- 1Institute of Psychology, University of Graz, Graz, Austria
- 2BioTechMed, Graz, Austria
- 3Otto Loewi Research Center, Division of Physiology, Medical University of Graz, Graz, Austria
- 4Institute of Physiotherapy, University of Applied Sciences FH-JOANNEUM, Graz, Austria
- 5Faculty of Human Sciences, Medical School Hamburg (MSH), Hamburg, Germany
Transcranial alternating current stimulation (tACS) is the application of subthreshold, sinusoidal current to modulate ongoing brain rhythms related to sensory, motor and cognitive processes. Electrophysiological studies suggested that the effect of tACS applied at an alpha frequency (8–12 Hz) was state-dependent. The effects of tACS, that is, an increase in parieto-occipital electroencephalography (EEG) alpha power and magnetoencephalography (MEG) phase coherence, was only observed when the eyes were open (low alpha power) and not when the eyes were closed (high alpha power). This state-dependency of the effects of alpha tACS might extend to the aging brain characterized by general slowing and decrease in spectral power of the alpha rhythm. We additionally hypothesized that tACS will influence the motor cortex, which is involved in motor skill learning and consolidation. A group of young and old healthy adults performed a serial reaction time task (SRTT) with their right hand before and after the tACS stimulation. Each participant underwent three sessions of stimulation: sham, stimulation applied at the individual participant’s alpha peak frequency or individual alpha peak frequency (iAPF; α-tACS) and stimulation with iAPF plus 2 Hz (α2-tACS) to the left motor cortex for 10 min (1.5 mA). We measured the effect of stimulation on general motor skill (GMS) and sequence-specific skill (SS) consolidation. We found that α-tACS and α2-tACS improved GMS and SS consolidation in the old group. In contrast, α-tACS minimally improved GMS consolidation but impaired SS consolidation in the young group. On the other hand, α2-tACS was detrimental to the consolidation of both skills in the young group. Our results suggest that individuals with aberrant alpha rhythm such as the elderly could benefit more from tACS stimulation, whereas for young healthy individuals with intact alpha rhythm the stimulation could be detrimental.
Introduction
Aging is defined as a persistent decline in the age-specific fitness components of an organism due to internal physiological deterioration (Galloway, 1993). In humans, one of the hallmarks of aging-associated deterioration is a deficit in motor performance which includes coordination, balance, and gait difficulties, as well as slowing and increased variability of movement (Seidler et al., 2010). The age-related motor deficits also extend to the learning of new motor skills and the modification of previously learned skills (King et al., 2013). The acquisition and consolidation of implicit motor sequence skills are reported to be preserved and impaired, respectively, while the reverse is observed for visuomotor adaptation skills (King et al., 2013). These motor behavioral changes in the elderly were suggested to be causally linked to the age-related structural deterioration in brain areas responsible for movement planning and execution. For instance, decreased gray matter volume in the cerebellum, caudate nucleus, prefrontal, parietal and sensorimotor cortices, as well as white matter deterioration in the corpus callosum, corticospinal tract and cerebellum are consistent findings in older adults when compared to young adults (Good et al., 2001; Salat et al., 2004; Raz et al., 2005; Ota et al., 2006; Sullivan et al., 2010). Furthermore, a significant decline in dopamine, acetylcholine, serotonin and norepinephrine-mediated neurotransmission in older adults have also been directly linked to a deficit in motor performance including skill learning (Seidler et al., 2010).
More recently, research has focused on the age-related changes in the oscillatory activity in the alpha frequency band and its significance for the neural control of movement during physiological aging. Several studies involving elderly participants identified voluntary movement planning and execution related electroencephalography (EEG) changes within the alpha frequencies in the parieto-occipital area (“classical” or posterior alpha rhythm) and the sensorimotor cortex (motor-cortical alpha, “mu,” or Rolandic rhythm). For instance, the absence of motor-cortical alpha and limited low beta lateralization (motor-related amplitude asymmetries or MRAA) in internal motor preparation was concomitant with slowed reaction time and suggested less efficient cerebral processes subserving free movement selection in older adults, which may indicate a reduced capacity for internally driven action with age (Deiber et al., 2014). Similarly, aging influenced the patterns of event-related desynchronization/synchronization (ERD/ERS). There were reports of larger alpha and beta ERD amplitude, increased spatial diffusion of motor-cortical alpha ERD over the parietal and frontal regions, and lengthening of motor-cortical alpha ERD duration during finger movements in the elderly subjects compared with young subjects (Derambure et al., 1993; Labyt et al., 2003; Deiber et al., 2014; Mary et al., 2015; Quandt et al., 2016). Cued finger movements, pinches, and the whole hand grip task also elicited a widespread spatial distribution and more uniform flat curve of alpha power decrease in the elderly compared to young participants in sensorimotor areas, which were linked to age-related changes in the neural coding of skilled motor behavior (Quandt et al., 2016). The post-movement rebound of magnetoencephalography (MEG) motor-cortical alpha and beta activity in the sensorimotor cortex, which was suggested to reflect plasticity changes, is also impaired in the aging brain (Mary et al., 2015). Furthermore, there is less increase in motor-cortical alpha power than in older adults during the inhibition of learned movements, which is believed to be due to a deficit in local inhibitory mechanisms within the sensorimotor cortices (Bönstrup et al., 2015). On the other hand, motor cortical potentials that preceded freely-executed voluntary finger or hand movements (lateralized readiness potential or LRP) were reported to increase in aged individuals. Specifically, an increase in the amplitude of response-locked LRPs was indicative of a slowed motor response (Feve et al., 1991; Yordanova et al., 2004; Roggeveen et al., 2007; Cespón et al., 2013).
Aging also affects alpha oscillatory activity in other brain regions. With regard to the posterior alpha rhythm (8–13 Hz), there was a marked reduction in amplitude, slowing of spontaneous oscillation, and declined reactivity (eye-opening) which correlated with global cognitive performance in the elderly (Babiloni et al., 2006; Ishii et al., 2017; Knyazeva et al., 2018). Older adults also showed reduced connectivity in the upper alpha band compared to young adults (Kikuchi et al., 2000; Vysata et al., 2014; Scally et al., 2018). Other changes due to aging include a significant alpha increase in frontal regions, mainly over the prefrontal cortex (PFC), which has also been observed in early Alzheimer’s disease patients (Kolev et al., 2002; Ishii et al., 2017). The shift of alpha from posterior to frontal regions has been associated with a compensatory mechanism. Older adults need to activate the PFC even to accomplish very easy tasks, whereas younger adults do not have to draw on this cortical resource (Mattay et al., 2002; Berchicci et al., 2012). The evidence so far, thus suggests a causal link between impaired alpha activity and cognitive and motor impairment in healthy aging, as well as a link to pathological conditions such as Alzheimer’s disease (Ishii et al., 2017; Koelewijn et al., 2017). It is therefore timely to develop interventions that can modulate the alpha rhythm in the elderly.
Transcranial alternating current stimulation (tACS) can directly modulate specific cortical oscillations and with it possibly cognitive and motor functions (Antal and Paulus, 2013). The exact neurophysiological mechanism behind the effects of tACS remains unclear. In animal models, it was shown that neuronal entrainment, that is, synchronization of endogenous (cortical) oscillations with the extrinsically applied rhythmic current, was possible (Fröhlich and McCormick, 2010; Ali et al., 2013; Schmidt et al., 2014; Aspart et al., 2018; Toloza et al., 2018). In healthy human subjects (simultaneous tACS-EEG recording) and epilepsy patients (simultaneous direct cortical stimulation and electrocorticography (ECoG) recording), alpha tACS led to an increase in alpha power (Helfrich et al., 2014; Alagapan et al., 2016). It has also been shown that the alpha power increase lasted minutes until hours after the stimulation, which was associated with entrainment echoes or spike-timing-dependent plasticity (Zaehle et al., 2010; Neuling et al., 2013; Strüber et al., 2015; Vossen et al., 2015; Alagapan et al., 2016; Kasten et al., 2016). Behaviorally, tACS at individual alpha peak frequency (iAPF) was shown to increase target detection and mental rotation performance of young healthy participants, as well as improved inhibitory abilities in elderly participants during a working memory task (Helfrich et al., 2014; Kasten and Herrmann, 2017; Borghini et al., 2018). Interestingly, the observed increase in alpha power at the parieto-occipital cortex was found to be state-dependent. An increase in EEG/ECoG alpha power and MEG phase coherence was only observed when the participant’s alpha power was low (eye-open condition) and not when the participant’s alpha power was high (eyes-closed condition; Neuling et al., 2013; Alagapan et al., 2016; Ruhnau et al., 2016). Considering that alpha activity is usually reduced in the elderly (Ishii et al., 2017), we may speculate that alpha tACS will be more beneficial for this age group.
In this study, we investigated the state-dependent effect of alpha tACS on motor consolidation. A group of healthy young and old participants performed a serial reaction time task (SRTT) with their right hand before and after tACS stimulation. During tACS, the current was delivered to the left motor cortex at the iAPF in one session (α-tACS) and above the iAPF (iAPF + 2 Hz) in another session (α2-tACS). So far, this is the first study trying to find behavioral evidence of the state-dependent effect of tACS applied in the alpha band. Pioneering studies in healthy young participants suggest that the effect of tACS is also modulated by concurrent motor-cortical alpha activity. For instance, 10 Hz tACS increased the size of motor evoked potential (MEP) only during motor imagery and had no effect without it (Feurra et al., 2013). This can be explained by the state-dependency of tACS as motor-cortical alpha and beta bands recorded over the somatosensory and motor cortex desynchronize (power decrease) during this task (Nam et al., 2011; Kim and Lee, 2015; Galdo-Alvarez et al., 2016). It follows, that the effect of tACS on SRTT performance should be increased due to the reduced alpha activity during the task (Alagapan et al., 2016). Therefore, we hypothesized that α-tACS and α2-tACS stimulation of the motor cortex will improve the consolidation of motor skills in old participants more than in young participants.
Materials and Methods
Participants
Twenty healthy young adults between 19 and 30 years old (10 males; mean age 23.8 ± 3.90 years) and fifteen healthy older adults between 55 and 67 years old (six males; mean age 61.66 ± 3.71 years) participated in the study. The young group was composed of university students and the old group was composed of retirees with 14.06 ± 2.93 mean years of education. All participants had a normal or corrected-to-normal vision and were right-handed according to the Edinburgh Handedness Inventory (Oldfield, 1971). Exclusion criteria included any history of chronic medical or neuropsychiatric disorders (e.g., depression, epilepsy, and stroke), learning disability, brain injuries, intake of maintenance medications, and contraindications to tACS such as metallic or electrical implants in the body or the head (Poreisz et al., 2007). Individuals who were familiar with activities involving repeated sequential finger movements such as professional musicians or video game players, as well as those who had previous experience with the SRTT were also excluded. The study was approved by the Ethics Committee of the Medical University of Graz and all performed experimental procedures conformed to the principles of the Helsinki Declaration regarding human experimentation. All participants provided written informed consent prior to the experiment and received monetary compensation (60 Euros) for their participation in the study.
