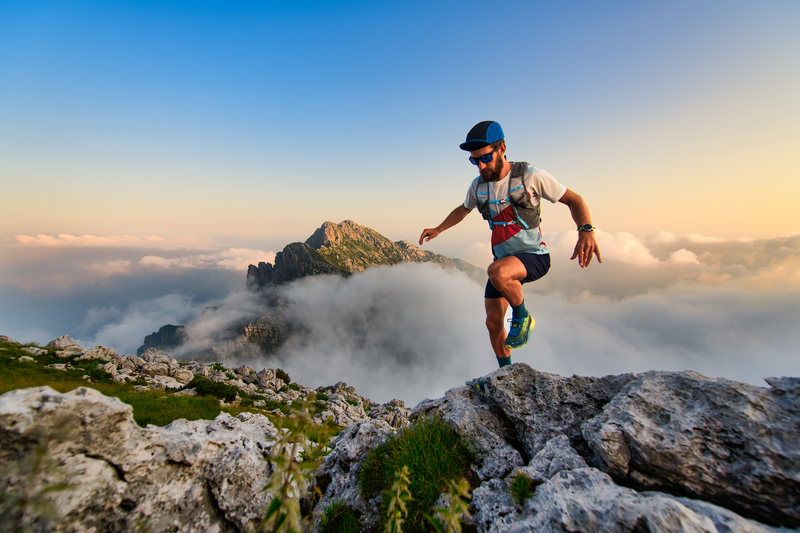
95% of researchers rate our articles as excellent or good
Learn more about the work of our research integrity team to safeguard the quality of each article we publish.
Find out more
ORIGINAL RESEARCH article
Front. Mol. Biosci. , 31 October 2023
Sec. Molecular Diagnostics and Therapeutics
Volume 10 - 2023 | https://doi.org/10.3389/fmolb.2023.1284623
This article is part of the Research Topic Machine Learning Approaches for Differential Diagnosis, Prognosis, Prevention, and Treatment of Digestive System Disorders View all 8 articles
Background: With a poor prognosis for affected individuals, pancreatic adenocarcinoma (PAAD) is known as a complicated and diverse illness. Immunocytes have become essential elements in the development of PAAD. Notably, sphingolipid metabolism has a dual function in the development of tumors and the invasion of the immune system. Despite these implications, research on the predictive ability of sphingolipid variables for PAAD prognosis is strikingly lacking, and it is yet unclear how they can affect PAAD immunotherapy and targeted pharmacotherapy.
Methods: The investigation process included SPG detection while also being pertinent to the prognosis for PAAD. Both the analytical capability of CIBERSORT and the prognostic capability of the pRRophetic R package were used to evaluate the immunological environments of the various HCC subtypes. In addition, CCK-8 experiments on PAAD cell lines were carried out to confirm the accuracy of drug sensitivity estimates. The results of these trials, which also evaluated cell survival and migratory patterns, confirmed the usefulness of sphingolipid-associated genes (SPGs).
Results: As a result of this thorough investigation, 32 SPGs were identified, each of which had a measurable influence on the dynamics of overall survival. This collection of genes served as the conceptual framework for the development of a prognostic model, which was carefully assembled from 10 chosen genes. It should be noted that this grouping of patients into cohorts with high and low risk was a sign of different immune profiles and therapy responses. The increased abundance of SPGs was identified as a possible sign of inadequate responses to immune-based treatment approaches. The careful CCK-8 testing carried out on PAAD cell lines was of the highest importance for providing clear confirmation of drug sensitivity estimates.
Conclusion: The significance of Sphingolipid metabolism in the complex web of PAAD development is brought home by this study. The novel risk model, built on the complexity of sphingolipid-associated genes, advances our understanding of PAAD and offers doctors a powerful tool for developing personalised treatment plans that are specifically suited to the unique characteristics of each patient.
Pancreatic adenocarcinoma is recognized as one of the most prevalent forms of primary malignancies globally (Huang C. et al., 2023; Huang X. et al., 2023). Notably, the complex interaction of inflammatory cascades plays a crucial role in the development of PAAD. This cancer develops from dysplastic nodules and progresses through a range of histopathological phases, each with unique molecular and cellular characteristics (Chi et al., 2023a). Despite sincere efforts to remove the tumor surgically (Li C. et al., 2023), PAAD patients typically experience poor prognosis results (Zhang B. et al., 2022; Zhang et al., 2023a; Cui et al., 2023). This bleak reality can be attributed to the PAAD’s substantial intratumoral and interpatient heterogeneity, which inexorably encourages the development of drug resistance events and disease recurrence (Baek and Lee, 2020; Chi et al., 2022a; Wen et al., 2023; Yu et al., 2023). Checkpoint inhibitor immunotherapy is a cutting-edge cancer treatment (Zhang L. et al., 2022; Su et al., 2022). Checkpoint inhibitors have emerged as pivotal agents in the management of PAAD, colorectal malignancies, and various other neoplastic diseases (Zhang P. et al., 2022; Zhang et al., 2023b; Zhang L. et al., 2023). Furthermore, PAAD’s complex interaction with the immunological environment has gained attention from researchers. The tailored RNA neoantigen vaccines treatment strategy for pancreatic cancer has received attention from recent clinical studies (Rojas et al., 2023).
However, the need for accurate identification and validation of reliable prognostic biomarkers poses a significant obstacle to the efficient translation of checkpoint immunotherapy to the PAAD scenario. This need stems from the urgent need to improve therapeutic strategies and, ultimately, patient outcomes. Therefore, there is still a need for novel biomolecular markers that predict patient prognoses, and their discovery might help PAAD enter a new age of individualized medication.
Sphingolipids, essential structural elements of cellular membranes, play a crucial and multidimensional function in the complex control of a variety of biological processes (Chi et al., 2022b). These include and go beyond important processes including cellular development, proliferation, directed migration, invasion, and even the metastatic cascade, particularly in the setting of malignancy (Ogretmen, 2018; Yuan et al., 2022a). As secondary messengers, they go beyond their structural function to exert control on cellular differentiation, senescence, programmed cell death, and general growth dynamics. Sphingomyelin, ceramide, sphingosine-1-phosphate, a substance reviewed in its name, and glycosphingolipids are among the fundamental components supporting the field of sphingolipids (Xiao et al., 2019; Sasset and Di Lorenzo, 2022). Dynamic changes in the complex sphingolipid biosynthesis pathway have the potential to delicately modify a variety of signaling cascades, dramatically affecting the course of carcinogenesis either as a promoter or an inhibitor (Guri et al., 2017; Muthusamy et al., 2020; Qi et al., 2021; Thayyullathil et al., 2021). In recent years, research on the role of sphingolipids in oncology has garnered significant attention, particularly in the context of tumor immunotherapy and chemotherapy (Zhang X. et al., 2023; Machy et al., 2023; Su et al., 2023). Studies have indicated that certain sphingolipids can modulate the immunogenicity of tumor cells, rendering them more susceptible to recognition and elimination by the immune system (Kozbor, 2010; Zhong et al., 2022). Furthermore, the alteration of sphingolipid composition on the membranes of immune cells has been demonstrated to enhance their activity, thereby augmenting their anti-tumor potential (Kue et al., 2012). These investigations provide robust support for the development of novel tumor immunotherapies. Sphingolipids also play a pivotal role in chemotherapy (Brachtendorf et al., 2019). Some studies have suggested that sphingolipids can influence the sensitivity of tumor cells to chemotherapeutic agents (Sousa et al., 2023). By regulating the levels of sphingolipids on cell membranes, it is possible to either increase or decrease the resistance of tumor cells to these drugs (Chiu et al., 2022). Recent studies have shown a significant correlation between some sphingolipid cohort members and the development of PAAD, providing predictive information about the course of the illness (Horejsi et al., 2023; Wilson et al., 2023). Despite the well-established importance of sphingolipids in the field of PAAD, there has been a noticeable lack of thorough investigations into the latent predictive value held by the SPGs, or short for sphingolipid orchestration genes, genes. A deeper and more thorough understanding of these genetic components has the potential to improve patient survival rates and increase response to treatment plans.
The evolution of bioinformatics has found extensive utility in the identification of biological markers and the diagnosis of diseases (Jin et al., 2021a; Jin et al., 2021b; Yan et al., 2021; Liu G. et al., 2022; Li et al., 2022). Nevertheless, exploration of genes associated with lipid bilayers remains notably limited. By using SPGs derived from the TCGA-PAAD cohort, our research aims to develop a reliable predictive model. Following the integration of this genetic data with essential clinicopathological factors, a nomogram is created that is designed to improve prognosis accuracy and provide customized therapeutic care methods. We meticulously validated the proposed nomogram using rigorous studies, such as time-dependent Receiver Operating Characteristic (ROC) and Decision Curve Analysis (DCA), to determine its clinical prognostic usefulness. These analytical methods allow for a thorough assessment of the nomogram’s performance, demonstrating its reliability for clinical settings. Our empirical findings suggest that sphingolipid-associated genes have the latent ability to predict the future course of individuals with PAAD. Additionally, the found genes provide up fresh possibilities as empirically confirmed indicators, hence increasing the pool of targets for targeted therapy approaches.
