- 1Department of Community Healthcare and Geriatrics, Nagoya University Graduate School of Medicine, Nagoya, Japan
- 2Institute of Innovation for Future Society, Nagoya University, Nagoya, Japan
- 3Liberal Arts and Sciences, Faculty of Engineering, Toyama Prefectural University, Toyama, Japan
- 4Department of Preventive Gerontology, Center for Gerontology and Social Science, National Center for Geriatrics and Gerontology, Obu, Japan
Insulin resistance (IR), diabetes mellitus (DM), sarcopenia, and cognitive dysfunction are thought to be mutually associated. We conducted a comprehensive assessment of the relationships among IR, gait speed, hyperglycemia, and DM by cross-sectionally analyzing the baseline data of an interventional study for cognitive preservation with physical exercise (the TOyota Preventional Intervention for Cognitive decline and Sarcopenia [TOPICS]). The participants (n = 444) were relatively healthy older individuals who had mild cognitive impairment without dementia, and 61 of the participants had DM. Slow gait speed and hyperglycemia were associated with cognitive dysfunction, mainly in the executive function domain, whereas IR was associated with memory impairment. The participants with DM had lower general cognition and executive function. Executive dysfunction in the DM participants seemed to be partly explained by hyperglycemia and/or slow gait speed. Our findings confirmed that IR, DM, sarcopenia, and cognitive dysfunction are mutually associated in complex ways. Understanding the mechanisms underlying these associations will lead to effective strategies to prevent and treat cognitive dysfunction in older individuals.
Introduction
Along with the increasingly aging populations in mainly developed countries, the number of individuals with cognitive impairment has grown, leading to increases in medical expenses as well as economic, physical, and psychological care-related burdens. There are no curative treatments for cognitive dysfunction at this time, and the prevention of cognitive decline is a critical issue. Toward this end, the identification of cognitive dysfunction risk factors (especially modifiable factors) is urgently needed, and interventional trials for such risk factors would be warranted in order to develop effective therapies.
Many studies have shown that individuals with diabetes mellitus (DM) have higher risks of dementia and cognitive dysfunction (Koekkoek et al., 2015). The mechanism that underlies DM-related cognitive dysfunction has not been fully elucidated, but several hypotheses have been put forward (Umegaki, 2014). Hyperglycemia is involved in the mechanism of DM-associated cognitive dysfunction (Geijselaers et al., 2015), as are other factors. Insulin resistance (IR) often leads to DM, and patients with DM sometimes have cognitive dysfunction. The results obtained in basic researches have suggested that systemic IR may induce brain IR, or a deficiency of insulin effects in the brain, which in turn may lead to the phosphorylation of tau and the production of amyloid beta, and to Alzheimer disease (AD)-related pathology (Bedse et al., 2015).
Muscle is a major target of insulin. Insulin binds to the receptors on the muscle cells to transport blood glucose into muscle (Ryder et al., 2001). In humans, muscle mass and performance decrease with age, via a phenomenon known as sarcopenia (Rosenberg, 1997). Sarcopenia is thus characterized by a loss of muscle mass and strength (Cruz-Jentoft et al., 2010). Because insulin has a catabolic effect, IR, which induces impairment of protein synthesis in muscle, is suspected to be involved in the development of sarcopenia (Umegaki, 2016). The diagnosis of sarcopenia is based on the reduction of muscle mass, but a loss in muscle power and a decline in physical performance are also required for the diagnosis.
Gait speed is often used as an index of physical performance. Several studies have reported that slower gait speed was associated with cognitive dysfunction, mainly in the executive function domain (Kearney et al., 2013; Demnitz et al., 2016), and that slower gait speed is predictive of adverse health events including dementia (Buchner et al., 1996; Guralnik et al., 2000). The age-associated muscle mass reduction in sarcopenia has also been reported to be associated with cognitive dysfunction (Nourhashémi et al., 2002; Doi et al., 2014). Moreover, Burns et al. (2010) reported that the age-associated reduction of lean muscle mass is accelerated in AD and is associated with brain atrophy and cognitive performance. Several studies have reported that DM is associated with sarcopenia or low muscle performance (Kearney et al., 2013; Demnitz et al., 2016). It may be speculated that DM-related cognitive dysfunction is at least partly associated with sarcopenia or low muscle performance, although there have been few studies examining these potential associations.
