- 1Laboratorio de Interacción Hospedero Patógeno, Unidad de Biología Molecular, Institut Pasteur de Montevideo, Montevideo, Uruguay
- 2Departamento de Química del Litoral, Universidad de la República, Paysandú, Uruguay
- 3Grupo de Resonancia Magnética Nuclear e Imagen en Biomedicina, Instituto Pluridisciplinar, Universidad Complutense de Madrid, Madrid, Spain
- 4Departamento de Química en Ciencias Farmacéuticas, Facultad de Farmacia, Universidad Complutense de Madrid, Madrid, Spain
- 5Centro de Investigación Biomédica en Red de Enfermedades Respiratorias (CIBERES), Instituto de Salud Carlos III, Madrid, Spain
- 6Centro de Referencia COVID 1, Hospital Español, Administración de Servicios de Salud del Estado, Montevideo, Uruguay
- 7Centro de Referencia COVID 2, Instituto Nacional de Ortopedia y Traumatología, Administración de Servicios de Salud del Estado, Montevideo, Uruguay
COVID-19 was the most significant infectious-agent-related cause of death in the 2020-2021 period. On average, over 60% of those admitted to ICU facilities with this disease died across the globe. In severe cases, COVID-19 leads to respiratory and systemic compromise, including pneumonia-like symptoms, acute respiratory distress syndrome, and multiorgan failure. While the upper respiratory tract and lungs are the principal sites of infection and injury, most studies on the metabolic signatures in COVID-19 patients have been carried out on serum and plasma samples. In this report we attempt to characterize the metabolome of lung parenchyma extracts from fatal COVID-19 cases and compare them with that from other respiratory diseases. Our findings indicate that the metabolomic profiles from fatal COVID-19 and non-COVID-19 cases are markedly different, with the former being the result of increased lactate and amino acid metabolism, altered energy pathways, oxidative stress, and inflammatory response. Overall, these findings provide additional insights into the pathophysiology of COVID-19 that could lead to the development of targeted therapies for the treatment of severe cases of the disease, and further highlight the potential of metabolomic approaches in COVID-19 research.
1 Introduction
As experienced during the 2020–2023 COVID-19 pandemic, SARS-CoV-2 infections can result in a variety of respiratory conditions, including pneumonias-like symptoms, acute respiratory distress syndrome (ARDS), and multiorgan failure (Chavez et al., 2021). Potential risk factors for mortality among patients admitted to ICU included age, obesity, and comorbidities such as hypertension, diabetes, and cardiovascular disease (Ejaz et al., 2020; Ahlström et al., 2021; Booth et al., 2021). It was also observed that the clinical symptoms of COVID-19 could be influenced by viral load as well as by respiratory and gut microbiota dysbiosis (Liu et al., 2020; Brosseau et al., 2021). While most of the patients diagnosed with COVID-19 attended the disease at home, 13%-14% needed hospitalization in moderate care facilities, and between 5%-6% were admitted to intensive care units (Verity et al., 2020; Gosangi et al., 2022). Hospital mortality was between 30%–60% in case series reported in the first wave, increasing significantly for patients admitted to the ICU who required mechanical ventilation (Abate et al., 2020; Bastos et al., 2021; Estenssoro et al., 2021; Kurtz et al., 2021; Ranzani et al., 2021; Dongelmans et al., 2022). Uruguay was no exception, and towards the end of 2020 the average number of new cases increased exponentially to over 400 cases per day (GUIAD-COVID-19, 2022). In addition, the most prevalent viral variant during the first wave was B.1.1.28 (now designated as P.6), and vaccines were not yet available (Moreno et al., 2020; Elizondo et al., 2021; Rego et al., 2021).
A number of studies have established that SARS-CoV-2 infections set off a chain of events that can lead to a cytokine storm, an immune system overreaction that may result in ARDS (Koçak Tufan et al., 2021), which is the most frequent complication of severe COVID-19 cases. However, there are still several aspects of the disease that remain unknown. In order to elucidate the pathophysiological effects of COVID-19 and improve clinical care through the selection of appropriate treatments, particularly for patients with severe manifestations of the disease, a thorough understanding of the metabolic alterations and early acute lung injury biomarkers are required.
Metabolomic profiling can complement the lack of knowledge regarding the molecular mechanisms underlying clinical manifestations and pathogenesis of COVID-19. Consequently, several studies have employed metabolomic approaches to better understand the metabolic pathways involved in COVID-19 pathogenesis (Ansone et al., 2021; Chen et al., 2022; Murali et al., 2023). Serum-based metabolomic studies in COVID-19 patients revealed altered glycolytic pathways as well as amino acid, lipid, and anaplerotic metabolism, suggesting an impact on energy pathways, inflammatory response, and oxidative stress, and confirming the systemic nature of the disease (Kimhofer et al., 2020; Lorente et al., 2021; Shi et al., 2021; Valdés et al., 2022). Additionally, metabolomic studies have been conducted in different biofluids, including sweat, saliva and used face masks, as well as exhaled breath, serum and plasma, to identify differential metabolites and metabolic changes associated with COVID-19 (Barberis et al., 2020; Barberis et al., 2021; Hasan et al., 2021). However, there are no studies focusing on changes in the metabolic profile in lung tissue, which is SARS-CoV-2 primary site of infection. In the present communication we use an NMR-based non-targeted metabolomics approach to characterize the metabolome of lung parenchyma from fatal COVID-19 cases and compare it with other fatal respiratory diseases. As discussed herein, we found statistically significant differences between metabolites related to energy metabolism and inflammatory processes, revealing a unique metabolic profile in the infected tissue.
2 Materials and methods
2.1 Sample acquisition and experimental design
The inclusion criteria comprised adults 18 years or older admitted to the ICU with respiratory sepsis and respiratory failure and which had received mechanical ventilation. Clinical information was obtained by retrospective chart review, and data of the Acute Physiology and Chronic Health disease Classification System II (APACHE-II) scores on admission, arterial oxygen pressure/inspired fraction of oxygen (PaO2/FiO2 or PAFI), the need of vasopressor support, renal or multiorgan failure, and the presence of comorbidities, such as diabetes, hypertension, or obesity, were collected. Fragments of lung tissue were collected during clinical autopsies performed on ICU patients deceased between November 2020 and February 2021 who had SARS-CoV-2 infection confirmed by RT-qPCR (n = 8). As stated above there was no vaccination strategy in place at the time, and therefore none of these patients had received immunization. In addition, lung fragments from non-COVID-19 deceased patients were collected between December 2016 and June 2018 at the same facility and with the same ethical safeguards. This group included microbiological and serological positive results for Klebsiella pneumoniae, Leptospira interrogans, and respiratory syncytial virus (n = 7). In all cases, tissue samples were obtained in the first 2 h post-mortem and stored at −80 °C until processed for NMR analysis.
