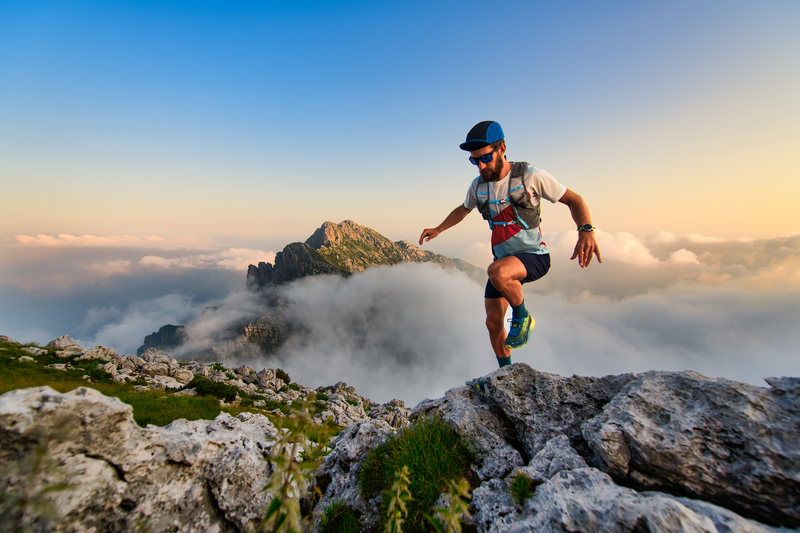
94% of researchers rate our articles as excellent or good
Learn more about the work of our research integrity team to safeguard the quality of each article we publish.
Find out more
SYSTEMATIC REVIEW article
Front. Aging Neurosci. , 10 November 2020
Sec. Parkinson’s Disease and Aging-related Movement Disorders
Volume 12 - 2020 | https://doi.org/10.3389/fnagi.2020.587396
This article is part of the Research Topic Biomarkers and Pathogenesis of Alpha-Synuclein in Parkinson's Disease View all 33 articles
Recent research regards the electroencephalogram (EEG) as a promising method to study real-time brain dynamic changes in patients with Parkinson's disease (PD), but a deeper understanding is needed to discern coincident pathophysiology, patterns of changes, and diagnosis. This review summarized recent research on EEG characterization related to the cognitive and motor functions in PD patients and discussed its potential to be used as diagnostic biomarkers. Thirty papers out of 220 published from 2010 to 2020 were reviewed. Movement abnormalities and cognitive decline are related to changes in EEG spectrum and event-related potentials (ERPs) during typical oddball paradigms and/or combined motor tasks. Abnormalities in β and δ frequency bands are, respectively the main manifestation of dyskinesia and cognitive decline in PD. The review showed that PD patients have noteworthy changes in specific EEG characterizations, however, the underlying mechanism of the interrelation between gait and cognitive is still unclear. Understanding the specific nature of the relationship is essential for development of novel invasive clinical diagnostic and therapeutic methods.
Parkinson's disease (PD) is the second most common neurodegenerative disease after Alzheimer's disease (AD) (Galvez et al., 2018). The degeneration of the basal nucleus nigrostriatal pathway leads to the loss of the dopaminergic neurons, which will result in multiple motor and non-motor symptoms (Martinez-Martin et al., 2011; Galvez et al., 2018). The motor symptoms mainly appear as resting tremor, postural instability, or gait impairment whilst patients would also demonstrate non-motor symptoms, such as depression, anxiety, sleep disorders, and cognitive dysfunctions (Latreille et al., 2015; Galvez et al., 2018). These consequential features of PD contribute to reduced quality of life and increased the risk of disability and mortality in patients with PD.
Gait is no longer regarded as an automated activity, and instead, the role of central nervous system (CNS) and the interaction between motion control and movement execution is drawn more and more attention. Studies in recent decades has revealed a relationship between cognitive dysfunction and motor symptoms of postural instability/gait disability (PIGD) (Martinez-Martin, 2011). Evidence have shown that cognitive functions, especially execution function and attention function, play significant roles in gait regulation (Maruyama and Yanagisawa, 2006; Amboni et al., 2008). Although the precise mechanism underlying PIGD, especially for severe symptoms, such as freezing of gait (FOG), are not completely understood. It has been shown that impaired cognitive functions can reduce motor autonomy of PD patients (Lewis and Barker, 2009), which comprises postural and gait stability (Robinovitch et al., 2013). Such cognitive dysfunctions (i.e., executive function impairment) are significantly related to motor symptoms (Maruyama and Yanagisawa, 2006; Amboni et al., 2008), suggesting that it can be considered as a predictor of PD.
Neuroimaging technologies have been widely used to study the regulation effect of cognition on motor control. Previous research has demonstrated that FOG might be related with dysfunction of the cortical frontal and parietal regions, and increased activation of the frontal region related to attention network has been observed in PD patients (Rushworth et al., 2002; Wager et al., 2004; Peterson et al., 2014; Maidan et al., 2016b). However, current studies have certain limitations as they were mostly performed in a constrained environment due to the immobility of scanner equipment.
Recent mobile brain/body imaging techniques based on electroencephalogram (EEG) allow the acquisition and real-time analysis of brain dynamics during active unrestrained movements (Nathan and Contreras-Vidal, 2016). The approach has been applied to distinguish brain dysfunctions of PD patients (Handojoseno et al., 2018; Wagner et al., 2019). In general, PD patients show a slowing tendency of global EEG activity where a decrease of beta and gamma power whilst an increase of power in the theta and alpha bands have been observed to be significantly related to cognitive processes, such as motivation, emotion, and decision-making (Handojoseno et al., 2013). Event-related potentials (ERPs) do not only reflect cognitive decline but also could indicate motor disorders in PD (Georgiev et al., 2015; Butler et al., 2017; Delval et al., 2018; Maidan et al., 2019).
Despite a certain amount of studies about characterizing EEG patterns in PD have been published, changes of EEG features related to cognition and motor dysfunctions have not been discussed in detail. Therefore, the aim of this article is to provide a literature review of characterizations of EEG signals related to PD's cognitive and motor functions and discuss its potential to be used as a novel method in clinic diagnosis.
This review follows a PICO search strategy based on the research question about the brain mechanism related to cognition and motor dysfunctions in PD. The EEG features of PD patients were summarized and compared to healthy controls, as well as other neurodegenerative diseases, such as DLB and AD.
In this review, PubMed, Web of Science databases and EMBASE were systematically searched for relevant literature from 2010 to 2020. Three sets of keywords were used for the literature search: (i) “Parkinson's disease”; (ii) “EEG” or “Electroencephalography”; (iii) “Dual task” or “cognition and motor” or “cognitive and motor.” Papers that have the term of keywords (i & ii & iii) located within the title and/or abstract were included in this review. In addition to the systematic electronic database search, a targeted search of bibliographies of relevant articles was also performed to identify any additional papers for inclusion.
Only original, full-text articles published in English between January 2010 and June 2020 that investigate the cognitive and motor impairments with scalp EEG analysis in patients with PD were considered in this review. Articles were excluded if they: (i) did not use scalp EEG signals to study features associated with cognitive and/or motor symptoms; (ii) studied on other neurological diseases, such as Alzheimer's disease, multiple system atrophy, Huntington's disease, and Binswanger disease; (iii) were review articles.
Using the search method mentioned above, a total of 152 articles were retrieved in the Web of Science database and 66 articles were retrieved in the PubMed database. After duplicate and unrelated papers were removed after initial screening, a total of 51 articles were excluded according to the criteria, of which, 38 were excluded according to the exclusion criteria (i), 8 according to the criteria (ii), 2 according to the criteria (iii) and 3 due to only healthy people participated in the studies. Two relevant articles were identified based on the targeted search in the review article. Therefore, a total of 30 articles were selected for inclusion in this review. A modified PRISMA diagram as shown in Figure 1 illustrates the screening and inclusion process.