DM is often associated with various metabolic alterations, such as hyperglycemia and IR, as well as with indices of sarcopenia, particularly low muscle performance (Umegaki, 2016; Zaslavsky et al., 2016). Both sarcopenia (Nourhashémi et al., 2002; Doi et al., 2014) and IR (Umegaki et al., 2008; Ekblad et al., 2015) have been associated with cognitive dysfunction in older subjects. Therefore, the underlying mechanisms of DM-related cognitive dysfunction may include or be associated with IR and low muscle function. However, the contributions of sarcopenia and IR to DM-related cognitive dysfunction have not been studied extensively. Thus, while it is anticipated that associations exist among IR, sarcopenia, and cognitive dysfunction, few studies have comprehensively assessed the associations these associations. In the present study we investigated the associations among IR, sarcopenia, and cognitive dysfunction to determine the contributions of IR and declining physical performance to the development of DM-related cognitive dysfunction.
Methods
This was a randomized control trial to assess the effects of different types of exercise (aerobic, resistance, or both) on cognition in older subjects with a slight decline of memory function based on the cross-sectional analysis of baseline data from the TOyota Preventional Intervention for Cognitive decline and Sarcopenia [TOPICS].
The study protocol was approved by our University's Ethics Committee (Graduate School of Medicine, Nagoya University; approval no. 2014-0155-2) and registered with the University Hospital Medical Information Network (UMIN) clinical trials registry (no. UMIN000014437). Written informed consent was obtained from all participants prior to their inclusion in the study.
Participants
Participants were screened with the use of a questionnaire comprised of 25 self-completed items (Arai and Satake, 2015), including three items concerning subjective cognitive decline: Q18, Do your family or friends point out your memory loss? (e.g., “You ask the same question over and over again”); Q19, Do you make a call by looking up phone numbers?; and Q20, Do you find yourself not knowing today's date? Respondents who answered yes to Q18 or Q20 or who answered no to Q19 were regarded as being at high risk of cognitive decline (Maki et al., 2012). The questionnaire was mailed to community-dwelling residents aged 65–85 years in selected areas in the city of Toyota, Aichi, Japan. Residents who met the criteria for being at risk of cognitive decline according to at least one of the three questionnaire items described above were recruited via a mailing describing the interventional study project.
The exclusion criteria were as follows: the subject (1) meets clinical criteria for dementia, (2) has any disability with respect to basic and instrumental activities of daily living (ADLs), (3) requires support or care from the Japanese public long-term care insurance system, (4) has a Mini-Mental State Examination (MMSE) score ≤19, (5) has severe visual impairment, (6) has been diagnosed with a neurodegenerative disease (e.g., Parkinson's disease), (7) has medical conditions for which exercise is contraindicated, (8) has a psychiatric disease (i.e., major depressive disorder), or (9) has a history of serious cardiovascular, musculoskeletal, respiratory, or cerebrovascular disease or other severe health issue.
DM was defined as a self-report of diagnosed DM, current use of antidiabetic medication, fasting plasma glucose ≥126 mg/dl, or HbA1c ≥6.5%. We excluded fasting blood glucose >140 mg/dl from the present analysis because it is not suitable for the assessment of IR in these subjects (Wallace et al., 2004) with homeostatic model assessment-insulin resistance (HOMA-IR; Matthews et al., 1985).
Measurements
A battery of neuropsychological assessments were performed by a group of well-trained clinical psychologists, speech therapists, and occupational therapists. Each score was rechecked by a single neuropsychologist who was blinded regarding the other data associated with the participants. The following tests were used. To assess memory, we used the Logical Memory II subtest of the WMS-R (Guo et al., 2009; Aisen et al., 2010); the MSE for general cognitive ability (Folstein et al., 1975); and the Logical Memory I subtests and Visual Reproduction I and II subtests of the WMS-R (Wechsler, 1987). For verbal fluency, we used the category fluency (animal naming) test and the letter fluency test. For working memory, we used the Digit Span (forward and backward) subtest and Visual Memory Span (forward and backward) subtest of the WMS-R for working memory. Finally, to assess processing speed, we used the Digit Symbol subtest of the Wechsler Adult Intelligence Scale-III (WAIS-III; Wechsler, 1997); and the Trail Making Test parts A and B (Spreen and Strauss, 1998).
Physical Function
We measured grip strength in the dominant hand of each participant with the use of a portable grip strength dynamometer (GRIP-D; Takei, Ltd., Niigata, Japan). Gait speed was assessed during normal walking, with the participant instructed to walk at his or her preferred speed (Doi et al., 2014).
Body Composition
We used a bioelectrical impedance data acquisition system (Inbody 430; Biospace Co., Seoul, Korea) to perform the bioelectrical impedance analyses. This system uses electrical current at three frequencies (5, 50, 250 kHz) to directly measure the amounts of extracellular and intracellular water in the human body. Using segmental body composition and muscle mass, each participant's appendicular skeletal muscle mass was determined and retained for further analysis. The skeletal muscle mass index (SMI) was calculated by dividing the participant's muscle mass by his or her height in meters squared (kg/m2).
