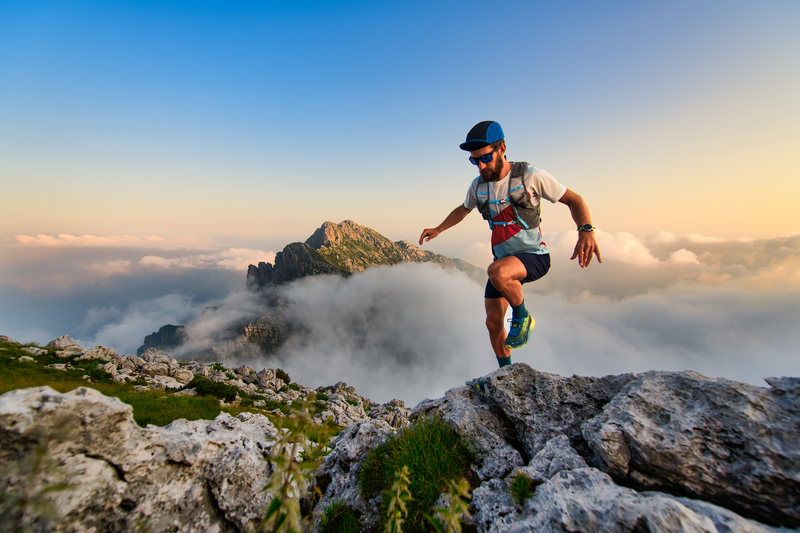
94% of researchers rate our articles as excellent or good
Learn more about the work of our research integrity team to safeguard the quality of each article we publish.
Find out more
OPINION article
Front. Mol. Biosci. , 26 October 2023
Sec. Genome Organization and Dynamics
Volume 10 - 2023 | https://doi.org/10.3389/fmolb.2023.1286716
This article is part of the Research Topic Transcriptional and Epigenetic Regulation in Normal and Aberrant Hematopoiesis View all 5 articles
DNA methylation (DNAm) commonly refers to the methylation of cytosines in the mammalian genome, which mainly occurs at CpG sites (cytosines followed by guanines). Its existence was initially predicted in 1948 (Hotchkiss, 1948) and the first analyses of eukaryotic DNAm states were performed in 1978 (Bird and Southern, 1978). Since then, and speeded up by recent genome-wide analyses, a large body of evidence suggests that proper DNAm is vital for hematopoiesis. For example, aberrations affecting the function of the DNAm machinery, e.g., DNA methyltransferases (DNMTs) and demethylating enzymes (TETs among others) (Figure 1A), have profound effects on the hematological system, from differentiation defects to malignant transformation, as reviewed by Gore and Weinstein (2016) and Medeiros et al. (2017). While DNAm is usually associated with gene expression changes, a clear link between these two layers is still lacking. Less commonly considered is that DNAm can be used as read out of other layers of information, such as cell identity (Ziller et al., 2013; Farlik et al., 2016; Loyfer et al., 2023) and cell proliferation (Yang et al., 2016; Youn and Wang, 2018; Zhou et al., 2018; Duran-Ferrer et al., 2020; Minteer et al., 2023). This, together with advancements in single-cell technologies, opens up new avenues to study cellular processes from the single-cell DNAm (scDNAm) perspective. In this opinion piece, we address the main processes that shape the DNA methylome during hematopoiesis and beyond, and provide insights into how to utilise scDNAm studies to extract underlying layers of biological information. Altogether, allowing to expand the opportunities for new discoveries in the single-cell era.
FIGURE 1. Summary of relevant biological and technological (single-cell) DNA methylation analyses aspects. (A) Main enzymes directly involved in DNAm modulation. TETs, Tet Methylcytosine Dioxygenases; TDG, Thymine DNA glycosylase; DNMTs, DNA methyltransferases. Deaminating enzymes such as APOBECs and AID were not included in this overview, as they play a less prominent role in this pathway. (B) Different sets of CpGs that can be analysed to retrieve underlying biological information. H3, histone 3; K4/9/27, lysine 4/9/27; me1/3, mono/trimethylation; ac, acetylation. (C) Graphical overview of overlapping coverage, indicated by the number of cells in which information of a specific CpG is available. Targeting scDNAm methods to a specific set of the CpGs in the genome increases overlapping coverage (right) in comparison to randomly covering the same percentage of CpGs in each cell (left). (D) Targeted methods reduce sequencing efforts and price, while increasing overlapping coverage. They can be based on targeting genomic features such as CpG dense regions in scRRBS and transposable elements in scTEM-seq (for this method the number of CpGs is an approximation based on the number of transposable elements covered per cell), or designed to capture information at specific genomic loci marked by their coordinates (sciMET-cap and scTAM-seq). The latter methods are most powerful to increase overlapping coverage, which we assume reaches a plateau when a low number of CpGs are analysed. This plateau is dependent on the technology and sequencing depth. We believe that this plateau is currently reached at around 900 CpGs, using scTAM-seq. With the speed of technological developments and decrease of sequencing costs this number will increase.
