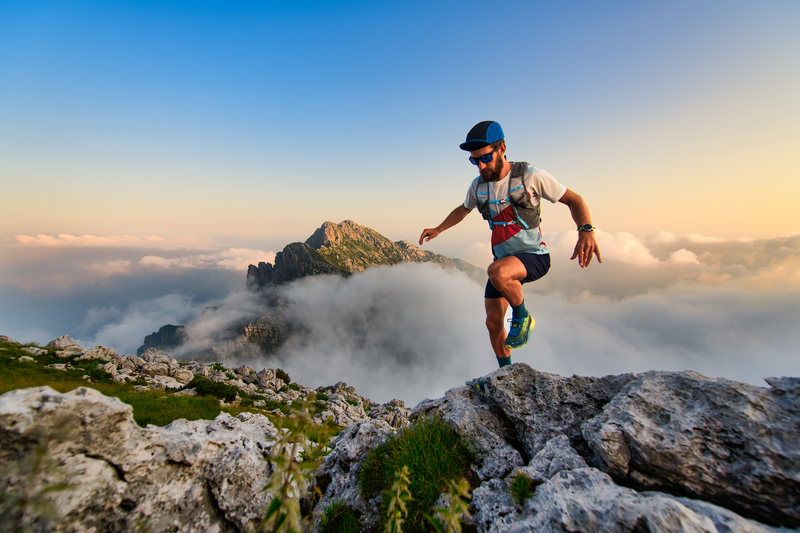
94% of researchers rate our articles as excellent or good
Learn more about the work of our research integrity team to safeguard the quality of each article we publish.
Find out more
ORIGINAL RESEARCH article
Front. Mol. Biosci. , 30 August 2024
Sec. Molecular Diagnostics and Therapeutics
Volume 11 - 2024 | https://doi.org/10.3389/fmolb.2024.1440276
Introduction: Actin-binding proteins (ABPs) are essential for the regulation of morphological plasticity required for tumor cells to metastasize. The aim of this study was to perform an unbiased bioinformatic approach to identify the key ABPs significantly associated with the metastatic potential of breast cancer cells.
Methods: Microarray data from 181 primary breast cancer samples from our hospital were used, and all genes belonging to the Gene Ontology term actin cytoskeleton organization were obtained from QuickGO. Association with metastasis-free survival probability was tested using Cox proportional hazards regression, and pairwise co-expression was tested by Pearson correlations. Differential expression between different subgroups was analyzed using Wilcoxon tests for dichotomous traits and Kruskal–Wallis tests for categorical traits. Validation was performed using four publicly available breast cancer datasets.
Results: ARHGAP25 was significantly associated with a low metastatic potential, and CFL1, TMSB15A, and ACTL8 were significantly associated with a high metastatic potential. A significantly higher expression of CFL1, TMSB15A, and ACTL8 mRNA was found in the more aggressive Her2-positive and triple-negative subtypes as well as in ER-negative samples. Also, these genes were co-expressed in the same tumors. However, only mRNA levels of CFL1 were increased in pN1 compared to pN0 patients. External validation revealed that CFL1 and TMSB15A had significant associations with consistent hazard ratios in two breast cancer cohorts, and among these, CFL1 exhibited the highest hazard ratios.
Conclusion: CFL1 showed the strongest correlation with the metastatic potential of breast tumors. Thus, targeted inhibition of CFL1 might be a promising approach to treat malignant breast cancer cells.
Distant metastasis is the leading cause of cancer-related death in breast cancer and most other solid tumors, and currently, no therapy is available to cure patients suffering from metastasis (Fares et al., 2020). Therefore, the identification and inhibition of metastasis-promoting genes in cancer populations is of great clinical relevance. In order to form distant metastases, tumor cells must undergo strong morphological changes to escape from the primary tumor, invade the tissue and blood vessels, and finally, extravasate from the vessels and form new metastases. This morphological plasticity requires remodeling of the actin cytoskeleton, which is controlled by many actin-binding proteins (ABPs). During amoeboid and mesenchymal migration, as well as during invasion, the tumor cells form different kinds of protrusions at the leading edges, requiring the formation, bundling, and cross-linking of actin filaments (F-actin) at the tips of the cells. Also, F-actin is cleaved to provide new actin monomers (G-actin) for F-actin elongation, and actin-myosin contraction provides the force needed for cell migration and invasion. In addition, some ABPs only bind to G-actin; they sequester monomeric actin or promote its polymerization to F-actin (Olson and Sahai, 2009; Pollard, 2016; Lappalainen et al., 2022).
