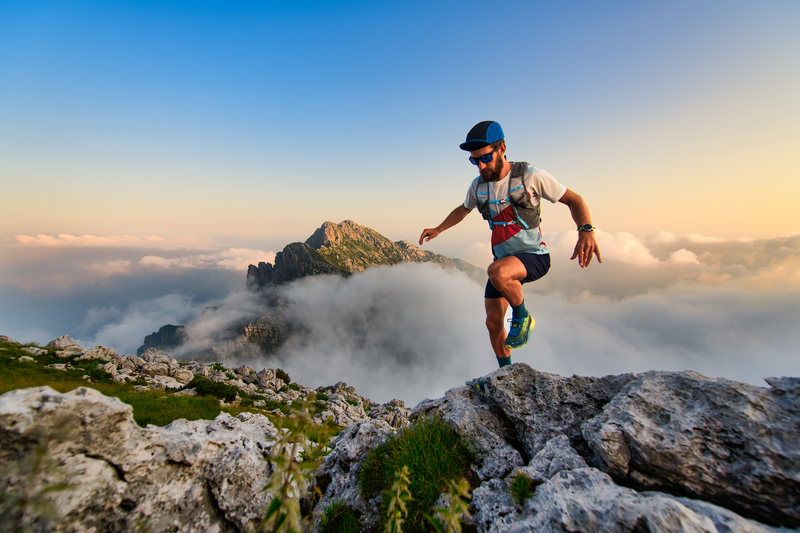
94% of researchers rate our articles as excellent or good
Learn more about the work of our research integrity team to safeguard the quality of each article we publish.
Find out more
ORIGINAL RESEARCH article
Front. Aging Neurosci. , 23 October 2020
Sec. Neurocognitive Aging and Behavior
Volume 12 - 2020 | https://doi.org/10.3389/fnagi.2020.511562
Post-stroke cognitive impairment (PSCI) is a common neuropsychiatric complication of stroke. Mounting evidence has demonstrated a connection between gut microbiota (GM) and neuropsychiatric disease. Our previous study revealed the changes in the GM in a mouse model of vascular dementia. However, the characteristic GM of PSCI remains unclear. This study aimed to characterize the GM of PSCI and explored the potential of GM as PSCI biomarkers. A total of 93 patients with ischemic stroke were enrolled in this study. The patients were divided into two groups according to their MoCA scores 3 months after stroke onset. Clinical data and biological variables were recorded. GM composition was analyzed using 16S ribosomal RNA sequencing, and the characteristic GM was identified by linear discriminant analysis Effect Size (Lefse). Our results showed that Proteobacteria was highly increased in the PSCI group compared with the post-stroke non-cognitive impairment (PSNCI) group, the similar alterations were also observed at the class, order, family, and genus levels of Proteobacteria. After age adjustments, the abundance of Firmicutes, and its members, including Clostridia, Clostridiales, Lachnospiraceae, and Lachnospiraceae_other, were significantly decreased in the age-matched PSCI group compared with the PSNCI group. Besides, the GM was closely associated with MoCA scores and the risk factors for PSCI, including higher baseline National Institute of Health Stroke Scale score, higher homocysteine (Hcy) level, higher prevalence of stroke recurrence, leukoaraiosis, and brain atrophy. The KEGG results showed the enriched module for folding, sorting and degradation (chaperones and folding catalysts) and the decreased modules related to metabolisms of cofactors and vitamins, amino acid, and lipid in PSCI patients. A significant correlation was observed between PSCI and the abundance of Enterobacteriaceae after adjustments (P = 0.035). Moreover, the receiver operating characteristic (ROC) models based on the characteristic GM and Enterobacteriaceae could distinguish PSCI patients from PSNCI patients [area under the curve (AUC) = 0.840, 0.629, respectively]. Our findings demonstrated that the characteristic GM, especially Enterobacteriaceae, might have the ability to predict PSCI in post-stroke patients, which are expected to be used as clinical biomarkers of PSCI.
Ischemic stroke is a major risk factor for cognitive impairment (Vijayan and Reddy, 2016b). The occurrence of cognitive impairment after stroke may be the result of vascular cognitive impairment or Alzheimer’s disease (AD) promoted by stroke, or both (Sun et al., 2014). Zekry et al. (2003) revealed that the infarcts in strategic regions are critical for the pathogenesis of cognitive impairment after stroke. Besides, stroke and cognitive impairment also share similar risk factors such as hypertension and diabetes mellitus, which contribute to cognitive impairment after stroke (Sun et al., 2014). Therefore, ischemic stroke is closely correlated with cognitive impairment. Post-stroke cognitive impairment (PSCI) is a common complication of stroke. In China, the prevalence of cognitive impairment 3 months after stroke ranges from 18 to 41.8% (Tang et al., 2006; Tu et al., 2014). PSCI is associated with poor clinical outcomes such as increased hospitalization, disability, and burden of care (Crichton et al., 2016), and functional impairment is more significant in stroke survivors with cognitive impairment. Previous studies have focused on the demographic, psychological, and biological variables influencing PSCI (Arba et al., 2017; Levine et al., 2018). However, the pathogenesis of PSCI remains unclear. Given that there is a prodromal period after stroke onset of 3 months or more before the development of PSCI (Ballard et al., 2003), it is of considerable significance to identify useful PSCI biomarkers.
Gut microbiota (GM) dysbiosis in neuropsychiatric disorders has been observed in human and animal studies. Recent studies showed that fecal microbial diversity and composition were significantly different between AD patients and healthy controls (Zhuang et al., 2018). The GM of AD patients was characterized by a higher abundance of bacteria inducing proinflammatory states, and a lower abundance of bacteria able to synthesize short-chain fatty acids (SCFAs) (Haran et al., 2019). The animal study confirmed the altered GM in a mouse model of AD, which was characterized by increased abundances of Verrucomicrobia and Proteobacteria, and decreased levels of Ruminococcus and Butyricicoccus (Zhang et al., 2017). Moreover, our previous study demonstrated that fecal microbiota transplantation could reduce AD symptoms in the APP/PS1 mouse model (Sun et al., 2019). Besides, patients with schizophrenia exhibited decreased GM diversity and microbial dysbiosis (Xu et al., 2019b), and transplantation of gut bacteria from schizophrenic patients into antibiotic-treated mice caused schizophrenia-like abnormal behaviors (Zhu et al., 2019). Increased abundances of opportunistic pathogens and decreased levels of butyrate-producing bacteria were identified as hallmarks of post-stroke GM dysbiosis (Yin et al., 2015). Animal studies indicated that the GM dysbiosis exacerbated the outcome of stroke, while transplantation of fecal microbiota or normalization of GM dysbiosis by antibiotics improved the outcome (Singh et al., 2016; Chen et al., 2019). Moreover, increasing evidence indicated the close correlation between the GM and cognitive impairment in different diseases (Bajaj et al., 2012; Carlson et al., 2018; Gao et al., 2019; Liu et al., 2019). However, the gut microbial characteristics in PSCI patients remain unclear.
Many studies used animal models to investigate the role of GM in the brain function, such as germ-free mice, and animal models treated with probiotics. For example, the germ-free mice showed impaired social behaviors (Diaz Heijtz et al., 2011; Neufeld et al., 2011), and structural alterations in the amygdala and prefrontal cortical (Stilling et al., 2015; Hoban et al., 2016). The previous study of germ-free animals had indicated that GM regulated neurogenesis, which modulated learning and memory (Ogbonnaya et al., 2015). Administration of probiotics to healthy rats and mice showed the alleviation of anxiety-like and depression-like behaviors (Dinan et al., 2013). Moreover, oral treatment with SCFAs could alleviate the impaired microglial function in germ-free animals, according to the previous study (Erny et al., 2015). Besides, fecal microbiota transplantation could transfer behavioral phenotypes (Collins et al., 2013). However, these studies were based on animal models, whether these findings of animal studies could be generalized to humans remained unclear. Therefore, there is a need to elucidate the relationship between GM and neuropsychiatric diseases in human studies.
In the present study, we aimed to investigate the GM composition in PSCI patients and GM’s association with MoCA scores and risk factors for PSCI. Besides, we further confirmed the characteristic GM of PSCI and its potential as a biomarker for the diagnosis of PSCI.
Ischemic stroke patients diagnosed and treated in the Second Affiliated Hospital and Yuying Children’s Hospital of Wenzhou Medical University from January to April 2019 were enrolled. The inclusion criteria were as follows: patients aged 40–90 years, ischemic stroke, with infarcts in non-strategic brain regions (including the subcortex, brain stem, and cerebellum). Exclusion criteria included the following: pre-existing dementia history, infarct of strategic regions (hippocampus, thalamus, frontal lobe, cingulate gyrus, angular gyrus, internal capsule, caudate nucleus), recent (within 3 months) use of antibiotics or probiotics, restrictive diet, gastrointestinal surgery, recent infection, psychosis such as schizophrenia or bipolar disease, severe life-threatening illnesses, communication deficits, and pregnancy. The Ethics Committee of the Second Affiliated Hospital of Wenzhou Medical University approved the study protocol, and all patients gave written informed consent.
