- 1Department of Respiratory Medicine, University Children’s Hospital Zurich, Zurich, Switzerland
- 2Department of Chemistry and Applied Biosciences, ETH Zurich, Zurich, Switzerland
- 3Department of Pulmonology, University Hospital Zurich, Zurich, Switzerland
- 4Division of Clinical Chemistry and Biochemistry, University Children’s Hospital Zurich, Zurich, Switzerland
Introduction: There is a need to improve the diagnosis and management of pediatric asthma. Breath analysis aims to address this by non-invasively assessing altered metabolism and disease-associated processes. Our goal was to identify exhaled metabolic signatures that distinguish children with allergic asthma from healthy controls using secondary electrospray ionization high-resolution mass spectrometry (SESI/HRMS) in a cross-sectional observational study.
Methods: Breath analysis was performed with SESI/HRMS. Significant differentially expressed mass-to-charge features in breath were extracted using the empirical Bayes moderated t-statistics test. Corresponding molecules were putatively annotated by tandem mass spectrometry database matching and pathway analysis.
Results: 48 allergic asthmatics and 56 healthy controls were included in the study. Among 375 significant mass-to-charge features, 134 were putatively identified. Many of these could be grouped to metabolites of common pathways or chemical families. We found several pathways that are well-represented by the significant metabolites, for example, lysine degradation elevated and two arginine pathways downregulated in the asthmatic group. Assessing the ability of breath profiles to classify samples as asthmatic or healthy with supervised machine learning in a 10 times repeated 10-fold cross-validation revealed an area under the receiver operating characteristic curve of 0.83.
Discussion: For the first time, a large number of breath-derived metabolites that discriminate children with allergic asthma from healthy controls were identified by online breath analysis. Many are linked to well-described metabolic pathways and chemical families involved in pathophysiological processes of asthma. Furthermore, a subset of these volatile organic compounds showed high potential for clinical diagnostic applications.
1 Introduction
Asthma is the most frequent chronic condition in children in the developed world. The disease is very heterogeneous in its presentation and clinical course. Due to the lack of a well-recognized and easy to apply diagnostic gold-standard (Gaillard et al., 2021), misdiagnosis is relatively common. Reported numbers range from 10% to 62% for underdiagnosis (Kaur et al., 1998; Siersted et al., 1998; van Gent et al., 2007) from 48% to 53% for overdiagnosis (Looijmans-van den Akker et al., 2016; Yang et al., 2017). This has negative impacts on asthma related morbidity, quality-of-life, medication side-effects, prognosis, and health costs. Therefore, the investigation of pediatric asthma and its associated molecular processes including airway inflammation is of high importance for the development of novel, much-needed diagnostic and monitoring applications.
Breath is known to contain several hundreds of metabolites that reflect metabolism as well as disease-specific mechanisms such as airway inflammation (Ferraro et al., 2018). Therefore, there is great interest in discovering endogenous exhaled organic compounds that are linked to diseases and their pathophysiological processes (Neerincx et al., 2017). One of the few clinical tests taking advantage of this is the quantification of exhaled fractional nitric oxide (FeNO), which can be measured in all age groups. FeNO is a biomarker for eosinophilic airway inflammation that is related to allergic asthma (Ferraro et al., 2018). This exemplifies the potential of applying breath analysis to further study allergic asthma and improve the diagnostic power of exhaled biomarkers.
Several breath analysis studies attempted to distinguish children with asthma from healthy controls by different techniques. Dallinga and colleagues compared exhaled breath of children with asthma and a healthy group by gas chromatography mass spectrometry and identified a small set of discriminatory volatile organic compounds (VOCs) that is potentially related to lipid peroxidation, including various hydrocarbons, xylene, benzoic acid, and butanoic acid (Dallinga et al., 2010). A pilot study from van Mastrigt et al. identified VOCs discriminating children with asthma, cystic fibrosis and healthy controls by using a broadband quantum cascade laser spectroscopy technique (van Mastrigt et al., 2016). The distinguishing compound classes included different carboxylic acids, esters, and ethers. Altogether, there is only little overlap between the detected metabolites of different studies and sometimes even conflicting results are reported. Therefore, standardization as well as external validation are challenges that need further research as summarized in recent reviews (Neerincx et al., 2017; Ferraro et al., 2018; Papamichael et al., 2021).
Secondary electrospray ionization high-resolution mass spectrometry (SESI/HRMS) is a technology applied for online breath analysis that links real-time measurements without sample preparation to high mass resolution (Gaugg et al., 2019). The latter strongly improves the confidence in compound identification of the detected mass-to-charge features (m/z features). Previous studies confirmed the potential of this technology to identify relevant exhaled organic compounds, including biological metabolites, and reveal altered molecular pathways for different respiratory diseases (Schwarz et al., 2016; Gaugg et al., 2019; Weber et al., 2020). A strength of SESI/HRMS lies in the detection of polar molecules with high molecular masses and low volatility (Gaugg et al., 2016; Bruderer et al., 2019; Chen et al., 2021). Furthermore, its applicability in children was confirmed in our previous study on cystic fibrosis (Weber et al., 2020).
The aim of this study was to identify metabolic signatures in exhaled breath consisting of discriminating organic compounds specific to allergic asthma in children by SESI/HRMS and to assess their biological context.
2 Materials and methods
2.1 Study design, participants, and clinical data
This observational cross-sectional study included children with allergic asthma and healthy controls, aged 5–18 years. Asthmatic patients from the outpatient clinic of the University Children’s Hospital Zürich, Switzerland, were recruited for this study. Asthma diagnosis was based on the recent ERS evidence-based practice guidelines (Gaillard et al., 2021) and only children with confirmed asthma were included. Allergic sensitization was defined by either a positive skin prick test or an allergen-specific IgE of >0.35 kU·L–1 by radioallergosorbent test or by ELISA for at least one common aeroallergen. Further, eligible patients were clinically stable enough to temporarily stop the inhalation of long-acting asthma medication at least 1 week before the measurements. Exclusion criteria were the inability to stop medication, and the presence of an acute respiratory infection during the last 2 weeks before the measurement. Clinical data was collected on the same day as breath analysis and is summarized together with anthropometric data in Table 1. Healthy controls without any chronic respiratory symptoms or known lung diseases were recruited from the public. The presence of an acute (respiratory) infection was an exclusion criterion for both groups. The measurement and recruitment period were in parallel and lasted for 15 months. Efforts were put into recruiting participants of both cohorts at a randomized schedule across daytime and throughout the study period. The sample size was based on our previous study with a similar design (Weber et al., 2020). All participants, where appropriate and parents gave their written informed consent in advance. The study was approved by the local ethics committee (KEK-ZH ID 2018–00441) and was conducted in accordance with the Declaration of Helsinki.