Fourteen papers enrolled only PD patients with and/or without motor or cognitive impairment in the experiments while the rest of studies aimed to identify difference in EEG features during cognitive and/or motion tasks for PD group compared with healthy control (HC) group (n = 14) or patient group with other neurodegenerative disease (n = 2), such as AD or Lewy body dementia (LBD). The PD patient demographic characteristics and clinical scores are summarized in Table 1.
More than half of the studies utilized motor and/or cognitive tasks, some of which combined oddball paradigm (Kotz and Gunter, 2015; Muente et al., 2015; Waechter et al., 2015; Tard et al., 2016; Possti et al., 2020) in order to analyze dual task execution difficulty and investigate the interrelation of the cognitive decline and motor impairment in PD patients. Motor tasks include the standardized Timed up and Go Test (Quynh Tran et al., 2016; Handojoseno et al., 2018), bilateral cyclical ankle movements (Yoshida et al., 2018), lower-limb pedaling motor task (Singh et al., 2020), and repetitive movements of a specific finger (Herz et al., 2014), while the common Simon task (van Wouwe et al., 2014; Singh et al., 2018), and other customized tasks that require more cognitive processing (Palmer et al., 2010; Yuvaraj et al., 2014; Melloni et al., 2015; Solis-Vivanco et al., 2015) were used to accomplish the cognitive tasks. Three other studies established simple auditory and/or visual oddball paradigms to assess PD cognitive and motor symptoms (Georgiev et al., 2015; Butler et al., 2017; Maidan et al., 2019). The characterizations of EEG signals include: EEG frequency spectrum characteristics, evoked potentials and cortical-muscle coherence (CMC). The remaining 11 papers (37%) used resting state EEG to investigate possible biomarkers for cognitive decline in PD patients (Schlede et al., 2011; Caviness et al., 2015, 2016; Latreille et al., 2015; Cozac et al., 2016; Utianski et al., 2016; Arnaldi et al., 2017), evaluate therapeutic effects (Galvez et al., 2018; Babiloni et al., 2019), and distinguish PDD patients from other dementia patients, such as AD and DLB (Babiloni et al., 2011; Bliwise et al., 2014). The EEG frequency spectrum characteristics (the spectral power and/or power density), the event-related desynchronization/synchronization (ERD/ERS), and the EEG connectivity were studied in rest state EEG while sleep EEG, microstate analysis and the grand total EEG (GTE) score were also investigated in PD patients.
This study has evaluated the temporal and spectral characteristics of EEG as well as cortico-muscular coherence (CMC) in PD patients. The definable slowing EEG activity can reflect decreased cognitive level of patients with PD while negative correlation between the GTE score and cognitive state was observed. Reduced ERPs represent the cognitive decline and attention deficits that can be associated with severity of motor symptoms. Abnormalities in β and δ frequency bands are the main manifestation of dyskinesia and cognitive decline in Parkinson's disease, respectively.
EEG spectral pattern is usually characterized in the range of 0–30 Hz with five internationally agreed frequency bands: δ (0–4 Hz), θ (5–7 Hz), α (8–13 Hz), β (14–30 Hz), and γ (>30 Hz) bands. It is one of the most studied EEG features in PD patients. Studies showed that cognitive deficits are correlated with a slowing of EEG frequencies. Compared to non-demented PD patients, PD patients with dementia (PDD) have increased amplitude in lower frequency bands (i.e., θ and δ bands) and decreased amplitude in higher frequency bands (i.e., α and β bands; Soikkeli et al., 1991; Stoffers et al., 2007; Caviness et al., 2015; Arnaldi et al., 2017; Geraedts et al., 2018). More studies revealed the increased activities in θ and δ bands of EEG in PD patients (Babiloni et al., 2011; Caviness et al., 2016). Cozac et al. (2016) analyzed low frequency bands and demonstrated that global relative median power (GRMP) spectra of θ band can be used to predict cognitive decline. Despite the attenuated mid-frontal θ activity associated with disease duration, PD patients exhibited increased central θ power and decreased occipital θ power (Palmer et al., 2010; Singh et al., 2018). The abnormality of posterior cortical θ rhythm based on cortical source mapping of resting state EEG was observed in Babiloni et al. (2011). The θ relative band power of PD patients with mild cognitive impairment (PD-MCI) was higher than that of cognitively normal PD patients (PD-CogNl). An increasing trend was observed in the δ band power from PD-CogNl to PD-MCI to PDD (Caviness et al., 2016). Longitudinal changes of neuropsychological test were mostly related to the change of δ power for PD patients with mild to moderate motor impairment (Caviness et al., 2015). PD patients had higher diffuse δ source compared to HC group which may lead to abnormal sources of central δ rhythms in PDD patients (Babiloni et al., 2011, 2019). The increased power in δ band could be the main feature of the deterioration of cognitive impairment, which is closely related to the incidence of PDD (Caviness et al., 2015, 2016).
Event-related desynchronization (ERD) and event-related synchronization (ERS) are considered to indicate the activation of the motor cortex during planning, executing and completing a movement (Heinrichs-Graham et al., 2014; Tard et al., 2016; Delval et al., 2018). Previous studies have shown the α band rhythm display an essential role in motor processing and enhanced β band oscillation may reflect motor control deficits during movement preparation (Cahn et al., 2013; Tard et al., 2016; Delval et al., 2018). The diminished β power ERD and prolonged β ERS in an attentional experiment suggest that the coupling between attention and motor preparation was impaired in PD-FOG (Tard et al., 2016). The relative bandpower in α band showed a decreasing trend in the longitudinal changes from PD-CogNl to PD-MCI to PDD as well as cortical changes were observed in PD patients (Babiloni et al., 2011, 2019; Caviness et al., 2016). PD patients had lower occipital α source compared to HC whilst PDD patients showed increased frontal-temporal α source and abnormal sources of posterior cortical β1 rhythm (Soikkeli et al., 1991; Babiloni et al., 2011, 2019). As the changes of β band power are related to gait initiation failure (GIF) events, abnormal β oscillation may be a biomarker of motor impairment in PD patients (Palmer et al., 2010; Quynh Tran et al., 2016).
The efficient neural communication between premotor and motor cortical areas was critical for motor control (Herz et al., 2014). The decrease in γ band power was observed in resting state EEG of PD patients (Stoffers et al., 2007). PD patients exhibited a loss of γ-γ coupling from the lateral premotor cortex (lPM) to the supplementary motor area (SMA) when performing repetitive movements of the right index finger at maximal rate. Cross-frequency coupling of the slow and fast band activities might facilitate information transmission across brain networks (Canolty and Knight, 2010). Kappaw was used to evaluate the weighted brain network and found the parameters increased in the θ and β bands while decreased in the δ and α bands in PD patients compared to healthy participants (Utianski et al., 2016). The results showed a hub shifting in the bands and peak background frequency exists in both higher and lower frequency bands. The shift from α/δ to θ/β coupling suggests a change in cortical network in PD (Utianski et al., 2016), and the enhancement of θ/β coupling from primary motor cortex (M1) to the lateral premotor cortex (LPM) is related to the improvement of levodopa-induced motor function (Herz et al., 2014). The brain graph theory network analysis based on EEG signals indicated that the network breakdown is associated with cognitive decline, manifested as less connectivity and decreased global network efficiency with a loss of highly connected cortical hubs (Utianski et al., 2016).
Changes of EEG spectrum can be associated with clinical assessment scales and motion performance. The power of θ and β bands are, respectively related to the scores of Montreal Cognitive Assessment (MoCA) and the motor part of the Unified Parkinson's Disease Rating Scale (UPDRS-III) (Singh et al., 2020). PD-FOG patients showed increased β power and decreased θ power in mid-frontal region when performing a lower-limb pedaling motor task needing intentional initiation and stopping of movement (Singh et al., 2020). The θ, α, and β activities in left posterior parietal cortex (PPC) were positively correlated to the motion parameters during single or dual task walking (conversation/email sending), indicating that the left PPC may be involved in sensory motor integration and gait control (Pizzamiglio et al., 2018). Studies also found that the power of δ, θ, and γ bands was significantly increased during the planning and execution stages of motor (particularly in the execution stage), followed by decreases in α, β, and low-γ bands after an execution cue (Combrisson et al., 2017; Delval et al., 2018).