2.2 NMR sample preparation and data acquisition
An adaptation of previously published methods was followed (Nakayasu et al., 2016). Briefly, lung tissue samples between 50 and 100 mg in wet weight were homogenized and extracted with 0.7 mL MeOH/H2O (4:3) in a bullet blender (Next Advance, United States). Subsequently, chloroform was added to reach a final CHCl3/MeOH/H2O ratio of 8:4:3, vortexed for 5 min, and centrifuged for 5 min at 5,000 g. The aqueous phases were lyophilized and resuspended in a phosphate buffer prepared in D2O (pH 7.4) (Dona et al., 2014).
Water-suppressed 1D-NOESY 1H NMR spectra of aqueous tissue extracts were obtained at 25 °C on a Bruker AVANCE III 500 operating at a 1H frequency of 500.13 MHz. A spectral width of 10 kHz, a data size of 32 K, and a total of 128 scans were employed to record each spectrum, using a relaxation delay of 4 s between scans. 1D-TOCSY and HSQC spectra were acquired and processed using parameters provided with the spectrometer.
2.3 NMR data processing
NMR data were processed and analyzed with MNova (version 14.0, MestreLab Research, S.L., Santiago de Compostela, Spain). Free induction decays were zero-filled to 64 K points and apodized with a 0.3 Hz exponential window function prior to Fourier transformation. All spectra were manually phase- and baseline-corrected, and referenced to the anomeric proton signal of α-glucose (5.22 ppm). Spectra were manually aligned, and the data was normalized to the total spectral area after excluding the residual water resonance region and regions without signals. No binning was employed to construct the data matrices used for the multivariate statistical analyses.
2.4 Metabolite identification and estimation of relative concentrations
Metabolites were identified by comparison of 1H NMR data against spectral repositories, including the Biological Magnetic Resonance Bank (BMRB) (Hoch et al., 2023), the Human Metabolome Database (HMDB) (Wishart et al., 2022), and Chenomx (version 9, Chenomx, Inc., Edmonton, Canada). When required, metabolite identification was confirmed with data from 1D-TOCSY and HSQC spectra.
Given the characteristics of lung parenchyma and the difficulties of obtaining precise dry weights in biologically-hazardous samples, variations in metabolite levels were estimated using relative concentrations. This figure was computed as the ratio between the area from individual metabolite 1H NMR signals and the total area of the spectrum.
2.5 Statistical analysis
Multivariate statistical analyses, including principal component analysis (PCA) and orthogonal partial least squares discriminant analysis (OPLS-DA), were carried out with the PLS_Toolbox package (version 8.5, Eigenvector Research Inc., Manson, WA, United States) implemented for MATLAB (revision 2014a, The MathWorks Inc., Natick, MA, United States). For all models, the data was mean-centered and scaled using a Pareto factor (Van Den Berg et al., 2006). Analysis of the data was first performed with PCA, which reduces data dimensionality and facilitates the identification of clusters or trends (Wold et al., 1987; Trygg and Wold, 2002; Trygg et al., 2006). The PCA scores plot was also employed to identify strong outliers outside the 95% significance region of Hotelling’s T2 ellipse. Cross-validation of OPLS-DA models was achieved using the random subset method, which involved 20 iterations over data split into 5 equally-sized parts. Receiver operating characteristic (ROC) curves were plotted, and areas under the curves were calculated to ensure the goodness of fit of the resulting models (Ekelund, 2012; Simundic, 2012). Permutation tests with 100 iterations were also performed to determine the degree of over-fitting and further validate the discriminant analyses (Ni et al., 2008). When needed, statistical total correlation spectroscopy (STOCSY) analyses were performed with an in-house MATLAB script based on the algorithm described elsewhere (Cloarec et al., 2005).
Pairwise t-test comparisons were carried out between continuous demographic variables as well as between the relative concentrations of all identified metabolites in COVID-19 and non-COVID-19 samples using GraphPad Prism (version 7.0, GraphPad Software, Inc., San Diego, CA, United States).
2.6 Metabolic pathways analyses
Metabolic pathway analysis was performed using the Pathway Analysis module of Metaboanalyst v.5.0 (Xia et al., 2011; Chong et al., 2019), which combines results from robust pathway enrichment analysis with pathway topology analysis to identify the most relevant pathways involved in the conditions under study (Aittokallio and Schwikowski, 2006; Kankainen et al., 2011). The selected pathway enrichment analysis method was GlobalAncova (Hummel et al., 2008), the node importance measure for topological analysis was out-degree centrality, and KEGG metabolic pathways were used as the backend knowledgebase.
3 Results
3.1 Clinical characteristics of study patients
All patients in this study had been diagnosed with pneumonia, presented respiratory sepsis, and exhibited high APACHE-II scores upon admission to the ICU (Table 1). They all required mechanical ventilation, and more than 80% were on vasopressor support. The average ICU stay was 17.6 ± 4.9 days for COVID-19, and 11.2 ± 8.3 for non-COVID-19 patients. When compared to non-COVID patients, those with COVID-19 had a higher percentage of comorbidities on admission (diabetes, hypertension, chronic obstructive pulmonary disease, and obesity) and a lower PAFI score.
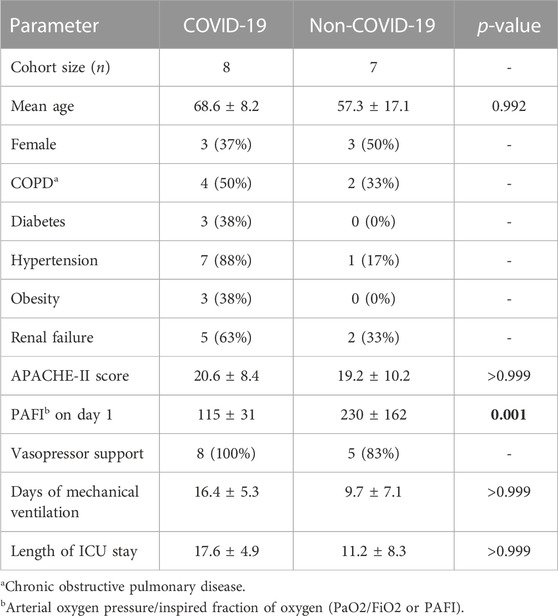
TABLE 1. Demographic and clinical characteristics of the study population upon admission in ICU. Variations in continuous variabbles with p-values <0.05 are indicated with bold numbers.
3.2 Metabolomic analysis
We initially compared 1H NMR profiles from lung tissue extracts from COVID-19 autopsies against those from non-COVID-19 autopsies (Figure 1). As shown in Figure 2A, a PCA derived from the 1H NMR data showed good discrimination between groups despite the low number of samples. Indeed, inspection of the loading plot from an OPLS-DA model obtained with the same data identified an important number of discriminating 1H signals (Figures 2B, C). Dereplication using a combination of STOCSY analyses, classical 1D and 2D NMR experiments, and comparison to data from various 1H spectral repositories allowed us to identify 21 metabolites (Figure 2C), 11 of which had significant differences in levels among the two cohorts (Table 2). The relative concentrations of the amino acids valine, alanine, methionine, glycine, tryptophane, phenylalanine, tyrosine, and asparagine were significantly increased in samples from COVID-19 patients. On the other hand, choline and glycerol-3-phosphate levels, as well as that of the metabolic intermediate succinate, were significantly lower among these samples.
