- 1Department of Dermatology and Venereology, The First Affiliated Hospital of Guangxi Medical University, Nanning, China
- 2Guangxi Key Laboratory of Major Infectious Disease Prevention and Control and Biosafety Emergency Response, Guangxi Centre for Disease Control and Prevention, Nanning, China
Objective: The observational association between circulating metabolites and systemic lupus erythematosus (SLE) has been well documented. However, whether the association is causal remains unclear. In this study, bidirectional Mendelian randomization (MR) was introduced to analyse the causal relationships and possible mechanisms.
Methods: We conducted a two-sample bidirectional MR study. A genome-wide association study (GWAS) with 7,824 participants provided data on 486 human blood metabolites. Outcome information was obtained from a large-scale GWAS summary, which contained 5,201 single nucleotide polymorphisms (SNPs) cases and 9,066 control cases of Europeans and yielded a total of 7,071,163 SNPs. The inverse variance weighted (IVW) model was recruited as the primary two-sample MR analysis approach, followed by sensitivity analyses such as the heterogeneity test, horizontal pleiotropy test, leave-one-out analysis, and linkage disequilibrium score (LDSC) regression.
Results: In this study, we discovered that 24 metabolites belonging to the lipid, carbohydrate, xenobiotic and amino acid superpathways may increase the risk of SLE occurrence (p < 0.05). In addition, the metabolic disorders of 51 metabolites belonging to the amino acid, energy, xenobiotics, peptide and lipid superpathways were affected by SLE (p < 0.05). Palmitoleate belonging to the lipid superpathway and isobutyrylcarnitine and phenol sulfate belonging to the amino acid superpathway were factors with two-way causation. The metabolic enrichment pathway of bile acid biosynthesis was significant in the forward MR analysis (p = 0.0435). Linolenic acid and linoleic acid metabolism (p = 0.0260), betaine metabolism (p = 0.0314), and glycerolipid metabolism (p = 0.0435) were the significant metabolically enriched pathways in the reverse MR analysis.
Conclusion: The levels of some specific metabolites may either contribute to the immune response inducing SLE, or they may be intermediates in the development and progression of SLE. These metabolites can be used as auxiliary diagnostic tools for SLE and for the evaluation of disease progression and therapeutic effects.
1 Introduction
The prevalence of systemic lupus erythematosus (SLE), a complex autoimmune multisystemic disease of great clinical heterogeneity, exhibits a wide range with rates varying between 29 and 7,713 per 100,000 individuals (Barber et al., 2021). The heterogeneity of SLE is not only different in patients with different individuals, different genders, different ages and different course of disease, but also in organ damage and corresponding manifestations, slow onset, overlapping damage, response to treatment, outcome and prognosis, even in identical twins (Bhaskar and Nagaraju, 2019; Wu et al., 2020). As autoimmunity starts before its clinical manifestations, the long time period between the onset of autoimmunity and final diagnosis means that some patients with SLE may develop irreversible, severe organ damage and even death (Nashi and Shmerling, 2021). Early diagnosis and treatment have become an urgent issue in SLE management (Ugarte-Gil et al., 2019). Because of the clinical complexity of lupus, identifying sensitive and specific biomarkers for its diagnosis and monitoring has been challenging. Therefore, the discovery of specific biomarkers is of great significance for the prognosis of SLE.
In the past decade, an increasing number of studies have found an association between metabolomics and SLE and other immune diseases, which provides new ideas for metabolic-derived biomarkers. Metabolomics is the study of small-molecule metabolites in samples or organs of living organisms. Cellular metabolic programs could affect the immune response by regulating the activation, proliferation, and differentiation of innate and adaptive immune cells. Many studies have shown that the dysregulation of the immune system is associated with changes in metabolite profiles (Teng et al., 2020). Metabolites can regulate the process of immune diseases by targeting the corresponding receptors (Wu et al., 2023a).
Metabolism is known for its intricate complexity at the biochemical level. Recent studies have added levels of complexity showing “moonlighting” functions of some metabolic enzymes, such as glyceraldehyde-3-phosphate dehydrogenase (GAPDH) regulating Interferon γ (IFN-γ) production, and metabolites, such as succinate or itaconate, with previously unsuspected immune signalling functions (Chang et al., 2013; Murphy and O'Neill, 2018). Therefore, screening out certain unreported metabolites associated with SLE by Mendelian randomization (MR) analysis has enlightening implications for exploring the mechanisms of pathophysiology.
While metabolic abnormalities affect the development of SLE, the disease also increases the metabolic burden. In an observational study (Ouyang et al., 2011), the levels of serum amino acids in SLE patients were decreased, including some glycolytic and ketogenic amino acids and citric acid, an intermediate metabolite of the tricarboxylic acid cycle, and the decrease in pyruvate, an intermediate product of glycolysis, indicates that SLE patients have energy metabolism disorders. This may be related to the enhanced protein catabolism and increased energy requirements that are present in a state of systemic inflammation.
Notably, cohort-based causal studies between metabolites and SLE are lacking. If differentially abundant metabolites are risk factors or protective factors for SLE, it is meaningful for the prediction of the disease and auxiliary diagnosis based on specific targets. MR analysis uses randomly occurring single nucleotide polymorphisms (SNPs) in human genes as mediation tools. This approach, which is similar to the randomized controlled trial (RCT) design, better ensures the randomization of the sampling. The establishment of exposure factors and outcome variables through instrumental variables can also better prove the causal relationship between them. At the same time, because metabolites may be substances that affect the occurrence of diseases, or may be substances produced after the occurrence of diseases, bidirectional MR analysis can better explain the causal direction of metabolites and diseases.
Therefore, this study collected relatively complete serum metabolome data and introduced MR analysis similar to the RCT design to interpret the causal relationship between SLE and related metabolites through bidirectional MR verification. In this research, MR methodology was used to evaluate the causal effects of genetically proxied metabolomics elements of interest on SLE by selecting metabolite-associated SNPs as instrumental variables (IVs) and to assess pooled metabolic pathways to explain the mechanism.
2 Materials and methods
2.1 Study design
The dataset that contains all the data in this study is available to the public on the database website. The genome-wide association study (GWAS) summary statistics that have already been published. The ethics committee at each institutional review board authorized all participants’ written informed permission in separate studies. No extra ethical approval or informed consent was required.
In the current study, we comprehensively evaluated the relationship between 486 serum metabolites and SLE based on a rigorous MR design. A scientific MR study must include the testing of the following three hypotheses: 1) genetic instrumental variables are strongly associated with the exposure of interest; 2) genetic instrumental variables should be irrelevant to the outcome and independent of any known or unknown confounding factors; and 3) the effect of instrumental variables on the results is mediated only by the interest exposure. Briefly, a bidirectional analysis strategy was utilized to select genetically significant SNPs for 486 human serum metabolites and SLE from a European population. To avoid sample overlap, the metabolites and SLE genetic information selected in this study were obtained from separate GWAS datasets. A schematic of this bidirectional MR study is shown in Figure 1.
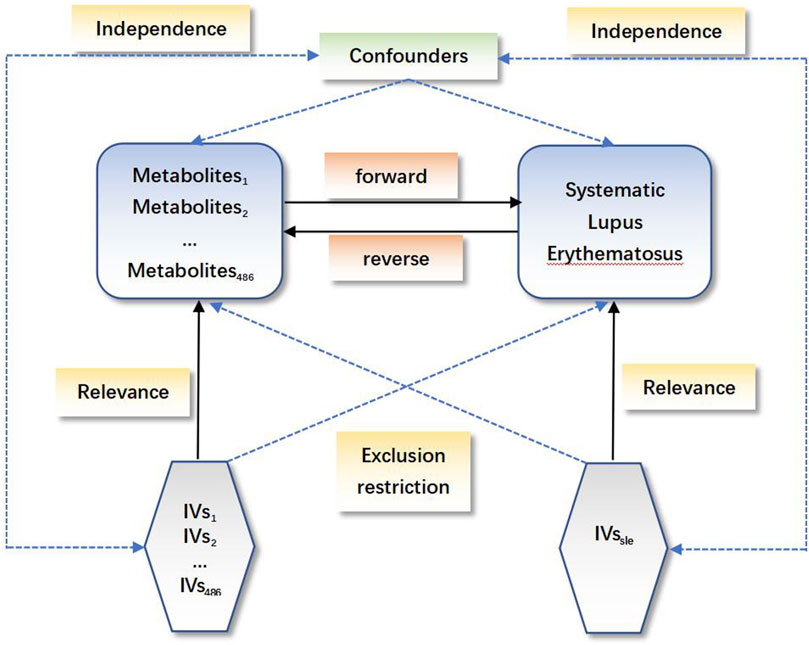
FIGURE 1. Schematic of the bidirectional Mendelian randomization (MR) analysis. Significant instrumental variables were selected for 486 differentially abundant metabolites and systemic lupus erythematosus, and the bidirectional causalities were probed. The three basic assumptions of MR analysis are illustrated in the acyclic graph.
