtta, M., Hansson, O., Andreasson, U., Hertze, J., Minthon, L., Nagga, K., et al. (2013). Evaluating amyloid-β oligomers in cerebrospinal fluid as a biomarker for Alzheimer’s disease. PLoS One 8:e66381. doi: 10.1371/journal.pone.0066381
Hughes, C. P., Berg, L., Danziger, W. L., Coben, L. A., and Martin, R. L. (1982). A new clinical scale for the staging of dementia. Br. J. Psychiatry 140, 566–572. doi: 10.1192/bjp.140.6.566
Jack, C. R., Jr., Bennett, D. A., Blennow, K., Carrillo, M. C., Dunn, B., Haeberlein, S. B., et al. (2018). NIA-AA research framework: toward a biological definition of Alzheimer’s disease. Alzheimers Dement. 14, 535–562. doi: 10.1016/j.jalz.2018.02.018
Jack, C. R., Jr., Bennett, D. A., Blennow, K., Carrillo, M. C., Feldman, H. H., Frisoni, G. B., et al. (2016). A/T/N: an unbiased descriptive classification scheme for Alzheimer disease biomarkers. Neurology 87, 539–547. doi: 10.1212/WNL.0000000000002923
Jack, C. R., Jr., Knopman, D. S., Jagust, W. J., Petersen, R. C., Weiner, M. W., Aisen, P. S., et al. (2013). Tracking pathophysiological processes in Alzheimer’s disease: an updated hypothetical model of dynamic biomarkers. Lancet Neurol. 12, 207–216. doi: 10.1016/S1474-4422(12)70291-0
Jessen, F., Amariglio, R. E., van Boxtel, M., Breteler, M., Ceccaldi, M., Chetelat, G., et al. (2014). A conceptual framework for research on subjective cognitive decline in preclinical Alzheimer’s disease. Alzheimers Dement. 10, 844–852. doi: 10.1016/j.jalz.2014.01.001
Kalheim, L. F., Fladby, T., Coello, C., Bjørnerud, A., and Selnes, P. (2018). [18F]-Flutemetamol uptake in cortex and white matter: comparison with cerebrospinal fluid biomarkers and [18F]-Fludeoxyglucose. J. Alzheimers Dis. 62, 1595–1607. doi: 10.3233/JAD-170582
Kwak, S. S., Washicosky, K. J., Brand, E., von Maydell, D., Aronson, J., Kim, S., et al. (2020). Amyloid-β42/40 ratio drives tau pathology in 3D human neural cell culture models of Alzheimer’s disease. Nat. Commun. 11:1377. doi: 10.1038/s41467-020-15120-3
LeVine, H., 3rd (1999). Quantification of beta-sheet amyloid fibril structures with thioflavin T. Methods Enzymol. 309, 274–284. doi: 10.1016/s0076-6879(99)09020-5
Luo, J., Wärmländer, S. K. T. S., Gräslund, A., and Abrahams, J. P. (2016). Cross-interactions between the Alzheimer disease amyloid-β peptide and other amyloid proteins: a further aspect of the amyloid cascade hypothesis. J. Biol. Chem. 291, 16485–16493. doi: 10.1074/jbc.R116.714576
Mattsson, N., Andreasson, U., Persson, S., Arai, H., Batish, S. D., Bernardini, S., et al. (2011). The Alzheimer’s association external quality control program for cerebrospinal fluid biomarkers. Alzheimers Dement. 7, 386.e6–395.e6. doi: 10.1016/j.jalz.2011.05.2243
McKhann, G. M., Knopman, D. S., Chertkow, H., Hyman, B. T., Jack, C. R., Jr., Kawas, C. H., et al. (2011). The diagnosis of dementia due to Alzheimer’s disease: recommendations from the National Institute on Aging-Alzheimer’s Association workgroups on diagnostic guidelines for Alzheimer’s disease. Alzheimers Dement. 7, 263–269. doi: 10.1016/j.jalz.2011.03.005
Müller, E. G., Edwin, T. H., Stokke, C., Navelsaker, S. S., Babovic, A., Bogdanovic, N., et al. (2019). Amyloid-β PET-correlation with cerebrospinal fluid biomarkers and prediction of Alzheimer s disease diagnosis in a memory clinic. PLoS One 14:e0221365. doi: 10.1371/journal.pone.0221365
Müller, C. B., Loman, A., Pacheco, V., Koberling, F., Willbold, D., Richtering, W., et al. (2008). Precise measurement of diffusion by multi-color dual-focus fluorescence correlation spectroscopy. EPL 83:46001.doi: 10.1209/0295-5075/83/46001
