- 1Luzern University of Applied Science and Art, Horw, Switzerland
- 2Ruhr-Universität Bochum, Bochum, Germany
- 3Glasgow Caledonian University, Glasgow, Scotland
- 4School of Business, Carroll University, Waukesha, WI, United States
Within the smart factory environment, we have a complex system of systems composed of Industry 4.0/digital technologies and assets with varying lifespans. Different degrees of innovation maturity and different lifespans of integrated assets within the industrial internet of things (IIOT) are considered problematic and lead to different perspectives on asset lifecycles as they impact significantly on the asset lifespans. This leads to the loss of a clear end-of-life phase defined in existing Product Lifecycle Management (PLM) models. Through an integrative literature review, this study introduces the concept of nested lifecycles that takes a systems perspective to asset management, and considers subsystems with different lifespans that must be managed holistically by different actors with different perspectives. Additionally, this study provides a framework to derive strategies for lifecycle management by allowing the nested lifecycles to be clearly identified and then addressed. From the theoretical perspective, the notion of nested lifecycles provides a novel viewpoint for the asset management and PLM research community. From the managerial perspective, the proposed framework will help managers identify why and where collaboration between different actors may create difficulties due to their varying outlooks and training.
Introduction
In today’s sustainability debate, and considering the responsibility of manufacturing companies, the view of ‘R-based approaches’ is becoming increasingly established (Bag et al., 2021). Today, these approaches comprise up to 10 principles, ranging from recycle, remanufacture and repair, to rethink, refuse (Potting et al., 2017). In the transformation from linear to circular value creation, these principles are used to utilize materials more sensibly, to extend the lifespan of products, and to manufacture and develop products more intelligently to replace the end-of-life phase “with reducing, alternatively reusing, recycling and recovering materials in production/distribution and consumption processes” (Kichherr et al., 2017). To achieve this, many manufacturing companies aim to leverage Smart Factory approaches (Herrmann et al., 2014). The shift to Smart Factories begins with the digital transformation of “everything related to production systems” (Roblek et al., 2021), starting with the assets themselves.
One promising trend in this digital transformation to Smart Factories is the emergence of Product-Service System (PSS) business models (Tukker and Tischner, 2006), in which product sales and consumption are taking a back seat to service that extends the life of assets (Vendrell-Herrero, 2021). Today, this trend is being reinforced by digitalization and, in many areas, it is the move to digital that makes it possible in the first place (Coreynen et al., 2017). Due to digitalization and the Industrial Internet of Things (IIoT) new complex systems in form of smart PSS (Kuhlenkötter et al., 2017) or smart service systems (Beverungen et al., 2019) emerge. Within such systems, hardware and software components of different actors are integrated into bundled offerings, leading to servitized business ecosystems, where value is co-created (Lightfoot et al., 2013). Especially for suppliers of long-lifespan products, this transformation seems to have a positive impact on firms’ performance (Vendrell-Herero, 2021). In the context of digitalization, the question arises of how to deal with the fact that products with long lifespans are combined with components with short lifespans. This has a considerable influence on PLM, where a systemic view is needed.
The discipline of Systems Engineering is concerned with the integration of discrete systems (Kossiakoff, 2014) to consider the entire system from a holistic point of view, mainly from a technical perspective. Lifecycles in this paper are considered from the systems development perspective, less so for management and control. Lifecycle management mainly focuses on single products or assets, rather than the system from which the products or assets result and are integrated. Further, PSS, according to Tukker (2004), focuses on the offering’s perspective rather than a holistic perspective from the firm regarding and considering not only the offering but also the entire organization’s activities for value creation.
Many researchers have investigated the role of lifecycle management of industrial equipment throughout the manufacturing sectors (Terzi et al., 2010). Product Lifecycle Management (PLM) is defined as a “systematic, controlled concept for managing and developing assets and product-related information”, according to Saaksvuori and Immonen (2005). Other studies (Hamraz, Caldwell and Clarkson, 2013) focus on engineering change management and design version control in use. Cerri and Terzi (2016) proposed a sustainability-focused lifecycle costing model at a system level, and consider the different phases of an asset’s life (e.g., beginning of life, middle of life, and end of life). The operational phase can be further subdivided into more discrete activities: operation, planned and routine maintenance, breakdown and repair, and asset renewal. West and Pascual (2015) advance this and consider the importance of lifecycles for the identification of value-driven service opportunities for sales across different phases of industrial assets’ life.
A distinction should be made between the concepts of asset lifecycle and lifespan. The lifecycle is concerned with the phased aging process of the asset and covers stages from the initial purchase to planned obsolescence (Table 1). By contrast, an asset’s lifespan defines the remaining useful operational life (Yaguo Lei, 2017) of the asset, which is typically considered in financial terms of investment, depreciation, and amortization, and is a key consideration in decisions to replace or refurbish.
Limited research has been conducted on the lifecycle impact of the IIoT when integrated with traditional equipment. Much has been written on the challenges associated with digitalization (Anderson, West and Harrison, 2021) and how to innovate digitally-enabled solutions or Smart Products. However, the conflict in product lifespan (or operational life) expectations (Vendrell-Herrero et al., 2021) stems in part from the different perspectives on PLM. For example, some components within a system may remain operational for decades with ongoing maintenance and repair, and other mechanical systems may need replacement more regularly, whereas some digital components have lifespans of just a few years. Limited research has been conducted on the lifecycle aspects of the entire system comprising many individual assets, in particular the lifespan aspect. Consequently, there are limited practitioner frameworks to operationally manage this beyond the established ISO 55001 standard (Ma, Zhou, and Sheng, 2014), which focusses upon risk mitigation and optimizing asset value at a process level.
The research arises from these apparent gaps in the literature, in particular the impact of such lifecycles as an interconnected system of nested lifecycles, incorporating both traditional and digital technologies, within a multi-actor industrial environment. Each actor has different perceptions of lifespan and asset obsolescence, based upon their intuition, and often without full awareness of adjacent operational functions, or the integrated lifecycles that are captive within the larger interconnected system.
Research question: What are the effects on PLM when bundling assets with diverse operational lifespans in digitally enabled product-service systems?
This study aims to explore this in the lifecycle management of interconnected assets that are typically found within a factory or production facility. In doing so, it will create a framework that can be used to support the long-term sustainability of the operation through a systems-based approach to asset management.
Research Framework and Methodology
An integrative literature review can be used to investigate a research gap and build a framework that can then be tested (Snyder, 2019). The literature that considers the different perspectives of the lifecycle and the implications for management is rather fragmented, although there is a weight of literature that focuses on lifecycle management during the beginning of life, often from a technological perspective (Terzi et al., 2010). The nesting of lifecycles and the inclusion of lifespan appears to be poorly integrated into the existing frameworks. For this reason, an integrative literature review has been chosen as the most appropriate approach, bounded by the operations within a factory, roles of various actors within a factory, and the asset lifespan within a factory, allowing the integration of knowledge from other disciplines (Snyder, 2019). To answer the research question and to address the gaps in the interdisciplinary space, our integrative literature review is divided into three phases to allow us to assess the problems that are faced:
1) The first part of the review is to understand the context of a factory, its purpose and its general operation. The section is relatively concise, nevertheless, it is required to provide the necessary boundary conditions for the following parts of the literature review.