The ABPs regulating these F-actin dynamics include proteins promoting elongation or formation of F-actin (Arp2/3-complex, formins, vasodilator-stimulated phosphoprotein (VASP)), bundling and/or cross-linking F-actin (Fascin, Plastins, Actinins, Inositol 1,4,5-trisphosphate 3-kinase-A (ITPKA)), capping actin (CAP-Z, gelsolin), binding to actin monomers (Thymosin, Profilin), or severe F-actin (Cofilin and Gelsolin). Most of these ABPs are inactive in resting cells and are stimulated by the RhoGTPases Rac-1, Cdc42, and Rho-A, but some of them are constitutively targeted to F-actin (Pollard, 2016; Windhorst et al., 2017).
Because of the important role of actin dynamic regulation for cancer cell metastasis, the expression and/or activity of several ABPs are upregulated in malignant cancer cells, and it could be shown that this upregulation is associated with cancer cell metastasis. These proteins include the actin-bundling proteins Fascin-1, L-Plastin, Cortactin, and ITPKA, the ENA/VASP proteins, the F-actin severing protein Cofilin 1, and many more (Olson and Sahai, 2009; Stevenson et al., 2012; Windhorst et al., 2017; Izdebska et al., 2020). Interestingly, most of these proteins are not expressed in the corresponding normal tissue and are thus suitable targets for tumor therapy (Stevenson et al., 2012; Windhorst et al., 2017; Izdebska et al., 2020).
An unbiased bioinformatic approach was conducted in this study to reveal which ABPs are most frequently expressed in breast cancer cells with high metastatic potential. Our goal was to identify those ABPs whose upregulation correlates most strongly with the potential of breast cancer cells to form metastases. Thereby, we intended to identify the most effective ABP targets for anti-cancer therapy.
The discovery (in-house) cohort included microarray data from 194 primary, untreated breast cancer patients, of whom metastasis-free survival data were available for 181 (Milde-Langosch et al., 2014). Briefly, all patients were treated at the University Medical Centre Hamburg-Eppendorf, Germany, Department of Gynecology between 1991 and 2002 and gave written approval for the utilization of their tissue samples and the reviewing of their medical records according to our investigational review board and ethics committee guidelines (Ethik-Kommission der Ärztekammer Hamburg, #OB/V/03). RNA was isolated from snap-frozen tissue samples, quantified using an Affymetrix (Santa Clara, CA, United States) HG-U133A array on a GeneChip System, and pre-processed as described elsewhere (Milde-Langosch et al., 2014).
Suitable validation cohorts with transcriptome data from primary breast cancer samples with follow-up of metastasis-free survival over at least 5 years were retrieved from the Gene Expression Omnibus (GEO) (Barrett et al., 2013). Four cohorts were identified and used for validation of the discovery findings, including GSE11121 (Schmidt et al., 2008), GSE6532 (Loi et al., 2007), GSE 2034 (Wang et al., 2005), and GSE21653 (Sabatier et al., 2011).
The pre-processing and data analysis were performed in R version 4.2.3. Expression intensities of the discovery cohort were log2-transformed and quantile-normalized. Microarray data from the validation cohorts were retrieved from GEO using the R/Bioconductor package GEOquery (Davis and Meltzer, 2007). The optimal pre-processing strategy for each dataset was evaluated for the effect on global variation based on principal component analysis (PCA). Probe intensities were log2-transformed and quantile-normalized for the studies GSE11121, GSE2034, and GSE21653. In the GSE6532 dataset, the PCA showed strong clusters of different batches represented by the variable characteristics_ch1.2, which was subsequently used as a batch variable in the batch effect removal function ComBat (Johnson et al., 2007) from the R/Bioconductor package sva (Leek et al., 2012).
All genes belonging to the Gene Ontology term “actin cytoskeleton organization” (GO:0030036) were obtained from QuickGO (Binns et al., 2009) and filtered for presence in the transcriptome dataset of the discovery cohort. The remaining list was manually filtered for those directly interacting with actin or belonging to the RhoGTPases and their regulators. The mRNA of each ABP was tested for association with metastasis-free survival probability using Cox proportional hazards regression adjusted for age at baseline examination. Genes with a false discovery rate (FDR) ≤ 0.05 were considered significant and considered potential candidates. The mRNA of these genes was tested for association with metastasis-free survival probability in the validation cohorts. Age at baseline examination was only used as a covariate in the Cox model in GSE6532 and GSE21653 because it was not available in GSE11121 and GSE 2034. Genes with a p-value ≤0.05 and a consistent effect direction, compared to the discovery, were considered candidates. Candidate gene expression was stratified based on the first quartile for hazard ratios smaller than one, and otherwise, the third quartile was used and visualized as Kaplan–Meier curves, including p-values from the log-rank tests.
