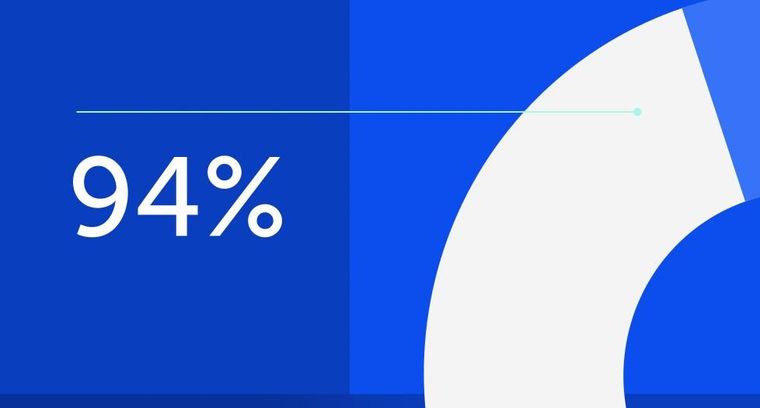
94% of researchers rate our articles as excellent or good
Learn more about the work of our research integrity team to safeguard the quality of each article we publish.
Find out more
ORIGINAL RESEARCH article
Front. Manuf. Technol., 12 July 2022
Sec. Software Technologies
Volume 2 - 2022 | https://doi.org/10.3389/fmtec.2022.921445
This article is part of the Research TopicHorizons in Manufacturing TechnologyView all 5 articles
Nowadays, the diffusion of digital and industry 4.0 (I4.0) technologies is affecting the manufacturing sector with a twofold effect. While on one side it represents the boost fastening the competitive advantage of companies, on the other hand it is often accompanied by several challenges that companies need to face. Among all, companies are required to invest in technologies to empower their production activities on the shopfloor without lagging behind their workforce in order to undertake a linear, aware, and structured path toward digitization. The extant literature presents some research conducted to support companies toward digitization, and they usually rely on maturity models in this intention. Nevertheless, few studies included the assessment of workforce skills and competencies in the overall assessment, and in this case, they provide a high level perspective of the investigation, mainly based on check lists which may limit the objectivity of the assessment, and usually they do not customize the assessment based on companies’ requirements. Therefore, considering the importance to balance investments in technologies with those in the workforce to move toward the same direction, this contribution aims to develop a structured, customizable, and objective skill assessment model. With this intention, it has been first clarified the set of job profiles required in I4.0, together with the needed related skills based on the extant literature findings; second, it has been identified the set of key criteria to be considered while performing the assessment of the workforce; third, it has been defined the method to be integrated in the maturity model to enable the initial setting of the weights of the criteria identified according to the company needs; and fourth, based on these findings, it has been developed the assessment model. The developed model facilitates the elaboration of the proper workforce improvement plans to be put in practice to support the improvement of the skills of the whole workforce based on company’s needs.
Nowadays, the diffusion of digital and industry 4.0 (I4.0) technologies is mainly affecting the manufacturing sector in two ways. On one side it represents the boost fastening the competitive advantage of companies (Gerbert, 2015), and on the other side it is often accompanied by some challenges that companies have to face. Among all, companies are required to invest in technologies to empower their production activities on the shopfloor without lagging behind their workforce in order to undertake a linear, aware, and structured path toward digitization (Oliveira et al., 2019). Indeed, people within the organizations must be kept updated in accordance with the internal technological advancements to ensure that they would be able to work in such an innovative environment (Hecklau et al., 2016; Longo, Nicoletti and Padovano, 2017). The extant literature presents some research that have been conducted in order to support companies toward digitization, and they usually rely on maturity models to support companies in undertaking the path in a structured way [e.g., (Schumacher, Erol and Sihn, 2016; De Carolis et al., 2017; Gökalp, Şener and Eren, 2017)] or organizational models (e.g., (Fantini, Pinzone and Taisch, 2020). Anyway, they tend to neglect the inclusion of the workforce skills in the overall assessment, although human resources and the related investments have been always considered fundamentals to improve organizations’ performances (Becker and Gerhart, 1996; Marimuthu, Arokiasamy and Ismail, 2009). Today, more than ever human workforce needs to be included in the strategic plans of manufacturing companies to keep high the competitive advantage of companies in the fourth industrial revolution (Gehrke et al., 2015; Hecklau et al., 2016). Actually, competency is defined as “any form of knowledge, skill, attitude, ability or learning objective that can be described in a context of learning, education, training or any specific business context” (Różewski and Małachowski, 2009), and thus, this concept is closely related to the learning and training processes associated to business contexts and it takes into account all the skills and capabilities needed for it. On the other side, “competence” has been defined as “the ability to apply knowledge, understanding and skills in performing to the standards required in employment. This includes solving problems and meeting changing demands” (Beaumont, 1996). Therefore, the competences are the ability to apply skills, and in this work it is going to be used the term “skills” as the main one to identify those skills to be owned for certain job positions, and thus to be assessed.
Actually, some efforts have been conducted to clarify what are the required skills to work in a smart and digital factory, even though in many cases the terms “skills” and “competencies” are still used as synonymous. To report some examples, Prifti et al. (2017) investigated the skills required by the employees with high levels of education, while Pinzone et al. (2017) and Acerbi, Assiani and Taisch (2019b) dug deeper in investigating the skills required by the operators and their managers focusing the attention over the operations. Starting from the knowledge about the required skills, which has been updated in this contribution, the workforce’s level in terms of skills should be evaluated to support companies in a structured and comprehensive way (Curtis, Hefley and Miller, 1995). Nevertheless, it not yet present an objective and customizable assessment model that can be easily deployed to the entire workforce enabling to obtain fast and objective results providing suggestions about room for improvement according to the workforce’s current state. Actually, skills are usually studied at a general point of view without entering in detail about the specific analyses dimensions to be investigated to ensure objectivity and completeness of the assessment. Indeed, most of them do not tailor the suggestions on company concrete needs, but they take as reference for improvement the most advanced levels of maturity to direct the improvement plans, such as the model proposed by Acerbi, Assiani and Taisch (2019a).
Therefore, this contribution aims to address this gap, extending the studies already published, by first clarifying which skills are required in discrete manufacturing companies that are undertaking or are willing to start any I4.0 initiative, and second by developing an assessment model to empower manufacturing companies to create a human-centric environment tailoring training activities on their current state. The objective of the research is aimed to be addressed by relying on the extant literature to develop an assessment model enabling to perform a reliable analysis about the workforce’s skill level allowing to make companies implement tailored countermeasures.
The remainder of the study is structured as follows. Section 2 describes the research methodology employed to develop a model covering the limits just identified. Section 3 elucidates the literature review results first about the new job profiles and the specific related skills required by manufacturing companies’ workforce, and second about the key characteristics of the already existing skill assessment models to clarify the structure of the model to be developed. Section 4 describes the assessment model development including the levels and the dimensions and discusses the results from the preliminary application. Last, Section 5 discusses the results and concludes the study by elucidating the main practical and theoretical contributions coming out from this research and the limitations to be addressed in future studies.
