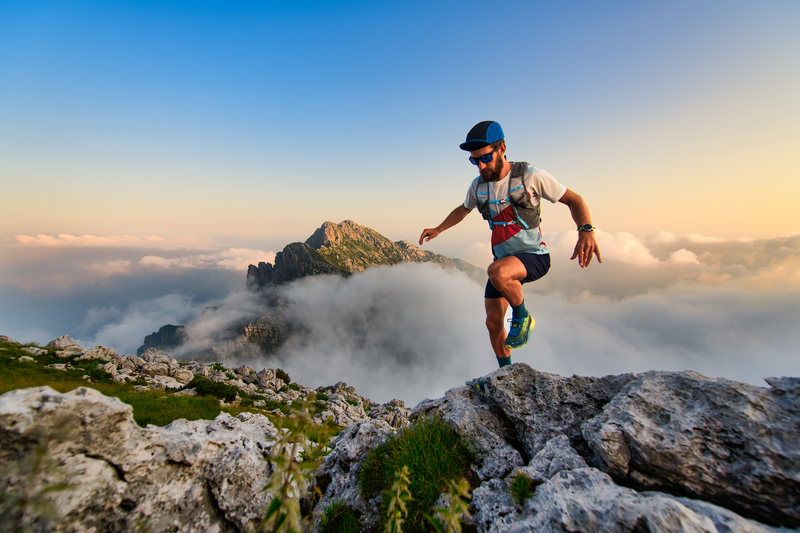
94% of researchers rate our articles as excellent or good
Learn more about the work of our research integrity team to safeguard the quality of each article we publish.
Find out more
ORIGINAL RESEARCH article
Front. Mol. Biosci. , 08 January 2025
Sec. Lipids, Membranes and Membranous Organelles
Volume 11 - 2024 | https://doi.org/10.3389/fmolb.2024.1504373
This article is part of the Research Topic Protein Regulation by Lipids View all 5 articles
This study investigates the dynamics of oleate hydratase (OhyA), a bacterial flavoenzyme from Staphylococcus aureus, and its interactions with lipid membranes, focusing on the factors influencing membrane binding and oligomerization. OhyA catalyzes the hydration of unsaturated fatty acids, playing a key role in bacterial pathogenesis by neutralizing host antimicrobial fatty acids. OhyA binds the membrane bilayer to access membrane-embedded substrates for catalysis, and structural studies have revealed that OhyA forms oligomers on membrane surfaces, stabilized by both protein-protein and protein-lipid interactions. Using fluorescence correlation spectroscopy (FCS), we examined the effects of membrane curvature and lipid availability on OhyA binding to phosphatidylglycerol unilamellar vesicles. Our results reveal that OhyA preferentially binds to vesicles with moderate curvature, while the presence of substrate fatty acids slightly enhanced the overall interaction despite reducing the binding affinity by 3- to 4-fold. Complementary phosphorus-31 (31P) NMR spectroscopy further demonstrated two distinct binding modes: a fast-exchange interaction at lower protein concentrations and a longer lasting interaction at higher protein concentrations, likely reflecting cooperative oligomerization. These findings highlight the reversible, non-stoichiometric nature of OhyA•membrane interactions, with dynamic binding behaviors influenced by protein concentration and lipid environment. This research provides new insights into the dynamic behavior of OhyA on bacterial membranes, highlighting that initial interactions are driven by lipid-mediated protein binding, while sustained interactions are primarily governed by the protein:lipid molar ratio rather than the formation of new, specific lipid-protein interactions. These findings advance our understanding of the biophysical principles underlying OhyA’s role in bacterial membrane function and virulence.
Membrane-targeting domains are essential for recruiting signaling molecules to membranes, and protein association with lipid membranes often occurs in response to extracellular or intracellular stimuli. However, these interactions are typically transient, allowing for precise temporal regulation of signaling pathways (Campagnola et al., 2015). The dynamic nature of these interactions also affects how proteins locate their target substrates, though the specifics of this process are not well understood. Peripheral membrane proteins play critical roles in key cellular processes such as signaling, cell division, and vesicle trafficking (Munro, 2002; DiNitto et al., 2003; Cho and Stahelin, 2005). These proteins associate with lipid membranes through various mechanisms, including lipid modifications and membrane-targeting domains. Their membrane binding sites can interact directly with specific lipid molecules, outer head groups (Stahelin, 2009), or the internal hydrocarbon backbone (Mulgrew-Nesbitt et al., 2006; Lomize et al., 2007; Murphy et al., 2009). Quantitative studies of protein-membrane affinity are crucial for unraveling the mechanics of these associations, understanding how the biophysical properties of membranes influence interactions, and determining the energetics of protein binding and insertion.
Oleate hydratase (OhyA) is a bacterial flavoenzyme that catalyzes water addition to cis double bonds of unsaturated fatty acids, producing hydroxylated fatty acids (hFA) (Radka et al., 2021b; Radka et al., 2024). OhyA activity facilitates a crucial step in the reduction of linoleic acid in commensal gut bacteria (Yang et al., 2017), and as a biocatalyst in the production of hFA intermediates (Prem et al., 2022). Commensal bacterial OhyA utilizes host unsaturated fatty acids to produce hFAs that work to reduce gut inflammation and bacterial membrane destabilization (Galbraith et al., 1971; Greenway and Dyke, 1979; Raychowdhury et al., 1985; Zheng et al., 2005; Miyamoto et al., 2015; Saika et al., 2019). Recent studies have displayed the role of OhyA as a virulence determinant in Staphylococcus aureus, a common human pathogen, and the primary cause of skin infection (Chalmers and Wylam, 2020). The initial response from the innate immune system, during a S. aureus infection, is for host cells to secrete antimicrobial peptides and fatty acids (Liu et al., 2018). To combat this response, S. aureus expresses OhyA to detoxify antimicrobial fatty acids in the host (Subramanian et al., 2019). A murine skin infection model has observed that OhyA activity represses the immune response, while OhyA disruption compromised S. aureus virulence (Radka et al., 2021a).
We study the S. aureus OhyA as a representative homolog (Robert et al., 2024). OhyA forms a homodimer in solution comprised of three key functional domains (Figure 1A): fatty acid lobe to bind unsaturated fatty acid substrate, FAD-binding lobe to bind coenzyme FAD, and carboxy terminus made of amphipathic helices that bind the membrane bilayer to interact with unsaturated fatty acid substrate (Radka et al., 2021b; Radka et al., 2024). The current model is the carboxy terminus transfers fatty acid from the membrane to the active site through a hydrophobic tunnel. Lipid binding studies demonstrated OhyA is a peripheral membrane-associated protein that directly interacts with the bilayer through surface electrostatic interactions between its carboxy terminus and the phosphate layer of the membrane (Radka et al., 2024). Structural analysis of an OhyA•membrane complex by cryo-electron microscopy showed OhyA assembles into higher order oligomers on the membrane surface, and the oligomers are stabilized by intermolecular interactions between adjacent OhyA dimers (Oldham et al., 2024). Thus, protein-protein and protein-lipid interactions both drive OhyA•membrane complex formation, but decomposition of their contributions to binding has not been investigated.
Figure 1. Purification and thermal stability of GFP-OhyA. (A), AlphaFold-generated dimer model of GFP-OhyA, with colors representing different functional domains. (B), GFP-OhyA appears as a 97 kDa monomer, with a purity of >95% as confirmed by denaturing gel electrophoresis. The theoretical molecular weight of the GFP-OhyA monomer is 96.8 kDa. (C), Gel filtration chromatogram (black) of GFP-OhyA, eluted from a Superose 6 Increase 10/300 GL column, overlaid with the molar mass distribution (green) from multi-angle light scattering (SEC-MALS). According to SEC-MALS, GFP-OhyA primarily exists as a 159.1 kDa dimer. (D), Thermal denaturation analysis of GFP-OhyA was performed to assess structural integrity of the protein. Assays (N = 5) were conducted with 1 mg/mL GFP-OhyA, and the data were fitted to the Boltzmann equation. The mean melting temperature is reported with ± S.D.