Four samples of normal pancreatic tissue and 179 cases of PAAD from the TCGA-PAAD cohort’s transcriptomic data were obtained from the TCGA repository (Zhai et al., 2020; Wang X. et al., 2022). Additionally, 179 PAAD tumor patients’ clinical data were gathered, a total of 179 PAAD patients were further filtered based on the completeness of survival time and age, as well as their survival status (Leek et al., 2012).
InnateDB stands as a publicly accessible repository, encompassing genes, proteins, experimentally validated interactions, and signaling pathways pertinent to the innate immune responses to microbial infections in humans. This repository augments the scope of innate immune interaction networks by amalgamating known interactions and pathways from major public databases with meticulously curated datasets into a centralized resource. Through the use of the InnateDB gateway (https://www.innatedb.com/index.jsp), 97 SPGs were assembled (Breuer et al., 2013).
In the context of this study, univariate Cox regression analysis was used to identify 32 SPGs that showed associations with PAAD patients’ survival rates. The “glmnet” R package (Song et al., 2022a) then made it easier to do LASSO regression analysis (Chi et al., 2023b; Ren et al., 2023), where the parameter was found using tenfold cross-validation (Zhao et al., 2023a). A multivariate Cox regression model was ultimately used to identify a group of ten key genes (Guan et al., 2023). Ten SPGs were used to create a risk signature by utilizing the best lambda scores and coefficients. Riskscore = SPTLC3*0.4708+STS*0.1031+KDSR*0.2274+SPHK1*0.1951+ARSJ*0.1008-ARSG*0.9889-CERS3*2.8795-CERS4*0.1164-SPHK2*0.3553-SMPD2*0.0189.
Utilizing the CIBERSORT and ssGSEA R scripts (Newman et al., 2015; Zhao S. et al., 2022; Song et al., 2022b; Ren et al., 2022) allowed for the measurement of infiltrating immune cell numbers. Immune cell type scores were calculated for individual samples using the CIBERSORT method (Zhao et al., 2023b). As a result, the predicted immune cell type scores were used to determine the scores for each sample. Furthermore, Spearman correlation analysis was used to look at the relationship between immune cell profiles and risk scores. The immune cell profiles of PAAD patients were used to inform the ssGSEA approach, which was then used to identify various risk categories for different people (Chi et al., 2023c).
The R package “pRRophetic” serves as a valuable tool for the application of gene expression data in predicting drug responses (Shen et al., 2022; Wang Z. et al., 2023). Its underlying principle relies on training models using established drug response data and subsequently employing these models to map new gene expression data onto predictions of drug responses. This approach contributes significantly to the realm of personalized medicine and the formulation of tailored pharmaceutical treatment strategies (Yuan et al., 2022b). The “pRRophetic” R program was used to calculate the IC50 of small molecule medicines.
The PAAD cell lines AsPC-1 and Panc-10.05, which were grown at 37 °C in a 5% CO2 atmosphere, were supplied by the ATCC firm. The culture media used was PRIM 1640 from Thermo Scientific, with 15% fetal bovine serum from Gibco as an addition (Song et al., 2021; Zhai et al., 2023).
The Cell Count Kit-8 (Dojindo, Japan) was utilized to determine the cell viability of AsPC-1 and Panc-10.05 cells. Cells were cultured at 37 °C with 5% CO2 for a period of 2 h after the addition of 10 L of CCK-8 reagent to each well. The subsequent measurement of optical density (OD) values at 450 nm ensued (Cai et al., 2022; Zhang Y. et al., 2023). Assessment of migration capability for AsPC-1 and Panc-10.05 cells was performed after a seeding interval of 48 h. Following that, the values of optical density (OD) at 450 nm were measured. After a 48-h seeding period, the capacity of AsPC-1 and Panc-10.05 cells to migrate was evaluated.
R version 4.1.3 was used to conduct all data analysis. The Student’s t-test was used to variables with a normal distribution, and Pearson’s correlation coefficient was used to evaluate inter-variable relationships. Statistical analysis of cellular experiments was conducted using GraphPad Prism 8 and SPSS Statistics v.27 software, with statistical analysis performed utilizing t-tests. The thresholds for statistical significance were p < 0.05*, p < 0.01**, and p < 0.001***, respectively.
The acquisition of a thorough set of 97 genes strongly associated with sphingolipid metabolism, painstakingly extracted from the prestigious InnateDB platform, served as the basis for our investigation. We obtained the necessary dataset from the TCGA database to provide a solid basis for our following studies as our investigation narrowed in on the complex landscape of PAAD. The “limma” R program was used to carefully go through the transcriptome data, finding genes within the sphingolipid pathway that had distinct expression patterns across the PAAD tumor samples and their corresponding surrounding normal tissues.
A thoughtful statistical methodology was used to identify a group of 35 SPGs that were associated to sphingolipids and showed notable expression difference (Figure 1A). The investigation of these SPGs went beyond simple expression alterations to include the critically important area of patient survival. Utilizing a comprehensive strategy, we evaluated the complex interactions between SPGs and the survival outcomes of PAAD patients using the analytical power of the “survival” and “survminer” R packages. A group of 32 SPGs that were closely linked to patient survival developed from the initial 35 SPGs (Figure 1B). Notably, a sizeable group of SPGs deviated from this general pattern and were negatively linked with good prognosis. These contrasts served as the foundation for the future creation of a PAAD prognostication prediction model. A model with possible therapeutic value was revealed by a careful Lasso analysis built around the 32 survival-associated SPGs (Figure 1C). It was crucial to confirm the predicted precision of this model. The model’s predictive accuracy was evaluated using time-dependent ROC curve analysis over many temporal horizons, including 1, 2, 3, and 5 years, all of which highlighted its dependability (Figure 1F). By using a dichotomization technique to divide the cohort of 179 PAAD patients into high- and low-risk categories based on median riskscore, the practical implications of this model were further highlighted. This line of demarcation clearly showed a perceptible survival disparity, with the high-risk group showing noticeably reduced survival (Figure 1E), where median survival durations of 1.3 and 2.8 years were recorded, respectively. Last but not least, we created a heatmap depicting the expression patterns of the top 10 SPGs across multiple riskscore groups to provide a visual summary of the expression trends within the context of riskscore stratification (Figure 1D).
FIGURE 1. Constructing a prognostic model based on sphingolipid-related genes in PAAD. (A) Differential gene screening was conducted to identify SPGs associated with PAAD. (B) 13 genes of prognostic significance, which we refer to as SPGs, were identified from the differential gene screening analysis. These SPGs demonstrated an association with survival in PAAD patients. (C) Utilizing the Lasso method, a prognostic model was constructed based on the identified SPGs. (D) The risk scores, survival status, and expression levels of the top 10-SPGs were plotted to visualize the distribution of prognostic risk. (E) Kaplan-Meier (KM) analysis was performed to further investigate the prognostic significance of the 10-SPGs in different PAAD subtypes. (F) The predictive efficiency of the prognostic model was evaluated using ROC analysis.
We conducted a thorough analysis of the transcriptional patterns of the ten SPGs in both normal and tumorous tissues using mRNA expression levels as a quantitative measure (Figure 2A). When compared to their nearby non-neoplastic counterparts, all 10 SPGs were evidently expressed differently in tumor tissues (p < 0.05), with SPHK1 particularly showing the highest level of expression. We subsequently examined the expression levels of these 10 SPGs among high-risk and low-risk subgroups in an effort to understand the underlying biological consequences of these observed patterns. We noticed a fascinating discrepancy between the ten SPGs in this subgroup’s expression pattern and the trend shown in Figure 1A, which is striking (Figure 2B). In order to get a deeper understanding, we used Kaplan-Meier survival curves to outline the relationship between each important SPG gene and the prognosis of PAAD patients. All 10 SPGs were shown to be statistically significantly correlated with patient prognosis according to this thorough research (p < 0.05). This highlights both their clinical importance and their potential to serve as prognostic indicators in PAAD, in addition to their clinical significance.
FIGURE 2. Expression levels of 10-SPGs. (A) Expression levels of 10-SPGs in PAAD tumor tissues and adjacent tissues. (B) Expression levels of 10-SPGs in PAAD risk subgroups. (*p < 0.05, **p < 0.01, ***p < 0.001).