2) The second part was designed to describe the roles of the actors within a factory. The internal actors were described based on Porter’s value chain, as it provides a framework for synthesizing and structuring research from a management perspective (de Mozota, 1998).
3) The third part was designed to identify the different lifespans of equipment for the factory and different subsystems within the factory. From the literature and online databases, a table of asset classes and lifespans has been created.
The final step (presented in Section 4 of this paper) follows on from the three phases of the literature review to build a framework to support the integration of the findings. In this form, researchers and practitioners can understand the difficulties of integrating different asset classes within a smart factory environment.
Integrative Literature Review
This section describes first the factory as a system, then moves to consider asset renewal within a smart factory based on each asset’s anticipated lifespan, before ending with a section on the actors who are active within a smart factory environment.
The Factory as a System
The primary activities of a typical factory mainly relates to the transformation of incoming materials into outbound goods (Porter, 1985). There are also supporting activities, such as quality control and warehousing, that are required to enable the primary activities. Figure 1 provides an overview of a typical production environment based on Porter (1985). According to Benotsmane et al. (2019) a smart factory is one that adapts to changes by integrating data from different sources to enable flexibility to respond to changes in demands. Both views, separated by over 30 years, represent similar views on the purpose of the factory. The use of digital technologies has become embedded within the traditional infrastructure of factories to improve quality and efficiency, and increase speed (Romero et al., 2016). On this basis, the literature has been assessed based upon the value chain, albeit focusing on the activities that are closely related with production, as seen in Table 2. Although the literature often focuses on greenfield Smart Factories, there is a growing appreciation for the evolution of a brownfield factory into a Smart Factory through the integration of new emerging digital technologies as part of Industry 4.0 (Jaspert et al., 2021; Mantravadi et al., 2022).

FIGURE 1. Example of production based on Porter’s value chain (adapted from Porter, 1985).
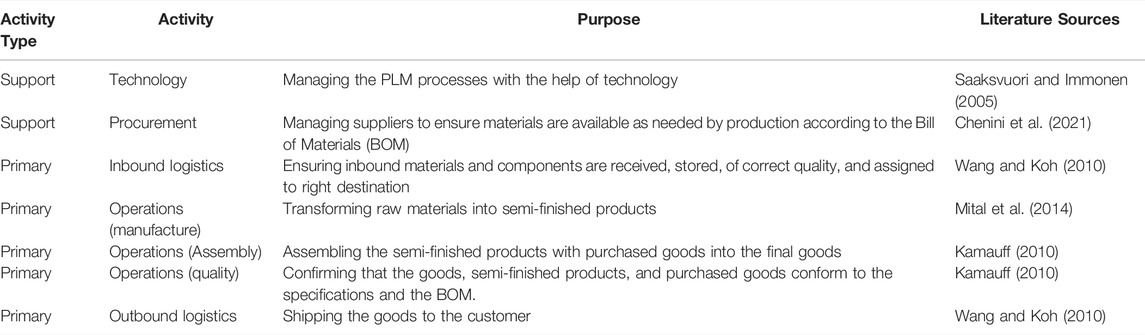
TABLE 2. Literature review of the factory as a system focused on operations (adapted from Porter, 1985).
In the context of research into Industry 4.0 and the implementation of the vision of networked smart factories, Sjödin et al. (2018) have identified different maturity stages for successful implementation of a smart factory. According to the authors, the three principles to advance across the maturity stages include 1) introduction of agile processes, 2) cultivation of digital people and thereby new skills and job profiles, and 3) modular configuration of technologies. The smart factory vision promises to help make processes more efficient, reduce operational costs, maximize quality and safety, and minimize resource use and environmental impact. Within the smart factory, reference models like RAMI 4.0 and IIRA emerged (Resman et al., 2019; Osterrieder, Budde and Friedli, 2020). These models are structured around layers that reach from the physical asset layer to a more abstract application layer, with sensor, connectivity and data layers in between, which can be considered for understanding the value generation in smart manufacturing (Porter and Heppelmann, 2014; Fleisch et al., 2015; Zheng et al., 2019; Jaspert et al., 2021).
Even smaller companies might benefit from implementing a smart factory, especially if they are open to the breadth of technologies and try to incorporate them along the value chain (Büchi, 2020). Examples of enabling technologies include internet of things cloud computing, big data analytics, and augmented reality (Büchi, 2020). These Industry 4.0 technologies can be integrated horizontally and/or vertically: the former for efficiency gains inside the organization, and the latter for easier and better communication with suppliers and customers for enhanced value propositions (Coreynen et al., 2017).
The value proposition is considered the most important building block of business models in the industrial internet of things (Dijkman et al., 2015). Successful companies are demonstrating how a stronger end-customer focus and networking via smart products can build close customer relationships and create service ecosystems that offer business potential (Breschi, 2017). In manufacturing, this type of change is described as servitization, which is being driven by digitalization and is seen as a promising transformation from a product-centric understanding of industry. Nevertheless, the question arises of how to continue to exploit previous competitive advantages. It is likely that tangible goods will continue to be a component in offerings (Porter and Heppelmann 2015). Hybrid service bundles tend to better meet customer needs and create competitive advantages (Anderson, West and Harrison, 2021). Product development will therefore remain an important pillar. To this end, it is also important to align production sites in the best possible way for the transformation towards hybrid offerings. The factory environment comprises numerous key components related to process automation, such as telemetry sensors, programmable logic controllers (PLC), Supervisory control and data acquisition (SCADA), Human-machine interfaces (HMI), and Distributed control systems (DCS). The integration of new digital technologies into these traditional infrastructure components is described in Table 3, based on the ANSI/ISA-95 standards (ANSI, 2008).
Roles Within a Smart Factory Environment
Smart factories and Industry 4.0 have brought new and changed job profiles and competencies into manufacturing industry (Pejic-Bach, 2020). These can be conceptualized as roles that are understood as “distinct technologically separable, value-added activities undertaken by firms or individuals” (Kambil and Short, 1994, p. 10). In multi-actor settings, actors can take on different roles depending on the situation, which is why a differentiation of roles, instead of actors is used in this paper (Ekman et al., 2016; Anke et al., 2020). The roles within the smart factory environment have been structured based on Porter’s value chain (Porter, 1985) and are described in Table 4 for the design and development phase and Table 5 for the operational phase of the PLM. The descriptions that each role takes, and their outlook, has been based on the literature. The outlook has been established from the breakdown between operational, tactical, and strategic thinking in a manufacturing environment according to Momme (2002), as this provides insights into their approach towards their role.