Blood Markers
A blood sample was obtained after the participant had fasted for ≥12 h. Serum samples were analyzed for total cholesterol, high-density lipoprotein cholesterol, triglyceride, glucose, glycosylated hemoglobin (HbA1c), and insulin. The apolipoprotein E (apoE) genotypes were classified into e2/e2, e2/e3, e3/e3, e2/e4, e3/e4, and e4/e4 based on the immunoblot technique using isoelectric focusing. We obtained the blood samples in the morning (between 9:30 a.m. and 12:00 p.m.). Insulin resistance was assessed by HOMA-IR (Matthews et al., 1985).
Statistical Analysis
Student's t-test was used for the comparison of continuous variables. The distribution of frequencies for categorical variables was analyzed with the Chi-square (χ2) test. We performed a multiple regression analysis to investigate the associations of cognitive performance and the factors of HOMA-IR values, gait speed, HbA1c, and DM diagnosis with adjustment for age, sex, number of school years, and apoE4 status. In the tests that revealed a significant association with DM, we performed further adjustment for any factors that were significantly associated with cognition among HOMA-IR values, gait speed, and HbA1c.
Results
Table 1 shows the background characteristics of the participants. In both males and females, the body mass index (BMI) values were significantly larger in the participants with DM. Among the participants with DM (n = 61), the blood glucose, HbA1c, insulin, and HOMAR-IR values were higher, but total cholesterol (T-chol) and high-density lipoprotein cholesterol (HDL-chol) were lower compared to those in the non-DM group (n = 383).
The body compositions and performance of the DM and non-DM groups were comparable, with the exception that gait speed was significantly lower (p = 0.024) in the DM group (Table 2).
In the cognitive assessment, the scores of MMSE, semantic fluency, digit span, digit symbol, TMT-A, and TMT-B were significantly lower in the DM group (Table 3).
The results of the multiple regression analysis (Table 4) showed that HOMA-IR was associated with logical memory II after adjustment for age, sex, school years, and apoE4 status. Gait speed was associated with logical memory I, semantic fluency, letter fluency, digit symbol, TMT-A, and TMT-B. The participants' HbA1c values were associated with semantic fluency, digit symbol, TMT-A, and TMT-B. We also observed that DM was associated with MMSE, semantic fluency, digit span, digit symbol, TMT-A, and TMT-B.
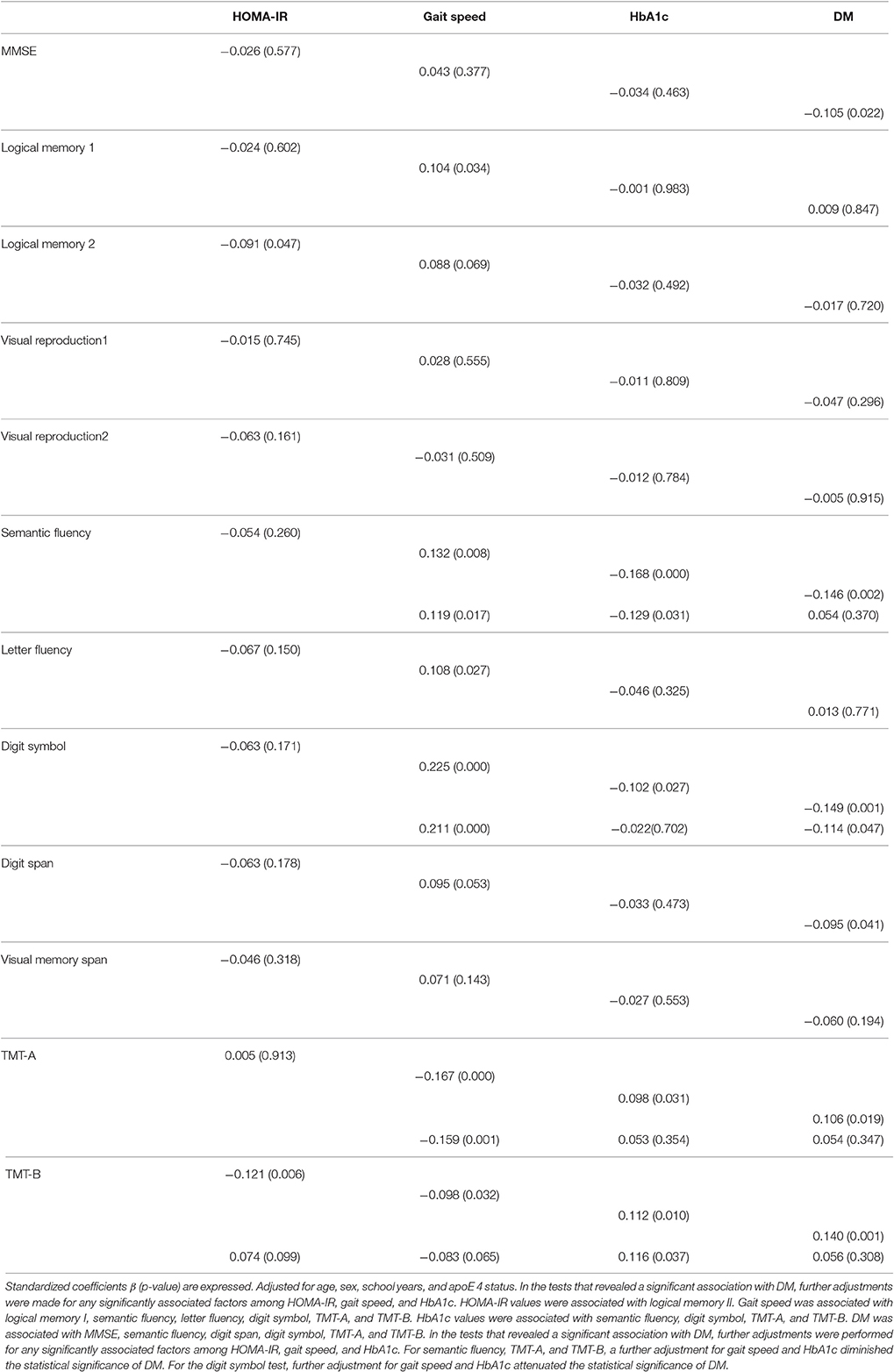
Table 4. Results of a multiple regression analysis of the associations between neurocognitive assessments and HOMA-IR, gait speed, HbA1c, and DM.
In the tests that revealed a significantly association with DM, we performed further adjustment for any factors that were significantly associated with cognition among HOMA-IR, gait speed, and HbA1c. For semantic fluency, TMT-A, and TMT-B, further adjustments for gait speed and HbA1c diminished the statistical significance of DM. For the digit symbol test, further adjustments for gait speed and HbA1c attenuated the statistical significance of DM (Table 4).
