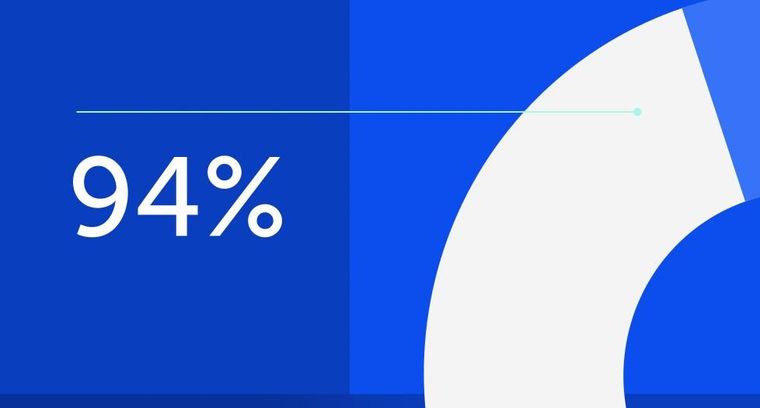
94% of researchers rate our articles as excellent or good
Learn more about the work of our research integrity team to safeguard the quality of each article we publish.
Find out more
EDITORIAL article
Front. Mol. Biosci., 17 January 2023
Sec. Molecular Recognition
Volume 10 - 2023 | https://doi.org/10.3389/fmolb.2023.1139471
This article is part of the Research TopicMechanisms, Thermodynamics and Kinetics of Ligand Binding Revealed from Molecular Simulations and Machine LearningView all 16 articles
Editorial on the Research Topic
Mechanisms, thermodynamics and kinetics of ligand binding revealed from molecular simulations and machine learning
Ligand binding plays an essential role in cellular signaling. Detailed understanding of the mechanisms, structures, thermodynamics and kinetics of ligand binding is central to drug discovery in the pharmaceutical industry and academia (Baron and McCammon, 2013; Peng et al., 2019). Despite this critical importance, such tasks remain challenging in computational chemistry and biophysics. Molecular docking has proven useful in rapid virtual screening of small molecules for drug discovery, although it is often difficult to fully incorporate receptor flexibility into the docking calculations. Recent developments in computing hardware and simulation algorithms have enabled molecular dynamics (MD) simulations to capture dynamic ligand binding and dissociation processes. These simulations can then be analyzed to compute both thermodynamic free energies and kinetic rates of ligand binding (Pang and Zhou, 2017; Tang et al., 2017; Nunes-Alves et al., 2020; Wang et al., 2022). In addition, Brownian dynamics simulations have been very efficient in generating a large number of ligand binding trajectories and estimating the binding kinetic rates (Huber and McCammon, 2019; Muñiz-Chicharro et al., 2022). Finally, emerging machine learning techniques have greatly enhanced molecular simulations and facilitated analysis of the simulation trajectories (Glielmo et al., 2021).
This Research Topic is focused on studies of the pathways, mechanisms, free energies and kinetics of ligand binding to target receptors. We encouraged both method development and application papers. Potential techniques used to address these problems include molecular docking, MD, Brownian dynamics, and machine learning approaches. Systems of interest broadly involve ligand binding to any type of receptors, including proteins, nucleic acids, materials, and so on.
Carloni et al. have reviewed recent major advancements in molecular simulation methodologies for predicting dissociation rate (koff), a parameter of fundamental importance in drug design. They further discuss the impact of the potential energy function models on the accuracy of the prediction, and provide a perspective from high-performance computing and machine learning for highly efficient and accurate prediction of the constants. Roussey and Dickson have uncovered important factors of host-guest unbinding through detailed analysis of a large dataset of simulation trajectories. They have found that differences in ion densities as well as guest-ion interactions strongly correlate with differences in the probabilities of reactive paths, and play a significant role in the guest unbinding.
Joshi et al. describe the extension of their clever method using curvilinear coordinate-based sampling to study the thermodynamics of rapamycin associating with the FKBP12 enzyme, the first step in the action of this antiproliferative agent. The method uses a multiple-walker umbrella sampling simulation approach to characterizing the protein–protein interaction energetics along the curvilinear paths, and yields binding free energies and mechanistic details of rapamycin binding with wild-type FKBP12 and modifications of these molecules.