Ono, K., and Tsuji, M. (2020). Protofibrils of amyloid-β are important targets of a disease-modifying approach for Alzheimer’s disease. Int. J. Mol. Sci. 21:952. doi: 10.3390/ijms21030952
Palmqvist, S., Janelidze, S., Quiroz, Y. T., Zetterberg, H., Lopera, F., Stomrud, E., et al. (2020). Discriminative accuracy of plasma phospho-tau217 for Alzheimer disease vs other neurodegenerative disorders. JAMA 324, 772–781. doi: 10.1001/jama.2020.12134
Parodi-Rullán, R., Ghiso, J., Cabrera, E., Rostagno, A., and Fossati, S. (2020). Alzheimer’s amyloidβ heterogeneous species differentially affect brain endothelial cell viability, blood-brain barrier integrity and angiogenesis. Aging Cell 19:e13258. doi: 10.1111/acel.13258
Perrin, R. J., Fagan, A. M., and Holtzman, D. M. (2009). Multimodal techniques for diagnosis and prognosis of Alzheimer’s disease. Nature 461, 916–922. doi: 10.1038/nature08538
Piazza, F., Greenberg, S. M., Savoiardo, M., Gardinetti, M., Chiapparini, L., and Raicher, I., et al. (2013). Anti-amyloid β autoantibodies in cerebral amyloid angiopathy-related inflammation: implications for amyloid-modifying therapies. Ann. Neurol. 73, 449–458. doi: 10.1002/ana.23857
Piazza, F., and Winblad, B. (2016). Amyloid-related imaging abnormalities (ARIA) in immunotherapy trials for Alzheimer’s disease: need for prognostic biomarkers? J. Alzheimers Dis. 52, 417–420. doi: 10.3233/JAD-160122
Pitschke, M., Prior, R., Haupt, M., and Riesner, D. (1998). Detection of single amyloid β-protein aggregates in the cerebrospinal fluid of Alzheimer’s patients by fluorescence correlation spectroscopy. Nat. Med. 4, 832–834. doi: 10.1038/nm0798-832
Radanovic, M., Oshiro, C. A., Freitas, T. Q., Talib, L. L., and Forlenza, O. V. (2019). Correlation between CSF biomarkers of Alzheimer’s disease and global cognition in a psychogeriatric clinic cohort. Braz. J. Psychiatry 41, 479–484. doi: 10.1590/1516-4446-2018-0296
Rascovsky, K., Hodges, J. R., Knopman, D., Mendez, M. F., Kramer, J. H., Neuhaus, J., et al. (2011). Sensitivity of revised diagnostic criteria for the behavioural variant of frontotemporal dementia. Brain 134, 2456–2477. doi: 10.1093/brain/awr179
Sachdev, P., Kalaria, R., O’Brien, J., Skoog, I., Alladi, S., Black, S. E., et al. (2014). Diagnostic criteria for vascular cognitive disorders: a VASCOG statement. Alzheimer Dis. Assoc. Disord. 28, 206–218. doi: 10.1097/WAD.0000000000000034
Santos, A. N., Ewers, M., Minthon, L., Simm, A., Silber, R. E., Blennow, K., et al. (2012). Amyloid-β oligomers in cerebrospinal fluid are associated with cognitive decline in patients with Alzheimer’s disease. J. Alzheimers Dis. 29, 171–176. doi: 10.3233/JAD-2012-111361
Savage, M. J., Kalinina, J., Wolfe, A., Tugusheva, K., Korn, R., Cash-Mason, T., et al. (2014). A sensitive Aβ oligomer assay discriminates Alzheimer’s and aged control cerebrospinal fluid. J. Neurosci. 34, 2884–2897. doi: 10.1523/JNEUROSCI.1675-13.2014
Selkoe, D. J., and Hardy, J. (2016). The amyloid hypothesis of Alzheimer’s disease at 25 years. EMBO Mol. Med. 8, 595–608. doi: 10.15252/emmm.201606210
Sengupta, P., Garai, K., Balaji, J., Periasamy, N., and Maiti, S. (2003). Measuring size distribution in highly heterogeneous systems with fluorescence correlation spectroscopy. Biophys. J. 84, 1977–1984. doi: 10.1016/S0006-3495(03)75006-1
Soto, C., and Pritzkow, S. (2018). Protein misfolding, aggregation and conformational strains in neurodegenerative diseases. Nat. Neurosci. 21, 1332–1340. doi: 10.1038/s41593-018-0235-9