Discussion
Our participants with DM had comparable muscle mass but significantly slower gait speed compared to those without DM. Slow gait speed and hyperglycemia have been associated with cognitive dysfunction, mainly in the executive function domain, whereas IR is associated with memory impairment. The participants with DM had lower general cognition and executive function. The executive dysfunction in the DM group seemed to be partly explained by hyperglycemia and/or slow gait speed.
DM has been reported to be a risk factor for lower muscle performance (Volpato et al., 2012; Kalyani et al., 2013). Several mechanisms are involved in DM-related sarcopenia (Umegaki, 2016). Although, muscle mass is not necessarily decreased in DM, muscle performance is impaired in DM (Guerrero et al., 2016). Our present finding that DM subjects had slower gait speed agreed in part with these observations.
In the current study DM subjects had significantly lower scores in general cognition, and in the executive function domain; they also had significantly lower scores in MMSE, semantic fluency, digit span, digit symbol, TMT-A, and TMT-B. It has been established that older individuals with DM have impaired cognition. In addition, a previous meta-analysis showed that executive dysfunction is common among individuals with DM (Palta et al., 2014), and general cognition measured by the MMSE was found to reduced in a DM group compared to the non-DM controls (Mogi et al., 2004).
We also observed that lower gait speed was associated with executive dysfunction. In fact, lower gait speed was associated with lower performance in a wide range of tests that measure executive function. Systematic reviews have confirmed the association between lower gait speed and executive dysfunction (Kearney et al., 2013; Demnitz et al., 2016), although the mechanism underlying this association remains to be elucidated. Because the design of our present investigation was cross-sectional, the cause-result relationship is unclear. However, several possible explanations for this relationship can be suggested. Walking requires complex coordination of the extremities, and thus executive dysfunction may slow the gait speed through impairments of attention, judgment, and/or coordination. Alternatively, impaired gait function may limit social interactions and engagement in leisure activities—both of which changes have been associated with cognitive decline—and it may increase the risk of depression (Shankar et al., 2013). As another possible explanation of the association between lower gait speed and executive dysfunction, recent research has revealed that muscle secretes several factors called myokines. Myokines regulate the lipid and glucose metabolism, and they are associated with insulin resistance through the control of inflammation (Eckardt et al., 2014).