Different biological processes shape the DNA methylome of normal and malignant cells. These comprise activation of lineage-specific, cell-stage specific, or tumor-specific enhancers, as well as stochastic errors of DNAm maintenance during cell division, and deposition of DNAm at polycomb-repressed regions. Here we focus on how DNAm changes affected by these different processes can be leveraged to infer other information, such as cell identity, proliferative and genome activation history, and the cell of origin of tumor cells. To understand how the abovementioned features can be extrapolated using this epigenetic mark, DNAm changes targeting regulatory elements and proliferation-associated epigenetic drift will be discussed. We believe that deeper insights into these processes and better knowledge of the CpGs that they target will allow for more comprehensive biological interpretations of large-scale DNAm studies.
DNAm changes in regulatory elements are widely studied since early observations that promoters of tumor suppressor genes (TSGs) are hypermethylated in cancer with p16 as hallmark example (Gonzalez-Zulueta et al., 1995; Herman et al., 1995). However, in many cases silencing of TSGs precedes promoter DNAm and demethylation does not necessarily reactivate gene expression as reviewed by Jones (2012). In addition, promoter DNAm is not directly linked to gene expression, but majorly depends on promoter CpG density (Weber et al., 2007). Nevertheless, for a fraction of promoters, DNAm and gene expression levels show clear correlations, for instance mediated by methylation sensitive transcription factors (TF) (Domcke et al., 2015; Maurano et al., 2015; Grand et al., 2021). Interestingly, with the advancement of genome-wide methods, the epigenomics field has opened up to explore DNAm at enhancers. Even though active enhancers harbour low DNAm levels (Stadler et al., 2011; Ziller et al., 2013), it remains unclear if DNA demethylation is necessary for enhancer activation. For most enhancers chromatin accessibility is not linked to low DNAm levels at the single-molecule level (Kreibich et al., 2023). This suggests that DNA demethylation is not instructive for activation of most enhancers. Nevertheless, enhancer DNAm is a powerful tool to study lineage specification and cell differentiation, both guided by TF programs driving unique enhancer activation patterns. In fact, it is becoming increasingly clear that differential DNAm among cell lineage and differentiation stages are mainly located in enhancers, as shown by detailed DNAm maps of hematopoietic cells (Farlik et al., 2016; Roy et al., 2021), as well as for a broad set of other cell types (Ziller et al., 2013; Loyfer et al., 2023). Enhancer DNAm can furthermore be used as an indirect readout of cellular TF activity, both in healthy and tumor cells. This can be achieved by applying TF motif enrichment analyses in differentially methylated regions, as shown for hematopoeitic cells types and their derived malignancies, as well as for non-hematopoietic cells (Ziller et al., 2013; Kulis et al., 2015; Farlik et al., 2016; Duran-Ferrer et al., 2020; Roy et al., 2021; Loyfer et al., 2023). Finally, genomic regions with low DNAm levels can represent inactive sites (lacking histone marks H3K27ac and H3K4me1) that were active enhancers during earlier cell stages. Clear examples are murine embryonic enhancers with low DNAm levels in adult tissues (Hon et al., 2013; Jadhav et al., 2019). Hence, low DNAm levels at these regions serve as an imprint of past enhancer activity and can be used to study cellular history.
The clear segregation of cell types and cell stages based on DNAm levels at enhancers implies that DNAm profiles can be very informative to determine the cellular origin of tumors. This is of particular interest in poorly differentiated tumors with unclear cell of origin, or in cases of metastasis where the primary tumor cannot be determined. In fact, ample examples exist where DNAm of tumor DNA or circulating cell-free DNA can be used as biomarker to diagnose tumors, as reviewed or analysed in large cohort studies (Liu et al., 2020; Barefoot et al., 2021; Danilova et al., 2022; Stackpole et al., 2022). Moreover, DNAm cannot only be exploited to study tumor cell of origin at the global cell type level, but also at the more detailed level of differentiation stage. For instance, different B-cell related tumors, such as chronic lymphocytic leukemia (CLL) and mantle cell lymphoma, can be further divided into subtypes originating from pre- or post-germinal center B cells (Kulis et al., 2012; Queiros et al., 2016), being relevant for clinical decision making and prognostic outcome.
Intriguingly, DNAm outside enhancers can also be leveraged to understand cellular history. Cell division, for example, is accompanied by global DNAm changes, leaving an epigenetic mark of proliferation. It is one of the main contributors that shape the DNA methylome in tumors as well as healthy cells (Yang et al., 2016; Youn and Wang, 2018; Zhou et al., 2018; Duran-Ferrer et al., 2020; Minteer et al., 2023). This proliferation-associated drift is characterized by stochastic loss of DNAm in heterochromatic regions (lacking the main regulatory histone marks or containing H3K9me3). In contrast, polycomb-repressed regions (marked by H3K27me3) stochastically accumulate DNAm upon proliferation (Duran-Ferrer et al., 2020). Importantly, while this is a relatively novel concept, the underlying observations are not new; global DNAm loss in tumors and DNAm gain at TSG promoters were already observed long ago (Feinberg and Vogelstein, 1983; Gama-Sosa et al., 1983; Gonzalez-Zulueta et al., 1995; Herman et al., 1995), followed by many similar findings. All these likely represent proliferation-associated drift. Of further note, the two contrasting behaviours - loss and gain of DNAm - at different genomic loci usually co-exist within a tumor, but some neoplasms show a clear bias for one of the two (e.g., hypermethylation in acute lymphoblastic leukemia and hypomethylation in multiple myelome) (Duran-Ferrer et al., 2020). Thirdly, while some CpGs targeted by proliferation-associated drift overlap with CpGs affected by ageing, others do not, suggesting that these two processes are related but not necessarily affect the same genomic regions (Duran-Ferrer et al., 2020; Minteer et al., 2023). Though the functional impact of proliferation-associated DNAm drift is still unknown, notion of this process is crucial to investigate cancer development and progression. It can, for example, be used to study the impact of genetic alterations on proliferation or as powerful independent prognostic marker (Duran-Ferrer et al., 2020). In contrast, it also can hamper DNAm interpretations. Particularly, the presence of partially methylated domains due to the stochastic nature of proliferation-associated drift limits the ability to distinguish functional hemi-methylation events, e.g., imprinted or mono-allelic changes, unless moving to the single-cell and/or single-molecule level.