Fluorescence correlation spectroscopy (FCS) is a powerful tool for rapid equilibrium analysis of fluorescent molecules and has been used to study the dynamics of protein-membrane binding interactions (Rhoades et al., 2006; Thomas et al., 2015; Kruger et al., 2017; Vesga et al., 2023), membrane toxin conformational alterations, and elucidate membrane-receptor binding (Chen et al., 2009; Harwardt et al., 2018; Ponmalar et al., 2019). FCS is a highly sensitive technique that measures the intensity fluctuation emitted by single molecules passing in and out of a focused light, yielding intensity fluctuations (Elson and Magde, 1974; Elson, 2011; Elson, 2013). The intensity fluctuations correspond to variations in the number of molecules interacting within, and diffusing through, the confocal plane (Elson and Magde, 1974). Evaluation of the decay of the intensity fluctuations enables the determination of diffusion coefficients and binding rate constants. Additionally, spectroscopic techniques detect signals from both aqueous and lipid phases simultaneously, eliminating the need for physical separation of these phases, which could disrupt equilibrium (Santos et al., 2003).
OhyA has shown a nanomolar binding affinity to phosphatidylglycerol, the dominant anionic phospholipid in the S. aureus membrane (Kuhn et al., 2015), in previous surface plasmon resonance (SPR) experiments (Radka et al., 2024). However, these experiments used large unilamellar vesicles (LUVs) composed of both phosphatidylglycerol and phosphatidylcholine due to the L1 sensor chip’s inefficiency in capturing anionic phospholipids. Moreover, SPR only allowed titration of one component (protein), limiting the analysis. In contrast, FCS does not require immobilization of components, allowing for experimental designs with varying concentrations of proteins and/or lipids and providing greater flexibility in the types of molecules that can be studied.
In this study, we employed a green fluorescent protein (GFP)-OhyA chimeric protein in FCS experiments to investigate the dynamics of OhyA binding phosphatidylglycerol unilamellar vesicles, focusing on the effects of membrane curvature and substrate availability on OhyA•membrane interactions. Our results revealed that GFP-OhyA shows a moderate preference for binding to LUVs over small unilamellar vesicles (SUVs), highlighting the role of membrane curvature in driving the interaction. Incorporating the 18:1Δ9 unsaturated fatty acid substrate into vesicles had only a minor impact on OhyA•membrane binding, reinforcing that curvature is the primary factor influencing membrane association. Titration experiments with varying protein and lipid concentrations identified two distinct binding modes: as protein concentration increased, OhyA accumulated on protein-loaded vesicles, whereas increasing the concentration of accessible lipids decreased the protein occupancy on individual vesicles. These findings offer new insights into the dynamic behavior of OhyA on membrane surfaces and suggest that protein distribution on membranes can be highly uneven due to non-stoichiometric interactions.
The gfp-ohyA gene was constructed to contain an amino terminal His6 tag followed by the gfp gene that was linked by a flexible linker (amino acid sequence GGGGSGGSS) to ohyA as previously described (Radka et al., 2024). The initiating methionine residues of GFP and OhyA were mutated to alanine residues to eliminate alternative transcription start sites during overexpression. Thus, the open reading frame encoded fMet-His6 tag-GFP-flexible linker-OhyA. The gfp-ohyA gene was cloned into the pET28a 5′-NcoI restriction site using the Gibson Assembly method to make pGFP-OhyA. Overexpression of gfp-ohyA is driven by the strong bacteriophage T7 promoter in this plasmid.
The pGFP-OhyA plasmid was transformed into Escherichia coli BL21 (DE3) cells, and isolates were selected on Luria broth agar plates containing 50 μg/μL kanamycin (Gold Biotechnology). The transformants were cultured in Luria broth with 50 μg/μL kanamycin at 37°C with shaking at 200 rpm. Once the cells reached an OD600 of 0.6, the temperature was lowered to 16°C, and protein expression was induced overnight with 1 mM isopropyl-β-D-thiogalactoside (Gold Biotechnology). After induction, the cells were harvested and lysed in a buffer containing 20 mM Tris, pH 8.0, 10 mM imidazole, 200 mM NaCl. The GFP-OhyA protein was purified from the lysate using nickel agarose beads (Gold Biotechnology) and eluted with a buffer containing 20 mM Tris, pH 8.0, 250 mM imidazole, and 200 mM NaCl. The eluted protein was further purified by gel filtration using a HiLoad Superdex 200 16/60 column (Cytiva Life Sciences) into a buffer with 20 mM Tris, pH 8.0, and 200 mM NaCl. The molecular weight of GFP-OhyA was estimated by SEC-MALS using a Superose 6 Increase 10/300 GL column (Cytiva Life Sciences) with three detectors connected in series: an Agilent 1200 UV detector (Agilent Technologies), a Wyatt DAN HELEOS II multi-angle light-scattering and a Wyatt Optilab T-rEX differential refractive index detector (Wyatt Technologies). The column was equilibrated in 20 mM Tris, 200 mM NaCl, pH 7.6, and 100 μL of GFP-OhyA (2 mg/mL) was injected at a flow rate of 0.5 mL/min at 25°C. Data were recorded and analyzed with the Wyatt Astra software (version 8), and plotted as a molar mass distribution superimposed on a chromatogram of A280 versus elution volume.
The thermal stability of GFP-OhyA was determined by a Sypro Orange-based fluorescence assay (Huynh and Partch, 2015). Solutions (30 μL) of GFP-OhyA (1 mg/mL) in 50 mM K2HPO4, 150 mM NaCl, pH 6, and 2.5 × Sypro Orange dye were added to the wells of a ThermoGrid optically clear PCR plate (Denville Scientific). The plates were centrifuged at 1,000 × g for 5 min and then analyzed by the ABI 7300 real-time PCR system as described previously (Radka et al., 2020). The temperature was ramped from 25°C to 95°C at 1°C/min with the fluorescence read six times at each temperature ramp. The resulting data were fitted to a Boltzmann sigmoidal equation to determine the melting point. The experiment was repeated five times, the thermal melting temperature of each replicate was determined independently, and the melting points from each replicate were averaged to determine the reported thermal melting point.
Charged unilamellar vesicles were prepared from three different lipid compositions: 100% anionic dioleoylphosphatidylglycerol (DOPG) (Avanti Polar Lipids), cationic dioleoylphosphatidylcholine (DOPC) (Avanti Polar Lipids), and a 99:1 mixture of DOPG and 18:1Δ9 (Ambeed) (DOPG/OA). For 100% DOPG or 100% DOPC vesicles, 60 μg of lipid was dissolved in chloroform and added to a 16 mm glass culture tube. For the DOPG/OA vesicles, 60 μg of DOPG and 2.12 μg of 18:1Δ9 were added to the glass culture tube. Micelles were prepared from 60 μg neutral triolein (Avanti Polar Lipids). The chloroform was evaporated under nitrogen gas using the Reacti-VapIII (Thermo Scientific, #TS-18826). The dried lipids were resuspended to a final concentration of 3 mM lipids in 50 mM KPO4, 150 mM NaCl, pH 6.0 and sonicated at 37∘C for at 5–10 min. Lipids were then manually extruded back and forth 75 times using a 10 mm filter support (Avanti Polar Lipids) fitted with either 0.03 μm or 0.1 μm polycarbonate membranes to produce SUVs or LUVs, respectively. The 0.1 μm polycarbonate membrane was used to prepare micelles. The hydrodynamic size and polydispersity of each lipid particle were measured using dynamic light scattering (DLS) with the RNA-LNP application of a Stunner instrument (Unchained Labs). Measurements were conducted under default settings: a 142° scattering angle, a 660 nm laser, and four 1 s acquisitions with automatic angle selection and outlier exclusion. At a lipid concentration of 3 μM, DLS confirmed that lipid particles remained monodisperse and did not aggregate, even at the highest lipid concentration used in the binding titration.