Patients were assessed by the Montreal Cognitive Assessment (MoCA) 3 months after stroke onset. The MoCA, characterized by excellent specificity and sensitivity (Zietemann et al., 2018), is currently the most widely used tool to assess cognitive function, and includes visuospatial/executive function, naming, attention, abstraction, language, delayed recall, and orientation. We used the score of the Informant Questionnaire on Cognitive Decline in the Elderly (cut-off value > 4.0) to exclude pre-existing dementia. Patients were identified as PSCI as follows: MoCA score < 26 points for patients with junior school education level or above, MoCA score < 21 points for patients with primary school education level, and MoCA score < 15 points for illiterate patients. The remaining patients were identified as post-stroke non-cognitive impairment (PSNCI).
We collected the demographic information, including sex, age, divorce rate, and educational level, physical activity, sleep deprivation, smoking and alcohol status, previous history of stroke, and dietary habit of each patient from an interview, and the height and weight of each patient were obtained to calculate the body mass index (BMI). We determined whether the patients had hypertension, diabetes mellitus, dyslipidemia, and atrial fibrillation by inquiring about the history of previous diseases and measuring patients’ blood pressure, blood glucose, blood lipid, and electrocardiogram, respectively. In addition, patients were examined by brain magnetic resonance imaging (MRI) scans to determine whether there was leukoaraiosis (LA) and brain atrophy, which was performed on a 1.5-T scanner (GE Discovery750, Milwaukee, United States) using standard protocols. We measured serum Hcy level (μmol/L) in each patient using standard enzymatic methods (A15 Random Access Analyzer, Biosystems, Spain). The professional neurologist assessed the National Institute of Health Stroke Scale (NIHSS) score and MoCA score of each patient.
All patients provided fresh stool within 1 week of admission. Stool samples were collected using the MiSeq Reagent kit (PE300 v3) and immediately transferred to the laboratory for repackaging within 15 min. The 200 mg feces samples were placed into a 2 ml sterile centrifuge tube and divided into three parts and labeled, respectively. All specimens were processed within 30 min after collection, and the samples were stored at −80°C. Fecal genomic DNA was extracted from stool samples using a DNA extraction kit (TIANGEN, TIANamp, China), according to the manufacturer’s methods, as described in previous studies (Li et al., 2008; Shkoporov et al., 2018). The fecal samples were lysed in lysis buffer, and we put VAHTS DNA Clean Beads in it, then homogenizing for 3–5 min in a vortex mixer (Qilinbeier Vortex-5), purified with 200 μl 80% ethanol, and eluted with 24 μl of elution buffer. The quantity of extracted genomic DNA was evaluated by 2% agarose gel, and DNA purity and concentration were determined by NanoDrop spectrophotometer (Thermo Fisher Scientific, United States). A260/A280 ratios were also measured to confirm the high-purity of the DNA yield. Then we stored the extracted DNA at −20°C.
The DNA extraction was followed by the amplification of the V3–V4 16S ribosomal RNA gene region, with the forward primer (5′-CCTACGGGNGGCWGCAG-3′) and the reverse primer (5′-GACTACHVGGGTATCTAATCC-3′), as described in the previous study (Bu et al., 2018). The PCR process was as follows: denaturation at 95°C for 30 s, annealing at 55°C for 30 s, extension at 72°C for 45 s, 25 cycles, and a final extension at 72°C for 5 min. Reaction system: 2 × Phanta Max Master Mix 25 μl, DNA template 5 μl, Nextera XT Index Primer 1 2 μl, Nextera XT Index Primer 2 2 μl, ddH2O 16 μl. PCR products were validated in a 2% agarose gel for single bands and expected sizes.
The DNA libraries were pooled and sequenced on a MiSeq Benchtop Sequencer (Illumina, Singapore, United States). For quality control, the reads without primers were discarded using cutadapt, version 1.11, and the chimeric reads removed. The processed pair-end reads were merged using PandaSeq, version 2.9, with default parameters, to generate representative complete nucleotide sequences. The overlapping areas of the paired-end reads were processed first, and low-quality reads (average Q < 20) and those containing ambiguous bases denoted by Ns were deleted. Vsearch was used to cluster high-quality sequences with a similarity cut-off of 0.97. We selected the sequences with the highest abundance in each class as the representative. The representative sequences were annotated (down to the genus) using the RDP classifier, version 2.12 (Whelan and Surette, 2017), and sequences which could not be assigned to any specific classification level were labeled as “unclassified.” QIIME was used to remove the Operational Taxonomy Units (OTUs) with only one sequence in all samples.
Bacterial diversity was determined by α-diversity (Shannon’s index and Simpson index) and β-diversity (Principal coordinates analysis, PCoA). The α-diversity indices were analyzed using the R software. A Mann-Whitney U-test or Kruskal Wallis H was performed to compare the α-diversity of groups. The β-diversity comparison was performed by analysis of similarities using the Bray-Curtis dissimilarity index. Significant P-values associated with microbial clades and functions were identified by linear discriminant analysis Effect Size (Lefse) (Qian et al., 2018). The Lefse analysis used the Kruskal-Wallis test (alpha value of 0.05) and a linear discriminant analysis score > 2 as thresholds. We used Phylogenetic Investigation of Communities by Reconstruction of Unobserved States (PICRUSt) to predict gene contents and metagenomic functional information according to the OTU table (Langille et al., 2013). We used the receiver operating characteristic (ROC) curves and the area under the curve (AUC) to verify the specificity and sensitivity of the characteristic GM in diagnosing PSCI, and investigate whether the characteristic GM could be regarded a biomarker for PSCI.
Statistical analysis was carried out using GraphPad Prism V.5.0.1 (La Jolla, CA, United States), the R software (V.3.5), Adobe Illustrator CC 2015 (Adobe Systems Incorporated, California, America), and SPSS, V.22 (SPSS, Chicago, United States). Categorical variables were presented as numbers and percentages and compared by chi-squared test. Continuous variables were described as mean and standard deviation or median and interquartile range, depending on the outcome of a Kolmogorov-Smirnov normality test, and compared by Student’s t-test or Mann-Whitney test, respectively. Mann-Whitney test was used to determine the significance of the difference between PSCI and PSNCI groups (i.e., PSCI vs. PSNCI; age-matched PSCI vs. PSNCI). We used multivariable logistic regression to determine the risk factors for PSCI and the representative microbiota associated with PSCI after adjustments for age and the risk factors. The probability cut-offs to enter or remove a variable were 0.05 and 0.1, respectively. Spearman rank correlation was used to analyze GM’s correlation with MoCA scores and the risk factors for PSCI. We further selected 29 PSCI patients as a subgroup of younger PSCI with average age similar to the PSNCI group. Randomization was stratified by age.
At first, a total of 135 stroke patients were enrolled, 14 patients were excluded due to unwillingness to participate in all the study procedures, eight patients were excluded due to missing data, and 20 patients were excluded according to the exclusion criteria, leaving 93 patients that could be analyzed (Figure 1). The patients’ demographic information and MoCA scores in the two groups (53 and 40 patients in the PSCI and PSNCI group, respectively) are summarized in Table 1. There were significant differences in terms of age, NIHSS and MoCA scores, stroke recurrence (not the first stroke), Hcy, LA, and brain atrophy between the two groups (P = 0.006, 0.001, < 0.001, < 0.001, < 0.001, < 0.001, < 0.001, respectively). After age-matched, the NIHSS and MoCA scores, stroke recurrence, Hcy, LA, and brain atrophy still exhibited the significant differences between the two groups (Supplementary Table S1). However, no significant difference was found in sex, divorce rate, education level, physical activity, sleep deprivation, diabetes mellitus, hypertension, dyslipidemia, atrial fibrillation, BMI, current smoking and alcohol status, and dietary risks between the two groups.
Table 1. The significant differences of demographic and clinical parameters between PSCI and PSNCI groups.
The comparisons of the sub-items of the MoCA score between the two groups were shown in Table 1. PSCI patients had lower scores in all sub-items, including visuospatial/executive function, naming, attention, language, abstraction, delayed recall, and orientation (all P < 0.005).