Figure 2 summarizes the included studies on EEG spectrum characteristics related to Parkinson's disease in this review. A significant interrelation was observed between β band power changes and dyskinesias, whilst abnormalities in θ and δ bands were associated to cognitive decline in PD patients. The changes of the spectrum characteristics can be used to assess the cognitive and motor abilities of PD patients during treatments as a dynamic and quantitative method.
Figure 2. The correlation between EEG spectral characteristics in different frequency bands and cognition and motor functions of PD patients. Green indicates that the EEG spectral characteristics is correlated with cognitive function; red indicates that it is correlated with motor performance; gray indicates that EEG spectral patterns are used to assess clinical treatment outcomes. 1, spectral power/power density; 2, event-related desynchronization/synchronization (ERD/ERS); 3, EEG connectivity; “↑,” the increase trend; “↓,” the decrease trend; “↕,” different trends of EEG spectral characteristics in various brain regions when cognitive and/or motor symptoms appear; “*” indicates that the gradual changes of EEG features of patients from PD-CogNl to PD-MCI to PDD manifest decrements in cognitive level.
Event-related potentials (ERPs) refer to event-related voltage changes in brain regions in response to specific stimuli (e.g., visual, auditory, and somatosensory stimuli). The ERPs provide a powerful method for exploring the ongoing EEG activity that are time-locked to sensory, motor, and cognitive events, and can be used as an electrophysiological indicator of cognitive function (Butler et al., 2017; Maidan et al., 2019). Table 2 summarizes ERP-related studies included in this review. The results demonstrate that changes of ERPs have been utilized to identify cognitive decline and abnormal attention distribution of PD patients, and can be applied to investigate the correlation between cognitive and motor functions. All studies investigated ERPs induced by visual or auditory stimuli (n = 10), and five studies also discussed event-related potentials while performing motor tasks (n = 5).
The P300 ERP is the most investigated neural markers of attention and cognition. Studies have shown that changes in the amplitude and latency of the P300 ERP are related to attention and cognitive decline (Georgiev et al., 2015; Maidan et al., 2019). An increase of P3b latency was observed in PD patients in visual and/or auditory oddball paradigms and associated with disease severity, cognitive dysfunction and impaired activities (Matsui et al., 2007; Da Silva Lopes et al., 2014). It is shown in Figure 3A that PD patients had a significantly smaller amplitude of P3b in random number generation (RNG) task compared to that in ordered number generation (ONG) task. The execution of RNG task may require greater attention resources, therefore less random behavior in PD patients was observed due to depleted attention resources (Muente et al., 2015). As there is a relationship between the decrease of P3a amplitude and the duration and severity of PD, the P3a is regarded as a potential cognitive marker for the development of mild to moderate PD patients (Solis-Vivanco et al., 2015). The changes of EEG in PD patients can be used to identify the drug effect during treatment in which P3a amplitude is mainly related to motor symptoms and dopaminergic medication (Georgiev et al., 2015). However, it should be noted that the P300 ERP is highly sensitive to cognitive decline and attention disorders, which may also occur in other neurodegenerative diseases, such as AD (Maidan et al., 2019). Further study on P300 in a large representative sample will be needed to support the potential use of P300 as a biomarker for PD patients.
Figure 3. Typical P300 changes in PD patients. (A) A more pronounced reduction in P3b amplitude during the RNG task was observed than that with ONG task (Muente et al., 2015). (B) Topographic maps of P3a in HC group and PD patients in three H&Y stages (stage 1, 2, 3) during an auditory oddball paradigm. The P3a showed a frontocentral distribution and its amplitude was negatively associated with the severity of PD (Solis-Vivanco et al., 2015). (C) The P300 amplitude is significantly smaller in PD patients (dash line) compared to those in young and older adults (solid black line and gray line, respectively) during walking (Maidan et al., 2019). HC, healthy control; H&Y, Hoehn and Yahr scale; RNG, random number generation; ONG, ordered number generation. (Image sources were adapted with granted copyright permission.) Figure 3A is available at IOS Press through Muente et al. (2015).
Another measure commonly used to study ERPs is Event-Related Spectral Perturbation (ERSP), which evaluated the spectral power within various frequency bands (Possti et al., 2020). Changes in brain dynamics in the power of different frequency bands, i.e., delta, theta, alpha, and beta bands, were observed when PD patients, healthy elder, and young subjects participated in an auditory oddball cognitive task performed during standing and walking on a treadmill (Possti et al., 2020). PD patients showed higher alpha and beta power during single and dual task compared to older adults, while an early lower delta power was also found during dual task. The phenomenon suggests that further modulation of readiness and attention is required in elders and PD patients and leads to larger recruitment of brain resources.
Unexpected changes in task-irrelevant auditory stimuli are usually accompanied by the elicited ERP components associated with attention orientation, such as novelty-P3a, mismatch negativity (MMN), reorientation negativity (RON). They are collectively referred as “distraction potential” and have been used to study the brain mechanism of involuntary attention (IA) in PD patients (Solis-Vivanco et al., 2011). As shown in Figure 3B, Solis-Vivanco et al. (2015) found that PD patients, especially those receiving anti-PD drug treatments, had reduced P3a amplitudes in the frontal, central, and temporal regions during distracting oddball tasks. The changes in novelty detection and/or the direction of attention were only observed in severe PD patients (Karayanidis et al., 1995; Georgiev et al., 2015; Solis-Vivanco et al., 2015). It suggests that the MMN might be potential in identifying patients with PD dementia (PDD) (Bronnick et al., 2010). Solis-Vivanco et al. (2011) also studied the role of RON in assessing cognitive function of PD patients. Comparing with drug-naïve patients, RON amplitude of patients receiving drug treatment was significantly higher and closer to HC group, indicating that dopamine therapy can also regulate the reorientation of attention. It is proven that there is a correlation between RON latency and the behavioral outcome of advanced PD patients (Georgiev et al., 2015; Solis-Vivanco et al., 2015). Despite the above-mentioned potentials, Kotz and Gunter (2015) firstly studied the P600 potential in one patient with advanced PD for reflecting language-related deficits.
Understanding the neural mechanism underlying the cognitive-motor interference may have implications for predicting the decrement in dual task performance. The P300 ERP is regarded as an index of the amount of resources allocated to stimulus processing. The reduced amplitude of P300 is associated with worse attentional concentration and the longer latency is related to motor and cognitive abnormalities (Polich, 2007; Maidan et al., 2019). PD patients showed a decrease amplitude and an increased peak latency of P300 during sitting and walking tasks as shown in Figure 3C, but its amplitude when performing oddball task during standing is similar to those in healthy elderly and young groups (Yilmaz et al., 2017; Maidan et al., 2019). There is no significant association between FOG symptom and ERPs. The ERPs elicited in auditory oddball paradigm may not be suitable as a biomarker of FOG symptoms (Tard et al., 2016).
The lateralized readiness potential (LRP) reflects the preparation of motor activity and it is modulated by participants' observed response. Due to the lack of motor preparation in PD caused by dysfunctions in supplementary motor area (SMA) (D'Ostilio et al., 2013), the LRP might occur earlier and have larger amplitude in PD-FOG when comparing to PD-nFOG (Butler et al., 2017). The increased activation in the prefrontal cortex area in the occurrence of FOG suggests that PD patients with FOG would need more attentional source (probably from the lateral premotor area) compared with PD-nFOG. The change in LRP reflects over-recruitment of the lateral premotor area in order to compensate for SMA deficits, and it also indicates that the main defect of FOG might occur in the motor preparation stage (Shine et al., 2013). As the LRP can be regarded as a movement-related brain potential, the results from van Wouwe et al. (2014) demonstrated that inhibition of motor cortex is important to alleviate PD symptoms. Another study also found that PD patients had reduced motor potential (MP) and impaired action-sentence compatibility effect (Melloni et al., 2015).