In this part, we systematically examined the effects of several signaling pathway activations on the complex dynamics driving tumor cell proliferation and development, as well as their complex interaction within the tumor microenvironment. A systematic comparison of gene expression levels was carried out in order to identify genes with differential expression patterns that could be used to identify cohorts with high-risk and low-risk profiles (Figure 3A). A constellation of pathways with notable enrichment found among the group of individuals who showed increased vulnerability to PAAD. These pathways, which are individually highlighted by their significant enrichment coefficients (Figure 3B), include but are not limited to those of major relevance, such as Cancer Immunotherapy. As we dug further, the interesting details of the high-risk subgroup’s transcriptional landscape were revealed by our investigation of Gene Ontology enrichment. Conspicuously elevated were the processes that control cell-cell junctions, which are essential for cellular adhesion and communication. On the other hand, there was a noticeable downregulation of pathways involved in the negative regulation of immune effectors. This subtle modulation, seen in Figure 3C, points to a well planned interaction between tumor cells and their immune environment. Additionally, a concentrated investigation into the genes that differed the most between the high-risk and low-risk groupings made it possible to identify crucial Gene Ontology pathways. The connections between these pathways and the underlying genetics of risk stratification were clearly shown in (Figures 3D,E), shedding light on proposed molecular mechanisms controlling various risk traits.
FIGURE 3. Gene ontology (GO) and Kyoto Encyclopedia of Genes and Genomes (KEGG) analysis (A) Volcano map screening for differential genes. (B) Mountain map showing the enriched KEGG pathway. (C–E) GO enrichment analysis.
Tumor growth is heavily influenced by the microenvironment of a tumor, particularly the immune system. Tumor cells can avoid immune surveillance when the immune system is not working correctly (Zhao Y. et al., 2022; Chi et al., 2022c). We performed dimensionality reduction and clustering using the Lasso method on a particular collection of 10-SPGs selected for PAAD patients. Our study’ results confirm the effectiveness of these 10-SPGs in differentiating between PAAD patients with different risk propensities (Figure 4A). The complicated patterns of immune infiltration among PAAD patients, stratified by various prognostic factors, were further investigated (Figures 4B–D). The ordering of risk score values, which reflects the proportionate distribution of various immune cell subtypes, in ascending order is an interesting finding (Figure 4B). Importantly, our research reveals that among PAAD patients categorized as high-risk, there is a noticeable infiltration of certain immune cell types, including CD4 memory resting T-cell and dormant Mast cells. The CD8 T-cell and Tregs within this subgroup, on the other hand, show a noticeable drop (Figures 4C,D). Comparing high-risk PAAD patients to those with low risk reveals that there is less immune cell infiltration, which may be a sign of a weaker response to ICB treatments. Our analysis goes further and looks at the relationship between the 10-SPGs and immune cell populations (Figure 5) in order to further understand this discovery. Multiple immune cell types’ expression patterns of 3 SPGs were shown to be significantly correlated (Figure 5A). Notably, the infiltration of CD8 T-cell is strongly positively correlated with CERS3 and KDSR (Figures 5B,C). This link raises the possibility of a relationship between the immune milieu and the expression of these sphingolipid genes in PAAD patients.
FIGURE 4. Identify immune landscape of PAAD based on sphingolipid signature. (A) UMAP demonstrates different immune profiles among PAAD subgroups. (B) Proportion of immune cells in PAAD tissues. (C, D) Differences in immune infiltration between PAAD subgroups.
FIGURE 5. Correlation between immune cells and 10-SPGS. (A) Heatmap was used to show the correlation between immune cells and 10-sphingolipid genes (10-SPGs). (B, C) Bar plots were used to illustrate the relationship between CERS4 and KDSR with immune cell infiltration.
According to our analysis’s preliminary findings, there are observable differences in the immunological milieu across cohorts at high and low risk. Particularly, there is a notable decrease in the infiltration of both Tregs and CD8 T-cell within the high-risk population. A milieu that is immunologically quiescent is produced by the convergence of these changed immune components, and this microenvironment in turn has differential effects on the effectiveness of the two different immunotherapeutic methods under review. It is significant to note that individuals with decreased 10-SPG cluster expression levels have a propensity for good responses to both PD-L1 and PD-1 blocking therapy (Figure 6). It is interesting to note that the 10-SPG cluster also shows promise as a prognosis tool, providing the capacity to prognosticate the accuracy of ICB therapy in the population of patients with PAAD (Figures 6A,B). When we focus on the molecular environment, a striking indication that predicts a poorer prognosis for PAAD patients is the increase in KDSR expression inside high-risk PAAD cancer tissues. This poor prognosis is thought to be caused by the concurrent increase in immune response activity (Figure 1B, 2B), a theory supported by the elevated immune response shown in this subgroup (Figure 7A). We used a comprehensive strategy to try to understand the differences in ICB responses between high-risk and low-risk PAAD patients. We connected KDSR expression levels with the complex dynamics of alcohol exposure using the Tumor Immune Dysfunction and Exclusion (TIDE) algorithm (Figure 7B). Surprisingly, this combination of factors showed that increased KDSR expression is still a reliable indicator of elevated immune response scores, unaffected by the subtleties of alcohol use. We looked further into the molecular factors after being intrigued by the effect of KDSR on immune response score. We also looked at the immune checkpoint expression patterns within the group that had been divided up by various SPG risk ratings. Surprisingly, a recurrent pattern showed that CD274, and CTLA4 were among the critical immunological checkpoints that were significantly upregulated in the high KDSR expression subgroup (Figures 7C,D). Finally, we utilized the computational capability of Cibersort analysis to fully assess the immunological milieu. Using this analytical method, we were able to compare the immune cell infiltration rates between the normal, low-risk, and high-risk PAAD tissue specimens (Figures 7E,F). The results of this research highlighted clear differences in immune cell infiltration across the various groups, providing better understandings of the complex interactions between immunological profiles and disease risk.
FIGURE 6. Immunotherapy response prediction. (A, B) Prediction of immune therapy response to anti-PD-1/CTLA-4 treatment in PAAD patients based on 10-SPGS.
FIGURE 7. The level of immune checkpoint in PAAD subtypes. (A) The TIDE score between KDSR subgroups. (B) Alcohol does not affect the effectiveness of immunotherapy. (C, D) There are differences in the expression of immune checkpoint markers between the high-risk and low-risk groups of PAAD. (E, F) CIBERSORT analysis revealed differences in immune infiltration between the subgroups.
Poor outcomes in cancer are significantly influenced by the recurrence of the disease through metastasis (Chen et al., 2019). We investigated the differences in drug response to chemotherapeutic drugs among subgroups distinguished by increased and lowered risk scores in the search for customized treatments for PAAD patients. Our study compared the IC50 values of five different chemotherapeutic drugs in the high-risk and low-risk clusters, two clearly defined subpopulations with different risk ratings (Figure 8, Supplementary Table S1). The findings of our investigation revealed significant differences in the IC50 measurements for a few drugs, including Axitinib. This finding suggests that those with high riskscores might potentially show increased vulnerability to this particular chemotherapy regimen.
We evaluated other PAAD cell lines’ risk scores based on the mRNA expression of each SPGs (Figure 9), in order to support our findings and provide another layer of validation. The Panc 10.05 and AsPC-1 cell lines were chosen to adequately reflect the two dichotomous groups of PAAD patients, defined by their high-risk and low-risk scores, respectively, in the context of drug responsiveness tests. Our CCK-8 test findings showed that AsPC-1 cells, a representative of the low-risk population, displayed a more pronounced sensitivity to Bicalutamide as compared to their Panc 10.05 counterparts, which was consistent with the predictions about drug responsiveness (Figure 10A). In contrast, Panc 10.05 cells were more sensitive to Axitinib compared with AsPC-1 cells (Figure 10B). This agreement supports the idea that Bicalutamide and Axitinib could develop into a good candidate for targeted, precision-oriented treatment approaches for PAAD patients (Figures 9C,D).
FIGURE 10. Drug sensitivity experiments. (A, C) The CCK-8 assay revealed the cytotoxic effects of Bicalutamide on Panc-10.05 and AsPC-1 cells at different concentrations. (B, D) The CCK-8 assay revealed the cytotoxic effects of Axitinib on Panc-10.05 and AsPC-1 cells at different concentrations. (E, F) T-cell proliferation induced by pancreatic cancer cells.
The work that came before it highlights the elevated immunoreactivity that high-risk PAAD patients show in comparison to their low-risk peers. We suggest that identifying high-risk PAAD cells based on genes associated to sphingolipids might result in a more robust stimulation of T-cell maturation. To test this hypothesis, we co-cultured T-cell with Panc 10.05 cells, which had a high riskscore, and AsPC-1 cells, which had a low risk score, for a period of 14 days. We then observed the level of T-cell activation and proliferation. Our findings demonstrate a significantly higher rate of T-cell proliferation in Panc 10.05 cells with a high risk score compared to AsPC-1 cells (Figures 10E,F), suggesting a potential relationship between Sphingolipid-related genes and the expression of surface-mutated proteins and, in turn, inducing a higher response to T-cell proliferation.