2.2 GWAS data for human serum metabolites
A genome-wide association aggregate dataset of 486 serum metabolites involved in this study was obtained by Shin et al. (2014). These data are publicly available from the GWAS server (http://metabolomics.helmholtz-muenchen.de/gwas/). The service platform collects relatively complete human serum metabolomics data. A total of 7,824 adults and approximately 2.1 million SNPs from two European cohorts (TwinsUK and KORA cohorts) were included in the GWAS analysis. Of the 486 metabolites, 309 are named metabolites that can be assigned to eight broad metabolic groups (amino acids, carbohydrates, cofactors and vitamins, energy, lipids, nucleotides, peptides, and xenobiotic metabolism), as defined by the kyoto encyclopedia of genes and genomes (KEGG) database (Kanehisa et al., 2012). The chemical properties of another 177 unknown metabolites have not been fully determined.
2.3 GWAS data for SLE
The summary data of SLE were obtained from the (https://gwas.mrcieu.ac.uk/) Integrative Epidemiology Unit (IEU) open GWAS project. The GWAS ID is ebi-a-GCST003156 (Bentham et al., 2015). In this GWAS meta-analysis, the summary data included 5,201 SLE cases and 9,066 control cases, yielding a total of 7,071,163 SNPs. We extracted SNPs by analysing vcf format files shared by this platform. The SLE patients from the European population were diagnosed according to the standard American College of Rheumatology classification criteria.
2.4 Selection of instrumental variables (IVs)
The selection of IVs in this MR analysis was based on 3 fundamental assumptions. First, for each metabolite, we set p < 1 × 10−5 as the genome-wide significance threshold to select strongly associated SNPs. Second, a clumping procedure implemented in R software was employed to identify the independent variants. R2 < 0.001 within a 500-kilobase (kb) distance was used for linkage-disequilibrium. Finally, to quantitatively verify whether the selected SNPs are strong instruments, we calculated the proportion of phenotypic variation explained (PVE) for each metabolite and the F statistic. Typically, a threshold of F > 10 is suggested for the following analysis (Burgess et al., 2013).
2.5 MR analysis
A standard inverse variance weighted (IVW) method was the main evaluation approach used for causal association exploration between metabolites and SLE (forward MR analysis and reverse MR analysis) in this analysis. MR-Egger and weighted median (WM) were secondary evaluation method. When the instrumental variables satisfy all three major hypotheses, the IVW method can provide a more accurate estimate of the causal effect of exposure and is considered the most efficient MR method. Nevertheless, if some IVs do not conform to the IV hypothesis, the analysis may give inaccurate results. Thus, we implemented the following sensitivity analyses: 1) the Q test was carried out with the IVW and MR‒Egger methods to detect possible violations of the hypothesis by the heterogeneity of the correlation between individual IVs (Cohen et al., 2015); 2) the MR‒Egger intercept was implemented to estimate the horizontal pleiotropy, ensuring that the genetic variation was independently related to the metabolite and SLE (Burgess and Thompson, 2017); 3) additional analyses such as the weighted median and weighted mode were applied to enhance the reliability and stability of hypothesis testing; and 4) we conducted an individual SNP analysis and leave-one-out test to evaluate the likelihood of relevance observed by individual SNPs.
2.6 Genetic correlation and direction validation
MR analysis may violate cause-effects under the premise of genetic correlation between the exposure and outcome of the research (O'Connor and Price, 2018; Reay et al., 2022). Although SNPs related to SLE were excluded in the selection of IVs, SNPs with no relevance may also impact the occurrence of SLE. Linkage disequilibrium score (LDSC) regression can compute the coinheritance by calling chi-squared statistics based on SNPs (Bulik-Sullivan et al., 2015). Therefore, to ensure that cause-effects were not confused by the coheritability of exposure with the outcome, LDSC was implemented to verify the genetic correlation between the differentially abundant serum metabolites and SLE.
2.7 Metabolic pathway analysis
Metabolic pathways were estimated using Web-based metconflict 5.0 (https://www.Metaboanalyst.ca/) (Chong and Xia, 2104). The pathway and enrichment analysis modules were applied to identify probable metabolite clusters or superpathways that may be associated with metabolic processes and the potential association with SLE. The small molecule pathway database (SMPDB) and the KEGG database were applied for reference. The significance level of the pathway was 0.05.
2.8 Intersection analysis
An intersection analysis was introduced to analyse the shared metabolites screened by the forward and reverse MR analyses and, in conjunction with potential pathway mechanisms, to evaluate the relationship between metabolic pathways and circulatory deterioration in SLE.
2.9 Statistical analysis
All MR analyses were performed using the “TwoSampleMR” package in R (version 4.3.0). LDSC was conducted by the “ldscr” package, and p < 0.05 was considered statistically significant. The odds ratio (OR) was used to estimate the magnitude and direction of the metabolic impact with its corresponding 95% confidence interval (CI).
3 Results
3.1 Influence of 486 serum metabolites on SLE (forward MR)
As the genome-wide significance threshold was p < 1 × 10−5 to select strongly associated SNPs, a total of 483 serum metabolites were selected. The IVs contained 7,881 SNPs in total, with a median of 12 SNPs. The F statistic values were all greater than 10, indicating that weak instrumental bias is unlikely to be significant. Except for 176 unnamed metabolites, the remaining 307 metabolites belong to 8 superpathways. The highest proportion of metabolites was lipids (122, 39.74%), followed by amino acids (74, 24.10%) and xenobiotics (39, 12.70%).
All metabolic analyses used IVW as the primary analytical methodology, with no evidence of heterogeneity and no weak instruments (Jin et al., 2020). From the primary results, 24 significantly associated metabolites were selected (p < 0.05 for IVW), in which 17 were positively associated with SLE and 7 were negatively associated with SLE. 2-Methoxyacetaminophen sulfate (p = 8.55 × 10−5) was the most significant metabolite, followed by palmitoleate (16:1n7) (p = 5.11 × 10−4) and ursodeoxycholate (p = 3.53 × 10−3) (Figure 2). Ten out of the 24 metabolites were unnamed. Among the 14 identified metabolites, 6 metabolites belonging to lipid pathways and 1,5-anhydroglucitol (1,5-AG) belonging to carbohydrate pathways were positively associated with SLE. 2-Methoxyacetaminophen sulfate, belonging to the xenobiotic pathway, exhibits serum protection. In addition to citrulline’s protective effect across the amino acid pathway, its other metabolic involvement may also be a risk factor for SLE (Figure 3). The results of the alternative MR analysis, Q test and sensitivity analysis for the 14 known metabolites are shown in Table 1. All IVs passed the sensitivity tests (p > 0.05).
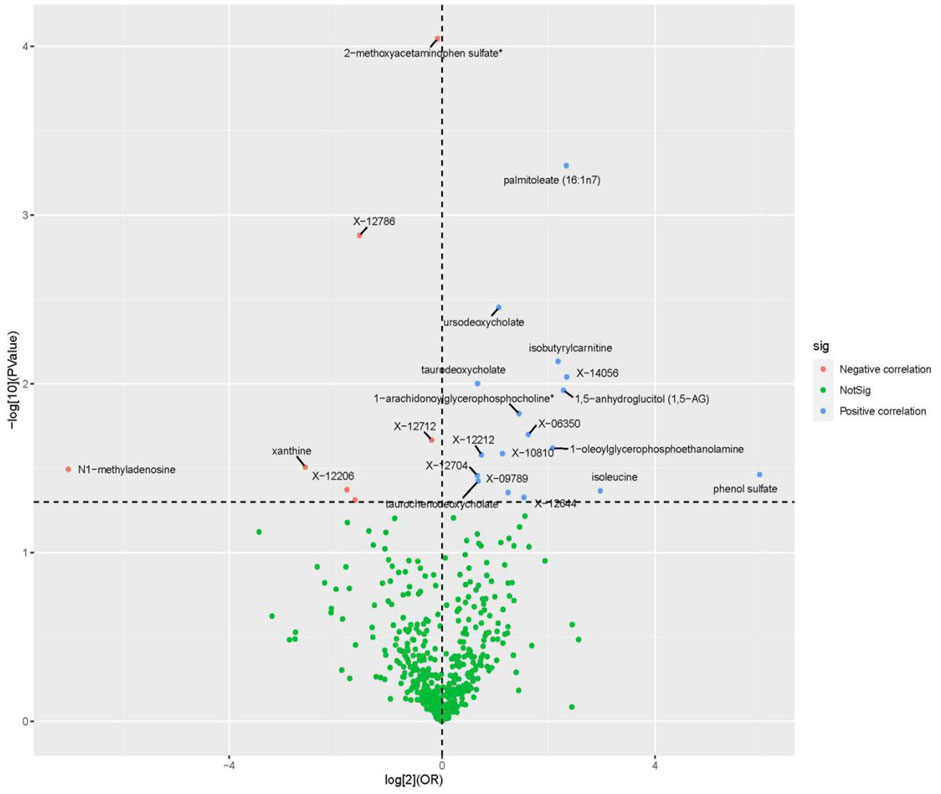
FIGURE 2. Volcano plot of correlations related to the influence of metabolites on SLE. This plot includes both odds ratios (ORs) in log 2 scale and p-values in -log 10 estimated by the inverse variance weighted method for SLE.