The mRNA of each potential candidate was tested for differential expression between different subgroups of the discovery cohort. Wilcoxon tests were applied for dichotomous traits, and Kruskal–Wallis tests were applied for categorical traits. Pairwise co-expression of all candidate genes was tested using Pearson correlations.
In order to identify the set of APBs upregulated in highly metastatic breast cancer cells, all annotations for the Gene Ontology term “actin cytoskeleton organization” (GO:0030036) were obtained from QuickGO (Binns et al., 2009) (Figure 1A). This category contained 666 unique genes, which were filtered for the presence in our transcriptome dataset derived from 181 breast cancer patients. Thereafter, a list of 342 genes was manually curated by selecting those directly interacting with actin (ABPs) or belonging to the RhoGTPases and their regulators (Figure 1B).
Figure 1. Study design of the discovery phase. (A) Ancestor chart of the Gene Ontology term “Actin cytoskeleton organization” (GO:0030036), which was used to select genes for the analysis. (B) Workflow and results for the discovery phase.
The in-house transcriptome dataset was used as a discovery cohort, and all 342 ABP-related genes were screened for associations to metastasis-free survival using Cox proportional hazards regression adjusted for age at baseline examination. For evaluation, we defined a false discovery rate (FDR) ≤ 0.05. Interestingly, only four genes were considered significant: Cofilin 1 (CFL1), Thymosin beta15A (TMSB15A), Rho GTPase activating protein 25 (ARHGAP25), and actin like 8 (ACTL8). Among these, a high mRNA expression of CFL1, TMSB15A, and ACTL8 was positively associated with metastasis formation, whereas ARHGAP25 was associated with lower metastatic rates.
Further, patients were divided into quartiles according to the mRNA level of each candidate gene, and Kaplan–Meier analyses and log-rank tests were performed using cut-offs described in materials and methods. Here, a significant association with metastasis-free survival was confirmed for CFL1 and TMSB15A, while no prognostic value could be observed for ACTL8 and ARHGAP25 (Figure 2).
Figure 2. Expression of candidate ABPs in relation to metastasis-free survival. The mRNA expression of candidate genes was stratified based on the second tertile for genes with a hazard ratio >1 (ACTL8, CFL1, TMSB15A) and based on the first tertile if the hazard ratio was <1 (ARHGAP25) and the relationship to metastasis-free survival probability was depicted in Kaplan–Meier curves. p-values refer to the log-rank test after dichotomization.
In summary, among 342 genes coding for ABPs, four are significantly associated with the probability of breast tumors forming distant metastases.
To analyze the relevance of ABP co-expression for malignancy in breast cancer, cluster analysis including all four candidates was performed within the following four subcohorts: Patients who developed metastasis before 5, between 5–10, between 10–15, or after 15 years. In Figure 3A each rectangle represents one patient, and dark brown represents a high ABP mRNA level, while dark blue represents a low ABP mRNA level. The result of this analysis revealed a clustering of high CFL1, TMSB15A, and ACTL8 in patients who developed metastasis within 5 years, and low levels in patients who did not suffer from metastasis within 15 years. For ARHGAP25, we observed the opposite pattern.
Figure 3. Cluster and co-expression analysis of ABPs. (A) The four ABPs whose mRNA expression significantly correlates with the metastatic potential of breast cancer cells were clustered according to the time-course of metastasis formation. (B) Pairwise correlations between mRNA expression of ABPs in tumor samples of the discovery cohort. Pearson correlation coefficients with a Bonferroni-corrected p-value >0.05 are crossed out.
In addition, correlation analysis of ABP transcripts revealed a significant positive association between ACTL8 with CFL1 and TMSB15A, suggesting the co-expression of these proteins in highly metastatic tumors. On the other hand, CFL1 and TMSB15A were not co-expressed, and ARHGAP25 did not show any co-expression with the other ABPs. Thus, mRNAs of ABPs show distinct co-expression profiles (Figure 3B).