Multivariable logistic regression was used to evaluate which variables could represent risk factors for PSCI. PSCI was independently associated with higher baseline NIHSS score [OR 1.553, 95% confidence interval (CI) 1.014–2.379, P = 0.043], higher Hcy level (OR 1.219, 95% CI 1.013–1.466, P = 0.036), higher prevalence of stroke recurrence (OR 4.042, 95% CI 1.293–12.634, P = 0.016), brain atrophy (OR 3.663, 95% CI 1.181–11.359, P = 0.025), and higher proportion of LA (OR 8.780, 95% CI 1.210–63.729, P = 0.032) after adjustment for age (Table 2).
Table 2. Multivariate logistic regression analysis of risk factor for PSCI after adjustment for age.
Analysis of the 16S ribosomal RNA sequencing gave a total of 197,660 OTUs, classified into 14 phyla, 28 classes, 50 orders, 97 families, and 243 genera. As shown in Supplementary Figure S1C, although no significant difference in gut bacterial communities between the PSCI and PSNCI groups was evident from the PCoA scatterplot, the relative abundances of some gut microbial taxa were significantly different between the two groups. At the phylum level, patients with PSCI had a significantly higher content of Proteobacteria (8.7 vs. 5.7%, P = 0.016, Figure 2A). At the class level, patients with PSCI had higher contents of Gammaproteobacteria (6.9 vs. 3.8%, P = 0.017, Figure 2B) and Bacilli (5.3 vs. 3.0%, P = 0.012, Figure 2B). At the order level, PSCI was associated with significantly higher abundances of Enterobacteriales (6.8 vs. 3.7%, P = 0.013, Figure 2C) and Lactobacillales (5.3 vs. 3.0%, P = 0.011, Figure 2C). At the family level, patients with PSCI had higher contents of Enterobacteriaceae (6.8 vs. 3.7%, P = 0.013, Figure 2D), Streptococcaceae (3.6 vs. 1.6%, P = 0.005, Figure 2D), and Lactobacillaceae (1.5 vs. 1.3%, P = 0.02, Figure 2D). At the genus level, PSCI patients had significantly higher levels of Streptococcus (3.6 vs. 1.6%, P = 0.005, Figure 2E), Klebsiella (2.3 vs. 0.6%, P = 0.002, Figure 2E), Lactobacillus (1.5 vs. 1.3%, P = 0.02, Figure 2E), Prevotella (14.0 vs. 10.2%, P = 0.01, Figure 2E), and Veillonella (1.05 vs. 0.23%, P = 0.022, Figure 2E); and lower contents of Roseburia (2.7 vs. 3.7%, P = 0.033, Figure 2E), f_Lachnospiraceae_other (1.9 vs. 2.8%, P = 0.008, Figure 2E) and Fusicatenibacter (0.17 vs. 0.40%, P = 0.0018, Figure 2E). However, no significant difference was found between the PSCI and PSNCI groups in fecal microbiota α-diversity (Supplementary Figures S1A,B). Furthermore, as shown in Figure 2H, the relative content of cystathionine-beta-lyase was significantly higher in the PSCI group compared with the PSNCI group (P = 0.011).
Figure 2. Comparison of the representative taxonomic abundance between post-stroke cognitive impairment (PSCI) and post-stroke non-cognitive impairment (PSNCI) groups. (A) Mann-Whitney U-test indicated the significant differences in phylum between the two groups and also in their corresponding class (B), order (C), family (D), and genus (E). (F) A cladogram of different taxonomic composition between PSCI patients and PSNCI patients. (G) linear discriminant analysis scores showed significant bacterial differences between PSCI patients and PSNCI patients. (H) Compare the functional Kyoto Encyclopedia of Genes and Genomes orthology of gut microbiota in PSCI and PSNCI groups. Mann-Whitney U-test indicated the significant differences between the two groups. o, order; f, family; g, genus. *P < 0.05, **P < 0.01.
After the groups were age-matched, although no significant difference in gut bacterial communities among the PSCI, PSNCI, and age-matched PSCI groups was evident from the PCoA scatterplot, the relative abundances of some gut microbial taxa were significantly different between age-matched PSCI and PSNCI groups (Supplementary Figure S3C). As shown in Supplementary Figures S4A–E, at the phylum level, age-matched PSCI patients had a significantly higher content of Proteobacteria (age-matched PSCI vs. PSNCI: 10.8 vs. 5.7%, P = 0.017), and lower abundance of Firmicutes (age-matched PSCI vs. PSNCI: 33.2 vs. 40.7%, P = 0.027). The similar alterations were also observed at the class, order, family, and genus levels of Proteobacteria and Firmicutes, including Gammaproteobacteria (age-matched PSCI vs. PSNCI: 9.1 vs. 3.8%, P = 0.040), Clostridia (age-matched PSCI vs. PSNCI: 21.8 vs. 29.8%, P = 0.056), Enterobacteriales (age-matched PSCI vs. PSNCI: 9.1 vs. 3.7%, P = 0.020), Clostridiales (age-matched PSCI vs. PSNCI: 21.8 vs. 29.8%, P = 0.056), Enterobacteriaceae (age-matched PSCI vs. PSNCI: 9.1 vs. 3.7%, P = 0.020), Klebsiella (age-matched PSCI vs. PSNCI: 3.2 vs. 0.7%, P = 0.031), and Lachnospiraceae_other (age-matched PSCI vs. PSNCI: 1.6 vs. 3.0%, P = 0.009). Besides, PSCI patients were also associated with a significantly higher abundance of Prevotella (age-matched PSCI vs. PSNCI: 19.6 vs. 10.2%, P = 0.021). However, no significant difference was found among the three groups in fecal microbiota α-diversity (Supplementary Figures S3A,B).
We further confirmed the characteristic GM using Lefse analysis. Of note, PSCI was associated with increased abundances of Enterobacteriaceae, Klebsiella of Enterobacteriales, and Lactobacillaceae, Streptococcaceae, Streptococcus, Lactobacillus of Lactobacillales and Prevotella, and decreased abundances of Fusicatenibacter and f_Lachnospiraceae_other (Figures 2F,G). After being adjusted for age, the age-matched PSCI and PSNCI groups showed significant differences in phylum Proteobacteria and Firmicutes. The abundances of Gammaproteobacteria, Enterobacteriales, Enterobacteriaceae, Klebsiella, and Prevotella were significantly higher, the proportions of Clostridia, Clostridiales, Lachnospiraceae, and Lachnospiraceae_other were lower in the age-matched PSCI group compared with PSNCI group (Supplementary Figures S4F,G).
The results showed that there was no significant difference in sex between the two groups (PSCI-male, n = 35, 66%; PSNCI-male, n = 27, 67.5%, P = 0.882). According to sex, stroke patients were divided into female and male subjects. The PCoA showed no significant difference in sex between the two groups (Supplementary Figure S5). Moreover, there was no significant difference in the relative abundance of the characteristic gut microbiome between the two groups (Supplementary Figure S6). Therefore, sex may have little effect on gut microbiota composition in this study.
We evaluated the functional differences in the microbiome of PSCI vs. PSNCI. As shown in Supplementary Table S2, the enriched orthologs in PSCI patients were folding, sorting and degradation (chaperones and folding catalysts), genetic information processing (protein folding and associated processing, transcription related proteins), energy metabolism (nitrogen metabolism, sulfur metabolism), metabolism (glycan biosynthesis and metabolism, nucleotide metabolism), enzyme families (protein kinases), carbohydrate metabolism (propanoate metabolism). In contrast, the increased pathways in PSNCI patients were metabolism of cofactors and vitamins (porphyrin and chlorophyll metabolism, pantothenate and CoA biosynthesis, nicotinate and nicotinamide metabolism, thiamine metabolism), amino acid metabolism (phenylalanine, tyrosine and tryptophan biosynthesis, arginine and proline metabolism, histidine metabolism, alanine, aspartate and glutamate metabolism, valine, leucine and isoleucine biosynthesis, valine, leucine, and isoleucine degradation), carbohydrate metabolism, lipid metabolism (primary bile acid biosynthesis, secondary bile acid biosynthesis, linoleic acid metabolism).
The Spearman rank correlation was used to confirm the correlation between MoCA scores and the GM at the genus level. As shown in Figure 3A, f_Lachnospiraceae_other (P < 0.001), Fusicatenibacter (P < 0.01), Parasutterella, Phascolarctobacterium, Clostridium_XVIII, and Butyricicoccus (P < 0.05) were positively associated with the MoCA score, while Klebsiella, Enterobacteriaceae_other (P < 0.01), Clostridium_sensu_stricto, Olsenella, Prevotella, Dialister, Enterococcus, and Alloprevotella (P < 0.05) showed negative correlation. Moreover, we further investigated the correlation between gut bacteria and the MoCA sub-items. As shown in Figure 3A, Fusicatenibacter was found to be positively associated with delayed recall, orientation, attention, abstraction (P < 0.05), and language (P < 0.01). f_Lachnospiraceae_other was positively correlated with naming, language, abstraction (P < 0.001), attention, visuospatial/executive function, delayed recall, and orientation (P < 0.01). In addition, Klebsiella was negatively associated with delayed recall (P < 0.01), attention, visuospatial/executive function, and naming (P < 0.05). Prevotella was negatively correlated with delayed recall, orientation (P < 0.05), and abstraction (P < 0.01). Escherichia/Shigella was negatively associated with naming (P < 0.05).