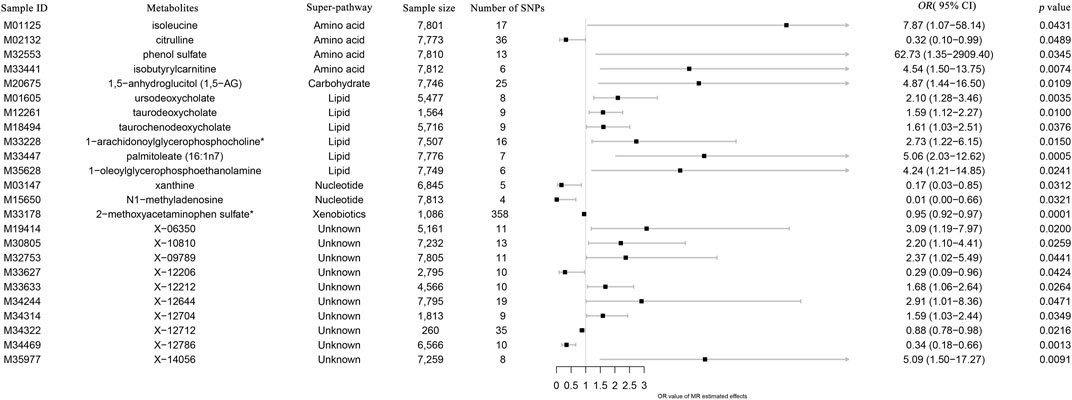
FIGURE 3. Forest plot of the causal effects of metabolites on the risk of SLE derived from the IVW method. OR, odds ratio; CI, confidence interval.
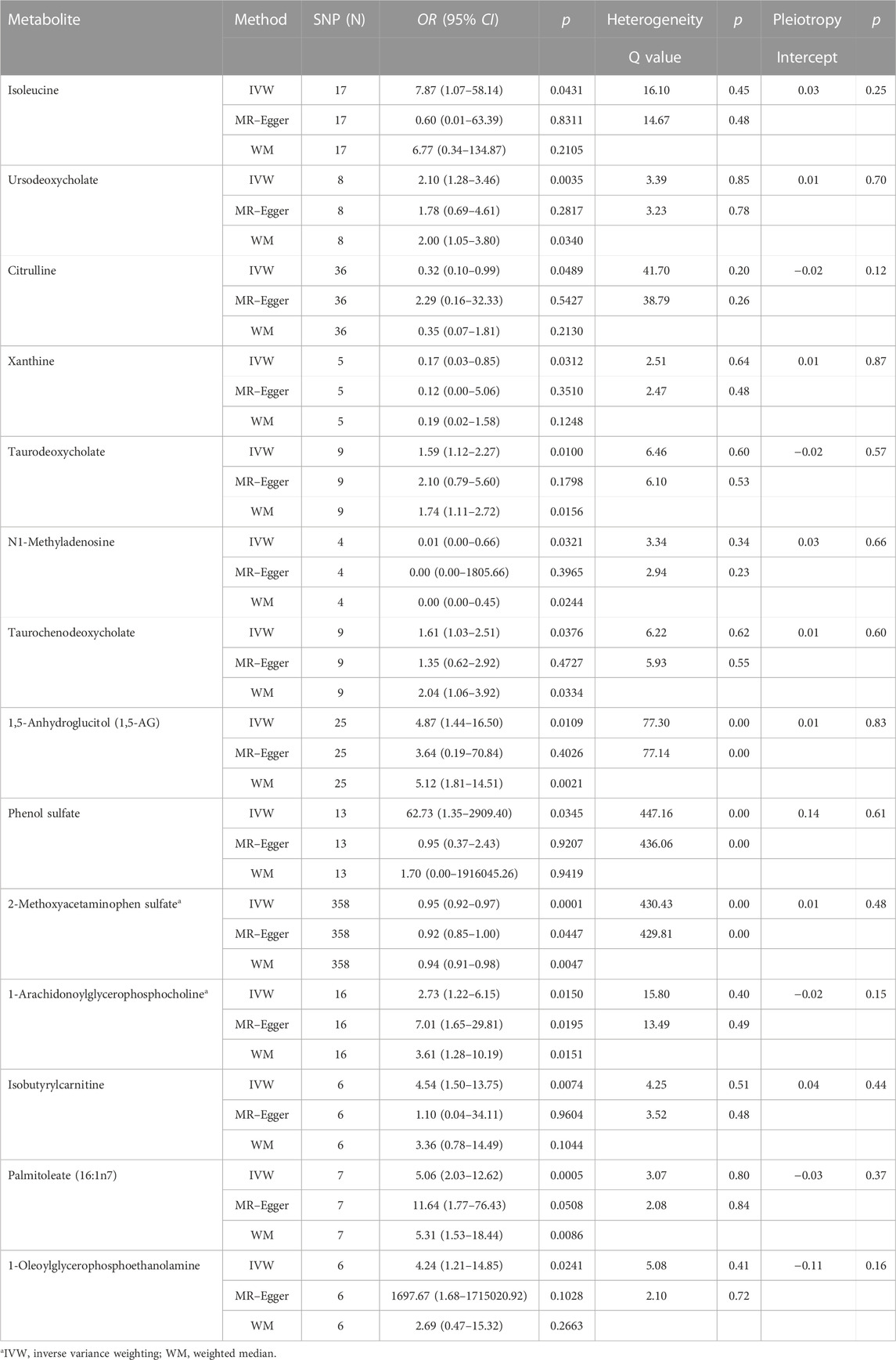
TABLE 1. The three MR model estimates of the causal relationships between 14 known metabolites and the risk of SLE and tests for heterogeneity and horizontal pleiotropy.
The results of LDSC analysis show weak evidence of a genetic correlation between SLE and ursodeoxycholate (rg = 0.0395, se = 0.2454, p = 0.8722), taurodeoxycholate (rg = −0.1601, se = 0.1715, p = 0.3503), N1-methyladenosine (rg = −0.0427, se = 0.362, p = 0.7538), 1,5-anhydroglucitol (1,5-AG) (rg = −0.0395, se = 0.0840, p = 0.6383), 1-arachidonoylglycerophosphocholine (rg = −0.0320, se = 0.1026, p = 0.7554), isobutyrylcarnitine (rg = 0.1165, se = 0.1219, p = 0.3392), palmitoleate (16:1n7) (rg = 0.2461, se = 0.1551, p = 0.1124), and 1-oleoylglycerophosphoethanolamine (rg = 0.1058, se = 0.2077, p = 0.6105), suggesting that the shared genetic component did not confound the MR estimates (Supplementary Table S1).
Fourteen metabolites significantly associated with SLE were entered into the Metabolic Analyzer 5.0 platform to determine various potential metabolic pathways involved in the pathogenesis of SLE. Among them, taurodeoxycholate and taurochenodeoxycholate were involved in the metabolic enrichment pathway of bile acid biosynthesis (p = 0.035) (Table 2). The metabolic mechanism formed by the above metabolites may be involved in the pathogenesis of SLE. Figure 4 exhibits the network of interactions among the metabolic pathways involved in this study.
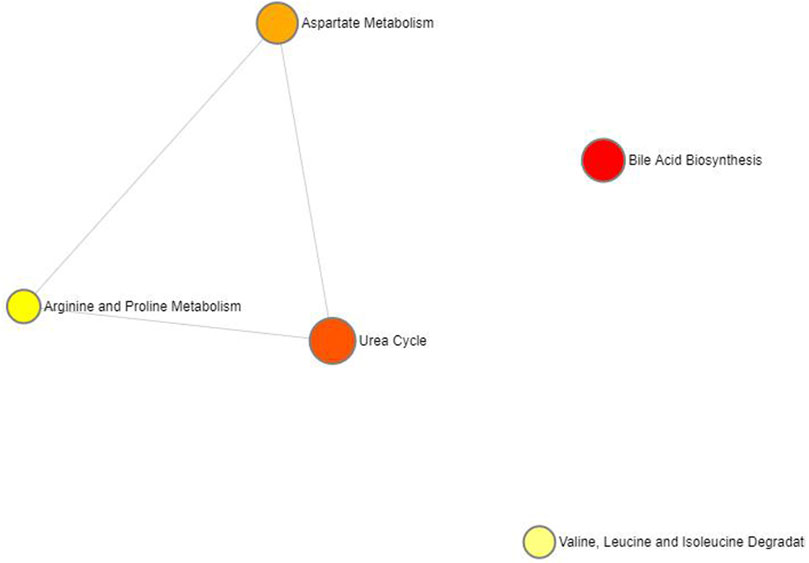
FIGURE 4. Network of enrichment pathways of the metabolites selected by forward MR. The colour ranges from light yellow to dark red, indicating the level of enrichment significance, and the size of the circle reflects the level of the enrichment ratio.
