- 1Life and Health Sciences Research Institute, School of Health Sciences, University of Minho, Braga, Portugal
- 2ICVS/3B’s – PT Government Associate Laboratory, Braga/Guimarães, Portugal
- 3Clinical Academic Center – Braga, Braga, Portugal
Normal brain aging is an inevitable and heterogeneous process characterized by a selective pattern of structural changes. Such heterogeneity arises as a consequence of cumulative effects over the lifespan, including stress and mood effects, which drive different micro- and macro-structural alterations in the brain. Investigating these differences in healthy age-related changes is a major challenge for the comprehension of the cognitive status. Herein we addressed the impact of normal aging, stress, mood, and their interplay in the brain gray and white matter (WM) structure. We showed the critical impact of age in the WM volume and how stress and mood influence brain volumetry across the lifespan. Moreover, we found a more profound effect of the interaction of aging/stress/mood on structures located in the left hemisphere. These findings help to clarify some divergent results associated with the aging decline and to enlighten the association between abnormal volumetric alterations and several states that may lead to psychiatric disorders.
Introduction
Normal brain aging is an inevitable, complex, and heterogeneous process, characterized by a selective pattern of structural and functional changes. With age, the whole brain itself and many of its specific structures present volumetric alterations, mostly reductions, white matter (WM) becomes less dense and loses integrity (Walhovd et al., 2011; Fjell et al., 2013). Specifically, “normal” brain aging has been consistently characterized by a noted overall atrophy associated with a decrease in brain volume and expansion of the cerebrospinal fluid (CSF) spaces (Tamnes et al., 2013). Although total brain volume is more correlated with age after 60 years old, gray matter (GM) volume decline may begin earlier and progress gradually, frequently associated with neuronal cell death, whereas WM may start later and progress more abruptly accompanying the myelin sheath deteriorating after the fourth decade of life (Dennis and Cabeza, 2008; Gunning-Dixon et al., 2009; Lemaitre et al., 2012; Fjell et al., 2013; Tamnes et al., 2013). Specifically, both GM and WM volumetric reductions seem to be greater in the cortex than in subcortical structures, associated to a greater spatial extent in WM, with the highest effects in GM frontal lobe and in the WM superior and medial frontal and anterior cingulate regions (Raz et al., 2005; Dennis and Cabeza, 2008; Gunning-Dixon et al., 2009; Salat et al., 2009b; Voineskos et al., 2010; Sala et al., 2012).
Notably, not only do such changes occur even in highly cognitive functioning individuals (Tamnes et al., 2013; Meunier et al., 2014), but during healthy aging, many domains become also less efficient, and the brain tends to respond to all these neural changes by engaging in continuous reorganizations to keep its homeostatic control and support cognitive functions, the so-termed “brain plasticity” (Park and Reuter-Lorenz, 2009; Lovden et al., 2013). Aging quality varies according to space (brain region), time (lifespan phase), subject (individual parameters), and external influences. Understanding and characterizing the structural brain changes across the lifespan using magnetic resonance imaging (MRI), taking into account the complex combination of distinct life experience, amongst which the exposure to stressful experience and variations in mood are major factors, became one of the most prominent challenges in the comprehension of the cognitive function in middle/late ages.
Despite the limited information on the stress and mood-induced structural alterations, most studies point to reduced volumes in stressed participants in the anterior cingulate cortex, hippocampus, and amygdala (van der Werff et al., 2013; Lucassen et al., 2014). Smaller prefrontal and anterior cingulate cortex volumes have also been observed in patients with major depressive disorder (Frodl et al., 2008) and in participants with prolonged stress (Blix et al., 2013; De Brito et al., 2013). Reduced volumes in the hippocampus, caudate, and putamen have also been reported in depressed subjects (Koolschijn et al., 2009; Kempton et al., 2011; van der Werff et al., 2013). Amygdala alterations were also observed in studies reporting larger volumes related with early stress exposure, including with hemispheric differences (Pruessner et al., 2010; van der Werff et al., 2013). On the other hand, volume decreases in amygdala have also been associated with early and late-onset depressed subjects (Burke et al., 2011; van Uden et al., 2011). Importantly, we previously found in young subjects that stress triggers an atrophy of the caudate and the orbitofrontal cortex, but a hypertrophy of the putamen, changes that were shown to be reversible and accompanied by a functional reorganization after a stress-free period (Soares et al., 2012). Curiously, there is a very limited amount of information regarding the stress and mood-effects on structural aspects on the WM.
Herein we addressed the impact of normal aging, stress, mood and their interplay in the brain GM and WM structure.
Materials and Methods
Ethics Statement
The present study was conducted in accordance with the principles expressed in the Declaration of Helsinki and was approved by the Ethics Committee of Hospital de Braga (Portugal). The study goals and tests were explained to all participants and all gave informed written consent.
Participants, Psychological Tests, and Cortisol Measurements
This study assessed a sample of 104 participants [52 males and 52 females, mean age 65.20 ± 8.07, minimum age 51, and maximum 82, 5.43 ± 3.84 mean years of education and mean of 26.66 ± 3.30 Mini-Mental State Examination (MMSE; Folstein et al., 1975)] selected from a representative sample of the Portuguese population in terms of age, gender, and education, of the SWITCHBOX Consortium project (Santos et al., 2013). Participants responded to a laterality test and to a questionnaire regarding perceived stress (Perceived Stress Scale – PSS; mean 21.49 ± 8.18; Cohen et al., 1983). Participants were further assessed with the Geriatric Depression Scale (GDS, long version; mean 10.91 ± 6.70; Yesavage et al., 1982) by a certified psychologist.
Data Acquisition and Processing
Participants were scanned on a clinical approved Siemens Magnetom Avanto 1.5 T (Siemens Medical Solutions, Erlangen, Germany) at Hospital de Braga using the Siemens 12-channel receive-only head coil. The imaging session included one structural T1 high-resolution anatomical sequence, 3D MPRAGE (magnetization prepared rapid gradient echo). This protocol was performed with the following scan parameters: repetition time (TR) = 2.730 s, echo time (TE) = 3.48 ms, 176 sagittal slices with no gap, flip angle (FA) = 7°, in-plane resolution = 1.0 mm× 1.0 mm and slice thickness = 1.0 mm.