In order to analyze whether the genes encoding ABPs are preferentially expressed by certain cancer subtypes, differential mRNA expression analyses were performed for lymph node status (N0: no lymph nodes are affected; N1: at least one lymph node is affected), estrogen receptor status (ER+/−: estrogen receptor-positive/negative) and the molecular subtype at baseline (luminal, HER2-positive, triple-negative breast cancer). As shown in Figure 4, all ABPs significantly correlating with the ability of the primary tumor to form distant metastases were preferentially expressed by HER2-positive and triple-negative breast cancer, even ARHGAP25 showing an inverse correlation with metastasis-free survival probability. Also, the mRNA levels of CFL1, TMSB15A, ACTL8, and ARHGAP25 were higher in ER− than ER+. However, only CFL1 mRNA was significantly higher in pN1 than in pN0 tumors.
Figure 4. Distributions of ABPs encoding gene expression by lymph node and estrogen receptor status at baseline and molecular breast cancer subtype. (A) The mRNA levels of ABPs were tested for differences regarding the ability of cancer cells to form lymph node metastasis; N0: No lymph node involvement; N1: At least one lymph node is involved. (B) Differential expression between estrogen receptor (ER) expression positive and negative breast cancer samples. (C) Differences between Her2-positive, luminal, and triple-negative breast cancer subtypes. In A-B, differences in the mean mRNA expression were tested using Wilcoxon tests, and in C, Kruskal–Wallis tests were performed. Statistical significance is indicated by ns (not significant) p-value >0.05, * p-value ≤0.05, ** p-value ≤0.01, *** p-value ≤0.001, **** p-value ≤0.0001.
In conclusion, the ABPs whose mRNA level is associated with a high metastatic potential are preferentially expressed by more aggressive breast cancer subtypes.
In order to validate the candidate genes in other breast cancer cohorts, their expression was tested for associations with metastasis-free survival probability in the GEO data sets GSE11121 (Schmidt et al., 2008), GSE6532 (Loi et al., 2007), GSE 2034 (Wang et al., 2005), and GSE21653 (Sabatier et al., 2011). Here, GSE6532 and GSE1653 included the same patient information as our “discovery” cohort, while only lymph node-negative patients were included in GSE11121 and GSE20234. Furthermore, GSE2034 only contained samples from ER-positive tumors, while in GSE11121, no data about the hormone status was available (Table 1). One of four candidate genes was captured in GSE6532, CFL1.
Table 1. Clinical data of Discovery and the publicly available breast cancer cohorts GSE11121, GSE6532, GSE2034, GSE21653.
Among the four initially identified ABPs in the discovery cohort (Figure 1), two met the replication criteria with a p-value ≤0.05 and a consistent direction of the hazard ratio compared to the discovery in at least two further cohorts (Figure 5A). The estimated hazard ratio of CFL1 mRNA was 8.4 (95% CI: 3.2–21.8) in the discovery, 4.2 (95% CI: 1.0–16.9) in GSE11121, and 4.0 (95% CI: 1.4–11.5) in GSE6532, while that of TMSB15A was 1.4 (95% CI: 1.2–1.6) in the discovery, 1.3 (95% CI: 1.1–1.6) in GSE11121, and 1.1 (95% CI: 1.0–1.3) in GSE 2034. On the other hand, Kaplan–Meier analysis revealed that only CFL1 showed a significant correlation with low metastasis-free survival probability in GSE6532 (Figure 5B).
Figure 5. Summary of metastasis-free survival analyses in the discovery and validation cohorts. (A) Forest plots of the four candidate genes showing hazard ratios, 95% confidence intervals, p-values, and sample sizes of the discovery and three to four published breast cancer cohorts. In GSE6532, only the mRNA of CFL1 was captured. (B) Kaplan–Meier curves stratified based on the second tertile of candidate genes meeting the validation criteria in relation to metastasis-free survival probabilities. Red rectangles highlight the validation cohorts that led to the successful validation of the corresponding genes.
In summary, among all ABPs analyzed in this study, a high mRNA level of Cofilin 1 showed the strongest association with the metastatic potential of breast cancer tumors (Figure 6).