The present contribution aims to develop a holistic and prescriptive assessment model enabling to support manufacturing companies in evaluating the current level of their workforce’s skills and competencies to then undertake tailored countermeasures relying on specific training methods. To address this goal, this research employs the Design Science Research (DSR) proposed by Peffers et al. (2006) integrated with the phases proposed by de Bruin et al. (2005), as proposed by and reported in Figure 1. This has been done with the goal to develop an objective and customizable maturity model.
FIGURE 1. Research methodology adapted from Peffers et al. (2006) and de Bruin et al. (2005).
The first phase, “scope”, has been clarified thanks to the extant literature, which additionally supported the definition of the problem to be addressed and the objectives of the solution to be developed. Based on these key elements, it has been set on the ground to design and populate the model, reflecting the “development” phase of the DSR. These phases have been addressed mainly through two separated literature reviews: 1) the first one on new job profiles and skills required in I4.0 context (see Chapter 3.1) and 2) the second one on skill assessment models (see Chapter 3.2).
Based on this knowledge, entering phase 2, an assessment model has been developed aiming at being objective and customizable facilitating the definition of workforce improvement plans. Indeed, on one side, the model has been populated based on the identification of job profiles and related skills required in a smart factory. On the other side, its configuration, in terms of maturity levels, dimensions, and variables to be assessed have been redefined though iterative investigation over the literature about the existing models. Indeed, the review has been extended from maturity models toward different types of assessment models to ensure to grasp the main peculiarities required in evaluating the workforce’s skills. This is the reason why this step allowed to clarify the criteria to be considered in this model and the weights to be given to the different criteria to keep a high customization level. Indeed, thanks to these analyses, it was possible to ensure a reliable assessment based on already developed and validated assessment models, by covering their main limitations.
The third phase, thus model demonstration evaluation and communication, has been conducted based on the interaction with experts in the field, both academic and industrial experts, and an initial industrial application. These external interactions facilitate the iterative improvement of the model and the update of the new set of skills identified by experts. Indeed, by using the model, it is possible to extend the list of skills and job profile by indirectly improving the assessment. Regarding the model validation in terms of robustness, reliability, and usefulness, it is currently mainly limited to the fact that the model has been developed as an extension of already existing and validated assessment models.
In the extant literature, there is some research aiming at classifying the types of skills required in a manufacturing environmental while embracing the I4.0 technologies. Table 1 reports some key classifications previously defined in the extant literature to highlight the main distinctions and needs in the I4.0 context when dealing with competencies and/or skills. Indeed, different types of clusters have been identified in previous researches and this puts the basis for the identification of the dominant ones that thus need to be considered in an assessment model.
As visible from these classifications, not only the technical and hard skills are required in the I4.0 era with the well-known technological advancements but also the soft and social ones are becoming more and more relevant to be empowered and diffused across the entire workforce (Cotet, Balgiu and Zaleschi Negrea, 2017) (Chaka, 2020). Indeed, a transformative path can be undertaken if all these aspects are kept under control.
Actually, the set of skills included in these clusters might be different according to the job profiles and according to the context in which the single company operates. Therefore, a review of the literature has been performed to evaluate, at least from a general point of view, the job profiles required by manufacturing companies in this era together with the Industry 4.0–related skills (both hard and soft skills). The results from the literature are reported in Table 2 as an extension of those identified in Acerbi, Assiani and Taisch (2019b).
Looking at Table 2, as just anticipated, it is evident how important is to back technical and methodological skills with more social and personal skills for all the identified job profiles. Indeed, the two major clusters here envisaged (i.e., hard and soft skills) are going to be employed in the assessment model that will be built in this contribution. More in detail, within the hard or technical skills are mapped also those skills needed to use the digital devices (e.g., a tablet), while regarding the soft skills are mapped those referred for instance to the leadership. In addition, Table 2 makes visible to companies the set of job profiles that should be present in a smart factory to be competitive. This facilitates the selection of the job profiles to be involved in the assessment about the current state of the company in terms of I4.0 skills making immediately evident on one side the lack of a certain set of job profiles inside the company, and on the other side, the set of skills to be assessed to evaluate whether those job profiles already present in the company have these fundamental skills.
In summary, Table 2 represents an updated taxonomy guiding manufacturing companies in understanding the needed new job profiles in an I4.0 context highlighting the related required skills per single job profile. These results are limited to the findings coming from the extant literature, and it is worth to be mentioned that these findings might be tailored based on the sector, country, and needs of each single company whose workforce is assessed to ensure to propose a proper and coherent improvement path.
Although several new job profiles emerged to be required in this era of digitalization and companies are required to keep under control their workforce in this context in the extant literature, there does not yet exists a univocal method for the evaluation of competencies and skills. This is true, especially when dealing with business environments characterized by organizational complexity, as it can be difficult to identify the competencies of people involved in the related processes. Draganidis and Mentzas (2006) developed a model identifying first the skills that the employees need to develop to improve their performances in their current job position or to be prepared for other jobs via promotion or transfer. The structure of the model enables also to perform a skill gap analysis thanks to the possibility to make the comparison between available and needed competencies of individuals or organizations, which facilitates to set the basis for an individual development plan to eliminate the gap. Among all, the most important variables to be considered during the development of a competency model are the use of skills dictionaries, or the creation of customized ones, and the proper use of competency identification, and verification methods which might be surveys, interviews, focus groups, or other (Russo, 2016).
Regarding the manufacturing sector, few works were found in the extant literature. Among all, in 2010, the Employability Skills Assessment Tool for manufacturing industry was developed (M. S. Rasul et al., 2010) with the aim to determine the most rational decisions for the employability of people. The Kepner–Tregoe method has been used to define the level of performance requested to the employees by setting the criteria needed in the decision making, listing the criteria according to the weight factors, calculating the scores for all the criteria, and compare the scores of all options to select the option with the highest score. The aim of this tool was to measure the employability level of an individual before becoming part of the workforce, by assessing those skills which appeared to be fundamental for a certain industry identified thanks to the use of ANOVA. Moreover, in 2016, Hecklau et al. (2016) developed a skill assessment model taking into account the challenges brought by I4.0 and their effects on existing and future jobs. The scale used to evaluate the identified skills is composed by five levels where 1 is nonexistence while 5 represents outstanding peculiarity. To show the skills required by the job position and the skills owned by the worker, a representation based on radar charts is used. Indeed, once the assessment is carried out, by using this visual tool, it is possible to easily identify the critical gaps, and this supports the identification of the ways in which filling these gaps such as the definition of tailored qualification strategies such as trainings and education to build specific competencies.