EEG signals can be segmented into many different short topographies (microstates) which can be clustered into a limited number of landscape (Koenig et al., 1999). EEG microstates reflect a transient and stable brain topology on a millisecond temporal level and provide a powerful perspective on the dynamic changes of the brain. It has been proved that EEG microstate features, especially its duration, were associated with the cognition and perception, and different cognitive functions were related to specific microstates (Britz et al., 2010; Van de Ville et al., 2010; Milz et al., 2016). Figure 4 illustrates that the EEG microstate analysis can be used for investigating brain activity changes in neurodegenerative diseases. Compared to AD and HC groups, patients with Lewy body dementia (LBD) have longer durations of all microstate classes and the number of distinct microstates per second is also reduced (Schumacher et al., 2019b). As the fluctuation of cognitive and attentional disorders is an important feature of Lewy body dementia, the severity of cognitive fluctuation is positively associated with the slowing of microstate dynamics (McKeith et al., 2005, 2017).
Figure 4. Microstate class topographies for dynamic brain activities in Alzheimer's disease (AD) and Lewy body dementia (LBD) patients compared to healthy control (HC) group. The EEG signal was divided into a group of short, non-overlapping and quasi-stable microstates where the microstates (A–E) were related to over 70% of changes in brain activities. P-values result from comparing the group topographies between groups using TANOVA. (Image source: Schumacher et al., 2019b: use permitted under the Creative Commons CC BY license).
The slowing of microstate dynamics indicates a relative loss of brain variability at resting state in patients with LBD (Schumacher et al., 2019b), which is consistent with the loss of brain network flexibility observed in previous fMRI studies (McIntosh et al., 2008; Jia et al., 2014; Schumacher et al., 2019a). The average duration of microstates in LBD patients is inversely related to dynamic functional connections between the basal ganglia and thalamus or large cortical networks, indicating that the dynamic interactions in the cortical-basal ganglia-thalamic loop participate in the regulation of global microstate dynamics and the subcortical abnormalities have a significant impact on the overall function of the whole brain network (Bell and Shine, 2016; Schumacher et al., 2018).
Recent studies show that sleep spindle and slow waves might facilitate understanding the mechanism of brain plasticity and the results have been related to human cognitive ability. However, the potential use of sleep EEG as a biomarker of cognitive decline in PD is still unclear (Latreille et al., 2015). In a longitudinal study, PD patients who develop dementia after 4.5 years of follow-up study showed decreased density and amplitude of sleep spindle in baseline polysomnography at the posterior cortical area: The PDD patients had most decrease in sleep spindles density, followed by PD patients without dementia, and the HC group has the least (Latreille et al., 2015). The result indicates that the sleep spindle density might be used a potential marker of cognitive decline in PD.
Cognitive fluctuation is a noteworthy feature of DLB rather than PD (Bliwise et al., 2014). In comparison of the coefficients of variation (COVs) based on the polysomnography, DLB patients have significantly greater cognitive fluctuation that PD patients even when they have similar level of cognitive impairment. Although PD patients have smaller amplitude of slow waves compared to healthy people, there is no significant difference observed between patients with different cognitive levels. Slow waves might not be suitable for predicting the cognitive function (Latreille et al., 2015).
The grand total EEG (GTE) score is a rating scale for clinical EEG analysis which can be used for the diagnosis of AD and LBD. It can distinguish between AD and other types of dementia (Pijnenburg et al., 2008). The original GTE score consists of six subscales: (i) frequency of rhythmic background activity, (ii) diffuse slow activity, (iii) reactivity of rhythmic background activity, (iv) paroxysmal activity, (v) focal abnormalities, and (vi) sharp wave activity. A negative relationship between scores (i)–(iii) and cognitive decline was observed in Schlede et al. (2011) by analyzing the correlation between GTE scores and cognitive levels in PD patients. The scores could be potential to identify MCI, which is a common non-motor symptom in PD and regarded as a transitional state between normal cognition and PDD.
The cortico-muscular coherence (CMC) reflects functional coupling between the cortical activity and muscle activity (Wagner et al., 2019). It has been widely used in studies to assess the recovery of motor function after stroke by quantifying interaction between the motor cortex and controlled muscles, however the CMC is rarely used in PD patients (Ushiyama et al., 2011; Zheng et al., 2018).
The findings in CMC features of PD patients are not yet consistent. Several studies have shown that PD patients exhibit motor abnormalities during ankle movement (Yoshida et al., 2018; Zheng et al., 2018). Although there was no difference in CMC even when movement performance significantly varied between PD patients and age-matched HC group (Andrykiewicz et al., 2007). The increased CMC of the midline cortical areas and tibialis anterior muscle in β band during maximum dorsiflexion and plantarflexion is opposite to results from previous study in which decreased β band CMC in PD patients during continuous isometric wrist extension was found (Omlor et al., 2007). Levels of cortico-muscular β band coherence during forearm contraction were found to be similar in patients with PD and HC group (Pollok et al., 2012). Different sensorimotor loops are responsible for upper and lower limb movements, which may explain the inconsistent results among the existing studies. The task-related and movement-related effect of CMC should be concerned when researchers investigate changes of functional interactions between brain activity and movement kinematics using CMC analysis.
PD patients have less global efficiency and functional connectivity of the brain network during the cognitive level declines (Utianski et al., 2016), which might be caused by pathological oscillations and abnormal regulation in the cortico-basal ganglia-thalamic-cortical pathways (Palmer et al., 2010; Herz et al., 2014). Studies have shown that the motor and cognitive dysfunction of PD patients can be related to abnormal changes in multiple pathways involving several brain regions, mainly including the basal ganglia (BG), thalamus, and frontal region. The changes of brain networks in PD were summarized in Figure 5.
Figure 5. Brain pathways involved in PD cognitive and motor dysfunctions. Neural information is transmitted from the cerebral cortex to the basal ganglia or thalamus and back to the cortex as shown in path 1. Path 2 demonstrates that the input from the thalamus in the basal ganglia is projected to the motor cortex or PPN, reflecting the pathological activities within the ganglia which can affect motor control process. The internal connection of the motor cortex in path 3 has a great influence on motor functions of PD patients. Path 4 and 5 are related to the striatum showing that the frontostriatal circuit and the basal ganglia-striatal circuit are both damaged in PD patients. PFC, prefrontal cortex; lPM, lateral premotor cortex; M1, primary motor cortex; SMA, supplementary motor area; PPN, pedunculopontine nucleus.
The BG circuits play a key role in high-level cognitive function and motor control (Melloni et al., 2015). The loss of dopamine impacts the network between the prefrontal cortex and BG which leads to inability to coordinate the selection and inhibition of conflict responses (Aron et al., 2007). The damage of the BG-cortical motor network consisting the loop from the frontal lobe to BG/thalamus and back to the cortex, may cause downstream influence on the cortical-subcortical connectivity and interfere with the cortical-subcortical motor network (Garcia and Ibanez, 2014; Melloni et al., 2015). Moreover, the cortical-BG-thalamic circuit is an important contributor to large-scale network communication in the brain, in which the dynamic interaction of the various components plays a role in the regulation of brain dynamics (Bell and Shine, 2016). Several different locations in the frontal area are connected to specific areas of the striatum and thalamus through parallel circuits (Cummings, 1995). Pathologic activities in the ganglia are input from the thalamus to the BG, and then projected to the motor cortex or pedunculopontine nucleus (PPN) (Lanciego et al., 2012; Hunnicutt et al., 2014; Yoshida et al., 2018). Dysfunction within these circuits lead to symptomatic progression in PD, such as cognitive issues.