Figure 3. The associations of gut microbiota (GM) with MoCA scores and the risk factors for PSCI. (A) Heatmap of spearman rank correlation analysis between GM and MoCA scores and its sub-variables. (B) Heatmap of spearman rank correlation analysis between GM and the risk factors for PSCI. Red means positive correlation and blue means negative correlation. *P < 0.05, **P < 0.01, ***P < 0.001, +P < 0.1, ++P < 0.05, +++P < 0.01.
As shown in Figure 3B, the stroke recurrence was negatively associated with Roseburia (P < 0.1) and Dorea (P < 0.05). LA was positively associated with Klebsiella (P < 0.05), while negatively associated with Paraprevotella (P < 0.01). Brain atrophy was positively correlated with Alistipes, Streptococcus, Clostridium_sensu_stricto (P < 0.01), Lactobacillus, Klebsiella, Odoribacter, Acidaminococcus, and Haemophilus (P < 0.05), but negatively correlated with Phascolarctobacterium (P < 0.05). The NIHSS score was positively associated with Klebsiella (P < 0.01), Veillonella, Clostridium_sensu_stricto, Enterobacteriaceae_other, and Prevotella (P < 0.05), while negatively associated with Fusicatenibacter (P < 0.1). Moreover, the Hcy level was positively correlated with Alloprevotella and Streptococcus (P < 0.05) but negatively correlated with Fusicatenibacter (P < 0.05).
In the multivariable logistic regression models (Supplementary Table S3), there was no significant association between the representative microbiota and PSCI in conditions of unadjusted and adjusted for age in models 1 and 2, respectively. However, we observed a significant correlation between PSCI and the abundance of Enterobacteriaceae after adjustment for age and risk factors for PSCI, including NIHSS score, stroke recurrence, Hcy, LA, and brain atrophy (P = 0.035). Moreover, the higher abundance of Enterobacteriaceae represented a closer association with PSCI (P = 0.010, OR = 59.721).
As shown in Supplementary Figure S2, the model based on the Lefse results after being age-matched, which represented the characteristic GM of PSCI, could effectively distinguish PSCI from PSNCI (AUCPSCI–PSNCI = 0.840, 95% CI: 0.760–0.920, P < 0.001; AUC age–matched PSCI–PSNCI = 0.858, 95% CI: 0.773–0.944, P < 0.001). The model based on the relative abundance of Enterobacteriaceae also showed the differentiating effect for PSCI (AUCPSCI–PSNCI = 0.629, 95% CI: 0.510–0.747, P = 0.038; AUCage–matched PSCI–PSNCI = 0.658, 95% CI: 0.524–0.792, P = 0.029). These results indicated that GM might contain valuable PSCI biomarkers.
In this study, we characterized the GM composition of PSCI patients. Although GM’s bacterial diversity in PSCI patients was similar to that of PSNCI patients, the microbial composition was distinct between the two groups. The abundance of Proteobacteria was highly increased in the PSCI group compared with the PSNCI group. Similar alterations were also observed at the class, order, family, and genus levels of Proteobacteria. After age adjustments, the abundance of Firmicutes, and its members, including Clostridia, Clostridiales, Lachnospiraceae, and Lachnospiraceae_other, were significantly decreased in the age-matched PSCI group compared with the PSNCI group. Moreover, we found GM’s close associations with MoCA scores and risk factors for PSCI, including NIHSS score, Hcy, stroke recurrence, LA, and brain atrophy. The abundance of Enterobacteriaceae showed a significant correlation with PSCI after adjustments for age and risk factors. Besides, the ROC model, which was based on the characteristic GM, could effectively distinguish PSCI patients from PSNCI patients. In particular, Enterobacteriaceae also showed the differentiating ability for PSCI. These results indicated that the GM might provide novel microbiome-related biomarkers for PSCI.
In this study, there were significant differences in terms of NIHSS score, stroke recurrence, Hcy, LA, and brain atrophy between the PSCI and PSNCI groups, and we also observed the associations of these risk factors with GM. Previous studies have revealed that the incidence of post-event dementia was positively correlated with stroke severity (Pendlebury et al., 2019), and GM dysbiosis was positively correlated with NIHSS scores in stroke patients (Xia et al., 2019). Besides, stroke recurrence was a significant contributor to cognitive impairment through its association with white matter hyperintensities (WMH) (Georgakis et al., 2019). In this study, PSCI patients contained a higher abundance of cystathionine beta-lyase, which was involved in the anabolism process of Hcy (Reveal and Paietta, 2013). Many bacteria, yeast, and plants contain the enzyme. Therefore, the changes in serum homocysteine in the PSCI group may be caused by many factors. Hcy levels were positively associated with the risk of cognitive impairment via upregulated pro-inflammatory cytokines, causing endothelial damage and having direct neurotoxic properties (Fang et al., 2014; Di Meco et al., 2018). Previous studies also reported that Hcy levels were associated with increased risk of severe deep and periventricular white matter lesions, contributing to poor cognitive performance (Vermeer et al., 2002), and the strong associations between increased Hcy levels and cognitive decline in patients with AD and Parkinson’s disease had been confirmed (Di Meco et al., 2018; Murray and Jadavji, 2019). Some researchers hypothesized that the Hcy/lipopolysaccharide (LPS) might mediate pyroptosis in the obese adipocytes due to the GM imbalance (Laha et al., 2018), and the altered microbiome in OSAHS patients was associated with Hcy (Ko et al., 2019). Besides, LA also contributed to cognitive deterioration by triggering the release of inflammatory factors (Kaffashian et al., 2016; Hainsworth et al., 2017). An earlier study had shown that generalized brain and hippocampal atrophy contributed to cognitive decline and specifically to memory deficits, through substantial neuronal loss (Fein et al., 2000). Many studies had indicated the alterations of GM in diseases associated with brain tissue atrophy, including AD (Liu et al., 2019) and multiple system atrophy (Wan et al., 2019). Our results supported the evidence from epidemiological studies that identified multiple risk factors for PSCI, including NIHSS score, Hcy, stroke recurrence, LA, and brain atrophy.
Decreased bacterial diversity is observed in various diseases, such as metabolic syndrome (Dabke et al., 2019) and neurodegenerative diseases (Zhang et al., 2017; Zhuang et al., 2018). Gut bacterial diversity is affected by factors such as lifestyle, age, metabolic diseases, and antibiotics (Gulden, 2018; Tengeler et al., 2018). A growing body of evidence has demonstrated that psychotropic drugs could affect the GM profile. Atypical antipsychotics induced a decrease in GM’s diversity and a significant increase in Lachnospiraceae abundance and a decrease in Akkermansia level in patients with bipolar disease (Flowers et al., 2017). In this study, we had already excluded the patients who had psychosis, such as schizophrenia or bipolar disease. Moreover, we also did not use psychotropic drugs to treat stroke patients during hospitalization. Therefore, we could eliminate the effects of psychotropic drugs on GM. Diet is one of the critical factors in regulating the GM profile. Different diets have different effects on the composition of GM. For example, the administration of a high-fat diet resulted in a decrease in Bacteroidetes and a significant increase in the abundance of Proteobacteria and Firmicute (Hildebrandt et al., 2009). In this study, we classified the diet as high fat, low in fruits, and low in vegetables. The results showed that there were no significant differences in diet between the two groups. Thus, the dietary effects on GM could be avoided. In this study, no significant difference in bacterial diversity was found between the two groups. The similarity in lifestyle between the two groups and the fact that both groups were composed of stroke patients might explain this result.