Another hypothesis is that shared causes of brain deficits may induce both executive dysfunction and slow gait speed. Subcortical ischemic vascular diseases are very common in older individuals and have been associated with both executive dysfunction (Jokinen et al., 2009), and slow gait speed (Rosano et al., 2005; Kreisel et al., 2013). A recent study also reported that amyloid beta deposition was associated with slow gait (Nadkarni et al., 2017). Alternatively, inflammation may be a shared pathology in both cognitive and physical dysfunction.
Systemic insulin resistance has been suggested to be associated with brain IR (Kullmann et al., 2012). Therefore, the association between systemic IR and cognitive dysfunction may be due to an impairment of insulin signaling in the brain. Insulin signaling in the brain inhibits glycogen synthase kinase 3 (GSK-3), which phosphorylates tau to induce neurofibrillary tangles (Kandimalla et al., 2016). A deficiency of insulin effects in the brain is thought to accelerate AD pathology. The first cognitive symptom in the disease process of AD is memory loss. The association that we observed between memory decline and IR in the present study's population partially agreed with the hypothesis that IR accelerates AD-related pathology in the brain.
Our study also indicated that HbA1c is associated with the results of several cognitive tests, mainly for executive function. Blood glucose itself may contribute to the cognitive decline in individuals with DM. HbA1c is a marker of the average blood glucose level over a relatively short term, i.e., 1–3 months (Parrinello and Selvin, 2014). High blood glucose may affect cognition relatively quickly. Or, many individuals with DM may tend to have a stable blood glucose status, and HbA1c at a certain point may represent the blood glucose status over longer periods.
As shown in Table 4, DM was associated with impairment in several neuropsychological assessments in the present analyses. However, the statistical significance of the associations between DM and lower scores in cognitive tests were diminished (semantic fluency, TMT-A, TMT-B) or attenuated (Digit symbol) by the adjustments for gait speed and/or HbA1c when these parameters were also associated with cognitions. The cognitive impairment (mainly in executive function) was associated with physical performance or blood glucose levels, and these factors at least partly explained the association between DM and cognitive dysfunction, which may suggest that the underlying mechanisms of DM-related cognitive dysfunction involve these factors—i.e., low physical function measured by slow gait speed and hyperglycemia represented by higher HbA1c.
An interventional study indicated that physical intervention was more effective in individuals with DM compared to non-DM subjects (Espeland et al., 2016). The current study was a baseline analysis of an interventional study which was originally designed to assess the contribution of physical exercise to cognitive preservation with physical exercise. The results regarding the effects of interventions in our cohort will be reported in the near future.
A major limitation of the present study was its cross-sectional design; because of this design, the cause-effect relationships were unclear. In addition, we analyzed the baseline data obtained in a randomized control trial with exercise intervention. Ideally, it would have been helpful to determine whether physical or metabolic improvement by exercise can lead to cognitive improvement. Other limitations of the study include the fact that the diagnosis of DM was based mainly on the participants' self-report. Finally, we did not fully account for medications that could potentially affect IR, since this information was lacking in the baseline database used.
All three factors that were significantly associated with cognitions in the current study—IR, gait speed, and hyperglycemia—are modifiable. Indeed, exercise may improve all three factors. In addition, antidiabetic agents improve hyperglycemia, and some medicines may improve IR. The above three factors were differently associated with different cognitive domains. A comprehensive approach to all three factors is warranted.
In conclusion, we assessed the associations among IR, hyperglycemia, DM, sarcopenia, and cognition. Our results showed that DM subjects had lower cognitive function compared to non-DM subjects. Among the factors assessed in the current study, IR was associated with memory impairment, while slow gait speed and hyperglycemia were associated with a wide-range of cognitive domains, but primarily executive function. The significance of the association between DM and cognitive dysfunction was attenuated upon adjustment for slow gait speed and hyperglycemia, which may suggest the involvement of these factors in the underlying mechanism of DM-related cognitive dysfunction. Further clarification of the mechanisms underlying these associations will contribute to the prevention of cognitive decline in the elderly.
Author Contributions
HU: design of the study, statistical analysis, manuscript writing, TM: design of trial, protocol development, data collection. KU: design of the study, data collection. XC, HS: review of study design and study supervision. TH: choice of measures and creation of intervention program. MK: design of trial, analysis plan, and critical revision of the manuscript. All authors have read and approved the final manuscript.
Conflict of Interest Statement
The TOPICS study is an investigator-initiated study funded by the Toyota Motor Corporation.