Insufficient motor preparation in PD patients is mainly caused by supplementary motor area (SMA) dysfunction and can be compensated by overrecruiting larger cortical areas, such as lateral premotor cortex (lPM) (D'Ostilio et al., 2013; Butler et al., 2017). Both lPM and SMA are located in Brodmann Area 6 (BA 6), while the primary motor cortex (M1) is in Brodmann Area 4 (BA 4). These three regions are all located near the precentral gyrus and the connections between them (shown as Path 3 in Figure 5) affect movement function in PD (Herz et al., 2014). Increased activities in the prefrontal cortex (PFC) has been observed in PD patients during dual-task walking, which indicates a potential compensation mechanism involving the recruitment of attention networks (Holtzer et al., 2015; Maidan et al., 2016a, 2019). The activation of the PFC has been proven to have an essential role in cognitive control (Singh et al., 2018) and also involve in initiation and execution of movement (Singh et al., 2020).
The EEG analysis provides parameters to quantitatively evaluate cognitive deficits related to the severity of PD, making it a promising approach to assess the therapeutic efficacy. Galvez et al. (2018) investigated the possibility of binaural-rhythmic sound simulation to be applied as an effective therapy using EEG data. The results showed that the decrease of θ power in PD patients after receiving auditory stimuli, demonstrating that the changes of EEG in PD patients could detect the drug effect before and after the treatment (Georgiev et al., 2015). The P3a amplitude is mainly related to motor symptoms and dopaminergic medication. Moreover, the onset of FOG could be identified by tracking the dynamic changes of EEG (Shine et al., 2014; Handojoseno et al., 2015, 2018). Handojoseno et al. (2018) established a classifier with a sensitivity of 85.86% and a specificity of 80.5% for the prediction of the transition period of FOG (5 s before FOG onset).
Studies have shown that EEG energy power, entropy, and signal correlation are significantly related to FOG symptom in PD. However, the relationship between cognitive and motor symptoms in PD is not well-understood. Current studies have a variety of sample size and experimental setting (Herz et al., 2014; Georgiev et al., 2015; Singh et al., 2018), thus leading to inconsistent conclusions. Further studies are needed to elucidate the relative contributions of EEG patterns on reflecting the cognitive and motor symptoms and their relationship. A better understanding of neural mechanism based on EEG analysis related to PD is crucial to develop novel invasive clinical diagnostic and therapeutic methods.
This paper reviewed recent 10 years studies related to cognitive and motor deficits in PD patients using EEG analysis. The studies show that PD patients have significant changes in specific EEG patterns compared to healthy people. Movement abnormalities and cognitive decline can be related to changes in EEG spectrum and ERPs during typical oddball paradigms and/or combined motor tasks. The unique time-frequency characteristics of EEG could provide further insight into disrupted sensorimotor networks in PD with motor deficits, such as FOG, dyskinesia, and dystonia in a real-world environment. However, the mechanism underlying interaction between gait and cognition still needs to be further studied.
The original contributions presented in the study are included in the article/supplementary material, further inquiries can be directed to the corresponding author/s.
LM, QW, XZ, and DM: conception and design of the study. QW and JP: acquisition, analysis, and interpretation of literatures. QW and LM: drafting the manuscript. All authors critically revised the draft and approved the final version.
This work was supported by National Key R&D Program of China (2016YFC1306501), Ministry of Science and Technology of China (2016YFC1306500 and 2016YFC1306504), the Science & Technology Development Fund of Tianjin Education Commission for Higher Education (2017ZD10), and Tianjin University Talent Support Grant (2020XRY-0014). National Natural Science Foundation of China (No. 82001921).
The authors declare that the research was conducted in the absence of any commercial or financial relationships that could be construed as a potential conflict of interest.
Amboni, M., Cozzolino, A., Longo, K., Picillo, M., and Barone, P. (2008). Freezing of gait and executive functions in patients with Parkinson's disease. Mov. Disord. 23, 395–400. doi: 10.1002/mds.21850
Andrykiewicz, A., Patino, L., Naranjo, J. R., Witte, M., Hepp-Reymond, M.-C., and Kristeva, R. (2007). Corticomuscular synchronization with small and large dynamic force output. BMC Neurosci. 8:101. doi: 10.1186/1471-2202-8-101
Arnaldi, D., De Carli, F., Fama, F., Brugnolo, A., Girtler, N., Picco, A., et al. (2017). Prediction of cognitive worsening in de novo parkinson's disease: clinical use of biomarkers. Mov. Disord. 32, 1738–1747. doi: 10.1002/mds.27190
Aron, A. R., Behrens, T. E., Smith, S., Frank, M. J., and Poldrack, R. A. (2007). Triangulating a cognitive control network using diffusion-weighted magnetic resonance imaging (MRI) and functional MRI. J. Neurosci. 27, 3743–3752. doi: 10.1523/JNEUROSCI.0519-07.2007
Babiloni, C., De Pandis, M. F., Vecchio, F., Buffo, P., Sorpresi, F., Frisoni, G. B., et al. (2011). Cortical sources of resting state electroencephalographic rhythms in parkinson's disease related dementia and alzheimer's disease. Clin. Neurophysiol. 122, 2355–2364. doi: 10.1016/j.clinph.2011.03.029
Babiloni, C., Del Percio, C., Lizio, R., Noce, G., Lopez, S., Soricelli, A., et al. (2019). Levodopa may affect cortical excitability in parkinson's disease patients with cognitive deficits as revealed by reduced activity of cortical sources of resting state electroencephalographic rhythms. Neurobiol. Aging 73, 9–20. doi: 10.1016/j.neurobiolaging.2018.08.010
Bell, P. T., and Shine, J. M. (2016). Subcortical contributions to large-scale network communication. Neurosci. Biobehav. Rev. 71, 313–322. doi: 10.1016/j.neubiorev.2016.08.036
Bliwise, D. L., Scullin, M. K., and Trotti, L. M. (2014). Fluctuations in cognition and alertness vary independently in dementia with lewy bodies. Mov. Disord. 29, 83–89. doi: 10.1002/mds.25707
Britz, J., Van De Ville, D., and Michel, C. M. (2010). BOLD correlates of EEG topography reveal rapid resting-state network dynamics. Neuroimage 52, 1162–1170. doi: 10.1016/j.neuroimage.2010.02.052