It has already been shown that a high level of several actin-binding proteins (ABP) correlates with breast cancer metastases (Izdebska et al., 2020). However, until now, a ranking of these proteins based on their importance in association with cancer malignancy has not been established. Because this approach enables the identification of the best-suited targets for cancer therapy, an unbiased bioinformatic method using five independent transcriptome data sets was conducted in this study to identify those ABPs with key roles in highly metastatic breast cancer cells. For this purpose, we selected all genes from the Gene Ontology term actin cytoskeleton organization and manually curated a list of ABPs directly interacting with actin (ABPs) or belonging to the RhoGTPases and their regulators. To analyze potential associations with the metastatic potential of breast cancer cells, their mRNA levels were correlated with the metastasis-free survival probability of breast cancer patients from our in-house cohort. Thereby, we identified four different genes, among which three were significantly associated with shorter (CFL1, TMSB15A, and ACTL8) and one with longer metastasis-free survival (ARHGAP25). In subsequent Kaplan–Meier analysis, two genes remained significant: CFL1 and TMSB15A.
Rho GTPase activating protein 25 (ARHGAP25) was the only ABP associated with a low metastatic potential of breast cancer cells. ARHGAP25 is a negative regulator of the RhoGTPase Rac1, and in neutrophils, it negatively regulates phagocytosis by controlling the actin cytoskeleton (Csepanyi-Komi et al., 2012). In lung cancer cells, a high ARHGAP25 level exhibits tumor suppressor activity and thus increases the overall survival of lung cancer patients (Xu et al., 2019; Shi et al., 2022). Also, in breast cancer tissues, ARHGAP25 is downregulated relative to normal tissues, and its knockdown in breast cancer cell lines decreased malignancy (Han et al., 2023). These data indicate that ARHGAP25 may exhibit tumor suppressor activity. However, our analysis did not show a significant association in Kaplan–Meier analysis, and the aggressive breast cancer phenotypes (Her2+ and triple-negative) showed a slightly increased ARHGAP25 mRNA level compared to luminal breast cancer. Furthermore, the result obtained from our discovery cohort could not be validated in further breast cancer cohorts. Therefore, future studies are necessary to validate the prognostic value of ARHGAP25 in breast cancer.
CFL1, TMSB15A, and ACTL8 were significantly associated with a high metastatic potential. Among these, CFL exhibited the highest hazard ratio of 8, and only CFL1 mRNA was significantly increased in pN1 compared to pN0 tumors. Cofilin 1 (CFL1) is an F-actin severing protein that promotes actin turnover and is, therefore, essential for cell motility. According to the current model, the actin subunits provided by Cofilin 1-mediated actin cleavage are used by the Arp2/3 complex and by VASP to elongate F-actin at the tips of cellular protrusions and thereby drive migration and invasion of cancer cells (Wang et al., 2007). Moreover, many studies showed a clear correlation between high CFL1 expression and aggressive progression of cancer cells [reviewed in Wang et al. (2007), Coumans et al. (2018), Sidani et al. (2007), Xu et al. (2021)], supporting our finding that CFL1 highly significantly correlates with the ability of breast cancer cells to form metastases.
In addition to CFL1, the mRNAs of TMSB15A and ACTL8 show significant HR results.
Like CFL1, they were more highly expressed in subtypes with more aggressive behavior, but their mRNA level was not significantly different between pN0 and pN1 tumors. Thymosins are G-actin sequestering proteins, saving a free cellular G-actin pool and thereby inhibiting F-actin polymerization. Together with the G-actin-binding protein Profilin, which promotes actin polymerization, the Thymosins control directed F-actin elongation, and that is why their binding to G-actin is tightly regulated by cellular stimulation. Darb-Esfahani et al. (2012) revealed that TMSB15A is a predictor of chemotherapy response in triple-negative breast cancer, and Zhang et al. (2017) found elevated levels of TMSB10 in breast cancer tissues as well as a significant correlation with metastasis status and poor prognosis. In melanoma cells, TMSB4 regulates focal adhesion formation as well as migration and invasion (Makowiecka et al., 2019). Thus, different Thymosin isoforms seem to be involved in the malignant progression of breast cancer cells. The cancer Testis antigen ACTL8 shows a similar hazard ratio as TMSB15A (1.36) and is co-expressed with CFL1 and TMSB15A, but it was not found to be significant in Kaplan–Meier analysis. ACTL8 is upregulated in different tumor types (Yang et al., 2022), and its knock-down inhibited malignancy of lung cancer A549 cells (Ma et al., 2019) as well as of triple-negative breast cancer cells (Fan et al., 2021). However, to our knowledge, its role in actin dynamics has not been investigated. Furthermore, among the ABPs whose high mRNA level showed an association with a high metastatic potential of the primary tumors, ACTL8 exhibited the lowest significance. Thus, similar to ARHGAP25, its prognostic value for breast cancer patients is rather weak.