According to a previous study, age is the confounding factor that may influence the GM composition. The age-related alterations in the GM composition include an increase of Proteobacteria, a decrease of the Firmicutes to Bacteroides, and a reduction of microbiota diversity (Vaiserman et al., 2017). The changes of GM may be associated with inflammation and endotoxin tolerance during the acute phase of stroke and myocardial infarction (Hernandez-Jimenez et al., 2017; Kowalska et al., 2018; Krishnan and Lawrence, 2019). In this study, the increased abundances of Klebsiella, Enterobacteriaceae, Enterobacteriales, Gammaproteobacteria of phylum Proteobacteria, and Prevotella were still found in age-matched PSCI patients compared with PSNCI patients. The previous study had indicated that the enrichment of Proteobacteria in the gut reflected dysbiosis of gut microbial community structure and risk of diseases (Shin et al., 2015). Moreover, the increased abundances of Proteobacteria, Gammaproteobacteria, Enterobacteriales, and Enterobacteriaceae could lead to the release of proinflammatory cytokine (Dinh et al., 2015; Shin et al., 2015; Sovran et al., 2018), and the proportions of these GM were negatively associated with cognitive function (Liu et al., 2019). The enriched network of taxa containing Gammaproteobacteria and Enterobacteriales was also observed in colorectal cancer (Peters et al., 2016) and AD patients (Liu et al., 2019), which was consistent with our study. A previous study on liver transplantation reported that the increased abundance of Klebsiella was associated with poor cognitive performance (Bajaj et al., 2017), which was in agreement with our results. Administration of Lactobacillus improved cognitive functions impaired by chronic restraint stress (Liang et al., 2015) and major depression (Rudzki et al., 2019). However, our results showed that patients with PSCI had more abundance of Lactobacillus. Thus, evidence from reports indicated that these gut bacteria might be closely related to PSCI.
We also found a significantly lower abundance of Firmicutes, and its members, including Clostridia, Clostridiales, Lachnospiraceae, and Lachnospiraceae_other, in age-matched PSCI patients compared with PSNCI patients. According to the previous study, the levels of Firmicutes and Clostridia were significantly reduced in humans with type 2 diabetes (Larsen et al., 2010). Besides, the decreased abundances of Firmicutes, Clostridia, Clostridiales, and Lachnospiraceae had been reported in AD patients (Liu et al., 2019). Lachnospiraceae was one of the most abundant known butyrate-producing bacteria in human GM (Hold et al., 2003; Zhang et al., 2019). SCFAs could improve learning and memory function (During et al., 2003), provide neuroprotection and neuroplasticity, and reduce β-amyloid plaques and microglia activation in animal models of AD (Dalile et al., 2019). Chen et al. demonstrated that transplanting fecal bacteria reduced infarct volume and cerebral edemas, and improved cognitive function in rat models of ischemic stroke (Chen et al., 2019). Our previous study also revealed that increasing the content of SCFAs could be a potential treatment for AD via fecal microbiota transplantation (Sun et al., 2019). However, whether inadequate SCFAs-producing bacteria were involved in PSCI still needs to be confirmed by future studies.
Besides, PSCI was associated with several modulations of the Kyoto Encyclopedia of Genes and Genomes (KEGG) pathways. The module for folding, sorting and degradation (chaperones and folding catalysts) progressively enriched in PSCI patients. According to early studies, molecular chaperones and protein-folding catalysts functioned as proinflammatory signals (Henderson and Pockley, 2010), and molecular chaperones were acting as receptors for the major pathogen-associated molecular patterns and LPS (Triantafilou and Triantafilou, 2005), which further induced inflammatory response. However, some evidence indicated that these proteins might have anti-inflammatory actions (Henderson and Pockley, 2010). Besides, the modules related to metabolisms of cofactors and vitamins, amino acid, and lipids were significantly lower in the PSCI patients, which were consistent with the findings in patients with AD (Li et al., 2019). According to the previous study, amino acid reduced inflammation, oxidative stress, and cell death in the gut (Liu et al., 2017). Moreover, the module for secondary bile acids of lipid metabolism could reduce macrophage inflammation and lipoprotein uptake to protect the blood vessels (Pols et al., 2011). These results suggested that multiple and complex communication pathways existed between GM and PSCI.
We also demonstrated the close correlation of GM with MoCA scores and risk factors for PSCI. Notably, we found that Klebsiella and Enterobacteriaceae_other of family Enterobacteriaceae were negatively correlated with the MoCA score, and positively associated with NIHSS score, LA, and brain atrophy. We further confirmed that the abundance of Enterobacteriaceae was closely associated with PSCI after adjusting age and risk factors. Furthermore, the ROC model, which was based on the characteristic GM, could effectively distinguish PSCI from PSNCI patients, and Enterobacteriaceae also exhibited the differentiating ability for PSCI. According to the previous studies, the abundances of Enterobacteriaceae and Escherichia/Shigella were increased in patients with AD, these gut bacteria were considered as pro-inflammatory bacteria and induced LPS accumulation, and mediated amyloid aggregation and inflammatory response (Li et al., 2019; Liu et al., 2019). Besides, the increased abundance of Enterobacteriaceae was associated with poor prognosis (Xu et al., 2019a). Thus, the increased abundance of Enterobacteriaceae might be significantly associated with PSCI. Due to the severity of PSCI, it is urgent to find biomarkers for PSCI diagnosis. Previous studies demonstrated that some microRNAs could achieve expected results in the diagnosis of PSCI (Huang et al., 2016; Wang et al., 2020). Besides, recent studies indicated that the imaging and multiple cellular changes had made significant progress in the diagnosis of neurological disease (Vijayan and Reddy, 2016a; Eyileten et al., 2018; Guo et al., 2018; Vijayan et al., 2018; Saba et al., 2019). However, few studies had tested their usefulness in the clinical trials, and the complexity of experimental operations with lower microRNAs detection sensitivity and specificity limited its clinical application. The alteration of GM composition involves many diseases, including neuropsychiatric diseases. However, the GM composition of PSCI is still largely unknown. This study showed that the characteristic GM could be used as a diagnostic biomarker for PSCI. further, combining other valuable biomarkers is also needed to improve the accuracy of PSCI diagnosis.
Several limitations of this study should be mentioned. Multiple variables influence GM composition, and it is difficult to achieve complete standardization for all patients. Meanwhile, patients enrolled in our study tended to have lower NIHSS scores, and we did not distinguish post-stroke dementia patients from PSCI non-dementia patients, which limited the representativeness of the study. The application on the outcome of the MoCA was somewhat overemphasized. In future studies, we will use more clinical scales such as Hastgawa Dementia Scale and Wechsler Memory Scale to verify our results. Moreover, we did not investigate the GM of these patients before cognitive decline and a healthy control group without stroke, as well as the long-term follow-up, which resulted in lacking the dynamic observation of the disease. Besides, our study was a single-center study in which the number of patients was still not enough. Thus, the conclusion that GM is closely associated with PSCI may not be made quickly. Age is a vital factor contributing to GM composition, and additional experiments with larger samples in age-matched groups for the PSCI and PSNCI are needed to verify the present results.
Despite these limitations, the study has some important strengths. First, this is one of the first studies characterizing the GM in patients with PSCI, filling the GM information gap in PSCI. Second, we also investigated the risk factors for PSCI and their associations with GM. The broader connections were established between GM and the risk factors, which contributed to a better understanding of GM’s role in PSCI. Third, this study gave new clues to explore the novel diagnostic biomarkers and interventions for PSCI.
In summary, our study assessed the GM composition of PSCI patients and further indicated that the characteristic GM, especially Enterobacteriaceae, might facilitate the diagnosis of PSCI.
The datasets generated for this study can be found in the NCBI Trace Archive NCBI Sequence Read Archive, SRA accession: PRJNA588869, Temporary Submission ID: SUB6532710, the SRA records will be accessible with the following link after the indicated release date: https://www.ncbi.nlm.nih.gov/sra/PRJNA588869, moreover, we have already uploaded the clinical data and 16s data to the additional files.
The studies involving human participants were reviewed and approved by The Ethics Committee of the Second Affiliated Hospital of Wenzhou Medical University. The patients/participants provided their written informed consent to participate in this study. Written informed consent was obtained from the individual(s) for the publication of any potentially identifiable images or data included in this article.
JS and JL conceived and designed the experiments. YL, TG, JZ, QG, XG, and XW performed the experiments and conducted the statistical analyses. All authors contributed to the article and approved the submitted version.
This work was supported by the National Natural Science Foundation of China (81871094).
The authors declare that the research was conducted in the absence of any commercial or financial relationships that could be construed as a potential conflict of interest.