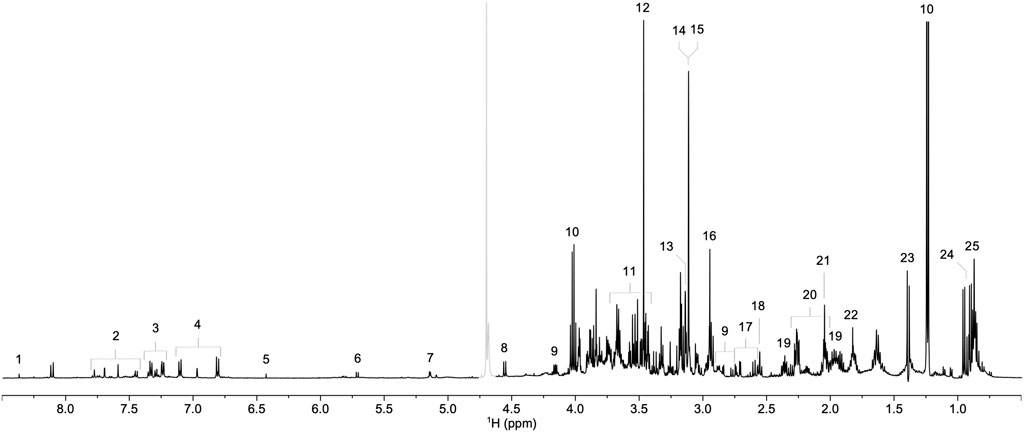
FIGURE 1. Representative 1H NMR spectrum of a lung parenchyma extract sample. Signals corresponding to formate (1), tryptophan (2), phenylalanine (3), tyrosine (4), fumarate (5), uracil (6), α-glucose (7), β-glucose (8), asparagine (9), lactate (10), glycerol-3-phosphate (11), glycine (12), betaine (13), choline (14), phosphocholine (15), creatine (16), citrate (17), pyruvate (18), glutamine (19), glutamate (20), methionine (21), acetate (22), alanine (23), valine (24), and isoleucine (25) are annotated. The grayed-out region corresponds to the residual HDO signal.
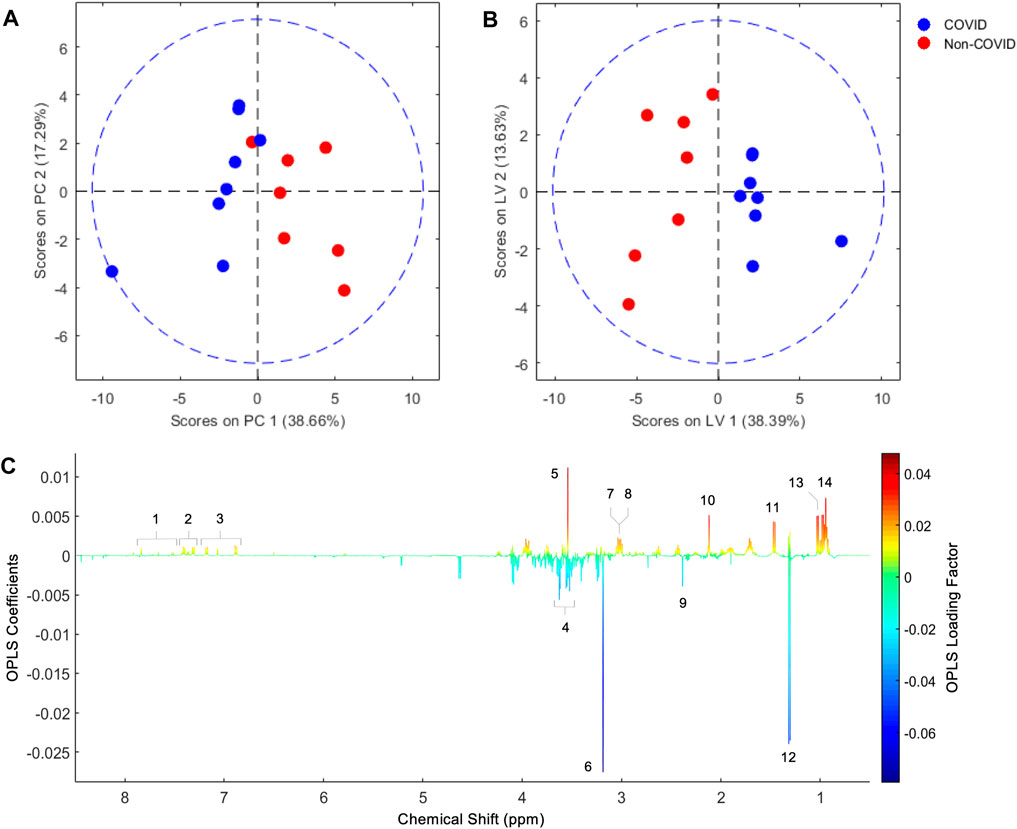
FIGURE 2. PCA score plot obtained from lung parenchyma extract 1H NMR data (A), and score and loading factor plots obtained from the OPLS-DA comparing COVID-19 and non-COVID samples (B and C, respectively). Metabolites that differentiate the COVID-19 from the non-COVID-19 cohorts are annotated in the loading factor plot, including tryptophan (1), phenylalanine (2), tyrosine (3), glycerol-3-phosphate (4), glycine (5), choline (6), creatine (7), asparagine (8), succinate (9), methionine (10), alanine (11), lactate (12), valine (13), and isoleucine (14). The OLPS-DA model had R2Y and Q2Y coefficients of 0.75 and 0.32, respectively, and its ROC curve had an AUC value of 0.98 (Supplementary Figures S1, S2).