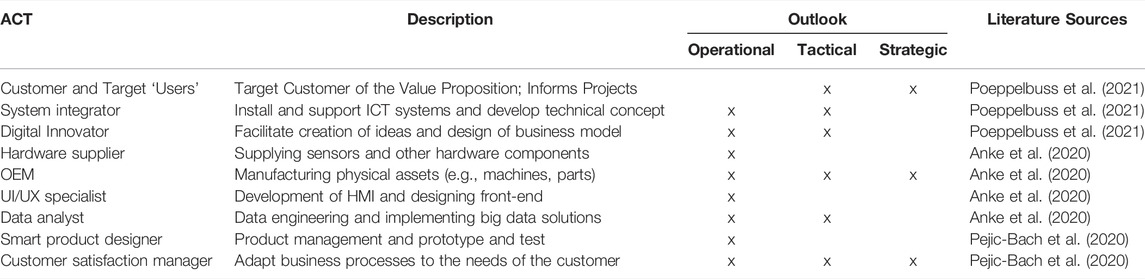
TABLE 4. Typical roles within the design and development phase (beginning of life) focused departments.
On reviewing the different roles, clearly defined ‘tribes’ of stakeholders emerge, arising from their different backgrounds and outlooks. Firstly the ‘Traditional Production’ tribe, such stakeholders are found mainly in sectors like engineering and manufacturing that follow more conservative stage-gate thinking with emphasis on iterative changes. Then the ‘Disruptive tribe’, these people are found mainly in information technology and internet-based organizations. They follow management approaches such as agile development, with an emphasis on incremental innovation with shorter change cycles via minimum viable products, resulting in a more cyclical and collaborative approach, which is more conducive to innovation. Meanwhile, traditional tribes follow waterfall and stage-gate methods, where change is addressed in more sequential steps. With regards to their respective experience, manufacturing heavy industries in physics, engineering, and other physical sciences dominate traditional stakeholders. Strategic long-term thinking is vital in this field. Meanwhile, disruptive stakeholders are in the information technology and digital sectors, where regulations or corporate governance rules are still nascent and some risk can be taken. Traditional stakeholders and their organizations deal with long lead times, strong intellectual property protection with extended quality analysis and release cycles. Meanwhile, disruptive industries follow a more agile, open-source approach, with a “perpetual beta” and fast corrective patching mindset. These general differences across stakeholders are a key consideration for lifecycle management, as this mismatch (and sometimes lack of awareness) of different intuitive perspectives can cause operational issues.
Asset Renewal Within a Factory
Asset renewal is the replacement (or refurbishment) of an existing asset or component with a new asset (e.g., new machine) capable of delivering the same level of service as the existing asset (NAMS Group, 2006). The asset renewal process should, in general terms, be closely related to the asset depreciation (Vangermeersch, 1971; Sigidov et al., 2016) and maintenance strategies (Kelly, 2006). The rate of asset depreciation should match the cost of asset renewal in the medium term to ensure the same level of performance from the system. The asset depreciation should be based on the estimate of useful life (or the asset’s lifespan). The asset depreciation period may be considered within an accounting environment and provides the framework for asset renewal within a factory (or a smart factory). The rate of depreciation can be impacted by the component’s lifespan and the maintenance strategy applied (i.e., preventative or predictive, repair or replace etc.). Within asset renewal the concept of obsolescence needs to be integrated, as obsolescence is often closely associated with innovation and technology maturity (Burns, 2010).
Besides mechanical asset renewal, the establishment of smart products creates the possibility of post-production reconfiguration (Abramovici et al., 2017). Products that have been delivered to a customer can be modified through smart retrofitting (Jaspert et al., 2021), but also through the continuous adaptation of software modules (Tomiyama et al., 2019), and ongoing ‘over the air’ updates to firmware (Nilsson and Larson, 2008). Such integrated views of asset renewal and reconfiguration enables new value creation in greenfield and brownfield factories. Understanding the smart factory or smart service systems as changing systems—whose use phase in the life cycle can be extended continuously through constant updates and reconfiguration—can have a significant impact on the 10Rs (Kirchherr et al., 2017) in the context of the circular economy. The increased integration of various asset classes, from long-lived mechanical components to short-lived software products, results in a complex network that must be managed. Figure 2 shows the different reconfiguration options with asset lifespans. It is notable that with a shift to newer digital technologies, there is a corresponding decrease in the lifespan, becoming more pronounced as the technology separates from the traditional production environment.
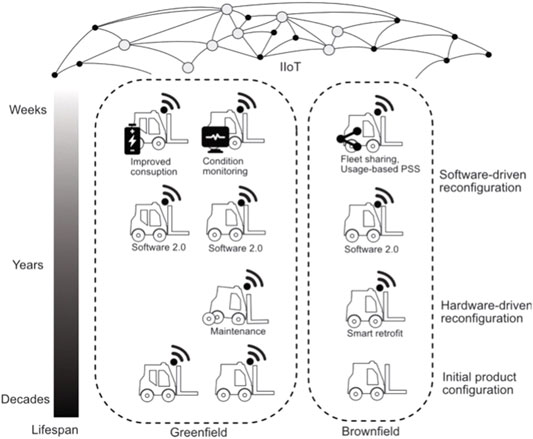
FIGURE 2. Reconfiguration of smart products and asset lifespan before renewal (based on Tomiyama et al., 2019).
As such, Table 6 provides an overview of different asset classes within a smart factory environment based on the literature and on a Japanese government database (NIES, n.d.). To distinguish asset classes, we used the layers introduced in section 3.1.
A Systems-Based Framework for Asset Life Management
Our contribution covers various facets of asset management in the implementation of smart products and product-service systems within the smart factory setting. As a contribution to research, the main areas considered were: asset lifecycle management, product lifecycle design, and the new role of the nested lifecycle manager.
Asset lifecycle management gives a detailed perspective on the lifecycles of different technologies and how they are related to innovation cycles. Assets with different innovation cycles (fast vs mature) should be considered distinctly in asset and lifecycle management and require different tools and processes. For the installed base, a field that often receives little attention, we provide a new perspective that enables further research, less oriented to OEM considerations but with a focus on the operational phase. In general, life cycles and lifespan are a poorly considered field of research. As other researchers from related fields have noted, this perspective can provide exciting insights that may be helpful in explaining existing challenges in the context of Industry 4.0 (Vendrell-Herrero et al., 2021).
A systems-based approach requires us to first consider the entire system by describing the boundary conditions before considering the subsystems (Blanchard, Fabrycky and Fabrycky, 1990). Within such a system it is then possible to consider the lifespan incongruences that have been identified in Section 3, around which it is now possible to consider the actors and their expectations that come from their individual roles. These shed light on the paradox that competing lifespans can provide when considering asset life management in a smart factory environment.