3.2 Influence of the 486 serum metabolites on SLE (reverse MR)
As the genome-wide significance threshold was p < 1 × 10−5, 21 significant SNPs were extracted as the IVs for SLE. The 486 serum metabolites were viewed as the outcomes. Of the 486 metabolites, 21 SNPs met the harmonization criteria. In addition, the F statistic values were all greater than 10, indicating that the weak instrumental bias is unlikely to be significant.
As no heterogeneity or weak instruments were observed, the IVW method was used as the primary estimation method for SLE casualties. From the primary results, 51 significantly associated metabolites were selected (p < 0.05 for IVW), among which 17 were positively associated with SLE, and 34 were negatively associated with SLE. 2-Methoxyacetaminophen sulfate (p = 1.55 × 10−4) was the most significant metabolite, followed by gamma-glutamyltyrosine (p = 3.12 × 10−4) and pentadecanoate (15:0) (p = 8.12 × 10−4) (Figure 5). Seventeen out of the 51 metabolites were unnamed. Among the 34 identified metabolites, 9 metabolites belonging to the amino acid pathway, phosphate belonging to the energy pathway and 2-hydroxyhippurate (salicylurate) belonging to the xenobiotic pathway were positively associated with SLE. Gamma-glutamyltyrosine and cyclo(leu-pro), belonging to the peptide pathway, exhibit serum protection. Twelve out of 21 metabolites across the lipid pathway have a protective effect, and the other 9 metabolites may also be risk factors for SLE (Figure 6). The results of the alternative MR analysis, Q test and sensitivity analysis for 14 known metabolites are shown in Table 3. Due to the influence of the number of IVs and the small confidence interval of the OR value, the p-values of the sensitivity analysis of lysine, 15-methylpalmitate (isobar with 2-methylpalmitate), glycerol and myristate (14:0) are less than 0.05, and a random effect model should be used.
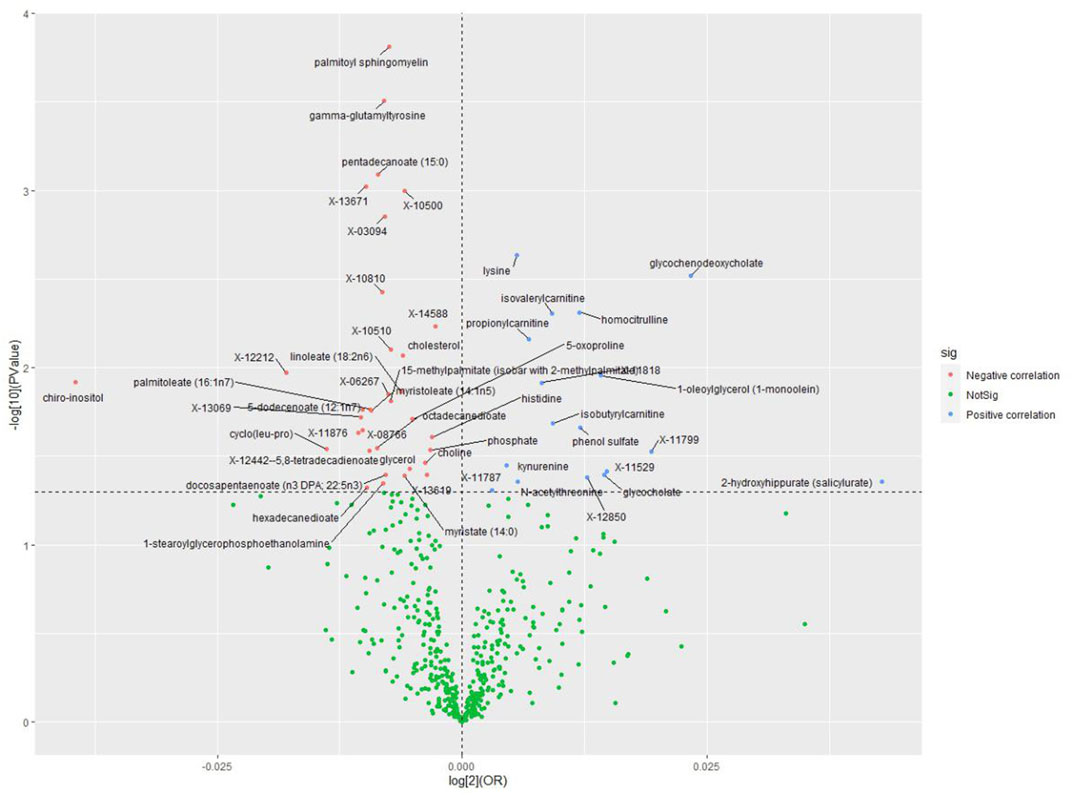
FIGURE 5. Volcano plot of correlations related to the influence of SLE on metabolites. This plot includes both odds ratios (ORs) in log 2 scale and p-values in -log 10 estimated by the inverse variance weighted method for SLE.
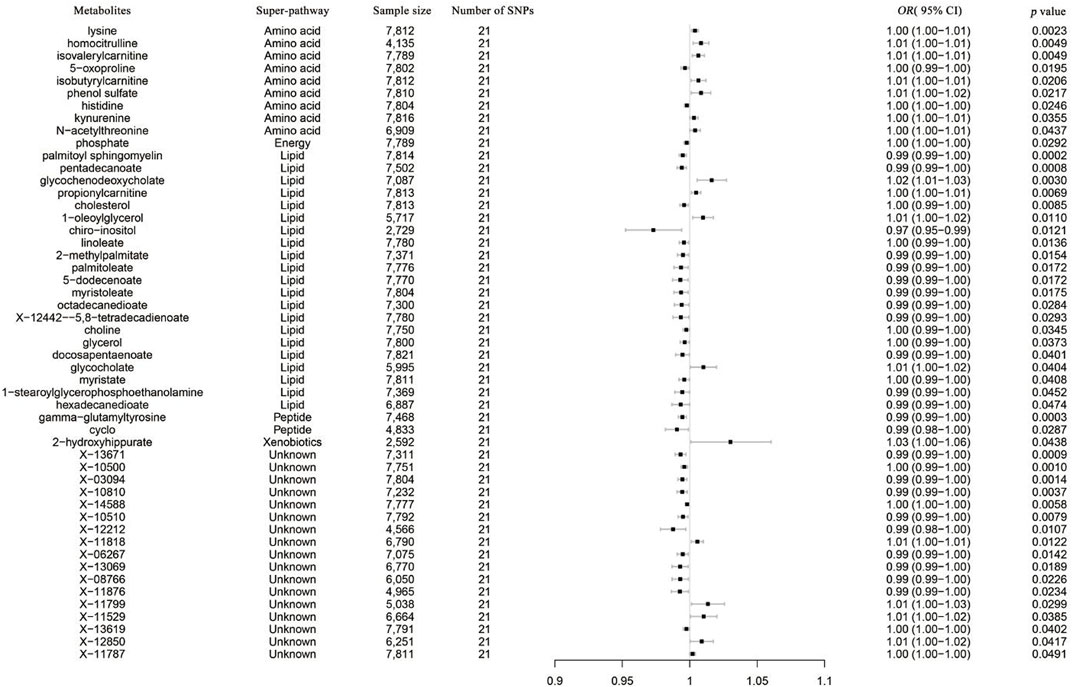
FIGURE 6. Forest plot of the causal effects of SLE on the risk of metabolites derived from the IVW method. OR, odds ratio; CI, confidence interval.