Bronnick, K. S., Nordby, H., Larsen, J. P., and Aarsland, D. (2010). Disturbance of automatic auditory change detection in dementia associated with parkinson's disease: a mismatch negativity study. Neurobiol. Aging 31, 104–113. doi: 10.1016/j.neurobiolaging.2008.02.021
Butler, J. S., Fearon, C., Killane, I., Waechter, S. M., Reilly, R. B., and Lynch, T. (2017). Motor preparation rather than decision-making differentiates parkinson's disease patients with and without freezing of gait. Clin. Neurophysiol. 128, 463–471. doi: 10.1016/j.clinph.2016.12.019
Cahn, B. R., Delorme, A., and Polich, J. (2013). Event-related delta, theta, alpha and gamma correlates to auditory oddball processing during vipassana meditation. Soc. Cogn. Affect. Neurosci. 8, 100–111. doi: 10.1093/scan/nss060
Canolty, R. T., and Knight, R. T. (2010). The functional role of cross-frequency coupling. Trends Cogn. Sci. 14, 506–515. doi: 10.1016/j.tics.2010.09.001
Caviness, J. N., Hentz, J. G., Belden, C. M., Shill, H. A., Driver-Dunckley, E. D., Sabbagh, M. N., et al. (2015). Longitudinal EEG changes correlate with cognitive measure deterioration in parkinson's disease. J. Parkinsons Dis. 5, 117–124. doi: 10.3233/JPD-140480
Caviness, J. N., Utianski, R. L., Hentz, J. G., Beach, T. G., Dugger, B. N., Shill, H. A., et al. (2016). Differential spectral quantitative electroencephalography patterns between control and Parkinson's disease cohorts. Eur. J. Neurol. 23, 387–392. doi: 10.1111/ene.12878
Combrisson, E., Perrone-Bertolotti, M., Soto, J. L. P., Alamian, G., Kahane, P., Lachaux, J. P., et al. (2017). From intentions to actions: neural oscillations encode motor processes through phase, amplitude and phase-amplitude coupling. Neuroimage 147, 473–487. doi: 10.1016/j.neuroimage.2016.11.042
Cozac, V. V., Chaturvedi, M., Hatz, F., Meyer, A., Fuhr, P., and Gschwandtner, U. (2016). Increase of EEG Spectral theta power indicates higher risk of the development of severe cognitive decline in parkinson's disease after 3 years. Front. Aging Neurosci. 8:284. doi: 10.3389/fnagi.2016.00284
Cummings, J. L. (1995). Anatomic and behavioral aspects of frontal-subcortical circuits. Ann. N. Y. Acad. Sci. 769, 1–13. doi: 10.1111/j.1749-6632.1995.tb38127.x
Da Silva Lopes, M., de Souza Melo, A., and Nobrega, A. C. (2014). Delayed latencies of auditory evoked potential P300 are associated with the severity of Parkinson's disease in older patients. Arq. Neuropsiquiatr. 72, 296–300. doi: 10.1590/0004-282X20140005
Delval, A., Braquet, A., Dirhoussi, N., Bayot, M., Derambure, P., Defebvre, L., et al. (2018). Motor preparation of step initiation: error-related cortical oscillations. Neuroscience 393, 12–23. doi: 10.1016/j.neuroscience.2018.09.046
D'Ostilio, K., Deville, B., Cremers, J., Grandjean, J., Skawiniak, E., Delvaux, V., et al. (2013). Role of the supplementary motor area in the automatic activation of motor plans in de novo Parkinson's disease patients. Neurosci. Res. 76, 173–177. doi: 10.1016/j.neures.2013.04.002
Galvez, G., Recuero, M., Canuet, L., and Del-Pozo, F. (2018). Short-term effects of binaural beats on eeg power, functional connectivity, cognition, gait and anxiety in Parkinson's disease. Int. J. Neural Syst 28:17500551. doi: 10.1142/S0129065717500551
Garcia, A. M., and Ibanez, A. (2014). Words in motion: motor-language coupling in Parkinson's disease. Transl. Neurosci. 5, 152–159. doi: 10.2478/s13380-014-0218-6
Georgiev, D., Jahanshahi, M., Dreo, J., Cus, A., Pirtosek, Z., and Repovs, G. (2015). Dopaminergic medication alters auditory distractor processing in Parkinson's disease. Acta Psychol. 156, 45–56. doi: 10.1016/j.actpsy.2015.02.001
Geraedts, V. J., Boon, L. I., Marinus, J., Gouw, A. A., van Hilten, J. J., Stam, C. J., et al. (2018). Clinical correlates of quantitative EEG in Parkinson disease a systematic review. Neurology 91, 871–883. doi: 10.1212/WNL.0000000000006473
Handojoseno, A. M. A., Naik, G. R., Gilat, M., Shine, J. M., Nguyen, T. N., Ly, Q. T., et al. (2018). Prediction of freezing of gait in patients with parkinson's disease using EEG signals. Stud. Health Technol. Inform 246, 124–131. doi: 10.3233/978-1-61499-845-7-124
Handojoseno, A. M. A., Shine, J. M., Nguyen, T. N., Tran, Y., Lewis, S. J. G., and Nguyen, H. T. (2013). “Using EEG spatial correlation, cross frequency energy, and wavelet coefficients for the prediction of freezing of gait in Parkinson's disease patients,” in 2013 35th Annual International Conference Of the IEEE Engineering In Medicine And Biology Society (Osaka: IEEE), 4263–4266.
Handojoseno, A. M. A., Shine, J. M., Nguyen, T. N., Tran, Y., Lewis, S. J. G., and Nguyen, H. T. (2015). Analysis and prediction of the freezing of gait using EEG brain dynamics. IEEE Trans. Neural Syst. Rehabil. Eng. 23, 887–896. doi: 10.1109/TNSRE.2014.2381254
Heinrichs-Graham, E., Wilson, T. W., Santamaria, P. M., Heithoff, S. K., Torres-Russotto, D., Hutter-Saunders, J. A. L., et al. (2014). Neuromagnetic evidence of abnormal movement-related beta desynchronization in parkinson's disease. Cereb. Cortex 24, 2669–2678. doi: 10.1093/cercor/bht121
Herz, D. M., Florin, E., Christensen, M. S., Reck, C., Barbe, M. T., Tscheuschler, M. K., et al. (2014). Dopamine replacement modulates oscillatory coupling between premotor and motor cortical areas in parkinson's disease. Cereb. Cortex 24, 2873–2883. doi: 10.1093/cercor/bht140
Holtzer, R., Mahoney, J. R., Izzetoglu, M., Wang, C. L., England, S., and Verghese, J. (2015). Online fronto-cortical control of simple and attention-demanding locomotion in humans. Neuroimage 112, 152–159. doi: 10.1016/j.neuroimage.2015.03.002
Hunnicutt, B. J., Long, B. R., Kusefoglu, D., Gertz, K. J., Zhong, H. N., and Mao, T. Y. (2014). A comprehensive thalamocortical projection map at the mesoscopic level. Nat. Neurosci. 17, 1276–1285. doi: 10.1038/nn.3780
Jia, H., Hu, X., and Deshpande, G. (2014). Behavioral relevance of the dynamics of the functional brain connectome. Brain Connect. 4, 741–759. doi: 10.1089/brain.2014.0300
Karayanidis, F., Andrews, S., Ward, P. B., and Michie, P. T. (1995). ERP indices of auditory selective attention in aging and Parkinson's disease. Psychophysiology 32, 335–350. doi: 10.1111/j.1469-8986.1995.tb01216.x
Koenig, T., Lehmann, D., Merlo, M. C. G., Kochi, K., Hell, D., and Koukkou, M. (1999). A deviant EEG brain microstate in acute, neuroleptic-naive schizophrenics at rest. Eur. Arch. Psychiatry Clin. Neurosci. 249, 205–211. doi: 10.1007/s004060050088