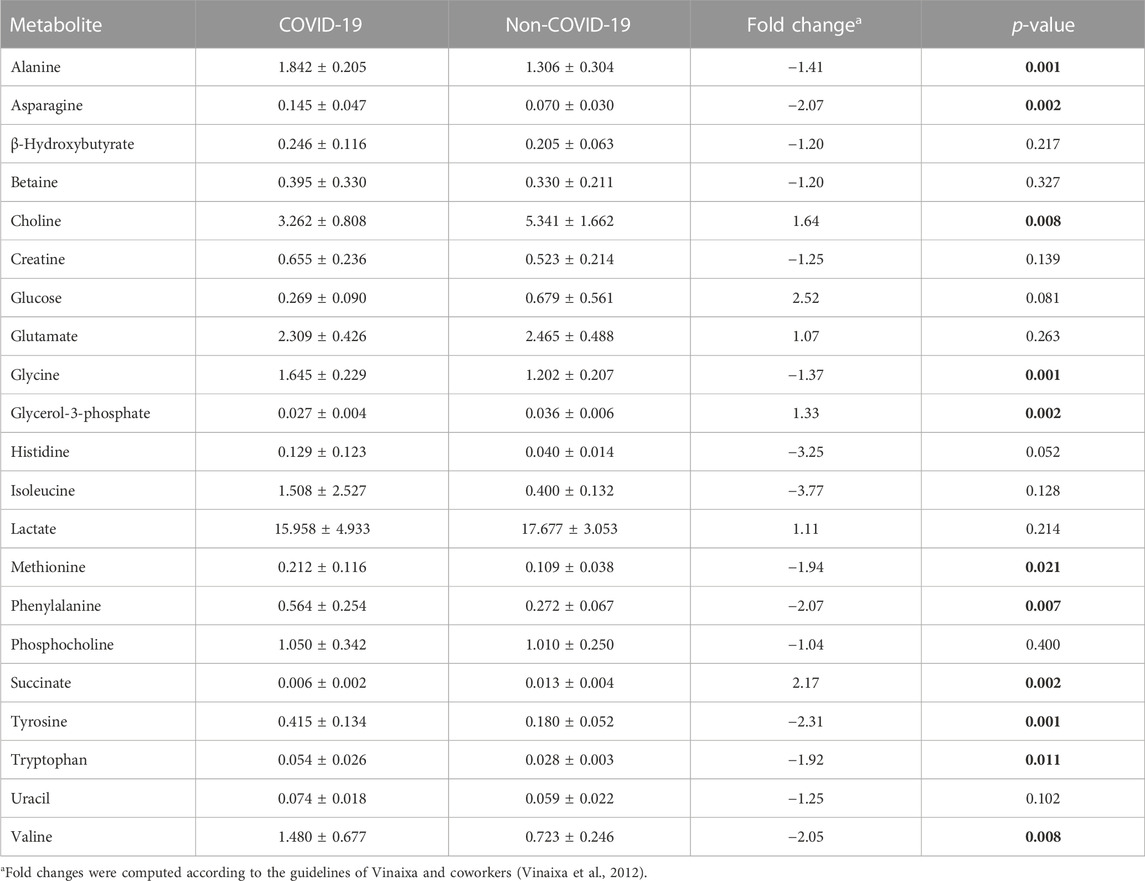
TABLE 2. Metabolite relative concentrations in COVID-19 and non-COVID-19 patients. Variations with p-values <0.05 are indicated with bold numbers.
3.3 Pathway analysis results
Metabolic pathway analysis was performed to identify the most relevant pathways involved in COVID-19 lung autopsy (Figure 3). This pathway analysis identified alterations in amino acids biosynthesis and degradation, anaplerotic alanine-aspartate-glutamate metabolism, glycine-serine-threonine metabolism, synthesis and degradation of ketone bodies and glycerophospholipid metabolism.
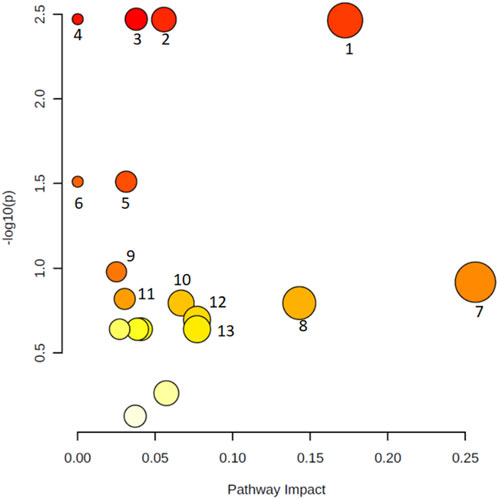
FIGURE 3. Metabolic pathway analysis of the set of metabolites identified in lung autopsies of COVID-19 patients and other fatal pneumonias. Y-axis represents the statistical p-values from enrichment analysis, and the X-axis represents the pathway impact value calculated from pathway topology analysis. The node colors represent the p-values (lowest: light yellow; highest: dark red) and the node radius indicate the pathway impact values. Dysregulated metabolic pathways include aminoacyl-tRNA biosynthesis (1), pantothenate and CoA biosynthesis (2), valine, leucine, and isoleucine degradation (3), valine, leucine, and isoleucine biosynthesis (4), alanine, aspartate, and glutamate metabolism (5), selenocompound metabolism (6), glycine, serine, and threonine metabolism (7), synthesis and degradation of ketone bodies (8), arginine and proline metabolism (9), butanoate metabolism (10), Cysteine and methionine metabolism (11), Glycerophospholipid metabolism (12), and glyoxylate and dicarboxylate metabolism (13).
4 Discussion
One of the most salient aspects from the results presented above is the general increase in the levels of essential amino acids, generally recognized as sepsis biomarkers (Mierzchala-Pasierb et al., 2020; Ahn et al., 2021), in patients with COVID-19. Indeed, branched chain amino acids (BCAAs), including isoleucine and valine (Table 2), are involved in stress, energy, and muscle metabolism (Neinast et al., 2019). BCAAs have different metabolic routes, with valine going solely to carbohydrates (glucogenic), leucine solely to fats (ketogenic), and isoleucine being both a glucogenic and a ketogenic amino acid. These metabolites can also regulate immune responses and influence viral infection (Atila et al., 2021). Hence, the maintenance of metabolic homeostasis is essential for the body’s normal physiological functioning, and disruptions in metabolic homeostasis could potentially facilitate virus infection. Our results in lung autopsies of COVID-19 patients show a significant enrichment in valine (Table 2). This is also evidenced by the metabolic pathway analysis, which revealed that valine, leucine, and isoleucine degradation and, to a lesser extent synthesis pathways, are significantly affected (Figure 3). High levels of BCAAs are associated with metabolic encephalopathy, often linked with respiratory suppression, epileptic seizures, and brain damage due to lack of oxygen (Ozturk et al., 2022). These results contrast those from a previous study conducted in serum, where the metabolic profiles of patients with ARDS due to COVID-19 and H1N1 were compared (Lorente et al., 2021). This report by Lorente and coworkers is particularly noteworthy, as it presents a footprint analysis in patients with the same severity of ARDS. On the other hand, most existing metabolomic studies contrast SARS-CoV-2 infected patients with healthy controls and cannot discern between metabolic dysregulations caused by the virus or the development of ARDS. These authors found that amino acid metabolism was decreased in COVID-19 patients, and the concentration of BCAAs, including isoleucine and valine, were also lower when compared with influenza A patients. Although different biofluids are commonly used for biomarker discovery, it is necessary to consider lung tissue metabolome as a complementary input. Indeed, it is not uncommon to find that certain metabolites are decreased in serum but increased in the tissue (Bernatchez and McCall, 2020).