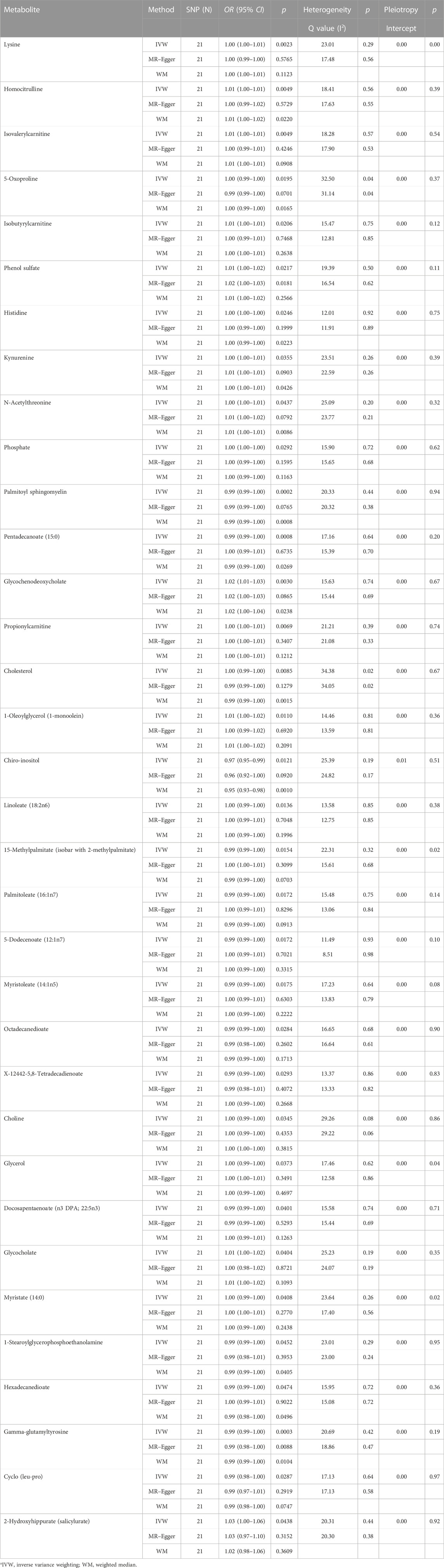
TABLE 3. The three MR model estimates of the causal relationships between the risk of SLE and 34 known metabolites and tests for heterogeneity and horizontal pleiotropy.
The results of the LDSC analysis show weak evidence of a genetic correlation between 36 metabolites and SLE (15 metabolites could not be used to estimate heritability because the genetic covariance matrix includes traits estimated to have negative heritability). Rg ranges from −0.3351 to 1.0997, the standard error from 0.0710 to 0.5204, and the p-value from 0.0023 to 0.9950. Except for phosphate belonging to the energy pathway (p = 0.0024) and X-13671 (unknown) (p = 0.0064), the genetic correlation of the other metabolites was not significant (p > 0.05), suggesting that these shared genetic components did not confound the MR estimates (Supplementary Table S2).
Thirty-three metabolites significantly influenced by SLE were put into the Metabolic Analyzer 5.0 platform to determine various potential metabolic pathways involved in the pathogenesis of SLE and immunomics. Among them, linoleic acid and docosapentaenoic acid (22n-6) were involved in the metabolic pathway of alpha-linolenic acid and linoleic acid metabolism (p = 0.0260), choline and phosphate were involved in the metabolic pathway of betaine metabolism (p = 0.0314), and glycerol and phosphate were involved in the metabolic pathway of glycerolipid metabolism (p = 0.0435) (Table 4). The metabolic mechanism formed by the above metabolites may be involved in the pathogenesis impacted by SLE. Figure 7 exhibits the network of interactions among the metabolic pathways involved in this analysis.
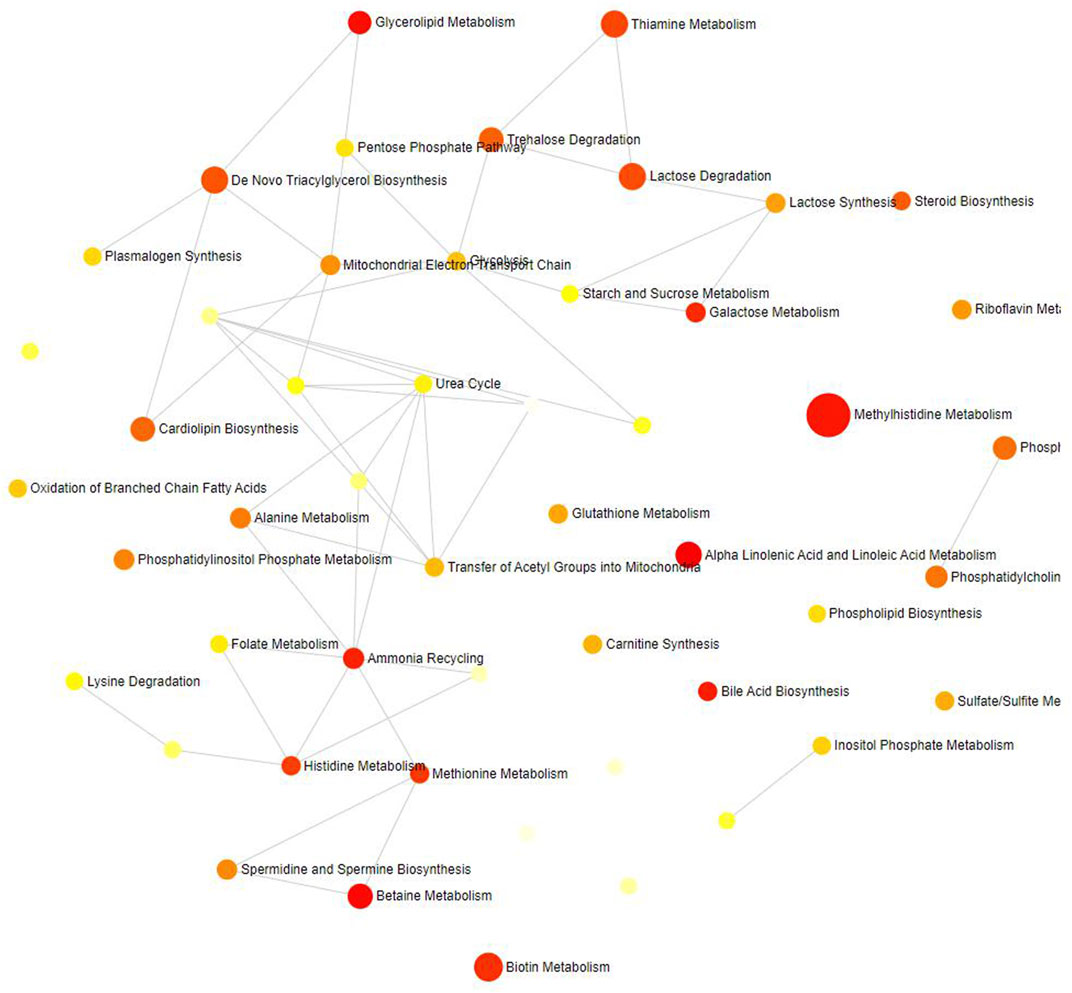
FIGURE 7. Network of enrichment pathways of the metabolites selected by reverse MR. The colour ranges from light yellow to dark red, indicating the level of enrichment significance, and the size of the circle reflects the level of the enrichment ratio.
3.3 Intersection between forward MR and reverse MR
Intersection analysis was introduced to analyse the shared metabolites screened by the forward and reverse MR analyses. Palmitoleate belonging to the lipid superpathway and isobutyrylcarnitine and phenol sulfate belonging to the amino acid superpathway appeared in the intersection (Figure 8).
4 Discussion
We elucidated the bidirectional causal relationship between metabolites and SLE by using genetic variation as the IVs in a two-sample MR model. We discovered that 24 metabolites belonging to the lipid, carbohydrate, xenobiotic and amino acid superpathways may increase the risk of SLE occurrence. In addition, the metabolic disorders of 51 metabolites belonging to the amino acid, energy, xenobiotics, peptide and lipid superpathways were affected by SLE. Palmitoleate belonging to the lipid superpathway and isobutyrylcarnitine and phenol sulfate belonging to the amino acid superpathway were factors with two-way causation.
In the first part of this study (forward MR), the metabolism of bile acids has aroused our great concern. Our study showed that the significantly altered metabolites (taurodeoxycholate, taurochenodeoxycholate, and ursodeoxycholate) were mapped to bile acid metabolism pathways (p = 0.035).
There is a subtle relationship between bile acid metabolism, gut microbes and the immune system (Cai et al., 2022; Collins et al., 2023). Bile acids are involved in processes including glucose and lipid metabolism, energy balance, and immune regulation through several key receptors such as the farnesoid X receptor (FXR), Takeda G protein-coupled receptor 5 (TGR5), pregnane X receptor (PXR), vitamin D receptor (VDR), sphingosine-1-phosphate receptor 2(SP1PR2), and retinoid-related orphan receptorγt (RORγt) (Jia et al., 2023). In the T cells of the intestine, bile acids affect the differentiation of Th17, Treg through the regulation of RORγt, FXR, and VDR receptors (Hang et al., 2019). Through Spearman’s correlation analysis showed good prediction of the SLEDAI score by bile acids (deoxycholic acid, glycocholic acid, and isohyodeoxycholic acid) and arachidonic acid, demonstrating a correlation between bile acids and SLE activity in a metabolomics study (He et al., 2020a). Our MR analysis indicated that individuals with high levels of bile acids (ursodeoxycholate, taurodeoxycholate, taurochenodeoxycholate) were more likely to develop SLE. Previous research on SLE found that chenodeoxycholic acid (CDCA) suppresses inflammatory cytokines and showing its association with SLE in MRL/lpr mice (Lian et al., 2012). Considering that bile acids are involved in the metabolic balance and immunoregulatory processes in multiple tissues, the exploration of the mechanism affecting systemic lupus erythematosus through the regulation of bile acids and their receptors will be the next step of our research.