To fluorescently label the vesicles or micelles for FCS standardization, 9.76 μL of a 1:100 dilution from a 1 mg/mL 18:1 Liss Rhod PE (Avanti Polar Lipids) stock solution was added to each lipid mixture in a 16 mm glass culture tube. The chloroform was evaporated under nitrogen gas, and the vesicles or micelles were then assembled as described above. This process produced Rhod PE-labeled SUVs, LUVs, and micelles for assessing free diffusion.
To determine the impact of protein concentration on binding, 0.014, 0.028, 0.056, 0.113, 0.22, 0.45, 0.9, 1.8 μM GFP-OhyA was mixed with 0.188 μM accessible lipid. To determine the impact of accessible lipid concentration on binding, 0.0115, 0.023, 0.047, 0.094, 0.188, 0.375, 0.75, 1.5, 3 μM lipid was mixed with 0.113 μM GFP-OhyA. The samples were 50 μL in volume and incubated in 50 mM KPO4, 150 mM NaCl, pH 6.0 for 5 m at 20°C before FCS data acquisition. The samples used for FCS standardization were 0.113 μM GFP-OhyA and 0.188 μM Rhod PE-labeled DOPC LUVs, DOPG SUVs and LUVs, DOPG/OA SUVs and LUVs, and triolein micelles.
FCS measurements were conducted using a custom-built microscope within the University of Kentucky Microscopy Core. Approximately 50 μL of freshly prepared, fluorescently labeled sample was placed onto an 18 × 18 mm Zeiss no. 1.5 coverslip, positioned inside a 35 mm Petri dish with an 18 mm hole (Cell E&G, Cat #: PDH00002-200). The Petri dish was mounted on a Nikon Eclipse Ti2 microscope equipped with a PicoQuant PicoHarp 300 Time-Correlated Single Photon Counting (TCSPC) system. A 532 nm laser, set at 1% power (29 μW), was used to excite the fluorescent labels, and a ×60 water immersion objective focused the laser beam on the sample. Photon detection was handled by the PicoHarp 300 TCSPC module. Each FCS result represents the average of three 60 s measurements. To avoid background interference from immobilized molecules on the coverslip, all measurements were taken at least 30 μm above the glass surface. Raw data was processed using SymPhoTime 64 (PicoQuant) software.
Laser power and pinhole diameter were calibrated using highly diluted GFP-OhyA and Rhod PE solutions, excited at 488 nm and 563 nm, respectively. At least five autocorrelation functions were obtained at 20°C for each condition, adjusting the laser irradiance to achieve consistent molecular brightness at 1% power. The measured laser power was 29 μW, and pinhole calibration ensured that the number of fluorescent molecules within the confocal plane (N) equaled 1.
All raw data analysis was performed through SymPhoTime 64. After recording the fluctuations of the times versus fluorescence intensity trace, which indicated the diffusing fluorescent species in the detection volume, the autocorrelation function is applied and defined as:
The parameter I (t) represents the intensity time trace (Hz). The brackets indicate averaging over time. The autocorrelation time refers to the total duration during which the fluorescent species remains within the confocal plane.
For our standard measurements, the autocorrelation data was further fitted with a pure diffusion model:
The parameter
For our experimental data, GFP-OhyA plus lipid particles, the autocorrelation data was further fitted with a 3D-triplet kinetics model comprising 2 components:
The parameter
We employed the single-molecule FCS technique to measure how vesicle addition affects protein diffusion, specifically by tracking the protein molecules rather than the vesicles themselves. The observed shift in protein diffusion time is caused by specific binding, as demonstrated by the side-by-side comparison of multiple lipid types (Section 3.2). The key readout from this experiment is the proportion of protein molecules that diffuse freely versus those with inhibited diffusion, which we interpret as the fraction of protein bound. It is important to note that we did not measure the number of protein molecules on each vesicle or the extent of the vesicle surface area covered.
DOPG (12 mM) was prepared in a buffer containing 150 mM NaCl, 1 mM bis-tris methane, pH 6.0, and 10% D₂O. GFP-OhyA was titrated into the DOPG solution at varying concentrations (3.9, 7.5, 16.7, 28.1, 42.9, 82.3, and 169.9 μM), using identical buffer conditions as the DOPG preparation.
31P NMR experiments were conducted at 298 K using a Bruker Avance 400 MHz spectrometer operating at 161.7 MHz. Proton decoupling was achieved with inverse-gated decoupling to minimize 1H–31P nuclear Overhauser effect (NOE) interactions. Data were collected using an observation frequency range of 65,789.48 Hz and a total of 64,000 data points. Acquisition parameters included a 90° pulse length and a 2 s pulse cycle. Approximately 22,000 accumulations were recorded before performing Fourier transformation of the free induction decay signal.
Two-dimensional 1H-13C spectra were acquired to analyze interactions between DOPG and GFP-OhyA. DOPG (12 mM) was prepared in a buffer containing 150 mM NaCl, 1 mM bis-tris methane, pH 6.0, and 10% D2O, with and without 169.9 μM GFP-OhyA. Spectra were recorded at 298 K using a Bruker Avance 600 MHz NMR spectrometer equipped with four RF channels and a 5 mm z-gradient TXI CryoProbe (Bruker BioSpin), and operated with a 13C detection frequency of 150 MHz. This setup allowed for detailed analysis of 1H-13C correlations to investigate molecular interactions between the lipid and protein under defined conditions.
The χ2 values were used to assess the quality of fit for FCS data modeling in SymPhoTime 64. Statistical analyses and mathematical modeling of the processed data were conducted using GraphPad Prism software version 10.3.0.
The specific binding with Hill slope model is defined by the equation:
The binding model describes the relationship between ligand concentration (X) and specific binding (Y), with key parameters providing insights into the binding process. X represents the ligand concentration, while Y denotes the specific binding. The maximum binding capacity, Bmax, reflects the total number of available binding sites, and the dissociation constant, KD, indicates the ligand concentration required to achieve half-maximum binding at equilibrium, representing the binding affinity. The Hill slope (h) provides information about cooperativity: when h = 1, there is no cooperativity, meaning ligand binding at one site does not influence others. A Hill slope h > 1 indicates positive cooperativity, where binding at one site enhances the likelihood of ligand binding at additional sites, while h < 1 suggests negative cooperativity, where binding at one site reduces the affinity of other sites or indicates the presence of multiple binding sites with differing affinities.
An amino-terminal His-tagged version of GFP-OhyA (Figure 1A) was expressed in Eschericia coli and purified by Ni+ affinity and gel filtration chromatography to obtain a homogenous 97 kDa protein (Figure 1B). GFP-OhyA eluted predominantly as a single species on a Superose 6 Increase 10/300 GL column (Figure 1C). Like OhyA, the apparent molecular weight of GFP-OhyA estimated by its anisotropic light scattering pattern indicated the protein is 80.6% dimer in solution (Figure 1C). The 46.99°C ± 0.29°C melting temperature of GFP-OhyA (Figure 1D) indicates its structural resistance to thermal denaturation is within 1.31°C of OhyA (Radka et al., 2024). These data indicate GFP-OhyA is a stable protein with the correct OhyA quaternary structure.