The Supplementary Material for this article can be found online at: https://www.frontiersin.org/articles/10.3389/fnagi.2020.511562/full#supplementary-material
Arba, F., Quinn, T., Hankey, G. J., Ali, M., Lees, K. R., Inzitari, D., et al. (2017). Cerebral small vessel disease, medial temporal lobe atrophy and cognitive status in patients with ischaemic stroke and transient ischaemic attack. Eur. J. Neurol. 24, 276–282. doi: 10.1111/ene.13191
Bajaj, J. S., Fagan, A., Sikaroodi, M., White, M. B., Sterling, R. K., Gilles, H., et al. (2017). Liver transplant modulates gut microbial dysbiosis and cognitive function in cirrhosis. Liver Transpl. 23, 907–914. doi: 10.1002/lt.24754
Bajaj, J. S., Hylemon, P. B., Ridlon, J. M., Heuman, D. M., Daita, K., White, M. B., et al. (2012). Colonic mucosal microbiome differs from stool microbiome in cirrhosis and hepatic encephalopathy and is linked to cognition and inflammation. Am. J. Physiol. Gastrointest. Liver Physiol. 303, G675–G685. doi: 10.1152/ajpgi.00152.2012
Ballard, C., Rowan, E., Stephens, S., Kalaria, R., and Kenny, R. A. (2003). Prospective follow-up study between 3 and 15 months after stroke: improvements and decline in cognitive function among dementia-free stroke survivors > 75 years of age. Stroke 34, 2440–2444. doi: 10.1161/01.STR.0000089923.29724.CE
Bu, X. L., Xiang, Y., Jin, W. S., Wang, J., Shen, L. L., Huang, Z. L., et al. (2018). Blood-derived amyloid-β protein induces Alzheimer’s disease pathologies. Mol. Psychiatry 23, 1948–1956. doi: 10.1038/mp.2017.204
Carlson, A. L., Xia, K., Azcarate-Peril, M. A., Goldman, B. D., Ahn, M., Styner, M. A., et al. (2018). Infant gut microbiome associated with cognitive development. Biol. Psychiatry 83, 148–159. doi: 10.1016/j.biopsych.2017.06.021
Chen, R., Xu, Y., Wu, P., Zhou, H., Lasanajak, Y., Fang, Y., et al. (2019). Transplantation of fecal microbiota rich in short chain fatty acids and butyric acid treat cerebral ischemic stroke by regulating gut microbiota. Pharmacol. Res. 148:104403. doi: 10.1016/j.phrs.2019.104403
Collins, S. M., Kassam, Z., and Bercik, P. (2013). The adoptive transfer of behavioral phenotype via the intestinal microbiota: experimental evidence and clinical implications. Curr. Opin. Microbiol. 16, 240–245. doi: 10.1016/j.mib.2013.06.004
Crichton, S. L., Bray, B. D., McKevitt, C., Rudd, A. G., and Wolfe, C. D. (2016). Patient outcomes up to 15 years after stroke: survival, disability, quality of life, cognition and mental health. J. Neurol. Neurosurg. Psychiatry 87, 1091–1098. doi: 10.1136/jnnp-2016-313361
Dabke, K., Hendrick, G., and Devkota, S. (2019). The gut microbiome and metabolic syndrome. J. Clin. Invest. 129, 4050–4057. doi: 10.1172/JCI129194
Dalile, B., Van Oudenhove, L., Vervliet, B., and Verbeke, K. (2019). The role of short-chain fatty acids in microbiota-gut-brain communication. Nat. Rev. Gastroenterol. Hepatol. 16, 461–478. doi: 10.1038/s41575-019-0157-3
Di Meco, A., Li, J. G., Barrero, C., Merali, S., and Pratico, D. (2018). Elevated levels of brain homocysteine directly modulate the pathological phenotype of a mouse model of tauopathy. Mol. Psychiatry 24, 1696–1706. doi: 10.1038/s41380-018-0062-0
Diaz Heijtz, R., Wang, S., Anuar, F., Qian, Y., Bjorkholm, B., Samuelsson, A., et al. (2011). Normal gut microbiota modulates brain development and behavior. Proc. Natl. Acad. Sci. U.S.A. 108, 3047–3052. doi: 10.1073/pnas.1010529108
Dinan, T. G., Stanton, C., and Cryan, J. F. (2013). Psychobiotics: a novel class of psychotropic. Biol. Psychiatry 74, 720–726. doi: 10.1016/j.biopsych.2013.05.001
Dinh, D. M., Volpe, G. E., Duffalo, C., Bhalchandra, S., Tai, A. K., Kane, A. V., et al. (2015). Intestinal microbiota, microbial translocation, and systemic inflammation in chronic HIV infection. J. Infect. Dis. 211, 19–27. doi: 10.1093/infdis/jiu409
During, M. J., Cao, L., Zuzga, D. S., Francis, J. S., Fitzsimons, H. L., Jiao, X., et al. (2003). Glucagon-like peptide-1 receptor is involved in learning and neuroprotection. Nat. Med. 9, 1173–1179. doi: 10.1038/nm919
Erny, D., Hrabe de Angelis, A. L., Jaitin, D., Wieghofer, P., Staszewski, O., David, E., et al. (2015). Host microbiota constantly control maturation and function of microglia in the CNS. Nat. Neurosci. 18, 965–977. doi: 10.1038/nn.4030
Eyileten, C., Wicik, Z., De Rosa, S., Mirowska-Guzel, D., Soplinska, A., Indolfi, C., et al. (2018). MicroRNAs as diagnostic and prognostic biomarkers in ischemic stroke-a comprehensive review and bioinformatic analysis. Cells 7:249. doi: 10.3390/cells7120249
Fang, P., Zhang, D., Cheng, Z., Yan, C., Jiang, X., Kruger, W. D., et al. (2014). Hyperhomocysteinemia potentiates hyperglycemia-induced inflammatory monocyte differentiation and atherosclerosis. Diabetes 63, 4275–4290. doi: 10.2337/db14-0809
Fein, G., Di Sclafani, V., Tanabe, J., Cardenas, V., Weiner, M. W., Jagust, W. J., et al. (2000). Hippocampal and cortical atrophy predict dementia in subcortical ischemic vascular disease. Neurology 55, 1626–1635. doi: 10.1212/wnl.55.11.1626
Flowers, S. A., Evans, S. J., Ward, K. M., McInnis, M. G., and Ellingrod, V. L. (2017). Interaction between atypical antipsychotics and the gut microbiome in a bipolar disease cohort. Pharmacotherapy 37, 261–267. doi: 10.1002/phar.1890
Gao, H., Jiang, Q., Ji, H., Ning, J., Li, C., and Zheng, H. (2019). Type 1 diabetes induces cognitive dysfunction in rats associated with alterations of the gut microbiome and metabolomes in serum and hippocampus. Biochim. Biophys. Acta Mol. Basis Dis. 1865:165541. doi: 10.1016/j.bbadis.2019.165541
Georgakis, M. K., Duering, M., Wardlaw, J. M., and Dichgans, M. (2019). WMH and long-term outcomes in ischemic stroke: a systematic review and meta-analysis. Neurology 92, e1298–e1308. doi: 10.1212/WNL.0000000000007142
Gulden, E. (2018). Lifestyle factors affecting the gut microbiota’s relationship with type 1 diabetes. Curr. Diab. Rep. 18:111. doi: 10.1007/s11892-018-1098-x
Guo, X., Yang, J., Liang, B., Shen, T., Yan, Y., Huang, S., et al. (2018). Identification of novel LncRNA biomarkers and construction of LncRNA-related networks in han chinese patients with ischemic stroke. Cell Physiol. Biochem. 50, 2157–2175. doi: 10.1159/000495058
Hainsworth, A. H., Minett, T., Andoh, J., Forster, G., Bhide, I., Barrick, T. R., et al. (2017). Neuropathology of white matter lesions, blood-brain barrier dysfunction, and dementia. Stroke 48, 2799–2804. doi: 10.1161/STROKEAHA.117.018101
Haran, J. P., Bhattarai, S. K., Foley, S. E., Dutta, P., Ward, D. V., Bucci, V., et al. (2019). Alzheimer’s disease microbiome is associated with dysregulation of the anti-inflammatory p-glycoprotein pathway. mBio 10:e00632-19. doi: 10.1128/mBio.00632-19
Henderson, B., and Pockley, A. G. (2010). Molecular chaperones and protein-folding catalysts as intercellular signaling regulators in immunity and inflammation. J. Leukoc. Biol. 88, 445–462. doi: 10.1189/jlb.1209779
Hernandez-Jimenez, E., Gutierrez-Fernandez, M., Cubillos-Zapata, C., Otero-Ortega, L., Rodriguez-Frutos, B., Toledano, V., et al. (2017). Circulating monocytes exhibit an endotoxin tolerance status after acute ischemic stroke: mitochondrial dna as a putative explanation for poststroke infections. J. Immunol. 198, 2038–2046. doi: 10.4049/jimmunol.1601594