2.2 Breath analysis
Online breath analysis was performed using a SESI source (SuperSESI, FIT FossilionTech, Madrid, Spain) connected to a high-resolution time-of-flight mass spectrometer (TripleTOF 5600+, AB Sciex, Concord, ON, Canada). Methodological details and instrumental settings were previously described by our group (Weber et al., 2020). Minor adaptations are specified below. Children were exhaling directly into the instrument in a sitting position. The breathing maneuver consisted of at least three long exhalations at a constant pressure of 5 mbar with short breaks in between. A single-use mouthpiece (product No. 100078, ACE Instruments, Germany) was connected to the ionization source, which was heated to 130°C, by a sterilizable, custom-made polytetrafluoroethylene adapter. Measurements were recorded in positive (4500 V) and negative (−4500 V) ionization mode between the m/z range of 50–500 Da. The accumulation time was set to 0.5 s per scan. The collisionally activated dissociation (CAD) gas was adjusted to 0 to avoid fragmentation. Temperatures of the MS were set to 0, gas 1 was used to pressurize the vial of the electrospray and set to 24, gas 2 was not connected to the SESI source, and the curtain gas was set to 10. The pulser frequency was adjusted to 23.983 kHz and the pulse 1 duration was 2 µs. A net flow of 0.3 L/min was defined by a mass flow controller (Alicat Scientific, Inc., Tucson, AZ, United States) at the exhaust of the ionization source. The nanoelectrospray was generated using silica emitters (50 cm length, 20 µm diameter, New Objective Inc., Woburn, MA, United States) and a 0.1% (v/v) aqueous formic acid solution (Optima LC/MS Grade, Thermo Fisher Scientific, Waltham, MA, United States). All participants were asked not to brush their teeth, consume any food, drinks (except for water), or chewing gums 1 h prior to the measurements (Weber et al., 2020).
2.3 Data preprocessing
All data were recalibrated in PeakView 2.2 (AB Sciex, Concord, ON, Canada) and processed in R version 4.1.1 (R Foundation for Statistical Computing, Vienna, Austria). The conversion and preprocessing of the raw data were done in the same way as described in our previous work (Weber et al., 2020). In brief, the raw mass spectra were resampled by interpolation (Δm/z: 0.0005, m/z range: 50–500 Da), peak picking was performed on the average mass spectra associated with exhalation and signal intensities of the m/z features were determined by trapezoidal integration. The intensities of the m/z features were normalized to the total ion current, log2-transformed and arranged into a data matrix of breath profiles for further analysis. More details on data preprocessing are given in the Supplementary Material.
2.4 Statistical analysis
To account for confounding influences and reduce the heterogeneity within the groups, batch adjustment was performed by applying surrogate variable analysis (SVA) (Leek and Storey, 2007) on the data matrix of breath profiles. Identification of differentially expressed m/z features when comparing cases and controls was assessed by the empirical Bayes moderated t-statistics test (Smyth, 2004). Correction for multiple hypothesis testing was conducted using Benjamini-Hochberg procedure (Benjamini and Hochberg, 1995) with significance threshold set to the adjusted p-value of 0.05 to determine the statistically significant features. Additionally, the ability of the breath profiles to classify samples as allergic asthmatic or healthy was assessed with the support vector machines algorithm [13] trained and tested in a 10 times repeated stratified 10-fold cross-validation. To avoid using all features for classifier development, Boruta feature selection (Kursa et al., 2010) was applied in each cross-validation iteration to include only the potentially discriminating features between the allergic asthmatic and the healthy control group. In order to prevent bias during cross-validation all preprocessing steps, feature selection and classifier development were strictly conducted on the training data sets, preventing any information leak from the left-out samples (Varma and Simon, 2006). Details on statistical analysis are given in the supplementary material.
2.5 Feature identification
For the 100 most significantly discriminative m/z features per study group, compound identification was based on MS2 spectra that were recorded directly from exhaled breath by SESI/HRMS with the same instrument set up. The settings of the SESI source and TripleTOF MS were identical to the ones described above for the MS1 full scan acquisition, with the following exceptions: the accumulation time was set to 1.0 s per scan and the CAD gas to 6. Precursors were selected with an isolation window of 0.7 Da. Collision energy for precursor fragmentation was set to 20 eV with a ramped energy spread of ± 10 eV. The MS2 spectra were analyzed by a workflow adapted from a published method (Kaeslin et al., 2021) to detect isotopes, adducts, and losses, and with the SIRIUS software (v4.9.9) (Dührkop et al., 2019) to assign putative molecular formulae and chemical structures. The putatively identified compounds were screened for their biological context and subgrouped into metabolic pathways or chemical families. Additionally, pathway enrichment analysis using the mummichog algorithm (MetaboAnalyst, v5.0) (Pang et al., 2021) was performed for further identification on all significant features including those without recorded MS2 spectra, without compound suggestions or with excluded MS2 spectra (exclusion criteria: see Supplementary Table S1). Lastly, the detected m/z features were compared with previously identified compounds from literature. The certainty of identification was indicated by an identification (ID) confidence level ranging from ID 1 to 5, as described by Schymanski and colleagues (Schymanski et al., 2014). More details on the identification approach are included in the supplementary material, including a schematic overview (Supplementary Figure S1).
3 Results
3.1 Participants and clinical data
Exhaled breath samples of 48 allergic asthma patients and 56 healthy control participants, in total 104 children, were included in this study. The age and body mass index values of the two cohorts were comparable, whereas the asthmatic group contained more males than the healthy one. Detailed clinical characteristics of the two individual study cohorts are shown in Table 1. The use of short-acting beta-agonists was allowed until the day before measurements. All children with asthma had a known allergic sensitization to at least one aero-allergen and the asthma severity ranged from mild to moderate. The FeNO values were significantly elevated in the allergic asthma cohort. Additionally, the forced expiratory volume in 1 s (FEV1) of the asthmatics was lower, while the forced vital capacity of the groups was comparable. Twelve children of the healthy control group showed an allergic sensitization according to the skin prick test, but only two reported symptomatic allergies.
3.2 Discriminative breath patterns and their metabolic associations
The pre-processing of the acquired mass spectra of the study subjects revealed 2,315 m/z features associated with exhaled breath. 375 m/z features were found to be significantly different between the two groups (Benjamini-Hochberg adjusted p < 0.05), of which 179 were upregulated and 196 downregulated in the allergic asthma group (Figure 1A). Among those, 134 were assigned to compounds. Inspection of the first two principal components (PCs) of the 134 putatively identified features revealed a moderate separation between the groups along the first PC (24% variance in the data, Figure 1B).
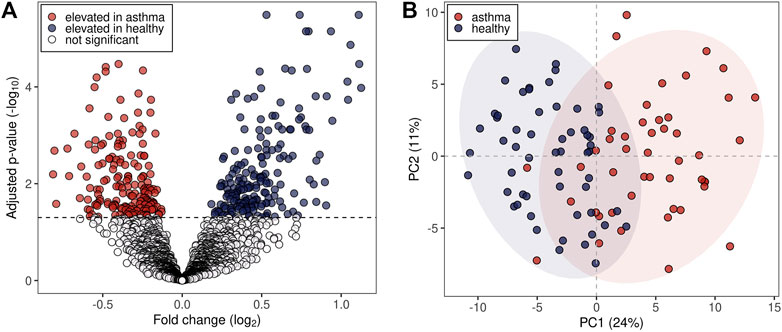
FIGURE 1. Statistical analysis of m/z features in breath profiles. (A) Volcano plot representing all detected 2,315 m/z features. Dashed line: Benjamini-Hochberg adjusted p-value of 0.05. (B) First two principal components (PCs) score plot of the 134 putatively identified m/z features. Blue dots represent healthy probands and red dots asthmatic patients. 95% data ellipses were added per group for visual depiction.