Before any data processing and analysis, all acquisitions were visually inspected by a certified neuroradiologist and confirmed that participants had no brain lesions and the acquisitions were not affected by critical head motion. Seven participants were excluded from the analysis based on the head motion and/or brain lesions.
Structural analysis based on segmentation of brain cortical and subcortical structures from T1 high-resolution anatomical data was performed using the Freesurfer toolkit version 5.1 (https://surfer.nmr.mgh.harvard.edu) running on an Ubuntu 12.04 LTS system. This software package implements a semi-automated segmentation workflow including processing stages such as spatial registration to the Talairach standard space, skull removal, normalization of WM intensity, tessellation of GM-WM segmentation, among others. For the cortical parcellation, two atlases are available: one gyral based atlas resulting in 68 structures (Desikan et al., 2006) and another considering the giral and sulcal parts as separate regions resulting in 148 different brain areas (Destrieux et al., 2010). For the present study the subcortical, WM, and gyral-based cortical segmentations were considered. The employed workflow has suffered several improvements in the past years (Fischl et al., 2002, 2004), is considered reliable across sessions, scanner platforms, updates and field strengths (Han and Fischl, 2007; Jovicich et al., 2009) and was already validated against manual segmentation procedures (Fischl et al., 2002).
Statistical Analyses
Statistical analyses [using the IBM SPSS Statistics software, v.22 (IBM, New York, NY, USA)] were performed with multiple regression models considering each volume as the dependent variable and age, gender, intracranial volume (ICV), PSS, GDS, and age∗PSS, age∗GDS and age∗PSS∗GDS interactions as independent variables. Additionally, in order to test any effect of the MMSE scores in the model, we included MMSE and the interaction MMSE∗GDS as independent variables. For each positive or negative correlation, the results were controlled for the other covariates. The key assumptions for multivariate linear regression analysis were met and the covariates were mean-centered to avoid multicollinearity issues (Aiken and West, 1991; Frazier et al., 2004).
Dissection of the two-way interactions was performed centring the PSS or GDS scores 1 SD below the mean, on the mean and 1 SD above the mean and assessing the age effect on brain volumetry in each model. In order to investigate the significant three-way interactions, the age effect was assessed in four different models: (1) centring both PSS and GDS scores one SD below the mean, (2) with the PSS scores centered one SD above the mean and GDS scores one SD below the mean, (3) PSS scores centered one SD below the mean and GDS one SD above the mean and (4) both variables centered one SD above the mean. Results were considered significant corrected for multiple comparisons using a False Discovery Rate (FDR) threshold of 0.05.
Results
Effect of Age, Stress, and Mood on Brain Volumetry
Volumetric analyses revealed that increased age was positively correlated with the volume of the choroid plexus (both sides), lateral ventricles and third ventricle and WM hypointensities. Most of the age correlations found were negative, including the total GM, cortical WM on both hemispheres, supratentorial volume, left accumbens, and both hippocampi (Table 1). The WM presented more alterations, but only negative correlations were observed with increasing age (Table 2). More specifically, significant volumetric decreases in the WM volume with increasing age were found in the orbitofrontal cortex, superior frontal, inferior and middle temporal, parahippocampal, posterior cingulate, and other frontal, parietal and temporal regions (Table 2).
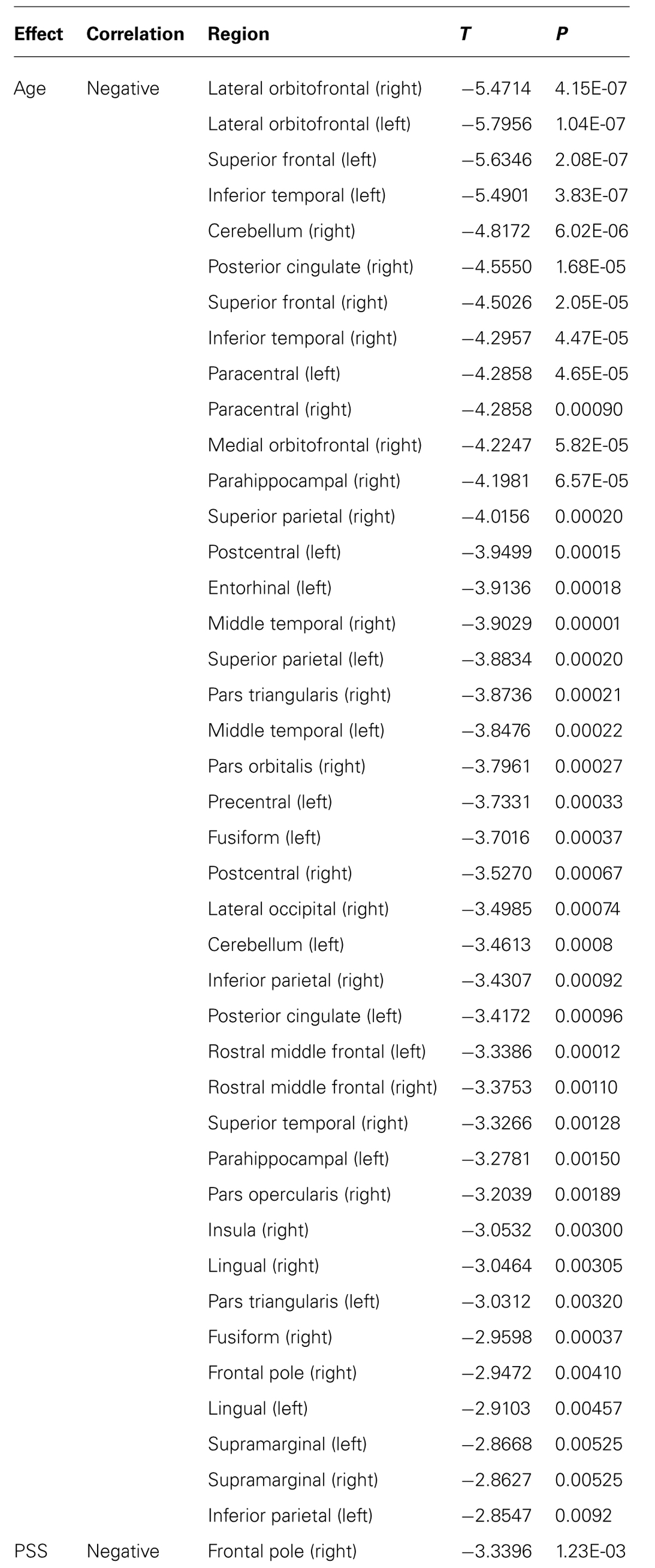
TABLE 2. Effect of age and stress on brain WM regional volumetry (corrected for multiple comparisons FDR 0.05).