The onset of diseases is frequently influenced by a multitude of factors, encompassing hormones (Luo et al., 2021), metabolic byproducts (Liu J. et al., 2023; Zhang et al., 2023f), inflammatory states (Xiao et al., 2020; Ni et al., 2021; Zhang et al., 2023g). Notably, certain determinants can precipitate the genesis of neoplasms. For instance, epigenetics is intricately intertwined with tumorigenesis (Wang J. et al., 2022; Zhang et al., 2023h), while microorganisms and immune cells play pivotal roles in shaping the course of neoplastic developments (Gong et al., 2023; Xia et al., 2023). Furthermore, the migratory propensity of tumor cells is closely associated with adverse prognoses and recurrent occurrences (Li Z. et al., 2023). A dangerous cancer known as pancreatic adenocarcinoma poses a serious threat to human life (Yuan et al., 2022c; Liu X. et al., 2023; Zhang et al., 2023i). The improvement of prognosis is a challenging endeavor because to the complex molecular pathways underlying PAAD. Single pharmacological therapies or single-targeted pathway interventions are ineffective in improving the prognosis of PAAD (Halbrook et al., 2023; Qi et al., 2023; Vahabi et al., 2023). As a result, using a wide variety of genes to build prognostic models turns out to be a more effective tactic. However, there are currently not enough effective biomarkers designed to achieve this goal. The need for further biomarkers is urgently needed to improve prognostic model accuracy and enable preventative actions against PAAD in its early stages.
Sphingolipids, a family of lipids, have a significant role in the maintenance of structural integrity and the sensitive management of membrane fluidity, as previously noted in studies (Zhang et al., 2023a). Cellular membranes are complex assemblages of various lipids. In particular, tumor cells’ metabolic needs are essential for their continued growth and survival inside the intricate tumor microenvironment (Soltani et al., 2021). Sphingolipids, recognized as bioactive molecules in the lipid repertory, have a diverse role in a variety of key cellular functions, acting not only as structural elements but also as essential mediators in cell signaling pathways (Gault et al., 2010). The thorough characterization and subsequent cloning of the essential metabolic enzymes controlling the complex homeostasis of sphingolipid components have been the focus of recent research endeavors. Their considerable influence on the molecular environment of cancer formation and the subtleties of therapy responses have been shown by this investigation (Snider et al., 2019). Recent research has illustrated the significance of sphingolipid metabolism in both lung cancer and breast cancer. Moreover, pivotal molecular targets associated with these malignancies have been pinpointed (Pei et al., 2023). In this setting, it is becoming clear that sphingolipid homeostasis disruption may play a role in the etiology of a variety of cancer morphologies, including the mysterious pancreatic adenocarcinoma. The tumor microenvironment (TME), which extends beyond the boundaries of specific cell populations, appears as a dynamic ecological niche and includes a variety of cellular and extracellular elements (Huang et al., 2023c), including tumor-associated macrophages (TAMs) (Wu et al., 2023), T-cell, and B-cell, each of which has a specific impact on the neoplastic milieu (Bejarano et al., 2021; Gong et al., 2022; Ch et al., 2023; Xiong et al., 2023). The TME is orchestrated by this complex interaction, which has a profound impact on the course of carcinogenesis and the effectiveness of treatments (Huan et al., 2023; Zhang et al., 2023j). A growing body of research has shown that the extraordinary variety within the TME supports the diverse responses seen in the context of different treatment approaches (Jin and Jin, 2020; Ng et al., 2020; Xiang et al., 2021; Chen et al., 2022).
Enrichment analyses, both using Gene Ontology (GO) and Kyoto Encyclopedia of Genes and Genomes (KEGG), have unveiled the involvement of sphingolipids in modulating the PI3K-AKT signaling pathway and T-cell proliferation pathways in the progression of PAAD. It is noteworthy that ongoing research has underscored the significant participation of sphingolipids and their associated enzymes in mediating the PI3K/AKT pathway’s influence on the growth of non-small cell lung cancer (NSCLC) cells (Gulhane and Singh, 2022). T-cell, pivotal actors in the context of NSCLC, play a vital role (Wang J. et al., 2023). These observations suggest that sphingolipids may exert their influence on the progression of PAAD by modulating the PI3K/AKT pathway and regulating T-cell activity.
Sphingolipids have been found to have a substantial role in cancer, intricately interacting with a variety of carcinogenic pathways (Ordonez et al., 2015; Liu et al., 2017; Cheng et al., 2018; Chi et al., 2022b; Liu C. et al., 2022; Kawai et al., 2022). Despite this acknowledgment, our knowledge of several genes involved in the regulation of sphingolipids is still limited, necessitating thorough exploration, particularly in light of their potential as therapeutic targets in clinical settings (Zhong et al., 2022; Zhang et al., 2023k). The cornerstone of a strong risk score signature in this study has been revealed to be a cohort of 10 genes, offering insight on their possible ramifications. In order to confirm these results, transwell tests and a wound-healing assay were carried out (Figures 9A,C,E). Given the likely function of increased SPGs expression in promoting the migratory potential of PAAD cells, our findings support the idea that sphingolipids could in fact constitute a feasible therapeutic approach for treating PAAD. The future importance of sphingolipid-focused treatments in the treatment of PAAD is thus highlighted by this study.
Changes in myelin metabolism have a significant impact on how chemosensitive neoplastic cells are, according to earlier studies. Axitinib sensitivity in people with PAAD who have elevated risk scores has been found to be particularly sensitive in the current study’s assessment of medication responsiveness (Figure 8). Using the CCK-8 test, as shown in Figure 10B, it was possible to verify the accuracy of drug predictions. The resulting results particularly highlights Axitinib’s potential eligibility as an effective treatment approach for people suffering from PAAD, particularly those who show an overexpression of SPGs (Figure 10D).
In earlier research projects, the use of gene expression profiling to classify tumor samples has been thoroughly verified (Brown and Elenitoba-Johnson, 2020; Jin et al., 2021c; Sirinukunwattana et al., 2021; Chi et al., 2022d; Jin et al., 2022). On the basis of the transcriptional levels displayed by 10 important sphingolipid-associated genes, we have attempted to classify a clinical cohort made up of PAAD patients against this scientific background. Through this methodical stratification, we have revealed striking differences in prognostic outcomes, highlighting in a profound way the strong predictive ability inherent to our genomic model, both in terms of prognosticating patient outcomes and in terms of prognosticating their responsiveness to therapeutic protocols across the spectrum from immunotherapeutic interventions to chemotherapeutic modalities. Covering the gamut from chemotherapeutic techniques to immunotherapeutic treatments. The quantity of knowledge so acquired has the potential to provide significant clinical guidance, enabling healthcare professionals to make well-informed therapy decisions for the cohort suffering from PAAD.
This study exhibits certain limitations. Despite the construction of a prognostic model based on sphingolipid-associated genes, the restricted size of the PAAD cohort hinders the extensive clinical application of this model. Furthermore, comprehensive in vitro experiments are imperative, whether for validating drug sensitivity or enriching the results concerning GO and KEGG signaling pathways. Predictions pertaining to immunotherapy necessitate a larger cohort of immunotherapy cases to substantiate their precision.
Our research efforts have resulted in the creation of a unique combination of 10 genes that make up a prognostic model. This development has the potential to help medical professionals precisely customize therapy regimens to each patient’s unique needs who has PAAD. In a related discovery, our research has shown a hitherto unknown connection between the complex environment of the immune system and the genes controlling sphingolipid metabolism. This paradigm-shifting relationship not only broadens our understanding at a fundamental level but also ushers in a new approach to immunotherapy. By focusing on the crucial sphingolipid genes, the possibility of making PAAD more susceptible to specific anti-tumor therapies emerges as an intriguing possibility deserving of future investigation.
The original contributions presented in the study are included in the article/Supplementary Material, further inquiries can be directed to the corresponding author.
Ethical approval was not required for the studies on humans in accordance with the local legislation and institutional requirements because only commercially available established cell lines were used.
TS: Data curation, Formal Analysis, Investigation, Methodology, Software, Visualization, Writing–original draft. ML: Visualization, Writing–original draft. YY: Conceptualization, Funding acquisition, Project administration, Supervision, Validation, Writing–review and editing.
The author(s) declare that no financial support was received for the research, authorship, and/or publication of this article.
All authors acknowledge the contributions from the TCGA project.
The authors declare that the research was conducted in the absence of any commercial or financial relationships that could be construed as a potential conflict of interest.
All claims expressed in this article are solely those of the authors and do not necessarily represent those of their affiliated organizations, or those of the publisher, the editors and the reviewers. Any product that may be evaluated in this article, or claim that may be made by its manufacturer, is not guaranteed or endorsed by the publisher.