Compound identification revealed several specific metabolic pathways and chemical families with many representatives for both study cohorts (Tables 2, 3). For the allergic asthma group, the chemical families of fatty acid metabolites and monosaccharides as well as the 2-oxocarboxylic acid metabolism and two amino acid pathways, i.e., lysine degradation and tyrosine metabolism, were elevated (Table 2). The relations of metabolites involved in some of these elevated pathways are visualized in Figure 2. For the diminished compounds, arginine pathways were found to be well represented, including both arginine and proline metabolism and arginine biosynthesis. Further, several compounds of the linoleic acid metabolism and of the chemical groups of aldehydes, amides, and fatty acids were identified (Table 3; Figure 3). A full list including more details about the putatively identified compounds can be found in Supplementary Table S2.
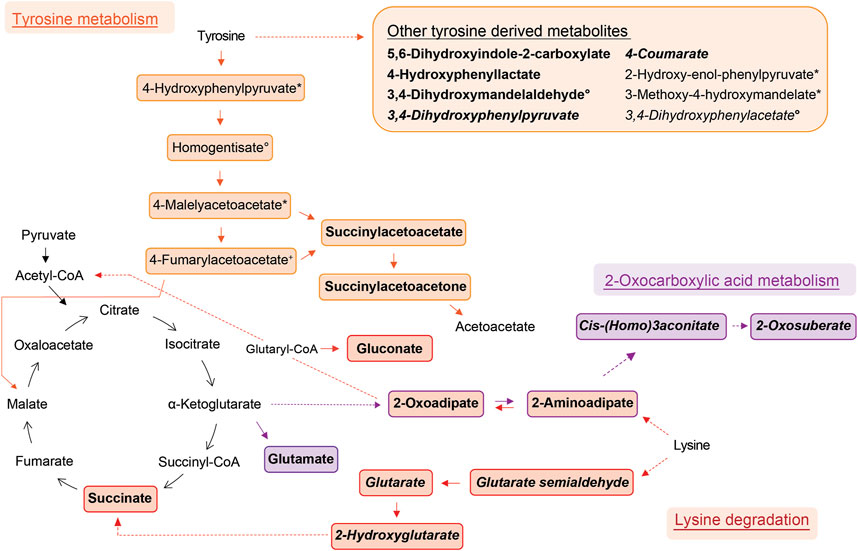
FIGURE 2. Schemes of metabolic pathways well-represented by compounds that were elevated in the allergic asthma group and putatively identified. Tyrosine derived metabolites besides the main degradation pathway in humans are summarised in the box. Two unrelated compounds of the 2-oxocarboxylic acid metabolism are not shown (see Table 2). Solid lines: direct metabolic relations; dashed lines: indirect metabolic relations (metabolites in between were not identified); colored: putatively identified compound; bold: identified by MS2, regular: identified based on exact mass and pathway mapping, or on literature; italic: metabolites from gut microbiota; *, °, +, #, ^, ‘: several possibilities for 1 m/z feature based on exact mass and pathway mapping.
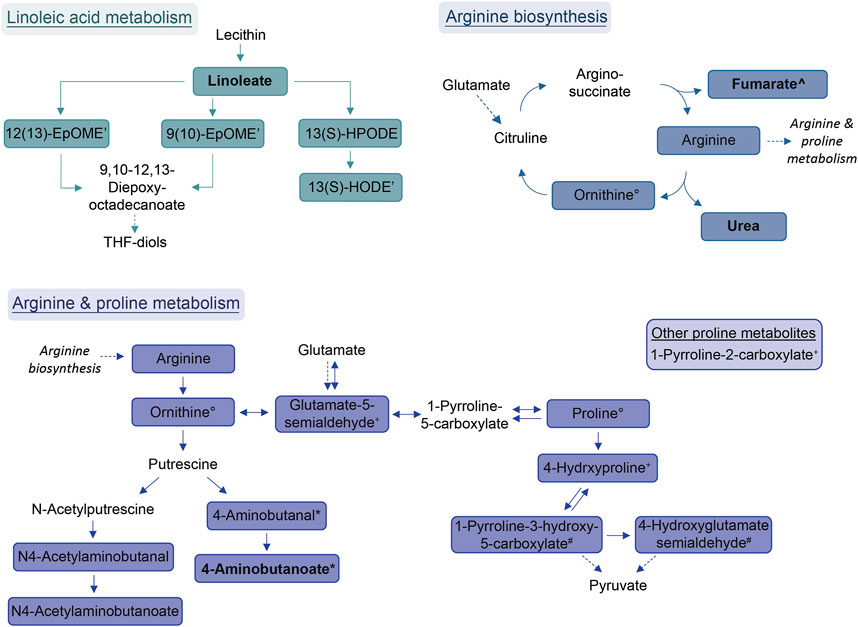
FIGURE 3. Schemes of metabolic pathways well-represented by compounds that were decreased in the allergic asthma group and putatively identified. Proline metabolism: One component is not directly connected to the displayed pathway and is shown in the box. Solid lines: direct metabolic relations; dashed lines: indirect metabolic relations; colored: putatively identified compound; bold: identified by MS2; regular: identified based on exact mass and pathway mapping, or on literature; *, °, +: several possibilities for 1 m/z feature based on exact mass and pathway mapping.
The assessment of the classification accuracy in discriminating between the allergic asthmatic and the healthy samples resulted in an area under the curve (AUC) of 0.83, 95% CI: 0.73–0.92, (Figure 4A; Supplementary Table S3; Supplementary Figure S2). When examining feature selection by the Boruta scheme (Kursa et al., 2010) within cross-validation, 57 (±8) m/z features were selected on average in each cross-validation iteration, many of which were putatively identified with the compound identification workflow above (Figure 4B). Compounds which were most frequently selected in LOOCV are presented in Figure 4C (for box plots see Supplementary Figure S2) and all the other selected metabolites can be found in Supplementary Table S4.
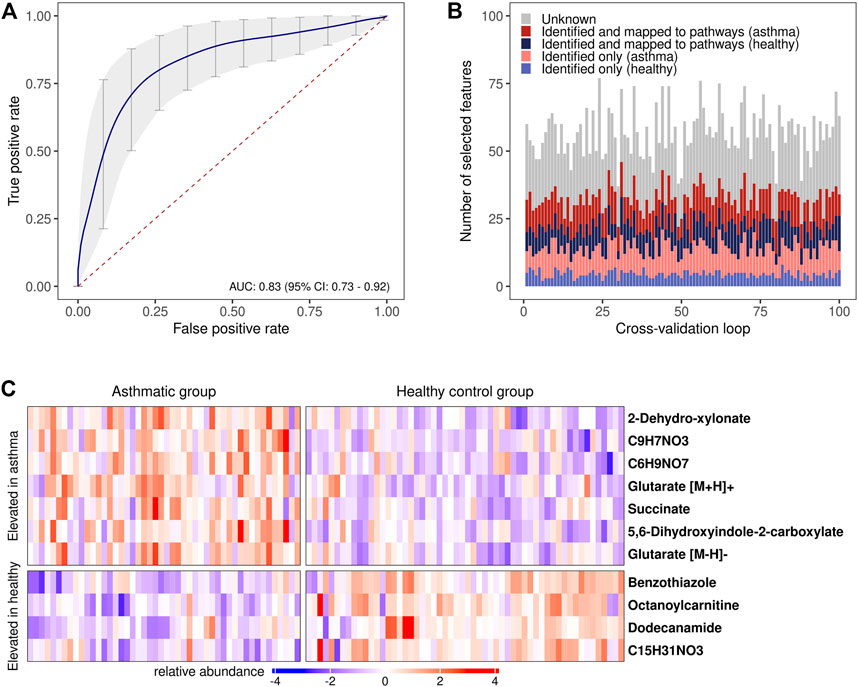
FIGURE 4. Disease prediction based on breath profiles. (A) Average receiver operating characteristic curve (ROC) with an average AUC of 0.83 resulting from the 10 times repeated 10-fold cross-validation. ROC curves resulting from predictions on each of the left-out data sets in the cross-validation were used to calculate the average ROC curve (Fawcett, 2006) (vertical averaging). Vertical grey bars: pointwise confidence intervals computed using bootstrapping (10.000 repetitions); red dashed line: line of no discrimination. (B) Stacked bar plots of the selected features in each cross-validation iteration. Red/blue color scheme: upregulated features in the allergic asthmatic/healthy group. (C) Heat map of the most frequently chosen features (standardized intensities) as predictors in the cross-validation. Columns: study participants; rows: m/z features with putatively identified compounds (right), a chemical formula is provided if compound identification was not possible.