In summary, in this section we have outlined the power of DNAm data to characterise important underlying biological features of normal and tumor cells. All stands or falls though, with selection of the right CpGs. Namely, to study cell identity, CpGs in enhancers marked by histone marks H3K27ac and H3K4me1 will be most powerful. In contrast, for proliferative history, CpGs in H3K9me3-and H3K27me3-marked regions need to be analysed, together with those in heterochromatic regions lacking main regulatory histone marks (Figure 1B). Of note, these regions can differ dependent on the biological system. To define them, detailed, cell-type specific histone-mark based chromatin state maps are needed. In this respect, large-scale efforts to generate reference epigenomes are invaluable (Roadmap Epigenomics et al., 2015; Stunnenberg et al., 2016), with the BLUEPRINT consortium being at the forefront of providing detailed characterisations of normal and malignant hematopoietic cells (Martens and Stunnenberg, 2013). They provide excellent resources enhancing the opportunities to read out underlying biological information from DNAm data.
As shown in the previous paragraphs, bulk DNAm studies have contributed to discoveries in normal hematological development and its derived malignancies. However, these analyses do not enable the exploration of cellular heterogeneity, nor the detection of rare cell types such as low abundant healthy or pre-malignant cell states, respectively arising during normal differentiation and tumor formation. Neither do they allow us to study DNAm patterns in isolated small cell populations, due to the high amount of input material needed. To overcome these limitations, the DNAm field has directed major efforts towards the development of single-cell technologies. In this section, we will give an overview of the main recent advancements of scDNAm methods and highlight key aspects to take into consideration to choose the appropriate method dependent on the biological question of interest. An overview of the highlighted methods and their main features can be found in Table 1.
TABLE 1. Overview of highlighted single-cell DNA methylation methods. Brief overview of the methods mentioned in the text with their main features.
Single-cell DNAm methods face multiple challenges, both from the biological and technical perspective. A biological bottleneck is the large number of CpGs present in every human cell, namely, two copies of roughly 29M CpGs. Therefore, analyzing the entire DNA methylome in large numbers of cells is very difficult, if not impossible, due to sequencing limitations. Covering all CpGs in a single cell would require 100–1,000 times more reads/cell in comparison with a scRNA-seq experiment. Even with extreme sequencing efforts (5–7M reads/cell), genome-wide single-cell methods can only cover a random set of 6%–7.5% of the total number of CpGs/cell (Luo et al., 2017; Mulqueen et al., 2018). Consequently, scDNAm methods suffer from high dropout rates, generating very sparse datasets. This sparsity is furthermore augmented by other technical reasons such as degradation of the DNA upon bisulfite treatment and PCR amplification biases or failures. Importantly, the high level of randomness of CpG coverage in genome-wide methods implies that it is unlikely to have the same CpGs covered in different cells, resulting in a low so-called overlapping coverage. In other words, for each cell one obtains information about a different set of CpGs (Figure 1C). This leads to the loss of single-CpG resolution when comparing DNAm readouts among cells, since binarization of the data into larger regions is necessary. A related biological limitation is that most CpGs have static DNAm levels; within each tissue only a minor fraction of CpGs show dynamic methylation (Kulis et al., 2015; Durek et al., 2016; Loyfer et al., 2023). This means that only a small fraction of the DNA methylome is informative. For the above-mentioned reasons, aiming to analyze the entire DNA methylome, as in genome-wide methods, hinders the ability to obtain information about biologically relevant CpGs.
Nevertheless, genome-wide bisulfite-based scDNAm methods, such as sci-MET (Mulqueen et al., 2018), sci-METv2 (Nichols et al., 2022), snmC-seq (Luo et al., 2017), snmC-seq2 (Luo et al., 2018) and Drop-BS (Zhang et al., 2023) offer exciting opportunities to perform hypothesis-free DNAm analyses, with high-throughput methods enabling readouts of thousands of cells. However, one must keep in mind that the overlapping coverage and resolution obtained with these methods largely depend on the sequencing depth one can afford. At high sequencing depth they are effective in distinguishing cell types. Hence, taking advantage of their unbiasedness, genome-wide methods have been used to build cell-type specific DNAm atlases (Luo et al., 2017), investigate hematopoiesis during embryonic development (Mukamel et al., 2022), and study hematopoietic stem cell populations (Hui et al., 2018). This illustrates their power to uncover new cell types and intermediate differentiation stages. Additionally, even with low sequencing depth we consider that they can be employed to study proliferation-associated drift, as this can be calculated using DNAm levels over large genomic regions. While sequencing depth is of major importance, increased sequencing efforts cannot control for the loss of information due to degradation of DNA during bisulfite conversion. For this reason, alternative genome-wide methods based on methylation-sensitive restriction enzymes (MSRE) have been developed, such as epi-gSCAR (Niemoller et al., 2021) and DARE (Viswanathan et al., 2019). MSRE-based methods improve overlapping coverage, but at the same time they are not completely unbiased, since CpGs should be located inside an MSRE restriction site to be studied. Furthermore, they are plate-based, which limits cellular throughput to a few cells per experiment, unless one can count on the use of a robot to automate the plate-based processing.