Among our four initially identified hits, only TMSB15A and CFL1 showed a significant association with metastasis-free survival in further validation cohorts. Both genes could be validated in the cohort GSE11121, which includes only nodal-negative patients who did not receive any systemic therapy. Interestingly, TMSB15A also showed a significant prognostic value in a second cohort (GSE 2034) with similar characteristics, and for CFL1, we observed a strong trend with a borderline significant value in this cohort. GSE2034 includes mainly ER-positive, luminal breast cancer patients. Additionally, a significant association with increased metastatic potential, identified in Kaplan–Meier and log-rank tests, could be determined for CFL1 in the GSE6532 cohort, showing similar characteristics to the discovery cohort. This cohort includes both luminal and basal subtype patients treated with standard hormonal therapy and chemotherapy.
In conclusion, among the ABPs identified in this study as being associated with a strong metastatic potential of breast cancer cells, CFL1 showed the most robust results. It exhibited the highest hazard ratio in our in-house and two published breast cancer cohorts. Also, a high CFL1 mRNA level was associated with malignant breast cancer subtypes, and CFL1 expression was highest in breast cancer populations that formed metastases within 5 years. In the literature, CFL1 has been described to play a key role in the malignant progression of cancer cells (reviewed in Wang et al. (2007), Coumans et al. (2018), and Xu et al. (2021)). Thus, among the ABPs associated with the malignant progression of cancer cells, Cofilin 1 seems to be a key player, and it would be of high interest to develop therapeutics specifically targeting Cofilin 1.
The datasets presented in this study can be found in online repositories. The names of the repository/repositories and accession number(s) can be found in the article/Supplementary Material.
The studies involving humans were approved by the Ethics Committee Board of Aerztekammer Hamburg, #OB/V/03. The studies were conducted in accordance with the local legislation and institutional requirements. The participants provided their written informed consent to participate in this study. Written informed consent was obtained from the individual(s) for the publication of any potentially identifiable images or data included in this article.
CM: writing–review and editing, software, methodology, investigation, formal analysis. LO-F: writing–review and editing, supervision. VM: writing–review and editing, resources. BS: writing–review and editing, resources. SW: writing–original draft, conceptualization.
The author(s) declare that financial support was received for the research, authorship, and/or publication of this article. This study was supported by the University Medical Center Hamburg-Eppendorf.
The authors declare that the research was conducted in the absence of any commercial or financial relationships that could be construed as a potential conflict of interest.
All claims expressed in this article are solely those of the authors and do not necessarily represent those of their affiliated organizations, or those of the publisher, the editors and the reviewers. Any product that may be evaluated in this article, or claim that may be made by its manufacturer, is not guaranteed or endorsed by the publisher.
The Supplementary Material for this article can be found online at: https://www.frontiersin.org/articles/10.3389/fmolb.2024.1440276/full#supplementary-material
Barrett, T., Wilhite, S. E., Ledoux, P., Evangelista, C., Kim, I. F., Tomashevsky, M., et al. (2013). NCBI GEO: archive for functional genomics data sets--update. Nucleic Acids Res. 41 (Database issue), D991–D995. doi:10.1093/nar/gks1193
Binns, D., Dimmer, E., Huntley, R., Barrell, D., O'Donovan, C., and Apweiler, R. (2009). QuickGO: a web-based tool for Gene Ontology searching. Bioinformatics 25 (22), 3045–3046. doi:10.1093/bioinformatics/btp536