Altered lipid metabolism is well known to occur in SLE patients. Especially in serum, a metabolomics study revealed that the identified lipids accounted for approximately 46.3% of the total identified metabolites, and the altered lipids in SLE patients were more than 65% of all changed metabolites (de Carvalho et al., 2008). Two observational studies from China and Brazil suggested that SLE can cause disorders of lipid metabolism [12,28]. In the present systematic MR study, we confirmed these associations of lipid metabolism (palmitoleat, isobutyrylcarnitine, 1-arachidonoylglycerophosphocholine, 1-oleoylglycerophosphoethanolamine) with the risk of SLE from a genetic perspective.
Citrullline was found to be protective as a differential metabolite in our MR analyses (p = 0.0489). No direct literature reports of citrulline and SLE have been retrieved. But previous studies have shown that increased citrulline directly binds to JAK2 and inhibits JAK2-STAT1 signalling (Nakayamada and Tanaka, 2022). The JAK-STAT signalling pathway is involved in the pathogenesis of multiple autoimmune diseases, including SLE. In an animal model of lupus, the JAK inhibitor tofacitinib improved clinical features, immune deregulation, and vascular dysfunction. It can therefore be speculated that increased citrulline, as a JAK2 inhibitor, may act as a protective factor in the pathogenesis of SLE.
1,5-AG is one of the main polyol sugars in the human body, is mainly derived from food and is hardly synthesized by the human body (Yamanouchi and Akanuma, 1994). When blood glucose levels are above the renal glucose threshold, the reabsorption of 1,5-AG in the kidney is competitively inhibited by glucose, which leads to a decrease in serum 1,5-AG levels. Measuring serum 1,5-AG levels can reflect the average blood glucose level in the last 1–2 weeks (Almeida et al., 2021). Previous studies showed that elevated levels of 1,5-AG in the immune microenvironment could inhibit macrophage proinflammatory polarization and promote survival of acute B lymphocyte leukemia cells in vitro through upregulation of C-X-C Motif Chemokine Ligand 14 (CXCL14) (Wu et al., 2023b). Our MR study identified that 1,5-AG acted as a pathogenic factor, leading to a high risk of SLE (p = 0.0109). Considering that the detection technology of 1, 5-AG is relatively stable and mature (Ortiz-Martínez et al., 2022), it has the potential to be used as a biomarker for predicting SLE to achieve clinical translation.
In a metabolomics study covering 4,569 differentially expressed metabolites identified in SLE, elevated levels of these metabolites were associated with the biosynthesis of valine, leucine, and isoleucine (Zeng et al., 2021). This study indicated that the metabolism of isoleucine is involved in the pathogenesis of SLE. By comparison, our MR study identified that isoleucine (p = 0.0431) also acted as a pathogenic factor, leading to a high risk of SLE.
For the other metabolites we screened, such as 2-methoxyacetaminophen sulfate, xanthine, N1-methyladenosine, we had not found reports related to SLE. A mounting array of studies has revealed that patients with SLE have abnormal metabolism compared to the general population (Teng et al., 2020). Due to limited understanding of the role about these metabolite in SLE, it would be a suggestive finding.
In the second part of this analysis (reverse MR), we found that alpha-linolenic acid and linoleic acid were the most significantly enriched metabolic pathways (p = 0.0260). The linolenic acid involved in this pathway is a new class of oxylipins that are produced by enzymatic or nonenzymatic oxidation of polyunsaturated fatty acids (PUFAs) (Kortz et al., 2014). Oxidized lipids play a very important role in the pathological and physiological environment, especially in inflammation and the immune response (Fullerton et al., 2014; Dennis and Norris, 2015). In SLE, several oxylipins have been identified to be significantly changed and critical for SLE pathophysiology, suggesting the potential utility of oxylipins as candidate biomarkers for SLE diagnosis and clinical treatment (Hye Khan et al., 2019; He et al., 2020b). Jingquan H et al. used targeted mass spectrometry analysis to explore the alteration of oxylipins in the serum of 98 SLE patients and 106 healthy controls (He et al., 2022). The negative correlation of linolenic acid with lupus nephritis and SLE disease activity is consistent with our study. This study further confirmed that SLE may be the cause of reduced linolenic acid metabolism.
This reverse MR analysis also revealed enrichment of the betaine metabolism pathway that was significantly associated with SLE (p = 0.0314). Although we did not find reports of a direct association between betaine and SLE, a study based on ultra-high-performance liquid chromatography-quadrupole time-of-flight mass spectrometry suggested that the betaine pathway differed significantly between rheumatoid arthritis patients and healthy controls (Zhu et al., 2022). Rheumatoid arthritis and SLE are autoimmune-related diseases. In fact, these two diseases share several clinical manifestations, serological profiles, and immunological characteristics. The third significant metabolic enrichment pathway of interest in the reverse MR analysis was glycerolipid metabolism (p = 0.0435). Glycerolipids are an important component of the cell membrane. Their activation not only has antioxidant effects (Matsufuji et al., 2000) but also has anti-inflammatory effects, especially in atherosclerosis caused by inflammatory factors (KaranionisH et al., 2002). It is well known that atherosclerosis is a major complication of SLE (Liu et al., 2022). The levels of circulating apoptotic endothelial cells are increased in SLE patients and are strongly linked to vascular dysfunction and increased tissue factor levels, suggesting an imbalance between endothelial cell damage and repair in individuals with SLE (Rajagopalan et al., 2004). The regulation of glycerolipids, as intermediate metabolites, is undoubtedly an innovative idea to prevent atherosclerosis in SLE patients.
In addition to the above significantly enriched pathways, this study specifically focused on three metabolites (palmitoleate, isobutyrylcarnitine and phenol sulfate) with bidirectional causal effects on SLE. Palmitoleic acid is a kind of free fatty acids (FFAs), and its correlation with SLE has been studied. A study used gas chromatography with mass spectrometry to identify the relationship between 24 FFAs and SLE (Shin et al., 2017). The results showed that palmitoleic acid levels were significantly elevated in SLE patients. Our causal study suggests that the elevation of palmitoleic acid levels may be due to metabolic abnormalities caused by the SLE immune response. In a study of serum metabolites and acute graft-versus-host disease (GVHD), isobutyrylcarnitine was found to be altered in patients with advanced GVHD (Reikvam et al., 2016). Carnitine is important for fatty acid transport and may also be important for the release of immunomodulatory cytokines. There is a lack of direct reports on the relationship between phenol sulfate and SLE. However, phenol sulfate is the main metabolite of intestinal microorganisms (Lustgarten et al., 2014), and the balance of intestinal microorganisms is closely related to the pathogenicity of SLE (Manfredo Vieira et al., 2018). Under the influence of the gut microbiota, the microbial pathways related to sulfur metabolism are altered in SLE patients (Tomofuji et al., 2021). In short, the above three metabolites may be involved in the whole process of SLE onset and development, and their metabolic mechanisms interact with SLE-related mechanisms.
Our study has several merits. In this observational study, the application of MR methodology efficiently decreases the potential confounding factors and reverses causality. The chronology of the causal chain of metabolites and SLE is clearer. Second, because a large sample for the MR analysis was recruited in this study, the statistical power of the results was increased. Third, to avoid bias from population stratification, this research limited the GWAS data to the major European ancestry cohorts. Furthermore, we adopted a two-way MR analysis process to more comprehensively explain the causal relationship between metabolites and SLE, as well as the mechanisms of the metabolic abnormalities associated with SLE onset and disease progression.
There are certain limitations to this study. First, the study was carried out using summary datasets, which makes it challenging to undertake stratified analysis. Some behavioural and biological characteristics are obscured. Second, it is possible that some metabolites associated with SLE may be excluded due to the level of pleiotropy of the IVs. Third, some important metabolites and pathways are ignored because they are not named or annotated in the pathway database. Therefore, the unproven metabolites still need to be explored in depth. In the future, we will continue to pay attention to the database, especially the characteristics of different types of SLE, and make more accurate prediction of influencing factors.
In conclusion, the results of this study indicate that the levels of some particular metabolites may either contribute to the immune response inducing SLE or may be intermediates in the development and progression of SLE. These metabolites can be used as auxiliary diagnostic tools for SLE and for the evaluation of disease progression and therapeutic effects. Future studies are necessary to derive more accurate inferences from our findings.
Data availability statement
The datasets presented in this study can be found in online repositories. The names of the repository/repositories and accession number(s) can be found in the article/Supplementary Material.
Ethics statement
Ethical approval was not required for the study involving humans in accordance with the local legislation and institutional requirements. Written informed consent to participate in this study was not required from the participants or the participants’ legal guardians/next of kin in accordance with the national legislation and the institutional requirements.