To overcome constraints related to the analysis of the entire DNA methylome, we and others have developed targeted scDNAm methods. We saw the opportunity of combining MSRE with a microfluidics system, the Tapestri platform from Mission Bio, and developed scTAM-seq (Bianchi et al., 2022). Our methodology builds upon an important development to reduce the needed sequencing depth (and thus the price) by targeting biological informative CpGs (Figure 1D). Other targeted methods have so far focused on covering specific functional regions in the genome such as regulatory regions in sciMET-cap (Acharya et al., 2023), promoters in scRRBS (Guo et al., 2013; Gaiti et al., 2019; Gu et al., 2021), or LINEs and SINEs in scTEM-seq (Hunt et al., 2022). While these methods do reduce sequencing requirements, they still suffer from low overlapping coverage. Furthermore, scRRBS and scTEM-seq are plate-based which limits cellular throughput as mentioned above. Yet, such approaches have shed important light onto DNAm dynamics in relation to clonal hematopoiesis and CLL (Gaiti et al., 2019; Izzo et al., 2020). To maximize overlapping coverage and allow cell comparisons of a highly informative set of methylation sites at the single-CpG level, we developed the microfluidics-based method scTAM-seq, using a rigorous selection of approximately 400 genomic sites. This method allowed us not only to read out distinct B-cell stages but also to capture the continuous process of memory B-cell formation (Bianchi et al., 2022). Of notice, this method requires prior knowledge of the system to design the CpG panel. Nevertheless, we believe that our method will open new doors to characterise complex, continuous biological systems with transitional cell states such as hematological differentiation (Velten et al., 2017; Weinreb et al., 2020), cellular reprogramming (Kong et al., 2022), as well as tumor development and plasticity (Kinker et al., 2020; Nadeu et al., 2022).
Overall, in this section, we have discussed the major aspects to consider when opting to use scDNAm methods. The most suitable method to profile the DNA methylome in single cells will depend on 1) the knowledge of the model, 2) the biological question to be answered, and 3) the budget. That being said, to obtain a global snapshot of the DNAm landscape or build cellular DNAm atlases, unbiased genome-wide methods are the most appropriate (Luo et al., 2018; Nichols et al., 2022; Zhang et al., 2023), followed by less-expensive targeted approaches such as scRRBS (Guo et al., 2013) or sciMET-cap (Acharya et al., 2023). Based on the number of input cells, either plate-based (low input) or droplet-based/combinatorial-indexing-based (high input) methods would be preferred. Finally, if one aims to study a small set of highly informative CpGs (up to 900) at single-CpG level, we believe that scTAM-seq is optimal (Bianchi et al., 2022). The overlapping coverage that can be achieved by this method allows the study of DNAm variability at individual genomic loci as well as their combinatorial DNAm behaviors in unprecedented detail.
The single-cell field has evolved extensively in recent years, providing highly informative atlases of many tissue types through large-scale initiatives such as the Human Cell Atlas (Regev et al., 2017). The field has largely focused on transcriptomic and chromatin accessibility readouts though, with single-cell explorations of the DNA methylome lagging far behind. This implies that our view of the DNA methylome at single-cell level is still in its infancy, while highly relevant information can be extracted from this epigenetic layer. In this opinion piece, we describe the underlying biological features that can be read out from DNAm data and we lined out the main developments of the scDNAm field in recent years. In this way, we aim to underline the biological relevance of DNAm studies in the single-cell era, an emerging field with many opportunities. Importantly, the scDNAm field is rapidly progressing and while we write this piece, new technologies are being developed with the aim to increase the targeted amount of CpGs, overlapping coverage, and resolution, while reducing sequencing efforts and therefore the costs. Furthermore, though out of the scope of this piece, techniques are developed to combine DNAm studies with other (epi)genetic layers of information (Viswanathan et al., 2019; Niemoller et al., 2021; Bianchi et al., 2022; Angermueller et al., 2016; Hou et al., 2016; Guo et al., 2017; Clark et al., 2018; Linker et al., 2019; Gu et al., 2021; Hunt et al., 2022). Such single-cell multi-omics technologies shall aid in better understanding of the correlations between DNAm and other molecular features such as somatic mutations, copy number variations, chromatin accessibility, gene expression, cell-surface proteins, and alternative splicing. In summary, with all recent biological insights and technical advancements, the scDNAm field has exciting times ahead, heading towards deep characterisations of complex biological processes such as cell differentiation, cellular reprogramming, and tumor formation, capturing the potential paths cells can follow within these continuous landscapes.
LA: Funding acquisition, Writing–original draft, Writing–review and editing. AB: Writing–original draft, Writing–review and editing, Funding acquisition. RB: Conceptualization, Funding acquisition, Supervision, Writing–original draft, Writing–review and editing.