Hildebrandt, M. A., Hoffmann, C., Sherrill-Mix, S. A., Keilbaugh, S. A., Hamady, M., Chen, Y. Y., et al. (2009). High-fat diet determines the composition of the murine gut microbiome independently of obesity. Gastroenterology 137, 1716–1724. doi: 10.1053/j.gastro.2009.08.042
Hoban, A. E., Stilling, R. M., Ryan, F. J., Shanahan, F., Dinan, T. G., Claesson, M. J., et al. (2016). Regulation of prefrontal cortex myelination by the microbiota. Transl. Psychiatry 6:e774. doi: 10.1038/tp.2016.42
Hold, G. L., Schwiertz, A., Aminov, R. I., Blaut, M., and Flint, H. J. (2003). Oligonucleotide probes that detect quantitatively significant groups of butyrate-producing bacteria in human feces. Appl. Environ. Microbiol. 69, 4320–4324. doi: 10.1128/aem.69.7.4320-4324.2003
Huang, S., Zhao, J., Huang, D., Zhuo, L., Liao, S., and Jiang, Z. (2016). Serum miR-132 is a risk marker of post-stroke cognitive impairment. Neurosci. Lett. 615, 102–106. doi: 10.1016/j.neulet.2016.01.028
Kaffashian, S., Soumare, A., Zhu, Y. C., Mazoyer, B., Debette, S., and Tzourio, C. (2016). Long-term clinical impact of vascular brain lesions on magnetic resonance imaging in older adults in the population. Stroke 47, 2865–2869. doi: 10.1161/STROKEAHA.116.014695
Ko, C. Y., Liu, Q. Q., Su, H. Z., Zhang, H. P., Fan, J. M., Yang, J. H., et al. (2019). Gut microbiota in obstructive sleep apnea-hypopnea syndrome: disease-related dysbiosis and metabolic comorbidities. Clin. Sci. 133, 905–917. doi: 10.1042/CS20180891
Kowalska, K., Klimiec, E., Weglarczyk, K., Pera, J., Slowik, A., Siedlar, M., et al. (2018). Reduced ex vivo release of pro-inflammatory cytokines and elevated plasma interleukin-6 are inflammatory signatures of post-stroke delirium. J. Neuroinflammation 15:111. doi: 10.1186/s12974-018-1156-y
Krishnan, S., and Lawrence, C. B. (2019). Old dog new tricks; revisiting how stroke modulates the systemic immune landscape. Front. Neurol. 10:718. doi: 10.3389/fneur.2019.00718
Laha, A., Majumder, A., Singh, M., and Tyagi, S. C. (2018). Connecting homocysteine and obesity through pyroptosis, gut microbiome, epigenetics, peroxisome proliferator-activated receptor gamma, and zinc finger protein 407. Can. J. Physiol. Pharmacol. 96, 971–976. doi: 10.1139/cjpp-2018-0037
Langille, M. G., Zaneveld, J., Caporaso, J. G., McDonald, D., Knights, D., Reyes, J. A., et al. (2013). Predictive functional profiling of microbial communities using 16S rRNA marker gene sequences. Nat. Biotechnol. 31, 814–821. doi: 10.1038/nbt.2676
Larsen, N., Vogensen, F. K., van den Berg, F. W., Nielsen, D. S., Andreasen, A. S., Pedersen, B. K., et al. (2010). Gut microbiota in human adults with type 2 diabetes differs from non-diabetic adults. PLoS One 5:e9085. doi: 10.1371/journal.pone.0009085
Levine, D. A., Wadley, V. G., Langa, K. M., Unverzagt, F. W., Kabeto, M. U., Giordani, B., et al. (2018). Risk factors for poststroke cognitive decline: the REGARDS study (reasons for geographic and racial differences in stroke). Stroke 49, 987–994. doi: 10.1161/strokeaha.117.018529
Li, B., He, Y., Ma, J., Huang, P., Du, J., Cao, L., et al. (2019). Mild cognitive impairment has similar alterations as Alzheimer’s disease in gut microbiota. Alzheimers Dement. 15, 1357–1366. doi: 10.1016/j.jalz.2019.07.002
Li, M., Wang, B., Zhang, M., Rantalainen, M., Wang, S., Zhou, H., et al. (2008). Symbiotic gut microbes modulate human metabolic phenotypes. Proc. Natl. Acad. Sci. U.S.A. 105, 2117–2122. doi: 10.1073/pnas.0712038105
Liang, S., Wang, T., Hu, X., Luo, J., Li, W., Wu, X., et al. (2015). Administration of Lactobacillus helveticus NS8 improves behavioral, cognitive, and biochemical aberrations caused by chronic restraint stress. Neuroscience 310, 561–577. doi: 10.1016/j.neuroscience.2015.09.033
Liu, P., Wu, L., Peng, G., Han, Y., Tang, R., Ge, J., et al. (2019). Altered microbiomes distinguish Alzheimer’s disease from amnestic mild cognitive impairment and health in a Chinese cohort. Brain Behav. Immun. 80, 633–643. doi: 10.1016/j.bbi.2019.05.008
Liu, Y., Wang, X., and Hu, C. A. (2017). Therapeutic potential of amino acids in inflammatory bowel disease. Nutrients 9:920. doi: 10.3390/nu9090920
Murray, L. K., and Jadavji, N. M. (2019). The role of one-carbon metabolism and homocysteine in Parkinson’s disease onset, pathology and mechanisms. Nutr. Res. Rev. 32, 218–230. doi: 10.1017/S0954422419000106
Neufeld, K. M., Kang, N., Bienenstock, J., and Foster, J. A. (2011). Reduced anxiety-like behavior and central neurochemical change in germ-free mice. Neurogastroenterol. Motil. 23, 255–264. doi: 10.1111/j.1365-2982.2010.01620.x
Ogbonnaya, E. S., Clarke, G., Shanahan, F., Dinan, T. G., Cryan, J. F., and O’Leary, O. F. (2015). Adult hippocampal neurogenesis is regulated by the microbiome. Biol. Psychiatry 78, e7–e9. doi: 10.1016/j.biopsych.2014.12.023
Pendlebury, S. T., Rothwell, P. M., and Oxford Vascular, S. (2019). Incidence and prevalence of dementia associated with transient ischaemic attack and stroke: analysis of the population-based oxford vascular Study. Lancet Neurol. 18, 248–258. doi: 10.1016/S1474-4422(18)30442-3
Peters, B. A., Dominianni, C., Shapiro, J. A., Church, T. R., Wu, J., Miller, G., et al. (2016). The gut microbiota in conventional and serrated precursors of colorectal cancer. Microbiome 4:69. doi: 10.1186/s40168-016-0218-6
Pols, T. W., Nomura, M., Harach, T., Lo Sasso, G., Oosterveer, M. H., Thomas, C., et al. (2011). TGR5 activation inhibits atherosclerosis by reducing macrophage inflammation and lipid loading. Cell. Metab. 14, 747–757. doi: 10.1016/j.cmet.2011.11.006
Qian, Y., Yang, X., Xu, S., Wu, C., Song, Y., Qin, N., et al. (2018). Alteration of the fecal microbiota in Chinese patients with Parkinson’s disease. Brain Behav. Immun. 70, 194–202. doi: 10.1016/j.bbi.2018.02.016
Reveal, B. S., and Paietta, J. V. (2013). Sulfur-regulated control of the met-2(+) gene of Neurospora crassa encoding cystathionine beta-lyase. BMC Res. Notes 6:259. doi: 10.1186/1756-0500-6-259
Rudzki, L., Ostrowska, L., Pawlak, D., Malus, A., Pawlak, K., Waszkiewicz, N., et al. (2019). Probiotic Lactobacillus Plantarum 299v decreases kynurenine concentration and improves cognitive functions in patients with major depression: a double-blind, randomized, placebo controlled study. Psychoneuroendocrinology 100, 213–222. doi: 10.1016/j.psyneuen.2018.10.010
Saba, L., Saam, T., Jager, H. R., Yuan, C., Hatsukami, T. S., Saloner, D., et al. (2019). Imaging biomarkers of vulnerable carotid plaques for stroke risk prediction and their potential clinical implications. Lancet Neurol. 18, 559–572. doi: 10.1016/S1474-4422(19)30035-3
Shin, N. R., Whon, T. W., and Bae, J. W. (2015). Proteobacteria: microbial signature of dysbiosis in gut microbiota. Trends Biotechnol. 33, 496–503. doi: 10.1016/j.tibtech.2015.06.011
Shkoporov, A. N., Ryan, F. J., Draper, L. A., Forde, A., Stockdale, S. R., Daly, K. M., et al. (2018). Reproducible protocols for metagenomic analysis of human faecal phageomes. Microbiome 6:68. doi: 10.1186/s40168-018-0446-z
Singh, V., Roth, S., Llovera, G., Sadler, R., Garzetti, D., Stecher, B., et al. (2016). Microbiota dysbiosis controls the neuroinflammatory response after stroke. J. Neurosci. 36, 7428–7440. doi: 10.1523/JNEUROSCI.1114-16.2016