Experimental Design and Procedures
The study was conducted in a single-blinded, randomized and sham-controlled design. Participants completed three randomized experimental sessions, two sessions with real tACS stimulation (applied at iAPF or “α-tACS” and iAPF + 2 Hz or “α2-tACS”) and one session with sham stimulation. To avoid carry-over effects, an interval of at least 1 week separated the experimental sessions. All experiments were carried out in the middle of the day (12:00–15:00) to ensure the highest alpha activity (Higuchi et al., 2001). The experiments were performed inside a dimly lit and sound-attenuated room. During the experiment, participants sat in a comfortable reclining chair with head and arm supports in front of a 19-inch computer monitor used to present the stimuli. Initially, the tACS electrodes were fixed underneath the EEG cap using rubber strips. In each session, a 5-min spontaneous resting-state EEG (eyes-open with central fixation) was recorded from three posterior electrodes to identify the participant’s iAPF before the stimulation. Participants were asked to relax and keep their eyes open during the measurements. The EEG data were immediately analyzed offline using a customized Matlab-based algorithm (Matlab R2016a, The MathWorks Inc., Natick, MA, USA) and the participant’s iAPF was identified from the Pz electrode. Participants were then asked to perform the SRTT as a baseline measure. After setting the individual stimulator frequency, the stimulation was started. To assess the impact of the stimulation on the early stages of motor skill consolidation, the participants performed the SRTT immediately after (0 min), 60 min and 120 min after stimulation. The EEG cap and tACS electrodes were removed during the first break. On average, each experimental session including the preparations and breaks lasted for 3 h.
EEG Recording
The EEG recording was conducted using BrainAmp standard amplifiers with the recording software BrainVision Recorder (Brain Products GmbH, Gilching, Germany). Continuous EEG signals were collected from three Ag-AgCl electrodes (Cz, Pz, and Oz) embedded in an elastic cap housing 32 channels labeled in accord with an extended international 10-20 system (Easycap, Falk Minow, Munich, Germany). The ground electrode was located on the forehead (FPz electrode). For recording, the reference electrode was placed at the left mastoid. A second reference channel was placed at the right mastoid to enable an offline re-referencing to the linked mastoids. For monitoring eye movements, three EOG channels were used, two were placed on the outer canthi of the eyes and one was placed superior to the nasion. EEG and EOG signals were digitized at a sampling rate of 500 Hz and band-pass filtered between 0.1 Hz to 100 Hz. An additional 50 Hz notch filter was applied. Electrode impedances were maintained below 5 kΩ for the EEG recording and below 10 kΩ for the EOG recording.
TACS Stimulation
tACS was delivered through a pair of saline-soaked (0.9%-NaCl) surface sponge electrodes connected to a battery-driven stimulator (ELDITH DC-stimulator, NeuroConn, Germany). The center of the first electrode (35 cm2, 0.04 mA/cm2 current density) was located underneath the C3 electrode of the EEG cap (10–20 EEG system) which corresponds to the location of the left primary motor cortex (left M1). This electrode was tangentially placed at a 45° angle relative to the central sulcus. A large second electrode (100 cm2, 0.01 mA/cm2 current density) was placed on the right contralateral supraorbital area (RO; Nitsche et al., 2007). The difference in the electrode sizes increased the focality of the stimulation since the current density was higher over the motor cortex than at the RO (Nitsche et al., 2007; Faria et al., 2011). Current flow modeling suggests that an adequate current can reach the motor cortex with a left M1-RO electrode montage without the influence of head fat distribution that may be different between young and old people (Miranda et al., 2006; Truong et al., 2013). The stimulation intensity was 1.5 mA (peak-to-peak current, no DC offset, no phase shift) and was applied for 10 min during the real tACS stimulation sessions. In one of the sessions, the current was delivered at the participant’s iAPF (α-tACS) determined from the Pz electrode. The stimulation parameters in this session were identical to the parameters we used in a recent study where we showed an increase in corticospinal excitability in both young and old individuals after α-tACS stimulation (Fresnoza et al., 2018). In another session, the current was delivered at the iAPF + 2 Hz (α2-tACS) in order to explore the frequency-specific effect of tACS on motor skill consolidation. To minimize the tingling skin sensation, the impedance during stimulation was maintained below 10 kΩ. In the sham stimulation session, the current was applied at α-tACS for only 30 s (with 10 s current ramping) and then switched off automatically without the participant’s awareness. This ensured that participants felt the same skin sensation in the sham condition as in the real stimulation conditions. In the present study, we used the iAPF determined from the posterior brain region as the stimulation frequency because of the significant role of the parietal cortex during motor preparation. The parietal cortex contributes to the temporal integration of sensory information into the movement sequence in order to ensure that each movement occurs after the successful completion of the preceding one (Catalan et al., 1998; Gongora et al., 2016). The sequence representation in the parietal cortex is then sent to the motor cortex for movement initiation (Yokoi et al., 2018). The posterior parietal cortex is also related to spatial goal-directed action planning and decisions of hand choice (Lindner et al., 2010; Oliveira et al., 2010; Petzschner and Krüger, 2012).
Serial Reaction Time Task (SRTT)
In the present study, we administered a modified version of the SRTT used in previous tDCS and tACS studies (Nitsche et al., 2003; Antal et al., 2008; Kuo et al., 2008; Pollok et al., 2015). Stimulus presentation and response recording were accomplished by using a program written in Psychopy (Psychology Software in Python, University of Nottingham; Peirce, 2009). A computer with a separate response box was used to record reaction times (RT) and error rate (ER). During the experiment, participants were seated in front of a computer screen. Their right-hand fingers rested on four horizontal buttons of a response box (index finger for button 1, middle finger for button 2, ring finger for button 3, and little finger for button 4; Figure 1). Each trial began with the presentation of four horizontally arranged square boxes of equal sizes in the center of the computer screen. The visual cue indicating the button (a cross that appeared in any of the four boxes) was presented in black on a white background. The participants were instructed to press a button of the response box that corresponded to the location of the cued box, as fast and as accurately as possible. Regardless of a correct or incorrect response, once a button was pressed, the cross disappeared and the trial ended. 500 ms later the cue appeared in a different box (Figure 1). One SRTT run was composed of seven blocks with 120 trials (button presses) each and took approximately 12–15 min to complete. Participants could take a break between blocks. In blocks 1 and 6, the appearance of the cross followed a pseudorandom or unpredictable order in which the cross was presented equally often in each position and never in the same position in two subsequent trials (random blocks). Blocks 2–5 and 7, were composed of 10 repetitions of the same 12-item button press sequence (1–2–1–4–2–3–4–1–3–2–4–3, sequential blocks). The participants were not informed about the existence of the repeating sequences. All participants performed four SRTT runs, one before stimulation (baseline) and three after stimulation (immediately after, 60 min and 120 min after stimulation). To test whether explicit knowledge of the sequences was acquired, the participants were asked after each run whether they had noticed a repeating sequence and if they could remember it. The RT for each trial is defined as the time difference (in milliseconds) between the onset of the cross (go signal) and the pressing of the correct button. On the other hand, the ER represents the ratio between the number of errors (wrong button presses and missing responses) within a block and the total number of trials on that block. The RTs and ERs before and after the stimulation were analyzed.
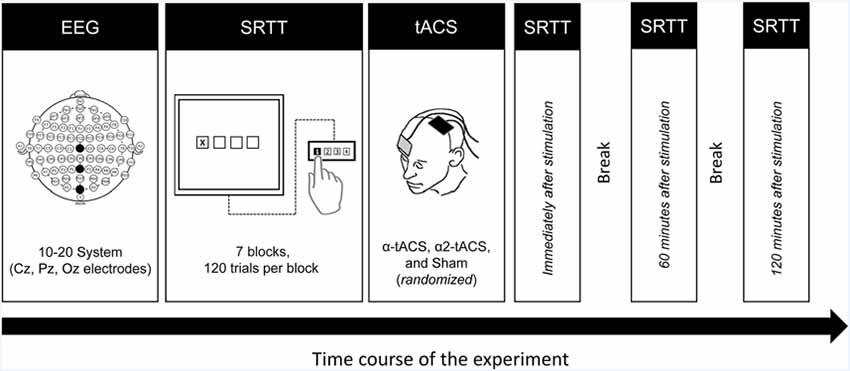
Figure 1. Experimental design. First, the participants underwent a 5-min resting-state EEG measurement to identify their individual alpha peak frequency (iAPF). Then they performed the SRTT task using their right hand. Immediately after the task, they received either α-tACS, α2-tACS or sham stimulation of the left motor cortex. They were retested immediately after, 60 min and 120 min after the stimulation. EEG, electroencephalography; SRTT, serial reaction time task; tACS, transcranial alternating current stimulation; α-tACS, tACS applied at iAPF; α2-tACS, tACS applied at iAPF + 2 Hz.
Statistical Analysis
EEG Data
The EEG data were preprocessed and analyzed offline using Brain Vision Analyzer software (version 2.01, Brain Products GmbH, Munich, Germany). First, data were offline re-referenced to a linked-mastoid reference. Then artifacts like eye blinks and other non-cerebral signals were removed using a thresholding method (WITHRESH function). Subsequently, the continuous data were epoched into 2,000 ms segments. Then, a Fast Fourier transformation (FFT) algorithm with a maximum resolution of 0.25 Hz (50% Hanning window) was used to calculate the power spectral density with a confidence interval boundary of 90% (Welch’s method). The iAPF was defined as the frequency where the maximum power was observed within the alpha range of 8–13 Hz (Klimesch, 1999).
SRTT Data
The statistical analysis of the behavioral data (RT and ER) was performed using SPSS 22.0 software (IBM SPSS Statistics, IBM Corp., Armonk, NY, USA). From our raw RT data, we computed two scores to capture motor learning consolidation, the general motor skill (GMS) and sequence skill (SS) score (Walker et al., 2003). ER was calculated for each block of all the SRTT runs. The GMS score represents the speeding up of RTs across sequential blocks due to practice (Savic and Meier, 2016). Specifically, the GMS score is the mean RT difference between two sequential blocks and served as a measure of GMS learning and consolidation. A positive GMS score means that a participant performed faster on the second sequential block, which represents learning and consolidation. Conversely, a negative GMS score means that a participant performed slower on the second block. The SS score, on the other hand, indicates the increase in RT when switching from sequential blocks to random blocks which immediately followed (Nissen and Bullemer, 1987; Salthouse et al., 1999; Brown et al., 2009; Urry et al., 2018). Task routine is assumed to be equal in both blocks so that the RT difference represents implicit motor sequence learning (Pascual-Leone et al., 1994). In our task, the SS score represents the RT difference between a random block and the preceding sequential block and served as a measure of SS learning and consolidation. A positive SS score means that the RTs are longer in the random block and represents learning and consolidation. Conversely, a negative SS score means that the RTs in the random block were shorter than those in a sequential block.