The Supplementary Material for this article can be found online at: https://www.frontiersin.org/articles/10.3389/fmolb.2023.1284623/full#supplementary-material
Baek, B., and Lee, H. (2020). Prediction of survival and recurrence in patients with pancreatic cancer by integrating multi-omics data. Sci. Rep. 10, 18951. doi:10.1038/s41598-020-76025-1
Bejarano, L., Jordao, M. J. C., and Joyce, J. A. (2021). Therapeutic targeting of the tumor microenvironment. Cancer Discov. 11, 933–959. doi:10.1158/2159-8290.CD-20-1808
Brachtendorf, S., El-Hindi, K., and Grosch, S. (2019). Ceramide synthases in cancer therapy and chemoresistance. Prog. Lipid Res. 74, 160–185. doi:10.1016/j.plipres.2019.04.002
Breuer, K., Foroushani, A. K., Laird, M. R., Chen, C., Sribnaia, A., Lo, R., et al. (2013). InnateDB: systems biology of innate immunity and beyond--recent updates and continuing curation. Nucleic Acids Res. 41, D1228–D1233. doi:10.1093/nar/gks1147
Brown, N. A., and Elenitoba-Johnson, K. S. J. (2020). Enabling precision oncology through precision Diagnostics. Annu. Rev. Pathol. 15, 97–121. doi:10.1146/annurev-pathmechdis-012418-012735
Cai, W., Zhang, Y., Jin, W., Wei, S., Chen, J., Zhong, C., et al. (2022). Procyanidin B2 ameliorates the progression of osteoarthritis: an in vitro and in vivo study. Int. Immunopharmacol. 113, 109336. doi:10.1016/j.intimp.2022.109336
Chen, T. W., Yin, F. F., Yuan, Y. M., Guan, D. X., Zhang, E., Zhang, F. K., et al. (2019). CHML promotes liver cancer metastasis by facilitating Rab14 recycle. Nat. Commun. 10, 2510. doi:10.1038/s41467-019-10364-0
Chen, X., Chi, H., Zhao, X., Pan, R., Wei, Y., and Han, Y. (2022). Role of exosomes in immune microenvironment of hepatocellular carcinoma. J. Oncol. 2022, 2521025. doi:10.1155/2022/2521025
Cheng, J. C., Wang, E. Y., Yi, Y., Thakur, A., Tsai, S. H., and Hoodless, P. A. (2018). S1P stimulates proliferation by upregulating CTGF expression through S1PR2-mediated YAP activation. Mol. Cancer Res. 16, 1543–1555. doi:10.1158/1541-7786.MCR-17-0681
Chi, H., Chen, H., Wang, R., Zhang, J., Jiang, L., Zhang, S., et al. (2023a). Proposing new early detection indicators for pancreatic cancer: combining machine learning and neural networks for serum miRNA-based diagnostic model. Front. Oncol. 13, 1244578. doi:10.3389/fonc.2023.1244578
Chi, H., Gao, X., Xia, Z., Yu, W., Yin, X., Pan, Y., et al. (2023b). FAM family gene prediction model reveals heterogeneity, stemness and immune microenvironment of UCEC. Front. Mol. Biosci. 10, 1200335. doi:10.3389/fmolb.2023.1200335
Chi, H., Jiang, P., Xu, K., Zhao, Y., Song, B., Peng, G., et al. (2022d). A novel anoikis-related gene signature predicts prognosis in patients with head and neck squamous cell carcinoma and reveals immune infiltration. Front. Genet. 13, 984273. doi:10.3389/fgene.2022.984273
Chi, H., Peng, G., Wang, R., Yang, F., Xie, X., Zhang, J., et al. (2022a). Cuprotosis programmed-cell-death-related lncRNA signature predicts prognosis and immune landscape in PAAD patients. Cells 11, 3436. doi:10.3390/cells11213436
Chi, H., Peng, G., Yang, J., Zhang, J., Song, G., Xie, X., et al. (2022b). Machine learning to construct sphingolipid metabolism genes signature to characterize the immune landscape and prognosis of patients with uveal melanoma. Front. Endocrinol. (Lausanne) 13, 1056310. doi:10.3389/fendo.2022.1056310
Chi, H., Xie, X., Yan, Y., Peng, G., Strohmer, D. F., Lai, G., et al. (2022c). Natural killer cell-related prognosis signature characterizes immune landscape and predicts prognosis of HNSCC. Front. Immunol. 13, 1018685. doi:10.3389/fimmu.2022.1018685
Chi, H., Yang, J., Peng, G., Zhang, J., Song, G., Xie, X., et al. (2023c). Circadian rhythm-related genes index: a predictor for HNSCC prognosis, immunotherapy efficacy, and chemosensitivity. Front. Immunol. 14, 1091218. doi:10.3389/fimmu.2023.1091218
Chi, H., Zhao, S., Yang, J., Gao, X., Peng, G., Zhang, J., et al. (2023). T-cell exhaustion signatures characterize the immune landscape and predict HCC prognosis via integrating single-cell RNA-seq and bulk RNA-sequencing. Front. Immunol. 14, 1137025. doi:10.3389/fimmu.2023.1137025
Chiu, C. C., Chen, Y. C., Bow, Y. D., Chen, J. Y., Liu, W., Huang, J. L., et al. (2022). diTFPP, a phenoxyphenol, sensitizes hepatocellular carcinoma cells to C(2)-ceramide-induced autophagic stress by increasing oxidative stress and ER stress accompanied by LAMP2 hypoglycosylation. Cancers (Basel) 14, 2528. doi:10.3390/cancers14102528
Cui, Y., Yuan, Q., Chen, J., Jiang, J., Guan, H., Zhu, R., et al. (2023). Determination and characterization of molecular heterogeneity and precision medicine strategies of patients with pancreatic cancer and pancreatic neuroendocrine tumor based on oxidative stress and mitochondrial dysfunction-related genes. Front. Endocrinol. (Lausanne) 14, 1127441. doi:10.3389/fendo.2023.1127441
Gault, C. R., Obeid, L. M., and Hannun, Y. A. (2010). An overview of sphingolipid metabolism: from synthesis to breakdown. Adv. Exp. Med. Biol. 688, 1–23. doi:10.1007/978-1-4419-6741-1_1
Gong, X., Chi, H., Strohmer, D. F., Teichmann, A. T., Xia, Z., and Wang, Q. (2022). Exosomes: a potential tool for immunotherapy of ovarian cancer. Front. Immunol. 13, 1089410. doi:10.3389/fimmu.2022.1089410
Gong, X., Chi, H., Xia, Z., Yang, G., and Tian, G. (2023). Advances in HPV-associated tumor management: therapeutic strategies and emerging insights. J. Med. Virol. 95, e28950. doi:10.1002/jmv.28950
Guan, H., Chen, X., Liu, J., Sun, J., Guo, H., Jiang, Y., et al. (2023). Molecular characteristics and therapeutic implications of Toll-like receptor signaling pathway in melanoma. Sci. Rep. 13, 13788. doi:10.1038/s41598-023-38850-y
Gulhane, P., and Singh, S. (2022). MicroRNA-520c-3p impacts sphingolipid metabolism mediating PI3K/AKT signaling in NSCLC: systems perspective. J. Cell Biochem. 123, 1827–1840. doi:10.1002/jcb.30319
Guri, Y., Colombi, M., Dazert, E., Hindupur, S. K., Roszik, J., Moes, S., et al. (2017). mTORC2 promotes tumorigenesis via lipid synthesis. Cancer Cell 32, 807–823. doi:10.1016/j.ccell.2017.11.011
Halbrook, C. J., Lyssiotis, C. A., Pasca di Magliano, M., and Maitra, A. (2023). Pancreatic cancer: advances and challenges. Cell 186, 1729–1754. doi:10.1016/j.cell.2023.02.014
Horejsi, K., Jin, C., Vankova, Z., Jirasko, R., Strouhal, O., Melichar, B., et al. (2023). Comprehensive characterization of complex glycosphingolipids in human pancreatic cancer tissues. J. Biol. Chem. 299, 102923. doi:10.1016/j.jbc.2023.102923
Huang, C., Li, H., Xu, Y., Xu, C., Sun, H., Li, Z., et al. (2023a). BICC1 drives pancreatic cancer progression by inducing VEGF-independent angiogenesis. Signal Transduct. Target Ther. 8, 271. doi:10.1038/s41392-023-01478-5
Huang, L., Jin, W., Bao, Y., Zeng, X., Zhang, Y., Zhou, J., et al. (2023c). Identification and validation of long noncoding RNA AC083900.1 and RP11-283C24.1 for prediction of progression of osteosarcoma. Mutat. Res. 827, 111828. doi:10.1016/j.mrfmmm.2023.111828
Huang, L., Sun, F., Liu, Z., Jin, W., Zhang, Y., Chen, J., et al. (2023). Probing the potential of defense response-associated genes for predicting the progression, prognosis, and immune microenvironment of osteosarcoma. Cancers (Basel) 15, 2405. doi:10.3390/cancers15082405
Huang, X., Chi, H., Gou, S., Guo, X., Li, L., Peng, G., et al. (2023b). An aggrephagy-related LncRNA signature for the prognosis of pancreatic adenocarcinoma. Genes (Basel) 14, 124. doi:10.3390/genes14010124