Sovran, B., Planchais, J., Jegou, S., Straube, M., Lamas, B., Natividad, J. M., et al. (2018). Enterobacteriaceae are essential for the modulation of colitis severity by fungi. Microbiome 6:152. doi: 10.1186/s40168-018-0538-9
Stilling, R. M., Ryan, F. J., Hoban, A. E., Shanahan, F., Clarke, G., Claesson, M. J., et al. (2015). Microbes & neurodevelopment–Absence of microbiota during early life increases activity-related transcriptional pathways in the amygdala. Brain Behav. Immun. 50, 209–220. doi: 10.1016/j.bbi.2015.07.009
Sun, J., Xu, J., Ling, Y., Wang, F., Gong, T., Yang, C., et al. (2019). Fecal microbiota transplantation alleviated Alzheimer’s disease-like pathogenesis in APP/PS1 transgenic mice. Transl. Psychiatry 9:189. doi: 10.1038/s41398-019-0525-3
Sun, J. H., Tan, L., and Yu, J. T. (2014). Post-stroke cognitive impairment: epidemiology, mechanisms and management. Ann. Transl. Med. 2:80. doi: 10.3978/j.issn.2305-5839.2014.08.05
Tang, W. K., Chan, S. S., Chiu, H. F., Ungvari, G. S., Wong, K. S., Kwok, T. C., et al. (2006). Frequency and clinical determinants of poststroke cognitive impairment in nondemented stroke patients. J. Geriatr. Psychiatry Neurol. 19, 65–71. doi: 10.1177/0891988706286230
Tengeler, A. C., Kozicz, T., and Kiliaan, A. J. (2018). Relationship between diet, the gut microbiota, and brain function. Nutr. Rev. 76, 603–617. doi: 10.1093/nutrit/nuy016
Triantafilou, M., and Triantafilou, K. (2005). The dynamics of LPS recognition: complex orchestration of multiple receptors. J. Endotoxin. Res. 11, 5–11. doi: 10.1179/096805105225006641
Tu, Q., Ding, B., Yang, X., Bai, S., Tu, J., Liu, X., et al. (2014). The current situation on vascular cognitive impairment after ischemic stroke in Changsha. Arch. Gerontol. Geriatr. 58, 236–247. doi: 10.1016/j.archger.2013.09.006
Vaiserman, A. M., Koliada, A. K., and Marotta, F. (2017). Gut microbiota: a player in aging and a target for anti-aging intervention. Ageing Res. Rev. 35, 36–45. doi: 10.1016/j.arr.2017.01.001
Vermeer, S. E., van Dijk, E. J., Koudstaal, P. J., Oudkerk, M., Hofman, A., Clarke, R., et al. (2002). Homocysteine, silent brain infarcts, and white matter lesions: the rotterdam scan Study. Ann. Neurol. 51, 285–289. doi: 10.1002/ana.10111
Vijayan, M., Kumar, S., Yin, X., Zafer, D., Chanana, V., Cengiz, P., et al. (2018). Identification of novel circulatory microRNA signatures linked to patients with ischemic stroke. Hum. Mol. Genet. 27, 2318–2329. doi: 10.1093/hmg/ddy136
Vijayan, M., and Reddy, P. H. (2016a). Peripheral biomarkers of stroke: focus on circulatory microRNAs. Biochim. Biophys. Acta 1862, 1984–1993. doi: 10.1016/j.bbadis.2016.08.003
Vijayan, M., and Reddy, P. H. (2016b). Stroke, vascular dementia, and Alzheimer’s disease: molecular links. J. Alzheimers Dis. 54, 427–443. doi: 10.3233/JAD-160527
Wan, L., Zhou, X., Wang, C., Chen, Z., Peng, H., Hou, X., et al. (2019). Alterations of the gut microbiota in multiple system atrophy patients. Front. Neurosci. 13:1102. doi: 10.3389/fnins.2019.01102
Wang, Z. Q., Li, K., Huang, J., Huo, T. T., and Lv, P. Y. (2020). MicroRNA Let-7i is a promising serum biomarker for post-stroke cognitive impairment and alleviated OGD-induced cell damage in vitro by regulating Bcl-2. Front. Neurosci. 14:215. doi: 10.3389/fnins.2020.00215
Whelan, F. J., and Surette, M. G. (2017). A comprehensive evaluation of the sl1p pipeline for 16S rRNA gene sequencing analysis. Microbiome 5:100. doi: 10.1186/s40168-017-0314-2
Xia, G. H., You, C., Gao, X. X., Zeng, X. L., Zhu, J. J., Xu, K. Y., et al. (2019). Stroke dysbiosis index (SDI) in gut microbiome are associated with brain injury and prognosis of stroke. Front. Neurol. 10:397. doi: 10.3389/fneur.2019.00397
Xu, R., Tan, C., Zhu, J., Zeng, X., Gao, X., Wu, Q., et al. (2019a). Dysbiosis of the intestinal microbiota in neurocritically ill patients and the risk for death. Crit. Care 23:195. doi: 10.1186/s13054-019-2488-4
Xu, R., Wu, B., Liang, J., He, F., Gu, W., Li, K., et al. (2019b). Altered gut microbiota and mucosal immunity in patients with schizophrenia. Brain Behav. Immun. 85, 120–127. doi: 10.1016/j.bbi.2019.06.039
Yin, J., Liao, S. X., He, Y., Wang, S., Xia, G. H., Liu, F. T., et al. (2015). Dysbiosis of gut microbiota with reduced trimethylamine-N-oxide level in patients with large-artery atherosclerotic stroke or transient ischemic attack. J. Am. Heart Assoc. 4:e002699. doi: 10.1161/JAHA.115.002699
Zekry, D., Duyckaerts, C., Belmin, J., Geoffre, C., Herrmann, F., Moulias, R., et al. (2003). The vascular lesions in vascular and mixed dementia: the weight of functional neuroanatomy. Neurobiol. Aging 24, 213–219. doi: 10.1016/s0197-4580(02)00066-0
Zhang, J., Song, L., Wang, Y., Liu, C., Zhang, L., Zhu, S., et al. (2019). Beneficial effect of butyrate-producing Lachnospiraceae on stress-induced visceral hypersensitivity in rats. J. Gastroenterol. Hepatol. 34, 1368–1376. doi: 10.1111/jgh.14536
Zhang, L., Wang, Y., Xiayu, X., Shi, C., Chen, W., Song, N., et al. (2017). Altered gut microbiota in a mouse model of Alzheimer’s disease. J. Alzheimers Dis. 60, 1241–1257. doi: 10.3233/JAD-170020
Zhu, F., Guo, R., Wang, W., Ju, Y., Wang, Q., Ma, Q., et al. (2019). Transplantation of microbiota from drug-free patients with schizophrenia causes schizophrenia-like abnormal behaviors and dysregulated kynurenine metabolism in mice. Mol. Psychiatry [Epub ahead of print]. doi: 10.1038/s41380-019-0475-4
Zhuang, Z. Q., Shen, L. L., Li, W. W., Fu, X., Zeng, F., Gui, L., et al. (2018). Gut microbiota is altered in patients with Alzheimer’s disease. J. Alzheimers Dis. 63, 1337–1346. doi: 10.3233/JAD-180176
Keywords: biomarkers, ischemic stroke, Enterobacteriaceae, cognitive impairment, gut microbiome
Citation: Ling Y, Gong T, Zhang J, Gu Q, Gao X, Weng X, Liu J and Sun J (2020) Gut Microbiome Signatures Are Biomarkers for Cognitive Impairment in Patients With Ischemic Stroke. Front. Aging Neurosci. 12:511562. doi: 10.3389/fnagi.2020.511562
Received: 12 November 2019; Accepted: 24 August 2020;
Published: 23 October 2020.
Edited by:
Panteleimon Giannakopoulos, Université de Genève, SwitzerlandReviewed by:
Murali Vijayan, Texas Tech University Health Sciences Center, United StatesCopyright © 2020 Ling, Gong, Zhang, Gu, Gao, Weng, Liu and Sun. This is an open-access article distributed under the terms of the Creative Commons Attribution License (CC BY). The use, distribution or reproduction in other forums is permitted, provided the original author(s) and the copyright owner(s) are credited and that the original publication in this journal is cited, in accordance with accepted academic practice. No use, distribution or reproduction is permitted which does not comply with these terms.
*Correspondence: Jiaming Liu, d3pqaWFtaW5nX2xpdUAxNjMuY29t; Jing Sun, c3Vuand6QDEyNi5jb20=
Disclaimer: All claims expressed in this article are solely those of the authors and do not necessarily represent those of their affiliated organizations, or those of the publisher, the editors and the reviewers. Any product that may be evaluated in this article or claim that may be made by its manufacturer is not guaranteed or endorsed by the publisher.
Research integrity at Frontiers
Learn more about the work of our research integrity team to safeguard the quality of each article we publish.