Fluorescence correlation spectroscopy (FCS) was used to monitor the binding of fluorescently labeled ligands to biomolecules (Figure 2A). Diffusion time (τD), which scales with the hydrodynamic radius of diffusing molecules, was calculated from the autocorrelation of fluorescence time traces. We first compared the diffusion times of GFP-OhyA and Rhodamine PE-labelled large unilamellar vesicles (LUVs) and micelles. Fluorescence bursts in the 60 s time trace correspond to molecules passing through the confocal plane (Figure 2B). The intensity and duration of these bursts reflect fluorophore concentration and residence time in the detection volume.
Figure 2. Microscope set-up and standard diffusion time measurements. (A), Diagram of Nikon Eclipse Ti2 microscope set-up utilizing the confocal laser system. The Nikon Eclipse Ti2 microscope is connected to a PicoHarp300 single-photon counter which transmits the fluorescent signal to the SymPhoTime 64 software for analysis. (B), Diagram of the illumination and detection volumes. Only fluorescent particles that diffuse through the confocal plane are detected by the photon counter for analysis. (C), Fitted ACFs for freely diffusing GFP-OhyA protein in 50 mM KPO4, 150 mM NaCl, pH 6.0. A simple diffusion model (SymPhoTime 64) was utilized to fit the ACF and calculate diffusion parameters for the standards.
Autocorrelation functions (ACF) derived from fluorescence time traces were used to determine diffusion times and molecular concentrations (Equations 1, 2). The best-fit normalized ACFs, G(τ), for GFP-OhyA is shown in Figure 2C, with a diffusion time of 0.032 ± 0.0012 ms. To evaluate lipid-binding preferences, we constructed LUVs of dioleoylphosphatidylglycerol (DOPG, anionic), dioleoylphosphatidylcholine (DOPC, cationic), and micelles of triolein (neutral lipid). Best fit ACFs for LUVs or micelles (Figures 3A–C; Table 1) exhibited longer diffusion times compared to free GFP-OhyA: 2.91 ± 0.31 ms for DOPG LUVs, 2.6 ± 0.27 ms for DOPC LUVs, and 0.65 ± 0.09 ms for triolein micelles. Dynamic light scattering measurements confirmed the lipid particles were monodisperse and of the expected size (Figure 3D). These results confirm that FCS effectively distinguishes free GFP-OhyA from lipid particle-bound protein based on shifts in diffusion time.
Figure 3. Standard diffusion time measurements for differing liposomal compositions. (A–C), Fitted ACFs for freely diffusing DOPG LUVs, DOPC LUVs, and Triolein micelles constructed from 0.188 μM of accessible lipid in 50 mM KPO4, 150 mM NaCl, pH 6.0. A simple diffusion model (SymPhoTime 64) was utilized to fit the ACF and calculate diffusion parameters for the standards. (D), Mass distribution curves for DOPG LUVs, DOPC LUVs, and Triolein micelles constructed from 3 μM of accessible lipid in 50 mM KPO4, 150 mM NaCl, pH 6.0. Measurements were collected via dynamic light scattering (DLS) analysis to confirm the size and homogeneity of each liposomal mixture.
Table 1. Fitted autocorrelation parameters diffusion time (τD), rate (D), reliability (χ2), and the mean hydrodynamic radius for lipid particle standards.
When GFP-OhyA binds its 18:1Δ9 (OA) substrate embedded in lipid membranes, its diffusion time increases–a hallmark of ligand binding to larger biomolecular complexes. Given the larger diameter of lipid particles relative to GFP-OhyA, we assumed that protein-loaded lipid particles diffuse with a similar coefficient as free particles. Mixing GFP-OhyA with lipid particles revealed a notable increase in diffusion time with DOPG LUVs but not DOPC LUVs or triolein micelles (Figure 4). Upon binding DOPG LUVs, GFP-OhyA diffusion time increased to 1.2 ± 0.12 ms and the ACF curve shape changed significantly Equation 3. Specifically, the upper plateau shifted downward, the lower plateau shifted rightward, and the slope connecting the plateaus broadened. These shape changes, combined with the shift to longer diffusion times, indicate that GFP-OhyA binds and interacts preferentially with anionic phospholipids.
Figure 4. DOPG alters the diffusion behavior of GFP-OhyA via binding interactions. Representative normalized autocorrelation functions of 0.22 μM GFP-OhyA mixed with DOPG LUVs, DOPG + 18:1Δ9 LUVs, DOPC, and Triolein micelles constructed from 0.188 μM accessible lipids at 100 nm. Autocorrelation functions were fitted using a 3D-triplet kinetics model (SymPhoTime 64) comprising two components, utilizing a Gaussian 3D distribution. The diffusion time for free GFP-OhyA (τD = 0.032 ms) was held constant. Values for χ2, τD, and D for each liposome composition and size are present in Table 1.
To investigate the effects of substrate availability and membrane curvature on GFP-OhyA binding, we prepared DOPG/OA LUVs and small unilamellar vesicles (SUVs) of DOPG and DOPG/OA (Figures 5A–D). Mixing GFP-OhyA with DOPG/OA LUVs increased its diffusion time to 2.6 ± 0.22 ms. With SUVs, diffusion times were 1.8 ± 0.42 ms for DOPG and 2.2 ± 0.14 ms for DOPG/OA Equation 3. While all DOPG-containing vesicles shifted the lower ACF plateau rightward and broadened the slope connecting the plateaus, only DOPG/OA LUVs caused a downward shift of the upper plateau (Figure 6). These findings indicate that GFP-OhyA interacts with all DOPG-containing vesicles, with larger LUVs exerting a more pronounced effect on the ACF curve than smaller SUVs. Although embedding OA substrates into vesicles had minimal impact on the substrate-free vesicle ACF curve’s shape, it consistently extended GFP-OhyA diffusion times.
Figure 5. Standard diffusion time measurements of OhyA-specific lipid compositions. (A–C), Fitted ACFs for freely diffusing DOPG SUVs, DOPG + 18:1Δ9 SUVs, and DOPG + 18:1Δ9 LUVs constructed from 0.188 μM accessible lipid in 50 mM KPO4, 150 mM NaCl, pH 6.0. A simple diffusion model (SymPhoTime 64) was utilized to fit the ACF and calculate diffusion parameters for the standards. (D), Mass distribution curves for DOPG SUVs, DOPG + 18:1Δ9 SUVs, and DOPG + 18:1Δ9 LUVs constructed from 3 μM accessible lipid in 50 mM KPO4, 150 mM NaCl, pH 6.0. Measurements were collected via dynamic light scattering (DLS) analysis to confirm the size and homogeneity of each liposomal mixture.
Figure 6. Protein•liposome binding alters the diffusion behavior of GFP-OhyA. Representative normalized autocorrelation functions of 0.22 μM GFP-OhyA mixed with DOPG SUVs, DOPG + 18:1Δ9 SUVs, DOPG LUVs, and DOPG + 18:1Δ9 LUVs constructed from 0.188 μM accessible lipids at 30 nm. Autocorrelation functions were fitted using a 3D-triplet kinetics model (SymPhoTime 64) comprising two components, utilizing a Gaussian 3D distribution. The diffusion time for free GFP-OhyA (τD = 0.032 ms) was held constant. Values for χ2, τD, and D for each liposome composition and size are present in Table 1.