Shinobu et al. have optimized practical protocols for a 2D replica-exchange MD (REMD) method that combines generalized replica exchange with solute tempering and replica-exchange umbrella sampling (gREST/REUS). As demonstrated on ligand binding to three protein kinase systems, the method ensures good random walks in the 2D replica spaces, which are important for enhanced sampling of kinase-inhibitor binding.
Chai et al. have carried out multi-microsecond length MD simulations of STK17B in three different states. They observed the conformational dynamics of its P-loop that could flip into the ADP-binding site upon the inhibitor binding to interact with inhibitors and the protein C-lobe, leading to strengthened communications between the C- and N-lobes. Their simulation results could be useful for designing highly selective inhibitors.
Zhang et al. have carried out MD simulations and Molecular Mechanics/Poisson–Boltzmann Surface Area (MM/PBSA) calculations and revealed stronger binding of rivaroxaban in the Y99C mutant of coagulation factor X than in the Y99A mutant. Their simulations have also shown that ligand binding may not only be a dynamic process but also a dynamic state involving multiple binding poses, which could be important for drug design. Cai et al. have performed MD simulations and absolute binding free energy calculations for exploring the drug resistance mechanism of epidermal growth factor receptor (EGFR), a target protein of many non-small cell lung cancer (NSCLC) drugs. They found the binding affinity of ATP to L858R/T790M mutant is higher than that to the L858R mutant, due to the significant changes of the protein conformation and the van der Waals interactions. Their findings could be valuable for designing new drugs for NSCLC.
Girame et al., Garcia-Borràs and Feixas have applied MD simulations to investigate changes in protonation states of in-pathway residues during protein-ligand binding processes. The authors found that binding of benzamidine to trypsin was infrequent when His57 was positively charged, where His57 was part of the catalytic triad and located more than 10 Å away from the gorge of the substrate binding pocket. Their findings illustrate the importance in properly accounting for protonation states of distal residues when using MD simulations to study ligand binding pathways.
Xue et al. have performed MD simulations on glucocorticoid receptor (GR) complexed with cofactor TIF2 and five different agonists. They have uncovered a communication mechanism between the ligand-binding and cofactor-binding pockets, and identified a pair of important residues (D590 and T739) in the allosteric communication pathway, which could be useful for GR-targeted drug discovery. Shen et al. have examined 130+ RORγ complex structures with different agonists and inverse agonists, identified specific changes in the contact interaction for distinguishing active and inactive conformations, and observed essential modes for separating allosteric binding vs canonical binding and active vs inactive structures. Their simulations and analyses have also revealed some essential contacts to the constitutive activity of RORγ.
Huang et al. have built the most likely 3D structures of alpha/beta hydrolase domain-containing 5 (ABHD5) and the ABHD5-ligand complexes by combining various computational and experimental methods. Their simulations have also identified three residues and some hydrophobic interactions important for protein structure, function and the interactions with ligands and membrane.
Xiao et al. have introduced the Protein Allosteric Sites Server (PASSer2.0), which uses a geometry-based algorithm and automated machine learning to predict allosteric sites. The authors tested a total of 204 proteins from the Allosteric Database (ASD) and ASBench database. The server performed well under multiple indicators. It will provide a valuable tool to facilitate allosteric drug discovery. McKay et al. have developed an essential dynamics ensemble docking (EDED) approach to identify the most relevant receptor conformations for virtual screening. They have demonstrated the approach on docking of small-molecule antagonists of the PAC1 class B GPCR. With four representative receptor models selected from simulations and screening of three million ZINC compounds and 23 experimentally validated ligands of PAC1, they show that EDED can effectively reduce the number of false positives and improve the accuracy of docking.
The paper “Big Data Analytics for Improved Prediction of Ligand Binding and Conformational Selection” by Gupta et al. continues the work by these authors to enhance our understanding of the binding of small molecules to proteins through the conformational selection mechanism. The authors make use of modern machine learning approaches and provide valuable tools for identifying proteins that utilize these mechanisms.
In summary, remarkable advances have been made in both method development and applications in computational predictions of ligand binding free energies and kinetics (especially the dissociation rate). Advanced MD simulations have revealed mechanisms of ligand recognition and associated protein conformational changes, which often involves allosteric modulation. Novel approaches have been developed to select important receptor conformations for molecular docking and improve the docking accuracy. A new server (PASSer2.0) has been developed for predicting allosteric sites in proteins based on machine learning. It will greatly facilitate allosteric drug discovery. These advances are expected to expand our capabilities in simulations of ligand binding and drug discovery.