Skill Acquisition Stage
To evaluate motor skills acquisition before the stimulation in both groups, a GMS score was calculated from block 2 (sequential) and block 7 (sequential) while an SS score was calculated from block 6 (random) and block 5 (sequential) of the first SRTT run. Statistical comparison of the GMS and SS scores was performed using an independent sample t-test (paired, two-tailed).
Skill Consolidation Stage
In order to measure the effect of tACS on the “off-line” consolidation of GMS, we calculated the in-between SRTT run GMS scores. Here, the GMS score represents the RT difference between block 7 of the first SRTT run and block 2 of the subsequent SRTT run. We used block 2 rather than block 1 of the next SRTT run because block 1 was random and the RT difference between block 7 and block 1 might underestimate the extent of GMS consolidation (Meier and Cock, 2014). Three GMS scores were determined: the RT difference before stimulation (block 7) and immediately after stimulation (block 2); immediately after (block 7) and 60 min after stimulation (block 2); 60 min after (block 7) and 120 min after stimulation (block 2). On the other hand, the effect of stimulation on the SS consolidation was assessed based on the SS scores calculated within the SRTT runs (RTs in block 6 minus the RTs in block 5). In total, we have four SS scores, one before and three after stimulation (immediately after, 60 min, and 120 min after stimulation).
The GMS scores, SS scores, and ERs were separately analyzed using linear mixed-effects modeling (LMM) with random-intercept. Linear models are robust alternatives to pure ANOVAs when dealing with unbalanced datasets like in our study (20 young and 15 old participants; Searle, 1988; Warton et al., 2016). The GMS and SS scores from incorrect trials and trials with RTs ±2 standard deviations (outliers) were excluded from the analysis. In the models, the GMS score, SS score, and ER served as the dependent variables and participants were included as a random factor. The between-subjects factor group (young vs. old) and the within-subjects factors stimulation (sham, α-tACS, and α2-tACS) and time (GMS score: 0 min, 60 min, and 120 min; SS score: baseline, 0 min, 60 min, and 120 min) were treated as fixed-effect covariates. For the GMS score, the score before the stimulation, calculated from two sequential blocks within the first SRTT run, was not included (GMS baseline score). Therefore, only the GMS scores (three) calculated between SRTT runs and SS scores (four) calculated within the SRTT runs using the raw RTs were entered into the models. In the separate model for the ER, blocks (1–7) were added as a within-subjects factor. Normal data distribution and homogeneity of variance test were conducted using Shapiro–Wilk and Levene’s test, respectively.
We performed a model selection procedure to determine the most parsimonious model for our data using a (forward) stepwise approach (Barr et al., 2013). We started with baseline models that only contained the random factor subject (to examine the individual variation in the dependent variable regardless of the other predictors) and then incrementally added the predictors (Singer and Willett, 2003). The within-subjects factors were then added to the model followed by the between-subjects factor group, as well as their respective interactions. By adding a factor to the model one-at-a-time, we were able to compare the Akaike Information Criterion (AIC) values that indicate model adequacy (Akaike, 1973). This method can determine overfitting in the model because it penalizes the likelihood function for having too many parameters. Model fit improvement or worsening was indicated by a 2-point decrease or increase in AIC value due to the addition of a factor, respectively (Burnham and Anderson, 2002). Maximum likelihood (ML) estimation (Compound Symmetry models) were used to estimate the parameters of the models. However, an AIC value only compares one model to the next and does not indicate the absolute fit of the model to the data, therefore we also calculated the Akaike weight of each model (Burnham and Anderson, 2002). The Akaike weights compare all possible models and determine which model will come out best most of the time. Factors with non-significant main effects were excluded in the final models except when they were involved in significant higher interactions. We calculated Cohen’s d as a measure of effect size (<0.2—trivial, ≥ 0.2—small, ≥ 0.5—medium and ≥ 0.8—large). Significant findings from the models were explored using post hoc comparisons (paired t-test, two-tailed, Bonferroni adjusted for multiple comparisons). Lastly, we tested collinearity in the final models by determining the tolerance and variance inflation factors. A p-value of < 0.05 was considered significant for all statistical analyses. All values are expressed as the mean ± standard error of the mean (SEM).
Results
Baseline EEG
The analysis of the EEG data from the Pz electrode revealed that there was no significant difference between groups and stimulation conditions in the mean iAPF and mean posterior alpha power prior to the stimulation (all ps > 0.05; Table 1).

Table 1. Baseline measurements: individual alpha peak frequency (iAPF), alpha power, GMS and SS scores.
Skill Acquisition Stage RT
In the first SRTT run, the mean RT for the sequential blocks gradually decreased for all participants. The RTs increased again in the second random block (block 6). The comparison of the calculated GMS scores (block 2–block 7) and SS scores (block 6–block 5) of the first SRTT run revealed no significant group or stimulation condition differences (all p = > 0.05; Table 1). Furthermore, no participant reported having been aware of the repeating sequence after the baseline SRTT measurement.
TACS Stimulation and Skill Consolidation Stage RT
Overall, the participants tolerated the experimental procedure well. Except for four young participants who reported phosphene sensations (flickering light in the right visual field) only at the beginning of the α2-tACS stimulation, there were no reports of headaches, dizziness or nausea during and after stimulation. In all stimulation conditions, the averaged stimulation frequency did not differ significantly between the groups (Table 1). At the end of each experimental session, all participants noted the presence of repeating sequences but nobody could verbally recall the exact pattern.
In the final models, we excluded the GMS scores from 1,911 incorrect trials (5.06% of the total data) and 1,337 outlier RTs (3.54% of the total data). Similarly, we excluded the SS scores from 3,619 incorrect trials (7.18% of the total data) and 1,042 outlier RTs (2.07% of the total data). Therefore, the final model for the GMS score contained 91.41% of the total data set while the model for the SS score contained 90.75% of the total data set. In the final models for the GMS and SS scores, we included all the factors because a full model did come out best most of the time based on the Akaike weight (Supplementary Table S1). For the model of the GMS scores, even though the stimulation and group interaction effect was not significant (F(2,20912.21) = 2.20, p = 0.111, d = 0.205), we included it in the final model because the individual main effects, as well as their higher interactions, were significant (Table 2). Similarly, the factor group (F(1,35.00) = 0.89, p = 0.353, d = 0.158) was included in the final model for the SS scores because all of its interaction effects were significant. Moreover, the addition of these factors did not worsen the model fit based on the AIC values (Supplementary Table S1). All modeled data were normally distributed after logarithmic transformation (Shapiro–Wilk test) and the variances were equal for each group (Levene’s test; all p > 0.05). Tolerance range and variance inflation factors were equal to 1.000 in the final models indicating that multicollinearity had no effect on the findings. In addition, we are certain that results of the final models having a lower number of participants in the old group do not undermine the final results and interpretation because similar results were obtained when their data was modeled together with the young group that contained the same number of participants.
GMS Consolidation
In our data, the RT difference between block 7 of the SRTT run before the stimulation and block 2 of the SRTT run immediately after stimulation served as the baseline GMS score. The results of the analysis revealed that the old group’s GMS scores were significantly higher than the young group’s after stimulation in all conditions except 60 min after α2-tACS stimulation (significant main effect of group: F(1,35.01) = 8.85, p = 0.005, d = 0.500; significant time, stimulation and group interactions: F(4,20910.83) = 5.99, p = <0.001, d = 0.478). In both groups, the GMS scores increased after stimulation (significant main effect of time: F(2,20908.28) = 4.80, p = 0.008, d = 0.151). However, the changes in the GMS scores showed a group and a stimulation-specific effect (significant main effect of stimulation: F(2,20912.12) = 6.52, p = 0.001, d = 0.226; significant time and stimulation interactions: F(4,20910.83) = 7.09, p = <0.001, d = 0.455; significant time and group interactions: F(2,20908.28) = 12.47, p = <0.001, d = 0.586; Figure 2). In the sham stimulation condition, the GMS scores increased and were significantly higher than the baseline 120 min after stimulation in both groups (young group: p = 0.036, old group: p = 0.005). The old group’s GMS scores were significantly higher compared to baseline 60 min (p = <0.001) and 120 min (p = <0.001) after α-tACS stimulation, as well as 60 min (p = 0.002) and 120 min (p = 0.042) after α2-tACS stimulation. In contrast, in the young group, the GMS score was only significantly higher than baseline 60 min (p = 0.028) after α2-tACS stimulation. When the real stimulation conditions were compared to sham, the results showed a significantly higher GMS score 120 min after α-tACS stimulation in both groups (young group—p = 0.030; old group—p = 0.003). On another hand, the GMS score was significantly higher than sham 120 min (p = <0.001) after α2-tACS stimulation in the old group, while the GMS score was significantly lower than sham (p = 0.031) 60 min after α2-tACS stimulation in the young group (Bonferroni corrected post hoc t-tests). In summary, α-tACS and α2-tACS improved GMS consolidation in the old group particularly 120 min after stimulation. In contrast, α-tACS had a minimal impact on GMS consolidation in the young group, while α2-tACS showed some detrimental effect on GMS consolidation 60 min after stimulation.