The authors declare that the research was conducted in the absence of any commercial or financial relationships that could be construed as a potential conflict of interest.
Acknowledgments
The TOPICS study is supported by the Center of Innovation Program (COI STREAM), the Ministry of Education, Culture, Sports, Science and Technology (MEXT), and the Japan Science and Technology Agency (JST).
References
Aisen, P. S., Petersen, R. C., Donohue, M. C., Gamst, A., Raman, R., Thomas, R. G., et al. (2010). Clinical core of the Alzheimer's disease neuroimaging initiative: progress and plans. Alzheimers Dement. 6, 239–246. doi: 10.1016/j.jalz.2010.03.006
Arai, H., and Satake, S. (2015). English translation of the Kihon Checklist. Geriatr. Gerontol. Int. 15, 518–519. doi: 10.1111/ggi.12397
Bedse, G., Di Domenico, F., Serviddio, G., and Cassano, T. (2015). Aberrant insulin signaling in Alzheimer's disease: current knowledge. Front. Neurosci. 9:204. doi: 10.3389/fnins.2015.00204
Buchner, D. M., Larson, E. B., Wagner, E. H., Koepsell, T. D., and de Lateur, B. J. (1996). Evidence for a non-linear relationship between leg strength and gait speed. Age Ageing 25, 386–391. doi: 10.1093/ageing/25.5.386
Burns, J. M., Johnson, D. K., Watts, A., Swerdlow, R. H., and Brooks, W. M. (2010). Reduced lean mass in early Alzheimer disease and its association with brain atrophy. Arch. Neurol. 67, 428–433. doi: 10.1001/archneurol.2010.38
Cruz-Jentoft, A. J., Baeyens, J. P., Bauer, J. M., Boirie, Y., Cederholm, T., Landi, F., et al. (2010). Sarcopenia: European consensus on definition and diagnosis: report of the European working group on sarcopenia in older people. Age Ageing 39, 412–423. doi: 10.1093/ageing/afq034
Demnitz, N., Esser, P., Dawes, H., Valkanova, V., Johansen-Berg, H., Ebmeier, K. P., et al. (2016). A systematic review and meta-analysis of cross-sectional studies examining the relationship between mobility and cognition in healthy older adults. Gait Posture 50, 164–174. doi: 10.1016/j.gaitpost.2016.08.028
Doi, T., Shimada, H., Makizako, H., Tsutsumimoto, K., Uemura, K., Anan, Y., et al. (2014). Cognitive function and gait speed under normal and dual-task walking among older adults with mild cognitive impairment. BMC Neurol. 14:67. doi: 10.1186/1471-2377-14-67
Eckardt, K., Görgens, S. W., Raschke, S., and Eckel, J. (2014). Myokines in insulin resistance and type 2 diabetes. Diabetologia 57, 1087–1099. doi: 10.1007/s00125-014-3224-x
Ekblad, L. L., Rinne, J. O., Puukka, P. J., Laine, H. K., Ahtiluoto, S. E., Sulkava, R. O., et al. (2015). Insulin resistance is associated with poorer verbal fluency performance in women. Diabetologia 58, 2545–2553. doi: 10.1007/s00125-015-3715-4
Espeland, M. A., Lipska, K., Miller, M. E., Rushing, J., Cohen, R. A., Verghese, J., et al. (2016). Effects of physical activity intervention on physical and cognitive function in sedentary adults with and without diabetes. J. Gerontol. A Biol. Sci. Med. Sci. doi: 10.1093/gerona/glw179. [Epub ahead of print].