It is of relevance to note that the adjustment with SVA captures the components of variability within the data and reduces any effect on the intensity levels of m/z features arising from other sources than the primary variables of interest (i.e., allergic asthma vs. healthy controls). Hence, any further subgroup analysis or correlation analysis to other clinical parameters could not be performed (Leek and Storey, 2007) Nevertheless, we decided to assess whether atopy by itself has an impact on the breath profiles by isolating the group of healthy samples and repeating our analysis pipeline to find differences between the healthy children with sensitization and the ones without. We found no significantly different features between the two groups (see Supplementary Figure S5).
4 Discussion
We present the first online breath analysis study performed by SESI/HRMS on a pediatric population with allergic asthma. The study revealed group-specific breath patterns with a large number of discriminative m/z features, many of which were putatively identified and could be grouped to metabolic pathways or chemical families. Moreover, some of the relevant compounds and pathways were previously published in metabolomic studies in pediatric asthma (Neerincx et al., 2017; Ferraro et al., 2018; Papamichael et al., 2021) or reported in SESI/HRMS studies (see Supplementary Table S2).
As described by Papamichael et al., an altered energy metabolism is expected in children with asthma due to the hypoxic environment, bronchoconstriction, and other associated changes as well as increased efforts for breathing (Papamichael et al., 2021). However, this explanation might not apply to the included asthmatic group of our study, as they did not suffer from acute exacerbations. The lung and gut microbiomes are also potential contributors to the pathophysiology of asthma (Barcik et al., 2020). Several previous breath analysis studies identified compounds and molecular pathways associated with pediatric asthma. However, some of the potential biomarkers were of exogenous origin and only a handful of them were consistently detected in more than one study (Neerincx et al., 2017; Ferraro et al., 2018; Papamichael et al., 2021). The pathways and chemical families identified in our study are biologically relevant and reflect both an altered state of energy metabolism as well as changes in products from the microbiome.
The metabolism of lysine was the most significantly elevated pathway in asthma and all associated compounds were identified based on direct MS2 spectra. Two different degradation pathways of lysine were found, one is taking place in humans and the other in the gut microbiota (Figure 2). The associated metabolites succinate and glutarate were unambiguously identified (ID1, see Table 2), and have been reported as associated with pediatric asthma in previous metabolomic studies in blood (Chang et al., 2015), urine (Saude et al., 2011), and breath (Carraro et al., 2018). Carraro et al. also reported a decreased level of oxoadipate in early asthma, which is not in line with our findings but could be explained by the different study design focusing on wheezing in preschool children (Carraro et al., 2018). However, a study linked an enzymatic complex involved in the lysine degradation pathway to the formation of reactive oxygen species from 2-oxoadipate (Jordan et al., 2019), which could potentially be a link to asthma pathophysiology.
Tyrosine metabolism was also significantly upregulated in the allergic asthmatic group. As illustrated in Figure 2, some of the metabolites belong to the main human degradation pathway whereas other tyrosine-derived metabolites are of human or microbiotic origin. An increased level of tyrosine in asthmatic children was reported in previous metabolomics studies (Saude et al., 2011; Papamichael et al., 2019; Tao et al., 2019). Additionally, the bacterial tyrosine metabolite 4-hydroxyphenylacetate was reported to be negatively correlated with the FEV1 in urine (Papamichael et al., 2019). It is hypothesized that high levels of tyrosine metabolism might be related to inflammation and oxidative stress in asthma (Papamichael et al., 2021). Also, tyrosine-derived catecholamines are important during conditions of stress and play a role in the regulation of the immune system (Barnes et al., 2015). In contrast to these findings, Carraro et al. reported a lower level of some tyrosine metabolites in children with early asthma compared to transient wheezers (Carraro et al., 2018).
The largest elevated group consisted of 20 fatty acid metabolites, including saturated and unsaturated dicarboxylic acids, ω-oxo-acids, hydroxy-acids, and alkanoic acids. All lysine metabolites are additionally fitting into this chemical family. A large part of these identified fatty acids were previously reported being decreased in chronic obstructive pulmonary disease exacerbations by SESI/HRMS and described as metabolites of the ω-oxidation, a minor pathway of the fatty acid oxidation (Gaugg et al., 2017a; 2019). Interestingly, important molecules in asthma pathophysiology including arachidonic acid, leukotrienes, and prostaglandins, although not detected in this study, are also common substrates of the cytochrome P450 ω-hydroxylases (Ni and Liu, 2021). Therefore, our findings support the hypothesis that ω-oxidation might be upregulated in allergic asthma. Butanoic and pentanoic acid are both identified (ID2, Supplementary Table S2) and were both reported to distinguish asthmatic from healthy children in previous studies (Dallinga et al., 2010; van Mastrigt et al., 2016; van Vliet et al., 2016). Furthermore, the findings from previous metabolomic studies of an altered fatty acid metabolism in asthma is supported by our data (Neerincx et al., 2017; Ferraro et al., 2018; Papamichael et al., 2021).
Further, the 2-oxocarboxylic acid metabolism was also elevated in the allergic asthmatic (Figure 2). 2-Oxoadipate and 2-aminoadipate are overlapping with the lysine degradation pathway and glutamate is a common metabolite involved in multiple metabolic pathways. The metabolism of 2-oxocarboxylic acids is solely happening in archaea, which are also represented in the gut microbiota. A review linked methanogenic archaea as potential important contributors to atopic diseases (Sereme et al., 2019). Additionally, 2-oxoadipate was previously detected as an exhaled metabolite from the gut microbiota in a mice model study (Lan et al., 2022).
Lastly, monosaccharides and derived metabolites were increased in allergic asthma. This difference in carbohydrate metabolism of asthmatic children is expected due to an altered energy demand and metabolism (Papamichael et al., 2021).
A recently published study comparing children with acute asthma exacerbations and healthy controls reported similar results to ours. Despite investigating urine by high-performance liquid chromatography mass spectrometry, they also reported an elevated level of tyrosine metabolism including gentisate and increased glucuronate as well as a downregulated linoleic acid metabolite and palmitic acid in children with acute asthma (Li et al., 2022).