In addition, many assessment methods have been developed for other fields such as the clinical one. To report some examples, there are performance-based tests proposed by van der Vleuten and Swanson (1990) and the practical examinations proposed in 1975 by the “Objective Structured Clinical Examination” (OSCE) which consisted in a standardized assessment of a person that is required to perform different clinical activities within a predefined timeframe. In the 90’s, Martin et al. (1997) and Reznick et al. (1997) developed the “Objective Structured Assessment of Technical Skills”, also called “OSATS”, which is based on three evaluation method: an operation-specific checklist composed of 20–40 items for which the rater indicates the ones completely and correctly performed by the candidate, a global rating scale composed by seven dimensions evaluated according to a 5-point scale in which extreme and middle points are also described by anchored behaviors tailored on the specific dimension under analysis, and a scoring system based on a pass/fail judgment. In the years, additional studies were conducted. Arora et al. (2011) studied the accuracy of surgeons technical and non-technical skills based on self-assessment, and Sedlack (2010) developed a tool to assess cognitive and motor skills during colonoscopy training called “Mayo Colonoscopy Skills Assessment Tool” (MCSAT)).
As emerged in the previous literature, the soft skills are essential in this path and several methods have been already developed in this regards as visible in Table 3 where those referred to hard skills are very few. More specifically, the analysis reported in Table 3 has the goal to clarify the main characteristics of the models reviewed which necessitate to be employed in the new model willing to be developed in this contribution with the goal to cover both soft and hard skills in an objective but customizable manner. The following pieces of information are gathered:
• Title: It is the title of the tool if it is indicated in the documents; otherwise, we reported the name of the document that illustrates it.
• Type of assessment: The assessment can be carried out by an evaluator or directly by the subject of the evaluation through a self-assessment.
• Scale: It is the typology of scale used for the assessment.
• Skills assessed: It includes the specific skills assessed thanks to the application of the tool or an indication of the skills typology (soft/hard skills) if further details are not available.
• Objective/sector of application: It reports the main scope of the assessment tool and the sector of application to highlight if there are tools developed for the manufacturing sector.
Summing up, although in the extant and dedicated literature several models to assess, both qualitatively and quantitatively, the levels of a competence have been developed, and it has never been formalized a univocal method in this regard, as all the existing models are based on different assumptions depending on the desired purposes. Indeed, as visible in Table 3, and in accordance with what stated by Glass and Metternich (2020), the evaluation of skills can be of different types: “Subjective or objective evaluation, open or closed evaluation, self-evaluation or external evaluation, summative or formative evaluation, direct or indirect evaluation, quantitative or qualitative evaluation”. Table 3 hence elucidates the possible scales that might be used for developing a unique model (i.e., open or closed evaluation), the possibility to create a self-assessment model or a model with a third party evaluator (i.e., self-evaluation or external evaluation), and the type of evaluation to be performed like a multiple choice questionnaire or exercises (i.e., quantitative or qualitative evaluation). Last, Table 3 also reports the type of skills already evaluated by these assessment models which highlight the still open gaps especially reflecting the limited view on both soft and hard skills in manufacturing (while in the medical sector, advancements in this direction have been made). This literature review about skill assessment methods created the basement upon which one can start building the developed model in terms of structure and also content. In particular, regarding the structure, a predominance of qualitative assessment methods was observed, based on self-assessment covering five scales of maturity. Regarding the content, the set of soft skills have been extended taking inspirations from the tool developed, for instance, by Guilland (2017). Even though the purposes of these assessments were different, the common characteristics of the models were employed in the assessment model developed in this contribution since these models were considered already established and validated, and thus, the correct structure for the proper involvement of the audience was already studied and used.
The analysis performed on these assessment models and reported in Table 3 has a high level perspective, thus, to enter more in detail, below two different existing methods developed for the evaluation of skills in different contexts will be displayed to deeper illustrate the key elements characterizing the existing skill assessment models. Indeed, these two models summarize the characteristics that emerged in the extant literature. In general, the first one is the simplest and it is often used in the literature to make a quick assessment, while the second is more articulated and oriented to a structured evaluation of competencies in a business context. To ensure a great level of transparency and completeness, an addition to these models has been made relying on the findings from the gray literature.
The first method is proposed by Mahatmavidya et al. (2018). The purpose of this research was to improve the quality of human resources, in a specific function, by starting from the assessment of workforce’s skills. In this case, data collection came from the interviews conducted with senior employees, who were asked to express an evaluation of the competencies of other employees in a scale 1–5. This model is based on the distinction between tacit and explicit knowledge and on the urge to convert the first into the second one based on the study proposed by Nonaka (2007), which described the following four phases to build up the model:
- Socialization: Competencies are identified and evaluated interviewing the senior employees.
- Externalization: Knowledge about competencies and the interviews’ results are documented.
- Combination: New knowledge is created through the combination of the answers of different responders.
- Internalization: The competence measurement design is shared through the entire department.
The second method, described by Russo (2016), is more structured and detailed, as it addresses directly the evaluation of skills in a business context, recognizing “the important impact that competency management has on improving the overall quality of the final product, and thus on customer satisfaction”.
In this model, each skill is defined through its three dimensions:
- knowledge, everything that can be learned through formative and training courses and involves cognitive processes;
- know-how, related to personal experience and working conditions;
- behavior, about human characters and traits driving someone to react in a certain way under certain circumstances.
According to (Russo, 2016) two different 0–5 scales (0 None, 1 Limited, 2 Basic, 3 Proficient, 4 Advanced, and 5 Expert), both available and required skills are assessed to highlight possible gaps and to make corrective actions to bridge them (Russo, 2016).
Last, as mentioned before, it was also taken inspiration from the gray literature to encounter the manufacturer’s needs. Especially the EBC consulting activities were reviewed. They highlighted the need to build 5-scale maturity model enabling to perform an assessment to evaluate the current gap in accordance to the company’s strategy, and in addition, they also underlined the need to make the assessment customizable and to cover a set of criteria while assessing the skills to facilitate objectivity (Castelli, 2016): 1) knowledge, 2) autonomy, 3) complexity, 4) variability, 5) responsibility, 6) proactivity, and 7) innovation.
All these studies provided a broad perspective over the models designed, without drilling down in assessing specifically the technical and soft skills that the workforce should have and without investigating the different job profiles required in the I4.0 era. Moreover, it was observed a greater attention over the soft skill assessment method rather than those concerning the technical skills. Actually, these studies provide different insights on how to build a complete model enabling the skills assessment, such as the need to create a maturity scale [e.g., (Hecklau et al., 2016)] to individualize the skills gap [e.g., (Bohlouli et al., 2017)], the need to evaluate ad hoc variables and criteria while assessing the level of skills of the workforce [e.g., (Castelli, 2016)], and to ensure an objective and customizable model based on the companies’ strategy [e.g., (Hecklau et al., 2016)].
The goal of this research, as previously mentioned, is to develop a model assessing whether the workforce masters to work in the manufacturing sector embracing the I4.0 paradigm by evaluating their skills level with the intention to structure ad hoc improvement plans to support the enhancement of workforce skills. Therefore, to address this objective, it emerged important to not limit the evaluation based on no/yes answers only, as done in the clinical sector, but rather to clarify the level at which the person is positioned. This level is reflected into the analysis of a series of competencies across maturity levels as proposed in several models (see Table 3). This facilitates the definition of an improvement path tailored on the needs of each specific person. The already developed models are characterized by a set of maturity levels and a set of dimensions of analysis. Looking at the maturity scales, these might be of different types. They differ for both the number of levels and the maturity level description. From this analysis, it was highlighted that the scales go from 3-point to 7-point, and among the 28 methods, for which a scale is indicated, one tool uses a 3-point scale, eight tools use a 4-point scale, 15 tools use a 5-point scale, three tools use a 6-point scale, and one tool use a 7-point scale (see Table 3). The number of levels in a scale is important because it should have the right level of detail. A scale with few levels could be not enough to have the right differentiation between elements ranked within the same class, but on the other hand, a scale with too many levels could create confusion if people are not able to discriminate between the levels (it is difficult to define the class in which categorize the elements because the classes are very similar).