Jin, M. Z., and Jin, W. L. (2020). The updated landscape of tumor microenvironment and drug repurposing. Signal Transduct. Target Ther. 5, 166. doi:10.1038/s41392-020-00280-x
Jin, W., Liu, Z., Zhang, Y., Che, Z., and Gao, M. (2021a). The effect of individual musculoskeletal conditions on depression: updated insights from an Irish longitudinal study on aging. Front. Med. (Lausanne) 8, 697649. doi:10.3389/fmed.2021.697649
Jin, W., Yang, Q., Chi, H., Wei, K., Zhang, P., Zhao, G., et al. (2022). Ensemble deep learning enhanced with self-attention for predicting immunotherapeutic responses to cancers. Front. Immunol. 13, 1025330. doi:10.3389/fimmu.2022.1025330
Jin, W., Yao, Q., Liu, Z., Cao, W., Zhang, Y., Che, Z., et al. (2021b). Do eye diseases increase the risk of arthritis in the elderly population? Aging (Albany NY) 13, 15580–15594. doi:10.18632/aging.203122
Jin, W., Zhang, Y., Liu, Z., Che, Z., Gao, M., and Peng, H. (2021c). Exploration of the molecular characteristics of the tumor-immune interaction and the development of an individualized immune prognostic signature for neuroblastoma. J. Cell Physiol. 236, 294–308. doi:10.1002/jcp.29842
Kawai, H., Osawa, Y., Matsuda, M., Tsunoda, T., Yanagida, K., Hishikawa, D., et al. (2022). Sphingosine-1-phosphate promotes tumor development and liver fibrosis in mouse model of congestive hepatopathy. Hepatology 76, 112–125. doi:10.1002/hep.32256
Kozbor, D. (2010). Cancer vaccine with mimotopes of tumor-associated carbohydrate antigens. Immunol. Res. 46, 23–31. doi:10.1007/s12026-009-8120-y
Kue, C. S., Jung, M. Y., Cho, D., and Kim, T. S. (2012). C6-ceramide enhances Interleukin-12-mediated T helper type 1 cell responses through a cyclooxygenase-2-dependent pathway. Immunobiology 217, 601–609. doi:10.1016/j.imbio.2011.10.021
Leek, J. T., Johnson, W. E., Parker, H. S., Jaffe, A. E., and Storey, J. D. (2012). The sva package for removing batch effects and other unwanted variation in high-throughput experiments. Bioinformatics 28, 882–883. doi:10.1093/bioinformatics/bts034
Li, C., Liu, Y. J. B., Ma, Y., and Yuan, M. (2023a). Efficacy and safety of acupuncture combined with herbal medicine for children and adolescents with Tourette syndrome: a Systematic Review and Meta-Analysis. Biomaterials Biosens. 2, 1–15. doi:10.1159/000534115
Li, M., Lan, F., Li, C., Li, N., Chen, X., Zhong, Y., et al. (2022). Expression and regulation network of HDAC3 in acute myeloid leukemia and the implication for targeted therapy based on multidataset data mining. Comput. Math. Methods Med. 2022, 4703524. doi:10.1155/2022/4703524
Li, Z., Zhou, H., Xia, Z., Xia, T., Du, G., Franziska, S. D., et al. (2023b). HMGA1 augments palbociclib efficacy via PI3K/mTOR signaling in intrahepatic cholangiocarcinoma. Biomark. Res. 11, 33. doi:10.1186/s40364-023-00473-w
Liu, C., Chen, K., Zhao, F., Xuan, L., Wang, Y., Xu, C., et al. (2022b). Occult infection with hepatitis B virus PreS variants synergistically promotes hepatocellular carcinoma development in a high-fat diet context by generating abnormal ceramides. BMC Med. 20, 279. doi:10.1186/s12916-022-02481-3
Liu, G., Xiong, D., Che, Z., Chen, H., and Jin, W. (2022a). A novel inflammation-associated prognostic signature for clear cell renal cell carcinoma. Oncol. Lett. 24, 307. doi:10.3892/ol.2022.13427
Liu, H., Ma, Y., He, H. W., Zhao, W. L., and Shao, R. G. (2017). SPHK1 (sphingosine kinase 1) induces epithelial-mesenchymal transition by promoting the autophagy-linked lysosomal degradation of CDH1/E-cadherin in hepatoma cells. Autophagy 13, 900–913. doi:10.1080/15548627.2017.1291479
Liu, J., Zhang, P., Yang, F., Jiang, K., Sun, S., Xia, Z., et al. (2023a). Integrating single-cell analysis and machine learning to create glycosylation-based gene signature for prognostic prediction of uveal melanoma. Front. Endocrinol. (Lausanne) 14, 1163046. doi:10.3389/fendo.2023.1163046
Liu, X., Tang, R., Xu, J., Tan, Z., Liang, C., Meng, Q., et al. (2023b). CRIP1 fosters MDSC trafficking and resets tumour microenvironment via facilitating NF-κB/p65 nuclear translocation in pancreatic ductal adenocarcinoma. Gut gutjnl-2022-329349. doi:10.1136/gutjnl-2022-329349
Luo, D., Jin, B., Zhai, X., Li, J., Liu, C., Guo, W., et al. (2021). Oxytocin promotes hepatic regeneration in elderly mice. iScience 24, 102125. doi:10.1016/j.isci.2021.102125
Machy, P., Mortier, E., and Birkle, S. (2023). Biology of GD2 ganglioside: implications for cancer immunotherapy. Front. Pharmacol. 14, 1249929. doi:10.3389/fphar.2023.1249929
Muthusamy, T., Cordes, T., Handzlik, M. K., You, L., Lim, E. W., Gengatharan, J., et al. (2020). Serine restriction alters sphingolipid diversity to constrain tumour growth. Nature 586, 790–795. doi:10.1038/s41586-020-2609-x
Newman, A. M., Liu, C. L., Green, M. R., Gentles, A. J., Feng, W., Xu, Y., et al. (2015). Robust enumeration of cell subsets from tissue expression profiles. Nat. Methods 12, 453–457. doi:10.1038/nmeth.3337
Ng, H. H. M., Lee, R. Y., Goh, S., Tay, I. S. Y., Lim, X., Lee, B., et al. (2020). Immunohistochemical scoring of CD38 in the tumor microenvironment predicts responsiveness to anti-PD-1/PD-L1 immunotherapy in hepatocellular carcinoma. J. Immunother. Cancer 8, e000987. doi:10.1136/jitc-2020-000987
Ni, M., Zhang, J., Sosa, R., Zhang, H., Wang, H., Jin, D., et al. (2021). T-cell immunoglobulin and mucin domain-containing protein-4 is critical for kupffer cell homeostatic function in the activation and resolution of liver ischemia reperfusion injury. Hepatology 74, 2118–2132. doi:10.1002/hep.31906
Ogretmen, B. (2018). Sphingolipid metabolism in cancer signalling and therapy. Nat. Rev. Cancer 18, 33–50. doi:10.1038/nrc.2017.96
Ordonez, R., Fernandez, A., Prieto-Dominguez, N., Martinez, L., Garcia-Ruiz, C., Fernandez-Checa, J. C., et al. (2015). Ceramide metabolism regulates autophagy and apoptotic cell death induced by melatonin in liver cancer cells. J. Pineal Res. 59, 178–189. doi:10.1111/jpi.12249
Pei, S., Zhang, P., Yang, L., Kang, Y., Chen, H., Zhao, S., et al. (2023). Exploring the role of sphingolipid-related genes in clinical outcomes of breast cancer. Front. Immunol. 14, 1116839. doi:10.3389/fimmu.2023.1116839
Qi, D., Song, X., Xue, C., Yao, W., Shen, P., Yu, H., et al. (2021). AKT1/FOXP3 axis-mediated expression of CerS6 promotes p53 mutant pancreatic tumorigenesis. Cancer Lett. 522, 105–118. doi:10.1016/j.canlet.2021.06.024
Qi, R., Bai, Y., Li, K., Liu, N., Xu, Y., Dal, E., et al. (2023). Cancer-associated fibroblasts suppress ferroptosis and induce gemcitabine resistance in pancreatic cancer cells by secreting exosome-derived ACSL4-targeting miRNAs. Drug Resist Updat 68, 100960. doi:10.1016/j.drup.2023.100960
Ren, J., Yuan, Q., Liu, J., Zhong, L., Li, H., Wu, G., et al. (2022). Identifying the role of transient receptor potential channels (TRPs) in kidney renal clear cell carcinoma and their potential therapeutic significances using genomic and transcriptome analyses. BMC Med. Genomics 15, 156. doi:10.1186/s12920-022-01312-x
Ren, Q., Zhang, P., Lin, H., Feng, Y., Chi, H., Zhang, X., et al. (2023). A novel signature predicts prognosis and immunotherapy in lung adenocarcinoma based on cancer-associated fibroblasts. Front. Immunol. 14, 1201573. doi:10.3389/fimmu.2023.1201573