In asset life management within the smart factory, responsible personnel should ensure that the products are suitably designed (Bakker, et al., 2014). According to Bakker, et al. (2014), products with short innovation cycles and immature technologies should be as easy to replace (and recycle) as possible. Plant components with long lifespans and mature technologies, on the other hand, should be designed for a long service life, with minimal use of materials, and be easy to maintain. The findings are summarized in Table 7. However, as described in Table 6, there are (sub) system components, e.g., bearings, seals, and car tires, that are considered consumable in nature, even though the technology may be considered mature.
Within the context of systems engineering (supporting with system boundaries) and the asset life management of the smart factory, the development of a framework becomes clearer when considering the literature findings. The first aspect is that the smart factory is a complex system that consists of many different actors with different perspectives, based on their individual and collective contexts. Here there is a clear difference when we consider a greenfield or a brownfield smart factory. The purpose of the factory remains to produce products efficiently, however over its lifespan the production will vary based on market, technology, and management changes (Lei, 2017; Mantravadi et al., 2022; Benotsmane et al., 2019).
The lifespan of a product or asset describes the expected duration of the object’s performance as planned (Vendrell-Herrero, 2021). In comparison, the lifecycle describes the lifespan in more detail, including the stages beginning-of-life (BOL), middle-of-life (MOL), and end-of-life (EOL); this concept provides the option of iteration, meaning that EOL activities can lead to a new BOL phase. Therefore, lifecycle management can be described as an activity to either reach or exceed the expected lifespan of a product or an asset. When considering or defining a system with many individual components and subsystems, then lifecycle management activities on the individual asset level, the application allows the lifespan concept to be applied. The application of either the lifecycle or lifespan concept can depend on the technological maturity of the component or the relative importance of the component within the system for value creation or destruction. The approach taken for the maintenance and asset management of complex systems is described by Kelly (2006), where the trade-offs between different approaches and the need to focus on the system and subsystems is considered. Also, it is clear that the BOL lifespan assumptions are design assumptions and that during the MOL phase these may need to be adapted. This approach to systems management also considers the obsolescence aspects, e.g., when a supplier stops producing a subsystem component and a firm needs to deal with the challenges it creates for long-term asset management.
The concept of digitally-enabled PSS can be applied to a smart factory because such a factory has all the main components related to such PSS, which are products, services, and digital technologies interacting with the purpose of value creation. Such a digitally-enabled PSS can become very complex, having many actors and products with different lifespans. The challenge of such a system orchestrator can be first, modelling the system and identifying system drivers for value creation and destruction. Second, establishing appropriate metrics for decision making from a systems perspective, and third, continuously evolving and adapting the system. The lifecycle management framework proposed in this paper aims to address the challenges stemming from continuously and sustainably evolving the digitally enabled product-service system.
There is clear interaction between the actors, and the various roles that they take, and the smart factory; yet each has different perspectives and different time horizons that they consider. This has been seen by considering the three horizons of operational, tactical, and strategic decision-making according to Momme (2002). Overlying this are the individual norms from each profession, which are not in alignment and are driven in part by the rhythm of their different professions, and in part by the norms of the technologies. The integrations between the different actors and the dominance of each actor depends on the position within the lifecycle (e.g., BOL, MOL, or EOL). The complexity is increased when considering the perspective of the asset owner and the supplier, as the factory itself may be in the MOL phase, yet the new machines that are being integrated into the factory are a new design and therefore at the late BOL phase.
The operational factory environment is dynamic (Benotsmane et al., 2019), yet the expectation of the operations manager is to maintain and standardize, and in doing so, optimize efficiency within a quality window (Romero et al., 2016). Processes to manage the mechanical, electrical and control system maintenance within a factory have become part of normal practices. The maintenance team understands the need for and the timing of maintenance since the machines themselves are of a mature technology. What is new in a smart factory is the integration of IoT technologies where technologies are less matured and development cycles significantly shortened.
The systems engineering (Kossiakoff 2014) approach to mechatronic systems has been well described (e.g., in the V-model) and many firms today develop such systems using traditional product development processes. Often these have been based on stage gate-like processes with clearly defined cycles. IoT-technologies are less mature and are on shorter lifecycles today, with much shorter development cycles and are often developed on more agile development processes. This creates a paradox in the development of technical solutions for smart factories, as it is necessary to understand these two approaches to innovation and lifecycle management. In an operational factory, the integration of these different lifecycles provides the potential for additional challenges as operations and maintenance managers understand the mechatronic systems and the lifecycles and equipment lifespans (Vendrell-Herrero, 2021; NIES, n.d.). They therefore face the challenge of having to adapt to the higher velocity of change with IoT technologies and the shorter lifespans they offer. Furthermore, the actors who deal with mechatronic systems in their roles are different to those who deal with the IoT technologies, often with a data science or information systems background.
Based on the differences seen in the literature of the lifecycles and the lifespans of equipment and the difference of the actors within a smart factory environment, the framework in Figure 3 has been developed.
Rather than considering only the system and the relevant subsystem of the different asset classes that constitute a production system, the framework integrates the perspective of the various actors and the asset mix within the smart factory. For the actors, it allows the decision-making horizons to be described visually and integrated into the Systems Engineering use case descriptions. The individual actor’s outlook drives expectations and norms, and a significant part comes from their decision-making horizons that are often considered ‘operational, tactical or strategic’. Additionally, the management team’s skillset within a factory environment is traditional, whereas the new skills needed for smart factories come from Industry 4 (Tomiyama et al., 2019). This creates challenges, as the working norms can create misunderstandings. Using a framework that describes these over the lifecycle of the factory and its subsystems will help managers identify why and where collaboration between different people may create difficulties due to different outlooks or training. Moving to consider the various asset classes, finance experts can provide insights into the replacement cycles, along with the Japanese database; alternatively, personal experience may be used to provide an initial view of lifespans. Nevertheless, an assessment of lifespan must be made with any capital investment, as finance will need to apply the appropriate depreciation rate to the new assets. For reliable asset management, it is crucial to understand and manage the lifecycle of important subsystems. With the integration of Industry four technologies, the lifespans have reduced due to rapid changes in technology as standards are established. The framework here is generic and would help to describe the individual assets and their subsystems. The visual approach here supports identifying possible nested lifecycles created due to the different levels of maturity or innovation in a particular system or subsystem.
Theoretical Implications
From a theoretical perspective, the concept of nested lifecycles provides an interesting viewpoint for asset management and the PLM research community in the context of a smart factory. This study further establishes the gap that the existing literature does not clearly distinguish between product lifecycle and product lifespan management concepts. By introducing the notion of nested lifecycles, this study argues that from a systems perspective, an asset’s lifecycle can be modeled as a nested structure of subsystem lifecycles where the lifespan of those subsystems can also vary. In line with Wellsandt et al. (2019), we also argue that researchers should consider integrating the nesting concept in the Lifecycle Modeling Language. Furthermore, this study provides a framework that will help researchers to develop and analyze case studies based around a specific asset from the system’s perspective by identifying the nested lifecycles resulting from different levels of maturity and innovation.