Another important thing emerged from this analysis is the predominance of tools based on self-assessment, also called self-rating, that is used in 22 cases out of 28 (Table 3). Indeed, most of these methods rely on a self-assessment approach which has its own drawback such as the probability that self-reporters try to appear better than what they really are. This happens especially when the assessment is used for the selection of students or workers for the admission to specific courses or during the hiring process. For this reason, a maturity scale should be developed having in mind to cope with the subjectivity issue emerged. In doing that, a normative scale is necessary as proposed by a consultant society (R-Group, 1995) which developed a 7-based scale (i.e., Initial, Minimal, Basic, Adequate, Evolved, Increased, and Maximum) to perform the assessment in a complete and objective way. Moreover, the clarification of the dimensions to be assessed together with the weights, which might be different according to the case/industry under assessment, is another relevant point allowing to build a complete and usable model not only limited to the evaluation of the required skills alone though a checklist that is not valuable for a transformation path.
Last, as developed by Hecklau et al. (2016), it would be beneficial to give back the results based on a radar chart—or similar visual support—which highlights immediately the key potential improvements. Indeed, in this way, it would be easier to explain and visualize the results obtained and identify the gap to be addressed.
The model developed in this contribution relies on the findings gathered from the extant literature reported before. It has been first decided to set the scale of maturity at five levels to ensure to be as much as possible complete but at the same time to avoid unnecessary levels which might become difficult to be analyzed in terms of concrete differences in respect with the other levels. Indeed, keeping as reference the six levels proposed by Russo (2016) and the five levels proposed by Acerbi, Assiani and Taisch (2019a), the maturity scale below was defined proposed ensuring to clearly differentiate each single levels from the others (see Table 4). More specifically, the first level, “basic”, represents the level according to which the person does not possess a certain skill, and the second level, “aware”, represents the level at which the person at least knows he/she should possess a certain skill even though he/she does not yet. Moving to the third level, “practiced”, we have a person that possesses, even though at a very basic level, a certain skill. The fourth level, “competent”, represents the level at which the possession of a skill is great, to finally reach the fifth level at which the person does not only possess a skill, but he/she is also able to teach it to other people.
Considering the importance emerged to define precise criteria for the skills evaluation, the list of criteria proposed in Castelli (2016) were deeply investigated to evaluate their alignment with the model’s goals. Starting with the “knowledge” criteria, which is already part of the definition of a competency (Gelb, 2004), this was considered the main dimension for the analysis reaching the concept of know-how when the knowledge can be transferred to third parties (Howells, 1996). Second, “Autonomy” is intended as one person’s ability to perform an assigned task without any external help or guidance. For this reason, it was taken into account as a dimension of a competency. The third criterion is “Complexity” that is intended as one person’s capability to cope with the intrinsic complexity of a specific task or context. Prins et al. (2008) in their work provide a useful example in this regard: a teacher in primary education can feel highly confident in her ability to control a class in a school of a rural area in a little town, but a definite lack of confidence when she’s supposed to control a class in a school sited in a big city; both contexts ask for similar competencies, but one would agree that the latter context is much more complex, due to the class size or the different social and economic background (Prins et al., 2008). Thus, the intrinsic complexity of a task is dependent on the specific context where it is required. This is the reason why complexity management was taken into account as the third criterion. In the same extent, “Variability” is intended as one person’s capability to cope with the intrinsic variability of a specific task, dealing with the unpredictability and unknown, and it was included as part of the evaluation. The fifth criterion is “Proactivity” that can be defined as the desire to learn everything about the world surrounding especially through experience, thus it is one person’s tendency and inclination to do, driven by interest and willingness to learn (Gelb, 2004), and thus it was included as well. The sixth criterion suggested by Castelli (2016) is “Responsibility”; however, no evidence was found in literature about the importance of responsibility in this regard, so it was not considered a dimension of competency. Conversely, a new criterion arose from the analysis of the literature. As a matter of fact, “commitment to catch and fix problems and to just-in-time operations is key” (Liker and Ogden, 2011) was considered important in this context thus, “commitment”, intended as one person’s capability to be constant and result-driven, especially when dealing with problematic tasks, was taken into account as the sixth criterion for the development of the model. Eventually, the dimension of “innovation” was analyzed. In this regard, a useful insight was given by Peterson (1971), in which innovation is defined as “Intellectual resourcefulness” (Peterson, 1971). Intellectual resourcefulness is given by the combination of capacity or confidence to engage in new learning and creativity in proposing problem solutions. For this reason, innovation was considered the seventh criterion to evaluate a competency in this research.
At the end, once clarified all the criteria required, it was decided to integrate the analysis of the technical skills with these criteria to ensure the highest level of completeness of the model, while keeping separated the soft skills analysis which instead is based only on the five levels of maturity without any specific criteria linked to them.
Once the skills have been identified (see Table 2 and the results from (Acerbi et al., 2019b) together with the criteria, and the maturity levels have been set, it was possible to identify how to weight the criteria to analyze in detail the technical skills according to the context. The proper method has been individualized in the AHP (Analytic Hierarchy Process) which facilitates the choice of weights once ensured that a criterion affects a certain issue for instance thought the ANOVA (Liu, Eckert and Earl, 2020). This method is based on pairwise comparisons among all the different pairs of criteria identified. In this regard, it is asked to the managers involved to provide a grade to indicate the importance of a certain criterion A with respect to another criterion B. This is done for all the pairs of criteria, according to the rating scale shown in Figure 2.
An example displaying the template used and how grades could be given, to understand how weights are calculated, is given in Figure 3.
FIGURE 3. Example displaying a hypothetical scenario to show how to calculate weights for the criteria.
As shown in Figure 3, consistency measures are calculated together with the CR (Consistency Ratio) to check the achievement of coherent results; in this case the green light corresponding to values of CR lower than 0.1, is needed to provide a consistent result. On the other hand, the values corresponding to the column “Average”, represent the weights that, according to the different grades associated to the different comparisons among criteria, are given to each one of them. Thus, in this precise scenario, provided as an example, the ranking of importance, and the correspondent weights for each criterion are illustrated in Figure 4.
FIGURE 4. Ranking and weights of criteria according to grades given in the example scenario considered.
Once the weights for each criterion are calculated through the AHP, the level of a single competency can be found through the Weighted Sum Method, according to the following formula (1):
where
- i, related to the different competencies;
- j, related to the different criteria;
- Pij = value of competency i according to the criterion j;
- pj = weight of criterion j;
- N = total number of criteria.