YM, C-EC, WZ and JM wrote the manuscript.
YM is supported by the National Institutes of Health (R01GM132572) and National Science Foundation (2121063). C-EC is supported by the National Institutes of Health (R01GM109045) and National Science Foundation (MCB1932984). JM is supported by the National Institutes of Health grant GM31749. WZ is supported by the National Science Foundation of China (82273851) and National Key R&D Program (2022YFA1004304).
The authors declare that the research was conducted in the absence of any commercial or financial relationships that could be construed as a potential conflict of interest.
All claims expressed in this article are solely those of the authors and do not necessarily represent those of their affiliated organizations, or those of the publisher, the editors and the reviewers. Any product that may be evaluated in this article, or claim that may be made by its manufacturer, is not guaranteed or endorsed by the publisher.
Baron, R., and Mccammon, J. A. (2013). Molecular recognition and ligand association. Annu. Rev. Phys. Chem. 64, 151–175. doi:10.1146/annurev-physchem-040412-110047
Glielmo, A., Husic, B. E., Rodriguez, A., Clementi, C., Noe, F., and Laio, A. (2021). Unsupervised learning methods for molecular simulation Data. Chem. Rev. 121, 9722–9758. doi:10.1021/acs.chemrev.0c01195
Huber, G. A., and Mccammon, J. A. (2019). Brownian dynamics simulations of biological molecules. Trends Chem. 1, 727–738. doi:10.1016/j.trechm.2019.07.008
Muñiz-Chicharro, A., Votapka, L. W., Amaro, R. E., and Wade, R. C. (2022). Brownian dynamics simulations of biomolecular diffusional association processes. WIREs Comput. Mol. Sci. n/a, e1649.
Nunes-Alves, A., Kokh, D. B., and Wade, R. C. (2020). Recent progress in molecular simulation methods for drug binding kinetics. Curr. Opin. Struct. Biol. 64, 126–133. doi:10.1016/j.sbi.2020.06.022
Pang, X., and Zhou, H. X. (2017). Rate constants and mechanisms of protein-ligand binding. Annu. Rev. Biophys. 46, 105–130. doi:10.1146/annurev-biophys-070816-033639
Peng, C., Wang, J., Yu, Y., Wang, G., Chen, Z., Xu, Z., et al. (2019). Improving the accuracy of predicting protein-ligand binding-free energy with semiempirical quantum chemistry charge. Future Med. Chem. 11, 303–321. doi:10.4155/fmc-2018-0207
Tang, Z., Roberts, C. C., and Chang, C. A. (2017). Understanding ligand-receptor non-covalent binding kinetics using molecular modeling. Front. Biosci. (Landmark Ed. 22, 960–981. doi:10.2741/4527
Keywords: ligand binding, thermodynamics, kinetics, molecular docking, molecular dynamics, brownian dynamics, machine learning, mechanisms
Citation: Miao Y, Chang C-EA, Zhu W and McCammon JA (2023) Editorial: Mechanisms, thermodynamics and kinetics of ligand binding revealed from molecular simulations and machine learning. Front. Mol. Biosci. 10:1139471. doi: 10.3389/fmolb.2023.1139471
Received: 07 January 2023; Accepted: 11 January 2023;
Published: 17 January 2023.
Edited and reviewed by:
Ray Luo, University of California, Irvine, United StatesCopyright © 2023 Miao, Chang, Zhu and McCammon. This is an open-access article distributed under the terms of the Creative Commons Attribution License (CC BY). The use, distribution or reproduction in other forums is permitted, provided the original author(s) and the copyright owner(s) are credited and that the original publication in this journal is cited, in accordance with accepted academic practice. No use, distribution or reproduction is permitted which does not comply with these terms.
*Correspondence: Yinglong Miao, bWlhb0BrdS5lZHU=; Chia-En A. Chang, Y2hpYWVuY0B1Y3IuZWR1; Weiliang Zhu, d2x6aHVAc2ltbS5hYy5jbg==; J. Andrew McCammon, am1jY2FtbW9uQHVjc2QuZWR1
Disclaimer: All claims expressed in this article are solely those of the authors and do not necessarily represent those of their affiliated organizations, or those of the publisher, the editors and the reviewers. Any product that may be evaluated in this article or claim that may be made by its manufacturer is not guaranteed or endorsed by the publisher.
Research integrity at Frontiers
Learn more about the work of our research integrity team to safeguard the quality of each article we publish.