Coumans, J. V. F., Davey, R. J., and Moens, P. D. J. (2018). Cofilin and profilin: partners in cancer aggressiveness. Biophys. Rev. 10 (5), 1323–1335. doi:10.1007/s12551-018-0445-0
Csepanyi-Komi, R., Sirokmany, G., Geiszt, M., and Ligeti, E. (2012). ARHGAP25, a novel Rac GTPase-activating protein, regulates phagocytosis in human neutrophilic granulocytes. Blood 119 (2), 573–582. doi:10.1182/blood-2010-12-324053
Darb-Esfahani, S., Kronenwett, R., von Minckwitz, G., Denkert, C., Gehrmann, M., Rody, A., et al. (2012). Thymosin beta 15A (TMSB15A) is a predictor of chemotherapy response in triple-negative breast cancer. Br. J. Cancer 107 (11), 1892–1900. doi:10.1038/bjc.2012.475
Davis, S., and Meltzer, P. S. (2007). GEOquery: a bridge between the gene expression Omnibus (GEO) and BioConductor. Bioinformatics 23 (14), 1846–1847. doi:10.1093/bioinformatics/btm254
Fan, S., Yan, S., Yang, Y., Shang, J., and Hao, M. (2021). Actin-like protein 8 promotes the progression of triple-negative breast cancer via activating PI3K/AKT/mTOR pathway. Onco Targets Ther. 14, 2463–2473. doi:10.2147/OTT.S291403
Fares, J., Fares, M. Y., Khachfe, H. H., Salhab, H. A., and Fares, Y. (2020). Molecular principles of metastasis: a hallmark of cancer revisited. Signal Transduct. Target Ther. 5 (1), 28. doi:10.1038/s41392-020-0134-x
Han, S., Jin, X., Hu, T., and Chi, F. (2023). ARHGAP25 suppresses the development of breast cancer by an ARHGAP25/Wnt/ASCL2 feedback loop. Carcinogenesis 44 (5), 369–382. doi:10.1093/carcin/bgad042
Izdebska, M., Zielinska, W., Halas-Wisniewska, M., and Grzanka, A. (2020). Involvement of actin and actin-binding proteins in carcinogenesis. Cells 9 (10), 2245. doi:10.3390/cells9102245
Johnson, W. E., Li, C., and Rabinovic, A. (2007). Adjusting batch effects in microarray expression data using empirical Bayes methods. Biostatistics 8 (1), 118–127. doi:10.1093/biostatistics/kxj037
Lappalainen, P., Kotila, T., Jegou, A., and Romet-Lemonne, G. (2022). Biochemical and mechanical regulation of actin dynamics. Nat. Rev. Mol. Cell Biol. 23 (12), 836–852. doi:10.1038/s41580-022-00508-4
Leek, J. T., Johnson, W. E., Parker, H. S., Jaffe, A. E., and Storey, J. D. (2012). The sva package for removing batch effects and other unwanted variation in high-throughput experiments. Bioinformatics 28 (6), 882–883. doi:10.1093/bioinformatics/bts034
Loi, S., Haibe-Kains, B., Desmedt, C., Lallemand, F., Tutt, A. M., Gillet, C., et al. (2007). Definition of clinically distinct molecular subtypes in estrogen receptor-positive breast carcinomas through genomic grade. J. Clin. Oncol. 25 (10), 1239–1246. doi:10.1200/JCO.2006.07.1522
Ma, S., Qiang, G., Shao, W., Liang, C., Wang, X., and Liu, D. (2019). Knockdown of actin-like 8 inhibits cell proliferation by regulating FOXM1, STMN1, PLK1, and BIRC5 in lung adenocarcinoma A549 cells. Transl. Cancer Res. 8 (5), 1975–1984. doi:10.21037/tcr.2019.09.33
Makowiecka, A., Malek, N., Mazurkiewicz, E., Mrowczynska, E., Nowak, D., and Mazur, A. J. (2019). Thymosin β4 regulates focal adhesion formation in human melanoma cells and affects their migration and invasion. Front. Cell Dev. Biol. 7, 304. doi:10.3389/fcell.2019.00304
Milde-Langosch, K., Karn, T., Schmidt, M., zu Eulenburg, C., Oliveira-Ferrer, L., Wirtz, R. M., et al. (2014). Prognostic relevance of glycosylation-associated genes in breast cancer. Breast Cancer Res. Treat. 145 (2), 295–305. doi:10.1007/s10549-014-2949-z
Olson, M. F., and Sahai, E. (2009). The actin cytoskeleton in cancer cell motility. Clin. Exp. Metastasis 26 (4), 273–287. doi:10.1007/s10585-008-9174-2
Pollard, T. D. (2016). Actin and actin-binding proteins. Cold Spring Harb. Perspect. Biol. 8 (8), a018226. doi:10.1101/cshperspect.a018226
Sabatier, R., Finetti, P., Cervera, N., Lambaudie, E., Esterni, B., Mamessier, E., et al. (2011). A gene expression signature identifies two prognostic subgroups of basal breast cancer. Breast Cancer Res. Treat. 126 (2), 407–420. doi:10.1007/s10549-010-0897-9