Kotz, S. A., and Gunter, T. C. (2015). Can rhythmic auditory cuing remediate language-related deficits in Parkinson's disease? Ann N Y Acad Sci. 1337, 62–68. doi: 10.1111/nyas.12657
Lanciego, J. L., Luquin, N., and Obeso, J. A. (2012). Functional neuroanatomy of the basal ganglia. Cold Spring Harb. Perspect. Med. 2:a009621. doi: 10.1101/cshperspect.a009621
Latreille, V., Carrier, J., Lafortune, M., Postuma, R. B., Bertrand, J.-A., Panisset, M., et al. (2015). Sleep spindles in Parkinson's disease may predict the development of dementia. Neurobiol. Aging 36, 1083–1090. doi: 10.1016/j.neurobiolaging.2014.09.009
Lewis, S. J. G., and Barker, R. A. (2009). A pathophysiological model of freezing of gait in Parkinson's disease. Parkinsonism Relat. Disord. 15, 333–338. doi: 10.1016/j.parkreldis.2008.08.006
Maidan, I., Fahoum, F., Shustak, S., Gazit, E., Patashov, D., Tchertov, D., et al. (2019). Changes in event-related potentials during dual task walking in aging and Parkinson's disease. Clin. Neurophysiol. 130, 224–230. doi: 10.1016/j.clinph.2018.11.019
Maidan, I., Nieuwhof, F., Bernad-Elazari, H., Reelick, M. F., Bloem, B. R., Giladi, N., et al. (2016a). The role of the frontal lobe in complex walking among patients with parkinson's disease and healthy older adults: an fNIRS study. Neurorehabil. Neural Repair. 30, 963–971. doi: 10.1177/1545968316650426
Maidan, I., Rosenberg-Katz, K., Jacob, Y., Giladi, N., Deutsch, J. E., Hausdorff, J. M., et al. (2016b). Altered brain activation in complex walking conditions in patients with Parkinson's disease. Parkinsonism Relat. Disord. 25, 91–96. doi: 10.1016/j.parkreldis.2016.01.025
Martinez-Martin, P. (2011). The importance of non-motor disturbances to quality of life in Parkinson's disease. J. Neurol. Sci. 310, 12–16. doi: 10.1016/j.jns.2011.05.006
Martinez-Martin, P., Rodriguez-Blazquez, C., Kurtis, M. M., Chaudhuri, K. R., and Grp, N. V. (2011). The impact of non-motor symptoms on health-related quality of life of patients with parkinson's disease. Mov. Disord. 26, 399–406. doi: 10.1002/mds.23462
Maruyama, T., and Yanagisawa, N. (2006). Cognitive impact on freezing of gait in Parkinson's disease. Parkinsonism Relat. Disord. 12, S77–S82. doi: 10.1016/j.parkreldis.2006.05.024
Matsui, H., Nishinaka, K., Oda, M., Kubori, T., and Udaka, F. (2007). Auditory event-related potentials in Parkinson's disease: prominent correlation with attention. Parkinsonism Relat. Disord. 13, 394–398. doi: 10.1016/j.parkreldis.2006.12.012
McIntosh, A. R., Kovacevic, N., and Itier, R. J. (2008). Increased brain signal variability accompanies lower behavioral variability in development. PLoS Comput. Biol. 4:e1000106. doi: 10.1371/journal.pcbi.1000106
McKeith, I. G., Boeve, B. F., Dickson, D. W., Halliday, G., Taylor, J.-P., Weintraub, D., et al. (2017). Diagnosis and management of dementia with lewy bodies fourth consensus report of the DLB consortium. Neurology 89, 88–100. doi: 10.1212/WNL.0000000000004058
McKeith, I. G., Dickson, D. W., Lowe, J., Emre, M., O'Brien, J. T., Feldman, H., et al. (2005). Diagnosis and management of dementia with lewy bodies - third report of the DLB consortium. Neurology 65, 1863–1872. doi: 10.1212/01.wnl.0000187889.17253.b1
Melloni, M., Sedeno, L., Hesse, E., Garcia-Cordero, I., Mikulan, E., Plastino, A., et al. (2015). Cortical dynamics and subcortical signatures of motor-language coupling in Parkinson's disease. Sci. Rep. 5:11899. doi: 10.1038/srep11899
Milz, P., Faber, P. L., Lehmann, D., Koenig, T., Kochi, K., and Pascual-Marqui, R. D. (2016). The functional significance of EEG microstates-associations with modalities of thinking. Neuroimage 125, 643–656. doi: 10.1016/j.neuroimage.2015.08.023
Muente, T. F., Joppich, G., Daeuper, J., Schrader, C., Dengler, R., and Heldmann, M. (2015). Random number generation and executive functions in parkinson's disease: an event-related brain potential study. J. Parkinsons Dis. 5, 613–620. doi: 10.3233/JPD-150575
Nathan, K., and Contreras-Vidal, J. L. (2016). Negligible motion artifacts in scalp electroencephalography (EEG) during treadmill walking. Front. Hum. Neurosci. 9:708. doi: 10.3389/fnhum.2015.00708
Omlor, W., Patino, L., Hepp-Reymond, M.-C., and Kristeva, R. (2007). Gamma-range corticomuscular coherence during dynamic force output. Neuroimage 34, 1191–1198. doi: 10.1016/j.neuroimage.2006.10.018
Palmer, S. J., Lee, P. W.-H., Wang, Z. J., Au, W.-L., and McKeown, M. J. (2010). theta, beta but not alpha-band EEG connectivity has implications for dual task performance in Parkinson's disease. Parkinsonism Relat. Disord. 16, 393–397. doi: 10.1016/j.parkreldis.2010.03.001
Peterson, D. S., Pickett, K. A., Duncan, R. P., Perlmutter, J. S., and Earhart, G. M. (2014). Brain activity during complex imagined gait tasks in Parkinson disease. Clin. Neurophysiol. 125, 995–1005. doi: 10.1016/j.clinph.2013.10.008
Pijnenburg, Y. A. L., Strijers, R. L. M., Made, Y. V., van der Flier, W. M., Scheltens, P., and Stam, C. J. (2008). Investigation of resting-state EEG functional connectivity in frontotemporal lobar degeneration. Clin. Neurophysiol. 119, 1732–1738. doi: 10.1016/j.clinph.2008.02.024
Pizzamiglio, S., Abdalla, H., Naeem, U., and Turner, D. L. (2018). Neural predictors of gait stability when walking freely in the real-world. J. Neuroeng. Rehabil. 15:11. doi: 10.1186/s12984-018-0357-z
Polich, J. (2007). Updating p300: an integrative theory of P3a and P3b. Clin. Neurophysiol. 118, 2128–2148. doi: 10.1016/j.clinph.2007.04.019
Pollok, B., Krause, V., Martsch, W., Wach, C., Schnitzler, A., and Sudmeyer, M. (2012). Motor-cortical oscillations in early stages of Parkinson's disease. J. Physiol. Lond. 590, 3203–3212. doi: 10.1113/jphysiol.2012.231316
Possti, D., Fahoum, F., Sosnik, R., Giladi, N., Hausdorff, J. M., Mirelman, A., et al. (2020). Changes in the EEG spectral power during dual-task walking with aging and Parkinson's disease: initial findings using event-related spectral perturbation analysis. J. Neurol. doi: 10.1007/s00415-020-10104-1
Quynh Tran, L., Handojoseno, A. M. A., Gilat, M., Nghia, N., Chai, R., Yvonne, T., et al. (2016). “Detection of gait initiation failure in parkinson's disease patients using EEG signals,” in 2016 38th Annual International Conference of the IEEE Engineering in Medicine and Biology Society, eds J. Patton, R. Barbieri, J. Ji, E. Jabbari, S. Dokos, R. Mukkamala, et al., (Orlando: IEEE), 1599–1602.