Managerial Implications
At present, the asset management focus within industry is based around ISO 55001 (Ma et al, 2014), which considers an asset’s value, environmental and social costs, risk, quality of service, and performance. Core asset decision-making frameworks need to be extended to incorporate “nested lifecycle management”. The perspectives of the various actors who are directly or indirectly involved in the management, operation, maintenance, or support of a smart factory need to be considered when designing a smart factory. This can be done visually and should consider actors who are outside the factory’s production area, as Industry 4.0 approaches demand a vertical and horizontal integration of information flows. The addition of the different assets provides an asset-focused view of the factory. It provides an understanding of the typical asset relational cycles based on the expected operational lifespans of systems and subsystems. The integration of some Industry 4-based components within a traditional factory environment can lead to misalignments of expectations between different actors due to their backgrounds and experiences. This may also shed some light on the expectations of obsolescence within the system. The asset visual should be completed for the whole of the factory. Another area of concern is the lack of clear demarcation of operational responsibilities that follow the adoption of newer technologies. Such responsibilities need to be clearly defined throughout the organization. Typically, the responsibility for Asset Management overlaps between Quality and Operations functions (Parra et al., 2021). A new role should be created to support nested asset lifecycles, taking a more holistic view of the entire factory as a system and supporting the integration of the different stakeholder perspectives, ensuring that lifecycles or lifespan issues are a key consideration with asset strategy.
This has a direct impact on the second field of smart product development, which should consider the lifespan of technologies in the design at an early stage to simplify asset management in the smart factory. Here, it is interesting to look at this in the same way as Bakker et al. (2014) see product lifecycle design. Companies should incorporate suitable lifecycle models and design decisions into development at an early stage. For new technologies and applications, innovation cycles are so short that the establishment of long lifespans does not make economic sense or is not yet technologically possible (e.g., batteries). In this case, however, it is important that these components have no influence on the parts and subsystems of a PSS that otherwise have a long lifespan (e.g., housing). It must be possible to simply renew, replace and recycle developing technologies within the PSS. Otherwise, there is a risk that the expected positive effects of digitalization and PSS on sustainability will turn into the opposite, resulting in rebound effects (Kjaer et al., 2018; 2019). This brings us to the third area, namely the question of personnel and the required skills of engaged actors. Asset lifespan consideration could make it important to establish new roles. So far, the focus has been strongly on digital skills and a change of job profiles in the context of Industry 4.0 (Jerman et al., 2020; Pejic-Bach et al., 2020). In previous work, the topic of lifespan management is underrepresented, and we propose, for example, the establishment of a nested lifecycle manager. Here, it would be necessary to clarify who can take on such a role or what skills they need. A Smart Factory is a dynamic system that is subject to ongoing changes and optimizations. A governance approach should be adopted comprising the key function stakeholders to oversee and manage proposed changes to the factory system, from asset beginning of life to renewal.
Conclusion and Recommendations
Much of the literature on product life cycle management is from the manufacturer’s perspective. However, in a Smart Factory, the distinctions of the supplier break down, as many different systems and subsystems may be represented. Furthermore, many different perspectives are represented within the factory environment with varying outlooks. For these reasons, we propose that it may be necessary for a new nested lifecycle management task to be included in the asset lifecycle and product lifecycle design. To support this, we have developed a framework based on the integrative literature that visually combines the outlooks of different actors and the lifespan of various assets, systems, and subsystems.
The framework requires testing and further refinement, and this paper aimed to develop a framework based on published theory, which has been fulfilled. Additionally, the tasks around managing what we have called ‘nested lifecycles’ need to be further described. This role needs to integrate asset lifecycle management and lifecycle design perspectives.
Data Availability Statement
The original contributions presented in the study are included in the article/Supplementary Material further inquiries can be directed to the corresponding author.
Author Contributions
SW (lead author) with contributions in all sections. ME with contributions in all sections. MA contributed to the overall concept, the introduction, the literature review, and the discussion. OS with contributions to the methodology and discussion. JP with contributions to the abstract and the conclusions. MK contributed to theoretical and managerial implication sections and PLM-related discussions throughout the paper.
Funding
Open access funding provided by Lucerne University of Applied Sciences and Arts.
Conflict of Interest
The authors declare that the research was conducted in the absence of any commercial or financial relationships that could be construed as a potential conflict of interest.
Publisher’s Note
All claims expressed in this article are solely those of the authors and do not necessarily represent those of their affiliated organizations, or those of the publisher, the editors and the reviewers. Any product that may be evaluated in this article, or claim that may be made by its manufacturer, is not guaranteed or endorsed by the publisher.
Acknowledgments
The authors would like to thank Lucerne University of Applied Sciences and Arts, Ruhr-Universität Bochum, Glasgow Caledonian University, and School of Business, Carroll University, Waukesha.
References
Abramovici, M., Göbel, J. C., and Savarino, P. (2017). Reconfiguration of Smart Products during Their Use Phase Based on Virtual Product Twins. CIRP Ann. 66 (1), 165–168. doi:10.1016/j.cirp.2017.04.042
Anderson, M., West, S., and Harrison, D. (2021). “Exploring Accidental Digital Servitization in an Industrial Context,” in IFIP International Conference on Advances in Production Management Systems (Cham: Springer), 126–135. doi:10.1007/978-3-030-85906-0_15
Anke, J., Poeppelbuss, J., and Alt, R. (2020). It Takes More Than Two to Tango: Identifying Roles and Patterns in Multi-Actor Smart Service Innovation. Schmalenbach Bus Rev. 72 (4), 599–634. doi:10.1007/s41464-020-00101-2
ANSI (2008). ANSI/ISA 95.00.01-2000: Enterprise-Control System Integration Part 1: Models and Terminology.
AWS (2021). Amazon Monitron FAQs. Available at: https://aws.amazon.com/de/monitron/faqs/(Retrieved December, 2021).