Tiiman, A., Jarvet, J., Graslund, A., and Vukojević, V. (2015). Heterogeneity and turnover of intermediates during amyloid-β (Aβ) peptide aggregation studied by fluorescence correlation spectroscopy. Biochemistry 54, 7203–7211. doi: 10.1021/acs.biochem.5b00976
Tiiman, A., Jelic, V., Jarvet, J., Jaremo, P., Bogdanovic, N., Rigler, R., et al. (2019). Amyloidogenic nanoplaques in blood serum of patients with Alzheimer’s disease revealed by time-resolved thioflavin T fluorescence intensity fluctuation analysis. J. Alzheimers Dis. 68, 571–582. doi: 10.3233/JAD-181144
Toledo, J. B., Xie, S. X., Trojanowski, J. Q., and Shaw, L. M. (2013). Longitudinal change in CSF Tau and Aβ biomarkers for up to 48 months in ADNI. Acta Neuropathol. 126, 659–670. doi: 10.1007/s00401-013-1151-4
Varrone, A., Asenbaum, S., Vander Borght, T., Booij, J., Nobili, F., Någren, K., et al. (2009). EANM procedure guidelines for PET brain imaging using [18F]FDG, version 2. Eur. J. Nucl. Med. Mol. Imaging 36, 2103–2110. doi: 10.1007/s00259-009-1264-0
Vukojević, V., Heidkamp, M., Ming, Y., Johansson, B., Terenius, L., and Rigler, R. (2008). Quantitative single-molecule imaging by confocal laser scanning microscopy. Proc. Natl. Acad. Sci. U S A 105, 18176–18181. doi: 10.1073/pnas.0809250105
Walsh, D. M., Hartley, D. M., Kusumoto, Y., Fezoui, Y., Condron, M. M., Lomakin, A., et al. (1999). Amyloid β-protein fibrillogenesis: structure and biological activity of protofibrillar intermediates. J. Biol. Chem. 274, 25945–25952. doi: 10.1074/jbc.274.36.25945
Wattmo, C., Blennow, K., and Hansson, O. (2020). Cerebro-spinal fluid biomarker levels: phosphorylated tau (T) and total tau (N) as markers for rate of progression in Alzheimer’s disease. BMC Neurol. 20:10. doi: 10.1186/s12883-019-1591-0
Williams, J. H., Wilcock, G. K., Seeburger, J., Dallob, A., Laterza, O., Potter, W., et al. (2011). Non-linear relationships of cerebrospinal fluid biomarker levels with cognitive function: an observational study. Alzheimers Res. Ther. 3:5. doi: 10.1186/alzrt64
Xue, C., Lin, T. Y., Chang, D., and Guo, Z. (2017). Thioflavin T as an amyloid dye: fibril quantification, optimal concentration and effect on aggregation. R. Soc. Open. Sci. 4:160696. doi: 10.1098/rsos.160696
Keywords: Alzheimer disease, amyloid, amyloid beta-peptides, amyloidogenic proteins, biomarkers, cerebrospinal fluid, fluorescence spectrometry, thioflavin T
Citation: Aksnes M, Tiiman A, Edwin TH, Terenius L, Bogdanović N, Vukojević V and Knapskog AB (2021) Comparison of Cerebrospinal Fluid Amyloidogenic Nanoplaques With Core Biomarkers of Alzheimer’s Disease. Front. Aging Neurosci. 12:608628. doi: 10.3389/fnagi.2020.608628
Received: 21 September 2020; Accepted: 09 December 2020;
Published: 08 January 2021.
Edited by:
Olivia Belbin, Sant Pau Institute for Biomedical Research, SpainReviewed by:
Fabrizio Piazza, University of Milano-Bicocca, ItalyAlan David Snow, Cognitive Clarity Inc., United States
Copyright © 2021 Aksnes, Tiiman, Edwin, Terenius, Bogdanović, Vukojević and Knapskog. This is an open-access article distributed under the terms of the Creative Commons Attribution License (CC BY). The use, distribution or reproduction in other forums is permitted, provided the original author(s) and the copyright owner(s) are credited and that the original publication in this journal is cited, in accordance with accepted academic practice. No use, distribution or reproduction is permitted which does not comply with these terms.
*Correspondence: Mari Aksnes, mari.aksnes@medisin.uio.no
† These authors share first authorship