Other metabolites found to be significantly more abundant in patients with COVID-19 were tyrosine, phenylalanine, and tryptophan. Absorption of the latter metabolite is mediated by angiotensin converting enzyme 2 (ACE2), the primary receptor of SARS-CoV-2, and has been recognized as a marker of inflammation in severe COVID-19 cases (Takeshita and Yamamoto, 2022). Similarly, elevated plasma or serum levels of tyrosine are observed in a variety of ailments, including hyperphenylalaninemia, sepsis, severe burns, transient tyrosinemia and hyperphenylalaninemia of the newborn, phlebotomus fever, viral hepatitis, or hepatic encephalopathy (Rosen et al., 1977; Watanabe et al., 1979; Rudnick and Ebach, 2004; Ansone et al., 2021). High levels of this non-essential amino acid synthetized from phenylalanine have also been detected in septic patients (Freund et al., 1978). Also, increased phenylalanine serum concentrations have been associated with immunological activation and an increased risk of cardiovascular events in sepsis and other viral infections (Ansone et al., 2021). This could be explained due to muscle tissue catabolism leading to amino acid release, which, together with the body’s differential metabolic capacity for different amino acids, results in their accumulation. Indeed, despite muscle tissue is easily able to oxidize BCAAs to support its own energy requirements, aromatic amino acids as well as sulfur-containing amino acids such as taurine, cysteine, and methionine are not as easily metabolized, and may account for the increase in the levels of tyrosine seen during sepsis (Freund et al., 1978). It has also been reported that as disease severity progresses, there is a significant increase in phenylalanine serum concentrations (Martínez-Gómez et al., 2022). Taken together with our results, these findings support the idea that these aromatic amino acids could be used as biomarkers of COVID-19 severity.
Additionally, succinate was found significantly depleted in COVID-19 patients. This metabolite plays a key role in hypoxia, where it acts inhibiting the prolyl hydroxylase domain-containing enzymes (PHD) (Yang et al., 2012). Under normal oxygenation, PHD constantly degrades the hypoxia-inducible transcription factor (HIF). This O2-sensitive factor mediates the response to hypoxia through the expression of genes that regulate cellular energy production, biosynthesis, cell growth, and redox homeostasis (Yang et al., 2014). In our cohort of severe COVID-19 patients lower initial PAFI scores were observed, indicating decreased blood oxygenation (Yang et al., 2012). While increased succinate levels would be expected in this scenario, it is known that mechanical ventilation periods like the ones experienced by our patients lead to succinate downregulation (Mussap and Fanos, 2021). As previously reported, these results indicate that despite high sensitivity, changes in succinate levels are not suitable indicators of disease severity or patient prognosis (Mussap and Fanos, 2021).
Choline levels were also found to be significantly lower in COVID-19 samples. This has also been reported in serum from severe COVID-19 patients, where an increase in the consumption of this trimethylamine caused by activation of macrophage innate immune receptors was linked to extracellular cytokine secretion (Sanchez-Lopez et al., 2019). The presence of pro-inflammatory components in bronchoalveolar lavage fluid is elevated even in severe COVID-19 patients treated with glucocorticoids, suggesting that slowing down the cytokine storm is a critical strategy for disease control (Barberis et al., 2020).
Similarly, we found a significant drop in glycerol-3-phosphate levels among COVID-19 samples. This phosphorylated polyol is tightly related to phospholipid metabolism, which is now known to be deregulated in COVID-19 patients based on serum metabolomic analyses (Shen et al., 2020; Shi et al., 2021). More importantly, it has been reported that the decrease in the levels of this species are directly related to severity in COVID-19 patients (Wu et al., 2020). Although the reduction in glycerol-3-phosphate concentration at the site of SARS-CoV-2 infection warrants further investigation, our results corroborate that this metabolite could be considered as a biomarker of severe manifestations of the disease.
Finally, lactate was the most widely expressed metabolite across both cohorts with no statistically significant differences between them. This finding is consistent with the known fact that high plasma lactate concentration is a marker of poor prognosis and an indicative of metabolic acidosis in critically ill patients, and was expected to be higher in both groups (Martha et al., 2021).
In conclusion, distinct metabolic signatures associated with energy metabolism and inflammatory pathways differentiate COVID-19 from fatal pneumonias caused by other respiratory infections. In particular, we found a significant increase in the levels of branched-chain, aromatic, and sulfur-containing amino acids in lung tissue from fatal COVID-19 cases. Many of these have been recognized as sepsis and inflammatory markers and are associated with lung injury, a condition that commonly leads to severe refractory hypoxemia and is one of the main causes of mortality in COVID-19 patients (Dhont et al., 2020; Donina, 2022; Ribeiro et al., 2022).
To our knowledge, this is the first comparative metabolomic study employing lung tissue samples from COVID-19 patients. In spite of the heterogeneity and wide range of symptoms observed, our findings provide additional insights into the pathogenesis of COVID-19 and have helped identify potential biomarkers for disease severity and treatment efficacy. Notwithstanding, the nature of the samples led to small cohorts affected differently by comorbidities. Some of these, such as diabetes, could have a sizable impact on the metabolic pathways identified as altered in our analyses (Felig et al., 1977). Therefore, the preliminary results reported in this work should be further corroborated in larger scale studies.
Data availability statement
The dataset presented in this study can be found at Mendeley Data with doi: 10.17632/bg45mx8rxd.1 (https://data.mendeley.com/datasets/bg45mx8rxd).
Ethics statement
The study was approved by the Research Bioethics and Ethics Committee from the Hospital Español, a state-managed public hospital which served as the principal COVID-19 reference in Uruguay during the pandemic. An informed consent form was signed by direct family members before autopsy.
Author contributions
JH: Data curation, Formal Analysis, Investigation, Methodology, Writing–original draft. AL-R: Data curation, Formal Analysis, Investigation, Methodology, Writing–review and editing. JLI-G: Conceptualization, Formal Analysis, Methodology, Writing–review and editing. FR: Investigation, Writing–review and editing. GM: Conceptualization, Data curation, Methodology, Writing–review and editing. GG: Funding acquisition, Project administration, Writing–review and editing. NN: Conceptualization, Funding acquisition, Investigation, Methodology, Project administration, Writing–original draft.
Funding
The author(s) declare financial support was received for the research, authorship, and/or publication of this article. This research was funded by the Agencia Nacional de Investigación e Innovación (ANII, award FSS_X_2019_1_155219), the Fondo para la Convergencia Estructural del MERCOSUR (FOCEM), and the Ministerio de Ciencia e Innovación (MCIN, award PID 2019-10656RJ-I00). Additional financial support was received from the Programa de Desarrollo de las Ciencias Básicas (PEDECIBA).
Acknowledgments
We wish to thank Prof. Gonzalo Moratorio for his recommendations regarding the preparation of lung parenchyma extracts from COVID-19 patients suitable for NMR experiments.
Conflict of interest
The authors declare that the research was conducted in the absence of any commercial or financial relationships that could be construed as a potential conflict of interest.
Publisher’s note
All claims expressed in this article are solely those of the authors and do not necessarily represent those of their affiliated organizations, or those of the publisher, the editors and the reviewers. Any product that may be evaluated in this article, or claim that may be made by its manufacturer, is not guaranteed or endorsed by the publisher.