In this way, the level of the identified skills for each job profile of a manufacturing company can be calculated in a scale from 1 to 5.
Based on this information, it is easy to evaluate the training needs of the person especially thanks to the criteria identified for the technical skills. Indeed, the criteria together with the maturity scale provide a deep and extensive analysis over the skills of the workforce and this allows to identify in a detailed way the type of need each single person has in improving a specific skill. Therefore, these results facilitate managers in setting specific development plans and ad hoc training activities for their workforce, which are based on concrete analysis of the workforce’s current state.
In summary, the evaluation of the workforce’s current state, to evaluate their capacity in operating in a smart and advanced context, needs to include both soft and technical skills across five levels of maturity (i.e., basic, aware, practiced, competent, and proficient). More specifically, the technical skills are required to be evaluated by looking at specific criteria to ensure high level of objectivity and coherence with the industry/case under assessment. The assessment needs to start with the evaluation of the soft skills identified considering their core position in this context. More in detailed, the skills evaluated are the integration of those identified in this contribution (see Table 2) and those identified in Acerbi, Assiani and Taisch (2019b)). Hence, once performed an assessment over the soft skills, framing the personality of a certain person, it is possible to dig deeper in the evaluation of the maturity of the technical or hard skills across the six criteria identified (i.e., knowledge, proactivity, commitment, complexity, autonomy, variability, and innovation). According to the company under assessment, these criteria can be weighted differently based on the opinion of the managers and the CEO, and this has an impact on the final results, thus on the final decisions about the workforce development plan. Indeed, for each skill, each criteria must be set before starting the assessment.
To perform the assessment, of both technical and soft skills, it is asked, to each person, to set his/her level of maturity for each of the competencies identified as relevant for his/her job profile. Indeed, an excel file where the list of skills per job profile is provided, and the self-assessment can be easily performed by each person within the company by replying at which level, according to him/her own perspective, is currently positioned. The standard definition of each level, at each question, facilitates the objectivity of the answers. In addition, to furtherly increase the objectivity of the assessment, the evaluation can be done on a specific person by a set of selected colleagues working usually with him/her and by the manager of his/her division or function. This peer review process might take longer time but ensures an alignment and potential comparison of the results limiting the biases caused by the single person perspective. On the hand instead, it might create competition and frustration among colleagues, and for this reason it is required to evaluate according to the case how to proceed evaluating among all the transparency and loyalty among people. In general, it is suggested to use anonymity in the peer review process to limit the pressure (Panadero and Maryam, 2019).
In all the cases, the excel file is used to report the questions, and this enables to easily link the answers and the weights of the criteria previously decided and set. Based on this model, the analysis of the entire workforce can be conducted, and this enables the creation of tailored development plans and training paths to ensure the alignment and the improvement of each person based on his/her current state assessed. The structure of the model is reported in Figure 5.
The model has been preliminary implemented within a discrete B2B SME Italian company, assessing the traditional manufacturing skills. This application enabled the validation of the structure of the model and it allowed to support the company in setting a proper training path for its workforce in order to align investments in I 4.0 technologies and training activities. The critical factors considered in the validation of the model were mainly two. The first one was referred to the capacity of the model in grasping the key skills to be assessed in accordance with the needs of the single company. The second one, was referred to the capability of the model in supporting the company in undertaking a tailored improvement path. Both the critical factors were properly covered in this initial application. Actually, the company was enthusiast about the possibility to set the criteria as they prefer and choose the skills to be assessed for each profile so that the training was set in accordance to the strategic objectives of the company. This great level of customization was considered a competitive advantage of the model in respect to the assessment models that they previously had used.
The entire model has been shared and discussed with experts in the field which expressed interest and positive feedback about the model. In particular, three industrial companies expressed their interest in a further evaluation of their workforce. The possibility to extend the application in several other industrial cases will facilitate the understanding of key characteristics representing the different industries. Indeed, it would be possible to modify the assessment based on the key profiles of the industries and the required skills to ensure to not be general in the assessment.
The advent of the new and advanced technologies, among which those developed under the I 4.0 paradigm, has twofold implications. On one side, they enabled to improve the competitiveness of several companies, but on the other side, they require to make the workforce be empowered in keeping high and improve their skills level to ensure the proper use of these technologies. Companies are struggling to find this new equilibrium and thus, the scientific world has to support them in this path by formalizing the new competencies required and the update of the job profiles to provide a reference to be followed. In addition, it is required to have a model allowing to assess the skills gap within companies to evaluate whether new required skills can be achieved and how. Therefore, this study contributes firstly in defining the new job profiles and the related required skills by relying on the extant literature extending an already developed study. Secondly, it contributes in the definition of an assessment model facilitating companies in assessing the current skills gap to undertake a structured path toward digitalization, by investigating and analyzing the already developed skill assessment models to develop this one. In this regard, it emerged the need to create a model including both soft and hard skills and reducing as much as possible the subjectivity of the assessment while ensuring a high customization level.
Therefore, the extant literature presents a plethora of skill assessment models especially focused on soft skills, but it lacks a complete assessment model enabling to assess both soft and hard skills by supporting a tailored improvement path according to both the person’s current state in a digital era and the strategic objective of the company. Literature proposed several scales to be used to assess the level of maturity of a certain skill, but this analysis was never backed by the investigation of the level of skills across a set of criteria especially as far as technical skills is concerned, although it emerged essential for a customizable, comprehensive, coherent and accurate analysis. These are the reasons why in the updated model proposed in this contribution these additions were made.
The present study contributes to the scientific literature by covering the preliminary gap identified about the necessity to have an updated view over the job profiles and the related needed skills requested in smart factories employing I4.0 technologies. Also, the study contributes to the development of a comprehensive, customizable and objective assessment model for skills in the I4.0 environment, to support the evaluation of owned skilled vs. desired/needed skills for the company’s strategic goals, to lead toward structured competence development plans within companies. The developed model is entirely based on the extant literature findings, starting from the new taxonomy built in this contribution about the skills and job profiles required in I4.0 era. Previous research proposed a set of skills to be kept under control in this context, but these were mainly linked to the traditional manufacturing job profiles and an updated analysis was required to ensure the reliability of the model here developed. In addition, the model has been implemented within a discrete B2B SME Italian company, assessing the traditional manufacturing skills. Moreover, the whole model has been shared and discussed with experts in the field which expressed interest and positive feedback on the model.
Both the structure and the content of the proposed model enable the deep and comprehensive assessment of the skills related to the just defined I4.0 job profiles allowing to clarify in a detailed way the current state of the workforce to set up tailored development plans and training paths. One of the main practical contribution of this article is to provide indications to companies on the directions they should pursue to first of all obtain higher benefits from I4.0 initiatives, thanks to the formalization of a new set of job profiles that better fit this incoming digital context. Second, to assess how far the extant employees profiles and skills are to reach such skill level and kind, through an ad hoc comprehensive assessment model for gap identification and pattern identification for competence development toward a more effective I4.0 skills establishment. The model facilitates managers to define the proper training path for each operator and employee empowering their strengths and coping with their key weaknesses identified though the assessment.