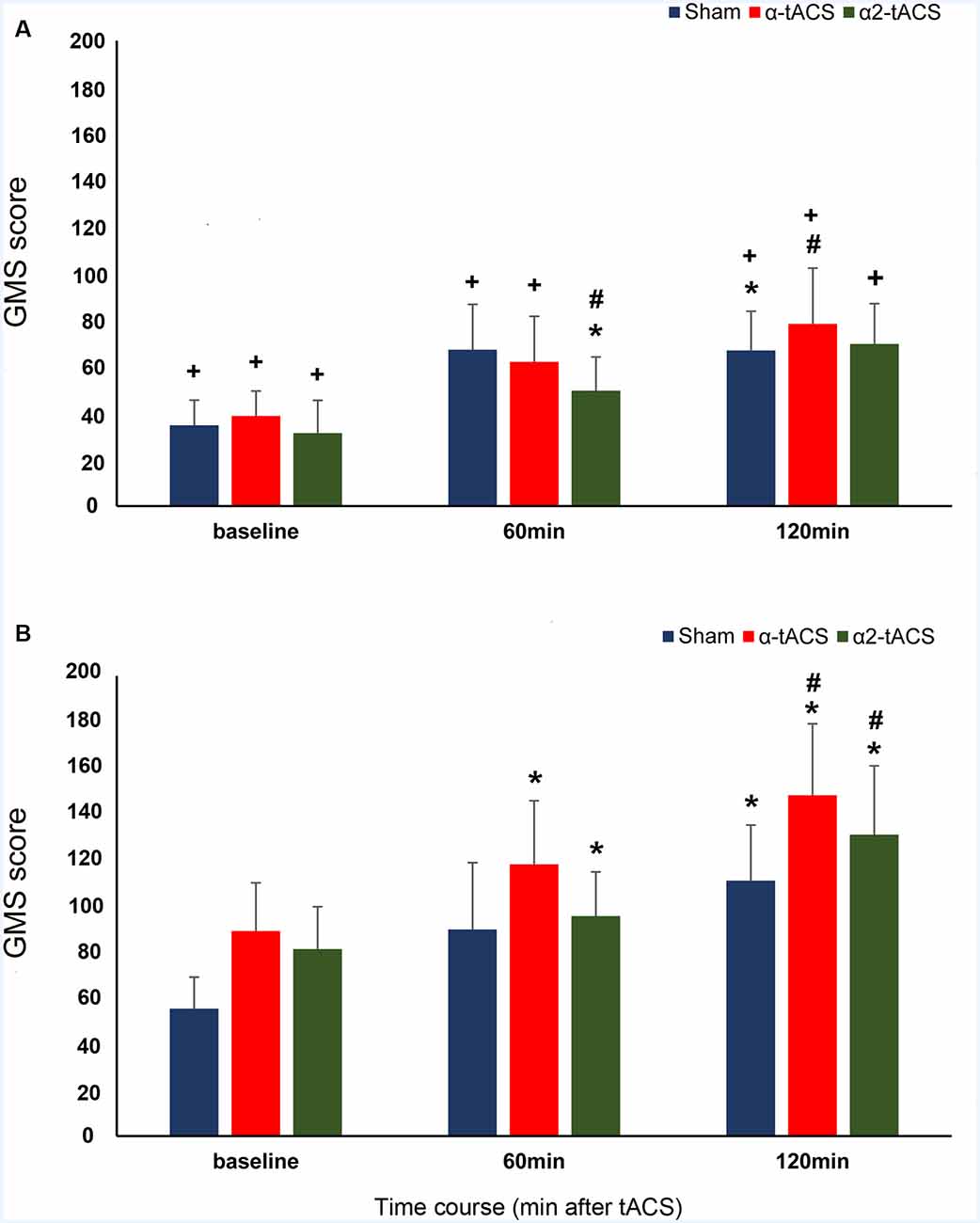
Figure 2. The effect of tACS stimulation on GMS consolidation. The y-axis displays the GMS scores in milliseconds (mean ± SEM). The GMS score is the difference in the reaction times (RT) from blocks with sequential trials (block 7 of the first SRTT run and block 2 of the following SRTT run). The x-axis displays the time points from which the GMS score was calculated. Baseline (RT difference between block 7 of the SRTT run before the stimulation and block 2 of the SRTT run immediately after stimulation), 60 min (RT difference between block 7 of the SRTT run immediately after stimulation and block 2 of the SRTT run 60 min after stimulation) and 120 min (RT difference between block 7 of the SRTT run 60 min after stimulation and block 2 of the SRTT run 120 min after stimulation). (A) Young group: α-tACS and α2-tACS stimulation had minimal impact on the GMS scores. (B) Old group: GMS scores significantly increased after α-tACS and α2-tACS stimulation and were significantly higher than sham 120 min after stimulation. The old group’s GMS scores were significantly higher than the young group’s for all time points except 60 min after α2-tACS stimulation. GMS, General motor skill; α-tACS, transcranial alternating current stimulation applied at iAPF; α2-tACS, transcranial alternating current stimulation applied at iAPF + 2 Hz, * = significant increase in GMS score compared to baseline, # = significant increase in GMS score compared to sham, + = significant difference between the young and the old group’s GMS score (Bonferroni corrected post hoc t-tests, paired, two-tailed, p < 0.05). Error bars denote SEM.
SS Consolidation
The analysis showed that SS scores changed significantly after the stimulation (significant main effect of time: F(3,33831.90) = 29.95, p = <0.001, d = 0.685). The stimulation-induced changes in SS scores were found to be group-specific because the magnitude was higher in the old group compared to the young group (significant time and group interactions: F(3,33831.90) = 3.42, p = 0.017, d = 0.177; Table 1 and Figure 3). A stimulation-specific effect can be observed on the SS scores as well (significant main effect of stimulation: F(2,33833.27) = 31.16, p = <0.001, d = 0.485; significant time and stimulation interactions: F(6,33831.97) = 3.98, p = 0.001, d = 0.204). In the young group, the SS scores were significantly lower immediately after (p = 0.006) and 120 min (p = <0.001) after α-tACS stimulation compared to sham at the same time points. In contrast, the SS scores were significantly higher than sham stimulation 60 (p = 0.001) and 120 min (p = <0.001) after α-tACS stimulation in the old group. Similarly, the SS scores were significantly lower than sham 120 min (p = 0.010) after α2-tACS stimulation in the young group, while the old group’s SS scores were significantly higher 60 min (p = <0.001) and 120 min (p = <0.001) after α2-tACS stimulation compared to sham (Bonferroni corrected post hoc t-tests). Moreover, although the old group exhibited overall higher SS scores than the young group after α-tACS and α2-tACS stimulation (significant stimulation and group interactions: F(2,33833.27) = 54.82, p = <0.001, d = 0.302), the SS scores significantly differed between the groups only immediately after α-tACS stimulation (significant time, stimulation and group interactions: F(6,33831.97) = 5.24, p = <0.001, d = 0.263). Here, the old group’s SS score was significantly higher (p = 0.046) than the young group’s SS score (Bonferroni corrected post hoc t-tests). In summary, the results showed better SS consolidation in the old group after α-tACS and α2-tACS stimulation compared to sham. On the other hand, both stimulation conditions had a detrimental effect on SS consolidation in the young group.
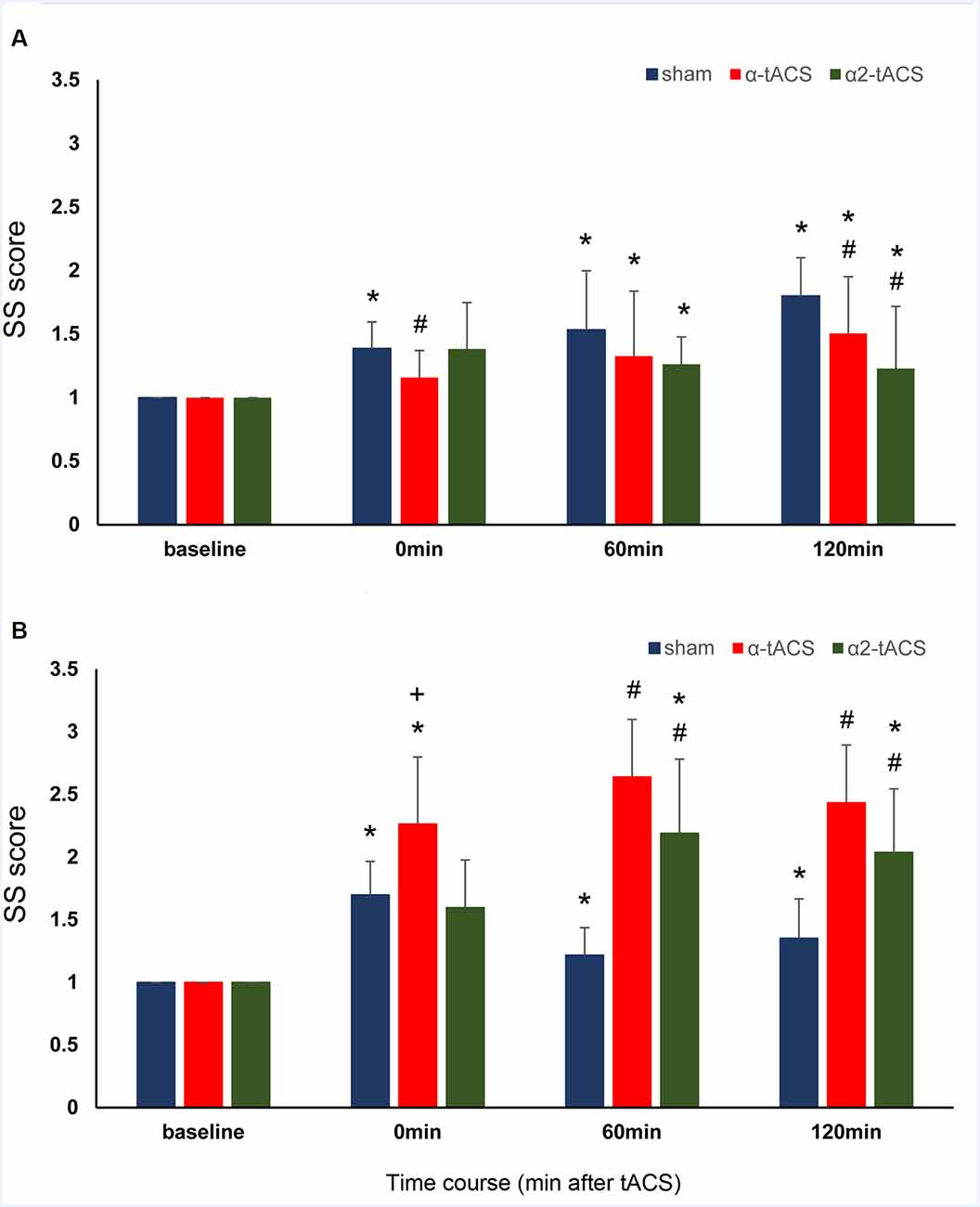
Figure 3. The effect of tACS stimulation on SS consolidation. The y-axis displays the SS scores (mean ± SEM) normalized with reference to the respective SS score before the stimulation (SS score after stimulation/SS score before stimulation). The SS score is the difference in the RT from the block with random trials (block 6) and a block with sequential trials (block 5) of the same SRTT run. The x-axis displays the time points before (baseline) and after stimulation (0 min or immediately after stimulation, 60 min and 120 min after stimulation). (A) Young group: SS scores were significantly lower than sham after α-tACS and α2-tACS stimulation. (B) Old group: SS scores significantly increased after α-tACS and α2-tACS stimulation and were significantly higher than sham 60 min and 120 min after stimulation. The old group’s SS scores were significantly higher than the young group’s immediately after α-tACS stimulation. SS, Sequence-specific skill; α-tACS, transcranial alternating current stimulation applied at iAPF; α2-tACS, transcranial alternating current stimulation applied at iAPF + 2 Hz, * = significant increase in SS score compared to baseline, # = significant increase in SS score compared to sham, + = significant difference between the young and the old group’s SS scores (Bonferroni corrected post hoc t-tests, paired, two-tailed, p < 0.05).
ER
The overall ERs in the study were low. Calculated overall ER was 3.43% and 3.01% in the young and old group, respectively. Relative to the total number of trials in each stimulation condition, the young group only made errors in 3.34% of the trials in the sham condition, 3.11% of the trials in the α-tACS condition and 3.26% of the trials in the α2-tACS condition. In the old group, there were only errors in 2.81% of the trials in the sham condition, 2.92% of the trials in α-tACS condition, and 2.62% of the trials in the α2-tACS condition. The results of the initial full model indicated that ERs did not significantly differ across stimulation conditions and between the groups (Supplementary Table S2). However, the model showed a significant main effect of time and blocks, as well as the interaction effects of group and blocks. Therefore, we ran and interpret a reduced LMM that contained the factor group, block and time as well as their interactions. The final/reduced model showed similar results (Supplementary Table S2). The main effect of time was significant (F(3,2869.06) = 17.74, p = <0.001, d = 0.353) indicating a significant increase in ER after stimulation. The main effect of block was also significant (F(3,2869.06) = 17.74, p = <0.001, d = 0.705) indicating the significant difference in ER between sequential and random blocks. Furthermore, the post hoc comparisons for the significant group and block interactions (F(6,2869.02) = 7.47, p = <0.001, d = 0.565) showed higher ERs in the young group (4.5%) compared with the old group (3.1%) only in block 1 (p = 0.026). Further comparisons revealed that these differences were also present during the consolidation stage (60 min: p = 0.048, 120 min: p = 0.029; Bonferroni corrected post hoc t-tests).