To further investigate the concentration-dependent binding of GFP-OhyA to vesicles, we used the SymPhoTime-derived ρ1 and ρ2 parameters from the fitted ACFs, reflecting the contributions of free and bound GFP-OhyA, respectively. The linear relationship between the GFP-OhyA concentrations used in the titration and those detected provides evidence that our measurements accurately correlate with the concentrations applied in each instance (Supplementary Figure S1). The fraction of GFP-OhyA bound was calculated and fitted to a specific binding with Hill slope model (Figure 7) (Equation 4). This binding model enables the extraction of the ligand concentration required to achieve half-maximum binding at equilibrium and fits a Hill slope to measure cooperativity. Results showed that substrate presence and lower membrane curvature influenced binding. At the highest GFP-OhyA concentration, the fraction bound to DOPG vesicles was 0.335 for SUVs (Figure 7A) and 0.442 for LUVs (Figure 7B). When DOPG/OA vesicles were used, these fractions increased to 0.412 for SUVs (Figure 7C) and 0.718 for LUVs (Figure 7D). Positive cooperativity was observed only when GFP-OhyA bound to substrate-free SUVs; in all other cases, the cooperativity was negative. These findings indicate that in both SUVs and LUVs, the presence of embedded substrate enhanced binding compared to substrate-free vesicles, despite reducing the binding affinity by 3- to 4-fold (from 79.9 to 247.4 nM for LUVs, and 117.1–447.0 nM for SUVs). The incomplete binding at high concentrations (fraction bound not reaching 1 asymptotically) suggests a reversible process with potential conformational states preventing complete vesicle saturation.
Figure 7. Fraction bound GFP-OhyA versus protein concentration. Fraction bound calculations were performed utilizing the autocorrelation function parameters ρ1 and ρ2 that describe the contribution of each diffusing species to the correlation function (bound GFP-OhyA vs. unbound GFP-OhyA). A constant accessible lipids concentration (0.188 μM) was used for protein titrations versus (A) DOPG LUVs, (B) DOPG SUVs, (C) DOPG + 18:1Δ9 LUVs, or (D) DOPG + 18:1Δ9 SUVs. Data are fitted to a specific binding with Hill slope model (GraphPad 10.3.0).
The influence of accessible lipid concentration on GFP-OhyA binding was analyzed similarly to the concentration-dependent experiments. A specific binding with Hill slope model was used to fit the protein fraction bound (Figure 8). Interestingly, as lipid concentration increased, GFP-OhyA binding decreased. For DOPG vesicles, the SUVs initially increased in bound fraction before decreasing from 0.618 to 0.170 (Figure 8A), while the LUVs showed a gradual decline in bound fraction from 0.547 to 0.289 (Figure 8B). In DOPG/OA vesicles, the SUVs fraction bound decreased from 0.444 to 0.157 (Figure 8C), and the LUVs bound fraction decreased from 0.573 to 0.217 (Figure 8D). Negative cooperativity was observed across all vesicle types. These results suggest that higher lipid concentrations may impede GFP-OhyA binding.
Figure 8. Fraction bound GFP-OhyA versus accessible lipid concentration (Lipidacc). Fraction bound calculations were performed utilizing the autocorrelation function parameters ρ1 and ρ2 that describe the contribution of each diffusing species to the correlation function (bound GFP-OhyA vs. unbound GFP-OhyA). A constant protein concentration (0.113 μM) was used for lipid titrations using (A) DOPG liposomes LUVs, (B) DOPG SUVs, (C) DOPG + 18:1Δ9 LUVs, or (D) DOPG + 18:1Δ9 SUVs. Data are fitted to a specific binding with Hill slope model (GraphPad 10.3.0).
We normalized our results by calculating the ratio of bound GFP-OhyA to accessible lipid concentration to understand the role of protein distribution in membrane binding. As protein concentration increases with a fixed accessible lipid concentration, the ratio of vesicle-bound protein to accessible lipid concentration rises hyperbolically, demonstrating positive cooperative binding across all DOPG vesicle types (Figure 9A). This curve shape, with a distinct maximum, indicates that the number of vesicles carrying large quantities of protein increases relative to the protein molecules in solution. This suggests a protein:lipid molar ratio-dependent binding mode where protein molecules assemble on individual vesicles.
Figure 9. Ratio of bound GFP-OhyA (GFP-OhyAmem) to accessible lipid (Lipidacc) compared to total GFP-OhyA and accessible lipid titrations. (A), The GFP-OhyAmem:Lipidacc ratio versus GFP-OhyA data were fitted to a specific binding with Hill slope model. A representation of binding is displayed below, depicting interactions between liposome (blue) and GFP-OhyA (green) at a high GFP-OhyA concentration. (B), GFP-OhyA mem:Lipidacc versus Lipidacc data were fitted to a specific binding with Hill slope model (GraphPad 10.3.0). A schematic for each binding mode is shown below each graph.
Conversely, increasing the lipid concentration while maintaining a constant protein concentration results is an exponential decline in the ratio of vesicle-bound protein to accessible lipid concentration, indicating negative cooperative binding across all DOPG vesicle types (Figure 9B). This curve, which exhibits a noticeable minimum, reflects a shift in the equilibrium between bound and unbound protein. As vesicle concentration increases, the protein-to-lipid molar ratio decreases, and the equilibrium shifts to a state with minimal protein bound, as the binding remains highly reversible. The apparent reduction in bound protein is not due to vesicles rendering bound protein undetectable by FCS, but rather to the equilibrium favoring the unbound state. This binding mode involves protein adsorption to the bilayer through electrostatic interactions, potentially disrupting protein assemblies or inhibiting their formation due to limited cooperativity or steric hindrance. Some protein molecules may bind to lipid sites in a way that excludes additional GFP-OhyA subunits from assembling, leaving unincorporated subunits unbound at fixed accessible lipid concentrations. This behavior underscores the dynamic and non-uniform nature of OhyA’s interaction with lipid membranes.
Notably, a membrane bilayer is essential for transitioning OhyA from discrete dimers to oligomeric ring assemblies that encircle vesicles (Radka et al., 2024; Oldham et al., 2024). The near-identical convergence of curves from both titration experiments indicates that OhyA’s association with the membrane is not significantly influenced by membrane curvature or substrate availability. Instead, protein-membrane binding is predominantly driven by protein:lipid molar ratio, reflecting the interplay between protein-protein and protein-lipid interactions.
Phosphorus-31 (31P) NMR spectroscopy was used to investigate the molecular interactions between GFP-OhyA and DOPG. Stacked NMR spectra (Figure 10A) revealed uniform and significant chemical shift changes (0.24 ppm) as the protein concentration increased, transitioning from the unbound state (0.68 ppm) to the bound state (2.14 ppm). These observations indicate fast-exchange binding of GFP-OhyA to DOPG that forms a slightly different size vesicle, characterized by a single sharp peak progressively shifting downfield with increasing protein concentrations.
Figure 10. 31P NMR spectrum of DOPG with GFP-OhyA. 31P NMR spectra were recorded as a function of increasing GFP-OhyA concentration. (A), Stacked plot gives the 31P NMR spectra of free DOPG (0.68 ppm) and protein-bound DOPG (up to 2.14 ppm). Peaks marked with † are due to fast-exchange interaction between GFP-OhyA and DOPG, and peaks marked with ‡ are due to the intermediate-exchange interaction. (B), Chemical shift data versus protein concentration from the fast-exchange binding interaction, fitted to a specific binding with Hill slope model (GraphPad 10.3.0).
At a protein concentration of 7.5 μM, a second smaller, broader peak appeared at 0.24 ppm, intensifying and broadening with increasing protein concentrations. This second peak, which shifts upfield of the unbound DOPG signal, remains at a constant chemical shift position and is consistent with intermediate-exchange binding with another vesicle of the same order.