The author(s) declare financial support was received for the research, authorship, and/or publication of this article. We acknowledge support from the Spanish Ministry of Science and Innovation to the EMBL partnership, from the Centro de Excelencia Severo Ochoa and the CERCA Programme/Generalitat de Catalunya. AB is supported by an FPI fellowship from the Spanish Ministry of Science and Innovation (PRE2019-087574). LA received funding from the European Union’s Horizon 2020 research and innovation programme under the Marie Skłodowska-Curie grant agreement number 101034290 and CEX2020-001049-S financed by MCIN/AEI/10.13039/501100011033. RB was supported by a Junior Leader Fellowship from the la Caixa foundation and grants from the Spanish Ministry of Science and Innovation (RTI2018-096359-A-I00) and the European Hematology Association (EHA, Advanced Research Grant), and is supported by grants from the European Research Council (101039265) and the Spanish Ministry of Science and Innovation (PID2021-123635NB-I00).
The authors declare that the research was conducted in the absence of any commercial or financial relationships that could be construed as a potential conflict of interest.
All claims expressed in this article are solely those of the authors and do not necessarily represent those of their affiliated organizations, or those of the publisher, the editors and the reviewers. Any product that may be evaluated in this article, or claim that may be made by its manufacturer, is not guaranteed or endorsed by the publisher.
Acharya, S. N., Nichols, R. V., Rylaarsdam, L. E., O'Connell, B. L., Braun, T. P., and Adey, A. C. (2023). Scimet-cap: high-throughput single-cell methylation analysis with a reduced sequencing burden. bioRxiv, 2023, 548718. doi:10.1101/2023.07.12.548718
Angermueller, C., Clark, S. J., Lee, H. J., Macaulay, I. C., Teng, M. J., Hu, T. X., et al. (2016). Parallel single-cell sequencing links transcriptional and epigenetic heterogeneity. Nat. Methods 13 (3), 229–232. doi:10.1038/nmeth.3728
Barefoot, M. E., Loyfer, N., Kiliti, A. J., McDeed, A. P., Kaplan, T., and Wellstein, A. (2021). Detection of cell types contributing to cancer from circulating, cell-free methylated DNA. Front. Genet. 12, 671057. doi:10.3389/fgene.2021.671057
Bianchi, A., Scherer, M., Zaurin, R., Quililan, K., Velten, L., and Beekman, R. (2022). scTAM-seq enables targeted high-confidence analysis of DNA methylation in single cells. Genome Biol. 23 (1), 229. doi:10.1186/s13059-022-02796-7
Bird, A. P., and Southern, E. M. (1978). Use of restriction enzymes to study eukaryotic DNA methylation: I. The methylation pattern in ribosomal DNA from Xenopus laevis. J. Mol. Biol. 118 (1), 27–47. doi:10.1016/0022-2836(78)90242-5
Clark, S. J., Argelaguet, R., Kapourani, C. A., Stubbs, T. M., Lee, H. J., Alda-Catalinas, C., et al. (2018). scNMT-seq enables joint profiling of chromatin accessibility DNA methylation and transcription in single cells. Nat. Commun. 9 (1), 781. doi:10.1038/s41467-018-03149-4
Danilova, L., Wrangle, J., Herman, J. G., and Cope, L. (2022). DNA-methylation for the detection and distinction of 19 human malignancies. Epigenetics 17 (2), 191–201. doi:10.1080/15592294.2021.1890885
Domcke, S., Bardet, A. F., Adrian Ginno, P., Hartl, D., Burger, L., and Schübeler, D. (2015). Competition between DNA methylation and transcription factors determines binding of NRF1. Nature 528 (7583), 575–579. doi:10.1038/nature16462
Duran-Ferrer, M., Clot, G., Nadeu, F., Beekman, R., Baumann, T., Nordlund, J., et al. (2020). The proliferative history shapes the DNA methylome of B-cell tumors and predicts clinical outcome. Nat. Cancer 1 (11), 1066–1081. doi:10.1038/s43018-020-00131-2
Durek, P., Nordström, K., Gasparoni, G., Salhab, A., Kressler, C., de Almeida, M., et al. (2016). Epigenomic profiling of human CD4(+) T cells supports a linear differentiation model and highlights molecular regulators of memory development. Immunity 45 (5), 1148–1161. doi:10.1016/j.immuni.2016.10.022
Farlik, M., Halbritter, F., Müller, F., Choudry, F. A., Ebert, P., Klughammer, J., et al. (2016). DNA methylation dynamics of human hematopoietic stem cell differentiation. Cell Stem Cell 19 (6), 808–822. doi:10.1016/j.stem.2016.10.019
Feinberg, A. P., and Vogelstein, B. (1983). Hypomethylation distinguishes genes of some human cancers from their normal counterparts. Nature 301 (5895), 89–92. doi:10.1038/301089a0
Gaiti, F., Chaligne, R., Gu, H., Brand, R. M., Kothen-Hill, S., Schulman, R. C., et al. (2019). Epigenetic evolution and lineage histories of chronic lymphocytic leukaemia. Nature 569 (7757), 576–580. doi:10.1038/s41586-019-1198-z
Gama-Sosa, M. A., Slagel, V. A., Trewyn, R. W., Oxenhandler, R., Kuo, K. C., Gehrke, C. W., et al. (1983). The 5-methylcytosine content of DNA from human tumors. Nucleic Acids Res. 11 (19), 6883–6894. doi:10.1093/nar/11.19.6883
Gonzalez-Zulueta, M., Bender, C. M., Yang, A. S., Nguyen, T., Beart, R. W., Van Tornout, J. M., et al. (1995). Methylation of the 5' CpG island of the p16/CDKN2 tumor suppressor gene in normal and transformed human tissues correlates with gene silencing. Cancer Res. 55 (20), 4531–4535.