Supplementary material
The Supplementary Material for this article can be found online at: https://www.frontiersin.org/articles/10.3389/fmolb.2023.1295216/full#supplementary-material
References
Abate, S. M., Ali, S. A., Mantfardo, B., and Basu, B. (2020). Rate of intensive care unit admission and outcomes among patients with coronavirus: a systematic review and Meta-analysis. PLoS One 15, e0235653. doi:10.1371/journal.pone.0235653
Ahlström, B., Frithiof, R., Hultström, M., Larsson, I. M., Strandberg, G., and Lipcsey, M. (2021). The Swedish COVID-19 intensive care cohort: risk factors of ICU admission and ICU mortality. Acta Anaesthesiol. Scand. 65 (4), 525–533. doi:10.1111/aas.13781
Ahn, S., Lee, S. H., Chung, K. Y., Ku, N. S., Hyun, Y., Chun, S., et al. (2021). Development and validation of a novel sepsis biomarker based on amino acid profiling. Clin. Nutr. 40 (6), 3668–3676. doi:10.1016/j.clnu.2021.05.008
Aittokallio, T., and Schwikowski, B. (2006). Graph-based methods for analysing networks in cell biology. Briefings Bioinforma. 7 (3), 243–255. doi:10.1093/bib/bbl022
Ansone, L., Briviba, M., Silamikelis, I., Terentjeva, A., Perkons, I., Birzniece, L., et al. (2021). Amino acid metabolism is significantly altered at the time of admission in hospital for severe COVID-19 patients: findings from longitudinal targeted metabolomics analysis. Microbiol. Spectr. 9 (3), e0033821. doi:10.1128/spectrum.00338-21
Atila, A., Alay, H., Yaman, M. E., Akman, T. C., Cadirci, E., Bayrak, B., et al. (2021). The serum amino acid profile in COVID-19. Amino acids 53 (10), 1569–1588. doi:10.1007/s00726-021-03081-w
Barberis, E., Amede, E., Khoso, S., Castello, L., Sainaghi, P. P., Bellan, M., et al. (2021). Metabolomics diagnosis of COVID-19 from exhaled breath condensate. Metabolites 11 (12), 847. doi:10.3390/metabo11120847
Barberis, E., Timo, S., Amede, E., Vanella, V. V., Puricelli, C., Cappellano, G., et al. (2020). Large-scale plasma analysis revealed new mechanisms and molecules associated with the host response to SARS-CoV-2. Int. J. Mol. Sci. 21 (22), 8623. doi:10.3390/ijms21228623
Bastos, L. S., Ranzani, O. T., Souza, T. M. L., Hamacher, S., and Bozza, F. A. (2021). COVID-19 hospital admissions: Brazil’s first and second waves compared’, Lancet Respir. Med. 9, e82-e83. doi:10.1016/S2213-2600(21)00287-3
Bernatchez, J. A., and McCall, L. (2020). Insights gained into respiratory infection pathogenesis using lung tissue metabolomics. PLOS Pathog. 16 (7), e1008662. doi:10.1371/journal.ppat.1008662
Booth, A., Reed, A. B., Ponzo, S., Yassaee, A., Aral, M., Plans, D., et al. (2021). Population risk factors for severe disease and mortality in COVID-19: a global systematic review and meta-analysis. PLOS ONE 16 (3), e0247461. doi:10.1371/journal.pone.0247461
Brosseau, L. M., Escandón-Vargas, K., Ulrich, A. K., Rasmussen, A. L., Roy, C. J., Bix, G. J., et al. (2021). Severe acute respiratory syndrome coronavirus 2 (SARS-CoV-2) dose, infection, and disease outcomes for coronavirus disease 2019 (COVID-19): a review. Clin. Infect. Dis. 75 (1), e1195–e1201. doi:10.1093/cid/ciab903
Chavez, S., Long, B., Koyfman, A., and Liang, S. Y. (2021). Coronavirus Disease (COVID-19): a primer for emergency physicians. Am. J. Emerg. Med. 44, 220–229. doi:10.1016/j.ajem.2020.03.036
Chen, W., Yao, M., Chen, M., Ou, Z., Yang, Q., He, Y., et al. (2022). Using an untargeted metabolomics approach to analyze serum metabolites in COVID-19 patients with nucleic acid turning negative. Front. Pharmacol. 13, 964037. doi:10.3389/fphar.2022.964037
Chong, J., Wishart, D. S., and Xia, J. (2019). Using MetaboAnalyst 4.0 for comprehensive and integrative metabolomics data analysis’, Curr. Protoc. Bioinforma. 68, e86. doi:10.1002/cpbi.86
Cloarec, O., Dumas, M., Craig, A. W., Barton, R., Trygg, J., Hudson, J., et al. (2005). Statistical total correlation spectroscopy: an exploratory approach for latent biomarker identification from metabolic 1H NMR data sets. Anal. Chem. 77 (5), 1282–1289. doi:10.1021/ac048630x
Dhont, S., Derom, E., Van Braeckel, E., Depuydt, P., and Lambrecht, B. N. (2020). The pathophysiology of ‘happy’ hypoxemia in COVID-19. Respir. Res. 21 (1), 198. doi:10.1186/s12931-020-01462-5
Dona, A. C., Jiménez, B., Schäfer, H., Humpfer, E., Spraul, M., Lewis, M. R., et al. (2014). Precision high-throughput proton NMR spectroscopy of human urine, serum, and plasma for large-scale metabolic phenotyping. Anal. Chem. 86 (19), 9887–9894. doi:10.1021/ac5025039
Dongelmans, D. A., Termorshuizen, F., Brinkman, S., Bakhshi-Raiez, F., Arbous, M. S., De Lange, D. W., et al. (2022). Characteristics and outcome of COVID-19 patients admitted to the ICU: a nationwide cohort study on the comparison between the first and the consecutive upsurges of the second wave of the COVID-19 pandemic in The Netherlands. Ann. Intensive Care 12 (1), 5. doi:10.1186/s13613-021-00978-3
Donina, Z. A. (2022). Causes of hypoxemia in COVID-19. J. Evol. Biochem. Phys. 58, 73–80. doi:10.1134/S0022093022010070
Ejaz, H., Alsrhani, A., Zafar, A., Javed, H., Junaid, K., Abdalla, A. E., et al. (2020). COVID-19 and comorbidities: deleterious impact on infected patients. J. Infect. Public Health 13, 1833–1839. doi:10.1016/j.jiph.2020.07.014
Ekelund, S. (2012). ROC curves—what are they and how are they used? Point of care. J. Near-patient Test. Technol. 11 (1), 16–21. doi:10.1097/poc.0b013e318246a642
Elizondo, V., Harkins, G. W., Mabvakure, B., Smidt, S., Zappile, P., Marier, C., et al. (2021). SARS-CoV-2 genomic characterization and clinical manifestation of the COVID-19 outbreak in Uruguay. Emerg. Microbes Infect. 10 (1), 51–65. doi:10.1080/22221751.2020.1863747
Estenssoro, E., Loudet, C., Rios, F., Edul, V. S. K., Plotnikow, G., Andrian, M., et al. (2021). Clinical characteristics and outcomes of invasively ventilated patients with COVID-19 in Argentina (SATICOVID): a prospective, multicentre cohort study. Lancet Respir. Med. 9 (9), 989–998. doi:10.1016/s2213-2600(21)00229-0
Felig, P., Wahren, L., Sherwin, R., and Palaiologos, G. (1977). Amino acid and protein metabolism in diabetes mellitus. Arch. Intern Med. 137 (4), 507–513. doi:10.1001/archinte.1977.03630160069014
Freund, H. R., Ryan, J. A., and Fischer, J. E. (1978). Amino acid derangements in patients with sepsis: treatment with branched chain amino acid rich infusions. Ann. Surg. 188 (3), 423–430. doi:10.1097/00000658-197809000-00017
Gosangi, B., Rubinowitz, A. N., Irugu, D., Gange, C., Bader, A., and Cortopassi, I. (2022). COVID-19 ARDS: a review of imaging features and overview of mechanical ventilation and its complications. Emerg. Radiol. 29 (1), 23–34. doi:10.1007/s10140-021-01976-5
GUIAD-COVID-19 (2022). Estadisticasuy. Available online: https://guiad-covid.github.io/estadisticasuy.html (accessed on July 26, 2021).