Folstein, M. F., Folstein, S. E., and McHugh, P. R. (1975). “Mini-mental state.” A practical method for grading the cognitive state of patients for the clinician. J. Psychiatr. Res. 12, 189–198. doi: 10.1016/0022-3956(75)90026-6
Geijselaers, S. L., Sep, S. J., Stehouwer, C. D., and Biessels, G. J. (2015). Glucose regulation, cognition, and brain MRI in type 2 diabetes: a systematic review. Lancet Diabetes Endocrinol. 3, 75–89. doi: 10.1016/S2213-8587(14)70148-2
Guerrero, N., Bunout, D., Hirsch, S., Barrera, G., Leiva, L., Henríquez, S., et al. (2016). Premature loss of muscle mass and function in type 2 diabetes. Diabetes Res. Clin. Pract. 117, 32–38. doi: 10.1016/j.diabres.2016.04.011
Guo, Q., Zhao, Q., Chen, M., Ding, D., and Hong, Z. (2009). A comparison study of mild cognitive impairment with 3 memory tests among Chinese individuals. Alzheimer Dis. Assoc. Disord. 23, 253–259. doi: 10.1097/WAD.0b013e3181999e92
Guralnik, J. M., Ferrucci, L., Pieper, C. F., Leveille, S. G., Markides, K. S., Ostir, G. V., et al. (2000). Lower extremity function and subsequent disability: consistency across studies, predictive models, and value of gait speed alone compared with the short physical performance battery. J. Gerontol. A Biol. Sci. Med. Sci. 55, M221–M231. doi: 10.1093/gerona/55.4.M221
Jokinen, H., Kalska, H., Ylikoski, R., Madureira, S., Verdelho, A., van der Flier, W. M., et al. (2009). Longitudinal cognitive decline in subcortical ischemic vascular disease—the LADIS Study. Cerebrovasc. Dis. 27, 384–391. doi: 10.1159/000207442
Kalyani, R. R., Tra, Y., Yeh, H. C., Egan, J. M., Ferrucci, L., and Brancati, F. L. (2013). Quadriceps strength, quadriceps power, and gait speed in older U.S. adults with diabetes mellitus: results from the National Health and Nutrition Examination Survey, 1999-2002. J. Am. Geriatr. Soc. 61, 769–775. doi: 10.1111/jgs.12204
Kandimalla, R., Thirumala, V., and Reddy, P. H. (2016). Is Alzheimer's disease a type 3 diabetes? A critical appraisal. Biochim. Biophys. Acta. doi: 10.1016/j.bbadis.2016.08.018. [Epub ahead of print].
Kearney, F. C., Harwood, R. H., Gladman, J. R., Lincoln, N., and Masud, T. (2013). The relationship between executive function and falls and gait abnormalities in older adults: a systematic review. Dement. Geriatr. Cogn. Disord. 36, 20–35. doi: 10.1159/000350031
Koekkoek, P. S., Kappelle, L. J., van den Berg, E., Rutten, G. E., and Biessels, G. J. (2015). Cognitive function in patients with diabetes mellitus: guidance for daily care. Lancet Neurol. 14, 329–340. doi: 10.1016/S1474-4422(14)70249-2
Kreisel, S. H., Blahak, C., Bäzner, H., Inzitari, D., Pantoni, L., Poggesi, A., et al. (2013). Deterioration of gait and balance over time: the effects of age-related white matter change–the LADIS study. Cerebrovasc. Dis. 35, 544–553. doi: 10.1159/000350725
Kullmann, S., Heni, M., Veit, R., Ketterer, C., Schick, F., Häring, H. U., et al. (2012). The obese brain: association of body mass index and insulin sensitivity with resting state network functional connectivity. Hum. Brain Mapp. 33, 1052–1061. doi: 10.1002/hbm.21268
Maki, Y., Ura, C., Yamaguchi, T., Murai, T., Isahai, M., Kaiho, A., et al. (2012). Effects of intervention using a community-based walking program for prevention of mental decline: a randomized controlled trial. J. Am. Geriatr. Soc. 60, 505–510. doi: 10.1111/j.1532-5415.2011.03838.x
Matthews, D. R., Hosker, J. P., Rudenski, A. S., Naylor, B. A., Treacher, D. F., and Turner, R. C. (1985). Homeostasis model assessment: insulin resistance and beta-cell function from fasting plasma glucose and insulin concentrations in man. Diabetologia 28, 412–419. doi: 10.1007/BF00280883
Mogi, N., Umegaki, H., Hattori, A., Maeda, N., Miura, H., Kuzuya, M., et al. (2004). Cognitive function in Japanese elderly with type 2 diabetes mellitus. J. Diabetes Complications 18, 42–46. doi: 10.1016/S1056-8727(03)00078-3
Nadkarni, N. K., Perera, S., Snitz, B. E., Mathis, C. A., Price, J., Williamson, J. D., et al. (2017). Association of brain amyloid-β with slow gait in elderly individuals without dementia: influence of cognition and apolipoprotein E ε4 genotype. JAMA Neurol. 74, 82–90. doi: 10.1001/jamaneurol.2016.3474
Nourhashémi, F., Andrieu, S., Gillette-Guyonnet, S., Reynish, E., Albarède, J. L., Grandjean, H., et al. (2002). Is there a relationship between fat-free soft tissue mass and low cognitive function? Results from a study of 7,105 women. J. Am. Geriatr. Soc. 50, 1796–801. doi: 10.1046/j.1532-5415.2002.50507.x
Palta, P., Schneider, A. L., Biessels, G. J., Touradji, P., and Hill-Briggs, F. (2014). Magnitude of cognitive dysfunction in adults with type 2 diabetes: a meta-analysis of six cognitive domains and the most frequently reported neuropsychological tests within domains. J. Int. Neuropsychol. Soc. 20, 278–291. doi: 10.1017/S1355617713001483
Parrinello, C. M., and Selvin, E. (2014). Beyond HbA1c and glucose: the role of nontraditional glycemic markers in diabetes diagnosis, prognosis, and management. Curr. Diab. Rep. 14:548. doi: 10.1007/s11892-014-0548-3
Rosano, C., Kuller, L. H., Chung, H., Arnold, A. M., Longstreth, W. T. Jr., and Newman, A. B. (2005). Subclinical brain magnetic resonance imaging abnormalities predict physical functional decline in high-functioning older adults. J. Am. Geriatr. Soc. 53, 649–654. doi: 10.1111/j.1532-5415.2005.53214.x
Rosenberg, I. H. (1997). Sarcopenia: origins and clinical relevance. J. Nutr. 127(5 Suppl.), 990S–991S.