Gore, A. V., and Weinstein, B. M. (2016). DNA methylation in hematopoietic development and disease. Exp. Hematol. 44 (9), 783–790. doi:10.1016/j.exphem.2016.04.013
Grand, R. S., Burger, L., Gräwe, C., Michael, A. K., Isbel, L., Hess, D., et al. (2021). BANP opens chromatin and activates CpG-island-regulated genes. Nature 596 (7870), 133–137. doi:10.1038/s41586-021-03689-8
Gu, H., Raman, A. T., Wang, X., Gaiti, F., Chaligne, R., Mohammad, A. W., et al. (2021). Smart-RRBS for single-cell methylome and transcriptome analysis. Nat. Protoc. 16 (8), 4004–4030. doi:10.1038/s41596-021-00571-9
Guo, F., Li, L., Li, J., Wu, X., Hu, B., Zhu, P., et al. (2017). Single-cell multi-omics sequencing of mouse early embryos and embryonic stem cells. Cell Res. 27 (8), 967–988. doi:10.1038/cr.2017.82
Guo, H., Zhu, P., Wu, X., Li, X., Wen, L., and Tang, F. (2013). Single-cell methylome landscapes of mouse embryonic stem cells and early embryos analyzed using reduced representation bisulfite sequencing. Genome Res. 23 (12), 2126–2135. doi:10.1101/gr.161679.113
Herman, J. G., Merlo, A., Mao, L., Lapidus, R. G., Issa, J. P., Davidson, N. E., et al. (1995). Inactivation of the CDKN2/p16/MTS1 gene is frequently associated with aberrant DNA methylation in all common human cancers. Cancer Res. 55 (20), 4525–4530.
Hon, G. C., Rajagopal, N., Shen, Y., McCleary, D. F., Yue, F., Dang, M. D., et al. (2013). Epigenetic memory at embryonic enhancers identified in DNA methylation maps from adult mouse tissues. Nat. Genet. 45 (10), 1198–1206. doi:10.1038/ng.2746
Hotchkiss, R. D. (1948). The quantitative separation of purines, pyrimidines, and nucleosides by paper chromatography. J. Biol. Chem. 175 (1), 315–332. doi:10.1016/s0021-9258(18)57261-6
Hou, Y., Guo, H., Cao, C., Li, X., Hu, B., Zhu, P., et al. (2016). Single-cell triple omics sequencing reveals genetic, epigenetic, and transcriptomic heterogeneity in hepatocellular carcinomas. Cell Res. 26 (3), 304–319. doi:10.1038/cr.2016.23
Hui, T., Cao, Q., Wegrzyn-Woltosz, J., O'Neill, K., Hammond, C. A., Knapp, D. J. H. F., et al. (2018). High-resolution single-cell DNA methylation measurements reveal epigenetically distinct hematopoietic stem cell subpopulations. Stem Cell Rep. 11 (2), 578–592. doi:10.1016/j.stemcr.2018.07.003
Hunt, K. V., Burnard, S. M., Roper, E. A., Bond, D. R., Dun, M. D., Verrills, N. M., et al. (2022). Sctem-seq: single-cell analysis of transposable element methylation to link global epigenetic heterogeneity with transcriptional programs. Sci. Rep. 12 (1), 5776. doi:10.1038/s41598-022-09765-x
Izzo, F., Lee, S. C., Poran, A., Chaligne, R., Gaiti, F., Gross, B., et al. (2020). DNA methylation disruption reshapes the hematopoietic differentiation landscape. Nat. Genet. 52 (4), 378–387. doi:10.1038/s41588-020-0595-4
Jadhav, U., Cavazza, A., Banerjee, K. K., Xie, H., O'Neill, N. K., Saenz-Vash, V., et al. (2019). Extensive recovery of embryonic enhancer and gene memory stored in hypomethylated enhancer DNA. Mol. Cell 74 (3), 542–554. doi:10.1016/j.molcel.2019.02.024
Jones, P. A. (2012). Functions of DNA methylation: islands, start sites, gene bodies and beyond. Nat. Rev. Genet. 13 (7), 484–492. doi:10.1038/nrg3230
Kinker, G. S., Greenwald, A. C., Tal, R., Orlova, Z., Cuoco, M. S., McFarland, J. M., et al. (2020). Pan-cancer single-cell RNA-seq identifies recurring programs of cellular heterogeneity. Nat. Genet. 52 (11), 1208–1218. doi:10.1038/s41588-020-00726-6
Kong, W., Fu, Y. C., Holloway, E. M., Garipler, G., Yang, X., Mazzoni, E. O., et al. (2022). Capybara: a computational tool to measure cell identity and fate transitions. Cell Stem Cell 29 (4), 635–649 e11. doi:10.1016/j.stem.2022.03.001
Kreibich, E., Kleinendorst, R., Barzaghi, G., Kaspar, S., and Krebs, A. R. (2023). Single-molecule footprinting identifies context-dependent regulation of enhancers by DNA methylation. Mol. Cell 83 (5), 787–802 e9. doi:10.1016/j.molcel.2023.01.017
Kulis, M., Heath, S., Bibikova, M., Queirós, A. C., Navarro, A., Clot, G., et al. (2012). Epigenomic analysis detects widespread gene-body DNA hypomethylation in chronic lymphocytic leukemia. Nat. Genet. 44 (11), 1236–1242. doi:10.1038/ng.2443