Some limitations, opening the way for future research, are worth to be reported.
• Although the model facilitates the detailed analysis of each single person inside a smart factory, it does not provide guidelines for specific training activities to be introduced. Indeed, at its current state, the model facilitates the decision maker (e.g., the manager or the CEO), to use in the proper way and with the adequate people the available types of training activities already adopted in their companies. In the future, it is expected to develop a model building customized training paths based to the results of the assessment enabling to make companies think about possible solutions never adopted before.
• The extant literature has been analyzed in a selective way without performing a systematic literature review. In future studies, to ensure to have grasped all the relevant models it might be useful to integrate the findings of this contribution with additional ones.
• The literature field of competence development models is extremely diverse and dispersed. In one side this study tries to bring insights from different theoretic field to learn the principles behind such models while in the other side focuses its analysis on the application field of engineering and digital manufacturing and I4.0. A comprehensive literature analysis from human resource management, psychological might bring additional insights to be further developed.
• Given the highly practical contribution of this work, one of the biggest limitations the authors are already working to overcome, is the validation of the model on the field. While the assessment model has been implemented within a discrete B2B SME Italian company, the model was assessing only traditional manufacturing skill, a complete application of the model toward the new set of I4.0 skills needs to be performed.
The study contributes to literature by providing a complete set of actual I4.0 skills needed to foster and support the fourth industrial revolution as well as to create a new taxonomy of such skills that from now on both academic and industrial world will be dealing with for long time to come. Also, the study contributes to the theoretical field of skill assessment models by introducing a 5-level maturity model, analyzing 2 dimensions of skills (i.e., technical, and soft) within which the technical skills are evaluated through a set of 6 criteria to ensure an objective assessment. This model is able to assess the as-is skill profile of a specific employee, as well as his/her gaps to be filled through competence development activities and training, in a customized and tailored way (thanks to the AHP portion of the model for relative dimension evaluation). The practical contributions of the study are clearly addressing an emerging need of companies, pushed to do the I4.0 leap without proper skills to support that.
The authors hope this work will be beneficial to advance both theoretical and practical discussion on the centrality and importance of people and workforce empowerment within this huge, incumbent, and unstoppable digital evolution that only started with I 4.0 to culminate with Industry 5.0, where people empowerment is the core.
The original contributions presented in the study are included in the article/Supplementary Material; further inquiries can be directed to the corresponding author.
All authors listed have made a substantial, direct, and intellectual contribution to the work and approved it for publication.
The authors declare that the research was conducted in the absence of any commercial or financial relationships that could be construed as a potential conflict of interest.
All claims expressed in this article are solely those of the authors and do not necessarily represent those of their affiliated organizations, or those of the publisher, the editors, and the reviewers. Any product that may be evaluated in this article, or claim that may be made by its manufacturer, is not guaranteed or endorsed by the publisher.
Acerbi, F., Assiani, S., and Taisch, M. (2019a). A Methodology to Assess the Skills for an Industry 4.0 Factory. IFIP International Conference on Advances in Production Management Systems. Springer International Publishing, 520–527. doi:10.1007/978-3-030-29996-5_60
Acerbi, F., Assiani, S., and Taisch, M. (2019b). “A Research on Hard and Soft Skills Required to Operate in a Manufacturing Company Embracing the Industry 4.0 Paradigm,” in XXIV Summer School “Francesco Turco” – Industrial Systems Engineering.
Achcaoucaou, F., Forgas-Coll, S., and Palau-Saumell, R. (2014). “Exploring the Use of an ICT-Based Tool for Assessing Competencies in Postgraduate Students,” in Innovation and Teaching Technologies (Cham: Springer International Publishing), 87–97. doi:10.1007/978-3-319-04825-3_9
Arora, S., Miskovic, D., Hull, L., Moorthy, K., Aggarwal, R., Johannsson, H., et al. (2011). Self vs Expert Assessment of Technical and Non-technical Skills in High Fidelity Simulation. Am. J. Surg. 202 (4), 500–506. doi:10.1016/J.AMJSURG.2011.01.024
Beard, D., Schwieger, D., and Surendran, K. (2008). Integrating Soft Skills Assessment through University, College, and Programmatic Efforts at an AACSB Accredited Institution. J. Inf. Syst. Educ. 19 (2), 229–204.
Beaumont, G. (1996). Review of 100 NVQs and SVQs. MoorfootSheffield UK: Department for Education and Employment. [Preprint].
Becker, B., and Gerhart, B. (1996). The Impact of Human Resource Management on Organizational Performance: Progress and Prospects. Amj 39 (4), 779–801. doi:10.5465/256712
Bharambe, Y., Mored, N., Mulchandani, M., Shankarmani, R., and Shinde, S. G. (2017). “Assessing Employability of Students Using Data Mining Techniques,” in 2017 International Conference on Advances in Computing, Communications and Informatics (ICACCI) (IEEE), 2110–2114. doi:10.1109/ICACCI.2017.8126157
Bohlouli, M., Mittas, N., Kakarontzas, G., Theodosiou, T., Angelis, L., and Fathi, M. (2017). Competence Assessment as an Expert System for Human Resource Management: A Mathematical Approach. Expert Syst. Appl. 70, 83–102. doi:10.1016/J.ESWA.2016.10.046
Bravo-Sanchez, C., Dorazio, V., Denmark, R., Heuer, A. J., and Parrott, J. S. (2018). Development of an Interprofessional Lean Facilitator Assessment Scale. J. Interprofessional Care 32 (3), 370–373. doi:10.1080/13561820.2017.1407300
Castelli, P. (2016). Dal concetto di competenza alla valutazione. EBC Consulting. https://www.bplus.it/dal-concetto-di-competenza-alla-valutazione/
Chaka, C. (2020). Skills, Competencies and Literacies Attributed to 4IR/Industry 4.0: Scoping Review. IFLA J. 46 (4), 369–399. doi:10.1177/0340035219896376
Cimini, C., Boffelli, A., Lagorio, A., Kalchschmidt, M., and Pinto, R. (2020). How Do Industry 4.0 Technologies Influence Organisational Change? an Empirical Analysis of Italian SMEs. Jmtm 32, 695–721. doi:10.1108/JMTM-04-2019-0135
Cotet, G., Balgiu, B., and Zaleschi Negrea, V. (2017). Assessment Procedure for the Soft Skills Requested by Industry 4.0. MATEC Web Conf. 121, 7005. doi:10.1051/matecconf/201712107005
Curtis, B., Hefley, W. E., and Miller, S. (1995). Overview of the People Capability Maturity Model. Pittsburgh, Pennsylvania.
de Bruin, T., Freeze, R., and Kulkarni, U. (2005). Understanding the Main Phases of Developing a Maturity Assessment Model. ACIS 2005 Proceedings [Preprint]. Available at: https://aisel.aisnet.org/acis2005/109 (Accessed December 4, 2020).