Discussion
In the present study, we demonstrated the impact of tACS stimulation on motor skill consolidation in young and old participants. The EEG data analysis revealed comparable posterior alpha oscillatory activity in both groups. Although age-related changes in the posterior alpha rhythm such as slowing and reduction in peak power are typically reported in older adults, our participants in the old group may not yet have undergone these age-related changes. This is possible because the age of these participants was not advanced (mean age 61.66 ± 3.71 years), and they were physically active and very healthy. However, tACS stimulation applied at comparable alpha frequencies affected the two types of motor skill consolidation differently in both groups. In particular, α-tACS and α2-tACS improved GMS and SS consolidation in the old group. In contrast, α-tACS minimally improved GMS consolidation but impaired SS consolidation in the young group. Furthermore, α2-tACS was detrimental to the consolidation of both skills in the young group.
The Acquisition of GMS and SS Skills Prior to the Stimulation
During the first SRTT run, the GMS score, SS score, and ER did not significantly differ between the groups indicating comparable skill learning. The participants also remained unaware of the test sequence, which is an indication that motor skill learning was largely implicit. The comparable group performance is not surprising because skill learning is generally preserved with age particularly when learning remains implicit and task complexity is low as in the SRTT task used here (Curran, 1997; Bennett et al., 2007; Brown et al., 2009; King et al., 2013; Meissner et al., 2016; Urry et al., 2018).
Effect of tACS on GMS Consolidation
In SRTT, GMS consolidation specifically refers to performance improvement across blocks with the same movement sequence. Improved performance is indicated by the gradual increase in GMS scores which is due to the reduction of RTs because of the participant’s growing expertise on the movement component of the sequence. GMS consolidation is assumed to be accompanied by several processes such as stabilization and enhancement of motor memories, as well as resistance to interference (Meier and Cock, 2014). Sequential trials require a stable motor memory of sequential (repeating) finger movements. In our results, we observed an increase in GMS scores after each break indicating the improvement in GMS consolidation in both groups even in the absence of stimulation (sham condition). However, statistical comparisons of the group’s scores revealed that consolidation was significantly higher in magnitude in the old group compared to the young group. This could indicate that motor memories are either robust, less susceptible to interference or more efficiently retrieved in our old participants (Brashers-Krug et al., 1996). Nevertheless, the improved consolidation we observed in the old group was unexpected because studies generally report either reduced (relative to young subjects) or complete absence of performance improvement during retesting of motor sequence tasks in older adults (Brown et al., 2009; King et al., 2013; Cornelis et al., 2016; Meissner et al., 2016). Late improvement (after 12 or 24-h off-line period) of GMS was also reported in elderly individuals (Brown et al., 2009; Nemeth and Janacsek, 2011). In our study, one possible reason that could explain the better performance of the old group compared to the young group during the consolidation stage is the ceiling effect that could have prevented the young group in further improving their skill performance (Berghuis et al., 2015; Centeno et al., 2018). This is plausible because the young group’s scores tended to plateau whereas the old group’s score trend-wise increased after sham stimulation (Figure 2). A ceiling effect in performance is less observed in the elderly as they tend to show either smaller improvements during motor practice or require extended periods of training to achieve skill levels comparable to those obtained by younger adults (Roig et al., 2014). Therefore, the absence of a ceiling effect may give the old participants more room for improvement during re-testing.
In the real stimulation conditions, we observed a group and stimulation-specific effect on GMS consolidation scores. In both groups, the stimulation applied at iAPF (α-tACS) improved the consolidation as indicated by the approximately linear increase in GMS scores that became significantly higher than the effect of sham 120 min after stimulation. The facilitating effect of α-tACS on the young group’s motor skill performance fits well with the findings of previous studies that stimulated the left motor cortex with 10 Hz tACS. Young subjects showed improved sequence acquisition and early motor skill consolidation during stimulation (Antal et al., 2008; Pollok et al., 2015). On the other hand, the increase in consolidation we observed in our old group after α-tACS is a novel finding. In theory, α-tACS stimulation could potentially facilitate the consolidation process in both groups because the α-tACS stimulation frequency closely matched the spectral peak of ongoing motor-cortical alpha (10 Hz) activity (Ali et al., 2013). Another possibility to account for the smaller GMS scores in our young group compared to the old group is movement slowing in the young group. Movement slowing after 10 Hz tACS stimulation of the motor cortex was observed in a fast finger tapping task in young adults (Wach et al., 2013). Indeed, a small GMS score is indicative of a slower response in block 2.
The group-specific effect on GMS consolidation was also evident after α2-tACS stimulation. The young group’s GMS scores were significantly lower compared to sham while the old group’s GMS scores were significantly higher compared to sham 60 and 120 min after stimulation, respectively. These results suggest that stimulation above the endogenous oscillatory frequency was detrimental to young participants but beneficial for older participants. Although post-stimulation EEG measurements were not conducted, which can be considered a potential limitation of the present study, the detrimental effect of α2-tACS stimulation in the young group might be explained by the results of entrainment studies. Cortical oscillations were shown to be strongly suppressed for stimulation frequencies between the dominant endogenous frequency and its first harmonic frequency (Ali et al., 2013). Also, the strongest entrainment is expected in areas that show a preference for the entraining frequency (Ruhnau et al., 2016). Neuronal entrainment is less likely in the α2-tACS condition because motor-cortical alpha rhythm in the motor cortex oscillates at 10 Hz and our stimulation frequency is more or less 12 Hz (iAPF + 2 Hz). Even if α2-tACS stimulation could entrain the natural frequency in the motor cortex to a value higher than its natural value, it could still be detrimental because the increased oscillatory activity might lead to a higher than necessary and therefore disruptive motoneuronal activity in the young group (Feurra et al., 2011a; Wach et al., 2013). On the other hand, although the magnitude was less compared to the effect of α-tACS, GMS consolidation in the old group also improved after α2-tACS stimulation. This might be explained by the finding that a mismatched stimulation frequency can succeed in increasing the endogenous oscillation (Schmidt et al., 2014). However, why did mismatched stimulation work for the old group but not for the young group? This might be due to differences between young and older adults in brain oscillations during motor processing. In a study by Quandt et al. (2016), young participants exhibited a clear and peaked modulation of movement-related power decrease in the alpha and upper beta band during a finger sequence task, whereas there was a more uniform flat curve of alpha power decrease in older adults.
Effect of tACS on SS Consolidation
In contrast to GMS consolidation, SS consolidation refers to performance improvement across sequenced and random trials. Specifically, the SS score refers to the increase in response times (RTs) when a random block directly followed a sequential block. Here, the resistance to the interfering memories of sequential finger movements might be the predominant reason for consolidation because the participants must inhibit the continued performance of the learned sequence. Indeed, compared to intelligence-matched controls, a core deficit in response inhibition is associated with slower RT’s especially in random trials in an SRTT task among the carrier of fragile X mental retardation 1 (FMR1) gene (Kraan et al., 2014). The lower accuracy in SRTT (including random trials) of individuals with attention deficit hyperactivity disorder (ADHD) is also ascribed to their impaired response inhibition (Pedersen and Ohrmann, 2018).
Similar to GMS consolidation, group and stimulation-specific effects on SS consolidation were evident in the two real tACS stimulation conditions. For instance, the young group’s SS scores after α-tACS were lower than those in the sham stimulation condition. In contrast, the old group’s SS scores were significantly higher after α-tACS than after sham stimulation for all time points. The group differences reached significance immediately after α-tACS stimulation. These results suggest that α-tACS stimulation is beneficial for the old group but detrimental for the young group. We would argue that the different effect of α-tACS on SS consolidation in both groups was due to the impact of stimulation on motor cortical inhibitory networks. In elderly adults, the inhibitory control of learned and automated motor response is suggested to be impaired due to age-dependent deterioration of motor cortical inhibitory networks. This deterioration is suggested to be reflected in EEG oscillatory changes over the sensorimotor regions particularly the motor cortical alpha power which is thought to be an index of inhibitory control (Hummel et al., 2002, 2004; Klimesch et al., 2007; Jensen and Mazaheri, 2010; Sauseng et al., 2013; Bönstrup et al., 2015). For instance, there is an impaired rebound of alpha power increase in the sensorimotor cortex after motor sequence learning and a reduced ability to inhibit inappropriate responses in older adults (Vallesi et al., 2010; King et al., 2013; Bönstrup et al., 2015; Mary et al., 2015). Interestingly, focal increases of oscillatory alpha activity were also absent over the primary motor cortex in dystonic patients who were known to have a deficit of inhibitory motor control (Hummel et al., 2002). Despite the absence of post-stimulation EEG measurement in our study, we may speculate that motor-cortical alpha power and inhibitory control is reduced in our old group compared to the young group after stimulation. Therefore, α-tACS-induced motor-cortical alpha power increase (through entrainment) will be more robust in elderly individuals because of low oscillatory power that could provide a bigger window of modulation. Behaviorally, the stimulation may increase inhibitory control crucial for tasks such as the SRTT because motor memories of sequential finger movements must be inhibited when performing random trials. Young adults typically exhibit a significant alpha power increase at the sensorimotor cortices during the consolidation of a skill that requires inhibition of the learned movements (Zhuang et al., 1997; Bönstrup et al., 2015). However, α-tACS have been shown to decrease cortical inhibition in young adults and therefore may cancel out the task-induced increase in inhibition (Wach et al., 2013; Fresnoza et al., 2018). If this is true, performance in blocks with random trials should be affected. Indeed, significantly higher ERs in block 1 were observed in the young group compared to the old group after α-tACS stimulation. The high ER could have been due to inefficient motor inhibition that leads to interference on the restabilization of task rule memory at the start of each SRTT run. Impaired inhibition was indicated by the low SS scores after α-tACS stimulation.
In the α2-tACS condition, although the magnitude is different, the direction of the stimulation after-effect on SS consolidation resembles that of α-tACS. α2-tACS stimulation of the motor cortex impaired and improved consolidation compared to sham in the young and old group, respectively. We believe that the same line of argumentation we presented for GMS consolidation can be applied to these results. For instance, in the young group, an increase in motor-cortical alpha power is not expected in the α2-tACS condition due to mismatched stimulation frequency (Ali et al., 2013; Ruhnau et al., 2016). This can negatively impact SS consolidation because of deficient cortical inhibition. On the other hand, improved consolidation after α2-tACS stimulation is possible in the old group because the mismatched stimulation frequency has the potential to increase alpha power in this age group (Schmidt et al., 2014). However, as our data showed, SS consolidation improvement was less in magnitude after α2-tACS compared to α-tACS in the old group. We argue that α2-tACS stimulation may induce an excessive increase in motor-cortical alpha power in the old group which by itself can be also detrimental to SS consolidation because of excessive inhibition. For example, very high prestimulus motor-cortical alpha activity could make the motor system less responsive to inputs from other brain regions (e.g., PFC) even for signals serving to inhibit an automatic motor action (Mazaheri et al., 2009). Higher intracortical inhibition in the older adults after α2-tACS could also reduce task-dependent plasticity which is crucial for motor skill consolidation (Mary et al., 2015).