Kulis, M., Merkel, A., Heath, S., Queirós, A. C., Schuyler, R. P., Castellano, G., et al. (2015). Whole-genome fingerprint of the DNA methylome during human B cell differentiation. Nat. Genet. 47 (7), 746–756. doi:10.1038/ng.3291
Linker, S. M., Urban, L., Clark, S. J., Chhatriwala, M., Amatya, S., McCarthy, D. J., et al. (2019). Combined single-cell profiling of expression and DNA methylation reveals splicing regulation and heterogeneity. Genome Biol. 20 (1), 30. doi:10.1186/s13059-019-1644-0
Liu, M. C., Oxnard, G. R., Klein, E. A., Swanton, C., and Seiden, M. V., (2020). Sensitive and specific multi-cancer detection and localization using methylation signatures in cell-free DNA. Ann. Oncol. 31 (6), 745–759. doi:10.1016/j.annonc.2020.02.011
Loyfer, N., Magenheim, J., Peretz, A., Cann, G., Bredno, J., Klochendler, A., et al. (2023). A DNA methylation atlas of normal human cell types. Nature 613 (7943), 355–364. doi:10.1038/s41586-022-05580-6
Luo, C., Keown, C. L., Kurihara, L., Zhou, J., He, Y., Li, J., et al. (2017). Single-cell methylomes identify neuronal subtypes and regulatory elements in mammalian cortex. Science 357 (6351), 600–604. doi:10.1126/science.aan3351
Luo, C., Rivkin, A., Zhou, J., Sandoval, J. P., Kurihara, L., Lucero, J., et al. (2018). Robust single-cell DNA methylome profiling with snmC-seq2. Nat. Commun. 9 (1), 3824. doi:10.1038/s41467-018-06355-2
Martens, J. H., and Stunnenberg, H. G. (2013). BLUEPRINT: mapping human blood cell epigenomes. Haematologica 98 (10), 1487–1489. doi:10.3324/haematol.2013.094243
Maurano, M. T., Wang, H., John, S., Shafer, A., Canfield, T., Lee, K., et al. (2015). Role of DNA methylation in modulating transcription factor occupancy. Cell Rep. 12 (7), 1184–1195. doi:10.1016/j.celrep.2015.07.024
Medeiros, B. C., Fathi, A. T., DiNardo, C. D., Pollyea, D. A., Chan, S. M., and Swords, R. (2017). Isocitrate dehydrogenase mutations in myeloid malignancies. Leukemia 31 (2), 272–281. doi:10.1038/leu.2016.275
Minteer, C. J., Thrush, K., Gonzalez, J., Niimi, P., Rozenblit, M., Rozowsky, J., et al. (2023). More than bad luck: cancer and aging are linked to replication-driven changes to the epigenome. Sci. Adv. 9 (29), eadf4163. doi:10.1126/sciadv.adf4163
Mukamel, Z., Lifshitz, A., Mittnenzweig, M., Chomsky, E., Schwartzman, O., Ben-Kiki, O., et al. (2022). DNA methyltransferases 3A and 3B target specific sequences during mouse gastrulation. Nat. Struct. Mol. Biol. 29 (12), 1252–1265. doi:10.1038/s41594-022-00885-6
Mulqueen, R. M., Pokholok, D., Norberg, S. J., Torkenczy, K. A., Fields, A. J., Sun, D., et al. (2018). Highly scalable generation of DNA methylation profiles in single cells. Nat. Biotechnol. 36 (5), 428–431. doi:10.1038/nbt.4112
Nadeu, F., Royo, R., Massoni-Badosa, R., Playa-Albinyana, H., Garcia-Torre, B., Duran-Ferrer, M., et al. (2022). Detection of early seeding of Richter transformation in chronic lymphocytic leukemia. Nat. Med. 28 (8), 1662–1671. doi:10.1038/s41591-022-01927-8
Nichols, R. V., O'Connell, B. L., Mulqueen, R. M., Thomas, J., Woodfin, A. R., Acharya, S., et al. (2022). High-throughput robust single-cell DNA methylation profiling with sciMETv2. Nat. Commun. 13 (1), 7627. doi:10.1038/s41467-022-35374-3
Niemoller, C., Wehrle, J., Riba, J., Claus, R., Renz, N., Rhein, J., et al. (2021). Bisulfite-free epigenomics and genomics of single cells through methylation-sensitive restriction. Commun. Biol. 4 (1), 153. doi:10.1038/s42003-021-01661-w
Queiros, A. C., Beekman, R., Vilarrasa-Blasi, R., Duran-Ferrer, M., Clot, G., Merkel, A., et al. (2016). Decoding the DNA methylome of mantle cell lymphoma in the light of the entire B cell lineage. Cancer Cell 30 (5), 806–821. doi:10.1016/j.ccell.2016.09.014
Regev, A., Teichmann, S. A., Lander, E. S., Amit, I., Benoist, C., Birney, E., et al. (2017). The human cell atlas. Elife, 6. doi:10.7554/eLife.27041
Roadmap Epigenomics, C., Kundaje, A., Meuleman, W., Ernst, J., Bilenky, M., Yen, A., et al. (2015). Integrative analysis of 111 reference human epigenomes. Nature 518 (7539), 317–330. doi:10.1038/nature14248
Roy, R., Ramamoorthy, S., Shapiro, B. D., Kaileh, M., Hernandez, D., Sarantopoulou, D., et al. (2021). DNA methylation signatures reveal that distinct combinations of transcription factors specify human immune cell epigenetic identity. Immunity 54 (11), 2465–2480 e5. doi:10.1016/j.immuni.2021.10.001