Bag, S., Gupta, S., and Kumar, S. (2021). Industry 4.0 Adoption and 10R advance Manufacturing Capabilities for Sustainable Development. Int. J. Prod. Econ. 231, 107844. doi:10.1016/j.ijpe.2020.107844
Bakker, C., Wang, F., Huisman, J., and Den Hollander, M. (2014). Products that Go Round: Exploring Product Life Extension through Design. J. Clean. Prod. 69, 10–16. doi:10.1016/j.jclepro.2014.01.028
Belhocine, L., Dahane, M., and Yagouni, M. (2021). Customer Behaviour–Based Multi-Objective Approach for the Recovery and Remanufacturing of Used Products: Application to Smartphone Reconditioning Process. Int. J. Adv. Manufacturing Tech. 117 (1), 125–146. doi:10.1007/s00170-021-07646-7
Benešová, A., and Tupa, J. (2017). Requirements for Education and Qualification of People in Industry 4.0. Proced. Manufact. 11, 2195–2202. doi:10.1016/j.promfg.2017.07.366
Benotsmane, R., Kovács, G., and Dudás, L. (2019). Economic, Social Impacts and Operation of Smart Factories in Industry 4.0 Focusing on Simulation and Artificial Intelligence of Collaborating Robots. Social Sciences 8 (5), 143. doi:10.3390/socsci8050143
Blanchard, B. S., Fabrycky, W. J., and Fabrycky, W. J. (1990). Systems Engineering and Analysis. Englewood Cliffs, NJ: Prentice-Hall, 4
Breschi, R., Freundt, T., Orebäck, M., and Vollhardt, K. (2017). The Expanding Role of Design in Creating an End-To-End Customer Experience. McKinzsey & Co. Available at: https://www.mckinsey.com/business-functions/operations/our-insights/the-expanding-role-of-design-in-creating-an-end-to-end-customer-experience (Accessed Dec 14, 2021).
Büchi, G., Cugno, M., and Castagnoli, R. (2020). Smart Factory Performance and Industry 4.0. Technol. Forecast. Soc. Change 150, 119790.doi:10.1016/j.techfore.2019.119790
Burns, B. (2010). “Re-evaluating Obsolescence and Planning for it,” in Longer Lasting Products—Alternatives to the Throwaway Society. Editor T. Cooper (Farnham, UK: Gower), 39–60.
Cerri, D., and Terzi, S. (2016). “Proposal of a Toolset for the Improvement of Industrial Systems' Lifecycle Sustainability through the Utilization of ICT Technologies,” in Computers in Industry 81, 47–54. doi:10.1016/j.compind.2015.09.003
Chenini, A., Iqbal, J., Qurrahtulain, K., Husain Mahmood, M. A., and Aldehayyat, J. S. (2021). Strategic Procurement, Supplier Integration, and Speed‐to‐market : The Mediating Role of Procurement lead‐time Performance and Manufacturing Performance. J. Public Aff. 21 (3), 1–2. doi:10.1002/pa.2248
Cooper, D. R., Skelton, A. C. H., Moynihan, M. C., and Allwood, J. M. (2014). Component Level Strategies for Exploiting the Lifespan of Steel in Products. Resour. Conservation Recycling 84, 24–34. doi:10.1016/j.resconrec.2013.11.014
Coreynen, W., Matthyssens, P., and Van Bockhaven, W. (2017). Boosting Servitization through Digitization: Pathways and Dynamic Resource Configurations for Manufacturers. Ind. Marketing Manage. 60, 42–53. doi:10.1016/j.indmarman.2016.04.012
de Mozota, B. B. (1998). Structuring Strategic Design Management: Michael Porter's Value Chain. Des. Manage. J. 9 (2), 26–31.10.1111/j.1948-7169.1998.tb00201.x
Dewett, T., and Jones, G. R. (2001). The Role of Information Technology in the Organization: a Review, Model, and Assessment. J. Manag. 27 (3), 313–346. doi:10.1177/014920630102700306
Dijkman, R. M., Sprenkels, B., Peeters, T., and Janssen, A. (2015). Business Models for the Internet of Things. Int. J. Inf. Manage. 35 (6), 672–678. doi:10.1016/j.ijinfomgt.2015.07.008
Ekman, P., Raggio, R. D., and Thompson, S. M. (2016). Service Network Value Co-creation: Defining the Roles of the Generic Actor. Ind. Marketing Manage. 56, 51–62. doi:10.1016/j.indmarman.2016.03.002
Fiero, C. (2019). DecemberIs Your SCADA System Aging? Maybe It's Time to Take Stock. Treatment Plant Operator. Available from https://www.tpomag.com/editorial/2019/12/is-your-scada-system-aging-maybe-its-time-to-take-stock (Retrieved December 14, 2021).
Fleisch, E., Weinberger, M., and Wortmann, F. (2015). “Business Models and the Internet of Things (Extended Abstract),” in Interoperability and Open-Source Solutions for the Internet of Things (Cham: Springer), 6–10. doi:10.1007/978-3-319-16546-2_2
Galloway, B., and Hancke, G. P. (2013). Introduction to Industrial Control Networks. IEEE Commun. Surv. Tutorials 15 (2), 860–880. doi:10.1109/SURV.2012.071812.00124
Hamraz, B., Caldwell, N. H. M., and Clarkson, P. J. (2013). A Holistic Categorization Framework for Literature on Engineering Change Management. Syst. Engin. 16 (4), 473–505. doi:10.1002/sys.21244
Hancke, G. P., Silva, B. C., and Hancke, G. P. (2013). The Role of Advanced Sensing in Smart Cities. Sensors (Basel) 13 (1), 393–425. doi:10.3390/s130100393
Herrmann, C., Schmidt, C., Kurle, D., Blume, S., and Thiede, S. (2014), Sustainability in Manufacturing and Factories of the Future. Int. J. Precis. Eng. Manuf.-Green Tech. 1, 283–292. doi:10.1007/s40684-014-0034-z
Honeywell Building Technologies (2021). WEB-HMI Touchscreen Monitors (Product Overview). WEB-HMI Touchscreen Monitors. Available from https://buildings.honeywell.com/us/en/products/by-brand/honeywell-bms-na/web-hmi-touchscreen-monitors (Retrieved December 14, 2021)
Itskos, G., Nikolopoulos, N., Kourkoumpas, D.-S., Koutsianos, A., Violidakis, I., Drosatos, P., et al. (2016). “Energy and the Environment,” in Environment and Development. Editors S. G. Poulopoulos, and V. J. Inglezakis. (Amsterdam: Elsevier), 363–452. doi:10.1016/B978-0-444-62733-9.00006-X
Jaspert, D., Ebel, M., Eckhardt, A., and Poeppelbuss, J. (2021). Smart Retrofitting in Manufacturing: A Systematic Review. J. Clean. Prod.312 127555 doi:10.1016/j.jclepro.2021.127555
Jerman, A., Pejić Bach, M., and Aleksić, A. (2020). Transformation towards Smart Factory System: Examining New Job Profiles and Competencies. Syst. Res. Behav. Sci. 37 (2), 388–402. doi:10.1002/sres.2657
Kalsoom, T., Ramzan, N., Ahmed, S., and Ur-Rehman, M. (2020). Advances in Sensor Technologies in the Era of Smart Factory and Industry 4.0. Sensors 20 (23), 6783. doi:10.3390/s20236783
Kambil, A., and Short, J. E. (1994). Electronic Integration and Business Network Redesign: a Roles-Linkage Perspective. J. Manage. Inf. Syst. 10, 59–83. doi:10.1080/07421222.1994.11518020
Kelly, A. (2006). Plant Maintenance Management Set. Oxford: Butterworth-Heinemann. doi:10.1016/B978-075066995-5.50035-7
Kirchherr, J., Reike, D., and Hekkert, M. (2017). Conceptualizing the Circular Economy: An Analysis of 114 Definitions. Resour. conservation recycling 127, 221–232. doi:10.1016/j.resconrec.2017.09.005
Kjaer, L. L., Pigosso, D. C. A., Niero, M., Bech, N. M., and McAloone, T. C. (2019). Product/Service‐Systems for a Circular Economy: The Route to Decoupling Economic Growth from Resource Consumption? J. Ind. Ecol. 23, 22–35. doi:10.1111/jiec.12747
Kleman, M. C., and Moyer, T. (2017). “Life Cycle Considerations for Microprocessor Relays,” in 2017 70th Annual Conference for Protective Relay Engineers (CPRE) (College stations (Texas): IEEE), 1–14. doi:10.1109/cpre.2017.8090036
Kossiakoff, Alexander. (2011). Systems Engineering : Principles and Practice. 2nd ed. Wiley-Interscience.