Conclusion
Our results show that motor skill consolidation can be enhanced with tACS stimulation of the motor cortex in elderly individuals. On the other hand, for young adults, tACS was detrimental to motor skill consolidation. The overall results of the present study suggest that the effects of tACS are age-dependent, that is, they depend on the overall level of alpha activity in the individual (Neuling et al., 2013). Our results suggest that tACS stimulation can remedy impaired oscillatory brain activity in order to improve motor and cognitive functions in the elderly, similar to earlier studies (Borghini et al., 2018). However, in determining the optimal stimulation frequency, it is important to individually identify the endogenous (dominant) resonant frequency for the underlying neuronal processes associated with the targeted brain area. In the primary motor cortex, 10 Hz tACS stimulation may increase “motor surround inhibition,” which is necessary for the selective activation of target muscles (e.g., the finger that should move) and the inhibition of nearby muscles (e.g., those fingers that should not move). This is necessary to produce the desired movements and prevent those unwanted (Naro et al., 2017). However, there are exceptions. For example, while cerebellar Purkinje cells’ average spike frequency is 10 Hz (Llinás, 2009), tACS applied at 10 Hz to the cerebellum was ineffective in modulating motor cortex excitability and motor performance probably because it had no or only a minimal effect on another type of inhibition called “cerebellum-brain inhibition” (CBI), as compared to 50 Hz tACS (Naro et al., 2017). In rabbits, motor behavioral effects (eyeblink) were induced at higher frequencies (30, 100 and 200 Hz) rather than 10 Hz (Márquez-Ruiz et al., 2016). Similarly, although tACS stimulation of the somatosensory cortex (SI) at alpha and high-gamma frequency was able to elicit tactile sensations (Feurra et al., 2011b), 10 Hz tACS did not modulate the ability to temporally discriminate between two subsequent tactile suprathreshold stimuli (Wittenberg et al., 2019). Overall, our data suggest that perturbations of neural oscillations can be beneficial for individuals with aberrant oscillations such as the elderly. On the other hand, for young healthy individuals with functional neural oscillation, minimal beneficial effects might be outweighed by detrimental effects.
Data Availability Statement
The datasets generated for this study are available on request to the corresponding author.
Ethics Statement
The studies involving human participants were reviewed and approved by Medical University of Graz. The patients/participants provided their written informed consent to participate in this study.
Author Contributions
SF, MC, EG, CK, UZ, and AI designed the study. SF, MC, and LB conducted the experiments and collected the data. SF, LB, and AI analyzed the data and wrote the manuscript. SF, MC, LB, EG, CK, UZ, and AI reviewed and approved the final version of the manuscript.
Funding
The present work was supported by BioTechMed, a government-supported initiative to promote research for health at three universities in Graz, Austria.
Supplementary Material
The Supplementary Material for this article can be found online at: https://www.frontiersin.org/articles/10.3389/fnagi.2020.00025/full#supplementary-material.
Conflict of Interest
The authors declare that the research was conducted in the absence of any commercial or financial relationships that could be construed as a potential conflict of interest.
References
Akaike, H. (1973). Information Theory and An Extension of the Maximum Likelihood Principle. New York, NY: Springer.
Alagapan, S., Schmidt, S. L., Lefebvre, J., Hadar, E., Shin, H. W., and Fröhlich, F. (2016). Modulation of cortical oscillations by low-frequency direct cortical stimulation is state-dependent. PLoS Biol. 14:e1002424. doi: 10.1371/journal.pbio.1002424
Ali, M. M., Sellers, K. K., and Frohlich, F. (2013). Transcranial alternating current stimulation modulates large-scale cortical network activity by network resonance. J. Neurosci. 33, 11262–11275. doi: 10.1523/JNEUROSCI.5867-12.2013
Antal, A., Boros, K., Poreisz, C., Chaieb, L., Terney, D., and Paulus, W. (2008). Comparatively weak after-effects of transcranial alternating current stimulation (tACS) on cortical excitability in humans. Brain Stimul. 1, 97–105. doi: 10.1016/j.brs.2007.10.001
Antal, A., and Paulus, W. (2013). Transcranial alternating current stimulation (tACS). Front. Hum. Neurosci. 7:317. doi: 10.3389/fnhum.2013.00317
Aspart, F., Remme, M. W. H., and Obermayer, K. (2018). Differential polarization of cortical pyramidal neuron dendrites through weak extracellular fields. PLoS Comput. Biol. 14:e1006124. doi: 10.1371/journal.pcbi.1006124
Babiloni, C., Binetti, G., Cassarino, A., Dal Forno, G., Del Percio, C., Ferreri, F., et al. (2006). Sources of cortical rhythms in adults during physiological aging: a multicentric EEG study. Hum. Brain Mapp. 27, 162–172. doi: 10.1002/hbm.20175
Barr, D. J., Levy, R., Scheepers, C., and Tily, H. J. (2013). Random effects structure for confirmatory hypothesis testing: keep it maximal. J. Mem. Lang. 68, 255–278. doi: 10.1016/j.jml.2012.11.001
Bennett, I., Howard, J., and Howard, D. (2007). Age-related differences in implicit learning of subtle third-order sequential structure. J. Gerontol. B Psychol. Sci. Soc. Sci. 62, P98–P103. doi: 10.1093/geronb/62.2.p98
Berchicci, M., Lucci, G., Pesce, C., Spinelli, D., and Di Russo, F. (2012). Prefrontal hyperactivity in older people during motor planning. NeuroImage 62, 1750–1760. doi: 10.1016/j.neuroimage.2012.06.031
Berghuis, K. M. M., Veldman, M. P., Solnik, S., Koch, G., Zijdewind, I., and Hortobágyi, T. (2015). Neuronal mechanisms of motor learning and motor memory consolidation in healthy old adults. Age 37:9779. doi: 10.1007/s11357-015-9779-8
Bönstrup, M., Hagemann, J., Gerloff, C., Sauseng, P., and Hummel, F. C. (2015). α oscillatory correlates of motor inhibition in the aged brain. Front. Aging Neurosci. 7:193. doi: 10.3389/fnagi.2015.00193
Borghini, G., Candini, M., Filannino, C., Hussain, M., Walsh, V., Romei, V., et al. (2018). α oscillations are causally linked to inhibitory abilities in ageing. J. Neurosci. 38, 4418–4429. doi: 10.1523/JNEUROSCI.1285-17.2018
Brashers-Krug, T., Shadmehr, R., and Bizzi, E. (1996). Consolidation in human motor memory. Nature 382, 252–255. doi: 10.1038/382252a0
Brown, R. M., Robertson, E. M., and Press, D. Z. (2009). Sequence skill acquisition and off-line learning in normal aging. PLoS One 4:e6683. doi: 10.1371/journal.pone.0006683
Burnham, K., and Anderson, D. (2002). Model Selection and Multimodel Inference: A Practical Information-Theoretic Approach. New York, NY: Springer-Verlag.
Catalan, M. J., Honda, M., Weeks, R. A., Cohen, L. G., and Hallett, M. (1998). The functional neuroanatomy of simple and complex sequential finger movements: a PET study. Brain 121, 253–264. doi: 10.1093/brain/121.2.253
Centeno, C., Medeiros, D., Beck, M. M., Lugassy, L., Gonzalez, D. F., Nepveu, J. F., et al. (2018). The effects of aging on cortico-spinal excitability and motor memory consolidation. Neurobiol. Aging 70, 254–264. doi: 10.1016/j.neurobiolaging.2018.06.035
Cespón, J., Galdo-Álvarez, S., and Díaz, F. (2013). Age-related changes in ERP correlates of visuospatial and motor processes. Psychophysiology 50, 743–757. doi: 10.1111/psyp.12063
Cornelis, C., De Picker, L. J., De Boer, P., Dumont, G., Coppens, V., Morsel, A., et al. (2016). Implicit motor sequence learning in schizophrenia and in old age: reduced performance only in the third session. Exp. Brain Res. 234, 3531–3542. doi: 10.1007/s00221-016-4751-0
Curran, T. (1997). Effects of aging on implicit sequence learning: accounting for sequence structure and explicit knowledge. Psychol. Res. 60, 24–41. doi: 10.1007/bf00419678
Deiber, M.-P., Sallard, E., Ibañez, V., Ludwig, C., and Barral, J. (2014). Aging and the lateralization of oscillatory activities related to external and internal motor preparation. J. Psychophysiol. 28, 136–147. doi: 10.1027/0269-8803/a000121
Derambure, P., Defebvre, L., Dujardin, K., Bourriez, J. L., Jacquesson, J. M., Destee, A., et al. (1993). Effect of aging on the spatio-temporal pattern of event-related desynchronization during a voluntary movement. Electroencephalogr. Clin. Neurophysiol. 89, 197–203. doi: 10.1016/0168-5597(93)90133-a
Faria, P., Hallett, M., and Miranda, P. C. (2011). A finite element analysis of the effect of electrode area and inter-electrode distance on the spatial distribution of the current density in tDCS. J. Neural Eng. 8:66017. doi: 10.1088/1741-2560/8/6/066017
Feurra, M., Bianco, G., Santarnecchi, E., Del Testa, M., Rossi, A., and Rossi, S. (2011a). Frequency-dependent tuning of the human motor system induced by transcranial oscillatory potentials. J. Neurosci. 31, 12165–12170. doi: 10.1523/JNEUROSCI.0978-11.2011
Feurra, M., Paulus, W., Walsh, V., and Kanai, R. (2011b). Frequency specific modulation of human somatosensory cortex. Front. Psychol. 2:13. doi: 10.3389/fpsyg.2011.00013
Feurra, M., Pasqualetti, P., Bianco, G., Santarnecchi, E., Rossi, A., and Rossi, S. (2013). State-dependent effects of transcranial oscillatory currents on the motor system: what you think matters. J. Neurosci. 33, 17483–17489. doi: 10.1523/JNEUROSCI.1414-13.2013
Feve, A. P., Bathien, N., and Rondot, P. (1991). Les potentiels corticaux liés au mouvement de l’homme âgé. Neurophysiol. Clin. 21, 281–291. doi: 10.1016/s0987-7053(05)80376-4