De Carolis, A., Macchi, M., Negri, E., and Terzi, S. (2017). “A Maturity Model for Assessing the Digital Readiness of Manufacturing Companies,” in APMS 2017. IFIP Advances in Information and Communication Technology (Springer International Publishing), 513, 13–20. doi:10.1007/978-3-319-66923-6_2
Schlaepfer, R. C., and Koch, M. (2015). Industry 4.0 Challenges and Solutions for the Digital Transformation and Use of Exponential Technologies. Report: Deloitte. Available at: https://www2.deloitte.com/content/dam/Deloitte/ch/Documents/manufacturing/ch-en-manufacturing-industry-4-0-24102014.pdf.
Draganidis, F., and Mentzas, G. (2006). Competency Based Management: a Review of Systems and Approaches. Inf. Manag. Comput. Secur. 14, 51–64. doi:10.1108/09685220610648373
Dragoumanos, S., Kakarountas, A., and Fourou, T. (2017). “Young Technology Entrepreneurship Enhancement Based on an Alternative Approach of Project-Based Learning,” in 2017 IEEE Global Engineering Education Conference (EDUCON) (IEEE), 351–358. doi:10.1109/EDUCON.2017.7942872
Erpenbeck, J. (2007). Handbuch Kompetenzmessung : erkennen, verstehen und bewerten von Kompetenzen in der betrieblichen, pädagogischen und psychologischen Praxis. Schäffer-Poeschel.
Fantini, P., Pinzone, M., and Taisch, M. (2020). Placing the Operator at the Centre of Industry 4.0 Design: Modelling and Assessing Human Activities within Cyber-Physical Systems. Comput. Industrial Eng. 139, 105058. doi:10.1016/j.cie.2018.01.025
Gehrke, L., Kuhn, A., Rule, D., and Moore, P. (2015). Industry 4.0 A Discussion of Qualifications and Skills in the Factory of the Future: A German and American Perspective.
Gelb, M. J. (2004). How to think like Leonardo da Vinci: Seven Steps to Genius Every Day. New York: Delta Trade Paperback.
Gerbert, P. (2015). Industry 4.0: The Future of Productivity and Growth in Manufacturing Industries.
Glass, R., and Metternich, J. (2020). Method to Measure Competencies - A Concept for Development, Design and Validation. Procedia Manuf. 45, 37–42. doi:10.1016/j.promfg.2020.04.056
Gökalp, E., Şener, U., and Eren, P. E. (2017). “Development of an Assessment Model for Industry 4.0: Industry 4.0-MM,” in Software Process Improvement and Capability Determination (Cham: Springer), 128–142. doi:10.1007/978-3-319-67383-7_10
Guilland, A. (2017). SOCCES Handbook - Assessment of Transversal Competences : Focus on Entrepreneurship, Sense of Initiative and Social Skills.
Hecklau, F., Galeitzke, M., Flachs, S., and Kohl, H. (2016). Holistic Approach for Human Resource Management in Industry 4.0. Procedia CIRP 54, 1–6. doi:10.1016/J.PROCIR.2016.05.102
Hening, D. A. (2016). Soft Skills Development of Engineering Students through Mentoring in Cooperative Education.
Howells, J. (1996). Tacit Knowledge. Technol. Analysis Strategic Manag. 8 (2), 91–106. doi:10.1080/09537329608524237
Ingols, C., and Shapiro, M. (2014). Concrete Steps for Assessing the "Soft Skills" in an MBA Program. J. Manag. Educ. 38 (3), 412–435. doi:10.1177/1052562913489029
Jerman, A., Pejić Bach, M., and Aleksić, A. (2019). Transformation towards Smart Factory System: Examining New Job Profiles and Competencies. Syst. Res. Behav. Sci. 37 (2). doi:10.1002/sres.2657
Leitao, P., Geraldes, C. A. S., Fernandes, F. P., and Badikyan, H. (2020). “Analysis of the Workforce Skills for the Factories of the Future,” in Proceedings - 2020 IEEE Conference on Industrial Cyberphysical Systems (ICPS 2020), 353–358. doi:10.1109/ICPS48405.2020.9274757
Liboni, L. B., Cezarino, L. O., Jabbour, C. J. C., Oliveira, B. G., and Stefanelli, N. O. (2019). Smart Industry and the Pathways to HRM 4.0: Implications for SCM. Supp Chain Mnagmnt 24 (1), 124–146. doi:10.1108/SCM-03-2018-0150
Liker, J. K., and Ogden, T. N. (2011). Lessons for Turning Crisis into Opportunity – Toyota under Fire: How Toyota Faced the Challenges of the Recall and the Recession to Come Out Stronger. McGraw-Hill.
Liu, Y., Eckert, C. M., and Earl, C. (2020). A Review of Fuzzy AHP Methods for Decision-Making with Subjective Judgements. Expert Syst. Appl. 161, 113738. doi:10.1016/j.eswa.2020.113738
Longo, F., Nicoletti, L., and Padovano, A. (2017). Smart Operators in Industry 4.0: A Human-Centered Approach to Enhance Operators' Capabilities and Competencies within the New Smart Factory Context. Comput. Industrial Eng. 113, 144–159. doi:10.1016/j.cie.2017.09.016
Mahatmavidya, P. A., Soesanto, R. P., Kurniawati, A., and Andrawina, L. (2018). “Characteristic and Competency Measurement Instrument Development for Maintenance Staff of Mechanical Expertise with SECI Method: A Case of Manufacturing Company,” in IOP Conference Series: Materials Science and Engineering (Institute of Physics Publishing), 012008. doi:10.1088/1757-899X/319/1/012008
Marimuthu, M., Arokiasamy, L., and Ismail, M. (2009). Human Capital Development and its Impact on Firm Performance: Evidence from Developmental Economics. Evidence Developmental Econ. 2.
Martin, J. A., Regehr, G., Reznick, R., Macrae, H., Murnaghan, J., Hutchison, C., et al. (1997). Objective Structured Assessment of Technical Skill (OSATS) for Surgical Residents. Br. J. Surg. 84 (2), 273–278. doi:10.1046/j.1365-2168.1997.02502.x
Matt, D. T., Orzes, G., Rauch, E., and Dallasega, P. (2020). Urban Production - A Socially Sustainable Factory Concept to Overcome Shortcomings of Qualified Workers in Smart SMEs. Comput. Industrial Eng. 139 (December), 105384–105410. doi:10.1016/j.cie.2018.08.035
Mckenzie, D. (2014). Hard Measurement of Soft Skills. Available at: http://blogs.worldbank.org/impactevaluations/hard-measurement-soft-skills.
Mohamed, A., Abdullah, D., and Mohd Dom, T. (2017). Soft Skills of Dental Students' Competence: What Is Important for Patients and How Do Students Fare? World J. Dent. 8 (33), 157–163. doi:10.5005/jp-journals-10015-1431
Müller-Frommeyer, L. C., Aymans, S. C., and Bargmann, C. (2017). Introducing Competency Models as a Tool for Holistic Competency Development in Learning Factories: Challenges, Example and Future Application. Procedia Manuf. 9, 307–314.