Rojas, L. A., Sethna, Z., Soares, K. C., Olcese, C., Pang, N., Patterson, E., et al. (2023). Personalized RNA neoantigen vaccines stimulate T cells in pancreatic cancer. Nature 618, 144–150. doi:10.1038/s41586-023-06063-y
Sasset, L., and Di Lorenzo, A. (2022). Sphingolipid metabolism and signaling in endothelial cell functions. Adv. Exp. Med. Biol. 1372, 87–117. doi:10.1007/978-981-19-0394-6_8
Shen, Y., Chi, H., Xu, K., Li, Y., Yin, X., Chen, S., et al. (2022). A novel classification model for lower-grade glioma patients based on pyroptosis-related genes. Brain Sci. 12, 700. doi:10.3390/brainsci12060700
Sirinukunwattana, K., Domingo, E., Richman, S. D., Redmond, K. L., Blake, A., Verrill, C., et al. (2021). Image-based consensus molecular subtype (imCMS) classification of colorectal cancer using deep learning. Gut 70, 544–554. doi:10.1136/gutjnl-2019-319866
Snider, J. M., Luberto, C., and Hannun, Y. A. (2019). Approaches for probing and evaluating mammalian sphingolipid metabolism. Anal. Biochem. 575, 70–86. doi:10.1016/j.ab.2019.03.014
Soltani, M., Zhao, Y., Xia, Z., Ganjalikhani Hakemi, M., and Bazhin, A. V. (2021). The importance of cellular metabolic pathways in pathogenesis and selective treatments of hematological malignancies. Front. Oncol. 11, 767026. doi:10.3389/fonc.2021.767026
Song, B., Chi, H., Peng, G., Song, Y., Cui, Z., Zhu, Y., et al. (2022b). Characterization of coagulation-related gene signature to predict prognosis and tumor immune microenvironment in skin cutaneous melanoma. Front. Oncol. 12, 975255. doi:10.3389/fonc.2022.975255
Song, B., Wu, P., Liang, Z., Wang, J., Zheng, Y., Wang, Y., et al. (2022a). A novel necroptosis-related gene signature in skin cutaneous melanoma prognosis and tumor microenvironment. Front. Genet. 13, 917007. doi:10.3389/fgene.2022.917007
Song, J., Cui, D., Wang, J., Qin, J., Wang, S., Wang, Z., et al. (2021). Overexpression of HMGA1 confers radioresistance by transactivating RAD51 in cholangiocarcinoma. Cell Death Discov. 7, 322. doi:10.1038/s41420-021-00721-8
Sousa, N., Geiss, C., Bindila, L., Lieberwirth, I., Kim, E., and Regnier-Vigouroux, A. (2023). Targeting sphingolipid metabolism with the sphingosine kinase inhibitor SKI-II overcomes hypoxia-induced chemotherapy resistance in glioblastoma cells: effects on cell death, self-renewal, and invasion. BMC Cancer 23, 762. doi:10.1186/s12885-023-11271-w
Su, K., Guo, L., Ma, W., Wang, J., Xie, Y., Rao, M., et al. (2022). PD-1 inhibitors plus anti-angiogenic therapy with or without intensity-modulated radiotherapy for advanced hepatocellular carcinoma: a propensity score matching study. Front. Immunol. 13, 972503. doi:10.3389/fimmu.2022.972503
Su, L., Chen, Y., Huang, C., Wu, S., Wang, X., Zhao, X., et al. (2023). Targeting Src reactivates pyroptosis to reverse chemoresistance in lung and pancreatic cancer models. Sci. Transl. Med. 15, eabl7895. doi:10.1126/scitranslmed.abl7895
Thayyullathil, F., Cheratta, A. R., Alakkal, A., Subburayan, K., Pallichankandy, S., Hannun, Y. A., et al. (2021). Acid sphingomyelinase-dependent autophagic degradation of GPX4 is critical for the execution of ferroptosis. Cell Death Dis. 12, 26. doi:10.1038/s41419-020-03297-w
Vahabi, M., Dehni, B., Antomas, I., Giovannetti, E., and Peters, G. J. (2023). Targeting miRNA and using miRNA as potential therapeutic options to bypass resistance in pancreatic ductal adenocarcinoma. Cancer Metastasis Rev. doi:10.1007/s10555-023-10127-w
Wang, J., Li, R. Z., Wang, W. J., Pan, H. D., Xie, C., Yau, L. F., et al. (2023b). CERS4 predicts positive anti-PD-1 response and promotes immunomodulation through Rhob-mediated suppression of CD8(+)Tim3(+) exhausted T cells in non-small cell lung cancer. Pharmacol. Res. 194, 106850. doi:10.1016/j.phrs.2023.106850
Wang, J., Yuan, Y., Tang, L., Zhai, H., Zhang, D., Duan, L., et al. (2022b). Long non-coding RNA-TMPO-AS1 as ceRNA binding to let-7c-5p upregulates STRIP2 expression and predicts poor prognosis in lung adenocarcinoma. Front. Oncol. 12, 921200. doi:10.3389/fonc.2022.921200
Wang, X., Zhao, Y., Strohmer, D. F., Yang, W., Xia, Z., and Yu, C. (2022a). The prognostic value of MicroRNAs associated with fatty acid metabolism in head and neck squamous cell carcinoma. Front. Genet. 13, 983672. doi:10.3389/fgene.2022.983672
Wang, Z., Yuan, Q., Chen, X., Luo, F., Shi, X., Guo, F., et al. (2023a). A prospective prognostic signature for pancreatic adenocarcinoma based on ubiquitination-related mRNA-lncRNA with experimental validation in vitro and vivo. Funct. Integr. Genomics 23, 263. doi:10.1007/s10142-023-01158-1
Wen, C., Zhang, L., Yang, Y., Jin, Y., Ren, D., Zhang, Z., et al. (2023). Specific human leukocyte antigen class I genotypes predict prognosis in resected pancreatic adenocarcinoma: a retrospective cohort study. Int. J. Surg. 109, 1941–1952. doi:10.1097/JS9.0000000000000264
Wilson, G. C., Patel, S. H., Wang, J., Xu, K., Turner, K. M., Becker, K. A., et al. (2023). Acid sphingomyelinase expression is associated with survival in resectable pancreatic ductal adenocarcinoma. J. Mol. Med. Berl. 101, 891–903. doi:10.1007/s00109-023-02331-6
Wu, X., Lu, W., Xu, C., Jiang, C., Zhuo, Z., Wang, R., et al. (2023). Macrophages phenotype regulated by IL-6 are associated with the prognosis of platinum-resistant serous ovarian cancer: integrated analysis of clinical trial and omics. J. Immunol. Res. 2023, 6455704. doi:10.1155/2023/6455704
Xia, Z., Chen, S., He, M., Li, B., Deng, Y., Yi, L., et al. (2023). Editorial: targeting metabolism to activate T cells and enhance the efficacy of checkpoint blockade immunotherapy in solid tumors. Front. Immunol. 14, 1247178. doi:10.3389/fimmu.2023.1247178
Xiang, X., Wang, J., Lu, D., and Xu, X. (2021). Targeting tumor-associated macrophages to synergize tumor immunotherapy. Signal Transduct. Target Ther. 6, 75. doi:10.1038/s41392-021-00484-9
Xiao, J., Huang, K., Lin, H., Xia, Z., Zhang, J., Li, D., et al. (2020). Mogroside II(E) inhibits digestive enzymes via suppression of interleukin 9/interleukin 9 receptor signalling in acute pancreatitis. Front. Pharmacol. 11, 859. doi:10.3389/fphar.2020.00859
Xiao, J., Lin, H., Liu, B., Xia, Z., Zhang, J., and Jin, J. (2019). Decreased S1P and SPHK2 are involved in pancreatic acinar cell injury. Biomark. Med. 13, 627–637. doi:10.2217/bmm-2018-0404
Xiong, J., Chi, H., Yang, G., Zhao, S., Zhang, J., Tran, L. J., et al. (2023). Revolutionizing anti-tumor therapy: unleashing the potential of B cell-derived exosomes. Front. Immunol. 14, 1188760. doi:10.3389/fimmu.2023.1188760
Yan, S., Jin, W., Ding, J., Yin, T., Zhang, Y., and Yang, J. (2021). Machine-intelligence for developing a potent signature to predict ovarian response to tailor assisted reproduction technology. Aging (Albany NY) 13, 17137–17154. doi:10.18632/aging.203032
Yu, L., He, R., and Cui, Y. (2023). Characterization of tumor microenvironment and programmed death-related genes to identify molecular subtypes and drug resistance in pancreatic cancer. Front. Pharmacol. 14, 1146280. doi:10.3389/fphar.2023.1146280
Yuan, Q., Deng, D., Pan, C., Ren, J., Wei, T., Wu, Z., et al. (2022b). Integration of transcriptomics, proteomics, and metabolomics data to reveal HER2-associated metabolic heterogeneity in gastric cancer with response to immunotherapy and neoadjuvant chemotherapy. Front. Immunol. 13, 951137. doi:10.3389/fimmu.2022.951137