The most prominent group of downregulated metabolites was associated with arginine and proline metabolism as well as arginine biosynthesis. Arginase, an enzyme that converts arginine into ornithine and urea is an important contributor to asthma pathophysiology (Maarsingh et al., 2011). According to Maarsingh et al., increased expression and activity of arginase in asthma mouse models resulted in the promotion of inflammatory processes, decreased arginine and increased ornithine and proline levels (Maarsingh et al., 2011). This is not completely in line with our findings where these downstream pathways are decreased in allergic asthma. However, a study about amino acids in blood serum of asthmatics reported decreased arginine, proline and ornithine levels (Morris et al., 2004).
Further, the linoleic acid metabolism was well-represented amongst the diminished compounds. While conjugated linoleic acid was consistently reported as having anti-inflammatory properties, the effect of linoleic acid especially on asthma is in dispute due to controversial observations in clinical trials (Wendell et al., 2014). Interestingly, a more recent study found genetically predicted linoleic acid to be associated with a lower risk for asthma, which is in line with our results (Zhao and Schooling, 2019).
Within the group of amides, palmitoylethanolamide (PEA) was found to be decreased in the allergic asthma group. This is in line with the well-studied anti-inflammatory effect of PEA (Clayton et al., 2021). More recently, also an inhibitory effect for the development of allergic airway symptoms was reported for PEA in mice (Roviezzo et al., 2017).
Another group of downregulated compounds was assigned to aldehydes. Aldehydes are indicative of oxidative stress and originate from lipid peroxidation (Jesenak et al., 2017), which is involved in asthma pathophysiology, and are thus expected to be increased in asthmatics. However, the results in literature on breath analysis in asthma are not consistent: Some studies observed an increased level of certain aldehydes in the asthmatic group (Gahleitner et al., 2013; van de Kant et al., 2013; Smolinska et al., 2014), whereas others reported unaltered or even decreased levels (Ibrahim et al., 2011; Sagdic et al., 2011; Caldeira et al., 2012; Riscassi et al., 2022). Beyond this, aldehydes are also used as common additives in cosmetics or food and are known environmental contaminants (Sinharoy et al., 2019), which could influence their exhaled concentrations. Furthermore, the annotation of aldehydes in our study was based solely on exact mass matches with previously published compounds by SESI/HRMS (see Supplementary Table S2).
Altogether, many of the enriched pathways that we reported either elevated or decreased in allergic asthma could be linked to previous findings of metabolomic studies using various methods for blood, urine, or breath analysis. This strengthens the putative compound identification performed in this work and supports the possible biological and diagnostic value of these metabolites.
Assessing the predictability of the disease with supervised machine learning in a 10 times repeated 10-fold cross-validation revealed an AUC of 0.83 (CI: 0.73–0.92), indicating that the metabolic profiles could be applied for potential diagnostic purposes. Some compounds that were allocated to subgroups of metabolic pathways or chemical families were frequently selected during cross-validation (Figure 4C; Supplementary Table S4) suggesting that a smaller group of compounds might not only be pathophysiologically relevant, but also has potential for diagnostic models. The two dicarboxylic acids and lysine metabolites, succinate and glutarate, are promising candidates and were unambiguously identified. Nevertheless, while efforts have been taken to prevent bias by preprocessing data in each cross-validation loop and reducing the dimensionality of the feature set for training the classifier with machine learning, the risk of overfitting cannot be completely ruled out (Vabalas et al., 2019). An independent and increased study cohort would be needed to help in validating the model performance and the selected predictors (Fijten et al., 2017).
Due to a rather large number of significant m/z features, a main focus was set on putative compound identification. We aimed at establishing an objective workflow that is based on matching direct MS2 spectra with database fragment spectra, adapted from previous work (Kaeslin et al., 2021), refined for a more extensive screening of suggested compounds, and expanded by pathway enrichment analysis to strengthen the feature annotation. A limitation in our identification approach is the lacking chromatographic separation in SESI/HRMS, which hinders the distinction of isomeric compounds. Further, with a minimum isolation window of 0.7 Da, co-fragmentation of several compounds with similar masses can occur, which complicates the annotation of fragment spectra. To address this, we excluded several MS2 spectra with insufficient quality from further analysis, as specified in Supplementary Table S1. The confidence of identification is only moderate for most compounds, as they are putatively annotated based on fragment spectra analysis with the SIRIUS software that uses computational power to determine chemical structures that potentially have a matching fragmentation pattern (Dührkop et al., 2019) or the exact mass comparison to the literature and/or known metabolites. Therefore, there is a general risk for misclassification, and the confirmation of the unambiguous chemical structures requires further time-consuming experiments, including the measurement of standards. Especially, the molecules that were annotated based on their exact mass or solely detected as adduct or loss species without their primary ion being amongst the significant features need further investigation. However, our aim for this study was to get a broad overview over the potentially involved metabolic pathways rather than accurately identifying a small set of single compounds.
A strength of this study design is that all enrolled patients were taken off long-term therapy at least 1 week prior to the study visit and did not take any short-acting relievers on the day of measurement. While direct breath analysis by SESI bypasses any contamination during sample preparation, this adds up to also diminish confounders and signal interferences from medications in exhaled breath. This is an important aspect, as it was previously shown that the methodology can detect drugs, including the asthma medication Salbutamol, in breath (Gaugg et al., 2017b; Chen et al., 2021; Singh et al., 2021).
While asthma is a heterogeneous disease with different phenotypes, this study focused only on allergic asthma, the most frequent phenotype in children. Therefore, our findings cannot be extrapolated to all forms of pediatric asthma. We included all sensitized healthy controls and all asthmatics with allergic comorbidities such as allergic eczema or hay fever in order to represent the real population for future applications. 21.4% of the healthy cohort had an allergic sensitization to at least one common aeroallergen, which is in line with the estimated prevalence in children (Kölli et al., 2022). However, performing subgroup analysis on the entire data set was not feasible since surrogate variable analysis is known to adjust for confounding influences and reduce heterogeneity in the data (e.g., demographic variations like age and sex, or disease heterogeneity) (Jaffe et al., 2015). We decided to apply SVA to adjust for unmodeled factors, since the study was conducted over a period of 15 months on a highly sensitive instrument. Therefore, despite a strict adherence to standard operating procedures, we had to assume that apart from demographic variation also unknown environmental or technical confounders might have impacted the m/z feature intensities. In order to nevertheless assess the interesting question whether the described markers and pathways might also be related to atopy by itself, we chose to perform an independent subgroup analysis in the healthy cohort. No significant features that could distinguish healthy children with allergic sensitization from the ones without could be found. Therefore, the identified metabolites and pathways represent promising candidate biomarkers for allergic asthma that need to be validated in a larger and independent study cohort.
This study confirms the applicability of SESI/HRMS to a pediatric population and shows its potential to distinguish children with allergic asthma from healthy controls based on their breath signatures. Moreover, well-represented metabolic pathways that are potentially linked to the pathophysiology of allergic asthma in children could be identified. A smaller subset of the differentiating compounds could possibly be used for predictive modelling. These findings might set the path for much-needed, non-invasive clinical applications to improve early diagnosis of asthma.
Data availability statement
TThe MS2 data generated for this study can be found in the FigShare repository at doi: 10.6084/m9.figshare.21946877. Due to ethical restrictions, other data is available upon request from the authors.