Kuhlenkötter, B., Wilkens, U., Bender, B., Abramovici, M., Süße, T., Göbel, J., et al. (2017). New Perspectives for Generating Smart PSS Solutions–Life Cycle, Methodologies and Transformation. Proced. CIRP 64, 217–222.10.1016/j.procir.2017.03.036
Lei, Y. (2017). “Remaining Useful Life Prediction,” in Intelligent Fault Diagnosis and Remaining Useful Life Prediction of Rotating Machinery (Elsevier), 281–358. doi:10.1016/b978-0-12-811534-3.00006-8
Lian, K.-Y., Hsiao, S.-J., and Sung, W.-T. (2013). Mobile Monitoring and Embedded Control System for Factory Environment. Sensors 13 (12), 17379–17413. doi:10.3390/s131217379
Lightfoot, H., Baines, T., and Smart, P. (2013). The Servitization of Manufacturing. Int. J. Operations Prod. Manage. 33, 1408–1434. doi:10.1108/ijopm-07-2010-0196
Lowe, J. (1993). Manufacturing Reform and the Changing Role of the Production Supervisor: the Case of the Automobile Industry*. J. Manage. Stud. 30 (5), 739–758. doi:10.1111/j.1467-6486.1993.tb00324.x
Ma, Z., Zhou, L., and Sheng, W. (2014). “September). Analysis of the New Asset Management Standard ISO 55000 and PAS 55,” in 2014 China International Conference on Electricity Distribution (CICED) (IEEE), 1668–1674.
Mantravadi, S., Møller, C., Li, C., and Schnyder, R. (2022). Design Choices for Next-Generation IIoT-Connected MES/MOM: An Empirical Study on Smart Factories. Robotics and Computer-Integrated Manufacturing 73, 102225. doi:10.1016/j.rcim.2021.102225
Mehta, P., Butkewitsch-Choze, S., and Seaman, C. (2018). Smart Manufacturing Analytics Application for Semi-continuous Manufacturing Process - a Use Case. Proced. Manufacturing 26, 1041–1052. ISSN 2351-9789. doi:10.1016/j.promfg.2018.07.138
Mital, A., Desai, A., Subramanian, A., and Mital, A. (2014). “The Significance of Manufacturing,” in Product Development. Second Edition (Elsevier), 3–19. doi:10.1016/B978-0-12-799945-6.00001-6
Mori, M., Hansel, A., and Fujishima, M. (2014). “Machine Tool,” in The International Academy for Production Engineering. CIRP Encyclopedia of Production Engineering. Editors L. Laperrière, and G. Reinhart (Berlin, Heidelberg: Springer), 792–801. doi:10.1007/978-3-642-20617-7_6533
NIES (2021). Lifespan Database for Vehicles, Equipment, and Structures: LiVES. National Institute for Environmental Studies. http://www.nies.go.jp/lifespan/(Retrieved December, 2021).
Nilsson, D. K., and Larson, U. E. (2008). “Secure Firmware Updates over the Air in Intelligent Vehicles,” in Proceedings of the (Beijing, China: IEEE Int. Conf. Commun. Workshop), Beijing, China, 380–384. doi:10.1109/iccw.2008.78
Olson, E. M., Walker, O. C., and Ruekert, R. W. (1995). Organizing for Effective New Product Development: The Moderating Role of Product Innovativeness. J. marketing 59 (1), 48–62. doi:10.1177/002224299505900105
Osterrieder, P., Budde, L., and Friedli, T. (2020). The Smart Factory as a Key Construct of Industry 4.0: A Systematic Literature Review. Int. J. Prod. Econ. 221, 107476. doi:10.1016/j.ijpe.2019.08.011
Park, B., Jeong, H., Huh, H., Kim, M., and Lee, S. (2018). Experimental Study on the Life Prediction of Servo Motors through Model-Based System Degradation Assessment and Accelerated Degradation Testing. J. Mech. Sci. Technol. 32 (11), 5105–5110. doi:10.1007/s12206-018-1007-x
Parra, C., González-Prida, V., Candón, E., De la Fuente, A., Martínez-Galán, P., and Crespo, A. (2021). “Integration of Asset Management Standard ISO55000 with a Maintenance Management Model,” in 14th WCEAM Proceedings. WCEAM 2019. Lecture Notes in Mechanical Engineering. Editors A. Crespo Márquez, D. Komljenovic, and J. Amadi-Echendu (Cham: Springer), 189–200. doi:10.1007/978-3-030-64228-0_17
Pejic-Bach, M., Bertoncel, T., Meško, M., and Krstić, Ž. (2002). Framework for Outsourcing Manufacturing: Strategic and operational implications,Momme, J. 50, 416–431. doi:10.1016/j.ijinfomgt.2019.07.014
PMMI Media Group (2014). PLC Lifecycle Management. Automation World. Available from https://www.automationworld.com/products/control/article/13311314/plc-lifecycle-management (Retrieved December 14, 2021).
Poeppelbuss, J., Ebel, M., and Anke, J. (2021). Iterative Uncertainty Reduction in Multi-Actor Smart Service Innovation. Electron. Markets, 1–29. doi:10.1007/s12525-021-00500-4
Porter, M. E. (1985). Competitive Advantage: Creating and Sustaining Superior Performance. New York: Simon & Schuster. ISBN 9781416595847.