Hasan, M. K., Suleiman, M. M., and Perez-Lopez, A. (2021). Metabolomics in the diagnosis and prognosis of COVID-19. Front. Genet. 12, 721556. doi:10.3389/fgene.2021.721556
Hoch, J. C., Baskaran, K., Burr, H., Chin, J., Eghbalnia, H. R., Fujiwara, T., et al. (2023). Biological magnetic resonance data bank. Nucleic Acids Res. 51, D368–D376. doi:10.1093/nar/gkac1050
Hummel, M., Meister, R., and Mansmann, U. (2008). GlobalANCOVA: exploration and assessment of gene group effects. Bioinformatics 24 (1), 78–85. doi:10.1093/bioinformatics/btm531
Kankainen, M., Gopalacharyulu, P., Holm, L., and Orešič, M. (2011). MPEA-metabolite pathway enrichment analysis. Bioinformatics 27 (13), 1878–1879. doi:10.1093/bioinformatics/btr278
Kimhofer, T., Lodge, S., Whiley, L., Gray, N. S., Loo, R. L., Lawler, N. G., et al. (2020). Integrative modeling of quantitative plasma lipoprotein, metabolic, and amino acid data reveals a multiorgan pathological signature of SARS-CoV-2 infection. J. Proteome Res. 19 (11), 4442–4454. doi:10.1021/acs.jproteome.0c00519
Koçak Tufan, Z., Kayaaslan, B., and Mer, M. (2021). COVID-19 and sepsis. Turkish J. Med. Sci. 51, 3301–3311. doi:10.3906/sag-2108-239
Kurtz, P., Bastos, L. S., Dantas, L. F., Zampieri, F. G., Soares, M., Hamacher, S., et al. (2021). Evolving changes in mortality of 13,301 critically ill adult patients with COVID-19 over 8 months. Intensive Care Med. 47 (5), 538–548. doi:10.1007/s00134-021-06388-0
Liu, Y., Yan, L., Wan, L., Xiang, T., Le, A., Liu, J., et al. (2020). Viral dynamics in mild and severe cases of COVID-19. Lancet Infect. Dis. 20 (6), 656–657. doi:10.1016/s1473-3099(20)30232-2
Lorente, J. A., Nin, N., Villa, P., Vasco, D., Miguel-Coello, A. B., Rodriguez, I. R., et al. (2021). Metabolomic diferences between COVID-19 and H1N1 influenza induced ARDS. Crit. Care 25 (1), 390. doi:10.1186/s13054-021-03810-3
Martha, J. W., Wibowo, A., and Pranata, R. (2021). Prognostic value of elevated lactate dehydrogenase in patients with COVID-19: a systematic review and meta-analysis. Postgrad. Med. J. 98, 422–427. doi:10.1136/postgradmedj-2020-139542
Martínez-Gómez, L. E., Ibarra-González, I., Fernández-Lainez, C., Tusie, T., Moreno-Macías, H., Martinez-Armenta, C., et al. (2022). Metabolic reprogramming in SARS-CoV-2 infection impacts the outcome of COVID-19 patients. Front. Immunol. 13, 936106. doi:10.3389/fimmu.2022.936106
Mierzchala-Pasierb, M., Lipinska-Gediga, M., Fleszar, M. G., Lesnik, P., Placzkowska, S., Serek, P., et al. (2020). Altered profiles of serum amino acids in patients with sepsis and septic shock - preliminary findings. Archives Biochem. biophysics 691, 108508. doi:10.1016/j.abb.2020.108508
Moreno, P., Moratorio, G., Iraola, G., Fajardo, A., Aldunate, F., Pereira-Gómez, M., et al. (2020). An effective COVID-19 response in South America: the Uruguayan Conundrum. medRxiv. doi:10.1101/2020.07.24.20161802
Murali, R., Wanjari, U. R., Mukherjee, A. G., Gopalakrishnan, A. V., Kannampuzha, S., Namachivayam, A., et al. (2023). Crosstalk between COVID-19 infection and kidney diseases: a review on the metabolomic approaches. Vaccines 11 (2), 489. doi:10.3390/vaccines11020489
Mussap, M., and Fanos, V. (2021). Could metabolomics drive the fate of COVID-19 pandemic? A narrative review on lights and shadows. Clin. Chem. Laboratory Med. (CCLM) 59 (12), 1891–1905. doi:10.1515/cclm-2021-0414
Nakayasu, E. S., Nicora, C. D., Sims, A. C., Burnum-Johnson, K. E., Kim, Y. H., Kyle, J. E., et al. (2016). MPLEx: a robust and universal protocol for single-sample integrative proteomic, metabolomic, and lipidomic analyses. mSystems 1 (3), e00043. doi:10.1128/msystems.00043-16
Neinast, M. D., Jang, C., Hui, S., Murashige, D., Chu, Q., Morscher, R. J., et al. (2019). Quantitative analysis of the whole-body metabolic fate of branched-chain amino acids. Cell Metab. 29 (2), 417–429. doi:10.1016/j.cmet.2018.10.013
Ni, N., Nandi, S., Kreyssig, A., Goldman, A. I., Mun, E. D., Bud’ko, S. L., et al. (2008). First-order structural phase transition inCaFe2As2. Phys. Rev. B 78 (1), 014523. doi:10.1103/physrevb.78.014523
Ozturk, A., Bayraktar, N., Bayraktar, M., Ibrahim, B., Bozok, T., and Resat, C. M. (2022). Evaluation of amino acid profile in serum of patients with Covid-19 for providing a new treatment strategy. J. Med. Biochem. 41 (4), 526–533. doi:10.5937/jomb0-37514
Ranzani, O. T., Bastos, L. S., Gelli, J. G. M., Marchesi, J. F., Baião, F. A., Hamacher, S., et al. (2021). Characterisation of the first 250 000 hospital admissions for COVID-19 in Brazil: a retrospective analysis of nationwide data. Lancet Respir. Med. 9 (4), 407–418. doi:10.1016/s2213-2600(20)30560-9
Rego, N., Salazar, C., Paz, M. A., Costábile, A., Fajardo, A., Ferrés, I., et al. (2021). Emergence and spread of a B.1.1.28-Derived P.6 lineage with Q675H and Q677H spike mutations in Uruguay. Viruses 13 (9), 1801. doi:10.3390/v13091801
Ribeiro, A., Mendonça, M., Sabina Sousa, C., Trigueiro Barbosa, M., and Morais-Almeida, M. (2022). Prevalence, presentation and outcomes of silent hypoxemia in COVID-19. Clinical medicine insights: circulatory, respiratory and pulmonary medicine. Clin. Med. Insights. Circ. Respir. Pulm. Med. 16, 11795484221082761. doi:10.1177/11795484221082761