Author contributions
LL: Conceptualization, Data curation, Formal Analysis, Investigation, Project administration, Software, Validation, Visualization, Writing–original draft, Writing–review and editing. WL: Data curation, Formal Analysis, Investigation, Validation, Visualization, Writing–review and editing. QM: Data curation, Formal Analysis, Investigation, Validation, Visualization, Writing–review and editing. YL: Conceptualization, Funding acquisition, Methodology, Project administration, Supervision, Writing–review and editing. ZC: Conceptualization, Formal Analysis, Funding acquisition, Methodology, Project administration, Software, Supervision, Writing–original draft, Writing–review and editing.
Funding
The author(s) declare financial support was received for the research, authorship, and/or publication of this article. This study was financially supported by the National Natural Science Foundation of China (Nos 81760561 and 82260656) in analysis and interpretation of data.
Acknowledgments
We are grateful to all the participants and investigators of the FinnGen Consortium, as well as to all investigators who contributed to the GWAS of modifiable risk factors.
Conflict of interest
The authors declare that the research was conducted in the absence of any commercial or financial relationships that could be construed as a potential conflict of interest.
Publisher’s note
All claims expressed in this article are solely those of the authors and do not necessarily represent those of their affiliated organizations, or those of the publisher, the editors and the reviewers. Any product that may be evaluated in this article, or claim that may be made by its manufacturer, is not guaranteed or endorsed by the publisher.
Supplementary material
The Supplementary Material for this article can be found online at: https://www.frontiersin.org/articles/10.3389/fmolb.2023.1281987/full#supplementary-material
Abbreviations
SLE, Systemic Lupus Erythematosus; RCT, Randomized Controlled Trial; MR, Mendelian Randomization; IVs, Instrumental Variables; GWAS, Genome-Wide Association Study; SNPs, Single Nucleotide Polymorphisms; LDSC, Linkage Disequilibrium Score; IVW, Inverse Variance Weighting; WM, Weighted Median; KEGG, Kyoto Encyclopedia of Genes and Genomes; SMPDB, Small Molecule Pathway Database; GAPDH, glyceraldehyde-3-phosphate dehydrogenase; IFN-γ, Interferon γ; FXR, farnesoid X receptor; TGR5, Takeda G protein-coupled receptor 5; PXR, pregnane X receptor; VDR, vitamin D receptor; SP1PR2, sphingosine-1-phosphate receptor 2; RORγt, retinoid-related orphan receptorγt; SLEDAI, systemic lupus erythematosus disease activity index; CXCL14, C-X-C Motif Chemokine Ligand 14; 1,5-AG, 1,5-anhydroglucitol; PUFAs, Polyunsaturated Fatty Acids; CDCA, chenodeoxycholic acid; FFAs, Free Fatty Acids; GVHD, Graft-versus-host Disease.
References
Almeida, L., Dhillon-LaBrooy, A., Carriche, G., Berod, L., and Sparwasser, T. (2021). CD4+ T-cell differentiation and function: unifying glycolysis, fatty acid oxidation, polyamines NAD mitochondria. J. Allergy Clin. Immunol. 148 (1), 16–32. doi:10.1016/j.jaci.2021.03.033
Barber, M. R. W., Drenkard, C., Falasinnu, T., Hoi, A., Mak, A., Kow, N. Y., et al. (2021). Global epidemiology of systemic lupus erythematosus. Nat. Rev. Rheumatol. 17 (9), 515–532. doi:10.1038/s41584-021-00668-1
Bentham, J., Morris, D. L., Graham, D. S. C., Pinder, C. L., Tombleson, P., Behrens, T. W., et al. (2015). Genetic association analyses implicate aberrant regulation of innate and adaptive immunity genes in the pathogenesis of systemic lupus erythematosus. Nat. Genet. 47 (12), 1457–1464. doi:10.1038/ng.3434
Bhaskar, LVKS, and Nagaraju, G. P. (2019). Clinical and immunogenetic aspects of systemic lupus erythematosus. Crit. Rev. Immunol. 39 (5), 343–360. doi:10.1615/CritRevImmunol.2020033247
Bulik-Sullivan, B., Finucane, H. K., Anttila, V., Gusev, A., Day, F. R., Loh, P. R., et al. (2015). An atlas of genetic correlations across human diseases and traits. Nat. Genet. 47 (11), 1236–1241. doi:10.1038/ng.3406
Burgess, S., and Thompson, S. G. (2017). Interpreting findings from Mendelian randomization using the MR-egger method. Eur. J. Epidemiol. 32 (5), 377–389. doi:10.1007/s10654-017-0255-x
Burgess, S., Butterworth, A., and Thompson, S. G. (2013). Mendelian randomization analysis with multiple genetic variants using summarized data. Genet. Epidemiol. 37 (7), 658–665. doi:10.1002/gepi.21758
Cai, J., Sun, L., and Gonzalez, F. J. (2022). Gut microbiota-derived bile acids in intestinal immunity, inflammation, and tumorigenesis. Cell Host Microbe 30 (3), 289–300. doi:10.1016/j.chom.2022.02.004
Chang, C. H., Curtis, J. D., Maggi, L. B., Faubert, B., Villarino, A. V., O'Sullivan, D., et al. (2013). Posttranscriptional control of T cell effector function by aerobic glycolysis. Cell 153 (6), 1239–1251. doi:10.1016/j.cell.2013.05.016
Chong, J., and Xia, J. (2104). Using MetaboAnalyst 4 0 for metabolomics data analysis, interpretation and integration with other omics data. Methods Mol. Biol. 11, 337–360. doi:10.1007/978-1-0716-0239-3_17
Cohen, J. F., Chalumeau, M., Cohen, R., Korevaar, D. A., Khoshnood, B., and Bossuyt, P. M. (2015). Cochran's Q test was useful to assess heterogeneity in likelihood ratios in studies of diagnostic accuracy. JClin Epidemiol. 68 (3), 299–306. doi:10.1016/j.jclinepi.2014.09.005
Collins, S. L., Stine, J. G., Bisanz, J. E., Okafor, C. D., and Patterson, A. D. (2023). Bile acids and the gut microbiota: metabolic interactions and impacts on disease. Nat. Rev. Microbiol. 21 (4), 236–247. doi:10.1038/s41579-022-00805-x
de Carvalho, J. F., Bonfá, E., and Borba, E. F. (2008). Systemic lupus erythematosus and "lupus dyslipoproteinemia. Autoimmun. Rev. 7 (3), 246–250. doi:10.1016/j.autrev.2007.11.016
Dennis, E. A., and Norris, P. C. (2015). Eicosanoid storm in infection and inflammation. Nat. Rev. Immunol. 15 (8), 511–523. doi:10.1038/nri3859
Fullerton, J. N., O'Brien, A. J., and Gilroy, D. W. (2014). Lipid mediators in immune dysfunction after severe inflammation. Trends Immunol. 35 (1), 12–21. doi:10.1016/j.it.2013.10.008
Hang, S., Paik, D., Yao, L., Kim, E., Trinath, J., Lu, J., et al. (2019). Bile acid metabolites control TH17 and Treg cell differentiation. Nature 576 (7785), 143–148. doi:10.1038/s41586-019-1785-z
He, J., Chan, T., Hong, X., Zheng, F., Zhu, C., Yin, L., et al. (2020a). Microbiome and metabolome analyses reveal the disruption of lipid metabolism in systemic lupus erythematosus. Front. Immunol. 11, 1703. doi:10.3389/fimmu.2020.01703
He, J., Chan, T., Hong, X., Zheng, F., Zhu, C., Yin, L., et al. (2020b). Microbiome and metabolome analyses reveal the disruption of lipid metabolism in systemic lupus erythematosus. Front. Immunol. 11, 1703. doi:10.3389/fimmu.2020.01703
He, J., Ma, C., Tang, D., Zhong, S., Yuan, X., Zheng, F., et al. (2022). Absolute quantification and characterization of oxylipins in lupus nephritis and systemic lupus erythematosus. Front. Immunol. 13, 964901. doi:10.3389/fimmu.2022.964901
Hye Khan, M. A., Stavniichuk, A., Sattar, M. A., Falck, J. R., and Imig, J. D. (2019). Epoxyeicosatrienoic acid analog EET-a blunts development of lupus nephritis in mice. Front. Pharmacol. 10, 512. doi:10.3389/fphar.2019.00512
Jia, W., Li, Y., Cheung, K. C. P., and Zheng, X. (2023). Bile acid signaling in the regulation of whole body metabolic and immunological homeostasis. Sci. China Life Sci. doi:10.1007/s11427-023-2353-0
Jin, H., Lee, S., and Won, S. (2020). Causal evaluation of laboratory markers in type 2 diabetes on cancer and vascular diseases using various mendelian randomization tools. Front. Genet. 11, 597420. doi:10.3389/fgene.2020.597420