Nugent, G., Barker, B., Grandgenett, N., and Adamchuk, V. I. (2010). Impact of Robotics and Geospatial Technology Interventions on Youth STEM Learning and Attitudes. J. Res. Technol. Educ. 42 (4), 391–408. doi:10.1080/15391523.2010.10782557
O'Neill, T. A., Deacon, A., Gibbard, K., Larson, N., Hoffart, G., Smith, J., et al. (2018). Team Dynamics Feedback for Post-secondary Student Learning Teams. Assess. Eval. High. Educ. 43 (4), 571–585. doi:10.1080/02602938.2017.1380161
The Organisation for Economic Co-operation and Development (2009). Take the Test Sample Questions from OECD’s Pisa Assessments Programme for International Student Assessment.
Oliveira, M., Arica, E., Pinzone, M., and Fantini, P. (2019). Human-Centered Manufacturing Challenges Affecting European Industry 4.0 Enabling Technologies. HCI Int. 11786. doi:10.1007/978-3-030-30033-3_39
Ousd (2018). California Healthy Kids Survey/Overview. Available at: https://www.ousd.org/domain/42 (Accessed July 11, 2018).
Panadero, E., and Maryam, A. (2019). An Empirical Review of Anonymity Effects in Peer Assessment, Peer Feedback, Peer Review, Peer Evaluation and Peer Grading. Assessment & Evaluation in Higher Education. [Preprint]. doi:10.1080/02602938.2019.1600186
Peffers, K., Tuunanen, T., Gengler, C. E., Rossi, M., Hui, W., Virtanen, V., et al. (2006). “The Design Science Research Process : A Model for Producing and Presenting Information Systems Research,” in 1st International Conference, DESRIST 2006 Proceedings (Claremont, CA, United States: Claremont Graduate University), 83–106.
Pejic-Bach, M., Bertoncel, T., Meško, M., and Krstić, Ž. (2020). Text Mining of Industry 4.0 Job Advertisements. Int. J. Inf. Manag. 50, 416–431. doi:10.1016/j.ijinfomgt.2019.07.014
Pinzone, M., Fantini, P., Perini, S., Garavaglia, S., Taisch, M., and Miragliotta, G. (2017). “Jobs and Skills in Industry 4.0: An Exploratory Research,” in IFIP: Advances in Production Management Systems. The Path to Intelligent, Collaborative and Sustainable Manufacturing P (Cham: Springer), 282–288. doi:10.1007/978-3-319-66923-6_33
Pontes, J., Geraldes, C. A. S., Fernandes, F. P., Sakurada, L., Rasmussen, A. L., Christiansen, L., et al. (2021). “Relationship between Trends, Job Profiles, Skills and Training Programs in the Factory of the Future,” in Proceedings of the IEEE International Conference on Industrial Technology, 2021-March, 1240–1245. doi:10.1109/ICIT46573.2021.9453584
Prifti, L., Knigge, M., and Kienegger, H. (2017). “A Competency Model for ‘Industrie 4.0’ Employees,,” in in 13th International Conference on Wirtschaftsinformatik, 46–60.
Prins, F. J., Nadolski, R., Berlanga, A., Drachsler, H., Koper, R., Hummel, H., et al. (2008). Competence Description for Personal Recommendations: The Importance of Identifying the Complexity of Learning and Performance Situations. Educ. Technol. Soc. 11, 141–152.
Ras, E., Wild, F., Stahl, C., and Baudet, A. (2017). “Bridging the Skills Gap of Workers in Industry 4.0 by Human Performance Augmentation Tools,” in Challenges and roadmap,” ACM International Conference Proceeding Series, 428–432. doi:10.1145/3056540.3076192
Rasul, M. S., Ismail, Y., Ismail, N., and Rajuddin, M. R. (2010). Development of Employability Skills Assessment Tool for Manufacturing Industry. J. Mek. (30), 48–61. Available at: https://www.researchgate.net/publication/46309154.
Reznick, R., Regehr, G., MacRae, H., Martin, J., and McCulloch, W. (1997). Testing Technical Skill via an Innovative "bench Station" Examination. Am. J. Surg. 173 (3), 226–230. doi:10.1016/S0002-9610(97)89597-9
Różewski, P., and Małachowski, B. (2009). “Competence Management in Knowledge-Based Organisation: Case Study Based on Higher Education Organisation,” in Lecture Notes in Computer Science (Springer), 358–369.
Russo, D. (2016). “Competency Measurement Model,” in Madrid: European Conference on Quality in Official Statistics.
Schumacher, A., Erol, S., and Sihn, W. (2016). A Maturity Model for Assessing Industry 4.0 Readiness and Maturity of Manufacturing Enterprises. Procedia CIRP 52, 161–166. doi:10.1016/J.PROCIR.2016.07.040
Sedlack, R. E. (2010). The Mayo Colonoscopy Skills Assessment Tool: Validation of a Unique Instrument to Assess Colonoscopy Skills in Trainees. Gastrointest. Endosc. 72 (6), 1125–1133. e3. doi:10.1016/j.gie.2010.09.001
Stefl, M. E. (2008). Common Competencies for All Healthcare Managers: the Healthcare Leadership Alliance Model. J. Healthc. Manag. 53 (6), 360–373. doi:10.1097/00115514-200811000-00004
van der Vleuten, C. P. M., and Swanson, D. B. (1990). Assessment of Clinical Skills with Standardized Patients: State of the Art. Teach. Learn. Med. 2 (2), 58–76. doi:10.1080/10401339009539432
Weiszbrod, T. (2015). Health Care Leader Competencies and the Relevance of Emotional Intelligence. Health Care Manag. 34 (2), 140–146. doi:10.1097/HCM.0000000000000060
Keywords: skills, competencies, skill gap, assessment model, industry 4.0, manufacturing
Citation: Acerbi F, Rossi M and Terzi S (2022) Identifying and Assessing the Required I4.0 Skills for Manufacturing Companies’ Workforce. Front. Manuf. Technol. 2:921445. doi: 10.3389/fmtec.2022.921445
Received: 15 April 2022; Accepted: 06 June 2022;
Published: 12 July 2022.
Edited by:
Dimitris Kiritsis, Swiss Federal Institute of Technology Lausanne, SwitzerlandReviewed by:
Joaquín Ordieres Meré, Polytechnic University of Madrid, SpainCopyright © 2022 Acerbi, Rossi and Terzi. This is an open-access article distributed under the terms of the Creative Commons Attribution License (CC BY). The use, distribution or reproduction in other forums is permitted, provided the original author(s) and the copyright owner(s) are credited and that the original publication in this journal is cited, in accordance with accepted academic practice. No use, distribution or reproduction is permitted which does not comply with these terms.
*Correspondence: Monica Rossi, bW9uaWNhLnJvc3NpQHBvbGltaS5pdA==
Disclaimer: All claims expressed in this article are solely those of the authors and do not necessarily represent those of their affiliated organizations, or those of the publisher, the editors and the reviewers. Any product that may be evaluated in this article or claim that may be made by its manufacturer is not guaranteed or endorsed by the publisher.
Research integrity at Frontiers
Learn more about the work of our research integrity team to safeguard the quality of each article we publish.