Rosen, H. S., Yoshimura, N. N., Hodgman, J. M., and Fischer, J. E. (1977). Plasma amino acid patterns in hepatic encephalopathy of differing etiology. Gastroenterology 72 (3), 483–487. doi:10.1016/s0016-5085(77)80261-8
Rudnick, D. A., and Ebach, D. R. (2004). Tyrosinemia. Encycl. Gastroenterology 2004, 538–541. doi:10.1016/b0-12-386860-2/00772-3
Sanchez-Lopez, E., Zhong, Z., Stubelius, A., Sweeney, S. R., Booshehri, L. M., Antonucci, L., et al. (2019). Choline uptake and metabolism modulate macrophage IL-1Β and IL-18 production. Cell Metab. 29 (6), 1350–1362. doi:10.1016/j.cmet.2019.03.011
Shen, B., Yi, X., Sun, Y., Bi, X., Du, J., Zhang, C., et al. (2020). Proteomic and metabolomic characterization of COVID-19 patient sera. Cell 182 (1), 59–72. doi:10.1016/j.cell.2020.05.032
Shi, D., Yan, R., Lv, L., Jiang, H., Lu, Y., Sheng, J., et al. (2021). The serum metabolome of COVID-19 patients is distinctive and predictive. Metabolism-clinical Exp. 118, 154739. doi:10.1016/j.metabol.2021.154739
Simundic, A. (2012). Diagnostic accuracy—Part 1: basic concepts sensitivity and specificity, ROC analysis, STARD statement. J. Near-patient Test. Technol. 11 (1), 6–8. doi:10.1097/poc.0b013e318246a5d6
Takeshita, H., and Yamamoto, K. (2022). Tryptophan metabolism and COVID-19-induced skeletal muscle damage: is ACE2 a key regulator? Front. Nutr. 9, 868845. doi:10.3389/fnut.2022.868845
Trygg, J., Holmes, E., and Lundstedt, T. (2006). Chemometrics in metabonomics. J. Proteome Res. 6 (2), 469–479. doi:10.1021/pr060594q
Trygg, J., and Wold, S. (2002). Orthogonal projections to latent structures (O-PLS). J. Chemom. 16 (3), 119–128. doi:10.1002/cem.695
Valdés, A., Moreno, L., Rello, S. R., Orduña, A., Bernardo, D., and Cifuentes, A. (2022). Metabolomics study of COVID-19 patients in four different clinical stages. Sci. Rep. 12 (1), 1650. doi:10.1038/s41598-022-05667-0
Van Den Berg, R. A., Hoefsloot, H. C. J., Westerhuis, J. A., Smilde, A. K., and Van Der Werf, M. J. (2006). Centering, scaling, and transformations: improving the biological information content of metabolomics data. BMC Genomics 7 (1), 142. doi:10.1186/1471-2164-7-142
Verity, R., Okell, L. C., Dorigatti, I., Winskill, P., Whittaker, C., Imai, N., et al. (2020). Estimates of the severity of coronavirus disease 2019: a model-based analysis. Lancet Infect. Dis. 20 (6), 669–677. doi:10.1016/s1473-3099(20)30243-7
Vinaixa, M., Samino, S., Saez, I., Duran, J., Guinovart, J. J., and Yanes, O. (2012). A guideline to univariate statistical analysis for LC/MS-Based untargeted metabolomics-derived data. Metabolites 2, 775–795. doi:10.3390/metabo2040775
Watanabe, A., Takesue, A., Higashi, T., and Nagashima, N. (1979). Serum amino acids in hepatic encephalopathy-effects of branched chain amino acid infusion on serum aminogram. Acta hepato-gastroenterologica 26 (5), 346–357.
Wishart, D. S., Guo, A., Oler, E., Wang, F., Anjum, A., Peters, H., et al. (2022). HMDB 5.0: the human metabolome Database for 2022. Nucleic acids Res. 50 (D1), D622–D631. doi:10.1093/nar/gkab1062
Wold, S., Esbensen, K. H., and Geladi, P. (1987). Principal component analysis. Chemom. Intelligent Laboratory Syst. 2 (1–3), 37–52. doi:10.1016/0169-7439(87)80084-9
Wu, D., Shu, T., Yang, X., Song, J. Y., Zhang, M., Yao, C., et al. (2020). Plasma metabolomic and lipidomic alterations associated with COVID-19. Natl. Sci. Rev. 7 (7), 1157–1168. doi:10.1093/nsr/nwaa086
Xia, J., Wishart, D. S., and Valencia, A. (2011). MetPA: a web-based metabolomics tool for pathway analysis and visualization. Bioinformatics 27 (13), 2342–2344. doi:10.1093/bioinformatics/btq418
Yang, M., Soga, T., Pollard, P. J., and Adam, J. (2012). The emerging role of fumarate as an oncometabolite. Front. Oncol. 2, 85. doi:10.3389/fonc.2012.00085
Keywords: biomarkers, COVID-19, ICU patients, lung parenchyma, NMR-based metabolomics
Citation: Hurtado JI, López-Radcenco A, Izquierdo-García JL, Rodríguez F, Moyna G, Greif G and Nin N (2023) A comparative NMR-based metabolomics study of lung parenchyma of severe COVID-19 patients. Front. Mol. Biosci. 10:1295216. doi: 10.3389/fmolb.2023.1295216
Received: 15 September 2023; Accepted: 30 October 2023;
Published: 15 November 2023.
Edited by:
Xavier Domingo-Almenara, Eurecat, SpainReviewed by:
Elizabeth R. Lusczek, University of Minnesota Twin Cities, United StatesAna Paula Valente, Federal University of Rio de Janeiro, Brazil
Copyright © 2023 Hurtado, López-Radcenco, Izquierdo-García, Rodríguez, Moyna, Greif and Nin. This is an open-access article distributed under the terms of the Creative Commons Attribution License (CC BY). The use, distribution or reproduction in other forums is permitted, provided the original author(s) and the copyright owner(s) are credited and that the original publication in this journal is cited, in accordance with accepted academic practice. No use, distribution or reproduction is permitted which does not comply with these terms.
*Correspondence: Nicolás Nin, niconin@hotmail.com; Guillermo Moyna, gmoyna@fq.edu.uy
†These authors have contributed equally to this work