Schmidt, M., Bohm, D., von Torne, C., Steiner, E., Puhl, A., Pilch, H., et al. (2008). The humoral immune system has a key prognostic impact in node-negative breast cancer. Cancer Res. 68 (13), 5405–5413. doi:10.1158/0008-5472.CAN-07-5206
Shi, F., Wu, J., Jia, Q., Li, K., Li, W., Shi, Y., et al. (2022). Relationship between the expression of ARHGAP25 and RhoA in non-small cell lung cancer and vasculogenic mimicry. BMC Pulm. Med. 22 (1), 377. doi:10.1186/s12890-022-02179-5
Sidani, M., Wessels, D., Mouneimne, G., Ghosh, M., Goswami, S., Sarmiento, C., et al. (2007). Cofilin determines the migration behavior and turning frequency of metastatic cancer cells. J. Cell Biol. 179 (4), 777–791. doi:10.1083/jcb.200707009
Stevenson, R. P., Veltman, D., and Machesky, L. M. (2012). Actin-bundling proteins in cancer progression at a glance. J. Cell Sci. 125 (Pt 5), 1073–1079. doi:10.1242/jcs.093799
Wang, W., Eddy, R., and Condeelis, J. (2007). The cofilin pathway in breast cancer invasion and metastasis. Nat. Rev. Cancer 7 (6), 429–440. doi:10.1038/nrc2148
Wang, Y., Klijn, J. G., Zhang, Y., Sieuwerts, A. M., Look, M. P., Yang, F., et al. (2005). Gene-expression profiles to predict distant metastasis of lymph-node-negative primary breast cancer. Lancet 365 (9460), 671–679. doi:10.1016/S0140-6736(05)17947-1
Windhorst, S., Song, K., and Gazdar, A. F. (2017). Inositol-1,4,5-trisphosphate 3-kinase-A (ITPKA) is frequently over-expressed and functions as an oncogene in several tumor types. Biochem. Pharmacol. 137, 1–9. doi:10.1016/j.bcp.2017.03.023
Xu, J., Huang, Y., Zhao, J., Wu, L., Qi, Q., Liu, Y., et al. (2021). Cofilin: a promising protein implicated in cancer metastasis and apoptosis. Front. Cell Dev. Biol. 9, 599065. doi:10.3389/fcell.2021.599065
Xu, K., Liu, B., and Ma, Y. (2019). The tumor suppressive roles of ARHGAP25 in lung cancer cells. Onco Targets Ther. 12, 6699–6710. doi:10.2147/OTT.S207540
Yang, P., Qiao, Y., Meng, M., and Zhou, Q. (2022). Cancer/testis antigens as biomarker and target for the diagnosis, prognosis, and therapy of lung cancer. Front. Oncol. 12, 864159. doi:10.3389/fonc.2022.864159
Keywords: actin, metastasis, actin-binding proteins, bioinformatics, breast cancer
Citation: Müller C, Oliveira-Ferrer L, Müller V, Schmalfeldt B and Windhorst S (2024) Transcriptome-based identification of key actin-binding proteins associated with high metastatic potential in breast cancer. Front. Mol. Biosci. 11:1440276. doi: 10.3389/fmolb.2024.1440276
Received: 30 May 2024; Accepted: 05 August 2024;
Published: 30 August 2024.
Edited by:
Oscar Maiques, Queen Mary University of London, United KingdomReviewed by:
Felipe Carlos Martin Zoppino, CONICET Institute of Medicine and Experimental Biology of Cuyo (IMBECU), ArgentinaCopyright © 2024 Müller, Oliveira-Ferrer, Müller, Schmalfeldt and Windhorst. This is an open-access article distributed under the terms of the Creative Commons Attribution License (CC BY). The use, distribution or reproduction in other forums is permitted, provided the original author(s) and the copyright owner(s) are credited and that the original publication in this journal is cited, in accordance with accepted academic practice. No use, distribution or reproduction is permitted which does not comply with these terms.
*Correspondence: Sabine Windhorst, cy53aW5kaG9yc3RAdWtlLmRl
Disclaimer: All claims expressed in this article are solely those of the authors and do not necessarily represent those of their affiliated organizations, or those of the publisher, the editors and the reviewers. Any product that may be evaluated in this article or claim that may be made by its manufacturer is not guaranteed or endorsed by the publisher.
Research integrity at Frontiers
Learn more about the work of our research integrity team to safeguard the quality of each article we publish.