The presence of both fast- and intermediate-exchange binding suggests two distinct binding events occur between GFP-OhyA and DOPG. At a protein-to-lipid concentration ratio slightly less than 1:50, the fast-exchange binding equilibrates, with no further chemical shift changes observed, while the intermediate-exchange peak intensity and broadening reaches its maximum. Two-dimensional 1H–13C HSQC NMR spectra were collected for DOPG LUVs in the presence and absence of GFP-OhyA. The hydrocarbon peaks of the lipids exhibited significant overlap in both conditions, indicating that the lipid structure and conformation remain unchanged upon protein binding (Supplementary Figure S2).
The dissociation constant (KD) for the fast-exchange binding was determined to be 3.181 ± 1.272 μM (Figure 10B). These results suggest that at low concentrations, GFP-OhyA transiently binds and releases from the membrane bilayer (fast exchange). In contrast, at higher protein concentrations, GFP-OhyA forms longer-lasting interactions on the bilayer as the peak intensity decreased, likely as part of an oligomeric complex.
We propose that OhyA exhibits cooperative switching (Duke et al., 2001), where the molecules dynamically transition between individual molecules and oligomers in response to the concentration of nearby membrane-bound proteins. This transition is driven by conformational changes that promote oligomer formation through interactions with other OhyA molecules on the same membrane bilayer. Oligomer assembly is stabilized by membrane binding and destabilized by dissociation. OhyA stochastically switches between these states, with the likelihood of each state dependent on the local concentration of membrane-bound proteins. Membrane binding shifts the equilibrium toward the oligomeric state, as coupling energy from protein nucleation on the bilayer induces conformational changes propagated through allosteric interactions.
The occupancy of membrane-binding sites by individual molecules is a nonlinear function of protein concentration, as membrane affinity increases with the amount of bound protein (Stefan and Le Novere, 2013). Small changes in lipid concentration can disrupt oligomer assembly by increasing the fraction of unbound protein, and separating individual molecules. Under these conditions, transient oligomers form and rapidly dissociate. At higher concentrations of membrane-bound proteins, stable oligomers predominate. At intermediate concentrations, the system exhibits bistability, oscillating between oligomeric assemblies and dispersed molecules. This model underscores the critical roles of membrane binding and cooperative protein-protein interactions in regulating OhyA’s oligomeric switch, driven by conformation-dependent interactions between adjacent protomers.
While the specific intermolecular interactions between OhyA and lipids that drive membrane binding have been previously identified, the equilibrium binding dynamics of OhyA in solution remain unexplored. Traditional binding models often assume a discrete stoichiometry and a Poisson distribution of particles that bind irreversibly, with unbound analyte levels rapidly declining as ligand concentration increases. However, modeling protein•membrane interactions poses a distinct challenge due to the multiple mechanisms influencing the collective biophysical properties of the interaction (White et al., 1998; Mulgrew-Nesbitt et al., 2006; Yeagle, 2014; Richens et al., 2015; Carravilla et al., 2020). Additionally, if binding is reversible, the unbound fraction cannot be ignored.
Using phosphorus-31 (31P) NMR spectroscopy, we observed two distinct binding events between GFP-OhyA and DOPG membranes. Fast-exchange binding, characterized by a significant chemical shift downfield of unbound DOPG, equilibrated at lower protein concentrations (KD = 3.181 ± 1.272 μM). In contrast, intermediate-exchange binding, associated with a distinct upfield peak and significant line broadening, became prominent at higher protein concentrations. These results suggest that GFP-OhyA exhibits transient, reversible interactions with the membrane bilayer at low concentrations and forms more stable, long-lasting interactions at higher concentrations, likely as part of an oligomeric complex.
In this study, we explored how OhyA binds to membrane bilayers in a non-uniform manner, demonstrating that protein distribution on membranes differs significantly when driven by electrostatic attraction versus cooperative assembly into oligomeric complexes. Using fluorescence correlation spectroscopy (FCS), we measured the free diffusion of GFP-OhyA and lipid particles to study OhyA-membrane binding dynamics. Titrations of GFP-OhyA and accessible lipids revealed concentration-dependent variations in OhyA binding to vesicles. Increasing the lipid concentration while keeping the protein concentration constant revealed an exponential decay in the ratio of vesicle-bound protein to accessible lipid concentration, consistent with a dynamic shift in equilibrium. This reflects a transition from cooperative oligomeric binding at higher protein-to-lipid ratios to a fast-exchange binding regime at lower ratios, where the reversible nature of binding limits the fraction of bound protein. As vesicle concentration increases, protein molecules bind primarily through electrostatic interactions, with some sites on the bilayer restricting further assembly of GFP-OhyA subunits due to steric or cooperative constraints.
To further characterize this binding behavior, we analyzed OhyA interactions at high protein-to-lipid ratios. While the binding curves did not exhibit an overtly biphasic pattern, this model enabled the identification of two distinct binding events: an initial fast-exchange binding mode followed by an intermediate-exchange cooperative oligomerization. This two-state binding was observed using 31P NMR and substrate-free vesicles, offering additional insight into the interplay between protein-protein and protein-lipid interactions.
We also examined vesicles of different sizes and compositions to assess whether membrane curvature or substrate specificity influences OhyA binding. Our findings revealed that OhyA exhibits a moderate preference for membranes with lower curvature, where the presence of unsaturated fatty acid substrates slightly enhanced the overall interaction. Notably, the fraction of bound molecules did not asymptotically approach one, likely due to the combined effects of reversible association, steric hindrance, and a dynamic turnover rate governing the interaction. Together, these results underscore the complexity of OhyA-membrane binding and highlight the importance of molar ratios and cooperative mechanisms in regulating protein assembly on lipid bilayers.
OhyA is enriched in S. aureus extracellular vesicles (EVs) formed in response to antimicrobial fatty acids (Kengmo Tchoupa and Peschel, 2020). Our findings indicate that increased membrane curvature has a modest impact on OhyA binding, implying that the curvature associated with vesiculation does not actively recruit OhyA into EVs. GFP-OhyA localization studies in S. aureus cells confirm its membrane association (Radka et al., 2024), suggesting that OhyA’s presence in EVs is likely due to its pre-existing membrane localization rather than active recruitment during vesicle formation.
OhyA requires soluble flavin adenine dinucleotide (FAD) for catalysis (Subramanian et al., 2019; Radka et al., 2021b), and its transient, less favorable interactions with curved vesicle membranes may enable brief excursions into the vesicular cytosol to acquire FAD. Although the impact of FAD binding on OhyA-membrane interactions is unclear, there is evidence of communication between protein domains, with conformational changes in the membrane binding domain occurring in tandem with alterations in the FAD lobe (Oldham et al., 2024; Radka et al., 2024).
In this study, we explored how lipids affect the cooperative biophysical properties of OhyA-membrane binding. Our results, including insights from 31P NMR, provide a dynamic perspective on the dual role of lipids as structural stabilizers for single peripheral membrane protein binding and as facilitators of protein complex assembly. We conclude that mole fraction is the primary driver of OhyA•membrane association.
The original contributions presented in the study are included in the article/Supplementary Material, further inquiries can be directed to the corresponding author.
WL: Conceptualization, Formal Analysis, Writing–original draft, Writing–review and editing, Investigation, Visualization, Methodology. RN: Writing–review and editing, Investigation. AZ: Investigation, Writing–review and editing. JB: Formal Analysis, Methodology, Writing–review and editing. VS: Investigation, Formal Analysis, Methodology, Visualization, Writing–review and editing. CR: Formal Analysis, Writing–review and editing, Investigation, Conceptualization, Funding acquisition, Project administration, Resources, Visualization, Writing–original draft.