Yuan, Q., Ren, J., Chen, X., Dong, Y., and Shang, D. (2022c). Contributions and prognostic performances of m7G RNA regulators in pancreatic adenocarcinoma. Chin. Med. J. Engl. 135, 2101–2103. doi:10.1097/CM9.0000000000002179
Yuan, Q., Zhang, W., and Shang, W. (2022a). Identification and validation of a prognostic risk-scoring model based on sphingolipid metabolism-associated cluster in colon adenocarcinoma. Front. Endocrinol. (Lausanne) 13, 1045167. doi:10.3389/fendo.2022.1045167
Zhai, X., Wang, W., Ma, Y., Zeng, Y., Dou, D., Fan, H., et al. (2020). Serum KIAA1199 is an advanced-stage prognostic biomarker and metastatic oncogene in cholangiocarcinoma. Aging (Albany NY) 12, 23761–23777. doi:10.18632/aging.103964
Zhai, X., Xia, Z., Du, G., Zhang, X., Xia, T., Ma, D., et al. (2023). LRP1B suppresses HCC progression through the NCSTN/PI3K/AKT signaling axis and affects doxorubicin resistance. Genes Dis. 10, 2082–2096. doi:10.1016/j.gendis.2022.10.021
Zhang, B., Huang, B., Zhang, X., Li, S., Zhu, J., Chen, X., et al. (2023i). PANoptosis-related molecular subtype and prognostic model associated with the immune microenvironment and individualized therapy in pancreatic cancer. Front. Oncol. 13, 1217654. doi:10.3389/fonc.2023.1217654
Zhang, B., Sun, J., Guan, H., Guo, H., Huang, B., Chen, X., et al. (2023a). Integrated single-cell and bulk RNA sequencing revealed the molecular characteristics and prognostic roles of neutrophils in pancreatic cancer. Aging (Albany NY) 15, 9718–9742. doi:10.18632/aging.205044
Zhang, B., Yuan, Q., Zhang, B., Li, S., Wang, Z., Liu, H., et al. (2022a). Characterization of neuroendocrine regulation- and metabolism-associated molecular features and prognostic indicators with aid to clinical chemotherapy and immunotherapy of patients with pancreatic cancer. Front. Endocrinol. (Lausanne) 13, 1078424. doi:10.3389/fendo.2022.1078424
Zhang, H., Ni, M., Wang, H., Zhang, J., Jin, D., Busuttil, R. W., et al. (2023g). Gsk3β regulates the resolution of liver ischemia/reperfusion injury via MerTK. JCI Insight 8, e151819. doi:10.1172/jci.insight.151819
Zhang, H., Zhai, X., Liu, Y., Xia, Z., Xia, T., Du, G., et al. (2023h). NOP2-mediated m5C modification of c-myc in an eif3a-dependent manner to reprogram glucose metabolism and promote hepatocellular carcinoma progression. Res. (Wash D C) 6, 0184. doi:10.34133/research.0184
Zhang, J., Peng, G., Chi, H., Yang, J., Xie, X., Song, G., et al. (2023j). CD8 + T-cell marker genes reveal different immune subtypes of oral lichen planus by integrating single-cell RNA-seq and bulk RNA-sequencing. BMC Oral Health 23, 464. doi:10.1186/s12903-023-03138-0
Zhang, L., Chen, C., Chai, D., Li, C., Guan, Y., Liu, L., et al. (2022b). The association between antibiotic use and outcomes of HCC patients treated with immune checkpoint inhibitors. Front. Immunol. 13, 956533. doi:10.3389/fimmu.2022.956533
Zhang, L., Kuang, T., Chai, D., Deng, W., Wang, P., and Wang, W. (2023c). The use of antibiotics during immune checkpoint inhibitor treatment is associated with lower survival in advanced esophagogastric cancer. Int. Immunopharmacol. 119, 110200. doi:10.1016/j.intimp.2023.110200
Zhang, P., Liu, J., Pei, S., Wu, D., Xie, J., Liu, J., et al. (2023b). Mast cell marker gene signature: prognosis and immunotherapy response prediction in lung adenocarcinoma through integrated scRNA-seq and bulk RNA-seq. Front. Immunol. 14, 1189520. doi:10.3389/fimmu.2023.1189520
Zhang, P., Pei, S., Gong, Z., Feng, Y., Zhang, X., Yang, F., et al. (2023k). By integrating single-cell RNA-seq and bulk RNA-seq in sphingolipid metabolism, CACYBP was identified as a potential therapeutic target in lung adenocarcinoma. Front. Immunol. 14, 1115272. doi:10.3389/fimmu.2023.1115272
Zhang, P., Pei, S., Liu, J., Zhang, X., Feng, Y., Gong, Z., et al. (2022c). Cuproptosis-related lncRNA signatures: predicting prognosis and evaluating the tumor immune microenvironment in lung adenocarcinoma. Front. Oncol. 12, 1088931. doi:10.3389/fonc.2022.1088931
Zhang, P., Pei, S., Wu, L., Xia, Z., Wang, Q., Huang, X., et al. (2023f). Integrating multiple machine learning methods to construct glutamine metabolism-related signatures in lung adenocarcinoma. Front. Endocrinol. (Lausanne) 14, 1196372. doi:10.3389/fendo.2023.1196372
Zhang, X., Zhuge, J., Liu, J., Xia, Z., Wang, H., Gao, Q., et al. (2023d). Prognostic signatures of sphingolipids: understanding the immune landscape and predictive role in immunotherapy response and outcomes of hepatocellular carcinoma. Front. Immunol. 14, 1153423. doi:10.3389/fimmu.2023.1153423
Zhang, Y., Jin, W., Chen, J., Wei, S., Cai, W., Zhong, Y., et al. (2023e). Gastrodin alleviates rat chondrocyte senescence and mitochondrial dysfunction through Sirt3. Int. Immunopharmacol. 118, 110022. doi:10.1016/j.intimp.2023.110022
Zhao, S., Chi, H., Yang, Q., Chen, S., Wu, C., Lai, G., et al. (2023b). Identification and validation of neurotrophic factor-related gene signatures in glioblastoma and Parkinson's disease. Front. Immunol. 14, 1090040. doi:10.3389/fimmu.2023.1090040
Zhao, S., Zhang, L., Ji, W., Shi, Y., Lai, G., Chi, H., et al. (2022a). Machine learning-based characterization of cuprotosis-related biomarkers and immune infiltration in Parkinson's disease. Front. Genet. 13, 1010361. doi:10.3389/fgene.2022.1010361
Zhao, S., Zhang, X., Gao, F., Chi, H., Zhang, J., Xia, Z., et al. (2023a). Identification of copper metabolism-related subtypes and establishment of the prognostic model in ovarian cancer. Front. Endocrinol. (Lausanne) 14, 1145797. doi:10.3389/fendo.2023.1145797
Zhao, Y., Wei, K., Chi, H., Xia, Z., and Li, X. (2022b). IL-7: a promising adjuvant ensuring effective T cell responses and memory in combination with cancer vaccines? Front. Immunol. 13, 1022808. doi:10.3389/fimmu.2022.1022808
Keywords: pancreatic carcinoma, machine learning, sphingolipid, immunotherapy response, prediction
Citation: Shi T, Li M and Yu Y (2023) Machine learning-enhanced insights into sphingolipid-based prognostication: revealing the immunological landscape and predictive proficiency for immunomotherapy and chemotherapy responses in pancreatic carcinoma. Front. Mol. Biosci. 10:1284623. doi: 10.3389/fmolb.2023.1284623
Received: 28 August 2023; Accepted: 17 October 2023;
Published: 31 October 2023.
Edited by:
Bin Jin, Shandong University, ChinaReviewed by:
Chen Li, Free University of Berlin, GermanyCopyright © 2023 Shi, Li and Yu. This is an open-access article distributed under the terms of the Creative Commons Attribution License (CC BY). The use, distribution or reproduction in other forums is permitted, provided the original author(s) and the copyright owner(s) are credited and that the original publication in this journal is cited, in accordance with accepted academic practice. No use, distribution or reproduction is permitted which does not comply with these terms.
*Correspondence: Yabin Yu, eXV5YWJpbmJpbkAxMjYuY29t
†These authors have contributed equally to this work
Disclaimer: All claims expressed in this article are solely those of the authors and do not necessarily represent those of their affiliated organizations, or those of the publisher, the editors and the reviewers. Any product that may be evaluated in this article or claim that may be made by its manufacturer is not guaranteed or endorsed by the publisher.
Research integrity at Frontiers
Learn more about the work of our research integrity team to safeguard the quality of each article we publish.