The separate impact of stress (PSS score) and mood (GDS score) on brain volumetry did not reveal any significant changes, except for a decreased in WM volumetry in the right frontal pole with lower PSS scores (Table 2). A negative correlation between MMSE and GDS scores was found (p = 0.0002), however, none significant alteration was found when including MMSE and MMSE∗GDS in the model.
Interactions of Age with Stress and Mood on Brain Volumetry
Tests for the two-way interaction between age and PSS revealed a significant interaction with the volume of the left frontal pole (p = 0.0024). Further analysis revealed that for lower PSS scores, decreased volumes were observed with increasing aging; however, as PSS scores get higher the slope of increase with age also increases. In the left temporal pole (p = 0.0061), for low GDS scores, its volume increases with age, while for medium to high GDS scores there is a reduction that gets more pronounced as the scores get higher (Table 3; Figure 1A).

TABLE 3. Effect of the interplay between stress and aging and stress and mood on brain volumetry (corrected for multiple comparisons FDR 0.05).
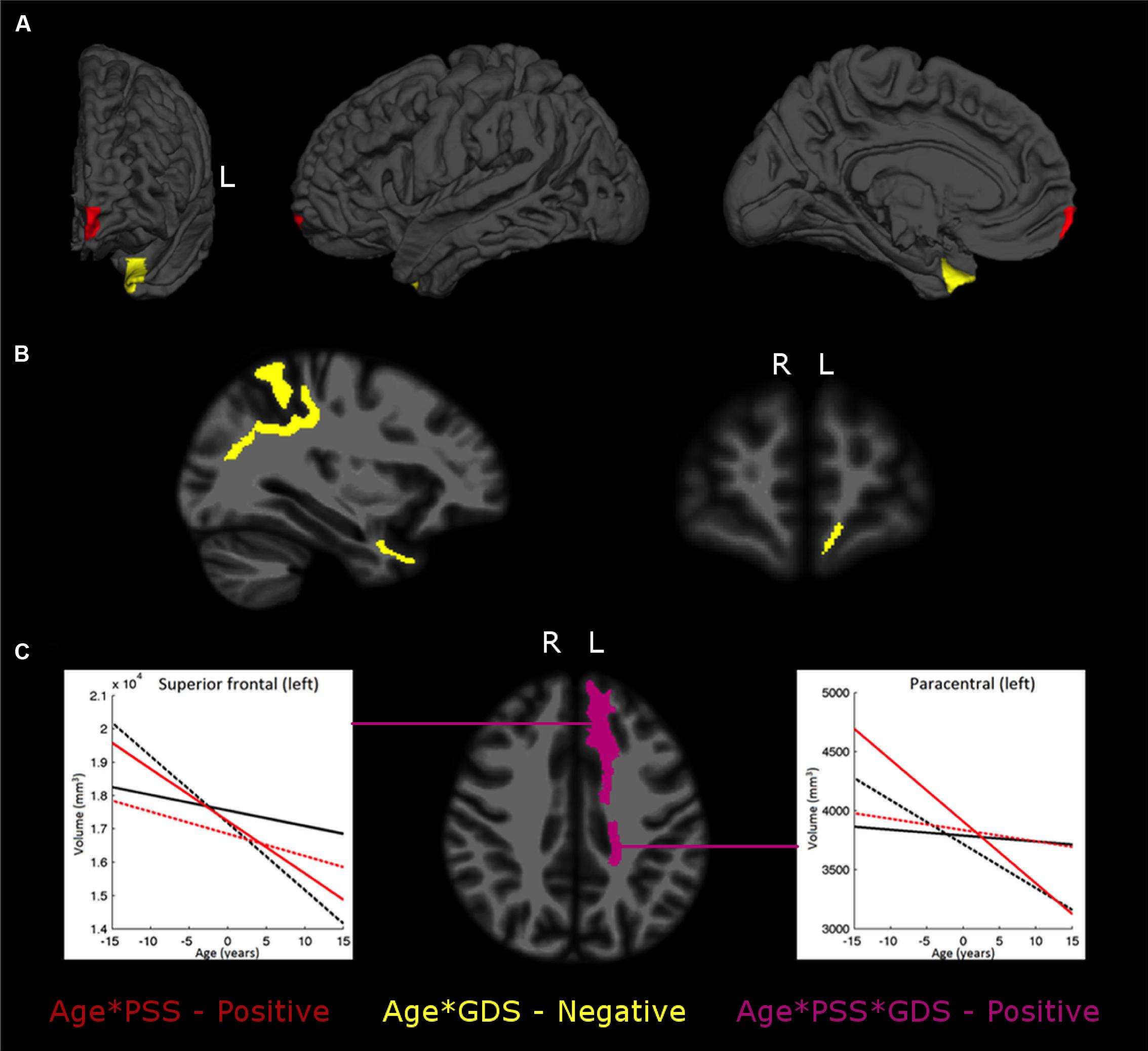
FIGURE 1. The interplay between stress or mood and aging in brain regional gray matter (GM) volumes (A), white matter (WM) regions (B), and the Age*PSS*GDS interactions (C). The images depict areas with significant interaction effects of age*PSS positive (in red), age*GDS negative (in yellow) and age*PSS*GDS positive (in violet). In (C) each graphic line is the regression line between age and regional volume for: Low PSS values (LPSS, black lines), combined with Low GDS (LPSS–LGDS, solid line), or with High GDS (LPSS–HGDS, dotted line); and High PSS value (HPSS, red lines), combined with Low GDS (HPSS–LGDS, solid line) or High GDS (HPSS–HGDS, dotted line).