Ryder, J. W., Gilbert, M., and Zierath, J. R. (2001). Skeletal muscle and insulin sensitivity: pathophysiological alterations. Front. Biosci. 6, D154–D163. doi: 10.2741/Ryder
Shankar, A., Hamer, M., McMunn, A., and Steptoe, A. (2013). Social isolation and loneliness: relationships with cognitive function during 4 years of follow-up in the English Longitudinal Study of Ageing. Psychosom. Med. 75, 161–170. doi: 10.1097/PSY.0b013e31827f09cd
Spreen, O., and Strauss, E. (1998). A Compendium of Neuropsychological Tests: Administration, Norms and Commentary, 2nd Edn. New York, NY: Oxford University Press.
Umegaki, H. (2014). Type 2 diabetes as a risk factor for cognitive impairment: current insights. Clin. Interv. Aging 9, 1011–1019. doi: 10.2147/CIA.S48926
Umegaki, H. (2016). Sarcopenia and frailty in older patients with diabetes mellitus. Geriatr. Gerontol. Int. 16, 293–299. doi: 10.1111/ggi.12688
Umegaki, H., Kawamura, T., Mogi, N., Umemura, T., Kanai, A., and Sano, T. (2008). Glucose control levels, ischaemic brain lesions, and hyperinsulinaemia were associated with cognitive dysfunction in diabetic elderly. Age Ageing 37, 458–461. doi: 10.1093/ageing/afn051
Volpato, S., Bianchi, L., Lauretani, F., Lauretani, F., Bandinelli, S., Guralnik, J. M., et al. (2012). Role of muscle mass and muscle quality in the association between diabetes and gait speed. Diabetes Care 35, 1672–1679. doi: 10.2337/dc11-2202
Wallace, T. M., Levy, J. C., and Matthews, D. R. (2004). Use and abuse of HOMA modeling. Diabetes Care 27, 1487–1495. doi: 10.2337/diacare.27.6.1487
Wechsler, D. (1987). Wechsler Memory Scale-Revised Manual. San Antonio, TX: The Psychological Corporation.
Wechsler, D. (1997). Wechsler Adult Intelligence Scale-Third Edition. London: The Psychological Corporation Limited.
Keywords: glycihemoglobine, HOMA-IR, gait speed, sarcopenia, executive function, memory
Citation: Umegaki H, Makino T, Uemura K, Shimada H, Hayashi T, Cheng XW and Kuzuya M (2017) The Associations among Insulin Resistance, Hyperglycemia, Physical Performance, Diabetes Mellitus, and Cognitive Function in Relatively Healthy Older Adults with Subtle Cognitive Dysfunction. Front. Aging Neurosci. 9:72. doi: 10.3389/fnagi.2017.00072
Received: 25 November 2016; Accepted: 08 March 2017;
Published: 23 March 2017.
Edited by:
Takeshi Ikeuchi, Niigata University, JapanReviewed by:
Mary Ann Ottinger, University of Houston, USANeha Sehgal, Children's Hospital of Philadelphia, USA
Copyright © 2017 Umegaki, Makino, Uemura, Shimada, Hayashi, Cheng and Kuzuya. This is an open-access article distributed under the terms of the Creative Commons Attribution License (CC BY). The use, distribution or reproduction in other forums is permitted, provided the original author(s) or licensor are credited and that the original publication in this journal is cited, in accordance with accepted academic practice. No use, distribution or reproduction is permitted which does not comply with these terms.
*Correspondence: Hiroyuki Umegaki, dW1lZ2FraUBtZWQubmFnb3lhLXUuYWMuanA=