Robinovitch, S. N., Feldman, F., Yang, Y. J., Schonnop, R., Leung, P. M., Sarraf, T., et al. (2013). Video capture of the circumstances of falls in elderly people residing in long-term care: an observational study. Lancet 381, 47–54. doi: 10.1016/S0140-6736(12)61263-X
Rushworth, M. F. S., Hadland, K. A., Paus, T., and Sipila, P. K. (2002). Role of the human medial frontal cortex in task switching: a combined fMRI and TMS study. J. Neurophysiol. 87, 2577–2592. doi: 10.1152/jn.2002.87.5.2577
Schlede, N., Zimmermann, R., Ehrensperger, M. M., Gschwandtner, U., Hardmeier, M., Hatz, F., et al. (2011). Clinical EEG in cognitively impaired patients with Parkinson's disease. J. Neurol. Sci. 310, 75–78. doi: 10.1016/j.jns.2011.05.034
Schumacher, J., Peraza, L. R., Firbank, M., Thomas, A. J., Kaiser, M., Gallaghe, P., et al. (2019a). Dynamic functional connectivity changes in dementia with lewy bodies and Alzheimer's disease. Neuroimage Clin. 22:101812. doi: 10.1016/j.nicl.2019.101812
Schumacher, J., Peraza, L. R., Firbank, M., Thomas, A. J., Kaiser, M., Gallagher, P., et al. (2018). Functional connectivity in dementia with lewy bodies: a within- and between-network analysis. Hum. Brain Mapp. 39, 1118–1129. doi: 10.1002/hbm.23901
Schumacher, J., Peraza, L. R., Firbank, M., Thomas, A. J., Kaiser, M., Gallagher, P., et al. (2019b). Dysfunctional brain dynamics and their origin in lewy body dementia. Brain 142, 1767–1782. doi: 10.1093/brain/awz069
Shine, J. M., Handojoseno, A. M. A., Nguyen, T. N., Tran, Y., Naismith, S. L., Nguyen, H., et al. (2014). Abnormal patterns of theta frequency oscillations during the temporal evolution of freezing of gait in Parkinson's disease. Clin. Neurophysiol. 125, 569–576. doi: 10.1016/j.clinph.2013.09.006
Shine, J. M., Matar, E., Ward, P. B., Bolitho, S. J., Gilat, M., Pearson, M., et al. (2013). Exploring the cortical and subcortical functional magnetic resonance imaging changes associated with freezing in Parkinson's disease. Brain 136, 1204–1215. doi: 10.1093/brain/awt049
Singh, A., Cole, R. C., Espinoza, A. I., Brown, D., Cavanagh, J. F., and Narayanan, N. S. (2020). Frontal theta and beta oscillations during lower-limb movement in Parkinson's disease. Clin. Neurophysiol. 131, 694–702. doi: 10.1016/j.clinph.2019.12.399
Singh, A., Richardson, S. P., Narayanan, N., and Cavanagh, J. F. (2018). Mid-frontal theta activity is diminished during cognitive control in Parkinson's disease. Neuropsychologia 117, 113–122. doi: 10.1016/j.neuropsychologia.2018.05.020
Soikkeli, R., Partanen, J., Soininen, H., Paakkonen, A., and Riekkinen, P. Sr. (1991). Slowing of EEG in Parkinson's disease. Electroencephalogr. Clin. Neurophysiol. 79, 159–165. doi: 10.1016/0013-4694(91)90134-P
Solis-Vivanco, R., Ricardo-Garcell, J., Rodriguez-Camacho, M., Prado-Alcala, R. A., Rodriguez, U., Rodriguez-Violante, M., et al. (2011). Involuntary attention impairment in early Parkinson's disease: an event-related potential study. Neurosci. Lett. 495, 144–149. doi: 10.1016/j.neulet.2011.03.058
Solis-Vivanco, R., Rodriguez-Violante, M., Rodriguez-Agudelo, Y., Schilmann, A., Rodriguez-Ortiz, U., and Ricardo-Garcell, J. (2015). The P3a wave: a reliable neurophysiological measure of Parkinson's disease duration and severity. Clin. Neurophysiol. 126, 2142–2149. doi: 10.1016/j.clinph.2014.12.024
Stoffers, D., Bosboom, J. L. W., Deijen, J. B., Wolters, E. C., Berendse, H. W., and Stam, C. J. (2007). Slowing of oscillatory brain activity is a stable characteristic of Parkinson's disease without dementia. Brain 130, 1847–1860. doi: 10.1093/brain/awm034
Tard, C., Dujardin, K., Bourriez, J.-L., Molaee-Ardekani, B., Derambure, P., Defebvre, L., et al. (2016). Attention modulation during motor preparation in Parkinsonian freezers: a time-frequency EEG study. Clin. Neurophysiol. 127, 3506–3515. doi: 10.1016/j.clinph.2016.09.014
Ushiyama, J., Suzuki, T., Masakado, Y., Hase, K., Kimura, A., Liu, M., et al. (2011). Between-subject variance in the magnitude of corticomuscular coherence during tonic isometric contraction of the tibialis anterior muscle in healthy young adults. J. Neurophysiol. 106, 1379–1388. doi: 10.1152/jn.00193.2011
Utianski, R. L., Caviness, J. N., van Straaten, E. C. W., Beach, T. G., Dugger, B. N., Shill, H. A., et al. (2016). Graph theory network function in Parkinson's disease assessed with electroencephalography. Clin. Neurophysiol. 127, 2228–2236. doi: 10.1016/j.clinph.2016.02.017
Van de Ville, D., Britz, J., and Michel, C. M. (2010). EEG microstate sequences in healthy humans at rest reveal scale-free dynamics. Proc. Natl. Acad. Sci. U.S.A. 107, 18179–18184. doi: 10.1073/pnas.1007841107
van Wouwe, N. C., van den Wildenberg, W. P. M., Claassen, D. O., Kanoff, K., Bashore, T. R., and Wylie, S. A. (2014). Speed pressure in conflict situations impedes inhibitory action control in Parkinson's disease. Biol. Psychol. 101, 44–60. doi: 10.1016/j.biopsycho.2014.07.002
Waechter, S. M., Fearon, C., McDonnell, C., Gallego, J., Quinlivan, B., Killane, I., et al. (2015). “The impact of dual tasking on cognitive performance in a Parkinson's disease cohort with and without freezing of gait: an EEG and behavioral based approach,” in 2015 7th International Ieee/Embs Conference on Neural Engineering (Montpellier), 1072–1075. doi: 10.1109/NER.2015.7146813
Wager, T. D., Jonides, J., and Reading, S. (2004). Neuroimaging studies of shifting attention: a meta-analysis. Neuroimage 22, 1679–1693. doi: 10.1016/j.neuroimage.2004.03.052
Wagner, J., Martinez-Canino, R., and Makeig, S. (2019). Trial-by-trial source-resolved EEG responses to gait task challenges predict subsequent step adaptation. Neuroimage 199, 691–703. doi: 10.1016/j.neuroimage.2019.06.018
Yilmaz, F. T., Ozkaynak, S. S., and Barcin, E. (2017). Contribution of auditory P300 test to the diagnosis of mild cognitive impairment in Parkinson's disease. Neurol. Sci. 38, 2103–2109. doi: 10.1007/s10072-017-3106-3
Yoshida, T., Masani, K., Zabjek, K., Popovic, M. R., and Chen, R. (2018). Dynamic cortical participation during bilateral, cyclical ankle movements: effects of Parkinson's disease. PLoS ONE 13:e0196177. doi: 10.1371/journal.pone.0196177
Yuvaraj, R., Murugappan, M., Ibrahim, N. M., Sundaraj, K., Omar, M. I., Mohamad, K., et al. (2014). Detection of emotions in Parkinson's disease using higher order spectral features from brain's electrical activity. Biomed. Signal Process. Control 14, 108–116. doi: 10.1016/j.bspc.2014.07.005
Keywords: Parkinson's disease (PD), electroencephalogram (EEG), motor disorders, cognitive decline, freezing of gait (FOG), dementia
Citation: Wang Q, Meng L, Pang J, Zhu X and Ming D (2020) Characterization of EEG Data Revealing Relationships With Cognitive and Motor Symptoms in Parkinson's Disease: A Systematic Review. Front. Aging Neurosci. 12:587396. doi: 10.3389/fnagi.2020.587396
Received: 05 August 2020; Accepted: 06 October 2020;
Published: 10 November 2020.
Edited by:
Liu Jun, Shanghai Jiao Tong University, ChinaCopyright © 2020 Wang, Meng, Pang, Zhu and Ming. This is an open-access article distributed under the terms of the Creative Commons Attribution License (CC BY). The use, distribution or reproduction in other forums is permitted, provided the original author(s) and the copyright owner(s) are credited and that the original publication in this journal is cited, in accordance with accepted academic practice. No use, distribution or reproduction is permitted which does not comply with these terms.
*Correspondence: Xiaodong Zhu, enhkMzUxNkB0bXUuZWR1LmNu; Dong Ming, cmljaGFyZG1pbmdAdGp1LmVkdS5jbg==
†These authors have contributed equally to this work and share first authorship
Disclaimer: All claims expressed in this article are solely those of the authors and do not necessarily represent those of their affiliated organizations, or those of the publisher, the editors and the reviewers. Any product that may be evaluated in this article or claim that may be made by its manufacturer is not guaranteed or endorsed by the publisher.
Research integrity at Frontiers
Learn more about the work of our research integrity team to safeguard the quality of each article we publish.