Fresnoza, S., Christova, M., Feil, T., Gallasch, E., Körner, C., Zimmer, U., et al. (2018). The effects of transcranial alternating current stimulation (tACS) at individual α peak frequency (iAPF) on motor cortex excitability in young and elderly adults. Exp. Brain Res. 236, 2573–2588. doi: 10.1007/s00221-018-5314-3
Fröhlich, F., and McCormick, D. A. (2010). Endogenous electric fields may guide neocortical network activity. Neuron 67, 129–143. doi: 10.1016/j.neuron.2010.06.005
Galdo-Alvarez, S., Bonilla, F. M., González-Villar, A. J., and Carrillo-de-la-Peña, M. T. (2016). Functional equivalence of imagined vs. Real performance of an inhibitory task: an EEG/ERP study. Front. Hum. Neurosci. 10:467. doi: 10.3389/fnhum.2016.00467
Galloway, A. (1993). The evolutionary biology of aging. By Michael R. Rose. New York: Oxford University Press. 1991. ix + 221 pp. ISBN 0-19–506133-0. $35.00 (cloth). Am. J. Phys. Anthropol. 91, 260–262. doi: 10.1002/ajpa.1330910217
Gongora, M., Bittencourt, J., Teixeira, S., Basile, L. F., Pompeu, F., and Droguett, E. L. (2016). Low-frequency rTMS over the parieto-frontal network during a sensorimotor task: the role of absolute β power in the sensorimotor integration. Neurosci. Lett. 611, 1–5. doi: 10.1016/j.neulet.2015.11.025
Good, C. D., Johnsrude, I. S., Ashburner, J., Henson, R. N. A., Friston, K. J., and Frackowiak, R. S. J. (2001). A voxel-based morphometric study of ageing in 465 normal adult human brains. NeuroImage 14, 21–36. doi: 10.1006/nimg.2001.0786
Helfrich, R. F., Schneider, T. R., Rach, S., Trautmann-Lengsfeld, S. A., Engel, A. K., and Herrmann, C. S. (2014). Entrainment of brain oscillations by transcranial alternating current stimulation. Curr. Biol. 24, 333–339. doi: 10.1016/j.cub.2013.12.041
Higuchi, S., Liu, Y., Yuasa, T., Maeda, A., and Motohashi, Y. (2001). Diurnal variations in α power density and subjective sleepiness while performing repeated vigilance tasks. Clin. Neurophysiol. 112, 997–1000. doi: 10.1016/s1388-2457(01)00527-2
Hummel, F., Andres, F., Altenmüller, E., Dichgans, J., and Gerloff, C. (2002). Inhibitory control of acquired motor programmes in the human brain. Brain 125, 404–420. doi: 10.1093/brain/awf030
Hummel, F., Saur, R., Lasogga, S., Plewnia, C., Erb, M., Wildgruber, D., et al. (2004). To act or not to act. Neural correlates of executive control of learned motor behavior. NeuroImage 23, 1391–1401. doi: 10.1016/j.neuroimage.2004.07.070
Ishii, R., Canuet, L., Aoki, Y., Hata, M., Iwase, M., Ikeda, S., et al. (2017). Healthy and pathological brain aging: from the perspective of oscillations, functional connectivity, and signal complexity. Neuropsychobiology 75, 151–161. doi: 10.1159/000486870
Jensen, O., and Mazaheri, A. (2010). Shaping functional architecture by oscillatory α activity: gating by inhibition. Front. Hum. Neurosci. 4:186. doi: 10.3389/fnhum.2010.00186
Kasten, F. H., and Herrmann, C. S. (2017). Transcranial alternating current stimulation (tACS) enhances mental rotation performance during and after stimulation. Front. Hum. Neurosci. 11:2. doi: 10.3389/fnhum.2017.00002
Kasten, F. H., Dowsett, J., and Herrmann, C. S. (2016). Sustained aftereffect of α-tACS lasts up to 70 min after stimulation. Front. Hum. Neurosci. 10:245. doi: 10.3389/fnhum.2016.00245
Kikuchi, M., Wada, Y., Koshino, Y., Nanbu, Y., and Hashimoto, T. (2000). Effect of normal aging upon interhemispheric EEG coherence: analysis during rest and photic stimulation. Clin. Electroencephalogr. 31, 170–174. doi: 10.1177/155005940003100404
Kim, H.-C., and Lee, J.-H. (2015). “Desynchronization of the mu oscillatory activity during motor imagery: a preliminary EEG-fMRI study,” in The 3rd International Winter Conference on Brain-Computer Interface (IEEE), New Jersey, USA, 1–2.
King, B., Fogel, S., Albouy, G., and Doyon, J. (2013). Neural correlates of the age-related changes in motor sequence learning and motor adaptation in older adults. Front. Hum. Neurosci. 7:142. doi: 10.3389/fnhum.2013.00142
Klimesch, W. (1999). EEG α and theta oscillations reflect cognitive and memory performance: a review and analysis. Brain Res. Rev. 29, 169–195. doi: 10.1016/s0165-0173(98)00056-3
Klimesch, W., Sauseng, P., and Hanslmayr, S. (2007). EEG α oscillations: the inhibition-timing hypothesis. Brain Res. Rev. 53, 63–88. doi: 10.1016/j.brainresrev.2006.06.003
Knyazeva, M. G., Barzegaran, E., Vildavski, V. Y., and Demonet, J.-F. (2018). Aging of human α rhythm. Neurobiol. Aging 69, 261–273. doi: 10.1016/j.neurobiolaging.2018.05.018
Koelewijn, L., Bompas, A., Tales, A., Brookes, M. J., Muthukumaraswamy, S. D., Bayer, A., et al. (2017). Alzheimer’s disease disrupts α and β-band resting-state oscillatory network connectivity. Clin. Neurophysiol. 128, 2347–2357. doi: 10.1016/j.clinph.2017.04.018
Kolev, V., Yordanova, J., Basar-Eroglu, C., and Basar, E. (2002). Age effects on visual EEG responses reveal distinct frontal α networks. Clin. Neurophysiol. 113, 901–910. doi: 10.1016/s1388-2457(02)00106-2
Kraan, C. M., Hocking, D. R., Bradshaw, J. L., Georgiou-Karistianis, N., Metcalfe, S. A., Archibald, A. D., et al. (2014). Symbolic sequence learning is associated with cognitive-affective profiles in female FMR1 premutation carriers. Genes Brain Behav. 13, 385–393. doi: 10.1111/gbb.12122
Kuo, M.-F., Unger, M., Liebetanz, D., Lang, N., Tergau, F., Paulus, W., et al. (2008). Limited impact of homeostatic plasticity on motor learning in humans. Neuropsychologia 46, 2122–2128. doi: 10.1016/j.neuropsychologia.2008.02.023
Labyt, E., Szurhaj, W., Bourriez, J.-L., Cassim, F., Defebvre, L., Destée, A., et al. (2003). Changes in oscillatory cortical activity related to a visuomotor task in young and elderly healthy subjects. Clin. Neurophysiol. 114, 1153–1166. doi: 10.1016/s1388-2457(03)00058-0
Lindner, A., Iyer, A., Kagan, I., and Andersen, R. A. (2010). Human posterior parietal cortex plans where to reach and what to avoid. J. Neurosci. 30, 11715–11725. doi: 10.1523/JNEUROSCI.2849-09.2010
Llinás, R. R. (2009). Inferior olive oscillation as the temporal basis for motricity and oscillatory reset as the basis for motor error correction. Neuroscience 162, 797–804. doi: 10.1016/j.neuroscience.2009.04.045
Márquez-Ruiz, J., Ammann, C., Leal-Campanario, R., Ruffini, G., Gruart, A., and Delgado-García, J. M. (2016). Synthetic tactile perception induced by transcranial alternating-current stimulation can substitute for natural sensory stimulus in behaving rabbits. Sci. Rep. 6:19753. doi: 10.1038/srep19753
Mary, A., Bourguignon, M., Wens, V., Op de Beeck, M., Leproult, R., De Tiège, X., et al. (2015). Aging reduces experience-induced sensorimotor plasticity. A magnetoencephalographic study. NeuroImage 104, 59–68. doi: 10.1016/j.neuroimage.2014.10.010
Mattay, V. S., Fera, F., Tessitore, A., Hariri, A. R., Das, S., Callicott, J. H., et al. (2002). Neurophysiological correlates of age-related changes in human motor function. Neurology 58, 630–635. doi: 10.1212/wnl.58.4.630
Mazaheri, A., Nieuwenhuis, I. L. C., van Dijk, H., and Jensen, O. (2009). Prestimulus α and mu activity predicts failure to inhibit motor responses. Hum. Brain Mapp. 30, 1791–1800. doi: 10.1002/hbm.20763
Meier, B., and Cock, J. (2014). Offline consolidation in implicit sequence learning. Cortex 57, 156–166. doi: 10.1016/j.cortex.2014.03.009
Meissner, S. N., Keitel, A., Südmeyer, M., and Pollok, B. (2016). Implicit motor sequence learning and working memory performance changes across the adult life span. Front. Aging Neurosci. 8:89. doi: 10.3389/fnagi.2016.00089
Miranda, P. C., Lomarev, M., and Hallett, M. (2006). Modeling the current distribution during transcranial direct current stimulation. Clin. Neurophysiol. 117, 1623–1629. doi: 10.1016/j.clinph.2006.04.009
Nam, C. S., Jeon, Y., Kim, Y.-J., Lee, I., and Park, K. (2011). Movement imagery-related lateralization of event-related (de)synchronization (ERD/ERS): motor-imagery duration effects. Clin. Neurophysiol. 122, 567–577. doi: 10.1016/j.clinph.2010.08.002
Naro, A., Bramanti, A., Leo, A., Manuli, A., Sciarrone, F., Russo, M., et al. (2017). Effects of cerebellar transcranial alternating current stimulation on motor cortex excitability and motor function. Brain Struct. Funct. 222, 2891–2906. doi: 10.1007/s00429-016-1355-1
Nemeth, D., and Janacsek, K. (2011). The dynamics of implicit skill consolidation in young and elderly adults. J Gerontol. B Psychol. Sci. Soc. Sci. 66, 15–22. doi: 10.1093/geronb/gbq063
Neuling, T., Rach, S., and Herrmann, C. S. (2013). Orchestrating neuronal networks: sustained after-effects of transcranial alternating current stimulation depend upon brain states. Front. Hum. Neurosci. 7:161. doi: 10.3389/fnhum.2013.00161
Nissen, M. J., and Bullemer, P. (1987). Attentional requirements of learning: evidence from performance measures. Cogn. Psychol. 19, 1–32. doi: 10.1016/0010-0285(87)90002-8
Nitsche, M. A., Doemkes, S., Karaköse, T., Antal, A., Liebetanz, D., Lang, N., et al. (2007). Shaping the effects of transcranial direct current stimulation of the human motor cortex. J. Neurophysiol. 97, 3109–3117. doi: 10.1152/jn.01312.2006
Nitsche, M. A., Schauenburg, A., Lang, N., Liebetanz, D., Exner, C., Paulus, W., et al. (2003). Facilitation of implicit motor learning by weak transcranial direct current stimulation of the primary motor cortex in the human. J. Cogn. Neurosci. 15, 619–626. doi: 10.1162/089892903321662994
Oldfield, R. C. (1971). The assessment and analysis of handedness: the Edinburgh inventory. Neuropsychologia 9, 97–113. doi: 10.1016/0028-3932(71)90067-4