Ethics statement
The studies involving human participants were reviewed and approved by Ethics committe of the canton of Zurich (Kantonale Ethikkommission Kanton Zürich). Written informed consent to participate in this study was provided by the participants’ legal guardian/next of kin.
Author contributions
Study design and concept: AM; Data acquisition: BS, RW, LW, and DI; Data processing and analysis: SM, BS, and RW; Data evaluation and interpretation: AM, SM, BS, and RW; Drafting of the manuscript: AM, SM, BS, and RW; Funding acquisition: AM, RZ, and MK; Resources: AM, RZ, MK, and NP; Review and editing of the manuscript: all authors.
Funding
This work was supported by the Swiss National Science Foundation (SNSF) [grant number 326030_177101/1]; the Evi Diethelm-Winteler Foundation; the Childhood Research Center of the University Children’s Hospital Zurich; the Heidi Ras Stiftung; the Zurich Foundation; and the Lotte and Adolf Hotz-Sprenger Stiftung.
Acknowledgments
We are very grateful to all the participants for participating in this study. We further like to thank the lung function team at the Children’s University Hospital Zurich for their support and Jérôme Kaeslin, ETH Zurich, for the helpful discussions on compound identification. This work is part of Zurich Exhalomics, a flagship project of “Hochschulmedizin Zürich”.
Conflict of interest
MK is a founder and board member of Deep Breath Intelligence AG (www.dbi.ch), a company that provides services in the field of breath analysis. RZ is advisor to Deep Breath Intelligence AG.
Publisher’s note
All claims expressed in this article are solely those of the authors and do not necessarily represent those of their affiliated organizations, or those of the publisher, the editors and the reviewers. Any product that may be evaluated in this article, or claim that may be made by its manufacturer, is not guaranteed or endorsed by the publisher.
Supplementary material
The Supplementary Material for this article can be found online at: https://www.frontiersin.org/articles/10.3389/fmolb.2023.1154536/full#supplementary-material
References
Barcik, W., Boutin, R. C. T., Sokolowska, M., and Finlay, B. B. (2020). The role of lung and gut microbiota in the pathology of asthma. Immunity 52, 241–255. doi:10.1016/j.immuni.2020.01.007
Barnes, M. A., Carson, M. J., and Nair, M. G. (2015). Non-traditional cytokines: How catecholamines and adipokines influence macrophages in immunity, metabolism and the central nervous system. Cytokine 72, 210–219. doi:10.1016/j.cyto.2015.01.008
Benjamini, Y., and Hochberg, Y. (1995). Controlling the false discovery rate: A practical and powerful approach to multiple testing. J. R. Stat. Soc. Ser. B Stat. Methodol. 57, 289–300. doi:10.1111/j.2517-6161.1995.tb02031.x
Bruderer, T., Gaisl, T., Gaugg, M. T., Nowak, N., Streckenbach, B., Müller, S., et al. (2019). On-line analysis of exhaled breath focus review. Chem. Rev. 119, 10803–10828. doi:10.1021/acs.chemrev.9b00005
Caldeira, M., Perestrelo, R., Barros, A. S., Bilelo, M. J., Morête, A., Câmara, J. S., et al. (2012). Allergic asthma exhaled breath metabolome: A challenge for comprehensive two-dimensional gas chromatography. J. Chromatogr. A 1254, 87–97. doi:10.1016/j.chroma.2012.07.023
Carraro, S., Bozzetto, S., Giordano, G., el Mazloum, D., Stocchero, M., Pirillo, P., et al. (2018). Wheezing preschool children with early-onset asthma reveal a specific metabolomic profile. Pediatr. Allergy Immunol. 29, 375–382. doi:10.1111/pai.12879
Chang, C., Guo, Z., He, B., and Yao, W. (2015). Metabolic alterations in the sera of Chinese patients with mild persistent asthma: A GC-MS-based metabolomics analysis. Acta. Pharmacol. Sin. 36, 1356–1366. doi:10.1038/aps.2015.102
Chen, X., Zhang, K., Yin, Z., Fang, M., Pu, W., Liu, Z., et al. (2021). Online real-time monitoring of exhaled breath particles reveals unnoticed transport of nonvolatile drugs from blood to breath. Anal. Chem. 93, 5005–5008. doi:10.1021/acs.analchem.1c00509
Clayton, P., Hill, M., Bogoda, N., Subah, S., and Venkatesh, R. (2021). Palmitoylethanolamide: A natural compound for health management. Int. J. Mol. Sci. 22, 5305. doi:10.3390/ijms22105305
Dallinga, J. W., Robroeks, C. M. H. H. T., van Berkel, J. J. B. N., Moonen, E. J. C., Godschalk, R. W. L., Jöbsis, Q., et al. (2010). Volatile organic compounds in exhaled breath as a diagnostic tool for asthma in children. Clin. Exp. Allergy 40, 68–76. doi:10.1111/j.1365-2222.2009.03343.x
Dührkop, K., Fleischauer, M., Ludwig, M., Aksenov, A. A., Melnik, A., Meusel, M., et al. (2019). Sirius 4: A rapid tool for turning tandem mass spectra into metabolite structure information. Nat. Methods. 16, 299–302. doi:10.1038/s41592-019-0344-8
Fawcett, T. (2006). An introduction to ROC analysis. Pattern. Recognit. Lett. 27, 861–874. doi:10.1016/j.patrec.2005.10.010
Ferraro, V., Carraro, S., Bozzetto, S., Zanconato, S., and Baraldi, E. (2018). Exhaled biomarkers in childhood asthma: Old and new approaches. Asthma. Res. Pract. 4, 9. doi:10.1186/s40733-018-0045-6
Fijten, R. R. R., Smolinska, A., Drent, M., Dallinga, J. W., Mostard, R., Pachen, D. M., et al. (2017). The necessity of external validation in exhaled breath research: A case study of sarcoidosis. J. Breath. Res. 12, 016004. doi:10.1088/1752-7163/aa8409
Gahleitner, F., Guallar-Hoyas, C., Beardsmore, C. S., Pandya, H. C., and Thomas, C. L. P. (2013). Metabolomics pilot study to identify volatile organic compound markers of childhood asthma in exhaled breath. Bioanalysis 5, 2239–2247. doi:10.4155/bio.13.184
Gaillard, E. A., Kuehni, C. E., Turner, S., Goutaki, M., Holden, K. A., de Jong, C. C. M., et al. (2021). European Respiratory Society clinical practice guidelines for the diagnosis of asthma in children aged 5–16 years. Eur. Respir. J. 58, 2004173. doi:10.1183/13993003.04173-2020
Gaugg, M. T., Bruderer, T., Nowak, N., Eiffert, L., Martinez-Lozano Sinues, P., Kohler, M., et al. (2017a). Mass-spectrometric detection of omega-oxidation products of aliphatic fatty acids in exhaled breath. Anal. Chem. 89, 10329–10334. doi:10.1021/acs.analchem.7b02092