Kanehisa, M., Goto, S., Sato, Y., Furumichi, M., and Tanabe, M. (2012). KEGG for integration and interpretation of large-scale molecular data sets. Nucleic Acids Res. 40, D109–D114. doi:10.1093/nar/gkr988
KaranionisH, C., Anionopoulou, S., and Demopoulos, C. A. (2002). Antithrombotic lipid minor constituents from vegetable oils comparison between olive oils and others. J. agriculural food Chem. 50 (5), 1150–1160. doi:10.1021/jf010923t
Kortz, L., Dorow, J., and Ceglarek, U. (2014). Liquid chromatography-tandem mass spectrometry for the analysis of eicosanoids and related lipids in human biological matrices: a review. J. Chromatogr. B Anal. Technol. Biomed. Life Sci. 964, 1–11. doi:10.1016/j.jchromb.2014.01.046
Lian, F., Wang, Y., Chen, J., Xu, H., Yang, X., Liang, L., et al. (2012). Activation of farnesoid X receptor attenuates liver injury in systemic lupus erythematosus. Rheumatol. Int. 32 (6), 1705–1710. doi:10.1007/s00296-011-1874-2
Liu, Y., Yu, X., Zhang, W., Zhang, X., Wang, M., and Ji, F. (2022). Mechanistic insight into premature atherosclerosis and cardiovascular complications in systemic lupus erythematosus. J. Autoimmun. 132, 102863. doi:10.1016/j.jaut.2022.102863
Lustgarten, M. S., Price, L. L., Chalé, A., and Fielding, R. A. (2014). Metabolites related to gut bacterial metabolism, peroxisome proliferator-activated receptor-alpha activation, and insulin sensitivity are associated with physical function in functionally-limited older adults. Aging Cell 13 (5), 918–925. doi:10.1111/acel.12251
Manfredo Vieira, S., Hiltensperger, M., Kumar, V., Zegarra-Ruiz, D., Dehner, C., Khan, N., et al. (2018). Translocation of a gut pathobiont drives autoimmunity in mice and humans. Science 359, 1156–1161. doi:10.1126/science.aar7201
Matsufuji, M., Taguchi, K., Inagaki, M., Higuchi, R., Ohta, S., and Yoshimoto, A. (2000). Glyceroglycolipids preventing tert-butylhydroperoxide-induced cell death from Microbacterium sp. and Corynebacterium aquaticum strains. JournalofBioscienceandBioengineering 89 (2), 170–175. doi:10.1016/s1389-1723(00)88732-0
Murphy, M. P., and O'Neill, L. A. J. (2018). Krebs cycle reimagined: the emerging roles of succinate and itaconate as signal transducers. Cell 174 (4), 780–784. doi:10.1016/j.cell.2018.07.030
Nakayamada, S., and Tanaka, Y. (2022). Pathological relevance and treatment perspective of JAK targeting in systemic lupus erythematosus. Expert Rev. Clin. Immunol. 18 (3), 245–252. doi:10.1080/1744666X.2022.2040988
Nashi, R. A., and Shmerling, R. H. (2021). Antinuclear antibody testing for the diagnosis of systemic lupus erythematosus. Med. Clin. North Am. 105 (2), 387–396. doi:10.1016/j.mcna.2020.10.003
O'Connor, L. J., and Price, A. L. (2018). Distinguishing genetic correlation from causation across 52 diseases and complex traits. Nat. Genet. 50 (12), 1728–1734. doi:10.1038/s41588-018-0255-0
Ortiz-Martínez, M., González-González, M., Martagón, A. J., Hlavinka, V., Willson, R. C., and Rito-Palomares, M. (2022). Recent developments in biomarkers for diagnosis and screening of type 2 diabetes mellitus. Curr. Diab Rep. 22 (3), 95–115. doi:10.1007/s11892-022-01453-4
Ouyang, X., Dai, Y., Wen, J. L., and Wang, L. X. (2011). ¹H NMR-based metabolomic study of metabolic profiling for systemic lupus erythematosus. Lu—pus 20, 1411–1420. doi:10.1177/0961203311418707
Rajagopalan, S., Somers, E. C., Brook, R. D., Kehrer, C., Pfenninger, D., Lewis, E., et al. (2004). Endothelial cell apoptosis in systemic lupus erythematosus: a common pathway for abnormal vascular function and thrombosis propensity. Blood 103 (10), 3677–3683. doi:10.1182/blood-2003-09-3198
Reay, W. R., Kiltschewskij, D. J., Geaghan, M. P., Atkins, J. R., Carr, V. J., Green, M. J., et al. (2022). Genetic estimates of correlation and causality between blood-based biomarkers and psychiatric disorders. Sci. Adv. 8 (14), 8969. doi:10.1126/sciadv.abj8969
Reikvam, H., Hatfield, K., and Bruserud, Ø. (2016). The pretransplant systemic metabolic profile reflects a risk of acute graft versus host disease after allogeneic stem cell transplantation. Metabolomics 12 (1), 12. doi:10.1007/s11306-015-0880-x
Shin, S. Y., Fauman, E. B., Petersen, A. K., Krumsiek, J., Santos, R., Huang, J., et al. (2014). An atlas of genetic influences on human blood metabolites. Nat. Genet. 46 (6), 543–550. doi:10.1038/ng.2982
Shin, T. H., Kim, H. A., Jung, J. Y., Baek, W. Y., Lee, H. S., Park, H. J., et al. (2017). Analysis of the free fatty acid metabolome in the plasma of patients with systemic lupus erythematosus and fever. Metabolomics 14 (1), 14. doi:10.1007/s11306-017-1308-6
Teng, X., Brown, J., Choi, S. C., Li, W., and Morel, L. (2020). Metabolic determinants of lupus pathogenesis. Immunol. Rev. 295 (1), 167–186. doi:10.1111/imr.12847
Tomofuji, Y., Maeda, Y., Oguro-Igashira, E., Kishikawa, T., Yamamoto, K., Sonehara, K., et al. (2021). Metagenome-wide association study revealed disease-specific landscape of the gut microbiome of systemic lupus erythematosus in Japanese. Ann. Rheum. Dis. 80 (12), 1575–1583. doi:10.1136/annrheumdis-2021-220687
Ugarte-Gil, M. F., González, L. A., and Alarcón, G. S. (2019). Lupus: the new epidemic. Lupus 28 (9), 1031–1050. doi:10.1177/0961203319860907
Wu, H., Chang, C., and Lu, Q. (2020). The epigenetics of lupus erythematosus. Adv. Exp. Med. Biol. 1253, 185–207. doi:10.1007/978-981-15-3449-2_7
Wu, Y., Zhao, M., Gong, N., Zhang, F., Chen, W., and Liu, Y. (2023a). Immunometabolomics provides a new perspective for studying systemic lupus erythematosus. Int. Immunopharmacol. 118, 109946. doi:10.1016/j.intimp.2023.109946
Wu, M., Zhang, S., Chi, C., Zhu, H., Ma, H., Liu, L., et al. (2023b). 1,5-AG suppresses pro-inflammatory polarization of macrophages and promotes the survival of B-ALL in vitro by upregulating CXCL14. Mol. Immunol. 158, 91–102. doi:10.1016/j.molimm.2023.05.003
Yamanouchi, T., and Akanuma, Y. (1994). Serum 1,5-anhydroglucitol (1,5 AG): new clinical marker for glycemic control. Diabetes Res. Clin. Pract. 24, S261–S268. doi:10.1016/0168-8227(94)90259-3
Zeng, L., Chen, N., Liao, J., Shen, X., Song, S., and Wang, F. (2021). Metabolic analysis of potential key genes associated with systemic lupus erythematosus using liquid chromatography-mass spectrometry. Comput. Math. Methods Med. 2021, 5799348. doi:10.1155/2021/5799348
Keywords: metabolites, systemic lupus erythematosus, correlations, Mendelian randomization, bidirectional
Citation: Li L, Li W, Ma Q, Lin Y and Cui Z (2023) Exploring the causal correlations between 486 serum metabolites and systemic lupus erythematosus: a bidirectional Mendelian randomization study. Front. Mol. Biosci. 10:1281987. doi: 10.3389/fmolb.2023.1281987
Received: 08 September 2023; Accepted: 30 October 2023;
Published: 09 November 2023.
Edited by:
Morana Jaganjac, Rudjer Boskovic Institute, CroatiaReviewed by:
Valeria Lodde, University of Sassari, ItalyUmesh Kumar, University of Innsbruck, Austria
Copyright © 2023 Li, Li, Ma, Lin and Cui. This is an open-access article distributed under the terms of the Creative Commons Attribution License (CC BY). The use, distribution or reproduction in other forums is permitted, provided the original author(s) and the copyright owner(s) are credited and that the original publication in this journal is cited, in accordance with accepted academic practice. No use, distribution or reproduction is permitted which does not comply with these terms.
*Correspondence: Youkun Lin, bGlueW91a3VuQGd4bXUuZWR1LmNu; Zhezhe Cui, Y3p6Njk5N0AxNjMuY29t