In the WM regional volumetry, only negative age∗GDS interactions were found (Table 4; Figure 1B). In the left frontal (p = 0.0018) and temporal pole (p = 0.0066), for low GDS scores, the WM volume increases with the age while for medium to high scores the volume decreases and the rate of decrease gets more prominent as the GDS scores get higher. In the left superior parietal (p = 0.0083) the WM volume decreases with age and this decrease gets more pronounced as GDS scores get higher.
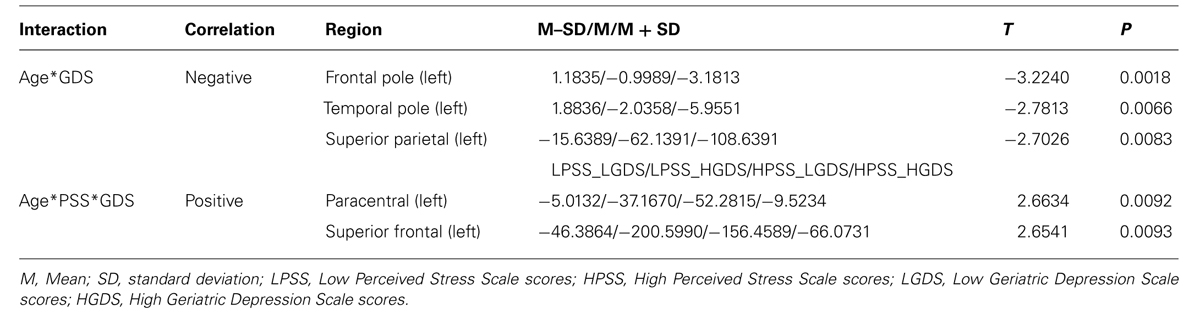
TABLE 4. Effect of the interplay between aging, stress, and mood on brain WM regional volumetry (corrected for multiple comparisons FDR 0.05).
The WM volume of the left paracentral (p = 0.0092) and the left superior frontal (p = 0.0093) regions showed three-way interactions, with both regions evidencing negative correlations between volume and age (Table 4; Figure 1C). Specifically, for low PSS scores, as GDS scores change from low to high the rate of volume decrease with age becomes more pronounced while for high PSS scores, the rate of volume decrease with age becomes less marked as GDS scores change from low to high.
Discussion
Several studies have consistently described the critical impact of the aging process, stress, and mood on brain volumetry. Nevertheless, most of the neuroimaging studies focused on the effect of individual elements, precluding the critical influence of the complex interplay among various processes. In this study, we dissected the influence of life events on brain GM and WM volumetry, namely stress (a more prolonged/chronic stress) and mood, throughout aging, and how they interplay and impact on brain structure.
With aging, we found a global, as well as a regional, pattern of volumetric GM and WM decrease, accompanied by an expansion of the ventricles, choroid plexus and CSF spaces, reflecting an atrophy of the brain parenchyma. Total and subcortical GM was decreased with age, including the left accumbens, both hippocampi and total cortical WM, frontal, temporal, occipital, and parietal WM regions also presented significant volumetric decreases with age. Similar findings have been consistently reported in the literature, both in cross-sectional and longitudinal studies (Raz et al., 2005; Smith et al., 2007; Abe et al., 2008; Fjell et al., 2009; Walhovd et al., 2011; Tamnes et al., 2013). Specifically, however, herein we found a higher decrease in global WM than GM, confirming a striker WM deterioration after the fifth decade (Gunning-Dixon et al., 2009; Lemaitre et al., 2012). This global WM volume decline, more pronounced in frontal regions (orbitofrontal, superior frontal, and rostral middle frontal) was paralleled by an increase with age of the WM hypointensities volume, a measure of lesion burden (Leritz et al., 2014).
It is well known that the stress impact is diverse on different life phases (Sousa and Almeida, 2012). Additionally, stress and mood are states known to be intrinsically connected and that interplay over the lifespan (Calabrese et al., 2009). In this study, and excluding any impact of the MMSE scores, in the volume of the left frontal pole GM, there was an inversion from decreases at low stress levels to increases at high levels with age. This result shows the critical stress impact in the frontal regions, especially at higher stress levels, leading to inversions from age-induced reductions to volume increases during aging (Cerqueira et al., 2007; Lupien et al., 2009). Importantly, behavioral stress affects, with possible reversibility, both structure and function of the prefrontal cortex, a region where neurons become less efficient with aging (McEwen and Morrison, 2013). On the other hand, on the left frontal pole WM, there is an increase in the volume reduction with age for higher depressive mood levels, in line with several previous findings (Konarski et al., 2008; Kong et al., 2014), showing the high susceptibility and variability of this region. Several studies have reported volumetric reductions in temporal regions associated with mood disorders (Drevets et al., 2008; Son et al., 2013) and herein we observed the depressive mood impact, especially at higher levels, in the increased volumetric reduction of the WM and GM in the left temporal pole. In this study we also found an increased volumetric reduction with age at high depressive mood levels in the left superior parietal WM, in line with the literature pointing to WM decreases in older patients with major depressive disorder (Zeng et al., 2012). Such lateralization effect, with more pronounced atrophy in structures localized in the left hemisphere, is in good line with previous studies on this topic (see for review, Cerqueira et al., 2008).
The most affected WM regions during aging by the interplay stress and mood are the left paracentral and the left superior frontal regions. Indeed, the superior frontal WM volume is known to present an accelerated decline with increasing age (Salat et al., 2009a). The volume of these regions decrease for all stress and mood level combinations, however, the decrease is much more pronounced for high stress and low mood levels. This indicates that for better mood (i.e., less depressive), the effect of stress is negative since it increases the negative relation between age and volume; this negative impact of stress does not seem to operate so obviously for subjects with higher depressive mood. These higher volumetric reductions with age, especially under high levels of stress, in WM of these regions, may be associated with the lower predisposition to action and extraception observed with increasing age. Importantly, the impact of stress and mood during the lifespan seems to be higher in the WM compared to the GM volumetry, in line with the described faster WM deterioration after the fifth decade of life (Gunning-Dixon et al., 2009).