Oliveira, F. T. P., Diedrichsen, J., Verstynen, T., Duque, J., and Ivry, R. B. (2010). Transcranial magnetic stimulation of posterior parietal cortex affects decisions of hand choice. Proc. Natl. Acad. Sci. U S A 107, 17751–17756. doi: 10.1073/pnas.1006223107
Ota, M., Obata, T., Akine, Y., Ito, H., Ikehira, H., Asada, T., et al. (2006). Age-related degeneration of corpus callosum measured with diffusion tensor imaging. NeuroImage 31, 1445–1452. doi: 10.1016/j.neuroimage.2006.02.008
Pascual-Leone, A., Grafman, J., and Hallett, M. (1994). Modulation of cortical motor output maps during development of implicit and explicit knowledge. Science 263, 1287–1289. doi: 10.1126/science.8122113
Pedersen, A., and Ohrmann, P. (2018). Impaired behavioral inhibition in implicit sequence learning in adult ADHD. J. Atten. Disord. 22, 250–260. doi: 10.1177/1087054712464392
Peirce, J. (2009). Generating stimuli for neuroscience using PsychoPy. Front. Neuroinform. 2:10. doi: 10.3389/neuro.11.010.2008
Petzschner, F. H., and Krüger, M. (2012). How to reach: movement planning in the posterior parietal cortex. J. Neurosci. 32, 4703–4704. doi: 10.1523/JNEUROSCI.0566-12.2012
Pollok, B., Boysen, A.-C., and Krause, V. (2015). The effect of transcranial alternating current stimulation (tACS) at α and β frequency on motor learning. Behav. Brain Res. 293, 234–240. doi: 10.1016/j.bbr.2015.07.049
Poreisz, C., Boros, K., Antal, A., and Paulus, W. (2007). Safety aspects of transcranial direct current stimulation concerning healthy subjects and patients. Brain Res. Bull. 72, 208–214. doi: 10.1016/j.brainresbull.2007.01.004
Quandt, F., Bönstrup, M., Schulz, R., Timmermann, J. E., Zimerman, M., Nolte, G., et al. (2016). Spectral variability in the aged brain during fine motor control. Front. Aging Neurosci. 8:305. doi: 10.3389/fnagi.2016.00305
Raz, N., Lindenberger, U., Rodrigue, K. M., Kennedy, K. M., Head, D., Williamson, A., et al. (2005). Regional brain changes in aging healthy adults: general trends, individual differences and modifiers. Cereb. Cortex 15, 1676–1689. doi: 10.1093/cercor/bhi044
Roggeveen, A. B., Prime, D. J., and Ward, L. M. (2007). Lateralized readiness potentials reveal motor slowing in the aging brain. J. Gerontol. B Psychol. Sci. Soc. Sci. 62, P78–P84. doi: 10.1093/geronb/62.2.p78
Roig, M., Ritterband-Rosenbaum, A., Lundbye-Jensen, J., and Nielsen, J. B. (2014). Aging increases the susceptibility to motor memory interference and reduces off-line gains in motor skill learning. Neurobiol. Aging 35, 1892–1900. doi: 10.1016/j.neurobiolaging.2014.02.022
Ruhnau, P., Neuling, T., Fuscá, M., Herrmann, C. S., Demarchi, G., and Weisz, N. (2016). Eyes wide shut: transcranial alternating current stimulation drives α rhythm in a state dependent manner. Sci. Rep. 6:27138. doi: 10.1038/srep27138
Salat, D. H., Buckner, R. L., Snyder, A. Z., Greve, D. N., Desikan, R. S., Busa, E., et al. (2004). Thinning of the cerebral cortex in aging. Cereb. Cortex 14, 721–730. doi: 10.1093/cercor/bhh032
Salthouse, T. A., McGuthry, K. E., and Hambrick, D. Z. (1999). A framework for analyzing and interpreting differential aging patterns: application to three measures of implicit learning. Aging Neuropsychol. Cogn. 6, 1–18. doi: 10.1076/anec.6.1.1.789
Sauseng, P., Gerloff, C., and Hummel, F. C. (2013). Two brakes are better than one: the neural bases of inhibitory control of motor memory traces. NeuroImage 65, 52–58. doi: 10.1016/j.neuroimage.2012.09.048
Savic, B., and Meier, B. (2016). How transcranial direct current stimulation can modulate implicit motor sequence learning and consolidation: a brief review. Front. Hum. Neurosci. 10:26. doi: 10.3389/fnhum.2016.00026
Scally, B., Burke, M. R., Bunce, D., and Delvenne, J.-F. (2018). Resting-state EEG power and connectivity are associated with α peak frequency slowing in healthy aging. Neurobiol. Aging 71, 149–155. doi: 10.1016/j.neurobiolaging.2018.07.004
Schmidt, S. L., Iyengar, A. K., Foulser, A. A., Boyle, M. R., and Fröhlich, F. (2014). Endogenous cortical oscillations constrain neuromodulation by weak electric fields. Brain Stimul. 7, 878–889. doi: 10.1016/j.brs.2014.07.033
Searle, S. R. (1988). Mixed models and unbalanced data: wherefrom, whereat and whereto? Commun. Stat. 17, 935–968. doi: 10.1080/03610928808829667
Seidler, R. D., Bernard, J. A., Burutolu, T. B., Fling, B. W., Gordon, M. T., Gwin, J. T., et al. (2010). Motor control and aging: links to age-related brain structural, functional, and biochemical effects. Neurosci. Biobehav. Rev. 34, 721–733. doi: 10.1016/j.neubiorev.2009.10.005
Singer, J. D., and Willett, J. B. (2003). Applied Longitudinal Data Analysis: Modeling Change and Event Occurrence Applied longitudinal data analysis: Modeling Change and Event Occurrence. New York, NY: Oxford University Press.
Strüber, D., Rach, S., Neuling, T., and Herrmann, C. S. (2015). On the possible role of stimulation duration for after-effects of transcranial alternating current stimulation. Front. Cell. Neurosci. 9:311. doi: 10.3389/fncel.2015.00311
Sullivan, E. V., Rohlfing, T., and Pfefferbaum, A. (2010). Quantitative fiber tracking of lateral and interhemispheric white matter systems in normal aging: relations to timed performance. Neurobiol. Aging 31, 464–481. doi: 10.1016/j.neurobiolaging.2008.04.007
Toloza, E. H. S., Negahbani, E., and Fröhlich, F. (2018). Ih interacts with somato-dendritic structure to determine frequency response to weak alternating electric field stimulation. J. Neurophysiol. 119, 1029–1036. doi: 10.1152/jn.00541.2017
Truong, D. Q., Magerowski, G., Blackburn, G. L., Bikson, M., and Alonso-Alonso, M. (2013). Computational modeling of transcranial direct current stimulation (tDCS) in obesity: impact of head fat and dose guidelines. NeuroImage Clin. 2, 759–766. doi: 10.1016/j.nicl.2013.05.011
Urry, K., Burns, N. R., and Baetu, I. (2018). Age-related differences in sequence learning: findings from two visuo-motor sequence learning tasks. Br. J. Psychol. 109, 830–849. doi: 10.1111/bjop.12299
Vallesi, A., McIntosh, A. R., Kovacevic, N., Chan, S. C. C., and Stuss, D. T. (2010). Age effects on the asymmetry of the motor system: evidence from cortical oscillatory activity. Biol. Psychol. 85, 213–218. doi: 10.1016/j.biopsycho.2010.07.003
Vossen, A., Gross, J., and Thut, G. (2015). α power increase after transcranial alternating current stimulation at α frequency (α-tACS) reflects plastic changes rather than entrainment. Brain Stimul. 8, 499–508. doi: 10.1016/j.brs.2014.12.004
Vysata, O., Kukal, J., Prochazka, A., Pazdera, L., Simko, J., and Valis, M. (2014). Age-related changes in EEG coherence. Neurol. Neurochir. Pol. 48, 35–38. doi: 10.1016/j.pjnns.2013.09.001
Wach, C., Krause, V., Moliadze, V., Paulus, W., Schnitzler, A., and Pollok, B. (2013). Effects of 10 Hz and 20 Hz transcranial alternating current stimulation (tACS) on motor functions and motor cortical excitability. Behav. Brain Res. 241, 1–6. doi: 10.1016/j.bbr.2012.11.038
Walker, M. P., Brakefield, T., Hobson, J. A., and Stickgold, R. (2003). Dissociable stages of human memory consolidation and reconsolidation. Nature 425, 616–620. doi: 10.1038/nature01930
Warton, D. I., Lyons, M., Stoklosa, J., and Ives, A. R. (2016). Three points to consider when choosing a LM or GLM test for count data. Methods Ecol. Evol. 7, 882–890. doi: 10.1111/2041-210x.12552
Wittenberg, M. A., Morr, M., Schnitzler, A., and Lange, J. (2019). 10 Hz tACS over somatosensory cortex does not modulate supra-threshold tactile temporal discrimination in humans. Front. Neurosci. 13:311. doi: 10.3389/fnins.2019.00311
Yokoi, A., Arbuckle, S. A., and Diedrichsen, J. (2018). The role of human primary motor cortex in the production of skilled finger sequences. J. Neurosci. 38, 1430–1442. doi: 10.1523/JNEUROSCI.2798-17.2017
Yordanova, J., Kolev, V., Hohnsbein, J., and Falkenstein, M. (2004). Sensorimotor slowing with ageing is mediated by a functional dysregulation of motor-generation processes: evidence from high-resolution event-related potentials. Brain 127, 351–362. doi: 10.1093/brain/awh042
Zaehle, T., Rach, S., and Herrmann, C. S. (2010). Transcranial alternating current stimulation enhances individual α activity in human EEG. PLoS One 5:e13766. doi: 10.1371/journal.pone.0013766
Keywords: transcranial alternating current stimulation, motor learning, alpha frequency, age-dependent, skill consolidation
Citation: Fresnoza S, Christova M, Bieler L, Körner C, Zimmer U, Gallasch E and Ischebeck A (2020) Age-Dependent Effect of Transcranial Alternating Current Stimulation on Motor Skill Consolidation. Front. Aging Neurosci. 12:25. doi: 10.3389/fnagi.2020.00025
Received: 16 July 2019; Accepted: 22 January 2020;
Published: 06 February 2020.
Edited by:
John F. Disterhoft, Northwestern University, United StatesReviewed by:
Jesus Cespon, Basque Center on Cognition, Brain and Language, SpainJosé M. Delgado-García, Universidad Pablo de Olavide, Spain
Copyright © 2020 Fresnoza, Christova, Bieler, Körner, Zimmer, Gallasch and Ischebeck. This is an open-access article distributed under the terms of the Creative Commons Attribution License (CC BY). The use, distribution or reproduction in other forums is permitted, provided the original author(s) and the copyright owner(s) are credited and that the original publication in this journal is cited, in accordance with accepted academic practice. No use, distribution or reproduction is permitted which does not comply with these terms.
*Correspondence: Shane Fresnoza, shane.fresnoza@uni-graz.at