The author(s) declare that financial support was received for the research, authorship, and/or publication of this article. This work was supported by National Institutes of Health, United States grants R00AI166116 (CR).
Fluorescence microscopy and data analysis was performed at the University of Kentucky Light Microscopy Core. We thank the College of Pharmacy PharmNMR Center for analytical support. PharmNMR is supported in part by NIH grants S10OD28690 and P20GM130456.
The authors declare that the research was conducted in the absence of any commercial or financial relationships that could be construed as a potential conflict of interest.
The author(s) declare that no Generative AI was used in the creation of this manuscript.
All claims expressed in this article are solely those of the authors and do not necessarily represent those of their affiliated organizations, or those of the publisher, the editors and the reviewers. Any product that may be evaluated in this article, or claim that may be made by its manufacturer, is not guaranteed or endorsed by the publisher.
The content is solely the responsibility of the authors and does not necessarily represent the official views of the National Institutes of Health.
The Supplementary Material for this article can be found online at: https://www.frontiersin.org/articles/10.3389/fmolb.2024.1504373/full#supplementary-material
OhyA, oleate hydratase; FCS, fluorescence correlation spectroscopy; DOPG, dioleoylphosphatidylglycerol; DOPC, dioleoylphosphatidylcholine; SUV, small unilamellar vesicle; LUV, large unilamellar vesicle; NMR, nuclear magnetic resonance.
Campagnola, G., Nepal, K., Schroder, B. W., Peersen, O. B., and Krapf, D. (2015). Superdiffusive motion of membrane-targeting C2 domains. Sci. Rep. 5, 17721. doi:10.1038/srep17721
Carravilla, P., Darre, L., Oar-Arteta, I. R., Vesga, A. G., Rujas, E., de Las Heras-Martinez, G., et al. (2020). The bilayer collective properties govern the interaction of an HIV-1 antibody with the viral membrane. Biophys. J. 118 (1), 44–56. doi:10.1016/j.bpj.2019.11.005
Chalmers, S. J., and Wylam, M. E. (2020). Methicillin-resistant Staphylococcus aureus infection and treatment options. Methods Mol. Biol. 2069, 229–251. doi:10.1007/978-1-4939-9849-4_16
Chen, Y., Munteanu, A. C., Huang, Y. F., Phillips, J., Zhu, Z., Mavros, M., et al. (2009). Mapping receptor density on live cells by using fluorescence correlation spectroscopy. Chemistry 15 (21), 5327–5336. doi:10.1002/chem.200802305
Cho, W., and Stahelin, R. V. (2005). Membrane-protein interactions in cell signaling and membrane trafficking. Annu. Rev. Biophys. Biomol. Struct. 34, 119–151. doi:10.1146/annurev.biophys.33.110502.133337
DiNitto, J. P., Cronin, T. C., and Lambright, D. G. (2003). Membrane recognition and targeting by lipid-binding domains. Sci. STKE 2003 (213), re16. doi:10.1126/stke.2132003re16
Duke, T. A., Le Novere, N., and Bray, D. (2001). Conformational spread in a ring of proteins: a stochastic approach to allostery. J. Mol. Biol. 308 (3), 541–553. doi:10.1006/jmbi.2001.4610
Elson, E. L. (2011). Fluorescence correlation spectroscopy: past, present, future. Biophys. J. 101 (12), 2855–2870. doi:10.1016/j.bpj.2011.11.012
Elson, E. L. (2013). 40 years of FCS: how it all began. Methods Enzymol. 518, 1–10. doi:10.1016/B978-0-12-388422-0.00001-7
Elson, E. L., and Magde, D. (1974). Fluorescence correlation spectroscopy. I. Conceptual basis and theory. Biopolymers 13(1), 1–27. doi:10.1002/bip.1974.360130102
Galbraith, H., Miller, T. B., Paton, A. M., and Thompson, J. K. (1971). Antibacterial activity of long chain fatty acids and the reversal with calcium, magnesium, ergocalciferol and cholesterol. J. Appl. Bacteriol. 34 (4), 803–813. doi:10.1111/j.1365-2672.1971.tb01019.x
Greenway, D. L., and Dyke, K. G. (1979). Mechanism of the inhibitory action of linoleic acid on the growth of Staphylococcus aureus. J. Gen. Microbiol. 115 (1), 233–245. doi:10.1099/00221287-115-1-233
Harwardt, M. I. E., Dietz, M. S., Heilemann, M., and Wohland, T. (2018). SPT and imaging FCS provide complementary information on the dynamics of plasma membrane molecules. Biophys. J. 114 (10), 2432–2443. doi:10.1016/j.bpj.2018.03.013
Huynh, K., and Partch, C. L. (2015). Analysis of protein stability and ligand interactions by thermal shift assay. Curr. Protoc. Protein Sci. 79 (28), 28.9.1–28.9.14. doi:10.1002/0471140864.ps2809s79
Kengmo Tchoupa, A., and Peschel, A. (2020). Staphylococcus aureus releases proinflammatory membrane vesicles to resist antimicrobial fatty acids. mSphere 5 (5), e00804-20–e00820. doi:10.1128/mSphere.00804-20
Kruger, D., Ebenhan, J., Werner, S., and Bacia, K. (2017). Measuring protein binding to lipid vesicles by fluorescence cross-correlation spectroscopy. Biophys. J. 113 (6), 1311–1320. doi:10.1016/j.bpj.2017.06.023
Kuhn, S., Slavetinsky, C. J., and Peschel, A. (2015). Synthesis and function of phospholipids in Staphylococcus aureus. Int. J. Med. Microbiol. 305 (2), 196–202. doi:10.1016/j.ijmm.2014.12.016
Liu, Q., Mazhar, M., and Miller, L. S. (2018). Immune and inflammatory reponses to Staphylococcus aureus skin infections. Curr. Dermatol. Rep. 7 (4), 338–349. doi:10.1007/s13671-018-0235-8
Lomize, A. L., Pogozheva, I. D., Lomize, M. A., and Mosberg, H. I. (2007). The role of hydrophobic interactions in positioning of peripheral proteins in membranes. BMC Struct. Biol. 7, 44. doi:10.1186/1472-6807-7-44
Miyamoto, J., Mizukure, T., Park, S. B., Kishino, S., Kimura, I., Hirano, K., et al. (2015). A gut microbial metabolite of linoleic acid, 10-hydroxy-cis-12-octadecenoic acid, ameliorates intestinal epithelial barrier impairment partially via GPR40-MEK-ERK pathway. J. Biol. Chem. 290 (5), 2902–2918. doi:10.1074/jbc.M114.610733
Mulgrew-Nesbitt, A., Diraviyam, K., Wang, J., Singh, S., Murray, P., Li, Z., et al. (2006). The role of electrostatics in protein-membrane interactions. Biochim. Biophys. Acta 1761 (8), 812–826. doi:10.1016/j.bbalip.2006.07.002
Munro, S. (2002). Organelle identity and the targeting of peripheral membrane proteins. Curr. Opin. Cell. Biol. 14 (4), 506–514. doi:10.1016/s0955-0674(02)00350-2
Murphy, J., Knutson, K., and Hinderliter, A. (2009). Protein-lipid interactions role of membrane plasticity and lipid specificity on peripheral protein interactions. Methods Enzymol. 466, 431–453. doi:10.1016/S0076-6879(09)66018-3