Gaugg, M. T., Engler, A., Nussbaumer-Ochsner, Y., Bregy, L., Stöberl, A. S., Gaisl, T., et al. (2017b). Metabolic effects of inhaled salbutamol determined by exhaled breath analysis. J. Breath. Res. 11, 046004. doi:10.1088/1752-7163/aa7caa
Gaugg, M. T., Gomez, D. G., Barrios-Collado, C., Vidal-de-Miguel, G., Kohler, M., Zenobi, R., et al. (2016). Expanding metabolite coverage of real-time breath analysis by coupling a universal secondary electrospray ionization source and high resolution mass spectrometry—A pilot study on tobacco smokers. J. Breath. Res. 10, 016010. doi:10.1088/1752-7155/10/1/016010
Gaugg, M. T., Nussbaumer-Ochsner, Y., Bregy, L., Engler, A., Stebler, N., Gaisl, T., et al. (2019). Real-time breath analysis reveals specific metabolic signatures of COPD exacerbations. Chest 156, 269–276. doi:10.1016/j.chest.2018.12.023
Ibrahim, B., Basanta, M., Cadden, P., Singh, D., Douce, D., Woodcock, A., et al. (2011). Non-invasive phenotyping using exhaled volatile organic compounds in asthma. Thorax 66, 804–809. doi:10.1136/thx.2010.156695
Jaffe, A. E., Hyde, T., Kleinman, J., Weinbergern, D. R., Chenoweth, J. G., McKay, R. D., et al. (2015). Practical impacts of genomic data “cleaning” on biological discovery using surrogate variable analysis. BMC Bioinform 16, 372. doi:10.1186/s12859-015-0808-5
Jesenak, M., Zelieskova, M., and Babusikova, E. (2017). Oxidative stress and bronchial asthma in children—causes or consequences? Front. Pediatr. 5, 162. doi:10.3389/fped.2017.00162
Jordan, F., Nemeria, N., and Gerfen, G. (2019). Human 2-oxoglutarate dehydrogenase and 2-oxoadipate dehydrogenase both generate superoxide/H2O2 in a side reaction and each could contribute to oxidative stress in mitochondria. Neurochem. Res. 44, 2325–2335. doi:10.1007/s11064-019-02765-w
Kaeslin, J., Micic, S., Weber, R., Müller, S., Perkins, N., Berger, C., et al. (2021). Differentiation of cystic fibrosis-related pathogens by volatile organic compound analysis with secondary electrospray ionization mass spectrometry. Metabolites 11, 773. doi:10.3390/metabo11110773
Kaur, B., Anderson, H. R., Austin, J., Burr, M., Harkins, L. S., Strachan, D. P., et al. (1998). Prevalence of asthma symptoms, diagnosis, and treatment in 12-14 year old children across Great Britain (international study of asthma and allergies in childhood, ISAAC UK). BMJ 316, 118–124. doi:10.1136/bmj.316.7125.118
Kölli, F., Breyer, M.-K., Hartl, S., Burghuber, O., Wouters, E. F. M., Sigsgaard, T., et al. (2022). Aero-allergen sensitization in the general population: Longitudinal analyses of the LEAD (lung heart social body) study. J. Asthma Allergy 15, 461–473. doi:10.2147/JAA.S349614
Kursa, M. B., Jankowski, A., and Rudnicki, W. R. (2010). Boruta – a system for feature selection. Fundam. Inf. 101, 271–285. doi:10.3233/FI-2010-288
Lan, J., Greter, G., Streckenbach, B., Arnoldini, M., Zenobi, R., and Slack, E. (2022). Non-invasive monitoring of microbiota and host metabolism using Secondary electrospray ionization-Mass spectrometry. bioRxiv 2022, 493434. doi:10.1101/2022.05.25.493434
Leek, J. T., and Storey, J. D. (2007). Capturing heterogeneity in gene expression studies by surrogate variable analysis. PLoS Genet. 3, 1724–1735. doi:10.1371/journal.pgen.0030161
Li, J., Li, X., Liu, X., Wang, X., Li, J., Lin, K., et al. (2022). Untargeted metabolomic study of acute exacerbation of pediatric asthma via HPLC-Q-Orbitrap-MS. J. Pharm. Biomed. Anal. 215, 114737. doi:10.1016/j.jpba.2022.114737
Looijmans-van den Akker, I., van Luijn, K., and Verheij, T. (2016). Overdiagnosis of asthma in children in primary care: A retrospective analysis. Br. J. Gen. Pract. 66, e152–e157. doi:10.3399/bjgp16X683965
Maarsingh, H., Dekkers, B. G. J., Zuidhof, A. B., Bos, I. S. T., Menzen, M. H., Klein, T., et al. (2011). Increased arginase activity contributes to airway remodelling in chronic allergic asthma. Eur. Respir. J. 38, 318–328. doi:10.1183/09031936.00057710
Morris, C. R., Poljakovic, M., Lavrisha, L., Machado, L., Kuypers, F. A., and Morris, S. M. (2004). Decreased arginine bioavailability and increased serum arginase activity in asthma. Am. J. Respir. Crit. Care. Med. 170, 148–153. doi:10.1164/rccm.200309-1304OC
Neerincx, A. H., Vijverberg, S. J. H., Bos, L. D. J., Brinkman, P., van der Schee, M. P., de Vries, R., et al. (2017). Breathomics from exhaled volatile organic compounds in pediatric asthma. Pediatr. Pulmonol. 52, 1616–1627. doi:10.1002/ppul.23785
Ni, K. di, and Liu, J. Y. (2021). The functions of cytochrome P450 ω-hydroxylases and the associated eicosanoids in inflammation-related diseases. Front. Pharmacol. 12, 716801. doi:10.3389/fphar.2021.716801
Pang, Z., Chong, J., Zhou, G., de Lima Morais, D. A., Chang, L., Barrette, M., et al. (2021). MetaboAnalyst 5.0: Narrowing the gap between raw spectra and functional insights. Nucleic Acids Res. 49, W388–W396. doi:10.1093/nar/gkab382
Papamichael, M. M., Katsardis, C., Erbas, B., Itsiopoulos, C., and Tsoukalas, D. (2019). Urinary organic acids as biomarkers in the assessment of pulmonary function in children with asthma. Nutr. Res. 61, 31–40. doi:10.1016/j.nutres.2018.10.004
Papamichael, M. M., Katsardis, C., Sarandi, E., Georgaki, S., Frima, E.-S., Varvarigou, A., et al. (2021). Application of metabolomics in pediatric asthma: Prediction, diagnosis and personalized treatment. Metabolites 11, 251. doi:10.3390/metabo11040251
Riscassi, S., Corradi, M., Andreoli, R., Maccari, C., Mercolini, F., Pescollderungg, L., et al. (2022). Nitric oxide products and aldehydes in exhaled breath condensate in children with asthma. Clin. Exp. Allergy 52, 561–564. doi:10.1111/cea.14066
Ritchie, M. E., Phipson, B., Wu, D., Hu, Y., Law, C. W., Shi, W., et al. (2015). Limma powers differential expression analyses for RNA-sequencing and microarray studies. Nucleic Acids Res. 43, e47. doi:10.1093/nar/gkv007
Roviezzo, F., Rossi, A., Caiazzo, E., Orlando, P., Riemma, M. A., Iacono, V. M., et al. (2017). Palmitoylethanolamide supplementation during sensitization prevents airway allergic symptoms in the mouse. Front. Pharmacol. 8, 857. doi:10.3389/fphar.2017.00857