Porter, M. E., and Heppelmann, J. E. (2014). How Smart, Connected Products Are Transforming Competition. Harv. Bus. Rev. 92 (11), 64–88. https://hbr.org/2014/11/how-smart-connected-products-are-transforming-competition
Potting, J., Hekkert, M. P., Worrell, E., and Hanemaaijer, A. (2017). Circular Economy: Measuring Innovation in the Product Chain. Utrecht: PBL Publishers.
Rassier, J. (2017). What’s the Lifespan of Hardware and Software? Netgain. Available from https://netgaincloud.com/blog/whats-the-lifespan-of-hardware-and-software/(Retrieved December 14, 2021).
Resman, M., Pipan, M., Simic, M., and Herakovic, N. (2019). A New Architecture Model for Smart Manufacturing: a Performance Analysis and Comparison with the RAMI 4.0 Reference Model. Adv. Produc Engineer Manag. 14, 153–165. doi:10.14743/apem2019.2.318
Roblek, V., Meško, M., Pušavec, F., and Likar, B. (2021). The Role and Meaning of the Digital Transformation as a Disruptive Innovation on Small and Medium Manufacturing Enterprises. Front. Psychol. 12. doi:10.3389/fpsyg.2021.592528
Romero, D., Bernus, P., Noran, O., Stahre, J., and Fast-Berglund, Å. (2016). “The Operator 4.0: Human Cyber-Physical Systems & Adaptive Automation towards Human-Automation Symbiosis Work Systems,” in IFIP International Conference on Advances in Production Management Systems (Cham: Springer), 677–686. doi:10.1007/978-3-319-51133-7_80
Saaksvuori, A., and Immonen, A. (2005). “Deployment of the PLM System,” in Product Lifecycle Management (Berlin, Heidelberg: Springer). doi:10.1007/3-540-26906-1_6
Schneider, J., Gaul, A. J., Neumann, C., Hogräfer, J., Wellßow, W., Schwan, M., et al. (2006). Asset Management Techniques. Int. J. Electr. Power Energ. Syst. 28 (9), 643–654. doi:10.1016/j.ijepes.2006.03.007
Sigidov, Y. I., Rybyantseva, M. S., Adamenko, A. A., and Yarushkina, E. A. (2016). Methodological Aspects of Depreciation as an Economic Category. Int. J. Econ. Financial Issues 6 (1S), 88–95.
Sjödin, D. R., Parida, V., Leksell, M., and Petrovic, A. (2018). Smart Factory Implementation and Process Innovation: A Preliminary Maturity Model for Leveraging Digitalization in Manufacturing Moving to Smart Factories Presents Specific Challenges that Can Be Addressed through a Structured Approach Focused on People, Processes, and Technologies. Research-Technology Manage. 61 (5), 22–31.
Snyder, H. (2019). Literature Review as a Research Methodology: An Overview and Guidelines. J. Business Res. 104, 333-339. doi:10.1016/j.jbusres.2019.07.039
Tan, S. Z., and Labastida, M. E. (2021). “Unified IIoT Cloud Platform for Smart Factory,” in Intelligent Systems Reference Library. Editors C. Toro, W. Wang, and H. Akhtar (Cham: Springer), Vol. 202. Implementing Industry 4.0, doi:10.1007/978-3-030-67270-6_3
Terzi, S., Bouras, A., Dutta, D., Garetti, M., and Kiritsis, D. (2010). Product Lifecycle Management – from its History to its New Role. Ijplm 4 (4), 360–389. doi:10.1504/IJPLM.2010.036489
Tomiyama, T., Lutters, E., Stark, R., and Abramovici, M. (2019). Development Capabilities for Smart Products. CIRP Ann. 68 (2), 727–750. doi:10.1016/j.cirp.2019.05.010
Tukker, A. (2004). Eight Types of Product-Service System: Eight Ways to Sustainability? Experiences from SusProNet. Bus. Strat. Env. 13 (4), 246–260. doi:10.1002/bse.414
Tukker, A., and Tischner, U. (2006). Product-services as a Research Field: Past, Present and Future. Reflections from a Decade of Research. J. Clean. Prod. 14 (17), 1552–1556. doi:10.1016/j.jclepro.2006.01.022
United Nations Environment Programme (2007). Life Cycle Management: A Business Guide to Sustainability. Paris: United Nations Environmental Program, UNEP DTIE. https://wedocs.unep.org/bitstream/handle/20.500.11822/7894/DTI0889PA.pdf.
Vangermeersch, R. (1971). A Historical Overview of Depreciation: US Steel, 1902-1970. Rev. Financial Econ. 7 (2), 56.
Vendrell-Herrero, F., Vaillant, Y., Bustinza, O. F., and Lafuente, E. (2021). Product Lifespan: The Missing Link in Servitization. Prod. Plann. Control., 1–17. doi:10.1080/09537287.2020.1867773
Wang, L., and Koh, S. C. (2010). Enterprise Networks and Logistics for Agile Manufacturing. 1st ed. London: Springer. doi:10.1007/978-1-84996-244-5
Wellsandt, S., Cattaneo, L., Cerri, D., Terzi, S., Corti, D., Norden, C., et al. (2019). “Life Cycle Management for Product-Service Systems,” in Models, Methods and Tools for Product Service Design (Cham: Springer), 29–43. doi:10.1007/978-3-319-95849-1_3
West, S., and Pascual, A. (2015). The Use of Equipment Life-Cycle Analysis to Identify New Service Opportunities. Aston Centre Servitization Res. Pract. 18, 235.
Winsystems Inc (2018). Industrial versus Commercial Computers. Winsystems. Available form: https://www.winsystems.com/industrial-versus-commercial-computers/(Retrieved December 14, 2021).
Wulfsberg, J. P., Verl, A.W., Wurst, K.-H., Grimske, S., Batke, C., and Heinze, T. (2013). Modularity in Small Machine Tools. Prod. Eng. Res. Devel. 7 (5), 483–490. doi:10.1007/s11740-013-0464-4
Keywords: smart factory, lifecycle management, asset renewal, product service system, nested lifecycle framework
Citation: West S, Ebel M, Anderson M, Stoll O, Poeppelbuss J and Khan M (2022) Nested Lifecycles-Improving the Visibility of Product Lifespans in Smart Factories. Front. Manuf. Technol. 2:837478. doi: 10.3389/fmtec.2022.837478
Received: 16 December 2021; Accepted: 16 March 2022;
Published: 25 April 2022.
Edited by:
Amit Kumar Jain, Glasgow Caledonian University, United KingdomCopyright © 2022 West, Ebel, Anderson, Stoll, Poeppelbuss and Khan. This is an open-access article distributed under the terms of the Creative Commons Attribution License (CC BY). The use, distribution or reproduction in other forums is permitted, provided the original author(s) and the copyright owner(s) are credited and that the original publication in this journal is cited, in accordance with accepted academic practice. No use, distribution or reproduction is permitted which does not comply with these terms.
*Correspondence: Shaun West, c2hhdW4ud2VzdEBoc2x1LmNo