Oldham, M. L., Zuhaib Qayyum, M., Kalathur, R. C., Rock, C. O., and Radka, C. D. (2024). Cryo-EM reconstruction of oleate hydratase bound to a phospholipid membrane bilayer. J. Struct. Biol. 216 (3), 108116. doi:10.1016/j.jsb.2024.108116
Ponmalar, I. I., Cheerla, R., Ayappa, K. G., and Basu, J. K. (2019). Correlated protein conformational states and membrane dynamics during attack by pore-forming toxins. Proc. Natl. Acad. Sci. U. S. A. 116 (26), 12839–12844. doi:10.1073/pnas.1821897116
Prem, S., Helmer, C. P. O., Dimos, N., Himpich, S., Bruck, T., Garbe, D., et al. (2022). Towards an understanding of oleate hydratases and their application in industrial processes. Microb. Cell Fact. 21 (1), 58. doi:10.1186/s12934-022-01777-6
Radka, C. D., Batte, J. L., Frank, M. W., Rosch, J. W., and Rock, C. O. (2021a). Oleate hydratase (OhyA) is a virulence determinant in Staphylococcus aureus. Microbiol. Spectr. 9 (3), e0154621. doi:10.1128/Spectrum.01546-21
Radka, C. D., Batte, J. L., Frank, M. W., Young, B. M., and Rock, C. O. (2021b). Structure and mechanism of Staphylococcus aureus oleate hydratase (OhyA). J. Biol. Chem. 296, 100252. doi:10.1074/jbc.RA120.016818
Radka, C. D., Frank, M. W., Yao, J., Seetharaman, J., Miller, D. J., and Rock, C. O. (2020). The genome of a Bacteroidetes inhabitant of the human gut encodes a structurally distinct enoyl-acyl carrier protein reductase (FabI). J. Biol. Chem. 295 (22), 7635–7652. doi:10.1074/jbc.RA120.013336
Radka, C. D., Grace, C. R., Hasdemir, H. S., Li, Y., Rodriguez, C. C., Rodrigues, P., et al. (2024). The carboxy terminus causes interfacial assembly of oleate hydratase on a membrane bilayer. J. Biol. Chem. 300 (2), 105627. doi:10.1016/j.jbc.2024.105627
Raychowdhury, M. K., Goswami, R., and Chakrabarti, P. (1985). Effect of unsaturated fatty acids in growth inhibition of some penicillin-resistant and sensitive bacteria. J. Appl. Bacteriol. 59 (2), 183–188. doi:10.1111/j.1365-2672.1985.tb03319.x
Rhoades, E., Ramlall, T. F., Webb, W. W., and Eliezer, D. (2006). Quantification of alpha-synuclein binding to lipid vesicles using fluorescence correlation spectroscopy. Biophys. J. 90 (12), 4692–4700. doi:10.1529/biophysj.105.079251
Richens, J. L., Lane, J. S., Bramble, J. P., and O'Shea, P. (2015). The electrical interplay between proteins and lipids in membranes. Biochim. Biophys. Acta 1848 (9), 1828–1836. doi:10.1016/j.bbamem.2015.03.017
Robert, J. N., Priscilla, C. L., Shannon, K. D., James, D. B., and Christopher, D. R. (2024). Independent evolution of oleate hydratase clades in Bacillales reflects molecular convergence. Front. Mol. Biosci. 11, 1485485. doi:10.3389/fmolb.2024.1485485
Saika, A., Nagatake, T., and Kunisawa, J. (2019). Host- and microbe-dependent dietary lipid metabolism in the control of allergy, inflammation, and immunity. Front. Nutr. 6, 36. doi:10.3389/fnut.2019.00036
Santos, N. C., Prieto, M., and Castanho, M. A. (2003). Quantifying molecular partition into model systems of biomembranes: an emphasis on optical spectroscopic methods. Biochim. Biophys. Acta 1612 (2), 123–135. doi:10.1016/s0005-2736(03)00112-3
Stahelin, R. V. (2009). Lipid binding domains: more than simple lipid effectors. J. Lipid Res. 50 (Suppl. l), S299–S304. doi:10.1194/jlr.R800078-JLR200
Stefan, M. I., and Le Novere, N. (2013). Cooperative binding. PLoS Comput. Biol. 9 (6), e1003106. doi:10.1371/journal.pcbi.1003106
Subramanian, C., Frank, M. W., Batte, J. L., Whaley, S. G., and Rock, C. O. (2019). Oleate hydratase from Staphylococcus aureus protects against palmitoleic acid, the major antimicrobial fatty acid produced by mammalian skin. J. Biol. Chem. 294 (23), 9285–9294. doi:10.1074/jbc.RA119.008439
Thomas, F. A., Visco, I., Petrasek, Z., Heinemann, F., and Schwille, P. (2015). Introducing a fluorescence-based standard to quantify protein partitioning into membranes. Biochim. Biophys. Acta 1848 (11 Pt A), 2932–2941. doi:10.1016/j.bbamem.2015.09.001
Vesga, A. G., Villegas, L., Vequi-Suplicy, C. C., Sorzano, C. O. S., and Requejo-Isidro, J. (2023). Quantitative characterization of membrane-protein reversible association using FCS. Biophys. J. 122 (11), 2285–2300. doi:10.1016/j.bpj.2023.01.026
White, S. H., Wimley, W. C., Ladokhin, A. S., and Hristova, K. (1998). Protein folding in membranes: determining energetics of peptide-bilayer interactions. Methods Enzymol. 295, 62–87. doi:10.1016/s0076-6879(98)95035-2
Yang, B., Gao, H., Stanton, C., Ross, R. P., Zhang, H., Chen, Y. Q., et al. (2017). Bacterial conjugated linoleic acid production and their applications. Prog. Lipid Res. 68, 26–36. doi:10.1016/j.plipres.2017.09.002
Yeagle, P. L. (2014). Non-covalent binding of membrane lipids to membrane proteins. Biochim. Biophys. Acta 1838 (6), 1548–1559. doi:10.1016/j.bbamem.2013.11.009
Keywords: oleate hydratase (OhyA), phospholipid, membrane bilayer, fluorescence correlation spectroscopy (FCS), phosphorus nuclear magnetic resonance (31P NMR), membrane binding, lipid-protein interaction
Citation: Lathram WA, Neff RJ, Zalla AN, Brien JD, Subramanian V and Radka CD (2025) Dissecting the biophysical mechanisms of oleate hydratase association with membranes. Front. Mol. Biosci. 11:1504373. doi: 10.3389/fmolb.2024.1504373
Received: 30 September 2024; Accepted: 16 December 2024;
Published: 08 January 2025.
Edited by:
Elena G. Govorunova, University of Texas Health Science Center at Houston, United StatesReviewed by:
Jitendriya Swain, UMR9004 Institut de Recherche en Infectiologie de Montpellier (IRIM), FranceCopyright © 2025 Lathram, Neff, Zalla, Brien, Subramanian and Radka. This is an open-access article distributed under the terms of the Creative Commons Attribution License (CC BY). The use, distribution or reproduction in other forums is permitted, provided the original author(s) and the copyright owner(s) are credited and that the original publication in this journal is cited, in accordance with accepted academic practice. No use, distribution or reproduction is permitted which does not comply with these terms.
*Correspondence: Christopher D. Radka, Y2hyaXN0b3BoZXIucmFka2FAdWt5LmVkdQ==
Disclaimer: All claims expressed in this article are solely those of the authors and do not necessarily represent those of their affiliated organizations, or those of the publisher, the editors and the reviewers. Any product that may be evaluated in this article or claim that may be made by its manufacturer is not guaranteed or endorsed by the publisher.
Research integrity at Frontiers
Learn more about the work of our research integrity team to safeguard the quality of each article we publish.