Stackpole, M. L., Zeng, W., Li, S., Liu, C. C., Zhou, Y., He, S., et al. (2022). Cost-effective methylome sequencing of cell-free DNA for accurately detecting and locating cancer. Nat. Commun. 13 (1), 5566. doi:10.1038/s41467-022-32995-6
Stadler, M. B., Murr, R., Burger, L., Ivanek, R., Lienert, F., Schöler, A., et al. (2011). DNA-binding factors shape the mouse methylome at distal regulatory regions. Nature 480 (7378), 490–495. doi:10.1038/nature10716
Stunnenberg, H. G., International Human Epigenome, C., and Hirst, M. (2016). The international human epigenome consortium: a blueprint for scientific collaboration and discovery. Cell 167 (5), 1145–1149. doi:10.1016/j.cell.2016.11.007
Velten, L., Haas, S. F., Raffel, S., Blaszkiewicz, S., Islam, S., Hennig, B. P., et al. (2017). Human haematopoietic stem cell lineage commitment is a continuous process. Nat. Cell Biol. 19 (4), 271–281. doi:10.1038/ncb3493
Viswanathan, R., Cheruba, E., and Cheow, L. F. (2019). DNA Analysis by Restriction Enzyme (DARE) enables concurrent genomic and epigenomic characterization of single cells. Nucleic Acids Res. 47 (19), e122. doi:10.1093/nar/gkz717
Weber, M., Hellmann, I., Stadler, M. B., Ramos, L., Pääbo, S., Rebhan, M., et al. (2007). Distribution, silencing potential and evolutionary impact of promoter DNA methylation in the human genome. Nat. Genet. 39 (4), 457–466. doi:10.1038/ng1990
Weinreb, C., Rodriguez-Fraticelli, A., Camargo, F. D., and Klein, A. M. (2020). Lineage tracing on transcriptional landscapes links state to fate during differentiation. Science 367 (6479), eaaw3381. doi:10.1126/science.aaw3381
Yang, Z., Wong, A., Kuh, D., Paul, D. S., Rakyan, V. K., Leslie, R. D., et al. (2016). Correlation of an epigenetic mitotic clock with cancer risk. Genome Biol. 17 (1), 205. doi:10.1186/s13059-016-1064-3
Youn, A., and Wang, S. (2018). The MiAge Calculator: a DNA methylation-based mitotic age calculator of human tissue types. Epigenetics 13 (2), 192–206. doi:10.1080/15592294.2017.1389361
Zhang, Q., Ma, S., Liu, Z., Zhu, B., Zhou, Z., Li, G., et al. (2023). Droplet-based bisulfite sequencing for high-throughput profiling of single-cell DNA methylomes. Nat. Commun. 14 (1), 4672. doi:10.1038/s41467-023-40411-w
Zhou, W., Dinh, H. Q., Ramjan, Z., Weisenberger, D. J., Nicolet, C. M., Shen, H., et al. (2018). DNA methylation loss in late-replicating domains is linked to mitotic cell division. Nat. Genet. 50 (4), 591–602. doi:10.1038/s41588-018-0073-4
Keywords: DNA methylation, single-cell technologies, hematopoiesis, hematological malignancies, epigenomics
Citation: Albinati L, Bianchi A and Beekman R (2023) The emerging field of opportunities for single-cell DNA methylation studies in hematology and beyond. Front. Mol. Biosci. 10:1286716. doi: 10.3389/fmolb.2023.1286716
Received: 31 August 2023; Accepted: 12 October 2023;
Published: 26 October 2023.
Edited by:
Jonas Samuel Jutzi, Harvard Medical School, United StatesReviewed by:
Mathijs Sanders, Erasmus MC Cancer Institute, NetherlandsCopyright © 2023 Albinati, Bianchi and Beekman. This is an open-access article distributed under the terms of the Creative Commons Attribution License (CC BY). The use, distribution or reproduction in other forums is permitted, provided the original author(s) and the copyright owner(s) are credited and that the original publication in this journal is cited, in accordance with accepted academic practice. No use, distribution or reproduction is permitted which does not comply with these terms.
*Correspondence: Renée Beekman, cmVuZWUuYmVla21hbkBjcmcuZXU=
†These authors have contributed equally to this work
Disclaimer: All claims expressed in this article are solely those of the authors and do not necessarily represent those of their affiliated organizations, or those of the publisher, the editors and the reviewers. Any product that may be evaluated in this article or claim that may be made by its manufacturer is not guaranteed or endorsed by the publisher.
Research integrity at Frontiers
Learn more about the work of our research integrity team to safeguard the quality of each article we publish.