This study presents also some important limitations. The analyses of stress and mood states were based only in psychological scales without any biological marker and indicator. Also, our sample included only middle aged to older adults and was a cross-sectional design, precluding a complete lifespan assessment, from childhood to elderly ages, and the evaluation of both individual differences and cohort effects. However, to the best of our knowledge there are no prior reports that evaluate the interplay between stress and mood on brain volumetry across the lifespan.
Conclusion
In this study we have shown the critical influence of stress and mood, especially at higher levels, in brain volumetry. High levels of stress and/or mood may accelerate the typical age-induced decline or alternatively reduce the aging impact. We showed also that for the effects of stress and mood in brain volumetry, timing is crucial. Indeed, the clarification of the stress and mood interplay during aging may help to explain some divergent results associated with the aging decline. Moreover, we expect also to enlighten the association between abnormal volumetric alterations and several states that may lead to psychiatric disorders (Drevets et al., 2008; Konarski et al., 2008; Kempton et al., 2011; Kroes et al., 2011; Durkee et al., 2013; Kuhn and Gallinat, 2013)
Author Contributions
José M. Soares and Paulo Marques contributed in literature search, figures, study design, data collection, data analysis, data interpretation, and writing. Ricardo Magalhães contributed in data collection and data analysis. Nadine C. Santos and Nuno Sousa contributed in study design, data interpretation, and writing.
Conflict of Interest Statement
The reviewer Josef Zihl declares that, despite having collaborated with co-authors Nadine C. Santos and Nuno Sousa, the review process was handled objectively. The authors declare that the research was conducted in the absence of any commercial or financial relationships that could be construed as a potential conflict of interest.
Acknowledgments
We are thankful to all study participants. This work was funded by the European Commission (FP7): “SwitchBox” (Contract HEALTH-F2-2010-259772) and co-financed by the Portuguese North Regional Operational Program (ON.2 – O Novo Norte) under the National Strategic Reference Framework (QREN), through the European Regional Development Fund (FEDER). José M. Soares, Paulo Marques, and Nadine C. Santos are supported by fellowships of the project “SwitchBox”; Ricardo Magalhães is supported by a fellowship from the project FCTANR/NEU-OSD/0258/2012 funded by FCT/MEC (www.fct.pt) and by ON.2 – ONOVONORTE – North Portugal Regional Operational Programme 2007/2013, of the National Strategic Reference Framework (NSRF) 2007/2013, through FEDER.
References
Abe, O., Yamasue, H., Aoki, S., Suga, M., Yamada, H., Kasai, K.,et al. (2008). Aging in the CNS: comparison of gray/white matter volume and diffusion tensor data. Neurobiol. Aging 29, 102–116. doi: 10.1016/j.neurobiolaging.2006.09.003
Aiken, L. S., and West, S. G. (1991). Multiple Regression: Testing and Interpreting Interactions. Newbury Park, CA: Sage.
Blix, E., Perski, A., Berglund, H., and Savic, I. (2013). Long-term occupational stress is associated with regional reductions in brain tissue volumes. PLoS ONE 8:e64065. doi: 10.1371/journal.pone.0064065
Pubmed Abstract | Pubmed Full Text | CrossRef Full Text | Google Scholar
Burke, J., Mcquoid, D. R., Payne, M. E., Steffens, D. C., Krishnan, R. R., and Taylor, W. D. (2011). Amygdala volume in late-life depression: relationship with age of onset. Am. J. Geriatr. Psychiatry 19, 771–776. doi: 10.1097/JGP.0b013e318211069a
Pubmed Abstract | Pubmed Full Text | CrossRef Full Text | Google Scholar
Calabrese, F., Molteni, R., Racagni, G., and Riva, M. A. (2009). Neuronal plasticity: a link between stress and mood disorders. Psychoneuroendocrinology 34(Suppl. 1), S208–S216. doi: 10.1016/j.psyneuen.2009.05.014
Pubmed Abstract | Pubmed Full Text | CrossRef Full Text | Google Scholar
Cerqueira, J. J., Almeida, O. F., and Sousa, N. (2008). The stressed prefrontal cortex. Left? Right! Brain Behav. Immun. 22, 630–638. doi: 10.1016/j.bbi.2008.01.005
Pubmed Abstract | Pubmed Full Text | CrossRef Full Text | Google Scholar
Cerqueira, J. J., Mailliet, F., Almeida, O. F., Jay, T. M., and Sousa, N. (2007). The prefrontal cortex as a key target of the maladaptive response to stress. J. Neurosci. 27, 2781–2787. doi: 10.1523/JNEUROSCI.4372-06.2007
Pubmed Abstract | Pubmed Full Text | CrossRef Full Text | Google Scholar
Cohen, S., Kamarck, T., and Mermelstein, R. (1983). A global measure of perceived stress. J. Health Soc. Behav. 24, 385–396. doi: 10.2307/2136404
De Brito, S. A., Viding, E., Sebastian, C. L., Kelly, P. A., Mechelli, A., Maris, H.,et al. (2013). Reduced orbitofrontal and temporal grey matter in a community sample of maltreated children. J. Child Psychol. Psychiatry 54, 105–112. doi: 10.1111/j.1469-7610.2012.02597.x
Pubmed Abstract | Pubmed Full Text | CrossRef Full Text | Google Scholar
Dennis, A. N., and Cabeza, R. (2008). “Neuroimaging of healthy cognitive aging,” in Handbook of Aging and Cognition, eds F. I. M. Craik and T. A. Salthouse (Mahwah, NJ: Erlbaum).