Sagdic, A., Sener, O., Bulucu, F., Karadurmus, N., Özel, H. E., Yamanel, L., et al. (2011). Oxidative stress status and plasma trace elements in patients with asthma or allergic rhinitis. Allergol. Immunopathol. Madr. 39, 200–205. doi:10.1016/j.aller.2010.07.006
Saude, E. J., Skappak, C. D., Regush, S., Cook, K., Ben-Zvi, A., Becker, A., et al. (2011). Metabolomic profiling of asthma: Diagnostic utility of urine nuclear magnetic resonance spectroscopy. J. Allergy Clin. Immunol. 127, 757–764.e1-6. doi:10.1016/j.jaci.2010.12.1077
Schwarz, E. I., Martinez-Lozano Sinues, P., Bregy, L., Gaisl, T., Garcia Gomez, D., Gaugg, M. T., et al. (2016). Effects of CPAP therapy withdrawal on exhaled breath pattern in obstructive sleep apnoea. Thorax 71, 110–117. doi:10.1136/thoraxjnl-2015-207597
Schymanski, E. L., Jeon, J., Gulde, R., Fenner, K., Ruff, M., Singer, H. P., et al. (2014). Identifying small molecules via high resolution mass spectrometry: Communicating confidence. Environ. Sci. Technol. 48, 2097–2098. doi:10.1021/es5002105
Sereme, Y., Mezouar, S., Grine, G., Mege, J. L., Drancourt, M., Corbeau, P., et al. (2019). Methanogenic archaea: Emerging partners in the field of allergic diseases. Clin. Rev. Allergy Immunol. 57, 456–466. doi:10.1007/s12016-019-08766-5
Siersted, H. C., Hansen, H. S., Mostgaard, G., Hyldebrandt, N., Rees, P. J., et al. (1998). Population based study of risk factors for underdiagnosis of asthma in adolescence: Odense schoolchild study. BMJ 316, 651–657. doi:10.1136/bmj.316.7132.651
Singh, K. D., Osswald, M., Ziesenitz, V. C., Awchi, M., Usemann, J., Imbach, L. L., et al. (2021). Personalised therapeutic management of epileptic patients guided by pathway-driven breath metabolomics. Commun. Med. 1, 21. doi:10.1038/s43856-021-00021-3
Sinharoy, P., McAllister, S. L., Vasu, M., and Gross, E. R. (2019). “Environmental aldehyde sources and the health implications of exposure,” in Aldehyde dehydrogenases: From alcohol metabolism to human health and precision medicine. Editors J. Ren, Y. Zhang, and J. Ge (Singapore: Springer Singapore), 35–52. doi:10.1007/978-981-13-6260-6_2
Smolinska, A., Klaassen, E. M. M., Dallinga, J. W., van de Kant, K. D. G., Jobsis, Q., Moonen, E. J. C., et al. (2014). Profiling of volatile organic compounds in exhaled breath as a strategy to find early predictive signatures of asthma in children. PLoS One 9, e95668. doi:10.1371/journal.pone.0095668
Smyth, G. K. (2004). Linear models and empirical Bayes methods for assessing differential expression in microarray experiments. Stat. Appl. Genet. Mol. Biol. 3, 1027. doi:10.2202/1544-6115.1027
Tao, J. L., Chen, Y. Z., Dai, Q. G., Tian, M., Wang, S. C., Shan, J.-J., et al. (2019). Urine metabolic profiles in paediatric asthma. Respirology 24, 572–581. doi:10.1111/resp.13479
Vabalas, A., Gowen, E., Poliakoff, E., and Casson, A. J. (2019). Machine learning algorithm validation with a limited sample size. PLoS One 14, e0224365. doi:10.1371/journal.pone.0224365
van de Kant, K. D. G., van Berkel, J. J. B. N., Jöbsis, Q., Lima Passos, V., Klaassen, E. M. M., van der Sande, L., et al. (2013). Exhaled breath profiling in diagnosing wheezy preschool children. Eur. Respir. J. 41, 183–188. doi:10.1183/09031936.00122411
van Gent, R., van Essen, L. E. M., Rovers, M. M., Kimpen, J. L. L., van der Ent, C. K., and de Meer, G. (2007). Quality of life in children with undiagnosed and diagnosed asthma. Eur. J. Pediatr. 166, 843–848. doi:10.1007/s00431-006-0358-y
van Mastrigt, E., Reyes-Reyes, A., Brand, K., Bhattacharya, N., Urbach, H. P., Stubbs, A. P., et al. (2016). Exhaled breath profiling using broadband quantum cascade laser-based spectroscopy in healthy children and children with asthma and cystic fibrosis. J. Breath. Res. 10, 026003. doi:10.1088/1752-7155/10/2/026003
van Vliet, D., Smolinska, A., Jöbsis, Q., Rosias, P. P. R., Muris, J. W. M., Dallinga, J. W., et al. (2016). Association between exhaled inflammatory markers and asthma control in children. J. Breath. Res. 10, 016014. doi:10.1088/1752-7155/10/1/016014
Varma, S., and Simon, R. (2006). Bias in error estimation when using cross-validation for model selection. BMC Bioinform 7, 91. doi:10.1186/1471-2105-7-91
Weber, R., Haas, N., Baghdasaryan, A., Bruderer, T., Inci, D., Micic, S., et al. (2020). Volatile organic compound breath signatures of children with cystic fibrosis by real-time SESI-HRMS. ERJ Open Res. 6, 00171–02019. doi:10.1183/23120541.00171-2019
Wendell, S. G., Baffi, C., and Holguin, F. (2014). Fatty acids, inflammation, and asthma. J. Allergy Clin. Immunol. 133, 1255–1264. doi:10.1016/j.jaci.2013.12.1087
Yang, C. L., Simons, E., Foty, R. G., Subbarao, P., To, T., and Dell, S. D. (2017). Misdiagnosis of asthma in schoolchildren. Pediatr. Pulmonol. 52, 293–302. doi:10.1002/ppul.23541
Keywords: volatile organic compounds (VOCs), metabolites, allergic asthma, children, breath analysis, SESI/HRMS
Citation: Weber R, Streckenbach B, Welti L, Inci D, Kohler M, Perkins N, Zenobi R, Micic S and Moeller A (2023) Online breath analysis with SESI/HRMS for metabolic signatures in children with allergic asthma. Front. Mol. Biosci. 10:1154536. doi: 10.3389/fmolb.2023.1154536
Received: 30 January 2023; Accepted: 16 March 2023;
Published: 31 March 2023.
Edited by:
Andras Szeitz, University of British Columbia, CanadaReviewed by:
Sven Schuchardt, Fraunhofer Institute for Toxicology and Experimental Medicine (FHG), GermanyMario Humberto Vargas, National Institute of Respiratory Diseases-Mexico (INER), Mexico
Copyright © 2023 Weber, Streckenbach, Welti, Inci, Kohler, Perkins, Zenobi, Micic and Moeller. This is an open-access article distributed under the terms of the Creative Commons Attribution License (CC BY). The use, distribution or reproduction in other forums is permitted, provided the original author(s) and the copyright owner(s) are credited and that the original publication in this journal is cited, in accordance with accepted academic practice. No use, distribution or reproduction is permitted which does not comply with these terms.
*Correspondence: Alexander Moeller, YWxleGFuZGVyLm1vZWxsZXJAa2lzcGkudXpoLmNo
†These authors have contributed equally to this work and share first authorship
‡These authors have contributed equally to this work and share last authorship