Desikan, R. S., Segonne, F., Fischl, B., Quinn, B. T., Dickerson, B. C., Blacker, D.,et al. (2006). An automated labeling system for subdividing the human cerebral cortex on MRI scans into gyral based regions of interest. Neuroimage 31, 968–980. doi: 10.1016/j.neuroimage.2006.01.021
Pubmed Abstract | Pubmed Full Text | CrossRef Full Text | Google Scholar
Destrieux, C., Fischl, B., Dale, A., and Halgren, E. (2010). Automatic parcel-lation of human cortical gyri and sulci using standard anatomical nomenclature. Neuroimage 53, 1–15. doi: 10.1016/j.neuroimage.2010.06.010
Pubmed Abstract | Pubmed Full Text | CrossRef Full Text | Google Scholar
Drevets, W. C., Price, J. L., and Furey, M. L. (2008). Brain structural and functional abnormalities in mood disorders: implications for neurocircuitry models of depression. Brain Struct. Funct. 213, 93–118. doi: 10.1007/s00429-008-0189-x
Pubmed Abstract | Pubmed Full Text | CrossRef Full Text | Google Scholar
Durkee, C. A., Sarlls, J. E., Hommer, D. W., and Momenan, R. (2013). White matter microstructure alterations: a study of alcoholics with and without post-traumatic stress disorder. PLoS ONE 8:e80952. doi: 10.1371/journal.pone.0080952
Pubmed Abstract | Pubmed Full Text | CrossRef Full Text | Google Scholar
Fischl, B., Salat, D. H., Busa, E., Albert, M., Dieterich, M., Haselgrove, C.,et al. (2002). Whole brain segmentation: automated labeling of neuroanatomical structures in the human brain. Neuron 33, 341–355. doi: 10.1016/S0896-6273(02)00569-X
Pubmed Abstract | Pubmed Full Text | CrossRef Full Text | Google Scholar
Fischl, B., Salat, D., Van Der Kouwe, A., Makris, N., Ségonne, F., and Dale, A. (2004). Sequence-independent segmentation of magnetic resonance images. Neuroimage 23, S69–S84. doi: 10.1016/j.neuroimage.2004.07.016
Pubmed Abstract | Pubmed Full Text | CrossRef Full Text | Google Scholar
Fjell, A. M., Walhovd, K. B., Fennema-Notestine, C., Mcevoy, L. K., Hagler, D. J., Holland, D.,et al. (2009). One-year brain atrophy evident in healthy aging. J. Neurosci. 29, 15223–15231. doi: 10.1523/JNEUROSCI.3252-09.2009
Pubmed Abstract | Pubmed Full Text | CrossRef Full Text | Google Scholar
Fjell, A. M., Westlye, L. T., Grydeland, H., Amlien, I., Espeseth, T., Reinvang, I.,et al. (2013). Critical ages in the life course of the adult brain: nonlinear subcortical aging. Neurobiol. Aging 34, 2239–2247. doi: 10.1016/j.neurobiolaging.2013.04.006
Pubmed Abstract | Pubmed Full Text | CrossRef Full Text | Google Scholar
Folstein, M. F., Folstein, S. E., and Mchugh, P. R. (1975). “Mini-mental state”. A practical method for grading the cognitive state of patients for the clinician. J. Psychiatr Res. 12, 189–198. doi: 10.1016/0022-3956(75)90026-6
Frazier, P. A., Tix, A. P., and Barron, K. E. (2004). Testing moderator and mediator effects in counseling psychology research. J. Couns. Psychol. 51, 115–134. doi: 10.1037/0022-0167.51.1.115
Frodl, T. S., Koutsouleris, N., Bottlender, R., Born, C., Jager, M., Scupin, I.,et al. (2008). Depression-related variation in brain morphology over 3 years: effects of stress? Arch. Gen. Psychiatry 65, 1156–1165. doi: 10.1001/archpsyc.65.10.1156
Pubmed Abstract | Pubmed Full Text | CrossRef Full Text | Google Scholar
Gunning-Dixon, F. M., Brickman, A. M., Cheng, J. C., and Alexopoulos, G. S. (2009). Aging of cerebral white matter: a review of MRI findings. Int. J. Geriatr. Psychiatry 24, 109–117. doi: 10.1002/gps.2087
Pubmed Abstract | Pubmed Full Text | CrossRef Full Text | Google Scholar
Han, X., and Fischl, B. (2007). Atlas renormalization for improved brain MR image segmentation across scanner platforms. IEEE Trans. Med. Imaging 26, 479–486. doi: 10.1109/TMI.2007.893282
Pubmed Abstract | Pubmed Full Text | CrossRef Full Text | Google Scholar
Jovicich, J., Czanner, S., Han, X., Salat, D., Van Der Kouwe, A., Quinn, B.,et al. (2009). MRI-derived measurements of human subcortical, ventricular and intracranial brain volumes: reliability effects of scan sessions, acquisition sequences, data analyses, scanner upgrade, scanner vendors and field strengths. Neuroimage 46, 177–192. doi: 10.1016/j.neuroimage.2009.02.010
Pubmed Abstract | Pubmed Full Text | CrossRef Full Text | Google Scholar
Kempton, M. J., Salvador, Z., Munafo, M. R., Geddes, J. R., Simmons, A., Frangou, S.,et al. (2011). Structural neuroimaging studies in major depressive disorder. Meta-analysis and comparison with bipolar disorder. Arch. Gen. Psychiatry 68, 675–690. doi: 10.1001/archgenpsychiatry.2011.60
Pubmed Abstract | Pubmed Full Text | CrossRef Full Text | Google Scholar
Konarski, J. Z., Mcintyre, R. S., Kennedy, S. H., Rafi-Tari, S., Soczynska, J. K., and Ketter, T. A. (2008). Volumetric neuroimaging investigations in mood disorders: bipolar disorder versus major depressive disorder. Bipolar Disord. 10, 1–37. doi: 10.1111/j.1399-5618.2008.00435.x
Pubmed Abstract | Pubmed Full Text | CrossRef Full Text | Google Scholar
Kong, L., Wu, F., Tang, Y., Ren, L., Kong, D., Liu, Y.,et al. (2014). Frontal-subcortical volumetric deficits in single episode, medication-naive depressed patients and the effects of 8 weeks fluoxetine treatment: a VBM-DARTEL study. PLoS ONE 9:e79055. doi: 10.1371/journal.pone.0079055
Pubmed Abstract | Pubmed Full Text | CrossRef Full Text | Google Scholar
Koolschijn, P. C., Van Haren, N. E., Lensvelt-Mulders, G. J., Hulshoff Pol, H. E., and Kahn, R. S. (2009). Brain volume abnormalities in major depressive disorder: a meta-analysis of magnetic resonance imaging studies. Hum. Brain Mapp. 30, 3719–3735. doi: 10.1002/hbm.20801
Pubmed Abstract | Pubmed Full Text | CrossRef Full Text | Google Scholar
Kroes, M. C., Rugg, M. D., Whalley, M. G., and Brewin, C. R. (2011). Structural brain abnormalities common to posttraumatic stress disorder and depression. J. Psychiatry Neurosci. 36, 256–265. doi: 10.1503/jpn.100077
Pubmed Abstract | Pubmed Full Text | CrossRef Full Text | Google Scholar
Kuhn, S., and Gallinat, J. (2013). Gray matter correlates of posttraumatic stress disorder: a quantitative meta-analysis. Biol. Psychiatry 73, 70–74. doi: 10.1016/j.biopsych.2012.06.029
Pubmed Abstract | Pubmed Full Text | CrossRef Full Text | Google Scholar
Lemaitre, H., Goldman, A. L., Sambataro, F., Verchinski, B. A., Meyer-Lindenberg, A., Weinberger, D. R.,et al. (2012). Normal age-related brain morphometric changes: nonuniformity across cortical thickness, surface area and gray matter volume? Neurobiol. Aging 33, e1–e9. doi: 10.1016/j.neurobiolaging.2010.07.013
Pubmed Abstract | Pubmed Full Text | CrossRef Full Text | Google Scholar
Leritz, E. C., Shepel, J., Williams, V. J., Lipsitz, L. A., Mcglinchey, R. E., Milberg, W. P.,et al. (2014). Associations between T1 white matter lesion volume and regional white matter microstructure in aging. Hum. Brain Mapp. 35, 1085–1100. doi: 10.1002/hbm.22236
Pubmed Abstract | Pubmed Full Text | CrossRef Full Text | Google Scholar
Lovden, M., Wenger, E., Martensson, J., Lindenberger, U., and Backman, L. (2013). Structural brain plasticity in adult learning and development. Neurosci. Biobehav. Rev. 37, 2296–2310. doi: 10.1016/j.neubiorev.2013.02.014
Pubmed Abstract | Pubmed Full Text | CrossRef Full Text | Google Scholar
Lucassen, P. J., Pruessner, J., Sousa, N., Almeida, O. F., Van Dam, A. M., Rajkowska, G.,et al. (2014). Neuropathology of stress. Acta Neuropathol. 127, 109–135. doi: 10.1007/s00401-013-1223-5
Pubmed Abstract | Pubmed Full Text | CrossRef Full Text | Google Scholar
Lupien, S. J., Mcewen, B. S., Gunnar, M. R., and Heim, C. (2009). Effects of stress throughout the lifespan on the brain, behaviour and cognition. Nat. Rev. Neurosci. 10, 434–445. doi: 10.1038/nrn2639
Pubmed Abstract | Pubmed Full Text | CrossRef Full Text | Google Scholar
McEwen, B. S., and Morrison, J. H. (2013). The brain on stress: vulnerability and plasticity of the prefrontal cortex over the life course. Neuron 79, 16–29. doi: 10.1016/j.neuron.2013.06.028
Pubmed Abstract | Pubmed Full Text | CrossRef Full Text | Google Scholar
Meunier, D., Stamatakis, E. A., and Tyler, L. K. (2014). Age-related functional reorganization, structural changes, and preserved cognition. Neurobiol. Aging 35, 42–54. doi: 10.1016/j.neurobiolaging.2013.07.003
Pubmed Abstract | Pubmed Full Text | CrossRef Full Text | Google Scholar
Park, D. C., and Reuter-Lorenz, P. (2009). The adaptive brain: aging and neurocognitive scaffolding. Annu. Rev. Psychol. 60, 173–196. doi: 10.1146/annurev.psych.59.103006.093656
Pruessner, J. C., Dedovic, K., Pruessner, M., Lord, C., Buss, C., Collins, L.,et al. (2010). Stress regulation in the central nervous system: evidence from structural and functional neuroimaging studies in human populations - 2008 curt richter award winner. Psychoneuroendocrinology 35, 179–191. doi: 10.1016/j.psyneuen.2009.02.016
Pubmed Abstract | Pubmed Full Text | CrossRef Full Text | Google Scholar
Raz, N., Lindenberger, U., Rodrigue, K. M., Kennedy, K. M., Head, D., Williamson, A.,et al. (2005). Regional brain changes in aging healthy adults: general trends, individual differences and modifiers. Cereb. Cortex 15, 1676–1689. doi: 10.1093/cercor/bhi044
Pubmed Abstract | Pubmed Full Text | CrossRef Full Text | Google Scholar
Sala, S., Agosta, F., Pagani, E., Copetti, M., Comi, G., and Filippi, M. (2012). Microstructural changes and atrophy in brain white matter tracts with aging. Neurobiol. Aging 33, 488–498.e2. doi: 10.1016/j.neurobiolaging.2010.04.027
Pubmed Abstract | Pubmed Full Text | CrossRef Full Text | Google Scholar
Salat, D. H., Greve, D. N., Pacheco, J. L., Quinn, B. T., Helmer, K. G., Buckner, R. L.,et al. (2009a). Regional white matter volume differences in nondemented aging and Alzheimer’s disease. Neuroimage 44, 1247–1258. doi: 10.1016/j.neuroimage.2008.10.030
Pubmed Abstract | Pubmed Full Text | CrossRef Full Text | Google Scholar
Salat, D. H., Lee, S. Y., Van Der Kouwe, A. J., Greve, D. N., Fischl, B., and Rosas, H. D. (2009b). Age-associated alterations in cortical gray and white matter signal intensity and gray to white matter contrast. Neuroimage 48, 21–28. doi: 10.1016/j.neuroimage.2009.06.074
Pubmed Abstract | Pubmed Full Text | CrossRef Full Text | Google Scholar
Santos, N. C., Costa, P. S., Cunha, P., Cotter, J., Sampaio, A., Zihl, J.,et al. (2013). Mood is a key determinant of cognitive performance in community-dwelling older adults: a cross-sectional analysis. Age 35, 1983–1993. doi: 10.1007/s11357-012-9482-y
Pubmed Abstract | Pubmed Full Text | CrossRef Full Text | Google Scholar
Smith, C. D., Chebrolu, H., Wekstein, D. R., Schmitt, F. A., and Markesbery, W. R. (2007). Age and gender effects on human brain anatomy: a voxel-based morphometric study in healthy elderly. Neurobiol. Aging 28, 1075–1087. doi: 10.1016/j.neurobiolaging.2006.05.018
Pubmed Abstract | Pubmed Full Text | CrossRef Full Text | Google Scholar
Soares, J. M., Sampaio, A., Ferreira, L. M., Santos, N. C., Marques, F., Palha, J. A.,et al. (2012). Stress-induced changes in human decision-making are reversible. Transl. Psychiatry 2, e131. doi: 10.1038/tp.2012.59
Pubmed Abstract | Pubmed Full Text | CrossRef Full Text | Google Scholar
Son, J. H., Han, D. H., Min, K. J., and Kee, B. S. (2013). Correlation between gray matter volume in the temporal lobe and depressive symptoms in patients with Alzheimer’s disease. Neurosci. Lett. 548, 15–20. doi: 10.1016/j.neulet.2013.05.021
Pubmed Abstract | Pubmed Full Text | CrossRef Full Text | Google Scholar
Sousa, N., and Almeida, O. F. (2012). Disconnection and reconnection: the morphological basis of (mal)adaptation to stress. Trends Neurosci. 35, 742–751. doi: 10.1016/j.tins.2012.08.006
Pubmed Abstract | Pubmed Full Text | CrossRef Full Text | Google Scholar
Tamnes, C. K., Walhovd, K. B., Dale, A. M., Ostby, Y., Grydeland, H., Richardson, G.,et al. (2013). Brain development and aging: overlapping and unique patterns of change. Neuroimage 68, 63–74. doi: 10.1016/j.neuroimage.2012.11.039
Pubmed Abstract | Pubmed Full Text | CrossRef Full Text | Google Scholar
van der Werff, S. J., van den Berg, S. M., Pannekoek, J. N., Elzinga, B. M., and van der Wee, N. J. (2013). Neuroimaging resilience to stress: a review. Front. Behav. Neurosci. 7:39. doi: 10.3389/fnbeh.2013.00039
Pubmed Abstract | Pubmed Full Text | CrossRef Full Text | Google Scholar
van Uden, I. W., van Norden, A. G., de Laat, K. F., van Oudheusden, L. J., Gons, R. A., Tendolkar, I.,et al. (2011). Depressive symptoms and amygdala volume in elderly with cerebral small vessel disease: the RUN DMC study. J. Aging Res. 2011, 647869. doi: 10.4061/2011/647869
Pubmed Abstract | Pubmed Full Text | CrossRef Full Text | Google Scholar
Voineskos, A., Rajji, T., Lobaugh, N., Miranda, D., Shenton, M., Kennedy, J.,et al. (2010). Age-related decline in white matter tract integrity and cognitive performance: a DTI tractography and structural equation modeling study. Neurobiol. Aging 33, 21–34. doi: 10.1016/j.neurobiolaging.2010.02.009
Pubmed Abstract | Pubmed Full Text | CrossRef Full Text | Google Scholar
Walhovd, K. B., Westlye, L. T., Amlien, I., Espeseth, T., Reinvang, I., Raz, N.,et al. (2011). Consistent neuroanatomical age-related volume differences across multiple samples. Neurobiol. Aging 32, 916–932. doi: 10.1016/j.neurobiolaging.2009.05.013
Pubmed Abstract | Pubmed Full Text | CrossRef Full Text | Google Scholar
Yesavage, J. A., Brink, T. L., Rose, T. L., Lum, O., Huang, V., Adey, M.,et al. (1982). Development and validation of a geriatric depression screening scale: a preliminary report. J. Psychiatr. Res. 17, 37–49. doi: 10.1016/0022-3956(82)90033-4
Zeng, L. L., Liu, L., Liu, Y., Shen, H., Li, Y., and Hu, D. (2012). Antidepressant treatment normalizes white matter volume in patients with major depression. PLoS ONE 7:e44248. doi: 10.1371/journal.pone.0044248
Pubmed Abstract | Pubmed Full Text | CrossRef Full Text | Google Scholar
Keywords: aging, stress, mood, volumetry, gray matter, white matter
Citation: Soares JM, Marques P, Magalhães R, Santos NC and Sousa N (2014) Brain structure across the lifespan: the influence of stress and mood. Front. Aging Neurosci. 6:330. doi: 10.3389/fnagi.2014.00330
Received: 14 June 2014; Accepted: 10 November 2014;
Published online: 24 November 2014.
Edited by:
P. Hemachandra Reddy, Texas Tech University, USAReviewed by:
Koteswara Rao Valasani, The University of Kansas, USAJosef Zihl, Ludwig Maximilian University, Germany
Copyright © 2014 Soares, Marques, Magalhães, Santos and Sousa. This is an open-access article distributed under the terms of the Creative Commons Attribution License (CC BY). The use, distribution or reproduction in other forums is permitted, provided the original author(s) or licensor are credited and that the original publication in this journal is cited, in accordance with accepted academic practice. No use, distribution or reproduction is permitted which does not comply with these terms.
*Correspondence: José M. Soares, Life and Health Sciences Research Institute, School of Health Sciences, University of Minho, Campus Gualtar, 4710-057 Braga, Portugal e-mail:am9zZXNvYXJlc0BlY3NhdWRlLnVtaW5oby5wdA==